- 1Department of Built Environment, Oslo Metropolitan University (OsloMet), Oslo, Norway
- 2Department of Civil and Architectural Engineering, Qatar University, Doha, Qatar
- 3Department of Materials, Mechanics, Management and Design, Delft University of Technology, Delft, Netherlands
Bridge infrastructure has great economic, social, and cultural value. Nevertheless, many of the infrastructural assets are in poor conservation condition as has been recently evidenced by the collapse of several bridges worldwide. The objective of this systematic review is to collect and synthesize state-of-the-art knowledge and information about how bridge information modeling, finite element modeling, and bridge health monitoring are combined and used in the creation of digital twins (DT) of bridges, and how these models could generate damage scenarios to be used by anomaly detection algorithms for damage detection on bridges, especially in bridges with cultural heritage value. A total of 76 relevant studies from 2017 up to 2022 have been taken into account in this review. The synthesis results show a consensus toward the future adoption of DT for bridge design, management, and operation among the scientific community and bridge practitioners. The main gaps identified are related to the lack of software interoperability, the required improvement of the performance of anomaly-detection algorithms, and the approach definition to be adopted for the integration of DT at the macro scale. Other potential developments are related to the implementation of Industry 5.0 concepts and ideas within DT frameworks.
1 Introduction
In 2018 the Morandi bridge collapsed in Genova, Italy, killing 43 people, forcing the displacement of 200 families living below the bridge, causing damages of EUR 422 million and yearly losses of EUR 784 million to the industry sector in the region (Xuequan, 2018). During the last 2 decades, the collapse of more than 120 bridges worldwide has caused major economic losses and casualties (Wang et al., 2022). A total of 9 661 structures representing 12.4% of all bridges and tunnels in Canada are reported to be in poor/very poor condition (Canada Infrastructure, 2019), whereas 46 ,154 bridges, equivalent to 7.5% of this kind of asset in the United States are considered structurally deficient (ASCE, 2021). In comparison, the percentages of deficient bridges in European countries such as France, Germany, and the United Kingdom are even higher, 39%, 30%, and 37%, respectively (European Commission et al., 2019). Besides, many old bridges are considered to have a Cultural Heritage (CH) value (Jiménez Rios and O’Dwyer, 2019) and some of them are even included in the UNESCO World Heritage List (World Heritage Centre, 2023) thanks to their outstanding universal cultural value. In addition to human and economic losses, the damage or collapse of a historical bridge also entails the painful loss of a cultural asset.
Because of the large number of existing bridges and the limited availability of human and economic resources (PIARC, 2023), it is not feasible to continuously inspect and assess the structural condition of every bridge using conventional methods. In the current practice, bridge inspections are performed on a code-prescriptive fixed-scheduled periodic basis varying between two to 6 years (EuroStruct, 2020). However, these periodic revisions have proven to be ineffective, as damage could appear after a periodic inspection and not be detected until the next one, leading to further deterioration of the bridge and increased cost of its eventual repair or replacement, if not to its collapse. In addition to the particular condition of a bridge, other factors can be considered in scheduling and performing bridge inspections. Most approaches consider the current and future usage of the bridge, its role in the transportation network, as well as other environmental, political, and social factors. It is of paramount importance to integrate CH values with bridge management methodologies, in agreement with international principles of conservation (Petzet, 2004), otherwise irreplaceable parts of our built environment may be lost forever.
A theoretical way to tackle the issue of insufficient resources at a network level, while adequately considering the CH value of a bridge, is to adopt a novel Digital Twin (DT) paradigm (Shabani et al., 2022). A DT of a bridge contains a virtual replica of a real-world bridge and a connectivity module that allows both the physical and virtual assets to be synchronized along the life cycle stages of the asset. The 3D geometry of the bridge can be created through a Bridge Information Modelling (BrIM) approach, whereas a structural twin can be constructed in Finite Element (FE) software. Sensors installed during a Bridge Health Monitoring (BHM) process can provide data about the environmental conditions, loads and response of the structure to those loads, either at local-element or global bridge scale. A series of damage and decay scenarios can be simulated on the virtual asset, which will reproduce the structural response of its physical counterpart through a series of FE models. This digital approach allows testing the bridge and generating the required data under several “normal” and “damaged” scenarios necessary for training Artificial Intelligence (AI) data-driven models such as Anomaly Detection Algorithms (ADAs) capable to detect damage in quasi-real time. The bridge management team or other stakeholders use the generated information to make an informed decision, thus optimizing the resources they have at their disposal. Therefore, the employment of a DT methodology leads to improved bridge performance and CH conservation, an increase in the bridge service life, and an eventual reduction of the maintenance and operation costs of the bridge network.
This systematic review aims to collect and synthesize state-of-the-art knowledge and information about how BrIM, FE, and BHM are combined and used in the creation of DTs of bridges and how the use of these models could generate damage scenarios to be employed by AI ADAs for damage detection on bridges (especially for bridges with high CH value). To this end, the proposed systematic review answers the following questions: i) what are the available ways to build bridge DTs based on BrIM, FEs, and BHM?; and ii) what are the available ADAs that could be used in the damage detection of conventional and CH bridges?
The value of this study lies in the need of having a comprehensive perspective of the current state of the art as the keystone for further research and development. The rest of this paper is organized as follows: Section 2 presents the methodology applied for the search strategy, bibliometric analysis, and synthesis of the found information, Section 3 contains the bibliometric results, and Section 4, the narrative synthesis. Finally, in Section 5 some conclusions are drawn, highlighting the gaps and further research suggestions derived from the systematic review work.
2 Methodology
The Preferred Reporting Items for Systematic Reviews and Meta-Analyses (PRISMA) 2020 methodology (Page et al., 2021a) was adopted. Thus, this systematic review has followed the checklist provided by PRISMA and a protocol was developed in accordance with the guidelines of the PRISMA-P Explanation and Elaboration (Page et al., 2021b). Following the guidelines, our systematic review protocol was registered in the Open Science Framework (OSF) Registries with registration number sh9b2 (Jiménez Rios et al., 2023b). The protocol of this systematic review can be consulted in Jiménez Rios et al. (2023d).
2.1 Search strategy
The quality of systematic reviews heavily relies on the search strategy implemented for the information retrieval process. Nevertheless, search strategies are commonly not adequately reported. This systematic review has adopted a search strategy methodology based on the PRISMA-S checklist (Rethlefsen et al., 2021) and it can be consulted in Jiménez Rios et al. (2023e).
The search strategy implemented was performed in Scopus because of its wide coverage of the literature, its high-quality content and its advanced data extraction capabilities (Elsevier, 2023). Initially, seven main keywords of interest were selected, namely, “bridge,” “digital twin,” “bridge information modeling,” “finite element methods,” “bridge health monitoring,” “anomaly detection algorithms” and “cultural heritage”). These keywords (and similar terms such as “bridge” and “bridges”) were combined to obtain six search queries in which every search combined a keyword with the “bridge” keyword. Thus, the queries obtained were.
• bridge* AND “digital twin*”
• bridge* AND (BrIM OR “bridge information model*“)
• bridge* AND (FEM OR FEA OR “finite element method*” OR “finite element analy*“)
• bridge* AND (“bridge health monitoring” OR “structural health monitoring”)
• bridge* AND (ADA OR “anomaly detection algorithm*“)
• bridge* AND (“cultural heritage” OR “monument* bridge*” OR “old bridge*” OR “ancient bridge*” OR “historic* bridge*“)
where * represents the wild character, AND and OR are Boolean operators, “⋅” are used to group individual words into multi-word keywords and (⋅) are used to group several similar terms. The six searches were limited to journal articles, conference papers, reviews, and book chapters, written in English, that were published after 2017, on the subject of Engineering. The searches were performed within the fields of title, abstract, and keywords. Table 1 presents the full queries used in the search, which was performed on 10/12/2022, and the respective number of records found for each one of them.
A total of 8 673 records were found. Detailed bibliometric information about all these records was downloaded from Scopus both in. ris and. csv format and has been made available in the open-source database Jiménez Rios et al. (2023a). Deduplication, filtering, screening, and eligibility assessment of all those records were carried out following PRISMA flow chart (Page et al., 2021a) (see Figure 1).
All duplicated records (based on the DOI number) and those records without a DOI number were removed. In total, 1 838 records were discarded after this first filtering. As a second filter, a search combination was performed. The initial six searches were combined using the AND operator (resulting in 15 new searches) to obtain relevant records dealing with at least three of the initially selected keywords (as the “bridge” keyword was used in all original six searches). Thus, 6 446 records were excluded and only 389 records remained, including the top-ten most cited papers from each one of the original searches (60 papers in total). The most cited papers were deemed to be of paramount importance to the state-of-the-art of the field due to their major impact on all related publications.
The selection phase (according to PRISMA 2020 item checklist #8) started at this point by manually screening the title and abstract of the remaining 389 records based on the authors’ criteria and previous knowledge of the field. Those records that did not fully fit within the scope of the review were excluded. Thus, 108 works remained and were subjected to full paper examination to assess their eligibility. From this list of 108 works, 2 were removed as they were duplicates, another 2 were excluded as they only dealt with the construction of new bridges, 12 more were not considered as they did not deal with DTs, and lastly, 16 papers were rejected as they were not related to bridges. As a result, a total of 76 studies were finally included in this systematic review.
2.2 Bibliometric analysis methodology
A bibliometric analysis represents a quantitative methodology by which meaningful insights can be obtained from large quantities of data (Broadus, 1987). The main outcomes of a bibliometric analysis are the identification of emerging research trends in a field, collaboration, and publication patterns, and exploration of literature structure (Koutsantonis et al., 2022). The approaches of a bibliometric analysis could be categorized into two main groups: performance analysis and science mapping (Solorzano and Plevris, 2022).
