- 1Department of Civil Engineering and Architecture, Faculty of Engineering, University of Pavia, Pavia, Italy
- 2University School for Advanced Studies (IUSS), Pavia, Italy
- 3Department of Civil Engineering, Faculty of Engineering, University of Porto, Porto, Portugal
Given the ambitious targets of carbon emission reduction set for the very near future, it is now expected that retrofitting operations on existing buildings aim both at reducing their operational energy consumption and at improving their seismic performance. Indeed, it is now well acknowledged that, if a sole energy efficiency upgrade is provided to a given building, in case of an earthquake occurrence, double economic and environmental losses will be experienced due to both the lost investment for energy retrofitting and the repair and retrofitting activities for post-earthquake damage. Moreover, social losses may also be experienced in terms of casualties, injured or homeless due to the seismic and structural deficiencies of the existing structure. To aid thus the process of a coupled seismic/energy renovation of the existing building stock, several multi-criteria decision-making (MCDM) approaches have been developed for the identification of optimal retrofitting solutions for buildings. Such procedures typically consider a range of economic, social, technical, and, more recently, environmental aspects that are assumed to be of interest to decision makers (e.g., installation cost, duration of works, architectural impact, need for specialised workers, etc.). The present study demonstrates the application to a case-study school building of two different MCDM approaches, which account for seismic vulnerability and energy efficiency, as well as related environmental impacts of buildings. The main differences between the two procedures are explored in terms of considered decision-making parameters and corresponding weights, rankings of retrofitting options and identification of the optimal retrofitting strategies.
1 Introduction
The recently adopted Paris Agreement on Climate represents the international commitment to mitigate the impacts of climate change by keeping the rise in the global average temperature ideally below 1.5°C above pre-industrial levels. Such ambitious target is expected to be achieved by at least halving global carbon emissions by 2030 and by reaching climate neutrality by 2050. A survey of the Buildings Performance Institute Europe (BPIE, 2011) showed that more than 40% of the European building stock was built before the 60s, lacking modern design requirements for seismic safety and energy efficiency and thus being responsible for significant global greenhouse gas (GHG) emissions, raw material depletion and waste production. Such buildings, mostly reinforced concrete (RC) moment-resisting frames with unreinforced masonry (URM) infills, are extremely vulnerable to seismic events, and, at the same time, have poor energy performance. Their expected unfavourable seismic behaviour is due to poor structural detailing, low-quality materials, and high likelihood of critical failure mechanisms, such as soft storey or short columns. At the same time, lack of proper thermal insulation of opaque elements, inefficient transparent components, obsolete and energy-consuming mechanical equipment, and high dependence from fossil fuels are amongst the major deficiencies of existing buildings from the energy performance viewpoint. The need to foster actions aimed at maintaining the building stock in operational, safe, and resilient conditions is thus of primary importance in the context of the current climate change emergency.
For the reasons above, retrofitting strategies on existing buildings should be aimed at reducing their operational energy consumption as well as at improving their seismic performance. However, it is now acknowledged that climate change-related risks (i.e., extreme weather events, floods, hurricanes, etc.) will progressively become more frequent and severe, thus their impact on the building stock will also be gaining ever-increasing importance, which calls for future research activities aimed at considering not only seismic risk, but also such climate change-induced risks in existing buildings’ assessment and retrofitting activities. In addition to the environmental impacts due to buildings’ operational life (which is also affected by the retrofitting operations), due to either energy consumption or to natural hazards, those embodied in the retrofit materials and components should be considered as well from their production to their end-of-life treatment (Passoni et al., 2022b). This kind of approach, referred to as life cycle thinking (LCT), envisages indeed the minimisation of environmental, economic, and social impacts of multiple buildings’ life cycle phases, through the use of operative tools, including life cycle assessment (LCA), life cycle cost analysis (LCCA), and social life cycle assessment (S-LCA) procedures. The use of recycled or reused, recyclable or reusable, local and durable materials, the limitation of damage due to natural hazards, the dry installation and disassembly processes, the limited duration of works, the opportunity to carry out outside-only works, as well as the levels of structural protection and energy efficiency improvement, are some of the main LCT-compliant (environmental, economic, and social) features of retrofitting techniques. It is thus evident that the LCT approach promotes a wider and holistic perspective in buildings’ renovation. Such LCT principles, as discussed in more detail in Passoni et al. (2022a), can be interpreted as new performance targets for buildings and be used as well at a preliminary stage of the design of a building, enabling a pre-screening of available retrofitting techniques with a view to avoid the adoption of unsustainable solutions.
In the recent years, the scientific community has made significant efforts to quantify earthquake-induced environmental impacts, which are typically not included in standard LCA procedures, demonstrating their relevance, especially in regions with a high level of seismic hazard (e.g., Belleri and Marini, 2016). In tandem, researchers have also recently been focusing on the design of combined/integrated energy and structural retrofitting solutions, acknowledging the importance of improving both performances towards increased sustainability and the advantage of shared construction activities (e.g., Terracciano et al., 2015; Marini et al., 2017; Bournas, 2018; ReLUIS - DPC 2019–21 project, 2019; Menna et al., 2021; Pohoryles et al., 2022). In this ongoing research and development context, several methodologies have been proposed in the recent literature for the identification of optimal seismic and/or energy retrofitting solutions for buildings. Procedures, such as seismic resilience-based assessments (Cimellaro, 2013), index-based methods (Requena-García-Cruz et al., 2019), cost-benefit analyses (Sousa and Monteiro, 2018; Cardone et al., 2019), sustainability-based methods (Pons et al., 2016) and multi-criteria decision-making (MCDM) approaches (Caterino et al., 2008; Formisano and Mazzolani, 2015; Kumar et al., 2017a; Kumar et al., 2017b; Gentile and Galasso, 2019), have been developed and applied to case-study buildings to identify optimal seismic or energy retrofit strategies. A comparative assessment of some of these procedures has been recently carried out by Carofilis et al. (2022). Furthermore, advanced optimisation methods have been employed to develop optimal designs of seismic or energy retrofit interventions considering either a single criterion or multiple criteria (Mauro et al., 2017; Park et al., 2018; Falcone et al., 2019; Di Trapani et al., 2020; Rosso et al., 2020). However, such methods, which do not necessarily consider seismic and energy retrofitting in an integrated manner, mostly account for economic, social, and technical criteria that are typically of interest to decision makers (e.g., installation costs, duration of works, architectural impact, need for specialised workers, etc.). Only recently, environmental indicators have been included among the relevant decisional variables in some MCDM methods (Caruso et al., 2021; Caruso et al., 2023; Clemett et al., 2022; Clemett et al., 2023).
This study demonstrates the application of two different MCDM approaches (i.e., those recently developed by Caruso et al., 2023; Clemett et al., 2023) to a case-study school building. Both methods account for building seismic vulnerability and energy efficiency, as well as the related environmental impacts. The main differences between the two procedures are thus investigated in terms of decision-making parameters and corresponding weights, rankings of retrofitting options and identification of the optimal retrofitting solutions. A hybrid approach, which combines decision variables, weighting factors, and procedures, is also applied to the given building to provide further insights into the two analysed MCDM methods. Lastly, the influence of the geographic location is explored as well, assuming the building as located in three different sites in Italy, all characterised by similar moderate-high levels of seismic hazard, but with cold (C), moderate (M), and warm (W) climate conditions.
