- 1Research Assistant. Laboratory for Future-Ready Infrastructure (FuRI Lab), School of Construction Management Technology. Purdue University, West Lafayette, IN, United States
- 2Master student. School of Construction Management Technology. Purdue University, West Lafayette, IN, United States
- 3Assistant Professor and Director of the laboratory for Future-Ready Infrastructure (FuRI Lab). School of Construction Management Technology. Purdue University, West Lafayette, IN, United States
- 4Associate Professor. School of Construction Management Technology. Purdue University, West Lafayette, IN, United States
The construction sector is traditionally affected by on-site errors that significantly impact both budget and schedule. To minimize these errors, researchers have long hypothesized the development of AR-enriched 4D models that can guide workers on components deployment, assembly procedures, and work progress. Such systems have recently been referred to as Advanced Building-Assistance Systems (ABAS). However, despite the clear need to reduce the on-site errors, an ABAS has not been implemented and tested yet. This is partially due to a limited comprehension of the current wealth of available sensing technologies in the construction industry. To bridge the current knowledge gap, this paper evaluates the capabilities of current use of sensing technologies for objects identification, location, and orientation. This study employs and illustrates a systematic methodology to select according to eight criteria and analyzed in three level the literature on the field to ensure comprehensive coverage of the topic. The findings highlight both the capabilities and constraints of current sensing technologies, while also providing insight into potential future opportunities for integrating advanced tracking and identification systems in the built environment.
1 Introduction
Building construction is a vital component of the US economy, representing about 4% of the national GDP in 2022 (BEA, 2023). Despite its strategic role, the construction industry is still challenged by a significant incidence of on-site errors and inefficiencies, which impact construction expenses and timelines (Jaafar et al., 2018). These challenges encompass, among others, the misplacement or misalignment of components and prolonged, inefficient searches for objects on-site, which are particularly relevant in assembly constructions, which constitute a significant market share in the US. (García de Soto et al., 2022).
Traditionally, errors and inefficiency in the identification and placement of components in construction have been considered unavoidable. However, with recent technological advancements providing control and support to on-site constructions, this is no longer the norm. Latest advancements in sensing technologies (Kumar et al., 2015), Internet of Things (IoT), 4D virtual representation (Pan et al., 2018) and augmented reality (Yan, 2022) have created the premises for the development of AR-enriched cyber-physical 4D models to support the workers during the construction, i.e., indicating the components to be deployed according to the work plan as well as the location and mode of assembly using augmented reality, and tracking the progress of the work to automatically update the Building Information Modeling (BIM) (Chen et al., 2020; Turkan et al., 2012).
Such a construction support system has recently been referred to (Suo et al., 2023) as Advanced Building-Assistance Systems (ABAS), mutating the well-established concept of Advanced Driving-Assistance Systems (ADAS) from the automotive industry (Li et al., 2021). ABAS build on three core sensing capabilities to constantly map the movements of objects in physical construction sites; these to identify, track, and orient components in real-time. Indeed, achieving synchronization of on-site assembly processes with the 4D model first necessitates the capability to: (i) locate specific objects, e.g., identifying a particular beam intended for installation in a specific part of the construction site among numerous others; (ii) track their movements, i.e., detect when the beam is moved from its original stack to its designated location in the construction; and (iii) recognize their orientation upon placement, e.g., detecting the alignment of the beam on all axes when laid in place. These sensing capabilities play a vital role in ensuring a consistent alignment of the physical construction process with the scheduled activities outlined in a 4D virtual model (Suo et al., 2023).
Despite the clear need to reduce the on-site errors and the wealth of sensing technologies to identify, track, and orient objects, recently become available, an ABAS system has not been implemented and tested yet. This is partially due to the notorious resistance of the construction sector to adopt technological advancements (Hunhevicz and Hall, 2020), which often stems from a limited comprehension of the existing technological capabilities that could enhance the construction industry.
To cover this gap, the aim (i.e., the research question) of the present paper is to address the current lack of understanding of the state of research on Automated Building Assembly Systems (ABAS). Establishing a common understanding of the current capabilities in performing these fundamental tasks on a construction site is essential for developing a cyber-physical interface capable of automatically synchronizing the movements of physical components with their virtual counterparts. With the goal of promoting the progress of Automated Building Assembly Systems (ABAS), this paper aims to address the current knowledge gap through a systematic analysis of how sensing technologies are currently applied to recognize, locate, and orient objects.
2 Methodology
In this section, the most cutting-edge works on the use of object identification, tracking and orientation recognition are analyzed using a systematic method.
In this work, we have systematically examined the current status of research on object identification, location, and orientation by selecting, categorizing, and analyzing relevant scientific publications in these fields. We chose papers that: (1) were published within the past 20 years, i.e., since January 2003; (2) addresses objects identification, tracking, and orientation; and (3) involve technologies that could be used on a construction site, i.e., that are used or can be used in outdoor or in a non-sterile environment. The selection was run using common database for scientific publications, including Google Scholar and Scopus. Then, the chosen articles were categorized according to 8 criteria: the year of publication, the sector of application, the type e and number of objects treated, the scope of the study, the sensing technology used, its state of development (i.e., the maturity of the technology) and its limitation for large scale deployment on a construction site. The choice of the criteria steams from the research question of the analysis. Therefore, some of the criteria were chosen to identify trends in the distribution of works over time, sector and objectives, and some to clarify the dependency of the applications to the state of the required technology, i.e., complexity and number of objects treated, type and maturity of the involved technology and potential barriers to implementing these solutions on a large scale, particularly in dynamic and complex environments like construction sites. The rational for the classification of each of the criteria is reported in Table 1.
Finally, the categorized papers were analyzed to uncover patterns, trends, and areas of deficiency in the field of objects identification, location, and orientation. The analysis has been designed to delve into the relationships and potential gaps within the development of the relevant sensing technologies to ABAS. To this scope the analysis was conducted across three distinct levels:
Level 1 - For each of the eight criteria, a frequency distribution of the classifications was produced, e.g., the number of publications on object tracking. The rationale behind this level of investigation was to identify overarching trends and prevailing themes, for establishing a fundamental framework upon which more detailed analyses could be constructed.