In this systematic review, the performance analysis was carried out by querying, filtering, and sorting the bibliographic database obtained from the search strategy, namely, using the 6835 records obtained after the first filtering phase, whereas the science mapping was performed using the VOSviewer v1.6.18 software (https://www.vosviewer.com/). Performance analysis is presented in terms of publications per year, most cited authors, most cited records, documents per country, keyword occurrence, and most used source for publication. On the other hand, science mapping focuses on analyzing the co-authorship relationships in terms of authors and countries, as well as the co-occurrence relationships between keywords (both author and index keywords). Keywords mapping allows visualizing the interconnections of core concepts and topics within a certain research area. For further insights into how the maps are created interested readers can consult (van Eck and Waltman, 2014) and the software manual (van Eck and Waltman, 2022).
2.3 Synthesis methodology
The information of the studies included in this systematic review has been qualitatively summarized in a narrative synthesis as the findings are characterized by heterogeneity. Data has been analyzed and classified within 5 major themes, namely,: i) Bridge DTs; ii) BrIM and FE modeling; iii) BHM, AI and ADAs; iv) Unmanned Aerial Vehicles (UAVs), satellite monitoring, and other DT-related emerging technologies for bridge inspection; and v) historical and CH bridges. Based on this classification, the findings of the systematic review are presented, the strengths and limitations of the studies are highlighted, their influence on practice and research is discussed, and future research recommendations are suggested.
3 Bibliometric analysis results and discussion
3.1 Performance analysis
Regarding the number of publications per year, Figure 2 shows that over 1 000 papers containing the keywords of interest of this systematic review were constantly published per year between 2017 and 2020. The trend though shows an increase in the number of publications from the last 2 years, with 25% and 50% increments on the number of yearly publications for the years 2021 and 2022, respectively.
Table 2 presents the top 20 most cited works (as of 10/12/2022, the day the search was conducted). The paper with the most citations is “Shaping the DT for design and production engineering” (Schleich et al., 2017) with a total of 644 citations. Nevertheless, after the filtering process shown in Figure 1, this paper was not included in this systematic review as it is not directly related to bridges. The paper included in this systematic review with the most citations is the second in the list, “Structural Health Monitoring Using Wireless Sensor Networks: A Comprehensive Survey” (Noel et al., 2017) with a total number of 273 citations in Scopus.
Another interesting metric related to citations is that of the most cited authors. This parameter considers the accumulated number of citations for all papers of an author. Thus, Wang, H., Li, H. and Bao, Y. are the most cited authors with 1308, 1276, and 1222 citations, respectively (see Figure 3).
Research is normally fostered at a national level by the National Research Council of each country. Figure 4 presents the countries with at least 100 publications in the field over the past 6 years. China is the country with the most publications (2599) followed by the United States and the United Kingdom with 1282 and 420 publications, respectively. The last country on the list is Turkey (out of the top 16 countries with more publications), with 105 publications. Note that the number of publications per country is based on the country of the authors’ affiliations, not on the nationality of the authors.
In terms of keywords occurrence, it is not surprising to find out that “bridges,” “FEM” and “SHM” are among the most frequently used keywords (based on the graphical information presented in Figure 5), as they were explicitly included in the search queries. On the other hand, the absence of terms such as “digital twins” and “bridge information modeling” may be explained by their relatively new adoption in the field, whereas the absence of keywords related to “cultural heritage” or “conservation” is directly tracked to the generalized lack of attention towards these topics by the engineering research community.
Most of the research considered in this systematic review has been published in three main scientific journals, namely, Engineering Structures, Journal of Bridge Engineering, and Lecture Notes in Civil Engineering, 445, 254, and 182 works in each one, respectively (see Figure 6). The total number of works concentrated in only these three main sources of publication represents 12.9% of the total number of records after deduplication found from the initial searches of this systematic review.
3.2 Science mapping
Co-author relationships are qualitatively analyzed using a network visualization map. Each circle in Figure 7 represents one of the top 100 authors with the most publications, as found after performing the search strategy described previously. The size of each circle depicts its strength or weight within the network, where larger circles correspond to authors with a larger number of publications. Moreover, the lines that are observed in this figure represent co-authorship links, in other words, who works mostly with whom. Analogously to the size of the items, the thickness of the links represents their strength, i.e., the strength of the co-authorship links of a given researcher with other researchers.
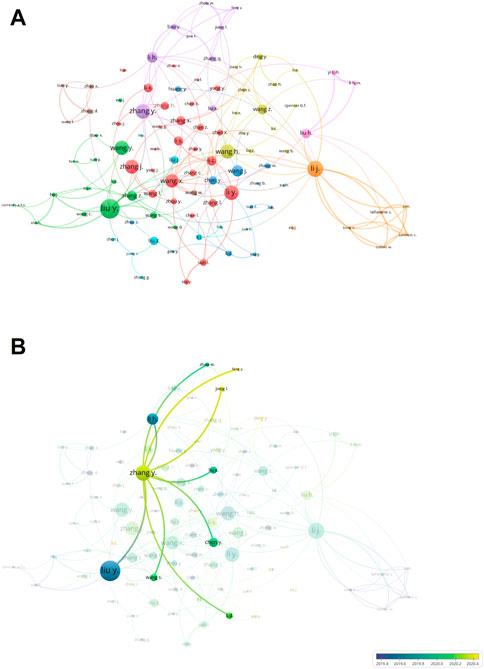
FIGURE 7. Co-authorship relationships in terms of authors: (A) Authors clusters and (B) Most recent publication averaged year per author, Zhang Y.
The items in Figure 7A are color-coded into nine different clusters based on network connectivity. Furthermore, Figure 7B shows an overlay visualization of the co-author relationships color-coded in terms of average publication year based on the scores assigned to each item of the network. It can be observed in Figure 7A that Liu Y. (green, 121 publications), Li J. (orange, 100 publications), Li Y. (red, 90 publications), Zhang Y. (pink, 90 publications) and Wang H. (yellow, 88 publications) are the centroids of the five more prominent clusters identified in the network. From these five networks, it can be seen in Figure 7B that the research group spear-headed by Zhang Y. is the one with the most recent average year of publication (2020.3).
Another interesting co-authorship relationship, now in terms of countries, is showcased in Figure 8. In this instance, three main clusters can be observed from Figure 8A where the strongest items are; China (pink, 2599 publications), United States (red, 1282 publications), followed by the United Kingdom and Italy (green, 420, and 415 publications, respectively). Among them, Italy is the country with the most recent average publication year (2020.09, see Figure 8B).
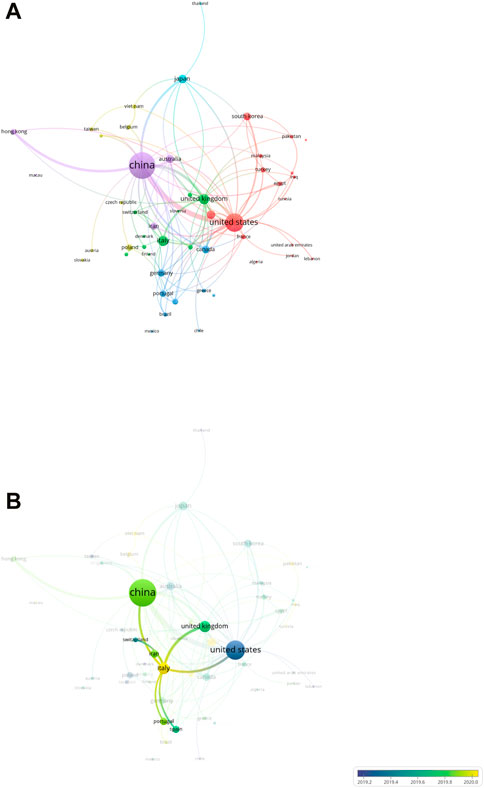
FIGURE 8. Co-authorship relationships in terms of countries: (A) Countries clusters and (B) country with the most recent average publication, Italy.
The co-occurrence relationships between keywords have similarly been analyzed through network and overlay visualization maps as displayed in Figure 9. The “bridges” keyword plays a predominant role in this network, which is not surprising because it is the main topic of interest in this systematic review. It is closely related to “SHM” and “Damage detection” as they belong to the same cluster (red) and have thick link lines (see Figure 9A). Regardless of its relatively small strength, “AI” has one of the most recent average publication years (2020.36), which shows its relatively new adoption in the field of bridge engineering (see Figure 9B).
4 Narrative synthesis and discussion
4.1 Bridge digital twins
The life-cycle stages of a bridge include: i) Planning and design; ii) Construction; iii) Inspection and maintenance; iv) Rehabilitation or replacement; and v) Demolition or decommissioning. Accounting for the entire life-cycle of a bridge within the DT paradigm requires the parallel evolution of both the digital and physical assets from the planning and design phase (inspection and maintenance for existing bridges) until the final demolition or decommissioning of the structure. For such purposes, deterioration models that can predict the progressive decay of the structural performance of the physical asset are of paramount importance (Cervenka et al., 2020; Jiang et al., 2021). Thus, Giorgadze et al. (2022) suggest an ontological modeling approach that includes not only components related to the structural elements of the bridge itself but also resources, processes, and risks related to the management and operation activities along the life of a bridge.
In terms of maturity, Shim et al. (2019) group DTs into three progressive categories based on their Level of Detail (LOD): i) partial DT (LOD 200–300, used during conceptual and detailed design/analysis); ii) clone DT (LOD 400, which provides construction information); and iii) augmented DT (LOD 500, capable of assisting during operation and management stages). Analogously, Kang et al. (2021) classify DT maturity into three progressive levels of complexity: functional, connected, and intelligent. Yet another classification based on the DT features and scopes is identified by Saback de Freitas Bello et al. (2022). In this threefold classification, a digital model replicates a physical asset but lacks data connectivity between the two, a digital shadow possesses automated one-way data connectivity between the physical and digital counterparts, and finally, in a digital twin the real-time data connectivity is granted in both directions and the digital asset evolves along with the physical one through its service life.