2 Multi-criteria decision-making (MCDM) approaches
The multi-criteria decision-making approach proposed by Clemett et al. (2023) considers the performance of alternative seismic/energy retrofitting strategies across a broad range of decision variables and uses a weighted average method to identify the optimal retrofitting solution. This approach follows indeed the technique for order preference by similarity to the ideal solution (TOPSIS), developed by Hwang and Yoon (1981), where the best option amongst a set of possible alternatives is identified as the one featuring the shortest distance with respect to an ideal solution and the farthest distance to a negative-ideal one, based on a relative weighting of decision-making criteria. One of the first applications of this procedure to compare alternative structural retrofit solutions for buildings is extensively described in Caterino et al. (2008).
The TOPSIS approach consists in the following steps. The decision maker is first asked to define a set of relevant criteria, which will be used to evaluate the relative performance of each retrofitting alternative considered. Each parameter of the set is then assigned a weighting factor based on how much important it is to the decision maker if compared to the other parameters (i.e., a higher weight factor indicates that a variable is assumed to be more important than one with a lower weight). In other words, weights are intended to amplify or de-amplify the relative importance of one decision variable with respect to another for each considered retrofitting solution. Weighting factors can be determined using rigorous methods, such as the analytical hierarchy procedure (AHP) (Saaty, 1980), or be based on engineering judgment. Once weights are defined for each alternative option, the ideal and least ideal solutions based on each single decision variable considered are identified, by taking for each criterion the best and worst performance values amongst the investigated options. These best and worst options can then be compared with all the other retrofitting options by calculating the n-space Euclidean distance between the decision matrix values for a single option and those of the ideal and least ideal solutions. Lastly, once the relative closeness of each alternative to the ideal solution is calculated, the one with the highest relative closeness (i.e., the farthest from the least ideal) is selected as the optimal solution. The relative closeness values vary between 0 and 1, with the unit value corresponding to the ideal solution.
The decision variables, and the corresponding weights, proposed in Clemett et al. (2023) for the identification of optimal seismic/energy retrofitting solutions according to the TOPSIS approach are the following:
• installation cost of the retrofit intervention (C1) (expressed in monetary terms), with a weighting factor of 0.15;
• post-retrofit life cycle costs (C2) (expressed in monetary terms), including contributions of expected seismic economic losses, energy costs, and maintenance cost of retrofit components, with a weighting factor of 0.19;
• post-retrofit life cycle carbon emissions (C3) (expressed in terms of equivalent carbon dioxide, or CO2e), including contributions of retrofitting intervention (installation, maintenance, and end of life), expected seismic environmental losses, and energy consumption impact, with a weighting factor of 0.18;
• annual probability of failure (C4), given by the seismic performance assessment, with a weighting factor of 0.14;
• duration of installation works (C5) (expressed in days), with a weighting factor of 0.13;
• architectural impact (C6), to be estimated through pairwise comparisons between the retrofitting alternatives, with a weighting factor of 0.06;
• need for specialised labour/design knowledge (C7), to be evaluated following a procedure analogous to the one used for C6, with a weighting factor of 0.05;
• required interventions at the foundations (C8), based on the maximum ratio of the vertical support reactions between the as-built configuration and each of the retrofitting alternatives, with a weighting factor of 0.1.
The weight vector indicated herein was determined using AHP and the professional judgment of the authors, assuming that the decision maker is the owner and operator of the building and that the retrofit interventions must be completed during the summer holiday period when the school is empty. A detailed discussion of additional assumptions for assigning weighting factors can be found in Carofilis et al. (2023) and Clemett et al. (2023). It is important, however, to underline that the choice of the weights is the largest source of uncertainty in this MCDM procedure, and it can significantly impact the results of the decision analysis, as demonstrated in Carofilis et al. (2023).
The MCDM approach proposed instead by Caruso et al. (2021), and further extended in Caruso et al. (2023), is, instead, based on four decision-making parameters and on the use of the so-called radar plots to (also visually) support the process of identification of optimal integrated retrofitting strategies. The considered decision variables are the following:
• post-retrofit life cycle costs (C) (expressed in monetary terms), including contributions of retrofitting intervention (installation, and end of life), expected seismic economic losses, and energy costs;
• post-retrofit life cycle carbon emissions (CE) (expressed in terms of CO2e), including contributions of retrofitting intervention (installation, and end of life), expected seismic environmental losses, and energy consumption impact;
• payback period (PB) of the retrofit investment (expressed in years);
• expected annual loss of life (EALL) due to seismic hazard (expressed in terms of fatalities per year).
Life cycle costs (C) and carbon emissions (CE) are evaluated throughout the post-retrofit building’s life by summing up the (economic and environmental) contributions of the retrofitting intervention (both installation and end of life), earthquake-induced losses, and operational energy consumption, and then normalised by the total floor area of the building and its expected post-retrofit life. The payback period of the retrofit investment indicates the number of years needed to fully pay back the initial investment through the monetary savings provided by the adopted retrofitting option (e.g., the reduction of seismic expected economic losses achieved through a seismic strengthening intervention). Finally, the expected annual fatalities can be estimated through the preferred casualty model (e.g., Coburn et al., 1992; ATC, 2018). Based on the above four parameters, the optimal retrofitting option for a given building can be determined through the use of radar plots by identifying the one with the smallest ensuing area, i.e., the one that concurrently minimises all four considered variables. For a proper representation of radar charts, the PB is normalised by the expected post-retrofit life of the given building, whilst the other three parameters are normalised by the as-built corresponding values, as shown in Section 4 for the case-study application. Differently from the procedure proposed by Clemett et al. (2023), in this second MCDM approach, any weighting factor is assigned to the decision variables, assuming all four parameters to be equally important.
3 Case-study building application
3.1 As-built building: integrated performance assessment
The case-study building is a school located in Isola del Gran Sasso d’Italia (Abruzzo, Italy), featuring a one-way RC moment resisting frame with URM infills. The structure was built in a period between 1960 and 1970, representing an example of typical Italian construction prior to the introduction of modern seismic design codes. The school is characterised by two aboveground stories and a small partial basement at the east end. The first and second floors each have areas of approximately 630 m2 and interstorey heights of 3.75 and 4.25 m, respectively. A more detailed description of the building, along with architectural plans and elevations, can be found in Prota et al. (2020). A numerical model of the case-study building for seismic performance assessment was developed using OpenSees (McKenna et al., 2010), as presented in Figure 1. The model consists of flexural elements (i.e., beams and columns), beam-column joints (BCJs), a staircase, and URM infills. Comprehensive information about the modelling of structural and non-structural elements of the case-study building can be found in the works of Carofilis et al. (2020), Carofilis et al. (2022). A preliminary seismic assessment, carried out through pushover analyses (as further described in Clemett et al., 2023), identified the moment capacity of the BCJs at which tensile cracking occurs and the shear capacity of the short columns adjacent to the URM infills and the ribbon windows as the most critical structural weaknesses of the building. Following the preliminary assessment, a detailed seismic loss assessment was performed, using multiple-stripe analysis (MSA), through the FEMA P-58 performance assessment and calculation tool (PACT) (ATC, 2018).
