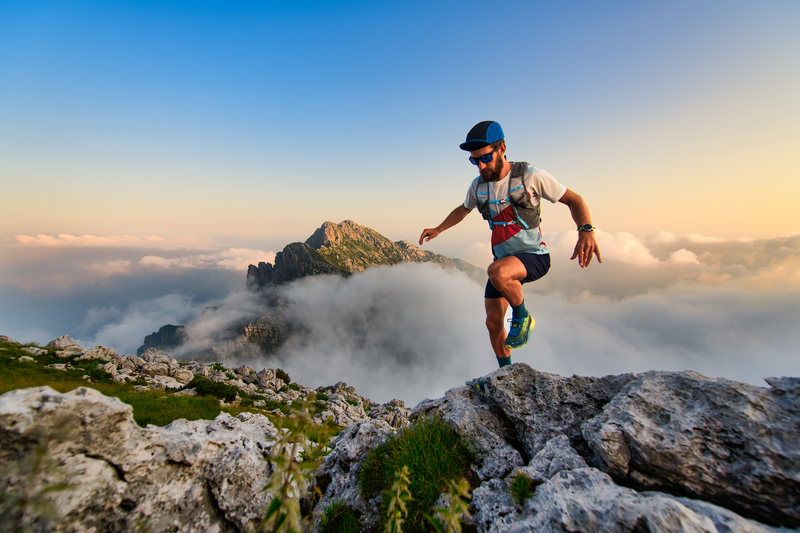
94% of researchers rate our articles as excellent or good
Learn more about the work of our research integrity team to safeguard the quality of each article we publish.
Find out more
ORIGINAL RESEARCH article
Front. Built Environ. , 08 July 2022
Sec. Coastal and Offshore Engineering
Volume 8 - 2022 | https://doi.org/10.3389/fbuil.2022.816020
Wave-induced pressure gradients and local accelerations are important interconnected physical mechanisms involving several hydrodynamic and morphodynamic coastal phenomena. Therefore, to provide a reliable and realistic hydrodynamic and morphodynamic simulation, the dependencies among different parameters, such as water level, pressure gradient, local acceleration, and sediment concentration, should be considered. Herein, a copula-based simulation is presented for modeling multivariate parameters and maintaining their statistical characteristics within the surf zone. Archimedean and elliptical copula families are applied to investigate the dependency construction between the parameters in two case studies: one from a field site on the east coast of Japan, and another from a large-scale laboratory barred beach profile. The dependency between variables is evaluated using Kendall’s τ correlation coefficient. The water level, pressure gradient, and local acceleration are shown to be significantly correlated. The correlation coefficients between the variables for the natural beach are lower than the laboratory data. The marginal probabilistic distribution functions and their joint probabilities are estimated to simulate the variables using a copula approach. The performance of the simulations is evaluated via the goodness-of-fit test. The analysis shows that the laboratory data are comparable to the field measurements, implying that the laboratory simulation results can be applied universally to model multivariable joint distributions with similar hydrodynamic conditions.
Surf zone sandbars are ephemeral nearshore bathymetric features that play a fundamental role in beach profile morphology (Ribas et al., 2012; Tabasi et al., 2017; Tabasi et al., 2018; Suzuki et al., 2018; Bryan et al., 2019). Surf zone sandbars enhance depth-induced wave breaking and subsequently initiate complex fluid motions generated by breaking waves in the surf zone. As a result of these fluid motions and the resulting sediment suspension and transport, the morphology of the nearshore can change rapidly. Because the morphological response of surf zone sandbars is governed by nearshore hydrodynamics, the prediction of surf zone sandbar morphological evolution is associated with the understanding of nearshore waves and current characteristics.
Field sites have been established along many coastlines to measure coastal hydrodynamic and morphodynamic characteristics, depending on the available budget and data necessity. Therefore, instruments for recording data may be deployed for a short or long duration. In some critical locations, for gathering data over seasons or years, instruments are installed permanently with the requirement for regular maintenance. However, owing to time and financial limitations, long-term data recording is not practical. Moreover, the gathered datasets may include missing data either due to the maintenance procedure of instruments, intentional pausing of recording to maintain the safety of instruments during coastal disasters or extreme events, and failure of instruments. Because of the abovementioned issues and coastal regions where no captured data are available, it is reasonable to generate data using different methods. The generated data should be modified and validated using the data recorded from a nearby location.
Generating data using different methods is important in turbulent environments, such as the surf zone, where not only do hydrodynamic parameters change abruptly, but also morphological components such as the sandbar shape change rapidly. Therefore, an improved prediction of hydrodynamic and morphodynamic parameters is closely associated with the understanding of several interconnected mechanisms, such as acceleration, velocity skewness, and pressure gradient, to obtain accurate surf zone sand bar shapes. Although several morphodynamic models have been proposed (e.g., Roelvink et al., 2010; Kuriyama, 2012; Tabasi et al., 2020), a comprehensive model that includes all interconnected mechanisms applicable to various coastal areas has not yet been developed. Researchers are attempting to include and evaluate several interconnected parameters in morphodynamic models. Suzuki et al. (2009) and Anderson et al. (2017) have shown that pressure gradients within sediment layers driven by waves and currents resulted in surf zone sandbar migrations. Subsequently, they investigated the pore water pressure gradient and current acceleration based on the wave height.