Level 2 – A dual interpolation was conducted on the most pivotal criteria identified in the Level 1 analysis, i.e., these that provided the most indicative patterns (e.g., if the analysis of a criteria show a particular concentration of research on a specific aspect over the others, that underscore a meaningful tendency, that criteria is retained for further interpolation in level 2), to create a frequency distribution that compares the classification of one criterion against another. For example, determining the number of publications related to tracking of construction elements.
Level 3 – A triple interpolation was conducted on the most critical criteria identified in the Level 1 analysis to generate a frequency distribution that compares one classification against the other two. This resulted in a distribution that represents the frequency of specific combinations of classification characteristics. For example, providing insights into the number of works that specifically target logistics infrastructure, using tracking technology, for TRL level.
3 Analysis of sensing technology for object identification, tracking and orientation recognition
The selected 72 articles are reported in Tables 2–4 alongside their specific classification for each of the 8 criteria. The results of the 3 levels analysis are further shown in Figures 1–5 and commented in the following text2.
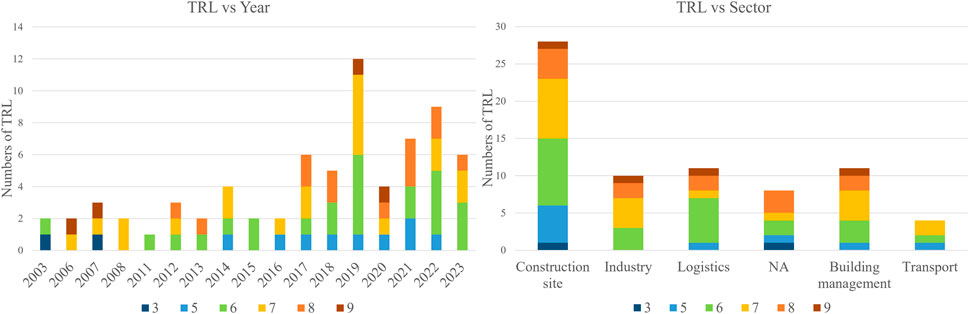
Figure 2. Level 2 analysis of the extrapolation between two classification characteristics, TRL and year (left), TRL and sector (right).
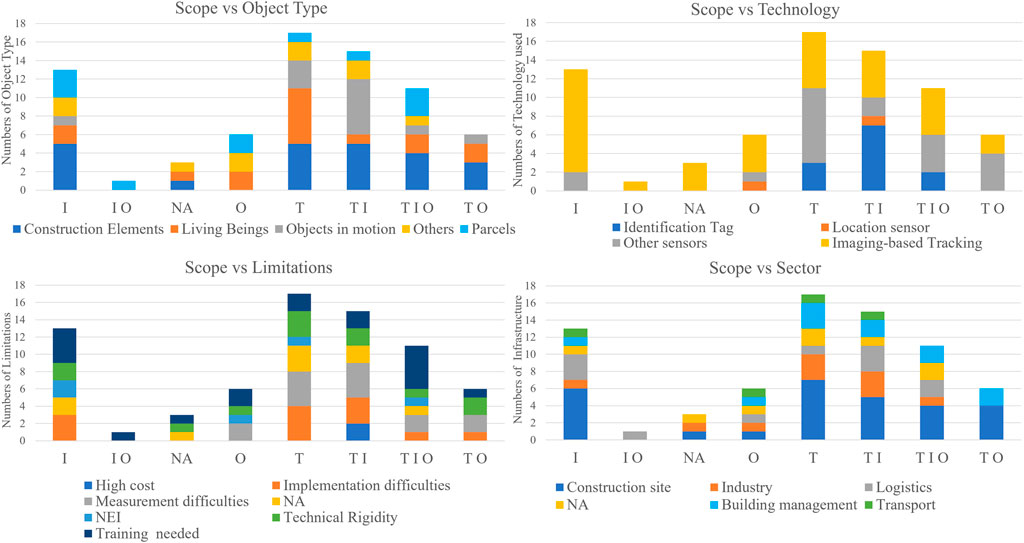
Figure 3. Level 2 analysis of the interpolation between scope and other four classification characteristics. (Abbreviation: tracking [T]; orientation [O]; identification [I] of the object; not specified [NA]; all combination of previously listed letters correspond the combined abbreviations).
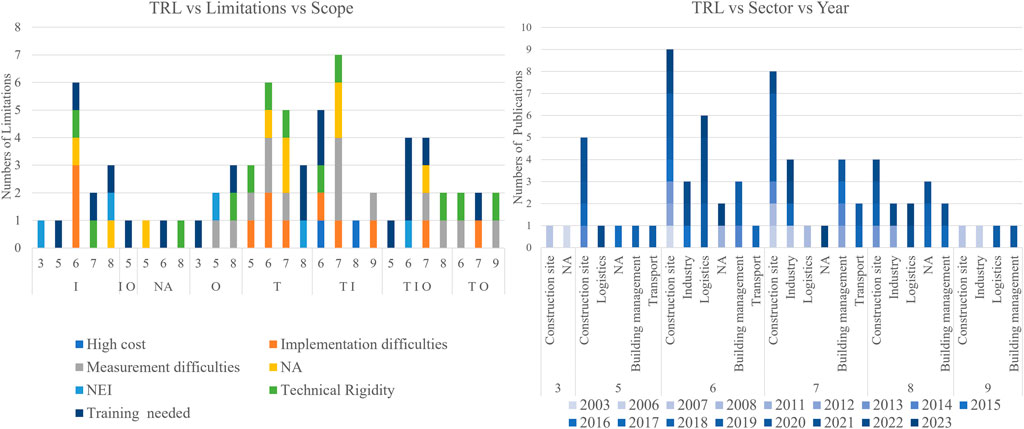
Figure 4. Level 3 analysis of the interpolation between TRL, scope and limitations (left), TRL, sector and year (right) (Abbreviation: tracking [T]; orientation [O]; identification [I] of the object; not specified [NA]; all combination of previously listed letters correspond the combined abbreviations)2.
