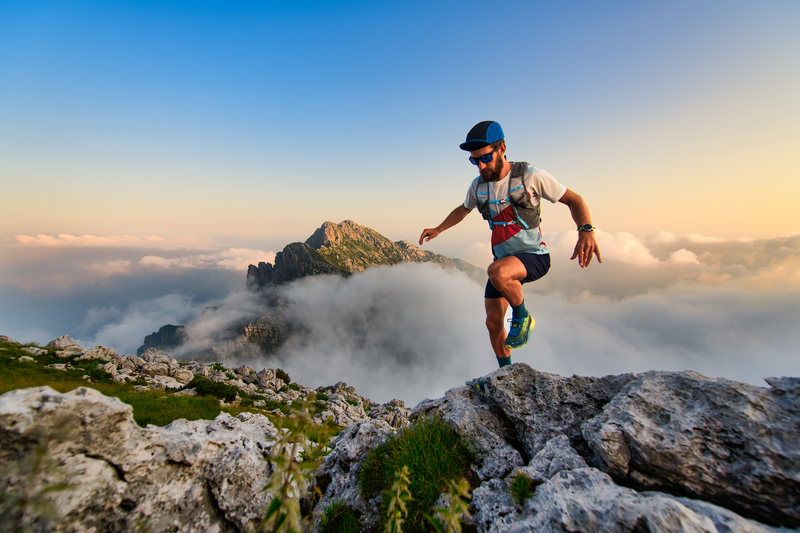
94% of researchers rate our articles as excellent or good
Learn more about the work of our research integrity team to safeguard the quality of each article we publish.
Find out more
ORIGINAL RESEARCH article
Front. Built Environ. , 27 February 2023
Sec. Construction Management
Volume 9 - 2023 | https://doi.org/10.3389/fbuil.2023.1079203
This article is part of the Research Topic Doctoral Research in Construction Management View all 10 articles
For decades, labor shortage has been a consistent struggle in the construction industry. At the same time, technological innovations have played a central role in the growth and development of an increasingly diverse construction industry. Existing research indicates that technological adoption is crucial for enhancing project productivity. Despite the importance and potential benefits of technology use, no research has yet studied how technology use on-site impact the worker’s performance and the difficulty of the process to access needed information. The objective of this paper is to analyze and understand the impact of on-site technology use on 1) the self-evaluated performance record (including safety, attendance, quality, productivity, and initiative), and 2) the difficulty of the process to access information, of construction workers and frontline supervisors in the construction industry. To achieve the research objective, 2,780 construction craft workers and frontline supervisors were surveyed using an online questionnaire. The survey participants were asked to self-evaluate 1) their work personal performance record (including safety, attendance, quality, productivity, and initiative), and 2) the difficulty of the process to receive or get access to information. The participants were additionally asked to specify whether different listed information technologies, material technologies, and equipment technologies are used on site. The collected data was then analyzed. Key findings indicate that the on-site use of several technologies had statistically significant impact in increased worker performance and information access. This study contributes to the body of knowledge by empirically quantifying the impact of on-site technology use on worker performance and information access in the construction industry.
The US construction industry stands at a crossroads. The aging infrastructure of the United States is in dire need for renewal as we face a major shortage of labor and craft workers (Construction Industry Institute, 2021). Recent trend data indicates that the construction workforce is aging fast causing an industry wide labor shortage (Construction Industry Institute, 2016). The consensus by industry experts is that the construction industry will look radically different over the next 20 years (Ribeirinho et al., 2020). With the increasing level of sophistication in the construction industry, companies are turning to innovative technologies and approaches to gain a competitive advantage (Renz et al., 2016). At the same time, construction technologies are being developed faster than the rate of their implementation, and the industry is yet to benefit from their full potential (Construction Industry Institute, 2021). An analysis by McKinsey and Company of Venture Capitalist (VC) investments trends has found that VC investment growth in construction technologies has outpaced VC investment growth in other industries by approximately 15-fold, with clear indications of continuing momentum (Bartlett et al., 2020).
Construction workers are the backbone of the construction industry, as they make up a sizable portion of overall production costs. Therefore, their performance and productivity is critical to the successful execution of construction projects (Okoro et al., 2017). An important issue facing the construction industry is the declining rate of worker productivity and performance (Shehata and El-Gohary, 2011). Because construction workers play a huge role in overall productivity, it is essential to understand the determinants of what impacts their performance. Factors involving tools and construction equipment, materials, and technologies were found to have a major impact on worker performance (Dai et al., 2009). Other determinants of worker performance and productivity include: increased level of supervision (Yates, 2006), communication and efficient information flow (Lingard et al., 2007), adequate financial compensation (Yates, 2006), proper work planning of construction tasks, materials, and methods (Gatti and Migliaccio, 2013), continuous work training and education, (Yates, 2006), and implementation of effective safety measures (Enshassi et al., 2009)
In addition to the importance of people on a construction project, information is considered the lifeblood of the modern construction industry (Nassereddine et al., 2022). Given the sheer volume of knowledge produced throughout the lifecycle of a construction project, the proper management of this information is critical due to the compartmentalized nature of the industry (Kazi and Koivuniemi, 2006; Dave and Koskela, 2009). Adequate information access has been found to add numerous benefits to the construction industry. Information access and information technologies enhance employee satisfaction, and improve external and internal collaboration and communication (Klinc et al., 2008). Information access and management improves work quality, simplifies complicated tasks, and increases worker productivity (Vasista and Abone, 2018). Within an organization, information management increases overall efficiency, and eases the process of disseminating necessary information to related projects, which in turn facilitates project completion on schedule per specification guidelines. Among team members, it allows the effective communication between workers and supervisors, and promotes collaboration, coordination and effective decision making (Prasanna and Raja Ramanna, 2014).
In the construction industry, while many attribute low productivity to craft workers, any problems are more likely to be caused by the lack of adequate supervision and failure of frontline supervisors to provide the necessary planning, information, support, and motivation (Howell, 1999). Frontline supervisors have a critical responsibility of ensuring safe and healthy work practices, and they create the missing communication link between management and the craft workforce (Uwakweh, 2005; Oswald and Lingard, 2019; Ramadan et al., 2022). Multiple studies have extensively highlighted the importance and major influence frontline supervisors have on construction productivity (Liberda et al., 2003; Hewage et al., 2011; Gerami Seresht and Fayek, 2019).