Various methods can be used to simulate water level, pressure gradients and accelerations. Fundamentally, the flow properties can be determined by solving the Navier-Stokes equations. However, due to turbulence terms in these equations, the exact solution of these equations is still incomplete except for very small control volumes. Nevertheless, these equations can be simplified by neglecting the term describing viscous motions of the flow to yield the Euler equations. Using intensive numerical models, pressure gradients and accelerations can be obtained using Euler’s inviscid momentum equations for a short duration. For solving these equations, the volume of fluid should be discretized or divided into several cells which is known as mesh. Open-source software (e.g., OpenFOAM, DualSPHysics) is readily available to obtain water level, pressure gradients, and accelerations via momentum equations (Brown et al., 2016; Lowe et al., 2019). However, depending on the required accuracy and the complexity of the flow, a more sophisticated discretization method with a high mesh resolution is required. Thus, the implementation of the numerical model will be more complicated. Because of the complicated model setup and code-compiling processes, the model computation time is often extremely long. Nevertheless, numerical simulations can be very useful approaches to predict and realize a wide variety of water environment processes.
Linear wave theory describes the propagation of waves or fluid surface changes assuming that the fluid is inviscid, incompressible, irrotational, and the surface of the fluid has a sinusoidal shape. However, the linear wave theory is valid for uniform small-amplitude waves and the linear wave model should be modified by including the effects of second-order nonlinearities such as Stokes drift, wave crest conservation, and mean mass conservation. Among the second-order wave approaches for solving the nonlinear waves, Stokes’s approach is very well-known. This approach proposes a solution for applying the boundary condition at the free surface position expanding the potential flow quantities in a Taylor series. Depending on the required accuracy, higher-order nonlinear wave approaches can be estimated accordingly. Valuable reviews about different wave theories and proposed models for the simulation of the water surface can be found in (Forristall, 2000; Tayfun and Fedele, 2007; Myrhaug et al., 2015).
Statistical methods are useful for simulating wave and wave-induced parameters such as wave heights, pressure gradients, and accelerations. For example, wave heights can be simulated using statistical-based wave spectra such as Neuman, JONSWAP, ITTC, and P-M spectrums. These spectra have been performed and evaluated in several coastal engineering studies such as Dawson et al. (1993); Ryabkova et al. (2019); Edwards et al. (2021). Similarly, Monte Carlo is a reliable simulation method (Bang Huseby et al., 2013; Clarindo et al., 2021) and can be used to account for the joint probability of correlated coastal parameters. Statistical multivariate regression models have been used to represent the relationships between dependent different parameters (Condon and Sheng, 2012). Although regression modeling is a very common method and almost all statistical packages are equipped with regression tools, the reliability of regression models generally decreases as the number of parameters increases.
Because of the complexities of coastal phenomena, the interdependency of coastal parameters should be considered in the calculations. In statistics, if the magnitude of one variable affects the magnitude of other variables, then the particular variable can be considered dependent. The dependency between two variables can be described using correlation coefficients. As mentioned above, owing to the importance of the dependency between variables, several methods have been proposed to model the bivariate joint probability of two variables. Plackett (1965) proposed a method for constructing a one-parameter bivariate distribution based on marginal functions. Vrijling and Bruinsma (1980) applied marginal distributions to model the significant wave height (
The copula approach, originally proposed by Sklar (1959), allows for the marginal distribution of dependent variables to be estimated separately based on a dependent structure, and numerous studies have been conducted to investigate the applicability to a range of problems. Nelsen (2006) described the construction of different copulas and their properties, whereas Schmidt (2006) provided a more concise guide into the theory of the copula approach. In coastal science and engineering, by De Waal and Van Gelder (2005) proposed the Burr–Pareto–logistic copula to model extreme significant wave heights and wave periods during severe storm events. De Michele et al. (2007) established a four-dimensional model for the analysis of significant wave height, storm duration, inter-arrival time, and wave direction. Salvadori et al. (2011) proposed multi-parameter multivariate extreme value (MEV) copulas to assess return periods and design qualities. Wahl et al. (2011) applied the Gumbel–Hougaard copula to analyze storm surge parameters. Subsequently, Wahl et al. (2012) extended the proposed bivariate statistical model to investigate the extreme significant wave height and peak sea level. Corbella and Stretch (2013) applied Archimedean and MEV copulas to simulate storm parameters such as peak wave period, storm duration, and inter-arrival time.
More recently, Li et al. (2014a) estimated coastal dune erosion along the Dutch coast via a statistical simulation of storm events developed by Li et al. (2014b), who employed a Gaussian copula to simulate the dependency relation of
The aim of this study is to provide a computationally efficient approach to model the joint probability and distribution for the height of the wave crest above the still-water level
The remainder of this paper is organized as follows: Section 2 describes the marginal distribution functions, the copula concept, and the description of the methodology for dependency construction using the copula approach. The methodology used in this study can be broadly categorized into two subsections, which are described in Sections 2 and 3. In Section 3, a summary of the experimental tests is presented prior to the results obtained from the laboratory tests, and the simulated and observed data are compared. Finally, the discussion and conclusions are presented in Sections 4 and 5, respectively.