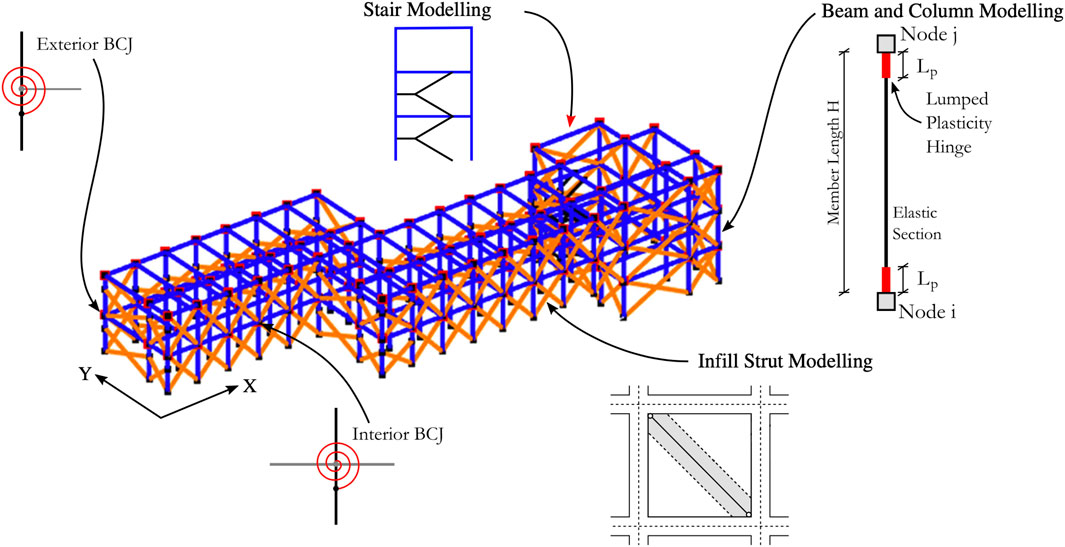
FIGURE 1. Three-dimensional representation of the numerical model developed in OpenSees (adapted from Clemett et al., 2023).
In tandem, the energy performance of the case-study building was assessed using the EDILCLIMA software (EDILCLIMA, 2021). Most of the modelling parameters were defined by local design codes based on the building’s occupancy type. Four different thermal zones, representing the basement, the ground floor, the first floor, and the stairwell, were included in the model. All thermal zones were assumed to be heated, with a heating set-point temperature of 20°C, supplied by a traditional natural gas boiler system. Domestic hot water was assumed to be provided by the same heating system used for space heating. Ventilation of the building was assumed to be provided naturally (i.e., no mechanical ventilation plant was considered). The thermal properties of the envelope elements, which are summarised in Clemett et al. (2023), were determined by defining the stratigraphy of each component’s constituent materials. Thermal bridges were also included to correct the two-dimensional heat flow that occurs at the intersections of different building’s elements (i.e., external wall elements, external and internal wall elements, external walls and RC columns, external walls and roof/interstorey floors/ground floor, external walls and windows).
3.2 Seismic/energy integrated retrofitting options
Following the preliminary seismic assessment, which identified the main structural deficiencies of the building, four seismic retrofit measures (SRMs) were designed according to the Italian building code (MIT, 2018) and analysed, as shown in Figure 2: S1—local strengthening of joints, columns, and beams with carbon fibre-reinforced polymer (CFRP); S2—global strengthening with concentric steel X-braces; S3—CFRP strengthening combined with concentric steel X-braces (S1+S2); S4—CFRP strengthening (S1) combined with viscous dampers. For all SRMs, URM infills were separated from the RC frames by the provision of a seismic gap to avoid column–infill interaction and reduce the shear forces acting on the columns.
Regarding the improvement of the energy performance of the building, three different combinations of energy retrofit measures (ERMs) were considered, as summarised in Figure 2: E1—roof insulation, installation of efficient LEDs and thermostatic valves on radiators; E2–E1 intervention coupled with external wall insulation with expanded polystyrene (EPS) panels; E3–E2 intervention coupled with installation of new efficient windows, floor insulation, condensing boiler, lighting control system and photovoltaic panels. Each considered energy retrofitting intervention aims to simultaneously reduce heat losses to the external environment and increase the energy efficiency of systems operating within the given building. From intervention E1 to E3 a higher level of performance requirements, as described in the Italian Ministerial Decree (Ministro Dello Sviluppo Economico, 2015), is achieved, though at the cost of an equally higher degree of invasiveness.
Lastly, all four seismic strengthening interventions were coupled with each energy upgrade option, leading to twelve possible combined retrofit alternatives (see Figure 2). Henceforth each coupled intervention will be referred to as SiEi, where Si and Ei correspond, respectively, to the reference number of the considered seismic and energy retrofit schemes. The detailed descriptions of the design procedures adopted for all retrofitting measures considered herein, together with any associated assumptions, are reported in previous research studies (Carofilis et al., 2020; Clemett et al., 2022; Clemett et al., 2023).
3.3 Retrofitted building: integrated performance assessment of the coupled retrofitting strategies
The seismic and energy performances of the twelve possible retrofitted configurations listed in Figure 2 were assessed using OpenSees (McKenna et al., 2010) and EDILCLIMA (EDILCLIMA, 2021), respectively, with the following assumptions:
• SRMs were assumed not to influence the energy performance of the retrofitted building (indeed, S1E1, S2E1, S3E1, S4E1 have the same energy performance);
• the influence of the ERMs on the structural model of the building was neglected, given that the considered types of measures mostly involve non-structural components (e.g., windows, lighting systems, etc.) that are not strongly influencing the structural behaviour of the building.
The impact of the SRMs on the seismic performance of the case-study structure was, first, preliminarily assessed through the N2 method and, subsequently, through a comprehensive FEMA P-58 performance-based seismic loss analysis. The results of the preliminary assessment through pushover analysis demonstrated an increase of the base shear capacity of the structure at the life safety limit state for all SRMs, with the largest improvements given by S3 and S4, and an increase of the interstorey drifts due to the separation of the URM infills from the RC frames (although still compliant with code-specified limits). The performance-based seismic assessment was then conducted through MSA, and, as a result, S4 provided the highest improvement, with a noticeable reduction of peak floor accelerations (PFAs) and peak storey drifts (PSDs) if compared to those of the as-built structure.