Meanwhile, the construction industry is plagued with numerous workforce challenges. The skilled craft labor shortage has been a consistent and unfortunate recurring struggle for the past 3 decades (Construction Industry Institute, 2016). Moreover, recent trends show that the construction workforce has been aging rapidly, aggravating the labor shortage challenge and negatively impacting project performance in terms of cost, schedule, and safety (Taylor et al., 2016). Additionally, the construction industry lacks workforce diversity, particularly gender diversity, where women make up only 4% of the construction craft workforce in the US (US Bureau of Labor Statistics, 2021; Ramadan et al., 2022). Furthermore, the industry has not been able to consistently attract and retain skilled workers due to industry image problems, insufficient training, and an aging workforce (Tucker et al., 1999).
To understand where the literature stands on the potential solutions and recommendations to address construction workforce challenges, the top 100 search results on this topic from “Google Scholar” were analyzed. While not all of these 100 academic papers contained relevant information, 23 academic papers were identified that included recommendations to mitigate the impact of workforce challenges. Some of these papers discussed single issue recommendations, while others discussed multiple recommendation. In total, these papers made 12 unique recommendations that were mentioned for 45 times across this sample of the literature. Figure 1 shows a word cloud of the main topics discussed in the literature to address workforce challenges in the construction industry.
Technology adoption and pursuing workforce diversity were the two leading topics discussed in the literature to minimize workforce challenges. Technology adoption was discussed 12 times while workforce diversity was mentioned 9 times. The overwhelming sentiment from the literature is that technology adoption can potentially increase productivity and workforce output While technology adoption is discussed in the literature as avenues to minimize workforce challenges, these academic studies do not attempt to study impact or potential benefits of technology adoption. For this reason, this research will focus on analyzing the impact of on-site technology use on construction workforce performance and information access.
Researchers have studied and discussed construction 4.0 technologies at length (Forcael et al., 2020; Ammar and Nassereddine, 2022), including the most recent developments in blockchain technologies (Li et al., 2019; Sadeghi et al., 2022a; Sadeghi et al., 2022b). In this study, based on a review of existing work on new technologies, and a workshop discussion among industry experts on technology use on construction sites, 13 technologies were identified for this research. The existing literature has highlighted the numerous benefits of such construction technologies. For instance, barcode scanning technology has been found to be one of the most effective methods of object recognition and information collection with high speed acquisition and low production cost (Yan et al., 2013). Augmented or Virtual reality allows for real time project visualization and data collection, improving spatial cognition, detection of design errors, enhancing productivity and quality, and improving communication and collaboration (Nassereddine, 2019; Nassereddine et al., 2020). Radio Frequency Identification (RFID) chip tracking facilitates the control of construction processes throughout the lifecycle of a project using the efficient tracking of construction materials, resources, equipment, components, and systems (Valero et al., 2015). Building Information Modeling (BIM) has been found to allow faster, more efficient, and more accurate planning and construction, increasing productivity as well as cost and time savings, and enabling simultaneous contribution to the system from all project stakeholders (Guo et al., 2017; Torres et al., 2018; Dadi et al., 2022). Artificial intelligence has helped contribute to the improvement of services and business operations, and improved automation processes of companies to give them a competitive advantage (Abioye et al., 2021). Drones have contributed to overall cost reduction and fewer project delays, improving the safety record, and provided the capability of generating high resolution aerial imagery used for more accurate data collection and surveying (McGuire et al., 2016; Hatoum and Nassereddine, 2022). Robotics and autonomous machinery use has facilitated construction processes, and has been found to considerably reduce costs and time spent on construction tasks, as well as improve the quality of executed product (Prasath Kumar et al., 2016; Hatoum and Nassereddine, 2020). Battery powered tools were shown to increase worker productivity and decrease workplace hazards and injuries. (Welch et al., 2015). Prefabrication/Modularization allows for faster on-site construction, reduced on-site work, improved quality, reduced energy and emissions, and reduced overall construction costs (Lopez and Froese, 2016). Finally, quick connection systems have been found to reduce work hours, decrease the number of necessary work packages, improve quality, and facilitate construction processes (Shan et al., 2012).
While the importance and benefits of technology use have been evaluated and highlighted in existing work, no research has yet studied how on-site technology use impacts the worker’s performance and ability to access needed information. This study attempts to bridge this gap of knowledge using a survey of construction workers that investigates the types of technologies used on site, the respondent’s performance record, and the difficulty of the information access process. The aim of this paper is to analyze and understand the impact of on-site technology use on 1) the self-evaluated personal performance record, and 2) the difficulty of the process to access information, of construction workers and frontline supervisors in the construction industry.
The objective of this paper is to analyze and understand the impact of on-site technology use on the worker’s performance (self-reported performance record including safety, attendance, quality, productivity, and initiative), and information access (the difficulty of the process to receive or get access to required information) for construction workers and frontline supervisors in the construction industry. To achieve the research objective, an online questionnaire was developed in “Qualtrics” and distributed to US construction workers. Among other questions, the survey asks construction workers about the types of technologies used on their construction sites, their performance, and how hard it is to gain access to information. The survey received 2,780 responses from all 50 states. Figure 2 details the geographic distribution of the responses. States contributing most to the survey were large population states including New York, California, Texas, Pennsylvania, and Illinois.
FIGURE 2. Geographic distribution of number of respondents across the states–generated by mapchart.net.
Overall, the responses have a gender breakdown of 94.8% male and 4.9% female. This breakdown is roughly representative of the US construction craft workforce, where, according to the bureau of labor statistics, females constituted 3.9% of the construction craft workforce in 2021 (US Bureau of Labor Statistics, 2021).
The breakdown of the respondents by age showed that 30% of them are over the age of 55, 25% are in the 45–54 age group, 25% are in the 35–44 age group, 17% are in the 25–34 age group, and 3% are under the age of 25. Of the survey respondents, 41% are craftspersons/journeymen, 14% are foremen, 13% are apprentices/helpers, 13% are superintendents, 7% are general foremen, 3% are project managers, and 9% indicated that they hold another title. By education, 42% of the respondents indicated that they have received some college education, 29% have a high school equivalent education or less, 16% have completed a technical program, 11% hold a bachelor’s degree or a graduate/post graduate degree, and 2% indicated that they have another type of education.