The empirical cumulative distribution function (ECDF) in statistics represents the cumulative distribution probability of the measured data and is defined as follows:
where
Theoretical cumulative distribution functions (TCDFs, also known as marginal functions) have been proposed to fit the cumulative distribution probability based on their statistical characteristics. Extreme value (EV), normal, generalized extreme value (GEV), logistic, Nakagami, Rician, Weibull, inverse Gaussian, and gamma distributions were tested for fitting. Supplementary Table S1 shows the equations of the TCDFs and their parameters selected for this study. The parameters of the TCDFs can be varied over a wide range to fit the curve of TCDFs with the ECDF. To achieve a good estimation of the parameters, the maximum likelihood estimation (MLE) method was used. The goodness of fit (GOF) between the TCDFs and ECDFs was assessed using the two-sample Kolmogorov–Smirnov (KS) test. The KS test, expressed as
where
The theoretical foundation for the application of copulas is derived from Sklar’s theorem (Sklar, 1959). According to Sklar’s theorem, a copula function
where
where
Based on Sklar’s theorem, the joint density for marginal distributions with densities of
where
In addition, the conditional distribution function can be derived via the partial differentiation of the joint distribution functions, as follows:
Let
One of the advantages of the copula compared with the other joint distribution models is the freedom to select any TCDFs for the variables; this is because the copula approach creates a dependency structure between correlated variables by selecting the TCDFs independently. Various types of bivariate parametric copula families and classes have been proposed. Each of these families or classes exhibits various characteristics. Among them, Archimedean and elliptical families, which are frequently applied in coastal engineering, were selected for this study.
To construct a dependence structure among the water level, local acceleration, and pressure gradient, Archimedean copulas (including the Clayton, Frank, and Gumbel–Hougaard copulas) and elliptical copulas (including the Gaussian and Student’s t copulas) were considered in this study. In general, these copulas encompass a wide range of dependency and correlation patterns. However, the compatibility of these copulas with the dependence variables must be ensured via GOF tests. The theoretical proofs and mathematical justifications for the construction of these copulas are presented below.
In practice, Archimedean copulas are frequently applied in several fields of study (Kwon and Lall, 2016; Bacigál et al., 2019; Garcia-Jorcano and Benito, 2020). Because the family of Archimedean copulas comprises a wide range of possible dependency patterns and properties, they can be easily constructed (Nelsen, 2006). Generally, an Archimedean copula based on an algebraic method can be written as follows:
where
where
Archimedean copulas present different and special tail dependencies based on their generator functions. For example, the Gumbel copula presents upper tail dependence and is more appropriate for data with significant dependencies at higher values than at low values. By contrast, the Clayton copula presents a lower tail dependence and is suitable for data with significant low-value dependencies, whereas the Frank copula presents no tail dependence and is an appropriate model for data with weak dependencies.
The Gaussian and Student’s t copulas, as the most typically recommended members of the elliptical copulas, were applied to simulate the variables in this study. The main advantage of elliptical copulas is that they can easily generalize to a high number of dimensions. The elliptical copulas mentioned above are presented below.
The Gaussian or normal copula is a member of the elliptical copula family. This copula can easily simulate a high number of dimensions as it is an n-variate distribution over the unit cube
where
Finally, the mathematical formulation of the Gaussian copula can be written as follows:
The Student’s t copula represents the dependence structure of a multivariate Student’s t distribution. In fact, the Student’s t distribution is a generalization of the Gaussian distribution, and the Gaussian copula is a limited version of the Student’s t copula with a limited degree of freedom. Compared with the Gaussian copula, the Student’s t copula performs better in capturing the dependence between variables in a dataset.
If
where
To simulate dependent multivariate data using a copula, the following procedures were implemented. First, the linear correlation coefficient was calculated to specify the linear correlation between variables. In this regard, Kendall’s
For Archimedean and elliptical copulas, Kendall’s
Similar to Kendall’s
Here,
Second, the TCDF of each variable was estimated and fitted to their calculated ECDFs. Subsequently, the data were fitted to different copulas using the MLE method. Next, random values between 0 and 1 were generated from the fitted copulas. Finally, the generated values were inverted to achieve the original scale data using inverse functions.
The field data employed in this study were based on a field experiment conducted by Suzuki et al. (2009) during a 5-day experiment on February 16–20, 2007, at the Hasaki Oceanographical Research Station (HORS), a research facility operated by the Port and Airport Research Institute in Japan. The HORS is located in Ibaraki Prefecture, approximately 100 km east of Tokyo, and faces the Pacific Ocean (Supplementary Figure S1). The shoreline orientation was 31° anticlockwise from the north. A 427-m-long pier equipped with different instruments for various field measurements was located perpendicular to the Hasaki shoreline. The HORS is situated on a sandy coast composed of fine sand with a median sediment grain of approximately 0.18 mm, as reported by Katoh and Yanagishima (1995). The mean water level during the experiment was 0.651 m, based on the datum level at the HORS (Tokyo Peil: 0.687 m). Additionally, the low and high water levels were -0.196 and 1.252 m, respectively. Hence, the HORS had a mean tide range of 1.5 m during the experiment. The offshore wave data was recorded at a depth of 23.4 m off the Kashima port.
