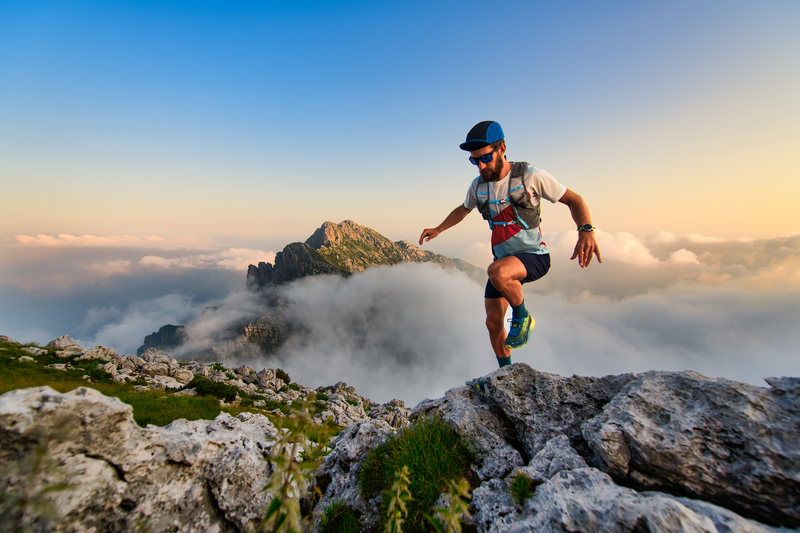
95% of researchers rate our articles as excellent or good
Learn more about the work of our research integrity team to safeguard the quality of each article we publish.
Find out more
REVIEW article
Front. Built Environ. , 18 February 2022
Sec. Indoor Environment
Volume 8 - 2022 | https://doi.org/10.3389/fbuil.2022.787289
This article is part of the Research Topic Innovative Human-Centric Investigations and Technologies for Human Wellbeing and Health in the Built Environment View all 8 articles
An understanding of indoor environmental quality (IEQ) and its effects on occupant well-being can inform building system design and operation. The use of wearables in field studies to collect subjective and objective health performance indicators (HPIs) from a large number of occupants could deliver important improvements in IEQ. To facilitate the use of wearables in IEQ studies, there is a need to identify which HPIs should be collected and to evaluate data accessibility from these devices. To address this issue, a literature review of previous IEQ studies was conducted to identify relationships between different IEQ factors and HPIs, with a focus on HPIs that were collected using wearables. A preliminary assessment of data accessibility from a selected wearable device (Fitbit Versa 2) was performed and documented. The review suggested the need to further investigate and collect sleep quality parameters, heart rate, stress response, as well as subjective ratings of comfort using wearables. The data accessibility assessment revealed issues related to missing data points and data resolution from the examined device. A set of recommendations is outlined to inform future studies.
The original energy crisis of the 1970s stimulated five decades of a nearly continuous focus on improving the energy efficiency of buildings. The systems used to provide heating, air conditioning, ventilation, and illumination in buildings continue to be radically transformed by this focus, along with other energy users such as appliances, water heaters, refrigeration units, computers, and other office equipment. Whereas early implementations of energy-efficient building technologies focused on achieving energy savings while maintaining some simplistic measures of performance (e.g., illuminance, temperature, humidity), today’s evaluations of building performance strive to account for a more holistic set of occupant experiences.
These occupant experiences are often expressed in terms of indoor environmental quality (IEQ). As embodied in the US Green Building Council’s Leadership in Energy and Environmental Design (LEED) Rating System, IEQ includes considerations of indoor air quality, thermal comfort, acoustic performance, quality interior lighting (glare control, color rendering, dimming), daylight exposure, and quality views to the outdoors (USGBC, 2021). Beyond energy savings, the justification for addressing these other attributes includes protecting occupant health; promoting occupant productivity, comfort, and well-being; reinforcing circadian rhythms; enhancing a connection to nature; and facilitating communications.
An understanding of IEQ and its effects on occupant well-being can inform building system design and operation (Bluyssen, 2013). Physical measurements of environmental attributes such as illuminance and sound levels are sometimes used to infer potential effects on occupants, but these inferences typically rely on general relationships between the IEQ measures and the occupant response. These responses can vary by building and occupant type (e.g., workers in an office may have a different response than nurses at a hospital). In field studies, subjective measures such as perceived comfort and satisfaction can be collected, but access to employees and budget constraints usually limit the ability to collect objective well-being indicators such as heart rate and activity level from occupants; these measures combined with subjective responses provide a more complete view of occupant responses to the environment.