The multi-scale nature of DTs is explored in the work presented by Lu et al. (2020), where they develop a hierarchical architecture to build a DT at both city and building levels. According to the authors’ vision, the DT of a bridge could as well be integrated within the DT of a city, and this city DT would eventually form part of a DT at a national level. Although this vision makes sense for urban bridges, the integration of most bridges as part of transportation networks in rural or natural areas would perhaps be more appropriate if a DT is created at the transportation network level (including DTs of roads, tunnels, etc.) which could additionally be benefited from traffic data sharing tools, as explored by Dan et al. (2022). This suggests that the direction for the creation of macro-DTs that integrate DT of individual infrastructure assets is still not clearly defined and it needs to be determined whether a geographical, systemic, or another kind of ontological integration approach would be more favorable for grouping bridge DTs into the macro-DT of an entire transportation network, country, or continent.
A key component for the successful implementation of the DT paradigm in bridge monitoring is the integration of Cloud Computing (CC) within the adopted framework. Jeong et al. (2019) build on top of the OpenBrIM schema proposed by Jeong et al. (2017) and develop an Infrastructure as a Service (IaaS)/Platform as a Service (PaaS) CC environment in the Microsoft Azure cloud platform where an open-source distributed NoSQL database (Apache Cassandra) was employed to ensure scalability, flexibility, fault-tolerance, and high-performing data management. IaaS was offered in the form of Virtual Machines (VMs) that can be scaled either vertically (increasing the computational capabilities of the VM) or horizontally (by adding extra VMs). Furthermore, Software as a Service (SaaS) is provided through an online platform from where the user can query the information of interest and download the model that can be regenerated in structural engineering software such as CSIBridge.
To further improve the performance of a DT, Dang et al. (2022) propose the implementation of an intermediate level defined as Fog Computing (FC, computing done in the data generation device itself), which is capable of filtering the great amount of data generated by BHM systems before transferring only the relevant data to the CC layer. They also recognize the need for having several sub-models as part of the digital replica of a DT, each suitable for particular tasks, namely, analytical models based on mechanics and probability theory capable of providing exact and fast results in terms of structural response, reliability and safety for relatively simple idealized structures, physics-based numerical models (i.e., FE) which can replicate the structural response of complex systems for undamaged/damaged scenarios, be used for prognosis purposes and to generate synthetically augmented data. These data, along with the ones collected from the BHM of the physical asset, can be exploited by a third type of data-driven model, capable of performing real-time damage detection.
Along with FC intermediate data filtering, the implementation of enhanced data acquisition techniques such as compressive sampling, suitable for sparse data signals (Bao et al., 2019a), can drastically reduce the amount of data that would be stored and analyzed in the DT. In terms of visual acquisition data, the amount of information required for processing could be reduced if appropriate compression techniques and image quality percentages are adequately determined as done, for example, by Ri et al. (2020). By using a BrIM model in combination with Genetic Algorithms (GA) and Discrete Event Simulation (DES), Nili et al. (2021) propose a simulation-based framework to optimize bridge intervention (maintenance, rehabilitation and replacement) considering crew limitations. The framework is developed using Microsoft Visual Studio environment, Microsoft Access for the data management and data query, Autodesk Navisworks Manage as the BrIM application software, GA engine for the planning and sequencing modules, and a DES engine of Symphony core service, with a customized. Net programming language code. Nevertheless, this framework lacks consideration for CH conservation philosophy and methodologies when applied to bridges with CH value.
4.2 BrIM and FE modeling
The concept of BrIM is the adaptation of Building Information Modeling (BIM) methodologies applied to bridges (McKenna et al., 2017), whereas Historical BIM (HBIM), has been developed having CH buildings in mind (Pepe et al., 2020). Based on the life-cycle stage of the bridge in which the BrIM model is built, it could be classified as either as-designed, if the model is produced since the planning and design stage, or as-built, if it is created after the construction phase, or as-is, if the model has been effectively interconnected with the physical asset and is capable of updating its status along the further life-cycle stages of the structure (Hosamo and Hosamo, 2022).
Therefore, by following a multi-level and multi-modal approach as suggested by Xiao et al. (2017), an augmented As-Is Historical Bridge Information Modeling (AI-HBrIM), would be the adequate tool to implement the BIM methodology for existing historical bridges within the context of the DT paradigm. It is estimated that the adoption of this approach could result in up to a 30% reduction in traffic-related costs and a 10% reduction in the overall management and operation activities along the entire life of a bridge (Saju, 2022).
A suitable methodology to keep AI-HBrIM digital models interconnected to the physical asset is through FE model updating. Using this approach, the FE model is informed by the actual measured data coming from the physical asset (Yu et al., 2022). Ramancha et al. (2020) implement an advanced Bayesian inference approach using Sequential Monte Carlo (SMC) simulations to update the material and damping model parameters of a full-scale reinforced-concrete column under dynamic loading based on the heterogeneous data collected by accelerometers, strain gauges, GPS displacements, and potentiometers. Similarly, by applying a Bayesian inference approach, Ghahari et al. (2022) successfully update an FE model including soil-structure interaction effects. This was possible thanks to the motion identification at foundation level based on the acceleration measurement data obtained from the BHM.
While nowadays there are several data formats (specific protocols for data storing and retrieving) and schema (organization and structure such as XML, STEP, etc.) proposed for achieving AI-HBrIM interoperability, the OpenBrIM Platform (OpenBrIM, 2023) seems to be the most up-to-date option, whereas Industry Foundation Classes (IFC) (IFC, 2023) development team is currently preparing a new standard (IFC5) including data definitions required for both buildings and bridges over their life cycle. Both OpenBrim and IFC are XML schema-based. In this regard, Jeong et al. (2017) expand the OpenBrIM standard by enriching it with libraries for structural elements (e.g., mesh, constraints, and coordinate systems), load and analysis conditions (e.g., vehicle loads, modal, static, and multi-step) and sensors (e.g., accelerometers, strain gauges, and thermistors). The input data is organized and stored in a NoSQL database and Python is used to create the interface between the database and the analysis software (CSI Bridge) by parsing the XML objects. On the other hand, Park et al. (2018) propose using the functional meaning of bridge components (i.e., column, beam, etc.) to improve the usability of IFC applied to bridges by exploiting IFC’s basic modular structure and its framework for the sharing of information between various areas of the construction industry.
Another practicality that has received attention from researchers is the initial geometry modeling process of the AI-HBrIM model. Lu and Brilakis (2019) propose an automatic geometry modeling method to advance the creation of HBrIM models characterized by a slicing-based object-fitting approach. They recreated the geometry of an existing concrete bridge using 3D solid elements in IFC format based on a pre-processed labeled point cluster, a work previously presented by the same authors in Lu et al. (2019). Although their work was limited to a LOD level of 250 and only four general bridge elements, namely, slab, pier cap, pier, and girder, they achieved an impressive time reduction in comparison with manual geometric modeling techniques currently in practice.
Also in this subject, McKenna et al. (2017) present a case study where 3D laser scanning was undertaken to capture as-is geometry and condition data using a Leica P20 pulse-based Terrestrial Laser Scanner (TLS). Scans are colored using imagery obtained from a Nikon D200 camera mounted on a Nodal Ninja bracket to create high-resolution 360° panoramic images and then processed using Leica Cyclone proprietary software to create a 3D solid Autocad model of the structure. Two approaches have been followed to transform the CAD model into an HBrIM one. Leica CloudWorx for Revit is used first and then Autodesk ReCap software. Most of the modeling work is done manually, though.
As an alternative to conventional geometry data capture of existing bridges necessary to build a DT, Rashidi and Karan (2018) propose a low-cost, automatic, videogrammetry methodology. It consists of videotaping the bridge from several views and directions to reduce occlusions, transforming the 2D images captured into a 3D points cloud through the use of a Patch-based Multi-View Stereo (PMVS) algorithm, applying computer vision algorithms to identify the bridge components and exporting those elements to an XML format compatible with major BrIM software (RM Bridge, LEAP Bridge Enterprise, AutoCAD Civil 3D, Revit Structure, and Tekla Structures).
Although limited to presenting the applications, challenges, and recommendations of BIM applied to transportation infrastructure (without integration within the DT methodology), Costin et al. (2018) present a comprehensive review of BIM. They highlight the lack of interoperability within the different tools and methodologies currently in practice (Del Rio et al., 2020; Bouzas et al., 2022; Polania et al., 2022) as one of the main needs to be addressed to facilitate the implementation of BIM on the field of transportation infrastructure. Other significant challenges are the assurance of data quality, methodology cost reduction, inherent limitations, and institutional barriers as well as resistance to change by the industry agents.
4.3 BHM, AI, and ADAs
BHM aims to improve asset performance by measuring and learning from in-service structural behavior (Ye et al., 2022). Moreover, in earthquake-prone countries, BHM supports emergency management actions (Limongelli et al., 2019) and it can even be used to provide real-time traffic information (Burrello et al., 2020). BHM systems are usually designed based on the structural response observed on an a-priori FE model (Ye et al., 2020). Although model-based BHM approaches (Gonen and Soyoz, 2021; Gonen et al., 2023) can predict future bridges’ structural response under idealized load scenarios, their use results unfeasible for real-time damage detection applications (due to the high computational resources and the relatively long simulation periods required). With the rapid surge and adoption of AI, a new BHM and damage detection paradigm have recently gained importance: the model-free, also known as data-driven, paradigm. Data-driven methodologies can provide quasi-real-time results when damage occurs, on the other hand, they require large data to be trained and it is difficult to assign physical meaning to the detected damage. Moreover, databases containing information from real damaged bridges are scarce, as highlighted by Kim et al. (2021).