Supplementary Figure S2 shows the cross-shore beach profile on the first day of the measurements as well as the instrumentation setup. Three instrument sets were used in the experiment to measure the water level, velocity, water pressure, and sediment concentration. The water levels were recorded using a capacitance-type wave gauge (CHT5-200, KENEK). The velocities were recorded using three Nortek acoustic Doppler velocimeters (ADVs) positioned 10 cm above the bed. Pressure gradients were estimated using data recorded by an array of pressure transducers composed of five sensors (BPR-A-50KPS, KYOWA). Sediment concentrations were recorded using three optical backscatter sensors (OBS-3, D&A Instrument Comp.) Additional details regarding the field measurements and instrumentation can be obtained from the paper by Suzuki et al. (2009). It is noteworthy that seabed erosion occurred after installation; consequently, the seabed level around the array of instruments decreased. Therefore, to avoid the effect of erosion, only data measured on the first day were used in this study.
In this study, experimental tests were conducted by Mieras et al. (2017) and Anderson et al. (2017) in a large-scale wave flume at the O. H. Hinsdale Wave Research Laboratory at the Oregon State University (Supplementary Figure S3). The flume had a length of 104 m, a width of 3.7 m, and a depth of 4.6 m, with a fixed barred beach profile constructed based on the average observed beach profile on 11 October 1994 from Duck, NC, during the Duck94 field experiment (Faria et al., 1997; Scott et al., 2005) conducted at the U.S. Army Corps of Engineering Field Research Facility.
The fixed barred profile comprised impermeable slopes of concrete slabs to ensure repeatability, and morphological changes did not cause the position of wave breaking to vary during the experiment. A sand pit composed of two sections was installed on the sandbar crest. The main section had a width of 3.66 m with a depth of 0.18 m. To install the experimental instruments, a subsection with a length and width of 1.2 m and a total depth of 0.61 m was installed beneath the main sand pit. The sand pit was filled with sediments of two different sizes. Because most of the trials were conducted using sediments with
A series of approximately 10 monochromatic waves with three different wave periods, i.e.,
The tests involved measurements of water surface elevation, pore water pressure, instantaneous bed bathymetry, and velocity. To measure the water surface elevations from offshore to onshore, 11 resistance-type wave gauges were deployed. The wave gauges starting from the toe of the beach profile, i.e., 17.7 m from the wave maker, were mounted along the flume wall at intervals of 3.65 m. A wave gauge positioned at the seaward boundary of the sandbar crest was deployed to record the wave transformation over the sandbar. In addition, a pressure gauge was installed immediately above the sediment bed to measure the water depth over the sandbar crest.
An array of 7 GE Druck PDCR 81 pressure transducers composed of a horizontal row of five pressure transducers and a vertical row of two pressure transducers was embedded beneath the sediment to observe the pore pressure and pressure gradient (Supplementary Figure S3). The pore pressure transducers were spaced equally at 20 mm intervals. A conductivity concentration profiler with a width of 5.6 mm and a thickness of 5.6 mm was installed in the sediment pit to measure the instantaneous bed bathymetry at 8 Hz with 1 mm resolution. Moreover, near-bed velocities were measured using a vertical array of Vectrino I ADVs mounted approximately 0.1 m above the initial bed level. Additional details regarding the experimental setup, instrumentation, and test conditions can available in Anderson et al. (2017), Mieras et al. (2017), and Mieras et al. (2019).
This research focuses on the dependency structure among the
As described in Section 3.1, the water level, velocity, water pressure, and sediment concentration were measured as field data. The pressure gradients for each water level based on the central difference scheme were estimated as follows:
where
where
Wave-by-wave analysis was conducted to assess the measured parameter behaviors. Supplementary Figure S4A,C,E show the wave-induced pressure gradients, local accelerations, and bottom sediment concentration for different water levels, starting from the still water to maximum water levels for each wave. By considering the maximum water level as
Anderson et al. (2017) used a method similar to that of Suzuki et al. (2010) to calculate the pressure gradient in both the horizontal and vertical directions. The horizontal gradients can be estimated as follows:
where
where
To assess the correlation between variables, Kendall’s
The marginal distributions or TCDFs, as a first step for simulating the correlated variables using the copula approach, should be determined. Therefore, the empirical probabilities for (
Supplementary Figures S7, S8 show the ECDFs of the experimental data (blue asterisks) and TCDFs applicable to non-dimensional (
The GEV distribution as a TCDF with three parameters showed excellent agreement with the laboratory experimental data. Meanwhile, the EV and logistic distributions with two parameters indicated excellent trends for the ECDFs involving the laboratory data, unlike the case for the field dataset calculations. The p-values from the KS tests for the field variable statistical investigations indicated no significant superiorities among the GEV, EV, and logistic distributions because their p-values exceeded 90%. However, the GEV distribution required two empirical parameters, and compared with the EV and logistic distributions, the computational procedure was more complicated. Meanwhile, the low p-values for the Nakagami and Gamma distributions indicated that they did not fit well with the laboratory data compared with the GEV, EV, and logistic distributions. Based on the p-values, the normal, Rician, and Weibull distributions demonstrated relatively good agreement with the laboratory data. However, it was difficult to select the appropriate distribution among them based on the KS test because they demonstrated similar characteristics.
Supplementary Figure S9A shows a comparison between the marginal functions for field and laboratory data based on p-values. The results indicate that the range of p-values was wide. Therefore, the marginal functions yielded different performances based on the dataset. By contrast, similar fitting parameters were achieved for each coastal parameter regardless of the study location (Supplementary Figure S9B).