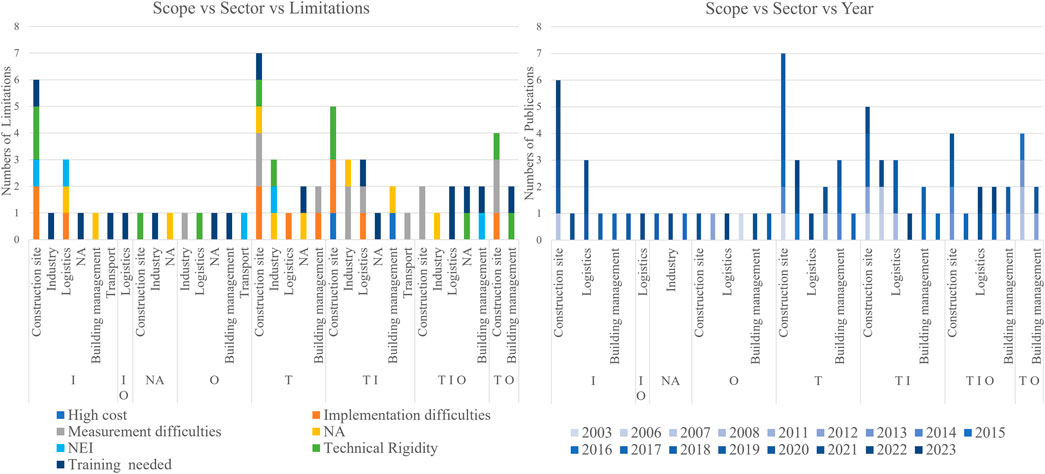
Figure 5. Level 3 analysis of the interpolation between scope, sector and limitations (left), scope, sector and year (right) (Abbreviation: tracking [T]; orientation [O]; identification [I] of the object; not specified [NA]; all combination of previously listed letters correspond the combined abbreviations)2.
3.1 Level 1 analysis
Level 1 analysis (Figure 1) shows how approximately one-third of the studies selected (37%) have been conducted within the construction sector and have been done with increasing consistency since 2019. Papers from this year on constitute 43% of the total publications in the two-decade span. The dominance of the construction sectors in the development and testing of solutions d for objects identification, tracking and orientation reflects the pressing need for ABAS in this domain characterized by a complex interplay of moving machinery, materials, and personnel. Moreover, more than half the studies focus on multiple objects, as a consequence of the need in many sectors of identify and track objects among multiple others (e.g., a wooden beam out of a stock). While, over half of the studies primarily focus on two types of objects: construction elements (e.g., wooden components for balloon frame constructions) and living beings (e.g., humans on industrial or construction sites, typically for safety reasons, or animals for farming purposes). In terms of technology used, imaging-based tracking, i.e., systems using computer vision for image capturing, background subtraction, bodies detection, bodies tracking and data association (Martani et al., 2017), emerges as the mode, with Radio Frequency Identification (RFID) following closely. The preference for these technologies reflects their affordable scalability - i.e., RFID are relatively inexpensive compared to other sensing technologies and allow for affordable large-scale deployments, while imaging-based tracking is cost invariant to the volume - and resistance–i.e., RFID are resistant and often reusable tags, while imaging-based tracking does not require deployment of sensors–which are valuable attributes in many site deployments. In terms of technology matureness, over 60% of studies have used technologies that are TRL 6 or above. This means that among the publications selected the vast majority used sensing technologies, at least on a prototype testing. This is not surprising as it confirms a known tendency in works addressing technology development and validation to focus on practical testing and deployments over only theoretical hypothesis, basic principles observation or concept formulation. Finally, an overarching limitation across various technologies, especially those involving Image-based tracking, is the need for training. Closely following this is the challenge of measurement difficulties, especially pronounced in the context of construction and work sites, where the dynamic and complex environment poses unique challenges to accurate measurement and tracking.
3.2 Level 2 analysis
Level 2 analysis involved a comprehensive evaluation of each criterion against all others. Among these, the most insightful results emerged from the interplay of the TRL and Scope, against the other criteria, which offer a comprehensive understanding of dynamics between technologies chosen in terms of maturity and purpose over time, sectors and objects, as well as the associated limitations.
In particular, the interpolation of the TRL with years of publication and sectors show how the maturity of the technologies chosen have remained stable over time and across fields of application. The results reported in Figure 2 clearly points that TRL 6, to 9 are consistently dominant among the works analyzed across time, without significant variations, i.e., no obvious tendency seems traceable of a progressive orientation toward either more mature or more experimental technologies in recent years. This is not surprising considering the tendency highlighted in the level 1 analysis to focus mostly on prototypes or large deployment testing over theoretical hypothesis. In particular, it is noticeable that the limited use of more experimental technologies (i.e., TRL 3, 4, and 5) is restricted to works developed for construction sites, logistics or theoretical studies. For example, a sensor-based material tracking system for construction components has recently been presented (Jung et al., 2023), that has so far only undergone lab-based testing of its individual components to validate their functionality (i.e., TRL 4).
The Scope has been interpolated with: type of objects, technology used, limitations and sector of application. Results in Figure 3 show how the works that have objects identification as a scope are predominantly applied on construction elements, logistic parcels and living beings; using largely imaging-based tracking as a technology; and deployed mostly on construction sites for construction components identification. For instance, in a Dubai construction project, imaging-based tracking was utilized to monitor the placement and orientation of pre-fabricated components. Cameras strategically positioned around the site, linked to AI algorithms, ensured that each component was correctly aligned according to architectural plans, thus enhancing accuracy and efficiency in the construction process (Guven and Ergen, 2019). Notably, the preference for imaging-based tracking can be attributed to its adaptability and proficiency in managing the dynamic and complex nature of construction and logistics environments. Given the multitude of objects and the non-delicate handling often observed on construction sites, the utilization of fragile sensors poses inherent risks. In this context, the robustness of imaging-based tracking systems offers a safer and more reliable alternative. In terms of limitations, a slight prevalence of training needs and implementation difficulties emerges. The works focusing on objects orientation are predominantly applied on logistic parcels and living beings (human specifically in this case to detect hazardous movement, such as falling from height); using almost exclusively Imaging-based tracking as a technology (11/13 times); and deployed mostly on industrial sites. In terms of limitations, the main concerns come from the training needs of the imaging-based tracking. The works that have objects tracking as a scope are predominantly applied on construction elements, logistic parcels and living beings; using a mix of imaging-based technology, Identification tags and others (e.g., programmable logic control, LADAR and optical sensors); and are deployed predominantly on construction sites (18/45 times) with a large spectrum of limitations. When considering works with multiple scopes it is interesting to notice how logistics grows in importance being the only sector involved in identification and orientation combined, and significantly present - along with building management and construction sites - both in works concerning identification and tracking and identification, orientation and tracking together.