Neves et al. (2017) present a data-driven damage detection approach based on Machine Learning (ML). They test their methodology and overcome the lack of large data by creating a synthetic database with the help of an FE model. The data set consists of accelerations from 300 simulations of healthy and two damage scenarios of a bridge, of which 150 are used for training of an unsupervised Artificial Neural Network (ANN) and the remaining 150 for validation and verification purposes. Even though their approach is effective, the authors list a series of necessary improvements before it could be put into practice, such as considering the effect of environmental and operational conditions, including multiple damage scenarios, extending it for damage location and dealing with factors such as minimum reliability levels (the CH value of the bridge must as well be considered) for the determination of the threshold value. In that regard, Kostic and Gül (2017) try to include environmental and operational effects in their proposed ANN damage detection methodology by implementing a time series analysis, which allows for the successful detection of damage under low levels of temperature-induced noise
By leveraging the mutual advantages of model-based and data-driven approaches, Zhang and Sun (2021) develop a physics-guided ML monitoring strategy. Their methodology consists of training an ANN using a baseline undamaged condition from observations of a bridge and enriched with damage scenarios data synthetically generated through a FE model. To detect damage, it uses the Normalized Frequency Change Ratios (NFCR) and the change of the first several mode shapes of the bridge, combined in a novel cross-entropy loss function. According to the authors, this mixed approach is not only capable of detecting damage, but also of locating and quantifying it.
While some authors have focused on the development of damage-detection data-driven methodologies, others have tried to improve the BHM, which is traditionally based on bridge instrumentation and results economically unfeasible for short and medium-span bridges. Sreevallabhan et al. (2017) present a comprehensive literature review of Structural Health Monitoring (SHM) using Wireless Sensor Networks (WSNs), which are a low-cost alternative to the wired sensor networks commonly used nowadays. Wang et al. (2022) explore the installation and operation of novel piezoelectric transducers, which use a Coda Wave Interferometry (CWI) technique, to assess the condition of existing concrete bridges based on waves generated by the passing vehicles.
On the other hand, OBrien et al. (2017) propose an indirect bridge monitoring approach based on the instrumentation of the vehicles driving through the bridge. This so-called drive-by monitoring provides acceleration data that can be decomposed into three main components; vehicle frequency, bridge natural frequency, and pseudo frequency associated with vehicle speed. These three components are obtained through the means of Empirical Mode Decomposition (EMD). Drive-by monitoring approaches have proved effective not only in damage detection but also in damage location. A research gap identified by them that needs to be addressed to improve the effectiveness of drive-by monitoring is the effect that road roughness has on indirect monitoring. More recently, Locke et al. (2020) present a drive-by monitoring approach capable of not only considering road roughness, which is modeled based on power spectral density functions (International Organization for Standardization, 2016), but also variable environmental and operational conditions.
Another alternative proposed for BHM cost reduction consists of the use of non-contact vision-based displacement sensors to measure bridge displacements, which is a parameter directly related to the stiffness of the structure. These approaches exploit a series of available template matching/registration techniques such as Up-sampled Cross Correlation (UCC), pattern matching, edge detection, Orientation Code Matching (OCM), Digital Image Correlation (DIC), Hough transforms, and RANSAC (Feng and Feng, 2017). More recently, Shao et al. (2020, 2021) propose a holographic visual sensor coupled with computer-vision-based algorithms in a non-contact displacement and vibration measurement system, capable of capturing bridge full-field displacement and vibrations. Nevertheless, vision-based displacement sensors’ efficiency highly relies on image quality, which is commonly affected by illumination variation, partial target occlusion, partial shading, and background disturbance, factors usually present in normal bridge operational conditions. In this regard, Shao et al. (2020) suggest the use of denoising and contractive auto encoders to reduce low image-quality errors and improve visual-based monitoring effectiveness.
The problem of damage detection in bridge monitoring may be presented like a simple classification problem, i.e., identifying whether there is or there is not any damage in the bridge. However, the individual and highly complex nature of bridges may result in different dynamic responses, which adds complexity to the task. Conventional classification approaches are rarely successful due to the important imbalance between normal and anomalous cases, resulting in too many false negatives. An excessive number of false negatives may hinder the detection of actual damages or substantial decay, ultimately affecting the performance of a bridge and, in critical cases, leading to its collapse. Conversely, a large number of false positives would lead to unnecessary spending of resources. By contrast, an acceptable number of false positives may be even desirable for damage detection on CH bridges, which could be obtained with the application of a fine-tuned ADA. Table 3 presents a compilation of the diverse ADAs methodologies found in this systematic review.
A comprehensive review of supervised learning, unsupervised learning, novelty detection, and deep neural network methodologies used for generalized damage detection is provided by Sun et al. (2020). Furthermore, Ye et al. (2019) list a series of deep learning techniques specifically used for crack detection, damage detection, loosened bolt detection, and damage state classification of bridges. Finally, a comprehensive list of damage detection methods classified either as model-based (FE model updating) or data-driven (ML and statistical methods) can be found in Vagnoli et al. (2018).
4.4 UAVs, satellite monitoring, and other DT-related emerging technologies for bridge inspection
One recurrent topic found in this systematic review is the use of UAVs both to capture bridge geometry and generate HBrIM models and to automatically detect damage and decay mechanisms based on visual computing techniques (Mongelli et al., 2017; Roselli et al., 2018). Over the past few years, the use of UAVs has increased thanks to the reduction of their costs, improvement of stability and maneuverability, as well as the development of more efficient visual computing techniques. They provide more advantages than manual inspections in terms of time, accuracy, safety, and costs (Albeaino et al., 2019). Furthermore, the GPS signal loss suffered by UAVs below bridge decks during inspections, has been overcome by the implementation of an array of navigation sensors such as optical, infrared, and ultrasonic sensors as proved by Kang and Cha (2018). The ongoing development of automatizing UAV flights would further boost the use of UAVs for bridge monitoring as it would result in operational time reductions and path re-usability.
For example, Perry et al. (2020) report UAVs as a key element of an automatic streamlined bridge inspection system capable of identifying and locating bridge surface defects, and generating as-built BrIM models for the storage and visualization of damage information. Their methodology includes photogrammetry software (Meshroom) for the creation of 3D point clouds and photorealistic models, Gaussian Mixture Model and Agglomerative Clustering using Python Scikit-learn for element identification along with the use of Revit and Dynamo for the creation of the AI-HBrIM model containing 3D geometry and damage cubes. However, their approach lacks dealing with the integration of FE and structural analysis tools.
Yoon et al. (2022) assess bridge condition based on images captured with a UAV from which damage is automatically detected through a mask Region-based Convolutional Neural Network (R-CNN) algorithm. The methodology proposed by these authors also included a FE model updating module based on a linear stiffness reduction corresponding to the level of bridge condition assessment, as per the damage grades defined in South Korean accepted codes.
The main limitation of visual-based damage detection techniques is that they can not identify sub-surface damages such as reinforcement corrosion and concrete delamination. These techniques need to be complemented with the application of remote sensing technologies such as Ground-Penetrating Radar (GPR), Infrared Thermography (IR) (Xu and Turkan, 2020), or the use of piezoelectric electromechanical sensors that can detect internal damage in a relatively inexpensive way (Na and Baek, 2018). Furthermore, in a comparative study between UAV photogrammetry scanning capabilities against a conventional TLS, Mohammadi et al. (2021) conclude that TLS provides more accurate results and is more suitable for the complex implementation of creating an AI-HBrIM model within the DT paradigm.
Another monitoring technology that has grown in importance over the past few years thanks to its capability for real-time remote monitoring of displacements in bridges is the Interferometry Synthetic Aperture Radar (InSAR). This technology has benefited from the increased number of available satellites and their specialized tools capable of performing millimeter-accuracy measurements. Alani et al. (2020) use InSAR in combination with GPR to assess the integrity of a historical masonry bridge and the effects that local floods have on its displacement seasonal trends. More recently, by taking advantage of the improvements in data processing techniques and the availability of larger SAR databases; Gagliardi et al. (2022) manage to detect the seasonal deformation components of a historical masonry bridge based on an enhanced Multi-Temporal InSAR (MT-InSAR) methodology. One more bridge satellite monitoring case, coupled with hydraulic monitoring of river conditions, is reported by Bianchi et al. (2022).
Regardless of the impressive advancements in UAVs, satellites, and AI applications experienced over the past few years, it is evident that the human component can not be entirely removed from any DT framework. In this regard, Karaaslan et al. (2022) develop a human-centered approach using Mixed Reality (MR) to improve the quality and effectiveness of conventional bridge inspections. This is achieved through the use of Hololens (https://www.microsoft.com/en-us/hololens), which provides the bridge inspector with visual information in real-time about the bridge condition and defects.
4.5 Historical and CH bridges
Historical bridges with CH value require an extra layer of care and special considerations from the part of bridge managers and operators as they not only play a key role in transportation networks but also hold important social, cultural, and artistic values (Pachón et al., 2018). Any intervention performed in this type of bridge must abide by the principles of evidence-based, minimum and incremental intervention, removable and distinguishable measures, and material compatibility established in the Venice Charter (ICOMOS, 1964) and strive to preserve the bridge’s authenticity (ICOMOS, 1994). Furthermore, guidelines and recommendations found in the ISCARSAH documents (ICOMOS-ISCARSAH, 2003a; ICOMOS-ISCARSAH, 2003b) and in the Annex I of the ISO 13822 standard (International Organization for Standardization, 2010) must be followed to ensure the correct conservation of such valuable assets.
Interventions on CH bridges must be performed by a multidisciplinary team as shown by the work done by Conde et al. (2017) and Bautista-De Castro et al. (2018). Conde et al. (2017) carry out a comprehensive field survey fully based on non-destructive testing techniques, followed by accurate and detailed 3D FE simulations calibrated using the results obtained from a dynamic identification campaign based on an operational modal analysis approach. Bautista-De Castro et al. (2018) perform TLS, ambient vibration tests, and minor destructive tests. These works result in the detailed assessment of the corresponding bridges’ structural condition and the determination of their acceptable safety level. The full adoption of a DT approach is desirable during interventions of CH bridges. A DT would have the ability to monitor in real-time the structure and detect any possible damage induced by the intervention procedure itself as validated by Andersen et al. (2019) in the case of the Henry Hudson I89 Bridge in New York, thus complying with the observational approach suggested by conservation guidance.