The Archimedean and elliptical copulas discussed in Sections 2.3.1 and 2.3.2, respectively, were employed to model the dependency between the variables. The joint probabilities for (
where
The calculated p-values imply that the field data can be analyzed using Archimedean and elliptical copulas. Among the Archimedean copulas, the Clayton and Frank copula generally performed better than the other member of the Archimedean family. Based on the results, it can be concluded that the Gumbel copula is not a good candidate for simulating the field data of (
Field Dataset
• (
• (
• (
Laboratory Dataset
• (
• (
• (
Researchers who investigated pore water pressure gradients such as Suzuki et al. (2010) indicated that the correlation among the water surface, pore pressure gradient, and horizontal velocity should be investigated in future studies. The results of this study show that the pressure gradient and current acceleration can be estimated using the wave height. Some morphodynamic models such as those developed by Madsen (1974) and Sleath (1999) demonstrated that the pressure gradient over the bar can be considered an important parameter for suspending sediments and can cause a momentary failure of the bed. The pressure gradients estimated in this study can be used for the calculation of the Sleath number which can be defined as
where
where
where
where
Supplementary Table S6 shows the hydrodynamic conditions and sheet flow thicknesses measured by Mieras et al. (2017). It was found that the range of values for
On the other hand, since there are several empirical equations proposed for the estimation of the Shields parameter, the results achieved by each equation should be different. Mieras et al. (2017) found a wide range for Shields parameter magnitudes using different proposed equations. That is due to the sensitivity of the Shields parameter equation to the estimation of the wave friction factor, fluid velocity amplitude and grain roughness. However, the correlation between the Sleath parameter and sheet flow thicknesses was assessed, in this study. A reasonable correlation between the Sleath parameter and the sheet flow thickness for the laboratory dataset was found. Supplementary Figure S14 show the result of simulated sheet flow thicknesses using the Sleath parameter by the copula method. Using wave heights and pressure gradients obtained in this study, it is possible to achieve a reasonable estimation for the wave-induced sheet flow thickness.
The effect of the pressure gradient on momentary bed failure was investigated by Anderson et al. (2017), who analyzed the initiation of erosion as well as the magnitude of erosion depth. The importance of the pressure gradient on momentary bed failure was revealed in numerical simulations (Cheng et al., 2017). Cheng et al. (2017) emphasized the importance of the horizontal pressure gradient for bed instability. Almost all of the beach profile morphodynamic models are including the Shields parameter without the involvement of the Sleath parameter (and therefore the pressure gradient) in the sediment transport modules. The accurate estimation of sediment erosion and sandbar formation is a sort of challenging issue for these models. Since the bed failure is a function of both Sleath and Shields parameters (Cheng et al., 2017). A more accurate simulated beach profile can be expected by using the Sleath parameter. Thus, the results generated by the copula method should be useful for the statistical-process based morphodynamic models.
Wave induced local acceleration has been employed in various sediment transport equations. The simulated data using the copula approach can be useful for all of the abovementioned purposes. In future studies, this approach can be extended to other coastal parameters. In addition, the effect of sediment size on copula-based simulations can be considered for the future studies.
In this study, a fully statistical model was used to investigate and simulate the interdependency of coastal parameters. Because the water level is one of the most important and well-known parameters for most coastal applications, this parameter and its effect on other coastal parameters should be considered. In addition, the effects of pressure gradient and acceleration on sediment transport mechanisms have been investigated extensively (e.g., Sleath, 1970; Madsen, 1978; Tabasi et al., 2021). To investigate the effects of different parameters on the sediment transport mechanism, Suzuki et al. (2009) and Mieras et al. (2017) conducted field measurements and large-scale experiments, respectively. The results highlighted the importance of the dependencies of
The copula approach was used to simulate the abovementioned parameters. To obtain a reliable simulation, a group of TCDFs was utilized to fit the variables. Multivariate dependency structures were constructed and fitted with Archimedean and elliptical copulas for (
The original contributions presented in the study are included in the article/Supplementary Material, further inquiries can be directed to the corresponding author.
MT: Simulation, data analysis and writing the manuscript. TS: Discussions of the data analysis and conduct the field experiments DC: Discussions of the data analysis and conduct the laboratory experiments.
The authors declare that the research was conducted in the absence of any commercial or financial relationships that could be construed as a potential conflict of interest.
All claims expressed in this article are solely those of the authors and do not necessarily represent those of their affiliated organizations, or those of the publisher, the editors and the reviewers. Any product that may be evaluated in this article, or claim that may be made by its manufacturer, is not guaranteed or endorsed by the publisher.
The Supplementary Material for this article can be found online at: https://www.frontiersin.org/articles/10.3389/fbuil.2022.816020/full#supplementary-material
Anderson, D., Cox, D., Mieras, R., Puleo, J. A., and Hsu, T. J. (2017). Observations of Wave‐induced Pore Pressure Gradients and Bed Level Response on a Surf Zone Sandbar. J. Geophys. Res. Oceans 122, 5169–5193. doi:10.1002/2016JC012557
Bacigál, T., Komorníková, M., and Komorník, J. (2019). State-of-the-art in Modeling Nonlinear Dependence Among Many Random Variables with Copulas and Application to Financial Indexes. Jamris 13, 84–91. doi:10.14313/JAMRIS/3-2019/31
Bang Huseby, A., Vanem, E., and Natvig, B. (2013). A New Approach to Environmental Contours for Ocean Engineering Applications Based on Direct Monte Carlo Simulations. Ocean. Eng. 60, 124–135. doi:10.1016/j.oceaneng.2012.12.034
Bitner-Gregersen, E. M., and Haver, S. (1989). “Joint Long Term Description of Environmental Parameters for Structural Response Calculation,” in 2nd International Workshop on Wave Hindcasting and Forecasting (Vancouver), August 11–16, 1991 (Edinburgh, The United Kingdom: ISOPE-I-91-031).