The energy performance of the building in each energy-retrofitted configuration was assessed in terms of primary energy consumption (PEC), annual equivalent CO2 emissions due to energy consumption, annual energy costs (AECs), and Italian energy class ratings, all summarised in Table 1. PEC represents the annual total energy consumption of the building, normalised by the floor area, and is used to determine the corresponding energy class rating. Energy classes are represented by a 10-letter scale (A4-G) and are assigned based on the performance of the building compared to that of a reference building with the same geometry and occupational class but with code-defined energy efficiency minimum requirements. By comparing the results obtained for the as-built configuration with the ones from each energy retrofitting intervention, the improvements of the energy performance of the case-study building are noticeable, progressively increasing from interventions E1 to E3, as expected.
Finally, a detailed seismic loss assessment was performed through the PACT calculation tool (ATC, 2018) for each combined seismic/energy retrofit interventions. The contribution of ERMs to seismic loss assessment was considered in terms of additional repair (economic and environmental) consequences of specific non-structural components and in terms of increased building reconstruction value (as illustrated in Table 2). Both parameters increase with the invasiveness of the intervention, from E1 to E3. It is also noted that the replacement cost and environmental impact (EI) were assumed, for simplicity, to be independent of the employed structural retrofit scheme.
The main results of the seismic loss assessment are shown in Table 3 in terms of expected annual loss (EAL), expected annual environmental impact (EAEI), annual probability of failure (APF), and expected annual loss of life (EALL), all of which will then be used within the two investigated MCDM methods. These results from the integrated assessment and retrofitting procedures shown in this Section were obtained considering the actual geographic location of the case-study building (i.e., site M).
4 Identification of optimal integrated retrofitting strategies
4.1 Decision-making variables
The decision variable (DV) values used in the Clemett et al. (2023) methodology, as well as the ranking (R) of each retrofitting option (Opt.) for site M, are summarised in Table 4. The alternative ranked as first, S4E3, represents the optimal solution, while the alternative ranked as twelfth (S1E2) is the worst-performing one.
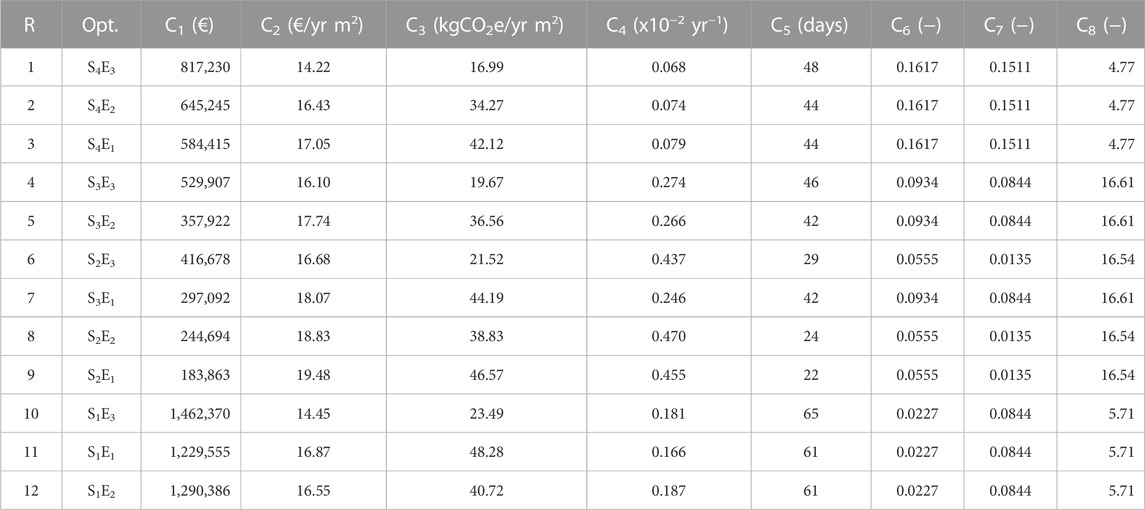
TABLE 4. Decision variable values used in the Clemett et al. methodology, and corresponding ranking.
In turn, Table 5 shows the decision variable values used in the Caruso et al. (2023) methodology, as well as the ranking of each alternative, while Figure 3 shows the radar plots for three coupled retrofitting interventions (namely, S1E1, S3E3, S4E3), representing the best and the worst solutions, and an intermediate one, still for site M, respectively. The red dashed lines refer to the as-built configuration, for which the normalised decision variables are equal to 1 (except for the PB, where the unit value refers to the expected post-retrofit life, which, in this case, was assumed as 20 years). Also when employing this second MDCM approach, option S4E3 resulted as the optimal solution, based on its smallest ensuing area in the radar plots.
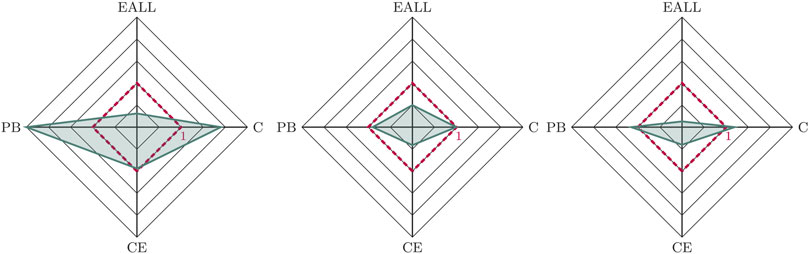
FIGURE 3. Examples of radar plots of three retrofitted configurations (i.e., S1E1, S3E3, S4E3) for site M, resulting from the Caruso et al. (2023) approach: normalised post-retrofit costs (C), carbon emissions (CE), payback period (PB) and expected annual loss of life (EALL).
In addition to the above MCDM methods, a hybrid approach that combines the decision variables and weighting factors of one procedure with the ranking approach of the other procedure was also applied to the given building. Specifically, each DV considered in the Clemett et al. (2023) approach was, first, normalised by the maximum DV value in the range of the considered retrofitting options, and then multiplied by the corresponding weighting factor (listed in Section 2). Radar plots, showing such normalised DV values, were then used to identify the optimal retrofitting solution based on the eight weighted parameters.
Table 6 summarises the rankings obtained through the application of all the three approaches, while Figure 4 shows the radar plots of the same three coupled retrofitting interventions indicated above (i.e., S1E1, S3E3, S4E3) obtained with the hybrid approach. It is noted that it was not possible to normalise the DVs with respect to the as-built configuration (as is done in Caruso et al., 2023), given that some parameters (i.e., installation cost, duration of works, architectural impact, need for specialised labour/design knowledge, and required interventions at the foundations) are null for the as-built configuration. For this reason, any red dashed line, such as the ones in Figure 3, is shown in Figure 4 to represent the as-built configuration.
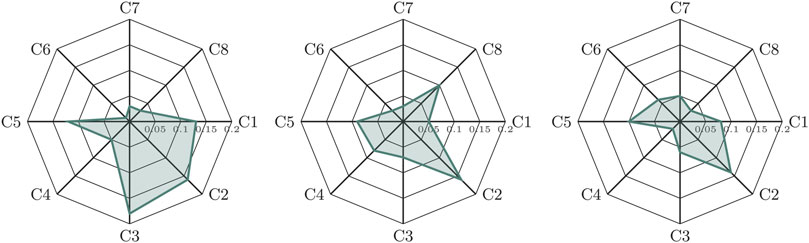
FIGURE 4. Examples of radar plots of three retrofitted configurations (i.e., S1E1, S3E3, S4E3) for site M, resulting from the hybrid approach: normalised installation cost (C1), post-retrofit life cycle costs (C2), post-retrofit life cycle carbon emissions (C3), annual probability of failure (C4), duration of works (C5), architectural impact (C6), need for specialised labour/design knowledge (C7), and required interventions at the foundations (C8).