Perhaps one of the most advanced tools for damage detection of bridges (in which the CH value is also considered), is the one presented by García-Macías and Ubertini (2020). Their MOVA/MOSS software is capable of automatically performing Operational Modal Analysis (OMA) and system identification through four different techniques: Enhanced Frequency Domain Decomposition (EFDD) and Polyreference Least Squares Complex Frequency Domain method (p-LSCF), both frequency-domain-based, Covariance driven Stochastic Subspace Identification (COV-SSI) and DATA-driven Stochastic Subspace Identification (DATA-SSI), these last two being time-domain-based. Subsequently, it executes frequency tracking and detects changes in the dynamic properties of the structure by applying statistical process control tools, namely, Hotelling, Multivariate Cumulative Sum (MCUSUM), and Multivariate Exponentially Weighted Moving Average (MEWMA). Finally, automatic damage detection is done through the implementation of the Pruned Exact Linear Time (PELT) Method. Their tool, unfortunately, is not part of any DT framework and compatibility issues may arise during integration with other modules of available frameworks.
Along with the conservation of CH value, a sustainable DT framework must as well account for robustness and resilience. Structural robustness is the capability of a structure to sustain a certain amount of damage without suffering full collapse, whereas the resilience of a structure refers to its ability to resist and withstand sudden shocks and sustained stresses, as well as its ability to recover from damage and continue to function effectively (Hajdin et al., 2018). Assessing both the robustness and resilience of a bridge implies dealing with a series of uncertainties related to material resistances and external loads. An adequate assessment methodology could be, as suggested by Futai et al. (2022), the implementation of reliability-based and risk-based performance indicators. It has been proved that modeling uncertainties can greatly be reduced by the adoption of a DT framework (Rojas-Mercedes et al., 2022).
5 Conclusion
Although the scope of not all research works found in this systematic review encompass the digital twin paradigm, and regardless of all the challenges and limitations still in place for its full deployment and implementation in real practice, there seems to be only one school of thought and a consensus toward the future adoption of digital twins for bridge design, management, and operation among the scientific community and bridge practitioners.
A suitable digital twin framework capable of accounting for the cultural heritage of existing bridges would be primarily based on the creation of an as-is historical bridge information model with fully inter-operable data, geometry, finite element, and data-driven modules. The as-is historical bridge information model would be kept interlinked to its physical asset counterpart through the implementation of a multi-metric bridge health monitoring system that constantly generates data about the structural, environmental, and operational conditions of the bridge. That data would be effectively generated by optimized sampling methodologies and would pass through an intermediate fog computing layer before its final processing at a cloud computing service.
Current research gaps in the practical development and implementation of digital twins are mainly related to i) the lack of interoperability among the different proprietary and open-source software used along the digital twin model generation pipeline; ii) performance improvement of currently available anomaly detection algorithms; and iii) the direction for the creation of macro-digital twins that integrate digital twins of individual infrastructure assets. The latter needs to be determined and the benefits/drawbacks of whether it is done at geographical, systemic, or another kind of ontological integration approach, needs to be assessed.
The digital twin paradigm was born within the Industry 4.0 era. Future potential developments in the field are related to the implementation of Industry 5.0 concepts and ideas within digital twin frameworks such as sustainability, human-centrism, and resilience (European Commission et al., 2022).
We are moving to a digital age where physical assets will increasingly have digital representations. New opportunities arise. Looking towards the future, it appears that digital twins in the built environment have the potential to play a significant role, in a wide range of applications throughout their entire life cycle. This is evidenced by the fast-growing occurrence of digital twin-related articles in the scientific literature during recent years. This study concludes that although some of the technologies discussed are relatively new and there are certainly several challenges to tackle, it has great potential to become an extremely positive force of change in the architecture, engineering, and construction industry. As engineers, it is our responsibility to facilitate the digital transformation of the architecture, engineering, and construction industry and to make it ready for the challenges and opportunities of the future, and digital twins are bound to play a pivotal role in this transformation.
Author contributions
All authors contributed to the conception and design of the study. AJ wrote the protocol and search strategy, carried out the search, organized the data and created the database, performed the bibliometric analysis, did the synthesis of the literature, and wrote the first draft of the manuscript. VP and MN supervised the work. All authors contributed to the manuscript revision, and read, and approved the submitted version. All authors have as well contributed to obtaining the necessary funding to carry out this work.
Funding
This project has received funding from the European Union’s Horizon 2020 research and innovation programme under the Marie Sklodowska-Curie grant agreement No. 101066739. The APC was funded by Oslo Metropolitan University.
Acknowledgments
The content of the manuscript has previously appeared online in the form of a preprint Jiménez Rios et al. (2023c).
Conflict of interest
The authors declare that the research was conducted in the absence of any commercial or financial relationships that could be construed as a potential conflict of interest.
Publisher’s note
All claims expressed in this article are solely those of the authors and do not necessarily represent those of their affiliated organizations, or those of the publisher, the editors and the reviewers. Any product that may be evaluated in this article, or claim that may be made by its manufacturer, is not guaranteed or endorsed by the publisher.
References
Alani, A. M., Tosti, F., Ciampoli, L. B., Gagliardi, V., and Benedetto, A. (2020). An integrated investigative approach in health monitoring of masonry arch bridges using gpr and insar technologies. NDT E Int. 115, 102288. doi:10.1016/j.ndteint.2020.102288
Albeaino, G., Gheisari, M., and Franz, B. W. (2019). A systematic review of unmanned aerial vehicle application areas and technologies in the aec domain. J. Inf. Technol. Constr. 24, 381–405. doi:10.36680/j.itcon.2019.020
Al-Ghalib, A. A. (2022). Structural damage detection of old ada steel truss bridge using vibration data. Struct. Control Health Monit. 29. doi:10.1002/stc.3098
An, Y., Chatzi, E., Sim, S.-H., Laflamme, S., Blachowski, B., and Ou, J. (2019). Recent progress and future trends on damage identification methods for bridge structures. Struct. Control Health Monit. 26. doi:10.1002/stc.2416
Andersen, J. E., Rex, S., and Gala, A., Hardesty & Hanover (2019). Structural health monitoring of henry hudson i89. Lyngby, Denmark: International Association for Bridge and Structural Engineering IABSE, 2121–2131. COWI.
Bao, Y., Chen, Z., Wei, S., Xu, Y., Tang, Z., and Li, H. (2019a). The state of the art of data science and engineering in structural health monitoring. Engineering 5, 234–242. doi:10.1016/j.eng.2018.11.027
Bao, Y., Tang, Z., Li, H., and Zhang, Y. (2019b). Computer vision and deep learning-based data anomaly detection method for structural health monitoring. Struct. Health Monit. 18, 401–421. doi:10.1177/1475921718757405
Bautista-De Castro, A., Sánchez-Aparicio, L. J., Ramos, L. F., Sena-Cruz, J., and González-Aguilera, D. (2018). Integrating geomatic approaches, operational modal analysis, advanced numerical and updating methods to evaluate the current safety conditions of the historical bôco bridge. Constr. Build. Mater. 158, 961–984. doi:10.1016/j.conbuildmat.2017.10.084
Bianchi, S., Biondini, F., D’Angelo, M., Ballio, F., Anghileri, M., Rosati, G., et al. (2022). “Satellite-based structural and hydraulic monitoring of a 50-year-old bridge over the oglio river in Italy,” in Lecture Notes in Civil engineering, 200, 380–389. doi:10.1007/978-3-030-91877-4_44
Bouzas, O., Cabaleiro, M., Conde, B., Cruz, Y., and Riveiro, B. (2022). Structural health control of historical steel structures using hbim. Autom. Constr. 140, 104308. doi:10.1016/j.autcon.2022.104308
Broadus, R. N. (1987). Toward a definition of “bibliometrics”. Scientometrics 12, 373–379. doi:10.1007/BF02016680
Burrello, A., Brunelli, D., Malavisi, M., and Benini, L. (2020). “Enhancing structural health monitoring with vehicle identification and tracking,” in 2020 IEEE International Instrumentation and Measurement Technology Conference (I2MTC) (Zurich, Switzerland: DEI, University of Bologna IIS, ETH, Institute of Electrical and Electronics Engineers Inc.), 9128641. doi:10.1109/I2MTC43012.2020.9128641
Canada Infrastructure (2019). Canadian infrastructure report card. Available at: http://canadianinfrastructure.ca/en/index.html.
Cervenka, J., Jendele, L., Zalsky, J., Pukl, R., Novak, D., Zhao, B., et al. (2020). Digital twin approach for durability and reliability assessment of bridges. Brno, Czech Republic: Dep. of Civil Engineering, Technical University of Brno, International Federation for Structural Concrete, 1840–1848.