3.3 Level 3 analysis
Also in the level 3 analysis, the most informative findings were uncovered by exploring the interaction between TRL and Scope with the other criteria (illustrated in Figures 4, 5 respectively). Figure 4 indicates a prevalence of technologies within TRL 6-8 across various scopes, with a relatively limited presence of more mature technologies at TRL 9. Several limitations could account for this trend. Works centered on identification, tracking, and their combination are significantly impacted by implementation challenges, technical rigidity, and measurement difficulties, while, works related to orientation often face challenges due to high costs. The need for extra training is ubiquitous across sectors, being a prevalent constraint in systems integrating Imaging-based tracking. These limitations frequently hinder these technologies from reaching the level of successful commercial systems, i.e., TRL 9. Additionally, it is noteworthy, as depicted in Figure 4 (right), that research endeavors spanning different sectors and TRLs have notably intensified over the past 7 years. While this trend is expected, the substantial acceleration in research activities within this field in recent years is striking. This trend is particularly evident in logistics, where only 1 out of 11 studies took place before 2016.
Figure 5 indicates that the construction research dominates, along with logistic, in works related to identification, tracking and a mix of the two. However, these applications face two main challenges: implementation complexities and technical rigidity. As an example, construction sites contend with issues such as dust, vibrations, and ever-changing environments, all of which can disrupt sensitive tracking devices. Another example details a residential building in New York that uses a cloud-based security system, allowing for remote monitoring and management, which enhances tenant security and operational efficiency (Sengupta et al., 2021). These implementation challenges are crucial as they can result in significant consequences. Construction projects often operate within tight budgets and schedules, where errors or inefficiencies can lead to substantial financial and time losses. For instance, misplacing a component due to tracking or identification errors can cause delays lasting several days. In terms of year of publication, also in Figure 5 (right) it is possible to appreciate the steep increase in works across various sectors and scopes in recent years. In this case the phenomenon is particularly evident in the construction sector, where 16 out of 23 studies, primarily focusing on Identification, tracking, or a combination of both, were conducted after 2016.
3.4 Contribute to the research question
In line with the research question presented in the introduction, this study contributed in covering the current gap in understanding the state of research on ABAS through a systematic literature review. The findings over the three levels analysis helped identifying the current trends, capabilities and limitations in the use of sensing technologies for recognizing, locating, and orienting objects that could be used for the development of in ABAS systems. Detailed conclusions from the results are provided in the next sections.
4 Conclusion
This study addresses the current lack of understanding of the state of research on ABAS by providing a comprehensive analysis of existing technologies, their applications, and the challenges they face. By identifying critical areas for improvement and potential future research directions, this paper contributes to the development of more effective and efficient ABAS solutions, ultimately promoting progress in the field. Specifically, based on all three levels of analysis, several notable conclusions can be drawn.
- Research in this domain has accelerated vigorously in recent years. Since 2019, there has been a discernible surge in identification and orientation research, not limited to the construction sector but also in building management, logistics and manufacturing industry. This growing trend highlights the urgent demand for innovative solutions across these sectors, emphasizing safety, productivity, and operational efficiency. The inherent challenges of each sector, like the complex nature of construction sites or the dynamic environment of logistics, are driving a shift towards the use of Imaging-based tracking techniques that are greatly adaptable to multifaceted environments.
- The construction sector stands out as one of the dominant areas among the research sectors involved. This prevalence is logically justified by the dynamic nature of construction environments, which involve intricate interactions among moving machinery, materials, and personnel. The research emphasis in this sector is unsurprising considering the significant advantages that integrating a proficient ABAS can offer, such as enhancing safety protocols, streamlining operations, and improving overall cost efficiency.
- The technologies utilized are characterized by a high level of maturity across various sectors. TRL 6 or above consistently dominates over time, showing no clear trend indicating a shift towards either more mature or experimental technologies. In particular, there is a consistent prevalence of TRL 5-7 overtime, albeit coupled with a modest presence of TRL 8 and 9. Current limitations in the use of highly impactful technologies, particularly visual technology across sectors, appears to possibly be responsible for the limited amount of TRL 9 applications.
- Five main limitations emerge to be recurrent across all applications and all sectors: works focused on identification, tracking, and their combination encounter significant obstacles related to implementation challenges, technical rigidity and measurement difficulties, while, initiatives involving orientation often encounter challenges due to high costs. In the aspect of technical rigidity, key challenges involve the need for multiple high-frequency RFID tags for precise tracking in warehouse automation systems, and the necessity for numerous GPUs for efficient real-time data processing in complex simulations. Regarding implementation issues, difficulties arise in machine vision systems that fail to recognize objects with diverse surface textures or colors, environmental monitoring systems providing inaccurate readings under extreme weather conditions. Measurement challenges are exemplified by GPS systems with limited range and accuracy in densely built urban areas. The necessity for additional training seems to be widespread. This is a common limitation of systems incorporating visual technologies, impacting projects across various scopes and sectors.
The practical challenges of implementing sensing technologies on construction sites are particularly noteworthy. Construction sites present unique challenges such as harsh environmental conditions, dynamic and cluttered workspaces, and the need for integration with existing workflows and safety protocols. These factors can significantly impact the performance and reliability of sensing technologies. Additionally, the high costs associated with the deployment and maintenance of these technologies pose a barrier to widespread adoption. Further exploration of these practical implications is crucial for advancing the field.