Methods and tools that can be utilized in the field to collect both subjective and objective well-being data from many occupants have the potential to improve our understanding of the relationships between IEQ and occupant responses, and ultimately to ensure that energy-efficient building technologies achieve significant savings while supporting occupant needs. Recent studies have begun exploring the use of non-invasive wearable devices, such as smartwatches, for administering micro ecological momentary assessments (μEMAs) (Intille et al., 2016; Jayathissa et al., 2019, 2020a). These devices can also gather objective data on measures such as sleep quality, physical activity, and heart rate; collectively referred to herein as health performance indicators (HPIs) (Allen et al., 2015). While the possibility of more easily collecting these HPIs while occupants are engaged in normal activities holds great promise, it also raises new challenges related to knowing which HPIs to collect, understanding precedents for measuring HPIs using wearables, and ensuring data accessibility from wearable devices.
This article aims to answer two questions: 1) which HPIs should be collected using wearables? 2) how accessible is the data? While there are several types of wearable devices that can be worn on the wrist, chest, as a headset, or as a clip-on sensor (Piwek et al., 2016; Taub et al., 2016), in this article the focus is on wrist or chest-mounted devices that can be worn by occupants while performing daily tasks in a field setting. In Section 2, relationships between IEQ factors and HPIs were summarized with a focus on identifying HPIs that can be collected using wearables. Second, data accessibility from a market-available wearable device (Fitbit Versa 2) is documented. Lastly, recommendations for data collection and data accessibility verification are described. The goal is to facilitate the use of wearables in future IEQ field studies where data can be collected from a large number of participants for extended durations. This can further our understanding of dose-response relationships and interactions between IEQ factors.
Well-being is a broad construct that includes physiological, psychological, and cognitive HPIs such as stress, sleep quality, comfort and satisfaction, mood, and cognitive performance. The relationships between these HPIs and IEQ factors were examined in previous studies (Veitch et al., 2008; Allen et al., 2016; Colenberg et al., 2020) using different approaches. Some of the studies used occupant questionnaires, with or without IEQ measurements (Altomonte and Schiavon, 2013; Allen et al., 2015), while others also included objectively-measured HPIs such as heart rate variability, cortisol levels, and sleep duration (Thayer et al., 2010; Boubekri et al., 2020).
Wearable devices can be used to collect localized IEQ measurements as well as subjective and objective HPIs that traditionally have been limited to laboratory settings or a small number of participants. Some examples include measuring illuminance at the eye, CO2 in the inhalation zone (Coulby et al., 2020; Salamone et al., 2021), EMAs, and electrodermal activity (Jayathissa et al., 2020a; Zhang et al., 2020). However, to effectively utilize wearables in IEQ studies, there is a need to identify IEQ factors and HPIs that need to be collected (Altomonte et al., 2020), explore the role of wearables for collecting these data, and discuss considerations for data collection. These issues are summarized and discussed through a literature review of previous relevant studies. Given that the focus was on the role of wearables, the review outlined in this section is not exhaustive and does not discuss all possible relationships between IEQ factors and HPIs.
Previous studies examined occupants’ comfort and satisfaction to determine comfortable and acceptable levels of different IEQ factors. A detailed questionnaire was often used at a single or few points in time to elicit responses evaluating satisfaction with various environmental factors (Choi et al., 2012a; Heinzerling et al., 2013; Elzeyadi et al., 2017; Park et al., 2019). While many IEQ factors can affect occupant’s comfort, a recent analysis of a large database revealed that satisfaction levels were lowest for sound privacy, noise level, and temperature (Graham et al., 2021). While detailed questionnaires can be helpful for general evaluations and for identifying sources of discomfort, they are unlikely to capture temporal variations in comfort and satisfaction as a result of changing IEQ.
To track such variations, EMAs on smartphones or wearables can be used (Wei et al., 2014; Konis and Annavaram, 2017; de Vries et al., 2021; Peeters et al., 2021). μEMAs can provide higher granularity, response rate, and are perceived to be less distracting compared to online questionnaires or EMAs administered on smart-phones (Intille et al., 2016; de Vries et al., 2021). Jayathissa et al. (2020b) demonstrated potential for using μEMAs on a Fitbit smartwatch to record thermal, lighting, and acoustical preferences (5–15 prompts per day). These preferences can be used to identify occupant and space profiles and make comfort predictions that could potentially be used to control building systems.