Lastly, to also investigate if the procedures of identifying optimal retrofitting strategies are affected by different combinations of climatic conditions and seismic hazard level, the case-study building was assumed as located in two more geographic locations in Italy other than Isola del Gran Sasso d’Italia, which feature similar moderate-high levels of seismic hazard, but have cold (C) and warm (W) climates. As already mentioned, Isola del Gran Sasso d’Italia (latitude 42.5056° N, longitude 13.6592° E) was considered as representative of a moderate climate (M-type) site, while Città di Castello (latitude 43.4700° N, longitude 12.2314° E) and Catania (latitude 37.5013° N, longitude 15.0742° E) were assumed as representative of C-type and W-type sites, respectively. Table 7 summarises the rankings obtained through the application of all the three approaches to all three geographic locations.
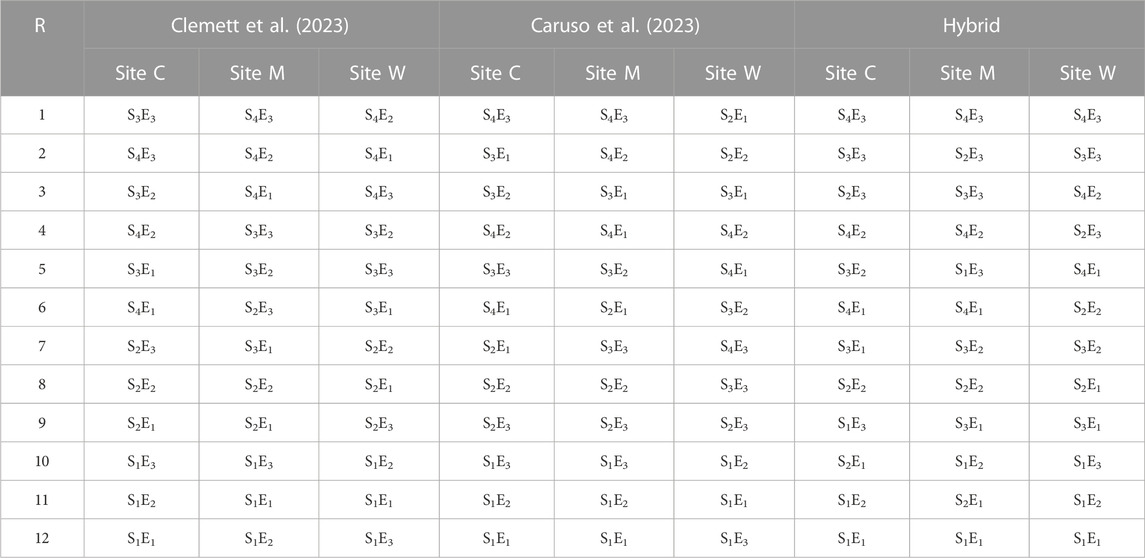
TABLE 7. Rankings comparison between the geographic locations considered, with cold (C), moderate (M), and warm (W) climates.
4.2 Methods comparison
From all the three methods analysed, it is observed that, for site M, the optimal solution is S4E3, which is the one coupling the global energy retrofitting intervention (roof, floors, and walls insulation + LEDs + thermostatic valves + new windows + condensing boiler + lighting control system + photovoltaic panels) and the structural intervention with CFRP and viscous dampers. Nevertheless, different rankings were achieved when applying the different methods in the three analysed locations as may be readily gathered from Table 7.
4.2.1 Overall ranking results
The ranking of alternatives obtained when applying the Clemett et al. (2023) methodology given in Table 4 indicates that, from the seismic viewpoint, S4 performs always better than S3, S3 better than S2, and S2 better than S1. Concerning the energy performances, similarly, E3 is always preferred over both E1 and E2. The surpassing of S2E3 over S3E1 on the ranking list demonstrates the higher benefits of the energy performance improvement of E3 with respect to the improved structural performance of S3. The top-ranking solution (S4E3) was observed to be the best solution based on four out of the eight decision variables considered, namely, post-retrofit life cycle costs (C2), post-retrofit life cycle carbon emissions (C3), annual probability of failure (C4) and required intervention at the foundations (C8). Table 5 illustrates that, when applying instead the Caruso et al. (2023) methodology, from the structural viewpoint, S1 is always the worst option, but the other solutions led to somehow different results. Indeed, S2 and S3 perform well in terms of life cycle costs and payback periods, moderately in terms of life cycle emissions, and inadequately in terms of EALL when compared to all other solutions. S4, instead, yields the lowest EALL values, and performs on average based on all the other variables. From the energy viewpoint, E3 is not always the best option, featuring very high costs (and, consequently, a high payback period) and environmental impacts due to the installation process. In addition, E3 solutions foresee seismic economic losses that are higher than costs of energy consumption, whereas the opposite occurs in terms of environmental impacts. Among the four considered decision variables, S4E3 was observed to be the best solution based on two of them, i.e., life cycle carbon emissions (CE) and expected annual loss of life (EALL). In fact, the absence of the EALL variable in the Clemett et al. (2023) methodology could justify, at least in part, some of the differences.
The rankings resulting for site M from the hybrid approach, instead, differ significantly from the other two (as shown in Table 6), except for the best solution (i.e., S4E3). Such a difference points out the strong influence of the ranking definition procedure adopted to identify the optimal solution between the range of options considered. Indeed, although the decision variables and the weighting factors are the same as those used in Clemett et al. (2023), the use of radar plots to select the option with the smallest ensuing area made the rankings change considerably.
4.2.2 Influence of climatic conditions
Table 7 shows the rankings obtained through the application of the three approaches to the other two sites, i.e., warm and cold sites (W and C, respectively). From the Clemett et al. (2023) methodology, the results for the warmer site W, where heating requirements are relatively modest and hence seismic retrofitting ends up playing a more impactful/dominant role in the integrated assessment, exhibit a clear trend compared to the other sites, being indeed grouped by structural retrofit scheme. From the Caruso et al. (2023) method, instead, preference is given to S2 especially due to its limited life cycle costs and payback period, as well as life cycle carbon emissions. In addition, from both methods, it appears that, in warmer climates, it may not always be necessary to provide high levels of energy efficiency, since the associated benefits do not always outweigh the costs when considered in an integrated seismic-energy MCDM framework. For what concerns the colder site C, from the Clemett et al. (2023) methodology, the increasing influence of the energy retrofitting schemes is evident in the rankings, whereas, from the Caruso et al. (2023) approach, high energy-efficiency retrofitting schemes are not all in the top rankings probably due to the higher balance of weights between seismic and energy related parameters. The hybrid approach still provides very different ranking trends if compared to the other two methods, with S4E3 resulting as the optimal retrofitting strategy for all three sites considered, and with measures achieving the highest levels of both seismic strengthening and energy efficiency always being in the top of the rankings. Examining the results across each of the sites and methodologies, it is noted that the rankings are significantly affected by the climatic conditions at the site of interest, and that, in general, as the heating demands increase, the impact of the energy retrofit alternatives on the rankings becomes more important, with the more energy-efficient retrofit options being the preferred solutions. It is seen as well that the S1 options are consistently ranked as the least preferred alternatives, mostly due to their high installation costs (around 2–6 times the cost of the other measures), while better results can be achieved through intervention techniques coupling CFRP with, e.g., X-braces or viscous dampers.