In this study, based on a review of existing work on new technologies, and a workshop discussion among industry experts on technology use on construction sites, 13 technologies were identified for this research. The survey asked the construction workforce to select all the identified information, material, and equipment technologies that they are using on their construction sites. The following is a list of technologies that can be selected.
• Information Technologies included the following: Barcode Scanning, Virtual or Augmented Reality (VR or AR), RFID Chip Tracking, Building Information Modeling (BIM), Artificial Intelligence, Robotics, and Drones. The respondents were allowed to list “Other” information technologies used on site
• Material Technologies included the following: Modularization/Prefabrication, and Quick Connection Systems. The respondents were allowed to list “Other” material technologies used on site
• Equipment Technologies included the following: Autonomous Machinery, New Hand Tools that were developed in the past 5 years, Battery Powered Tools, and New Types of Construction equipment that were developed in the past 5 years. The respondents were allowed to list “Other” equipment technologies used on site.
Finally, the survey asked construction workers the two following questions relating to their performance record and the level of difficulty to gain access to information.
• For the last year, please rate your personal performance record (including safety, attendance, quality, productivity, and initiative) on a scale from 0 to 10, 0 being “weak”, and 10 being “superior”.
• On a scale of 0–10, 0 being “instantaneous access”, and 10 being “very hard”, how hard is the process to receive or get access to required information?
A higher value on the response to the personal performance record indicates a more positive outcome, while a lower value on the response to how hard the process to receive or get access to required information indicates a more positive outcome.
The data collected through “Qualtrics” was downloaded in an Excel format. The dataset was secured and protected using a password to ensure the data is not manipulated or accessed by unauthorized personnel to ensure data integrity. A copy of the dataset was stored separately as a backup. The data was cleaned, sorted and analyzed using Python code. To assess the reliability and internal consistency of the survey data, a Cronbach’s alpha was calculated. The survey had a Cronbach’s alpha of 0.735 with a 95% confidence interval of (0.719,0.750), which is above the 0.7 acceptable threshold.
To compare 1) the personal performance record and 2) the difficulty of the process to receive or get access to information based on whether each technology is used on site, the data was grouped based on whether the technology is used on site or not. The corresponding average of the performance record and difficulty level of accessing information is calculated and compared for the two groups.
To test if the difference between two groups is statistically significant, the non-parametric Mann-Whitney U- test was performed to obtain the p-value. This test was used to err on the conservative side of the statistical analysis due to large discrepancies in sample sizes of two groups being compared (Gibbons and Chakraborti, 1991). The students’ t-test was avoided because of the large discrepancies in sample sizes. If sample sizes in both conditions are equal, the t-test is very robust against unequal variances. However, if sample sizes are unequal, unequal variances can influence the Type 1 error rate of the students’ t-test by either increasing or decreasing the Type 1 error rate from the nominal alpha significance level. In such cases, the Mann-Whitney U-test is better behaved and performs better than the t-test (Gibbons and Chakraborti, 1991). For this reason, the Mann-Whitney U-test is more appropriate in this statistical analysis. A significance level, α, of 0.1 is considered for statistical significance (i.e., 90% confidence level).
Moreover, the total number of technologies used on site for each respondent is calculated. The average personal performance record, and the average difficulty of the process to receive or get access to information is calculated for each total. The Kendall tau-b correlation coefficient was used to test if there is a relationship between the total number of technologies uses on site and 1) the average personal performance record and 2) the average difficulty of the process to receive or get access to information. The p-value of the Kendall tau-b correlation coefficient is obtained and compared to the significance level, α, of 0.1.
This analysis will be performed for 1) the survey population as a whole representing the overall construction workforce, 2) among frontline supervisors as one group, and 3) among craft workers as another group.
Finally, a k-means cluster analysis is performed to identify which technologies had the highest impact in improving the 1) personal performance record and 2) easing the process to receive or gain access to information for the overall construction workforce. Ranking the technologies according to their scores of “personal performance record” and the “difficulty of the process to receive or gain access to information” does not necessarily mean that the first score is statistically higher or lower than the second. Therefore, cluster analysis was used to organize the data into categories or levels. Cluster analysis is a statistical method used to group data by comparing each candidate technology to the other technologies already in the cluster. If the difference between the candidate technology and the other technology already in the cluster is significant, then the candidate technology is assigned to a different cluster.
Survey respondents were asked what kind of “Information”, “Material”, and “Equipment technologies they are using on site among the 2,602 survey participants who responded to these questions. Figures 3–5 show the current levels of different “Information”, “Material”, and “Equipment” technology use respectively on construction sites in the United States.
Among information technologies, as can be seen in Figure 3, Barcode Scanning and BIM have the highest use percentage on construction sites at 23% and 21% respectively. Drones are used on 9% of construction sites, Virtual or Augmented Reality is used on 7% of construction sites, Robotics are used on 6% of sites, and RFID Chip Tracking is used on only 5% of construction sites. Artificial Intelligence had the lowest use percentage at 2%. Still, 12% of the respondents indicated that they use other types of information technologies that were not among the listed technologies including GPS, tablets, laser alignment, and telematics, etc.
Among Material technologies, as can be seen in Figure 4, Prefabrication/Modularization is used on 42% of construction sites, while Quick Connection Systems are used on 17% of construction sites. Still, 8% of the respondents indicated that they use other types of material technologies that were not among the listed technologies including pre-cast concrete, composite materials, and advanced fastening systems, etc.
Among Equipment technologies, as can be seen in Figure 5, Battery Powered Tools have the highest use percentage on construction sites at 87%. New Hand Tools that were developed in the past 5 years are used on 22% of sites. New Types of Construction Machinery that were developed in the past 5 years are used on 11% of sites. Only 2% of respondents indicated that Autonomous Machinery is being used on their sites. Still, 3% of the respondents indicated that they use other types of equipment technologies that were not among the listed technologies including robotic total station, CNC, and edge-banding machines, etc.