Conde, B., Ramos, L. F., Oliveira, D. V., Riveiro, B., and Solla, M. (2017). Structural assessment of masonry arch bridges by combination of non-destructive testing techniques and three-dimensional numerical modelling: Application to vilanova bridge. Eng. Struct. 148, 621–638. doi:10.1016/j.engstruct.2017.07.011
Costin, A., Adibfar, A., Hu, H., and Chen, S. S. (2018). Building information modeling (bim) for transportation infrastructure - literature review, applications, challenges, and recommendations. Autom. Constr. 94, 257–281. doi:10.1016/j.autcon.2018.07.001
Dan, D., Ying, Y., and Ge, L. (2022). Digital twin system of bridges group based on machine vision fusion monitoring of bridge traffic load. IEEE Trans. Intell. Transp. Syst. 23, 22190–22205. doi:10.1109/TITS.2021.3130025
Dang, H., Tatipamula, M., and Nguyen, H. X. (2022). Cloud-based digital twinning for structural health monitoring using deep learning. IEEE Trans. Indust. Inf. 18, 3820–3830. doi:10.1109/TII.2021.3115119
Del Rio, I., Cabaleiro, M., Conde, B., Riveiro, B., Caamaño, J. C., El Naggar, H., et al. (2020). Hbim application to historical steel structures: The case study of lapela bridge. Vigo, CP 36208, Spain: Department of Materials Engineering Applied Mechanics and Construction, School of Industrial Engineering, University of Vigo, Avestia Publishing, 160–1–160–7. doi:10.11159/icsect20.160
Döhler, M., Hille, F., and Mevel, L. (2018). Vibration-based monitoring of civil structures with subspace-based damage detection. Intelligent Syst. Control Autom. Sci. Eng. 92, 307–326. doi:10.1007/978-3-319-68646-2_14
European CommissionJoint Research Centre Gkoumas, K., Balen, M., Grosso, M., Pekár, F., et al. (2019). Research and innovation in bridge maintenance, inspection and monitoring: A European perspective based on the transport research and innovation monitoring and information system (TRIMIS). Publications Office. doi:10.2760/16174
European CommissionDirectorate-General for Research and Innovation Renda, A., Schwaag Serger, S., Tataj, D., Morlet, A., et al. (2022). Industry 5.0, a transformative vision for europe: Governing systemic transformations towards a sustainable industry. Publications Office of the European Union. doi:10.2777/17322
Febrianto, E., Butler, L., Girolami, M., and Cirak, F. (2022). Digital twinning of self-sensing structures using the statistical finite element method. Data-Centric Eng. 3, e31. doi:10.1017/dce.2022.28
Feng, D., and Feng, M. Q. (2017). Experimental validation of cost-effective vision-based structural health monitoring. Mech. Syst. Signal Process. 88, 199–211. doi:10.1016/j.ymssp.2016.11.021
Futai, M. M., Bittencourt, T. N., Carvalho, H., and Ribeiro, D. M. (2022). Challenges in the application of digital transformation to inspection and maintenance of bridges. Struct. Infrastruct. Eng. 18, 1581–1600. doi:10.1080/15732479.2022.2063908
Gagliardi, V., Ciampoli, L. B., D’Amico, F., Alani, A. M., Tosti, F., Benedetto, A., et al. (2022). “Remote sensing measurements for the structural monitoring of historical masonry bridges,” in Lecture Notes in Civil engineering, 200, 632–641. doi:10.1007/978-3-030-91877-4_72
García-Macías, E., and Ubertini, F. (2020). Mova/moss: Two integrated software solutions for comprehensive structural health monitoring of structures. Mech. Syst. Signal Process. 143, 106830. doi:10.1016/j.ymssp.2020.106830
Ghahari, F., Malekghaini, N., Ebrahimian, H., and Taciroglu, E. (2022). Bridge digital twinning using an output-only bayesian model updating method and recorded seismic measurements. Sensors 22, 1278. doi:10.3390/s22031278
Giorgadze, I. M., Vahdatikhaki, F., and Voordijk, J. H. (2022). “Conceptual modeling of lifecycle digital twin architecture for bridges: A data structure approach,” in 39th International Symposium on Automation and Robotics in Construction (ISARC 2022) (Netherlands: Department of Construction Management Engineering, University of Twente, International Association for Automation and Robotics in Construction IAARC), 199–206.
Gonen, S., and Soyoz, S. (2021). “Dynamic identification of masonry arch bridges using multiple methodologies,” in Special topics in structural dynamics & experimental techniques. Editor D. S. Epp (Cham: Springer International Publishing), Vol. 5, 37–47.
Gonen, S., Demirlioglu, K., and Erduran, E. (2023). “Modal identification of a railway bridge under train crossings: A comparative study,” in Dynamics of Civil structures. Editors H. Y. Noh, M. Whelan, and P. S. Harvey (Cham: Springer International Publishing), Vol. 2, 33–40.
Hajdin, R., Powers, N., Frangopol, D. M., Al-Mahaidi, R., and Caprani, C. (2018). Managing existing bridges - on the brink of an exciting future. Faculty of Civil Engineering, University of Belgrade, CRC Press/Balkema, 63–87.
Hosamo, H. H., and Hosamo, M. H. (2022). Digital twin technology for bridge maintenance using 3d laser scanning: A review. Adv. Civ. Eng. 2022, 1–15. doi:10.1155/2022/2194949
ICOMOS (1964). International charter for the conservation and restoration of monuments and sites. London: ICOMOS.
ICOMOS (1994). “The nara document on authenticity,” in Ratified at Nara Conference on Authenticity in Relation to the World Heritage Convention, held at Nara, Japan, from 1-6 November, 1–6.
ICOMOS-ISCARSAH (2003a). “Principles for the analysis, conservation and structural restoration of architectural heritage,” in Ratified by the ICOMOS 14th General Assembly Victoria Falls, Zimbabwe.
ICOMOS-ISCARSAH (2003b). Recommendations for the analysis, conservation and structural restoration of architectural heritage. Available at: https://iscarsah.files.wordpress.com/2023/01/part-ii-e28093-guidelines.pdf.
International Organization for Standardization (2010). Iso 13822:2010 bases for design of structures - assessment of existing structures. Available at: https://www.iso.org/standard/46556.html.
International Organization for Standardization (2016). Iso 8608:2016 mechanical vibration: Road surface profiles: Reporting of measured data. Available at: https://www.iso.org/standard/71202.html.
Jeong, S., Hou, R., Lynch, J. P., Sohn, H., and Law, K. H. (2017). An information modeling framework for bridge monitoring. Adv. Eng. Softw. 114, 11–31. doi:10.1016/j.advengsoft.2017.05.009
Jeong, S., Hou, R., Lynch, J. P., Sohn, H., and Law, K. H. (2019). A scalable cloud-based cyberinfrastructure platform for bridge monitoring. Struct. Infrastructure Eng. 15, 82–102. doi:10.1080/15732479.2018.1500617
Jiang, F., Ding, Y., Song, Y., Geng, F., and Wang, Z. (2021). Digital twin-driven framework for fatigue life prediction of steel bridges using a probabilistic multiscale model: Application to segmental orthotropic steel deck specimen. Eng. Struct. 241, 112461. doi:10.1016/j.engstruct.2021.112461
Jiménez Rios, A., and O’Dwyer, D. (2019). “External post-tensioning system for the strengthening of historical stone masonry bridges,” in Structural analysis of historical constructions. Editors R. Aguilar, D. Torrealva, S. Moreira, M. A. Pando, and L. F. Ramos (Cham: Springer International Publishing), 1566–1574.
Jiménez Rios, A., Plevris, V., and Nogal, M. (2023a). Bibliographic data from the digital twin anomaly detection decision-making for bridge management systematic review. This project has received funding from the European Union’s Horizon 2020 research and innovation programme under the Marie Sklodowska- Curie grant agreement No 101066739. doi:10.5281/zenodo.7548017
Jiménez Rios, A., Plevris, V., and Nogal, M. (2023b). Digital twin anomaly detection decision-making for bridge management (dtadd). doi:10.17605/OSF.IO/SH9B2
Jiménez Rios, A., Plevris, V., and Nogal, M. (2023c). Dtadd systematic review preprint. This project has received funding from the European Union’s Horizon 2020 research and innovation programme under the Marie Sklodowska- Curie grant agreement No 101066739. doi:10.5281/zenodo.7673718
Jiménez Rios, A., Plevris, V., and Nogal, M. (2023d). Dtadd systematic review protocol (amended). This project has received funding from the European Union’s Horizon 2020 research and innovation programme under the Marie Sklodowska- Curie grant agreement No 101066739. doi:10.5281/zenodo.7546576
Jiménez Rios, A., Plevris, V., and Nogal, M. (2023e). Dtadd systematic review search strategy (amended). This project has received funding from the European Union’s Horizon 2020 research and innovation programme under the Marie Sklodowska- Curie grant agreement No 101066739. doi:10.5281/zenodo.7546557
Kang, D., and Cha, Y.-J. (2018). Autonomous uavs for structural health monitoring using deep learning and an ultrasonic beacon system with geo-tagging. Computer-Aided Civ. Infrastruct. Eng. 33, 885–902. doi:10.1111/mice.12375
Kang, J.-S., Chung, K., and Hong, E. J. (2021). Multimedia knowledge-based bridge health monitoring using digital twin. Multimedia Tools Appl. 80, 34609–34624. doi:10.1007/s11042-021-10649-x
Karaaslan, E., Zakaria, M., and Catbas, F. N. (2022). Mixed reality-assisted smart bridge inspection for future smart cities. Orlando, FL, United States: Department of Civil, Environmental, and Construction Engineering, University of Central Florida, Elsevier, 261–280. doi:10.1016/B978-0-12-817784-6.00002-3
Kim, C.-W., Zhang, F.-L., Chang, K.-C., McGetrick, P. J., and Goi, Y. (2021). Ambient and vehicle-induced vibration data of a steel truss bridge subject to artificial damage. J. Bridge Eng. 26. doi:10.1061/(ASCE)BE.1943-5592.0001730
Kostic, B., and Gül, M. (2017). Vibration-based damage detection of bridges under varying temperature effects using time-series analysis and artificial neural networks. J. Bridge Eng. 22. doi:10.1061/(ASCE)BE.1943-5592.0001085
Koutsantonis, D., Koutsantonis, K., Bakas, N. P., Plevris, V., Langousis, A., and Chatzichristofis, S. A. (2022). Bibliometric literature review of adaptive learning systems. Sustainability 14, 12684. doi:10.3390/su141912684
Li, L., Lei, B., and Mao, C. (2022). Digital twin in smart manufacturing. J. Indust. Inf. Integr. 26, 100289. doi:10.1016/j.jii.2021.100289
Limongelli, M. P., Dolce, M., Spina, D., Guéguen, P., Langlais, M., Wolinieck, D., et al. (2019). “S 2 hm in some European countries,” in Springer tracts in Civil engineering, 303–343. doi:10.1007/978-3-030-13976-6_13
Liu, Q., Zhang, H., Leng, J., and Chen, X. (2019). Digital twin-driven rapid individualised designing of automated flow-shop manufacturing system. Int. J. Prod. Res. 57, 3903–3919. doi:10.1080/00207543.2018.1471243
Locke, W., Sybrandt, J., Redmond, L., Safro, I., and Atamturktur, S. (2020). Using drive-by health monitoring to detect bridge damage considering environmental and operational effects. J. Sound Vib. 468, 115088. doi:10.1016/j.jsv.2019.115088
Lu, R., and Brilakis, I. (2019). Digital twinning of existing reinforced concrete bridges from labelled point clusters. Autom. Constr. 105, 102837. doi:10.1016/j.autcon.2019.102837
Lu, R., Brilakis, I., and Middleton, C. R. (2019). Detection of structural components in point clouds of existing rc bridges. Computer-Aided Civ. Infrastruct. Eng. 34, 191–212. doi:10.1111/mice.12407
Lu, Q., Parlikad, A. K., Woodall, P., Don Ranasinghe, G., Xie, X., Liang, Z., et al. (2020). Developing a digital twin at building and city levels: Case study of west cambridge campus. J. Manag. Eng. 36. doi:10.1061/(ASCE)ME.1943-5479.0000763
Mao, J.-X., Wang, H., Feng, D.-M., Tao, T.-Y., and Zheng, W.-Z. (2018). Investigation of dynamic properties of long-span cable-stayed bridges based on one-year monitoring data under normal operating condition. Struct. Control Health Monit. 25, e2146. doi:10.1002/stc.2146
McKenna, T., Minehane, M., O’Keeffe, B., O’Sullivan, G., and Ruane, K. (2017). Bridge information modelling (brim) for a listed viaduct. Proc. Institution Civ. Eng. Bridge Eng. 170, 192–203. doi:10.1680/jbren.16.00007
Meixedo, A., Ribeiro, D., Santos, J., Calçada, R., and Todd, M. D. (2022a). Real-time unsupervised detection of early damage in railway bridges using traffic-induced responses. Struct. Integr. 21, 117–142. doi:10.1007/978-3-030-81716-9_6
Meixedo, A., Santos, J., Ribeiro, D., Calçada, R., and Todd, M. D. (2022b). Online unsupervised detection of structural changes using train-induced dynamic responses. Mech. Syst. Signal Process. 165, 108268. doi:10.1016/j.ymssp.2021.108268
Mohammadi, M., Rashidi, M., Mousavi, V., Karami, A., Yu, Y., and Samali, B. (2021). “Case study on accuracy comparison of digital twins developed for a heritage bridge via uav photogrammetry and terrestrial laser scanning,” in 10th International Conference on Structural Health Monitoring of Intelligent Infrastructure, SHMII 10 (Italy: Department of Information Engineering and Computer Science, University of Trento, International Society for Structural Health Monitoring of Intelligent Infrastructure, ISHMII), 1713–1720.