4.2.3 Ranking approach
The application of the two considered methods highlighted some relevant differences, not only in the rankings of retrofitting options, but also in the approach used. Although the two methods do estimate post-retrofit life cycle costs and carbon emissions in similar manners, concerning cost parameters, it is noted that, in Clemett et al. (2023), the installation cost (C1) is separated from other life cycle costs (C2), while in Caruso et al. (2023) it is included in the parameter C. On the other hand, regarding environmental parameters, C3, which refers to the total life cycle environmental impacts in Clemett et al. (2023), is defined similarly to life cycle carbon emissions (CE) in Caruso et al. (2023). A significant difference between the methods is related to the weights assigned to each variable and the ensuing results. In the Caruso et al. (2023) method, all four variables are assumed as equally important, thus a 25%-weight is assigned to each of them. On the other hand, in the Clemett et al. (2023) method, economic parameters (i.e., C1+C2) have a total weight of 34%, whereas life-cycle environmental impacts and annual probability of failure have weights of 18% and 14%, respectively. The overall similarity between the rankings resulting from the two methods (as seen in Table 6) may be related to the fact that the highest weights in the Clemett et al. (2023) method are assigned to (economic, environmental, and social) decision variables that are similar to those used in the Caruso et al. (2023) approach. Furthermore, in Caruso et al. (2023) the post-retrofit life is assumed to be 20 years, whereas in Clemett et al. (2023) the code-based nominal building life is considered (i.e., 75 years for a school building). As another point, Clemett et al. (2023) consider the economic and environmental contributions of maintenance of retrofit components in C2 and C3, which are instead currently unaccounted for in the current version of the procedure proposed by Caruso et al. (2023). Lastly, in the Clemett et al. (2023) approach a relative comparison is made between the options considered, i.e., the optimal solution is identified based on the relative closeness to an ideal solution, which is the best in the considered range of options. Differently, in the Caruso et al. (2023) approach, every retrofitting option is compared to the as-built configuration (through the normalisation of decision variables).
The demonstrative application to the case-study building of a hybrid approach also underlined the need to further investigate which decision variables should be used to aid the process of identification of optimal integrated retrofitting solutions. Namely, two different types of decision variables can be potentially identified. The first would include “objective” parameters, whose importance is not assigned by decision makers based on personal opinion, but is instead acknowledged by professionals to be relevant in a decision-making framework (e.g., the reduction of buildings environmental impacts is now worldwide recognised as of primary importance for the future of our planet and society, although not necessarily a priority for an individual decision maker). The second would include “subjective” parameters, which are instead strictly related to the needs of the decision maker and to the specific building features and requirements (including, e.g., economic budget, duration of works, architectural impact, need for occupants relocation, etc.), and could thus be considered in a pre-screening phase (Passoni et al., 2022a), where available retrofitting techniques are filtered based on the decision-maker requests.
5 Conclusion
Several multi-criteria decision-making (MCDM) approaches have been recently proposed in the scientific literature for the identification of optimal retrofitting strategies for existing buildings. The present study demonstrates the application to a case-study building of two alternative MCDM approaches, namely, those recently developed by Caruso et al. (2023) and Clemett et al. (2023), which consider economic, environmental, and social aspects that are assumed to be of interest to decision makers when planning retrofitting activities (e.g., running costs and environmental impacts, duration of works, architectural impact, need for specialised workers, etc.). An existing school building, located in Isola del Gran Sasso d’Italia (Central Italy), representative of typical Italian construction prior to the introduction of modern seismic and energy design codes, was provided with twelve integrated retrofitting options, and the two MCDM approaches were used to select the optimal seismic/energy retrofitting strategy. For the site with moderate climatic conditions (i.e., site M), the optimal solution resulted by the application of both methodologies was identified as S4E3, i.e., the one coupling the global energy retrofitting intervention (roof, floors, and walls insulation + LEDs + thermostatic valves + new windows + condensing boiler + lighting control system + photovoltaic panels) and the structural intervention with CFRP and viscous dampers.
Despite the global agreement shown in the rankings of Table 6, some relevant differences between the approaches were found through their application to the case-study building, mostly in terms of the type of decision variables considered, the definition of economic decision variables and expected post-retrofit building life, the weighting factors assigned to each decision variable, and the methodology with which the alternative retrofitting options are compared. It is noted that a direct quantitative comparison between the decision-making parameters used in both methods is not straightforward, given that they are all defined in different ways.
First, concerning economic parameters, Clemett et al. (2023) consider two different economic variables, i.e., the costs of retrofit installation (C1) and the expected annual costs (C2), whereas a global life cycle economic estimate (C) is used in Caruso et al. (2023). On the contrary, C3, which refers to the total life cycle environmental impacts in Clemett et al. (2023), is defined similarly to life cycle carbon emissions (CE) in Caruso et al. (2023). For what concerns weighting factors, Caruso et al. (2023) allocate the same importance to all four variables, thus leading to a 25%-weight each, while, in the Clemett et al. (2023) method, different weights are assigned to each of the decision variables. Namely, economic parameters (C1+C2) are assigned a total weight of 34%, whereas life-cycle environmental impacts (C3) and annual probability of failure (C4) have weights of 18% and 14%, respectively. The overall similarity between the rankings, as visible in Table 6, may be explained by the fact that the highest weights in the Clemett et al. (2023) method are allocated to the decision variables that are more similar to those used in the Caruso et al. (2023) approach. Another different assumption is made in terms of post-retrofit life, which, in Caruso et al. (2023), is assumed to be 20 years, whereas in Clemett et al. (2023) the code-based nominal building life is considered (i.e., 75 years for a school building). Moreover, Caruso et al. (2023) neglect the costs and environmental impacts due to maintenance of retrofitting components, whose contribution, however, could be included in future applications. Lastly, in the Clemett et al. (2023) approach, the alternative options are evaluated based on a relative comparison, thus the optimal solution is identified based on the relative closeness to an ideal solution, being the best in the considered set of options. Differently, in the Caruso et al. (2023) approach, every retrofitting option is compared to the as-built configuration by normalising all the decision variables of interest.