Survey Respondents were asked to evaluate 1) their personal performance record and 2) the level of difficulty of the process to receive or get access to information on a scale of 0–10 each. This section details and compares 1) the average performance record and 2) the difficulty of information access among respondents who indicated that each specified technology is used on site, and respondents who indicated those technologies are not used. The p-value of the Mann-Whitney U- test is presented to examine if the differences in performance record and difficulty of information access are statistically significant. This analysis is performed three times: 1) the survey population as a whole representing the overall construction workforce, 2) among frontline supervisors as one group, and 3) among craft workers as another group.
The impact of technology use on 1) the personal performance record and 2) the difficulty to receive or get access to required information for the overall construction workforce, based on whether the respondents indicated a technology is used or not, is presented in Table 1 and Table 2. Each table shows the average response for the personal performance record and the average difficulty of information access respectively for each group. The tables present the sample size “N” for each group and the p-value of the Mann-Whitney U-test to examine whether any difference in the averages is statistically significant or not. This analysis was performed among 2,602 respondents representing the overall construction workforce.
TABLE 1. Average personal performance record of the overall construction workforce based on technology use on a scale of 0–10.
TABLE 2. Average difficulty of information access of the overall construction workforce based on technology use on a scale of 0–10.
Table 1 shows the average personal performance record of the overall construction workforce based on technology use on a scale of 0–10.
The results of Table 1 show that, on average, for the overall construction workforce, the use of 12 of the 13 technologies had a positive impact on the personal performance record of the respondents. Out of these 12 technologies, only nine had results that were statistically significant. The information technologies that had a statistically positive impact on performance include Barcode Scanning, Virtual or Augmented Reality, RFID Chip Tracking, and Building Information Modeling. Both material technologies, Prefabrication/Modularization and Quick Connection Systems had a statistically positive impact on performance. The equipment technologies that had a statistically positive impact on performance include New Hand Tools, and New Construction Machinery developed in the past 5 years. While the use of Artificial Intelligence indicated a decrease in the personal performance record, that result is not statistically significant.
Table 2 shows the average difficulty of information access of the overall construction workforce based on technology use on a scale of 0–10.
The results of Table 2 show that, on average, for the overall construction workforce, the use of 11 of the 13 listed technologies had a positive impact on the information access of the respondents. Out of these 11 technologies, only seven had results that were statistically significant. The information technologies that had a statistically positive impact on information access include Barcode Scanning, Virtual or Augmented Reality, RFID Chip Tracking, Building Information Modeling and Drones. Only Prefabrication/Modularization of the material technologies had a statistically positive impact on information access. Only Battery Powered tools of the equipment technologies had a statistically positive impact on information access. While the use of Quick Connection Systems, and New Construction Machinery indicated an increase in the difficulty to access information, only the impact of New Construction Machinery was statistically significant.
A correlation analysis was carried out to examine if there is any relationship between the total number of technologies and 1) the average personal performance record and 2) average difficulty of information access for the overall construction workforce. The results of the analysis show a strong positive Kendall tau-b correlation coefficient of 0.8181 between the number of technologies used on site and the average personal performance record of the respondents of the overall construction workforce. This means that an increase in the number of technologies used is likely to increase the respondent’s average personal performance record. The p-value of the Kendall tau-b is 0.00004 indicating that the correlation coefficient is statistically significant. Additionally, there is a decent negative Kendall tau-b correlation coefficient of −0.5151 between the number of technologies used on site and the average difficulty of information access of the respondents of the overall construction workforce. This means that an increase in the number of technologies used is likely to decrease the respondent’s average difficulty to access information. The p-value of the Kendall tau-b is 0.02098 indicating that the correlation coefficient is statistically significant.
The impact of technology use on 1) the personal performance record and 2) the difficulty to receive or get access to required information for the frontline supervisors of the construction workforce based on whether the respondents indicated a technology is used or not is presented in Table 3 and Table 4. Each table shows the average response for the personal performance record and the average difficulty of information access respectively for each group. The tables present the sample size “N” for each group, and the p-value of the Mann-Whitney U-test to examine whether any difference in the averages is statistically significant. This analysis was performed among 1,138 respondents representing the frontline supervisors of the construction workforce.
TABLE 3. Average personal performance record of frontline supervisors based on technology use on a scale of 0–10.
TABLE 4. Average difficulty of information access of frontline supervisors based on technology use on a scale of 0–10.
Table 3 shows the average personal performance record of the frontline supervisors of the construction workforce based on technology use on a scale of 0–10.
The results of Table 3 show that, on average, for the frontline supervisors of the construction workforce, the use of 12 of the 13 listed technologies had a positive impact on the personal performance record of the respondents. Out of these 12 technologies, only four had results that were statistically significant. The information technologies that had a statistically positive impact on performance include Barcode Scanning and Virtual or Augmented Reality. Only Quick Connection Systems of the material technologies had a statistically positive impact on performance, and only the New Hand Tools developed in the past 5 years of the equipment technologies had a statistically positive impact on performance. While the use of Artificial Intelligence indicated a decrease in the personal performance record, that result is not statistically significant.
Table 4 shows the average difficulty of information access of the frontline supervisors of the construction workforce based on technology use on a scale of 0–10.
The results of Table 4 show that, on average, for the frontline supervisors of the construction workforce, the use of nine of the 13 listed technologies had a positive impact on the information access of the respondents. Out of these nine technologies, only seven had results that were statistically significant. The information technologies that had a statistically positive impact on information access include Barcode Scanning, Virtual or Augmented Reality, RFID Chip Tracking, Building Information Modeling, and Drones. Both material technologies, Prefabrication/Modularization and Quick Connection Systems had a statistically positive impact on information access. Yet, none of the listed equipment technologies had a statistically positive impact on information access. While the use of four technologies indicated an increase in the difficulty to access information, only the impact of New Construction Machinery was statistically significant.
A correlation analysis was carried out to examine if there is any relationship between the total number of technologies and 1) the average personal performance record and 2) average difficulty of information access for the frontline supervisors of the construction workforce. The results of the analysis a strong positive Kendall tau-b correlation coefficient of 0.8182 between the number of technologies used on site and the average personal performance record of the respondents of frontline supervisors of the construction workforce. This means that an increase in the number of technologies used is likely to increase the respondent’s average personal performance record. The p-value of the Kendall tau-b is 0.00013 indicating that the correlation coefficient is statistically significant. Additionally, there is a small negative Kendall tau-b correlation coefficient of −0.2727 between the number of technologies used on site and the average difficulty of information access of the respondents of the overall construction workforce. This means that an increase in the number of technologies used is likely to decrease the respondent’s average difficulty to access information. However, the p-value of the Kendall tau-b is 0.28296 indicating that the correlation coefficient is not statistically significant.