Future research should focus on overcoming the identified limitations, particularly in improving the technical robustness and implementation feasibility of sensing technologies. Addressing the current challenges and tailoring solutions to specific industry needs could yield significant breakthroughs in the coming years. Recent advancements in AI-related Imaging-based tracking (Hamledari et al., 2017; Nguyen et al., 2020) are poised to overcome many of these limitations. It is foreseeable that upon overcoming the existing training challenges linked to Imaging-based tracking, the possibilities for identification, tracking, and orientation in the construction sector will greatly broaden. Combined with the advancement of augmented reality-enriched 4D models, this could facilitate the creation of Advanced Building-Assistance Systems (ABAS) capable of guiding workers in component deployment, assembly procedures, and work progress. Future research on several key areas would be needed, including.
- Enhancing the robustness of sensing technologies to ensure reliable performance across diverse and challenging environments.
- Evaluating and reducing the costs associated with these technologies to make their implementation more economically viable. This includes a thorough cost-benefit analysis to determine the financial feasibility and identify potential cost-saving measures.
- Developing strategies to make the implementation of these technologies more feasible and cost-effective.
- Creating user-friendly interfaces and systems that minimize the need for extensive training.
Author contributions
JS: Writing–original draft, Writing–review and editing. SW: Writing–original draft. VG: Writing–original draft. AP: Writing–original draft. CM: Writing–review and editing. HD: Writing–review and editing.
Funding
The author(s) declare that no financial support was received for the research, authorship, and/or publication of this article.
Acknowledgments
The authors acknowledge the support received from the organizing committee of the Cambridge Symposium on Applied Urban Modelling (AUM 2023). The feedback received during this event on the preliminary idea of this work has been instrumental in its development.
Conflict of interest
The authors declare that the research was conducted in the absence of any commercial or financial relationships that could be construed as a potential conflict of interest.
Publisher’s note
All claims expressed in this article are solely those of the authors and do not necessarily represent those of their affiliated organizations, or those of the publisher, the editors and the reviewers. Any product that may be evaluated in this article, or claim that may be made by its manufacturer, is not guaranteed or endorsed by the publisher.
Footnotes
1Source: https://esto.nasa.gov/trl/.
2Since the years are progressive, the legends for this classification characteristic is color graduated to facilitate visualizing the progression, i.e., lighter regions signify more remote years, with values increasing as the shade darkens. This is trusted to reduces the cognitive load on the reader, making it easier to focus on the data itself rather than deciphering the meaning of multiple colors.
References
Akhavian, R., and Behzadan, A. H. (2015). Construction equipment activity recognition for simulation input modeling using mobile sensors and machine learning classifiers. Adv. Eng. Inf. Collect. Intell. Model. Analysis, Synthesis Innovative Eng. Decis. Mak. 29 (4), 867–877. doi:10.1016/j.aei.2015.03.001
Al Jaberi, S. M., Patel, A., and AL-Masri, A. N. (2023). Object tracking and detection techniques under GANN threats: a systemic review. Appl. Soft Comput. 139, 110224. doi:10.1016/j.asoc.2023.110224
Alzahrani, B. A., and Irshad, A. (2022). An improved IoT/RFID-enabled object tracking and authentication scheme for smart logistics. Wirel. Pers. Commun. 129 (1), 399–422. doi:10.1007/s11277-022-10103-7
Angah, O., and Chen, A. Y. (2020). Tracking multiple construction workers through deep learning and the gradient based method with re-matching based on multi-object tracking accuracy. Automation Constr. 119, 103308. doi:10.1016/j.autcon.2020.103308
Arif, O., Ray, S. J., Vela, P. A., and Teizer, J. (2013). Potential of time-of-flight range imaging for object identification and manipulation in construction. J. Comput. Civ. Eng. 28 (6), 06014005. doi:10.1061/(ASCE)CP.1943-5487.0000304
BEA (2023). U.S. Bureau of economic analysis (BEA). Available at: https://www.bea.gov/(Accessed October 20, 2023).
Brownjohn, J. M. W., Xu, Y., and Hester, D. (2017). Vision-based bridge deformation monitoring. Front. Built Environ. 3, Frontiers. doi:10.3389/fbuil.2017.00023
Chen, Q., Adey, B. T., Haas, C., and Hall, D. M. (2020). Using look-ahead plans to improve material flow processes on construction projects when using BIM and RFID technologies. Constr. Innov. 20 (3), 471–508. doi:10.1108/CI-11-2019-0133
Contigiani, M., Pietrini, R., Mancini, A., and Zingaretti, P. (2016). Implementation of a tracking system based on UWB technology in a retail environment, 1–6.
Dror, E., Zhao, J., Sacks, R., and Seppänen, O. (2019) “Indoor tracking of construction workers using BLE: mobile beacons and fixed gateways vs,” in Fixed beacons and mobile gateways, 831–842.
Edwards, C., Morales, D. L., Haas, C., Narasimhan, S., and Cascante, G. (2023). Digital twin development through auto-linking to manage legacy assets in nuclear power plants. Automation Constr. 148, 104774. doi:10.1016/j.autcon.2023.104774
Ergen, E., Akinci, B., and Sacks, R. (2007). Tracking and locating components in a precast storage yard utilizing radio frequency identification technology and GPS. Automation Constr. 16 (3), 354–367. doi:10.1016/j.autcon.2006.07.004
Escorcia, V., Dávila, M. A., Golparvar-Fard, M., and Niebles, J. C. (2012). Automated vision-based recognition of construction worker actions for building interior construction operations using RGBD cameras. Am. Soc. Civ. Eng. 98, 879–888. doi:10.1061/9780784412329.089
Esteve-Taboada, J. J., and Garcıa, J. (2003). Detection and orientation evaluation for three-dimensional objects. Opt. Commun. 217 (1), 123–131. doi:10.1016/S0030-4018(03)01125-8
Fang, Q., Li, H., Luo, X., Ding, L., Luo, H., Rose, T. M., et al. (2018a). Detecting non-hardhat-use by a deep learning method from far-field surveillance videos. Automation Constr. 85, 1–9. doi:10.1016/j.autcon.2017.09.018
Fang, W., Ding, L., Zhong, B., Love, P. E. D., and Luo, H. (2018b). Automated detection of workers and heavy equipment on construction sites: a convolutional neural network approach. Adv. Eng. Inf. 37, 139–149. doi:10.1016/j.aei.2018.05.003
Galna, B., Barry, G., Jackson, D., Mhiripiri, D., Olivier, P., and Rochester, L. (2014). Accuracy of the Microsoft Kinect sensor for measuring movement in people with Parkinson’s disease. Gait and Posture 39 (4), 1062–1068. doi:10.1016/j.gaitpost.2014.01.008
Gao, B., and Yuen, M. M. F. (2011). Passive UHF RFID packaging with electromagnetic band gap (EBG) material for metallic objects tracking. IEEE Trans. Components, Packag. Manuf. Technol. 1 (8), 1140–1146. doi:10.1109/TCPMT.2011.2157150
García de Soto, B., Agustí-Juan, I., Joss, S., and Hunhevicz, J. (2022). Implications of Construction 4.0 to the workforce and organizational structures. Int. J. Constr. Manag. 22 (2), 205–217. doi:10.1080/15623599.2019.1616414
Goodrum, P. M., McLaren, M. A., and Durfee, A. (2006). The application of active radio frequency identification technology for tool tracking on construction job sites. Automation Constr. 15 (3), 292–302. doi:10.1016/j.autcon.2005.06.004
Guven, G., and Ergen, E. (2019). A rule-based methodology for automated progress monitoring of construction activities: a case for masonry work. J. Inf. Technol. Constr. (ITcon) 24 (11), 188–208.