The exemplificative application of a hybrid procedure, combining decision variables and weighting factors of both MCDM approaches, was also carried out. The ensuing rankings proved to be very different when compared to those from the other two methods (Table 6). Indeed, although the decision variables and the weighting factors are the same as those used in Clemett et al. (2023), the use of radar plots to select the option with the smallest ensuing area made the rankings change considerably. This difference highlighted the need to further explore the possibility of distinguishing between two types of decision variables; one corresponding to objective parameters, whose importance is not assigned by a decision maker based on personal opinion, but is instead considered as relevant by practitioners, and the other associated to subjective parameters, which are strictly related to the needs of the decision maker and the specific building considered.
Moreover, to investigate how different combinations of climatic conditions and seismic hazard level could influence the results, the case-study building was assumed as located in two more geographic sites in Italy other than Isola del Gran Sasso d’Italia, which feature similar moderate-high levels of seismic hazard but have cold (C) and warm (W) climates. Città di Castello (Central Italy) and Catania (Southern Italy) were assumed as representative of C-type and W-type sites, respectively. Across each of the sites and methodologies, the rankings resulted as significantly affected by the climatic conditions at the site of interest. In general, for increasing heating demands, the impact of the energy retrofit alternatives on the rankings becomes more important, with the more energy-efficient retrofit options being the preferred solutions. S1 options are consistently ranked as the least preferred alternatives, mostly due to their high installation costs, while better results can be achieved through intervention techniques coupling CFRP with, e.g., X-braces or viscous dampers.
Future developments of this study include further investigation on the optimal decision variables (and corresponding weighting factors) to be used in MCDM frameworks, through additional case-study building applications, as well as on the possibility to include other types of natural hazards (e.g., climate change-induced risks) in the integrated assessment and retrofitting procedures for existing buildings.
Data availability statement
The original contributions presented in the study are included in the article, further inquiries can be directed to the corresponding author.
Author contributions
All authors (MC, RC, RP, and RM) were involved in conceptualization, methodology, analysis, data curation, draft preparation, review and editing of the manuscript. RP and RM were involved in project administration and funding acquisition. All authors contributed to the article and approved the submitted version.
Funding
This research was funded by the Italian Civil Protection Department (Dipartimento della Protezione Civile), within the framework of the ReLUIS-DPC 2022–2024 research project, more specifically its WP5 activities (focused on the development and verification of integrated seismic and energy retrofitting solutions) and by the Portuguese Foundation for Science and Technology, through the project SERENE—2022.08138.PTDC.
Conflict of interest
The authors declare that the research was conducted in the absence of any commercial or financial relationships that could be construed as a potential conflict of interest.
Publisher’s note
All claims expressed in this article are solely those of the authors and do not necessarily represent those of their affiliated organizations, or those of the publisher, the editors and the reviewers. Any product that may be evaluated in this article, or claim that may be made by its manufacturer, is not guaranteed or endorsed by the publisher.
References
Applied Technology Council (ATC) (2018). Seismic performance assessment of buildings, volume 1-methodology, rep. No. FEMA P-58-1, federal emergency management agency (FEMA): washing-ton, D.C. Available at: https://femap58.atcouncil.org/reports (Accessed July, 2023).
Belleri, A., and Marini, A. (2016). Does seismic risk affect the environmental impact of existing buildings? Energy Build. 110, 149–158. doi:10.1016/j.enbuild.2015.10.048
Bournas, D. A. (2018). Concurrent seismic and energy retrofitting of RC and masonry building envelopes using inorganic textile-based composites combined with insulation materials: a new concept. Compos. B Eng. 148, 166–179. doi:10.1016/j.compositesb.2018.04.002
Building Performance Institute Europe (BPIE) (2011). Europe’s buildings under the microscope: A country-by-country review of the energy performance of the buildings. Brussel, Belgium: Building Performance Institute Europe.
Cardone, D., Gesualdi, G., and Perrone, G. (2019). Cost-benefit analysis of alternative retrofit strategies for RC frame buildings. J. Earthq. Eng. 23, 208–241. doi:10.1080/13632469.2017.1323041
Carofilis, W., Clemett, N., Gabbianelli, G., O’Reilly, G., and Monteiro, R. (2023). Influence of parameter uncertainty in multi-criteria decision-making when identifying optimal retrofitting strategies for RC buildings. J. Earthq. Eng. 27 (7), 1769–1794. doi:10.1080/13632469.2022.2087794
Carofilis, W., Gabbianelli, G., and Monteiro, R. (2022). Assessment of multi-criteria evaluation procedures for identification of optimal seismic retrofitting strategies for existing RC buildings. J. Earthq. Eng. 26 (11), 5539–5572. doi:10.1080/13632469.2021.1878074
Carofilis, W., Perrone, D., O’Reilly, G. J., Monteiro, R., and Filiatrault, A. (2020). Seismic retrofit of existing school buildings in Italy: performance evaluation and loss estimation. Eng. Struct. 225, 111243. doi:10.1016/j.engstruct.2020.111243
Caruso, M., Pinho, R., Bianchi, F., Cavalieri, F., and Lemmo, M. (2023). Multi-criteria decision-making approach for optimal seismic/energy retrofitting of existing buildings. Earthq. Spectra 39 (1), 191–217. doi:10.1177/87552930221141917
Caruso, M., Pinho, R., Bianchi, F., Cavalieri, F., and Lemmo, M. T. (2021). Integrated economic and environmental building classification and optimal seismic vulnerability/energy efficiency retrofitting. Bull. Earthq. Eng. 19, 3627–3670. doi:10.1007/s10518-021-01101-4
Caterino, N., Iervolino, I., Manfredi, G., and Cosenza, E. (2008). Multi-criteria decision making for seismic retrofitting of RC structures. J. Earthq. Eng. 12 (4), 555–583. doi:10.1080/13632460701572872
Cimellaro, G. P. (2013). “Resilience-based design (RBD) modelling of civil infrastructure to assess seismic hazards,” in Handbook of seismic risk analysis and management of Civil infrastructure systems (Elsevier), 268–303. doi:10.1533/9780857098986.2.268
Clemett, N., Carofilis, W., Gabbianelli, G., O’Reilly, G. J., and Monteiro, R. (2023). Optimal combined seismic and energy efficiency retrofitting for existing buildings in Italy. J. Struct. Eng. 149 (1), 04022207. doi:10.1061/(ASCE)ST.1943-541X.0003500
Clemett, N., Carofilis, W., O'Reilly, G. J., Gabbianelli, G., and Monteiro, R. (2022). Optimal seismic retrofitting of existing buildings considering environmental impact. Eng. Struct. 250, 113391. doi:10.1016/j.engstruct.2021.113391
Coburn, A. W., Spence, R. J., and Pomonis, A. (1992). “Factors determining human casualty levels in earthquakes: mortality prediction in building collapse,” in Proceedings of the 10th world conference on earthquake engineering, madrid, 19–24 july (New York: CRC Press), 5989–5994.