The impact of technology use on the 1) personal performance record, and 2) the difficulty to receive or get access to required information for craft workers of the construction workforce, based on whether the respondents indicated a technology is used or not is presented in Table 5 and Table 6. Each table shows the average response for the personal performance record and the average difficulty of information access respectively for respondents of each group. The tables present the sample size “N” for each group, and the p-value of the Mann-Whitney U-test to examine whether any difference in the averages is statistically significant. This analysis was performed among 1,394 respondents representing the craft workforce of the construction workforce.
TABLE 5. Average personal performance record of craft workers based on technology use on a scale of 0–10.
TABLE 6. Average difficulty of information access of craft workers based on technology use on a scale of 0–10.
Table 5 shows the average personal performance record of the craft workers of the construction workforce based on technology use on a scale of 0–10.
The results of Table 5 show that, on average, for the craft workers of the construction workforce, the use of nine of the 13 listed technologies had a positive impact on the personal performance record of the respondents. Yet, only three of these nine technologies had results that were statistically significant. The information technologies that had a statistically positive impact on performance include Virtual or Augmented Reality and RFID Chip Tracking. Only Quick Connection Systems of the material technologies had a statistically positive impact on performance, and none the equipment technologies had a statistically positive impact on performance. While the use of four technologies indicated on average, a decrease in the personal performance record, only Robotics had a statistically significant impact.
Table 6 shows the average difficulty of information access for the craft workers of the construction workforce based on technology use on a scale of 0–10.
The results of Table 6 show that, on average, for the craft workers of the construction workforce, only four of all the listed technologies had a positive impact on the information access of the respondents. However, out of these four, only Battery Powered Tool, had a statistically positive impact on information access. Among the other nine technologies that had a negative impact on information access, only the results of the Quick Connection Systems was statistically significant.
A correlation analysis was carried out to examine if there is any relationship between the total number of technologies and 1) the average personal performance record and 2) average difficulty of information access for the craft workers of the construction workforce. The results of the analysis show a strong positive Kendall tau-b correlation coefficient of 0.7078 between the number of technologies used on site and the average personal performance record of the respondents of the craft workers of the construction workforce. This means that an increase in the number of technologies used is likely to increase the respondent’s average personal performance record. The p-value of the Kendall tau-b is 0.00152 indicating that the correlation coefficient is statistically significant. Additionally, there is a decent negative Kendall tau-b correlation coefficient of −0.4848 between the number of technologies used on site and the average difficulty of information access of the respondents of the overall construction workforce. This means that an increase in the number of technologies used is likely to decrease the respondent’s average difficulty to access information. The p-value of the Kendall tau-b is 0.02098 indicating that the correlation coefficient is statistically significant.
The average personal performance record and average difficulty of information access when each specified technology is used is calculated, sorted and ranked in descending order of positivity. The k-means clustering analysis allows the finding of the number of clusters that give the most parsimonious balance between minimizing the number of clusters and the minimum sum of square errors within each cluster. To determine the number of clusters, the “elbow” method was used (Hothorn and Everitt, 2006) as can be seen in the scree plot of Figure 6. For instance, the scree plot indicates that the number of clusters technologies based on the difficulty of information access is 3, after which the decrease in the sum of squares is roughly constant.
FIGURE 6. Scree plot for the k-means cluster analysis of the 13 technologies and the difficulty of information access.
The k-means cluster analysis indicated the number of technology clusters is three for each of the personal performance record and the level of difficulty of information access. The plot in Figure 7 of the average personal performance record (left), and the average level of difficulty of information access (right) of the 13 technologies, also visually indicates the data of each is clustered into three groups.
FIGURE 7. Plot of technologies against the personal performance record (left) and difficulty to access information (right) showing three technology clusters each.
The results of the k-means cluster analysis and the visual representation of the plot of the technologies for the personal performance record in Figure 7 both identify the three technology clusters. Cluster one had three technologies that showed great potential for personal performance including Virtual or Augmented Reality, RFID Chip Tracking, and Drones. Cluster two included nine technologies that had some potential for personal performance, while cluster 3, which only included Artificial Intelligence, showed limited potential for personal performance.
The results of the k-means cluster analysis and the visual representation of the plot of the technologies for the information access in Figure 7 both identify the three technology clusters. Cluster one had four technologies that showed great potential for information access including BIM, Drones, Virtual or Augmented Reality, and RFID Chip Tracking. Cluster two had eight technologies that showed some potential for information access including Barcode Scanning, Artificial Intelligence, Prefabrication/Modularization, Battery Powered Tools, New Hand Tools, Quick Connection Systems, Robotics, and Autonomous Machinery, while cluster 3, which showed limited potential for information access only included New Types of Construction Machinery that was developed in the last 5 years.
Having a better understanding of the impact of different technologies on worker performance, and ability to access information, is critical to helping construction industry leaders identify avenues that improve construction productivity as we face the challenges of a decades long labor shortage in the industry. This study presents an in-depth statistical analysis of the impact the use of 13 technologies has on the construction workforce of the United States. Using the data collected from frontline supervisors and craft professionals, respondents identified different kinds of technologies being used, and then self-evaluated their own personal performance record, and the difficulty of the process to receive or get access to needed information. Worker performance and the ability to access information was compared among those who indicated specific technologies are used on their construction sites, and those indicated that it is not used. The results of this analysis for the overall construction workforce clearly show that technology use is overwhelming beneficial for worker performance, where 12 of the 13 of the technologies, on average, caused an increase in performance, nine of which had a statistically significant positive impact, including five information technologies, both material technologies, and two equipment technologies. Moreover, 11 of the 13 technologies, on average, eased the process of information access, seven of which had a statistically significant positive impact, including five information technologies, one material technology, and one equipment technology.