Hamledari, H., McCabe, B., and Davari, S. (2017). Automated computer vision-based detection of components of under-construction indoor partitions. Automation Constr. 74, 78–94. doi:10.1016/j.autcon.2016.11.009
Han, S., and Lee, S. (2013). A vision-based motion capture and recognition framework for behavior-based safety management. Automation Constr. 35, 131–141. doi:10.1016/j.autcon.2013.05.001
Han, S., Wang, H., Yu, E., and Hu, Z. (2023). ORT: occlusion-robust for multi-object tracking. Fundam. Res. doi:10.1016/j.fmre.2023.02.003
Heißmeyer, S., Overmeyer, L., and Müller, A. (2012) “Indoor positioning of vehicles using an active optical infrastructure,” in 2012 international conference on indoor positioning and indoor navigation (IPIN), 1–8.
Hoang, N.-D. (2019). Automatic detection of asphalt pavement raveling using image texture based feature extraction and stochastic gradient descent logistic regression. Automation Constr. 105, 102843. doi:10.1016/j.autcon.2019.102843
Holmström, E., Raatevaara, A., Pohjankukka, J., Korpunen, H., and Uusitalo, J. (2023). Tree log identification using convolutional neural networks. Smart Agric. Technol. 4, 100201. doi:10.1016/j.atech.2023.100201
Huang, R., Gu, J., Sun, X., Hou, Y., and Uddin, S. (2019). A rapid recognition method for electronic components based on the improved YOLO-V3 network. Electronics 8 (8), 825. doi:10.3390/electronics8080825
Huang, Z., Yang, S., Zhou, M., Gong, Z., Abusorrah, A., Lin, C., et al. (2021). Making accurate object detection at the edge: review and new approach. Artif. Intell. Rev. 55 (3), 2245–2274. doi:10.1007/s10462-021-10059-3
Huang, Z., Zhu, J., Yang, L., Xue, B., Wu, J., and Zhao, Z. (2015). Accurate 3-D position and orientation method for indoor mobile robot navigation based on photoelectric scanning. IEEE Trans. Instrum. Meas. 64 (9), 2518–2529. doi:10.1109/TIM.2015.2415031
Hunhevicz, J. J., and Hall, D. M. (2020). Do you need a blockchain in construction? Use case categories and decision framework for DLT design options. Adv. Eng. Inf. 45, 101094. doi:10.1016/j.aei.2020.101094
Iqbal, J., Munir, M. A., Mahmood, A., Ali, A. R., and Ali, M. (2021). Leveraging orientation for weakly supervised object detection with application to firearm localization. Neurocomputing 440, 310–320. doi:10.1016/j.neucom.2021.01.075
Jaafar, M. H., Arifin, K., Aiyub, K., Razman, M. R., Ishak, M. I. S., and Samsurijan, M. S. (2018). Occupational safety and health management in the construction industry: a review. Int. J. Occup. Saf. Ergonomics 24 (4), 493–506. doi:10.1080/10803548.2017.1366129
Jang, W.-S., and Skibniewski, M. J. (2008). A WIRELESS NETWORK SYSTEM FOR AUTOMATED TRACKING OF CONSTRUCTION MATERIALS ON PROJECT SITES. J. Civ. Eng. Manag. 14 (1), 11–19. doi:10.3846/1392-3730.2008.14.11-19
Jaselskis, E. J., and El-Misalami, T. (2003). Implementing radio frequency identification in the construction process. J. Constr. Eng. Manag. 129 (6), 680–688. doi:10.1061/(ASCE)0733-9364(2003)129:6(680)
Jeong, I. cheol, Bychkov, D., Hiser, S., Kreif, J. D., Klein, L. M., Hoyer, E. H., et al. (2017). Using a real-time location system for assessment of patient ambulation in a hospital setting. Archives Phys. Med. Rehabilitation 98 (7), 1366–1373.e1. doi:10.1016/j.apmr.2017.02.006
Jung, S., Jeoung, J., Lee, dong-eun, Jang, H.-S., and Hong, T. (2023) “Visual–auditory learning network for construction equipment action detection,” in Computer-aided civil and infrastructure engineering. doi:10.1111/mice.12983
Khosrowpour, A., Niebles, J. C., and Golparvar-Fard, M. (2014). Vision-based workface assessment using depth images for activity analysis of interior construction operations. Automation Constr. 48, 74–87. doi:10.1016/j.autcon.2014.08.003
Kim, M., and Kim, Y. (2022). Parcel classification and positioning of intelligent parcel storage system based on YOLOv5. Appl. Sci. 13 (1), 437. doi:10.3390/app13010437
Kovar, R. N., Brown-Giammanco, T. M., and Lombardo, F. T. (2018). Leveraging remote-sensing data to assess garage door damage and associated roof damage. Front. Built Environ. 4 (Frontiers). doi:10.3389/fbuil.2018.00061
Kumar, P., Morawska, L., Martani, C., Biskos, G., Neophytou, M., Di Sabatino, S., et al. (2015). The rise of low-cost sensing for managing air pollution in cities. Environ. Int. 75, 199–205. doi:10.1016/j.envint.2014.11.019
Lavner, Y., Cohen, R., Ruinskiy, D., and Ijzerman, H. (2016) “Baby cry detection in domestic environment using deep learning,” in 2016 IEEE international conference on the science of electrical engineering (ICSEE), 1–5.