Brown, S. A., Greaves, D. M., Magar, V., and Conley, D. C. (2016). Evaluation of Turbulence Closure Models under Spilling and Plunging Breakers in the Surf Zone. Coast. Eng. 114, 177–193. doi:10.1016/j.coastaleng.2016.04.002
Bryan, K. R., Davies-Campbell, J., Hume, T. M., and Gallop, S. L. (2019). The Influence of Sand Bar Morphology on Surfing Amenity at New Zealand Beach Breaks. J. Coast. Res. 87, 44. doi:10.2112/SI87-005.1
Cheng, Z., Hsu, T.-J., and Calantoni, J. (2017). SedFoam: A Multi-Dimensional Eulerian Two-phase Model for Sediment Transport and its Application to Momentary Bed Failure. Coast. Eng. 119, 32–50. doi:10.1016/j.coastaleng.2016.08.007
Clarindo, G., Teixeira, A. P., and Guedes Soares, C. (2021). Environmental Wave Contours by Inverse FORM and Monte Carlo Simulation with Variance Reduction Techniques. Ocean. Eng. 228, 108916. doi:10.1016/j.oceaneng.2021.108916
Condon, A. J., and Sheng, Y. P. (2012). Optimal Storm Generation for Evaluation of the Storm Surge Inundation Threat. Ocean. Eng. 43, 13–22. doi:10.1016/j.oceaneng.2012.01.021
Corbella, S., and Stretch, D. D. (2013). Simulating a Multivariate Sea Storm Using Archimedean Copulas. Coast. Eng. 76, 68–78. doi:10.1016/j.coastaleng.2013.01.011
Dawson, T. H., Kriebel, D. L., and Wallendorf, L. A. (1993). Breaking Waves in Laboratory-Generated JONSWAP Seas. Appl. Ocean Res. 15, 85–93. doi:10.1016/0141-1187(93)90023-Q
De Michele, C., Salvadori, G., Passoni, G., and Vezzoli, R. (2007). A Multivariate Model of Sea Storms Using Copulas. Coast. Eng. 54, 734–751. doi:10.1016/j.coastaleng.2007.05.007
De Waal, D. J., and Van Gelder, P. H. A. J. M. (2005). Modelling of Extreme Wave Heights and Periods through Copulas. Extremes 8, 345–356. doi:10.1007/s10687-006-0006-y
Edwards, S. J., Troesch, A. W., and Collette, M. (2021). Estimating Extreme Characteristics of Stochastic Non-linear Systems. Ocean. Eng. 233, 109042. doi:10.1016/j.oceaneng.2021.109042
Faria, A. F. G., Thornton, E. B., and Stanton, T. P. (1997). Small-scale Morphology Related to Wave and Current Parameters over a Barred Beach. Proc. Coast. Eng. Conf. 1, 3391–3404. doi:10.1061/9780784402429.262
Forristall, G. Z. (2000). Wave Crest Distributions: Observations and Second-Order Theory. J. Phys. Oceanogr. 30, 1931–1943. doi:10.1175/1520-0485(2000)030<1931:wcdoas>2.0.co;2
Garcia-Jorcano, L., and Benito, S. (2020). Studying the Properties of the Bitcoin as a Diversifying and Hedging Asset through a Copula Analysis: Constant and Time-Varying. Res. Int. Bus. Finance 54, 101300. doi:10.1016/j.ribaf.2020.101300
Jane, R., Dalla Valle, L., Simmonds, D., and Raby, A. (2016). A Copula-Based Approach for the Estimation of Wave Height Records through Spatial Correlation. Coast. Eng. 117, 1–18. doi:10.1016/j.coastaleng.2016.06.008
Katoh, K., and Yanagishima, S. (1995). “Changes of Sand Grain Distribution in the Surf Zone,” in Coastal Dynamics - Proceedings of the International Conference, 12-16 June, 2017 (Helsingør, Denmark: Ocean Dynamics).