This analysis was broken down among frontline supervisors and craft workers. For frontline supervisors, 12 of the 13 technologies resulted in an increase in performance. Yet, only four of those had a statistically significant positive impact, including two information technologies, one material technology, and one equipment technology. However, frontline supervisors mostly benefited from the use of technologies, specifically, information technologies, in the area of information access, where nine of the 13 technologies eased the process to receive or get access to information, seven of which had a statistically significant positive impact, including five information technologies, both material technologies, and none of the equipment technologies.
As for craft workers, nine of the 13 technologies resulted in an increase in performance. However, only three of those had a statistically significant positive impact, including two information technologies, one material technology, and none of the equipment technologies. On the other hand, craft workers did not greatly benefit from the use of technologies to ease the process of information access. Specifically, only four technologies, on average, eased the process to receive or get access to information, but only one had a statistically significant positive impact. Only two of the information technologies, on average, had a slightly positive impact on easing the process of information access, but none were statistically significant. None of the information technologies that indicated a negative impact were statistically significant either. All of the information technologies listed are sophisticated and advanced technologies that require training and expertise to operate. Potentially, the lack of training and familiarity with such systems that are not widely adopted across the industry may have hampered the realization of any benefits among craft workers. Also, information captured by such technologies are usually handled and operated at a higher level than craft workers (foremen, general foremen, superintendents, etc.), and therefore the benefit of such systems was realized at the level of frontline supervisors as the previous section indicated, where five of the seven listed information technologies yielded a statistically significant positive impact on the process of information access.
Moreover, the analysis clearly shows that there is a statistically significant strong correlation between the total number of technologies implemented and used on site and the average respondent’s personal performance record. This means that on average, the personal performance record improves as the number of technologies used increases. This is true for the overall construction workforce, frontline supervisors, and craft workers. On the other hand, there is a decent negative correlation between the total number of technologies implemented and used on site and the average difficulty to receive or get access to required information. This means that on average, the difficulty of information access decreases as the number of technologies used increases. This correlation is statistically significant for the overall construction workforce and the craft workers, but not for frontline supervisors. While the previous analysis showed that the use of specific technologies does not greatly ease the process of information access among craft workers, the use of multiple or an increasing number of technologies does. Industry leaders aiming to enhance worker performance and ease information access should consider implementing an increasing number of technologies to gain the desired benefits.
A cluster analysis of technologies identified three technologies with great potential for a strong performance record (cluster 1), eight technologies for some potential for performance (cluster 2) and one technology with limited potential for performance (cluster 3). As for information access, the cluster analysis identified four information technologies that had a great potential for decreasing the difficulty of information access (cluster 1), seven technologies for some potential for decreasing the difficulty of information access (cluster 2), and one technology with limited potential for decreasing the difficulty of information access (cluster 3). Of note, Virtual or Augmented Reality, RFID Chip Tracking, and Drones, are three technologies that are common in cluster one for performance and cluster one for information access. These three technologies are likely to have a huge positive impact for worker performance and information access on a project site. Leaders in the construction industry should consider implementing these technologies before others to maximize wanted benefits if the investment resources are limited.
Over the past decade, technological innovations have played a key role in the growth and advancement of an increasingly diverse construction industry. While, research has extensively studied the use of technology, little existing work has studied its direct impact on worker performance. The objective of this paper is to analyze and understand the impact of on-site technology use on the worker’s self-evaluated personal performance record, and the difficulty of the process to receive or get access to information in the construction industry. Using data from a survey of 2,780 construction workers, statistical analysis showed there are substantial benefits to the use and implementation of several technologies. This study found that on average, for the overall construction workforce, nine of the 13 technologies had a statistically positive impact on worker performance, and seven of the 13 technologies had a statistically positive impact on the process to receive or get access to needed information. Furthermore, increasing the number of technologies used is statistically correlated with improving the average performance and decreasing the difficulty of information access.
While this study presents a robust statistical analysis of the impact of technology use on personal performance and information access, the study does have certain limitations. The survey of construction workers does not ask any multiple-choice questions or open-ended questions that discuss specific benefits, challenges, or perceptions of the used technologies. Therefore, while this analysis can empirically measure which technologies have a positive impact on performance and information access, the study can not answer the questions related to why certain technologies yield improvements and others do not. Future research can build on this study and attempt to answer these specific questions, to build a roadmap for the leaders of the construction industry to identify critical technologies that will maximize their benefits based on their specific construction needs.
The raw data supporting the conclusions of this article will be made available by the authors, without undue reservation.
All listed authors have made a substantial, direct, and intellectual contribution to the content of this paper.
The authors declare that part of this study received funding from Construction Industry Institute (CII) as part of research project RT-370. Timothy R.B. Taylor a co-author in this paper, was the principal investigator of RT-370. The funder was not involved in the study design, collection, analysis, interpretation of data, the writing of this article or the decision to submit it for publication.
The authors gratefully acknowledge the Construction Industry Institute (CII) for their valuable support and partial funding for the data collection of this research project, and the college of engineering at the University of Kentucky for continuous support. The authors would also like to thank all the survey participants, without whom this research would not be possible. Any opinions, findings, conclusions and recommendations expressed by the authors in this paper do not necessarily reflect the views of the University of Kentucky or the Construction Industry Institute.
The authors declare that the research was conducted in the absence of any commercial or financial relationships that could be construed as a potential conflict of interest.
All claims expressed in this article are solely those of the authors and do not necessarily represent those of their affiliated organizations, or those of the publisher, the editors and the reviewers. Any product that may be evaluated in this article, or claim that may be made by its manufacturer, is not guaranteed or endorsed by the publisher.
Abioye, S. O., Oyedele, L. O., Akanbi, L., Ajayi, A., Davila Delgado, J. M., Bilal, M., et al. (2021). Artificial intelligence in the construction industry: A review of present status, opportunities and future challenges. J. Build. Eng. 44, 103299. doi:10.1016/j.jobe.2021.103299
Ammar, A., and Nassereddine, H. (2022). Blueprint for construction 4.0 technologies: A bibliometric analysis. IOP Conf. Ser. Mater. Sci. Eng. 1218 (1), 012011. doi:10.1088/1757-899X/1218/1/012011
Bartlett, K., Blanco, J. L., Fitzgerald, B., Johnson, J., and Ribeirinho, M. J. (2020). Rise of the platform era: The next chapter in construction technology. McKinsey and Company. Avaliable At: https://www.mckinsey.com/industries/private-equity-and-principal-investors/our-insights/rise-of-the-platform-era-the-next-chapter-in-construction-technology.