Mongelli, M., De Canio, G., Roselli, I., Malena, M., Nacuzi, A., De Felice, G., et al. (2017). 3D photogrammetric reconstruction by drone scanning for FE analysis and crack pattern mapping of the “bridge of the towers”, spoleto. Key Eng. Mat.747, 423–430. doi:10.4028/www.scientific.net/kem.747.423
Moughty, J. J., and Casas, J. R. (2017). A state of the art review of modal-based damage detection in bridges: Development, challenges, and solutions. Appl. Sci. Switz. 7, 510. doi:10.3390/app7050510
Na, W. S., and Baek, J. (2018). A review of the piezoelectric electromechanical impedance based structural health monitoring technique for engineering structures. Sensors Switz. 18, 1307. doi:10.3390/s18051307
Neves, A. C., González, I., Leander, J., and Karoumi, R. (2017). Structural health monitoring of bridges: A model-free ann-based approach to damage detection. J. Civ. Struct. Health Monit. 7, 689–702. doi:10.1007/s13349-017-0252-5
Nili, M. H., Taghaddos, H., and Zahraie, B. (2021). Integrating discrete event simulation and genetic algorithm optimization for bridge maintenance planning. Automation Constr. 122, 103513. doi:10.1016/j.autcon.2020.103513
Noel, A. B., Abdaoui, A., Elfouly, T., Ahmed, M. H., Badawy, A., and Shehata, M. S. (2017). Structural health monitoring using wireless sensor networks: A comprehensive survey. IEEE Commun. Surv. Tutorials 19, 1403–1423. doi:10.1109/COMST.2017.2691551
Obrien, E. J., Malekjafarian, A., and González, A. (2017). Application of empirical mode decomposition to drive-by bridge damage detection. Eur. J. Mech. A/Solids 61, 151–163. doi:10.1016/j.euromechsol.2016.09.009
OpenBrIM (2023). “Openbrim platform where bridge engineering happens,” in OpenBrIM platform - engineering on cloud.
Pachón, P., Castro, R., García-Macías, E., Compan, V., and Puertas, E. (2018). E. torroja’s bridge: Tailored experimental setup for shm of a historical bridge with a reduced number of sensors. Eng. Struct. 162, 11–21. doi:10.1016/j.engstruct.2018.02.035
Page, M. J., McKenzie, J. E., Bossuyt, P. M., Boutron, I., Hoffmann, T. C., Mulrow, C. D., et al. (2021a). The prisma 2020 statement: An updated guideline for reporting systematic reviews. BMJ 372, n71. doi:10.1136/bmj.n71
Page, M. J., Moher, D., Bossuyt, P. M., Boutron, I., Hoffmann, T. C., Mulrow, C. D., et al. (2021b). Prisma 2020 explanation and elaboration: Updated guidance and exemplars for reporting systematic reviews. BMJ 372, n160. doi:10.1136/bmj.n160
Park, S. I., Park, J., Kim, B.-G., and Lee, S.-H. (2018). Improving applicability for information model of an ifc-based steel bridge in the design phase using functional meanings of bridge components. Appl. Sci. Switz. 8, 2531. doi:10.3390/app8122531
Pepe, M., Costantino, D., and Garofalo, A. R. (2020). An efficient pipeline to obtain 3d model for hbim and structural analysis purposes from 3d point clouds. Appl. Sci. Switz. 10, 1235. doi:10.3390/app10041235
Perry, B. J., Guo, Y., Atadero, R., and van de Lindt, J. W. (2020). Streamlined bridge inspection system utilizing unmanned aerial vehicles (uavs) and machine learning. Meas. J. Int. Meas. Confed. 164, 108048. doi:10.1016/j.measurement.2020.108048
Petzet, M. (2004). International Charters for Conservation and restoration = Chartes Internationales sur la conservation et la restauration = Cartas internacionales sobre la conservacion y la restauracion. ICOMOS.
PIARC (2023). Piarc asset management manual. World Road Association PIARC. Asset Management Manual — Asset Management ManualA guide for practitioners.
Polania, D. R., Tondolo, F., Osello, A., Fonsati, A., De Gaetani, C., Trincianti, C., et al. (2022). “Digitalization processes and bridge information modeling for existing bridges,” in Lecture Notes in Civil engineering, 200, 944–953. doi:10.1007/978-3-030-91877-4_108
Ramancha, M. K., Astroza, R., Conte, J. P., Restrepo, J. I., Todd, M. D., and Mao, Z. (2020). Bayesian nonlinear finite element model updating of a full-scale bridge-column using sequential Monte Carlo. Santiago, Chile: Facultad de Ingeniería y Ciencias Aplicadas, Universidad de los Andes, Springer, 389–397. doi:10.1007/978-3-030-47638-0_43
Rashidi, A., and Karan, E. (2018). Video to brim: Automated 3d as-built documentation of bridges. J. Perform. Constr. Facil. 32. doi:10.1061/(ASCE)CF.1943-5509.0001163
Ravizza, G., Ferrari, R., Rizzi, E., Dertimanis, V., Chatzi, E. N., Papadrakakis, M., et al. (2020). “An integrated monitoring strategy for current condition assessment of historic bridges,” in XI International Conference on Structural Dynamics (Stefano-Franscini-Platz 5, Zürich, CH-8093, Switzerland: ETH Zürich, Institute of Structural Engineering, Department of Civil, Environmental and Geomatic Engineering, European Association for Structural Dynamics), 1373–1387.