Di Trapani, F., Malavisi, M., Marano, G. C., Sberna, A. P., and Greco, R. (2020). Optimal seismic retrofitting of reinforced concrete buildings by steel-jacketing using a genetic algorithm-based framework. Eng. Struct. 219, 110864. doi:10.1016/j.engstruct.2020.110864
EDILCLIMA (EDILCLIMA Software and Engineering) (2021). EC700 calcolo prestazioni energetiche degli edifici—versione 11. Borgomanero, Italy: EDILCLIMA software and engineering. Available at: https://www.edilclima.it/ (Accessed July, 2023).
Falcone, R., Carrabs, F., Cerulli, R., Lima, C., and Martinelli, E. (2019). Seismic retrofitting of existing RC buildings: A rational selection procedure based on genetic algorithms. Structures 22, 310–326. doi:10.1016/j.istruc.2019.08.006
Formisano, A., and Mazzolani, F. M. (2015). On the selection by MCDM methods of the optimal system for seismic retrofitting and vertical addition of existing buildings. Comput. Struct. 159 (5451), 1–13. doi:10.1016/j.compstruc.2015.06.016
Gentile, R., and Galasso, C. (2019). Shedding some light on multi-criteria decision making for seismic retrofitting of RC buildings. Seced conference 2019: Earthquake risk and engineering towards a resilient world. London: Greenwich.
Hwang, C. L., and Yoon, K. (1981). “Multiple attribute decision making,” in Lecture notes in economics and mathematical systems (Berlin, Germany: Springer-Verlag, Berlin), 186.
Kumar, A., Sah, B., Deng, Y., He, X., Kumar, P., and Bansal, R. (2017a). Application of multi-criteria decision analysis tool for design of a sustainable micro-grid for a remote village in the Himalayas. J. Eng. 2017 (13), 2108–2113. doi:10.1049/joe.2017.0702
Kumar, A., Sah, B., Singh, A. R., Deng, Y., He, X., Kumar, P., et al. (2017b). A review of multi criteria decision making (MCDM) towards sustainable renewable energy development. Renew. Sust. Energ. Rev. 69, 596–609. doi:10.1016/j.rser.2016.11.191
Marini, A., Passoni, C., Belleri, A., Feroldi, F., Preti, M., Metelli, G., et al. (2017). Combining seismic retrofit with energy refurbishment for the sustainable renovation of RC buildings: A proof of concept. Eur. J. Environ. Civ. 26 (7), 2475–2495. doi:10.1080/19648189.2017.1363665
Mauro, G., Menna, C., Vitiello, U., Asprone, D., Ascione, F., Bianco, N., et al. (2017). A multi-step approach to assess the lifecycle economic impact of seismic risk on optimal energy retrofit. Sustainability 9 (6), 989. doi:10.3390/su9060989
McKenna, F., Scott, M. H., and Fenves, G. L. (2010). Nonlinear finite-element analysis software architecture using object composition. J. Comput. Civ. Eng. 24 (1), 95–107. doi:10.1061/(asce)cp.1943-5487.0000002
Menna, C., Del Vecchio, C., Di Ludovico, M., Mauro, G. M., Ascione, F., and Prota, A. (2021). Conceptual design of integrated seismic and energy retrofit interventions. J. Build. Eng. 38, 102190. doi:10.1016/j.jobe.2021.102190
Ministero delle Infrastrutture e dei Trasporti (Mit), (2018). Aggiornamento delle Norme Tecniche per le costruzioni (Gazzetta Ufficiale della Repubblica Italiana No.42). Rome, Italy.
Ministro Dello Sviluppo Economico (2015). Decreto interministeriale 26 Giugno 2015, Applicazione delle metodologie di calcolo delle prestazioni energetiche e definizione delle prescrizioni e dei requisiti minimi degli edifici. Rome: Italian Government.
Park, H. S., Hwang, J. W., and Oh, B. K. (2018). Integrated analysis model for assessing CO2 emissions, seismic performance, and costs of buildings through performance-based optimal seismic design with sustainability. Energy Build. 158, 761–775. doi:10.1016/j.enbuild.2017.10.070
Passoni, C., Caruso, M., Marini, A., Pinho, R., and Landolfo, R. (2022a). The role of life cycle structural engineering in the transition towards a sustainable building renovation: available tools and research needs. Build 12 (8), 1107. doi:10.3390/buildings12081107
Passoni, C., Palumbo, E., Pinho, R., and Marini, A. (2022b). The LCT challenge: defining new design objectives to increase the sustainability of building retrofit interventions. Sustainability 14 (14), 8860. doi:10.3390/su14148860
Pohoryles, D. A., Bournas, D. A., Da Porto, F., Caprino, A., Santarsiero, G., and Triantafillou, T. (2022). Integrated seismic and energy retrofitting of existing buildings: A state-of-the-art review. J. Build. Eng. 61, 105274. doi:10.1016/j.jobe.2022.105274
Pons, O., De la Fuente, A., and Aguado, A. (2016). The use of MIVES as a sustainability assessment MCDM method for architecture and civil engineering applications. Sustainability 8, 460. doi:10.3390/su8050460
Prota, A., Di Ludovico, M., Del Vecchio, C., and Menna, C. (2020). Progetto DPC-ReLUIS 2019-2021 WP5: Interventi di rapida esecuzione a basso impatto ed integrati.
Requena-García-Cruz, M. V., Morales-Esteban, A., Durand-Neyra, P., and Estêvão, J. M. C. (2019). An index-based method for evaluating seismic retrofitting techniques. Application to a reinforced concrete primary school in Huelva. PLoS One 14 (4), e0215120. doi:10.1371/journal.pone.0215120
Rosso, F., Ciancio, V., Dell’Olmo, J., and Salata, F. (2020). Multi-objective optimization of building retrofit in the Mediterranean climate by means of genetic algorithm application. Energy Build. 216, 109945. doi:10.1016/j.enbuild.2020.109945
Sousa, L., and Monteiro, R. (2018). Seismic retrofit options for non-structural building partition walls: impact on loss estimation and cost-benefit analysis. Eng. Struct. 161, 8–27. doi:10.1016/j.engstruct.2018.01.028
Keywords: building renovation, seismic loss assessment, energy performance assessment, environmental impact, optimal retrofitting strategies, multi-criteria decision-making
Citation: Caruso M, Couto R, Pinho R and Monteiro R (2023) Decision-making approaches for optimal seismic/energy integrated retrofitting of existing buildings. Front. Built Environ. 9:1176515. doi: 10.3389/fbuil.2023.1176515
Received: 28 February 2023; Accepted: 31 July 2023;
Published: 11 August 2023.
Edited by:
Naida Ademovic, University of Sarajevo, Bosnia and HerzegovinaReviewed by:
Antonio Formisano, University of Naples Federico II, ItalyAristidis Papachristidis, 3DR Engineering Software Ltd., Greece
Copyright © 2023 Caruso, Couto, Pinho and Monteiro. This is an open-access article distributed under the terms of the Creative Commons Attribution License (CC BY). The use, distribution or reproduction in other forums is permitted, provided the original author(s) and the copyright owner(s) are credited and that the original publication in this journal is cited, in accordance with accepted academic practice. No use, distribution or reproduction is permitted which does not comply with these terms.
*Correspondence: Martina Caruso, martina.caruso@unipv.it