Construction Industry Institute (2016). Is There a demographic labor Cliff that will affect project performance (RT-318-11). Construction Industry Institute.
Construction Industry Institute (2021). Workforce 2030: What you Need to know now about your future workforce (final report FR-370). Construction Industry Institute.
Dadi, G. B., Nassereddine, H., Taylor, T. R., Griffith, R., and Ramadan, B. (2022). Technological capabilities of departments of transportation for digital project management and delivery. NCHRP Synthesis Report.
Dai, J., Goodrum, P. M., and Maloney, W. F. (2009). Construction craft workers’ perceptions of the factors affecting their productivity. J. Constr. Eng. Manag. 135 (3), 217–226. doi:10.1061/(asce)0733-9364(2009)135:3(217)(2009)135:3(217)
Dave, B., and Koskela, L. (2009). Collaborative knowledge management—a construction case study. Automation Constr. 18 (7), 894–902. doi:10.1016/j.autcon.2009.03.015
Enshassi, A., Mohamed, S., and Abushaban, S. (2009). Factors affecting the performance of construction projects in the gaza strip. J. Civ. Eng. Manag. 15 (3), 269–280. doi:10.3846/1392-3730.2009.15.269-280
Forcael, E., Ferrari, I., Opazo-Vega, A., and Pulido-Arcas, J. A. (2020). Construction 4.0: A literature review. Sustainability 12 (22), 9755. doi:10.3390/su12229755
Gatti, U. C., and Migliaccio, G. C. (2013). “A study on the influence of construction workers’ physiological status and jobsite environment on behaviour and performance,” in 49th ASC annual international conference proceedings (Associated Schools of Construction), 10–13.
Gerami Seresht, N., and Fayek, A. R. (2019). Factors influencing multifactor productivity of equipment-intensive activities. Int. J. Prod. Perform. Manag. 69 (9), 2021–2045. doi:10.1108/IJPPM-07-2018-0250
Gibbons, J. D., and Chakraborti, S. (1991). Comparisons of the mann-whitney, student’s t, and alternate t tests for means of normal distributions. J. Exp. Educ. 59 (3), 258–267. doi:10.1080/00220973.1991.10806565
Guo, F., Jahren, C. T., Turkan, Y., and David Jeong, H. (2017). Civil integrated management: An emerging paradigm for civil infrastructure project delivery and management. J. Manag. Eng. 33 (2), 04016044. doi:10.1061/(ASCE)ME.1943-5479.0000491
Hatoum, M. B., and Nassereddine, H. (2020). “Developing a framework for the implementation of robotics in construction enterprises,” in EG-ICE 2020 workshop on intelligent computing in engineering (Berlin, Germany: EGICE), 10.
Hatoum, M. B., and Nassereddine, H. (2022). “The use of Drones in the construction industry: Applications and implementation,” in 39th international symposium on automation and robotics in construction (ISARC). doi:10.22260/ISARC2022/0077
Hewage, K. N., Gannoruwa, A., and Ruwanpura, J. Y. (2011). Current status of factors leading to team performance of on-site construction professionals in Alberta building construction projects. Can. J. Civ. Eng. 38 (6), 679–689. doi:10.1139/l11-038
Hothorn, T., and Everitt, B. S. (2006). A handbook of statistical analyses using R. Chapman and Hall/CRC.
Howell, G. A. (1999). What is lean construction? IGLC7. Berkeley, CA, USA: University of California.
Kazi, A. S., and Koivuniemi, A. (2006). “Sharing through social interaction: The case of YIT construction ltd,” in Real-life knowledge management: Lessons from the field (Elsevier), 63–80.
Klinc, R., Dolenc, M., and Turk, Ž. (2008). “Possible benefits of WEB 2.0 to construction industry,” in CIB W78 2008 international conference on information technology in construction (Santiago, Chile: Participation).
Li, J., Greenwood, D., and Kassem, M. (2019). Blockchain in the built environment and construction industry: A systematic review, conceptual models and practical use cases. Automation Constr. 102, 288–307. doi:10.1016/j.autcon.2019.02.005
Liberda, M., Ruwanpura, J., and Jergeas, G. (2003). “Construction productivity improvement: A study of human, management and external issues,” in Construction research congress (ASCE). doi:10.1061/40671(2003)5
Lingard, H., Brown, K., Bradley, L., Bailey, C., and Townsend, K. (2007). Improving employees’ work-life balance in the construction industry: Project alliance case study. J. Constr. Eng. Manag. 133133 (10), 80710–80815. 807. doi:10.1061/(asce)0733-9364(2007)133:10(807)
Lopez, D., and Froese, T. M. (2016). Analysis of costs and benefits of panelized and modular prefabricated homes. Procedia Eng. 145, 1291–1297. doi:10.1016/j.proeng.2016.04.166
McGuire, M., Rys, M. J., and Rys, A. (2016). A study of how unmanned aircraft systems can support the Kansas Department of Transportation’s efforts to improve efficiency, safety, and cost reduction. Report.
Nassereddine, H. (2019). Design, development and validation of an augmented reality-enabled production strategy process for the construction industry. The University of Wisconsin-Madison.
Nassereddine, H., Bou Hatoum, M., Urban, H., and Harald, U. (2020). “A comprehensive map for integrating augmented reality during the construction phase,” in Proceedings of the creative construction E-conference (Budapest: University of Technology and Economics), 56–64. doi:10.3311/CCC2020-069
Nassereddine, H., Hanna, A. S., Veeramani, D., and Lotfallah, W. (2022). Augmented reality in the construction industry: Use-cases, benefits, obstacles, and future trends. Front. Built Environ. 8, 730094. doi:10.3389/fbuil.2022.730094
Okoro, C. S., Musonda, I., and Agumba, J. (2017). Identifying determinants of construction worker performance on construction sites: A literature review. Int. J. Innovation, Manag. Technol. 8, 60–63. doi:10.18178/ijimt.2017.8.1.703
Oswald, D., and Lingard, H. (2019). Development of a frontline H&S leadership maturity model in the construction industry. Saf. Sci. 118, 674–686. doi:10.1016/j.ssci.2019.06.005
Prasanna, S. V. S. N. D. L., and Raja Ramanna, T. (2014). Application of ICT benefits for building project management using ISM Model. Int. J. Res. Eng. Technol. 3 (6), 68–78.