Lee, Y. H., and Marsic, I. (2018). Object motion detection based on passive UHF RFID tags using a hidden Markov model-based classifier. Sens. Bio-Sensing Res. 21, 65–74. doi:10.1016/j.sbsr.2018.10.005
Lee, Y.-J., and Park, M.-W. (2019). 3D tracking of multiple onsite workers based on stereo vision. Automation Constr. 98, 146–159. doi:10.1016/j.autcon.2018.11.017
Li, T., Huang, B., Li, C., and Huang, M. (2019). Application of convolution neural network object detection algorithm in logistics warehouse. J. Eng. 2019 (23), 9053–9058. doi:10.1049/joe.2018.9180
Li, X., Lin, K.-Y., Meng, M., Li, X., Li, L., Hong, Y., et al. (2021). Composition and application of current advanced driving assistance system: a review. arXiv. doi:10.48550/arXiv.2105.12348
Liang, H., and Seo, S. (2022). Automatic detection of construction workers’ helmet wear based on lightweight deep learning. Appl. Sci. 12 (20), 10369. doi:10.3390/app122010369
Liu, Q. Q., and Li, J. B. (2019). Orientation robust object detection in aerial images based on R-NMS. Procedia Comput. Sci. Proc. 9th Int. Conf. Inf. Commun. Technol. [ICICT-2019] Nanning 154, 650–656. Guangxi, China January 11-13, 2019. doi:10.1016/j.procs.2019.06.102
Liu, S., Li, X., Gao, M., Cai, Y., Nian, R., Li, P., et al. (2018). Embedded online fish detection and tracking system via YOLOv3 and parallel correlation filter. OCEANS 2018 MTS/IEEE Charlest. 1–6. doi:10.1109/OCEANS.2018.8604658
Malburg, L., Rieder, M.-P., Seiger, R., Klein, P., and Bergmann, R. (2021). Object detection for smart factory processes by machine learning. Procedia Comput. Sci. 12th Int. Conf. Ambient Syst. Netw. Technol. (ANT)/4th Int. Conf. Emerg. Data Industry 4.0 (EDI40)/Affil. Work. 184, 581–588. doi:10.1016/j.procs.2021.04.009
Martani, C., Stent, S., Acikgoz, S., Soga, K., Bain, D., and Jin, Y. (2017). Pedestrian monitoring techniques for crowd-flow prediction. Proc. Institution Civ. Eng. - Smart Infrastructure Constr. 170 (2), 17–27. doi:10.1680/jsmic.17.00001
Mathew, M. P., and Mahesh, T. Y. (2022). Leaf-based disease detection in bell pepper plant using YOLO v5. SIViP 16 (3), 841–847. doi:10.1007/s11760-021-02024-y
M’hand, M. A., Boulmakoul, A., Badir, H., and Lbath, A. (2019). A scalable real-time tracking and monitoring architecture for logistics and transport in RoRo terminals. Procedia Comput. Sci. 10th Int. Conf. Ambient Syst. Netw. Technol. (ANT 2019)/2nd Int. Conf. Emerg. Data Industry 4.0 (EDI40 2019)/Affil. Work. 151, 218–225. doi:10.1016/j.procs.2019.04.032
Najafi, M., Nadealian, Z., Rahmanian, S., and Ghafarinia, V. (2019). An adaptive distributed approach for the real-time vision-based navigation system. Measurement 145, 14–21. doi:10.1016/j.measurement.2019.05.015
Nguyen, T.-N., Dakpé, S., Ho Ba Tho, M.-C., and Dao, T.-T. (2020). Real-time computer vision system for tracking simultaneously subject-specific rigid head and non-rigid facial mimic movements using a contactless sensor and system of systems approach. Comput. Methods Programs Biomed. 191, 105410. doi:10.1016/j.cmpb.2020.105410
Pan, M., Linner, T., Pan, W., Cheng, H., and Bock, T. (2018). A framework of indicators for assessing construction automation and robotics in the sustainability context. J. Clean. Prod. 182, 82–95. doi:10.1016/j.jclepro.2018.02.053
Rashid, K. M., and Louis, J. (2019). Construction equipment activity recognition from IMUs mounted on articulated implements and supervised classification. Am. Soc. Civ. Eng. 130–138. doi:10.1061/9780784482445.017
Ren, H., Liu, W., and Lim, A. (2014). Marker-based surgical instrument tracking using dual kinect sensors. IEEE Trans. Automation Sci. Eng. 11 (3), 921–924. doi:10.1109/TASE.2013.2283775
Roberts, D., and Golparvar-Fard, M. (2019). End-to-end vision-based detection, tracking and activity analysis of earthmoving equipment filmed at ground level. Automation Constr. 105, 102811. doi:10.1016/j.autcon.2019.04.006
Roddick, T., Kendall, A., and Cipolla, R. (2019). Orthographic feature transform for monocular 3D object detection. arXiv. doi:10.48550/arXiv.1811.08188
Rohrig, C., and Spieker, S. (2008). Tracking of transport vehicles for warehouse management using a wireless sensor network. IEEE/RSJ International Conference on Intelligent Robots and Systems, 3260–3265.
Sengupta, A., Budvytis, I., and Cipolla, R. (2021) “Probabilistic 3D human shape and pose estimation from multiple unconstrained images in the wild,” in 2021 IEEE/CVF conference on computer vision and pattern recognition (CVPR). USA: IEEE: Nashville, TN, 16089–16099.