Kuriyama, Y. (2012). Process-based One-Dimensional Model for Cyclic Longshore Bar Evolution. Coast. Eng. 62, 48–61. doi:10.1016/j.coastaleng.2011.12.001
Kwon, H.-H., and Lall, U. (2016). A Copula-Based Nonstationary Frequency Analysis for the 2012-2015 Drought in California. Water Resour. Res. 52, 5662–5675. doi:10.1002/2016WR018959
Li, F., Gelder, P. H. A. J. M. V., Vrijling, J. K., Callaghan, D. P., Jongejan, R. B., and Ranasinghe, R. (2014a). Probabilistic Estimation of Coastal Dune Erosion and Recession by Statistical Simulation of Storm Events. Appl. Ocean Res. 47, 53–62. doi:10.1016/j.apor.2014.01.002
Li, F., van Gelder, P. H. A. J. M., Ranasinghe, R., Callaghan, D. P., and Jongejan, R. B. (2014b). Probabilistic Modelling of Extreme Storms along the Dutch Coast. Coast. Eng. 86, 1–13. doi:10.1016/j.coastaleng.2013.12.009
Lin, Y., Dong, S., and Tao, S. (2020). Modelling Long-Term Joint Distribution of Significant Wave Height and Mean Zero-Crossing Wave Period Using a Copula Mixture. Ocean. Eng. 197, 106856. doi:10.1016/j.oceaneng.2019.106856
Lowe, R. J., Buckley, M. L., Altomare, C., Rijnsdorp, D. P., Yao, Y., Suzuki, T., et al. (2019). Numerical Simulations of Surf Zone Wave Dynamics Using Smoothed Particle Hydrodynamics. Ocean. Model. 144, 101481. doi:10.1016/j.ocemod.2019.101481
Madsen, O. S. (1974). Stability of a Sand Bed under Breaking Waves. Coast. engng. Proc. 2, 776–794. doi:10.1061/9780872621138.048
Madsen, O. S. (1978). Wave-Induced Pore Pressures and Effective Stresses in a Porous Bed. Géotechnique 28, 377–393. doi:10.1680/geot.1978.28.4.377
Mieras, R. S., Puleo, J. A., Anderson, D., Cox, D. T., and Hsu, T. J. (2017). Large‐scale Experimental Observations of Sheet Flow on a Sandbar under Skewed‐asymmetric Waves. J. Geophys. Res. Oceans 122, 5022–5045. doi:10.1002/2016JC012438
Mieras, R. S., Puleo, J. A., Anderson, D., Hsu, T. J., Cox, D. T., and Calantoni, J. (2019). Relative Contributions of Bed Load and Suspended Load to Sediment Transport under Skewed‐Asymmetric Waves on a Sandbar Crest. J. Geophys. Res. Oceans 124, 1294–1321. doi:10.1029/2018JC014564
Myrhaug, D., Ong, M. C., and Holmedal, L. E. (2015). Suspended Sediments Due to Random Waves Including Effects of Second Order Wave Asymmetry and Boundary Layer Streaming. Ocean. Eng. 104, 414–421. doi:10.1016/j.oceaneng.2015.05.022
Plackett, R. L. (1965). A Class of Bivariate Distributions. J. Am. Stat. Assoc. 60, 516–522. doi:10.1080/01621459.1965.10480807
Repko, A., Van Gelder, P. H. A. J. M., Voortman, H. G., and Vrijling, J. K. (2004). Bivariate Description of Offshore Wave Conditions with Physics-Based Extreme Value Statistics. Appl. Ocean Res. 26, 162–170. doi:10.1016/j.apor.2004.10.001
Ribas, F., Swart, H. E., Calvete, D., and Falqués, A. (2012). Modeling and Analyzing Observed Transverse Sand Bars in the Surf Zone. J. Geophys. Res. 117. doi:10.1029/2011JF002158
Roelvink, J. A., Reniers, A. J. H. M., Van Dongeren, A. R., van Thiel de Vries, J. S. M., Lescinski, J., and McCall, R. T. (2010). XBeach Model Description and Manual. Available at: http://scholar.google.com/scholar?hl=en&btnG=Search&q=intitle:XBeach+Model+Description+and+Manual#0.
Ryabkova, M., Karaev, V., Guo, J., and Titchenko, Y. (2019). A Review of Wave Spectrum Models as Applied to the Problem of Radar Probing of the Sea Surface. J. Geophys. Res. Oceans 124, 7104–7134. doi:10.1029/2018JC014804
Salvadori, G., De Michele, C., and Durante, F. (2011). On the Return Period and Design in a Multivariate Framework. Hydrol. Earth Syst. Sci. 15, 3293–3305. doi:10.5194/hess-15-3293-2011
Salvadori, G., Durante, F., De Michele, C., Bernardi, M., and Petrella, L. (2016). A Multivariate Copula-Based Framework for Dealing with Hazard Scenarios and Failure Probabilities. Water Resour. Res. 52, 3701–3721. doi:10.1002/2015WR017225
Schmidt, T. (2006). “Coping with Copulas,” in Copulas - from Theory to Applications in Finance (London: Bloomberg Press).
Scott, C. P., Cox, D. T., Maddux, T. B., and Long, J. W. (2005). Large-scale Laboratory Observations of Turbulence on a Fixed Barred Beach. Meas. Sci. Technol. 16, 1903–1912. doi:10.1088/0957-0233/16/10/004
Sellés Valls, S. (2019). A Vine-Based Approach for Defining Critical Infrastructure Loads. Available at: https://repository.tudelft.nl/islandora/object/uuid%3A9a133d6d-00ca-4fcc-a244-b88215dfd578.
Shields, A. (1936). Anwendung der Aehnlichkeitsmechanik und der Turbulenzforschung auf die Geschiebebewegung. Technology 26, 26.
Sklar, A. (1959). Fonctions de Reparition a n Dimensions et Leurs Marges. Paris: Publ. l’Institute Stat. l’Universite Paris.