Prasath Kumar, V. R., Balasubramanian, M., and Jagadish Raj, S. (2016). Robotics in construction industry. Indian J. Sci. Technol. 9 (23), 95974. doi:10.17485/ijst/2016/v9i23/95974
Ramadan, B. A., Taylor, T. R. B., Real, K. J., and Goodrum, P. (2022a). The construction industry from the perspective of the worker’s social experience. Constr. Res. Congr. 2022, 611–621. doi:10.1061/9780784483985.062
Ramadan, B., Nassereddine, H., Taylor, T. R. B., Real, K., and Goodrum, P. (2022b). “Impact of skill proficiencies on frontline supervision practices in the construction industry,” in Proceedings of the creative construction E-conference (Creative Construction Conference). doi:10.3311/CCC2022-024
Renz, A., Solas, M. Z., Buhler, M., Gerbert, P., Castagnino, S., and Rothballer, C. (2016). Shaping the future of construction. A breakthrough in mindset and technology. World Economic Forum.
Ribeirinho, M. J., Mischke, J., Strube, G., Sjödin, E., Blanco, J. L., Palter, R., et al. (2020). The Next Normal in Construction: How disruption is reshaping the world’s largest ecosystem. McKinsey and Company.
Sadeghi, M., Mahmoudi, A., and Deng, X. (2022a). Adopting distributed ledger technology for the sustainable construction industry: Evaluating the barriers using Ordinal Priority Approach. Environ. Sci. Pollut. Res. 29 (7), 10495–10520. doi:10.1007/s11356-021-16376-y
Sadeghi, M., Mahmoudi, A., Deng, X., and Luo, X. (2022b). Prioritizing requirements for implementing blockchain technology in construction supply chain based on circular economy: Fuzzy Ordinal Priority Approach. Int. J. Environ. Sci. Technol. 2022, 1–22. doi:10.1007/s13762-022-04298-2
Shan, Y., Goodrum, P., Haas, C., and Caldas, C. (2012). “Assessing productivity improvement of quick connection systems in the steel construction industry using building information modeling (BIM),” in Construction research congress 2012 (ASCE), 1135–1144. doi:10.1061/9780784412329.114
Shehata, M. E., and El-Gohary, K. M. (2011). Towards improving construction labor productivity and projects’ performance. Alexandria Eng. J. 50 (4), 321–330. doi:10.1016/j.aej.2012.02.001
Taylor, T., Karimi, H., Goodrum, P., and Albattah, M. (2016). Is There a demographic labor Cliff that will affect project performance (RT-318-1). Construction Industry Institute.
Torres, H. N., Ruiz, J. M., Chang, G. K., Anderson, J. L., and Garber, S. I. (2018). Automation in highway construction part I: Implementation challenges at state transportation departments and success stories (FHWA-HRT-16-030). United States: Federal Highway Administration Office of Infrastructure.
Tucker, R. L., Haas, C. T., Glover, R. W., Alemany, C., Carley, L. A., Rodriguez, A. M., et al. (1999). Key workforce challenges Facing the American construction industry an interim assessment (report No. 3). Center for Construction Industry Studies.
US Bureau of Labor Statistics (2021). Employed persons by detailed occupation, sex, race, and hispanic or latino ethnicity. Avaliable At: https://www.bls.gov/cps/cpsaat11.htm.
Uwakweh, B. O. (2005). Effect of foremen on construction apprentice. J. Constr. Eng. Manag. 131 (12), 1320–1327. doi:10.1061/(asce)0733-9364(2005)131:12(1320)(2005)131:12(1320)
Valero, E., Adán, A., and Cerrada, C. (2015). Evolution of RFID applications in construction: A literature review. Sensors 15 (7), 15988–16008. doi:10.3390/s150715988
Vasista, T. G., and Abone, A. (2018). Benefits, barriers and applications of information communication technology in construction industry: A contemporary study. Int. J. Eng. Technol. 7 (327), 492–499. doi:10.14419/ijet.v7i3.27.18004
Welch, L. S., Russell, D., Weinstock, D., and Betit, E. (2015). Best practices for health and safety technology transfer in construction: Practices for Health and Safety Technology. Am. J. Industrial Med. 58 (8), 849–857. doi:10.1002/ajim.22456
Yan, Y., Li, Q., Cao, M., Chen, H., and Xue, J. (2013). “Application research of two-dimensional barcode in information construction of colleges,” in Proceedings of the 2012 international conference on information technology and software engineering (ICIT), 71–80.
Keywords: construction, technology, performance, information access, impact
Citation: Ramadan B, Nassereddine H, Taylor TRB and Goodrum P (2023) Impact of technology use on workforce performance and information access in the construction industry. Front. Built Environ. 9:1079203. doi: 10.3389/fbuil.2023.1079203
Received: 25 October 2022; Accepted: 17 February 2023;
Published: 27 February 2023.
Edited by:
Timothy O. Olawumi, Edinburgh Napier Univesrsity, United KingdomReviewed by:
Jamal Younes Omran, Tishreen University, SyriaCopyright © 2023 Ramadan, Nassereddine, Taylor and Goodrum. This is an open-access article distributed under the terms of the Creative Commons Attribution License (CC BY). The use, distribution or reproduction in other forums is permitted, provided the original author(s) and the copyright owner(s) are credited and that the original publication in this journal is cited, in accordance with accepted academic practice. No use, distribution or reproduction is permitted which does not comply with these terms.
*Correspondence: Bassam Ramadan, YmFyYTIzNUB1a3kuZWR1
Disclaimer: All claims expressed in this article are solely those of the authors and do not necessarily represent those of their affiliated organizations, or those of the publisher, the editors and the reviewers. Any product that may be evaluated in this article or claim that may be made by its manufacturer is not guaranteed or endorsed by the publisher.
Research integrity at Frontiers
Learn more about the work of our research integrity team to safeguard the quality of each article we publish.