Shaari, A. M., and Nor, N. S. M. (2017). Position and orientation detection of stored object using RFID tags. Procedia Eng. Adv. Material and Process. Technol. Conf. 184, 708–715. doi:10.1016/j.proeng.2017.04.146
Skibniewski, M., Jang, W.-S., and Chair, A. (2007). Localization technique for automated tracking of construction materials utilizing combined RF and ultrasound sensor interfaces. doi:10.1061/40937(261)78
Song, J., Haas, C. T., Caldas, C., Ergen, E., and Akinci, B. (2006). Automating the task of tracking the delivery and receipt of fabricated pipe spools in industrial projects. Automation Constr. 15 (2), 166–177. doi:10.1016/j.autcon.2005.03.001
Suo, J., Martani, C., Faddoul, A. G., Suvarna, S., and Gunturu, V. K. (2023). “State-of-the-art in the use of responsive systems for the built environment,” in Life-Cycle of Structures and Infrastructure Systems. (CRC Press), 2128–2135.
Tao, J., Wang, H., Zhang, X., Li, X., and Yang, H. (2017) “An object detection system based on YOLO in traffic scene,” in 2017 6th international conference on computer science and network technology (ICCSNT), 315–319.
Teizer, J., Caldas, C., and Haas, C. (2007). Real-time three-dimensional occupancy grid modeling for the detection and tracking of construction resources. J. Constr. Eng. Manag. 133, 880–888. doi:10.1061/(asce)0733-9364(2007)133:11(880)
Thiede, S., Ghafoorpoor, P., Sullivan, B. P., Bienia, S., Demes, M., and Dröder, K. (2022). Potentials and technical implications of tag based and AI enabled optical real-time location systems (RTLS) for manufacturing use cases. CIRP Ann. 71 (1), 401–404. doi:10.1016/j.cirp.2022.04.023
Turkan, Y., Bosche, F., Haas, C. T., and Haas, R. (2012). Automated progress tracking using 4D schedule and 3D sensing technologies. Automation Constr. Plan. Future Cities-Selected Pap. 2010 eCAADe Conf. 22, 414–421. doi:10.1016/j.autcon.2011.10.003
Turkan, Y., Bosché, F., Haas, C. T., and Haas, R. (2014). Tracking of secondary and temporary objects in structural concrete work. Constr. Innov. 14 (2), 145–167. doi:10.1108/CI-12-2012-0063
Valente, F. J., and Neto, A. C. (2017) “Intelligent steel inventory tracking with IoT/RFID,” in 2017 IEEE international conference on RFID technology and application (RFID-TA), 158–163.
Vu, T.-T.-H., Pham, D.-L., and Chang, T.-W. (2023). A YOLO-based real-time packaging defect detection system. Procedia Comput. Sci. 4th Int. Conf. Industry 4.0 Smart Manuf. 217, 886–894. doi:10.1016/j.procs.2022.12.285
Wang, X., Zhao, Q., Jiang, P., Zheng, Y., Yuan, L., and Yuan, P. (2022). LDS-YOLO: a lightweight small object detection method for dead trees from shelter forest. Comput. Electron. Agric. 198, 107035. doi:10.1016/j.compag.2022.107035
Wang, Z., Zhang, Q., Yang, B., Wu, T., Lei, K., Zhang, B., et al. (2020). Vision-based framework for automatic progress monitoring of precast walls by using surveillance videos during the construction phase. J. Comput. Civ. Eng. 35. doi:10.1061/(asce)cp.1943-5487.0000933
Weerasinghe, I., Ruwanpura, J., Boyd, J., and Habib, A. (2012). Application of microsoft kinect sensor for tracking construction workers. 37, 858, 867. doi:10.1061/9780784412329.087
Yan, W. (2022). Augmented reality instructions for construction toys enabled by accurate model registration and realistic object/hand occlusions. Virtual Real. 26 (2), 465–478. doi:10.1007/s10055-021-00582-7
Yan, X., Zhang, H., and Gao, H. (2022). Mutually coupled detection and tracking of trucks for monitoring construction material arrival delays. Automation Constr. 142, 104491. doi:10.1016/j.autcon.2022.104491
Zhang, J., Zi, L., Hou, Y., Wang, M., Jiang, W., and Deng, D. (2020). A deep learning-based approach to enable action recognition for construction equipment. Adv. Civ. Eng. 2020, e8812928. doi:10.1155/2020/8812928
Zhao, J., Pikas, E., Seppänen, O., and Peltokorpi, A. (2021a). Using real-time indoor resource positioning to track the progress of tasks in construction sites. Front. Built Environ. 7 (Frontiers). doi:10.3389/fbuil.2021.661166
Zhao, J., Zheng, Y., Seppänen, O., Tetik, M., and Peltokorpi, A. (2021b). Using real-time tracking of materials and labor for kit-based logistics management in construction. Front. Built Environ. 7 (Frontiers). doi:10.3389/fbuil.2021.713976
Zhao, K., Wang, Y., Zhu, Q., and Zuo, Y. (2022). Intelligent detection of parcels based on improved faster R-CNN. Appl. Sci. 12 (14), 7158. doi:10.3390/app12147158
Keywords: computer vision, location sensing, positioning, object identification, tracking, communication and control systems, orientation, building and construction management
Citation: Suo J, Waje S, Gunturu VKT, Patlolla A, Martani C and Dib HN (2024) The rise of digitalization in constructions: State-of-the-art in the use of sensing technology for advanced building-assistance systems. Front. Built Environ. 10:1378699. doi: 10.3389/fbuil.2024.1378699
Received: 30 January 2024; Accepted: 19 August 2024;
Published: 10 September 2024.
Edited by:
Olli Seppänen, School of Engineering, Aalto University, FinlandReviewed by:
Natalia E. Lozano-Ramírez, Pontificia Universidad Javeriana, ColombiaMohammad Mehdi Ghiai, University of Louisiana at Lafayette, United States
Gaetano Di Mino, University of Palermo, Italy
Copyright © 2024 Suo, Waje, Gunturu, Patlolla, Martani and Dib. This is an open-access article distributed under the terms of the Creative Commons Attribution License (CC BY). The use, distribution or reproduction in other forums is permitted, provided the original author(s) and the copyright owner(s) are credited and that the original publication in this journal is cited, in accordance with accepted academic practice. No use, distribution or reproduction is permitted which does not comply with these terms.
*Correspondence: Jiaqi Suo, c3VvakBwdXJkdWUuZWR1