Rethlefsen, M. L., Kirtley, S., Waffenschmidt, S., Ayala, A. P., Moher, D., Page, M. J., et al. (2021). Prisma-s: An extension to the prisma statement for reporting literature searches in systematic reviews. Syst. Rev. 10, 39. doi:10.1186/s13643-020-01542-z
Ri, S., Tsuda, H., Chang, K., Hsu, S., Lo, F., and Lee, T. (2020). Dynamic deformation measurement by the sampling moiré method from video recording and its application to bridge engineering. Exp. Tech. 44, 313–327. doi:10.1007/s40799-019-00358-4
Rojas-Mercedes, N., Erazo, K., and Di Sarno, L. (2022). Seismic fragility curves for a concrete bridge using structural health monitoring and digital twins. Earthq. Struct. 22, 503–515. doi:10.12989/eas.2022.22.5.503
Roselli, I., Malena, M., Mongelli, M., Cavalagli, N., Gioffrè, M., De Canio, G., et al. (2018). Health assessment and ambient vibration testing of the “ponte delle torri” of spoleto during the 2016-2017 central ItItalyeismic sequence. J. Civ. Struct. Health Monit. 8, 199–216. doi:10.1007/s13349-018-0268-5
Saback de Freitas Bello, V., Popescu, C., Blanksvärd, T., Täljsten, B., Pellegrino, C., Faleschini, F., et al. (2022). “Framework for bridge management systems (bms) using digital twins,” in Lecture Notes in Civil engineering, 200, 687–694. doi:10.1007/978-3-030-91877-4_78
Saju, S. (2022). “Smart bridges based on bridge information modelling,” in 2022 IEEE International Conference on Signal Processing, Informatics, Communication and Energy Systems (SPICES) (Trivandrum, India: Bridges design unit, chief design office, Kerala Public Works Department, Institute of Electrical and Electronics Engineers Inc.), 279–284. doi:10.1109/SPICES52834.2022.9774179
Schleich, B., Anwer, N., Mathieu, L., and Wartzack, S. (2017). Shaping the digital twin for design and production engineering. CIRP Ann. - Manuf. Technol. 66, 141–144. doi:10.1016/j.cirp.2017.04.040
Shabani, A., Skamantzari, M., Tapinaki, S., Georgopoulos, A., Plevris, V., and Kioumarsi, M. (2022). 3d simulation models for developing digital twins of heritage structures: Challenges and strategies. Procedia Struct. Integr. 37, 314–320. doi:10.1016/j.prostr.2022.01.090
Shao, S., Zhou, Z., Deng, G., Du, P., Jian, C., and Yu, Z. (2020). Experiment of structural geometric morphology monitoring for bridges using holographic visual sensor. Sensors Switz. 20, 1187. doi:10.3390/s20041187
Shao, S., Deng, G., Zhou, Z., Rizzo, P., and Milazzo, A. (2021). Structural geometric morphology monitoring for bridges using holographic visual sensor. Lect. Notes Civ. Eng. 128, 3–13. doi:10.1007/978-3-030-64908-1_1
Shim, C.-S., Dang, N.-S., Lon, S., and Jeon, C.-H. (2019). Development of a bridge maintenance system for prestressed concrete bridges using 3d digital twin model. Struct. Infrastructure Eng. 15, 1319–1332. doi:10.1080/15732479.2019.1620789
Solorzano, G., and Plevris, V. (2022). Computational intelligence methods in simulation and modeling of structures: A state-of-the-art review using bibliometric maps. Front. Built Environ. 8. doi:10.3389/fbuil.2022.1049616
Soman, R., Kyriakides, M., Onoufriou, T., and Ostachowicz, W. (2018). Numerical evaluation of multi-metric data fusion based structural health monitoring of long span bridge structures. Struct. Infrastructure Eng. 14, 673–684. doi:10.1080/15732479.2017.1350984
Sreevallabhan, K., Chand, B. N., Ramasamy, S., Vijayaraghavan, R., Senthilnathan, K., Saral, A. M., et al. (2017). “Structural health monitoring using wireless sensor networks,” in IEEE Communications Surveys & Tutorials (Vellore-, Tamil Nadu, 632014, India: School of Electrical Engineering, VIT University, Institute of Physics Publishing). doi:10.1088/1757-899X/263/5/052015
Sun, L., Shang, Z., Xia, Y., Bhowmick, S., and Nagarajaiah, S. (2020). Review of bridge structural health monitoring aided by big data and artificial intelligence: From condition assessment to damage detection. J. Struct. Eng. (United States) 146. doi:10.1061/(ASCE)ST.1943-541X.0002535
Tang, Z., Chen, Z., Bao, Y., and Li, H. (2019). Convolutional neural network-based data anomaly detection method using multiple information for structural health monitoring. Struct. Control Health Monit. 26, e2296. doi:10.1002/stc.2296
Ubertini, F., Comanducci, G., Cavalagli, N., Laura Pisello, A., Luigi Materazzi, A., and Cotana, F. (2017). Environmental effects on natural frequencies of the san pietro bell tower in perugia, Italy, and their removal for structural performance assessment. Mech. Syst. Signal Process. 82, 307–322. doi:10.1016/j.ymssp.2016.05.025
Vagnoli, M., Remenyte-Prescott, R., and Andrews, J. (2018). Railway bridge structural health monitoring and fault detection: State-of-the-art methods and future challenges. Struct. Health Monit. 17, 971–1007. doi:10.1177/1475921717721137
van Eck, N. J., and Waltman, L. (2014). Visualizing bibliometric networks. Cham: Springer International Publishing, 285–320. doi:10.1007/978-3-319-10377-8_13
Wang, X., Niederleithinger, E., and Hindersmann, I. (2022). The installation of embedded ultrasonic transducers inside a bridge to monitor temperature and load influence using coda wave interferometry technique. Struct. Health Monit. 21, 913–927. doi:10.1177/14759217211014430
Weinstein, J. C., Sanayei, M., and Brenner, B. R. (2018). Bridge damage identification using artificial neural networks. J. Bridge Eng. 23. doi:10.1061/(ASCE)BE.1943-5592.0001302
Xiao, R., Lian, Y., Sun, B., Zhao, X., Liu, Z., Tang, P., et al. (2017). “Method of bridge structural analysis based on bridge information modeling,” in ASCE International Workshop on Computing in Civil Engineering 2017 (United States: School of Sustainable Engineering and the Built Environment, Arizona State Univ., American Society of Civil Engineers ASCE), 92–100. P.O. Box 873005, Tempe, AZ 85287-3005. doi:10.1061/9780784480823.012
Xu, Y., and Turkan, Y. (2020). Brim and uas for bridge inspections and management. Eng. Constr. Archit. Manag. 27, 785–807. doi:10.1108/ECAM-12-2018-0556
Xuequan, M. (2018). Morandi bridge absence costs Italy ”784 mln euros a year in lost gdp”. Report. Xinhua.
Ye, X. W., Jin, T., and Yun, C. B. (2019). A review on deep learning-based structural health monitoring of civil infrastructures. Smart Struct. Syst. 24, 567–585. doi:10.12989/sss.2019.24.5.567
Ye, S., Lai, X., Bartoli, I., and Aktan, A. E. (2020). Technology for condition and performance evaluation of highway bridges. J. Civ. Struct. Health Monit. 10, 573–594. doi:10.1007/s13349-020-00403-6
Ye, C., Kuok, S.-C., Butler, L. J., and Middleton, C. R. (2022). Implementing bridge model updating for operation and maintenance purposes: Examination based on UK practitioners’ views. Struct. Infrastructure Eng. 18, 1638–1657. doi:10.1080/15732479.2021.1914115
Yoon, H., Shin, J., and Spencer, B. F. (2018). Structural displacement measurement using an unmanned aerial system. Computer-Aided Civ. Infrastruct. Eng. 33, 183–192. doi:10.1111/mice.12338
Yoon, S., Lee, S., Kye, S., Kim, I.-H., Jung, H.-J., and Spencer, B. F. (2022). Seismic fragility analysis of deteriorated bridge structures employing a uav inspection-based updated digital twin. Struct. Multidiscip. Optim. 65, 346. doi:10.1007/s00158-022-03445-0
Yu, S., Li, D., and Ou, J. (2022). Digital twin-based structure health hybrid monitoring and fatigue evaluation of orthotropic steel deck in cable-stayed bridge. Struct. Control Health Monit. 29. doi:10.1002/stc.2976
Zhang, Z., and Sun, C. (2021). Structural damage identification via physics-guided machine learning: A methodology integrating pattern recognition with finite element model updating. Struct. Health Monit. 20, 1675–1688. doi:10.1177/1475921720927488
Zhang, H., Liu, Q., Chen, X., Zhang, D., and Leng, J. (2017). A digital twin-based approach for designing and multi-objective optimization of hollow glass production line. IEEE Access 5, 26901–26911. doi:10.1109/ACCESS.2017.2766453
Glossary
ADA Anomaly Detection Algorithm
AI Artificial Intelligence
ANN Artificial Neural Network
AI-HBrIM As-Is Historical Bridge Information Modeling
BIM Building Information Modeling
BHM Bridge Health Monitoring
BrIM Bridge Information Modelling
CH Cultural Heritage
CC Cloud Computing
CWI Coda Wave Interferometry
COV-SSI Covariance driven Stochastic Subspace Identification
DATA-SSI DATA-driven Stochastic Subspace Identification
DIC Digital Image Correlation
DES Discrete Event Simulation
DT Digital Twin
EMD Empirical Mode Decomposition
EFDD Enhanced Frequency Domain Decomposition
FC Fog Computing
FE Finite Element
GA Genetic Algorithms
GPR Ground-Penetrating Radar
HBIM Historical BIM
IFC Industry Foundation Classes
IR Infrared Thermography
IaaS Infrastructure as a Service
InSAR Interferometry Synthetic Aperture Radar
LOD Level of Detail
ML Machine Learning
MR Mixed Reality
MT-InSAR Multi-Temporal InSAR
MCUSUM Multivariate Cumulative Sum
MEWMA Multivariate Exponentially Weighted Moving Average
NFCR Normalized Frequency Change Ratios
OSF Open Science Framework
OMA Operational Modal Analysis
OCM Orientation Code Matching
PMVS Patch-based Multi-View Stereo
PaaS Platform as a Service
p-LSCF Polyreference Least Squares Complex Frequency Domain
PRISMA Preferred Reporting Items for Systematic Reviews and Meta-Analyses
PELT Pruned Exact Linear Time
R-CNN Region-based Convolutional Neural Network
SMC Sequential Monte Carlo
SaaS Software as a Service
SHM Structural Health Monitoring
TLS Terrestrial Laser Scanner
UAVs Unmanned Aerial Vehicles
UCC Up-sampled Cross Correlation
VM Virtual Machine
WSN Wireless Sensor Network.
Keywords: bridges, digital twins, anomaly detection algorithms, finite element method, cultural heritage conservation, bridge information modeling, bridge health monitoring
Citation: Jiménez Rios A, Plevris V and Nogal M (2023) Bridge management through digital twin-based anomaly detection systems: A systematic review. Front. Built Environ. 9:1176621. doi: 10.3389/fbuil.2023.1176621
Received: 28 February 2023; Accepted: 28 March 2023;
Published: 07 April 2023.
Edited by:
Giovanni Castellazzi, School of Engineering, University of Bologna, ItalyReviewed by:
Annalaura Vuoto, University of Minho, PortugalMarco Funari, University of Surrey, United Kingdom
Copyright © 2023 Jiménez Rios, Plevris and Nogal. This is an open-access article distributed under the terms of the Creative Commons Attribution License (CC BY). The use, distribution or reproduction in other forums is permitted, provided the original author(s) and the copyright owner(s) are credited and that the original publication in this journal is cited, in accordance with accepted academic practice. No use, distribution or reproduction is permitted which does not comply with these terms.
*Correspondence: Alejandro Jiménez Rios, alejand@oslomet.no