Sleath, J. F. A. (1999). Conditions for Plug Formation in Oscillatory Flow. Cont. Shelf Res. 19, 1643–1664. doi:10.1016/S0278-4343(98)00096-X
Sleath, J. F. A. (1970). Wave-Induced Pressures in Beds of Sand. J. Hydr. Div. 96, 367–378. doi:10.1061/jyceaj.0002325
Suzuki, T., Inami, Y., Yanagishima, S., Sakihama, S., and Cox, D. (2018). OBSERVATIONS OF SEDIMENT PARTICLE MOVEMENTS UNDER ACCRETIVE WAVE CONDITIONS. Int. Conf. Coast. Eng. 36, 68. doi:10.9753/icce.v36.sediment.68
Suzuki, T., Mori, N., and Cox, D. T. (2009). Statistical Modeling of Near-Bed Pressure Gradients Measured on a Natural Beach. Coast. Eng. J. 51, 101–121. doi:10.1142/S0578563409001965
Suzuki, T., Shin, S., Cox, D. T., and Mori, N. (2010). Spatiotemporal Characteristics of Near-Bed Pressure Gradients on a Barred Beach. J. Waterw. Port. Coast. Ocean. Eng. 136, 327–336. doi:10.1061/(asce)ww.1943-5460.0000055
Swart, D. H. (1974). Offshore Sediment Transport and Equilibrium Beach Profiles. Delft: Institutional Repository. doctoral thesis.
Tabasi, M., Soltanpour, M., Jayaratne, R., Shibayama, T., and Okayasu, A. (2018). A NUMERICAL MODEL OF CROSS-SHORE BEACH PROFILE EVOLUTION: THEORY, MODEL DEVELOPMENT AND APPLICABILITY. Int. Conf. Coast. Eng. 36, 69. doi:10.9753/icce.v36.papers.69
Tabasi, M., Soltanpour, M., and Jayaratne, R. (2017). “Study and Modeling of Cross-Shore Sediment Transport at Zarabad Fishery Port,” in 37th IAHR World Congress (Kuala Lumpur: Cross-shore Sediment Transport), 3256–3265.
Tabasi, M., Soltanpour, M., Suzuki, T., and Jayaratne, R. (2020). Modeling of Berm Formation and Erosion at the Southern Coast of the Caspian Sea. Int. Conf. Coast. Eng. 19, 19. doi:10.9753/icce.v36v.papers.19
Tabasi, M., Yamada, M., and Suzuki, T. (2021). Laboratory Investigation of the Influence of Sediment Compaction on Wave-Induced Pore Pressure Gradient and Sediment Concentration for a Sandbar. J. Jpn. Soc. Civ. Eng. 77, 397–402. doi:10.2208/kaigan.77.2_i_397
Tayfun, M. A., and Fedele, F. (2007). Wave-height Distributions and Nonlinear Effects. Ocean. Eng. 34, 1631–1649. doi:10.1016/j.oceaneng.2006.11.006
Vrijling, J. K., and Bruinsma, J. (1980). Hydraulic Boundary Conditions. Hydraulic. Aspects Of Coastal Structures. Developments In Hydraulic, 2, 109–133.
Wahl, T., Jensen, J., and Mudersbach, C. (2011). A Multivariate Statistical ModeL for Advanced Storm Surge Analyses in the North Sea. Int. Conf. Coast. Eng. 1, 19. doi:10.9753/icce.v32.currents.19
Wahl, T., Mudersbach, C., and Jensen, J. (2012). Assessing the Hydrodynamic Boundary Conditions for Risk Analyses in Coastal Areas: A Multivariate Statistical Approach Based on Copula Functions. Nat. Hazards Earth Syst. Sci. 12, 495–510. doi:10.5194/nhess-12-495-2012
Wilson, K. C. (1989). Friction of Wave-Induced Sheet Flow. Coast. Eng. 12, 371–379. doi:10.1016/0378-3839(89)90013-6
Keywords: wave-induced pressure gradient 1, local acceleration, surf zone, copula, multivariate analysis, barred beach
Citation: Tabasi M, Suzuki T and Cox DT (2022) A Copula-Based Simulation of Wave-Induced Pore Water Pressure Gradient and Local Acceleration Within Surf Zone for Natural and Laboratory Barred Beach Profiles. Front. Built Environ. 8:816020. doi: 10.3389/fbuil.2022.816020
Received: 16 November 2021; Accepted: 13 June 2022;
Published: 08 July 2022.
Edited by:
Barbara Zanuttigh, University of Bologna, ItalyReviewed by:
Sebastian Solari, Universidad de la República, UruguayCopyright © 2022 Tabasi, Suzuki and Cox. This is an open-access article distributed under the terms of the Creative Commons Attribution License (CC BY). The use, distribution or reproduction in other forums is permitted, provided the original author(s) and the copyright owner(s) are credited and that the original publication in this journal is cited, in accordance with accepted academic practice. No use, distribution or reproduction is permitted which does not comply with these terms.
*Correspondence: Mohammad Tabasi, dGFiYXNpLW1vaGFtbWFkLXJuQHludS5qcA==
Disclaimer: All claims expressed in this article are solely those of the authors and do not necessarily represent those of their affiliated organizations, or those of the publisher, the editors and the reviewers. Any product that may be evaluated in this article or claim that may be made by its manufacturer is not guaranteed or endorsed by the publisher.
Research integrity at Frontiers
Learn more about the work of our research integrity team to safeguard the quality of each article we publish.