Sick building syndrome (SBS) symptoms describe building-related symptoms experienced by building occupants that are relieved or go away after leaving the building. These symptoms can be influenced by environmental and personal factors and can be classified as (1) upper respiratory and mucosal symptoms such as dry or itchy eyes; (2) lower respiratory irritation like cough; (3) neurophysiological symptoms such as headache and mental fatigue; and (4) skin irritation symptoms like itching or reddening (Apte et al., 2000; Bluyssen et al., 2016; Sakellaris et al., 2020). Several studies associated air quality factors such as CO2, NO2, nicotine, particulate matter (PM), volatile organic compounds (VOCs), and microbial contamination with SBS symptoms (Erdmann et al., 2002; Menzies et al., 2003; Mitchell et al., 2007; Colton et al., 2014; Azuma et al., 2018). Additionally, air temperature and relative humidity were also linked to SBS symptoms (Arundel et al., 1986; Fang et al., 2004; Seppänen and Fisk, 2006; Wolkoff, 2017). To the authors’ knowledge, previous studies have not used μEMAs to check SBS symptoms. This is expected because participants typically report the frequency of experiencing symptoms over the past month using detailed questionnaires (Apte et al., 2000).
In addition to ratings of comfort and satisfaction, variations in sleepiness can be captured using EMAs. Generally, lighting has been shown to decrease subjective sleepiness, but this response may vary by time of the day (Vetter et al., 2021). One of the studies that had a different conclusion was a 3-weeks study where higher illuminance at the eye led to negative effects on sleepiness in the spring and no effects in the winter (Peeters et al., 2021). This study evaluated sleepiness using the Karolinska Sleepiness Scale (Åkerstedt and Gillberg, 1990) that was completed eight times a day using EMAs on mobile phones. This highlights the importance of capturing temporal and seasonal variations in sleepiness. In addition to lighting, high noise levels can affect self-reported tiredness (self-reported number of yawns in the last 10 min) and motivation, compared to low noise (Jahncke et al., 2011).
Previous field studies utilized wearables that track activity (actigraphs) to track sleep in relation to variations in light intensity and spectral power distribution (de la Iglesia et al., 2015; Wams et al., 2017; Cain et al., 2020; Peeters et al., 2020). For example, a study by Boubekri et al. (2020) used a wrist-worn device to track sleep duration over a week and found a significant increase of 37 min in sleep duration associated with working in an office that had optimized daylight and views, compared to roller shades. Sleep quality parameters such as duration of deep sleep, number of awakenings, sleep efficiency, and sleep onset latency—all assessed using actigraphy—can be negatively affected by other IEQ factors such as CO2 levels (Akimoto et al., 2021) and air temperature (Pan et al., 2012; Caddick et al., 2018).
The stress response can be elicited by several IEQ factors. Thayer et al. (2010) examined two aspects of physiological stress, circadian variations in heart rate variability and morning rise in cortisol, for a group of 60 participants working in a traditional or a modern office building. They found that physical features of the work environment such as lighting, views, acoustics, and air quality may affect both physiological stress indicators. In another study, Razjouyan et al. (2020) used a chest-worn sensor to examine the effects of relative humidity in offices on the stress response. They found significant effects of relative humidity on heart rate variability indices while occupants were in the office. Participants that spent the majority of their work time with relative humidity between 30 and 60% experienced 25% less stress, compared to those that spent the majority of their time in relative humidity levels under 30%. The chest-worn sensor also estimated sleep quality, with the authors finding a significant indirect effect of relative humidity on sleep quality, mediated by the stress response.
Other studies have shown that noise levels can affect the stress response. Physiological stress (indicated by skin conductance levels) and respiratory rate increased at higher noise levels, moderated by working experience (Shafiee Motlagh et al., 2018). Furthermore, cognitive stress self-reported using the cognitive stress scale was lower in open offices in a condition with enhanced sound absorption (Seddigh et al., 2015; Colenberg et al., 2020). Other factors such as workstation type and outdoor view type were also found to affect stress response (Kaplan, 1995; Bjørnstad et al., 2015; Lindberg et al., 2018).
Increased CO2 levels can have negative physiological and cognitive effects (Azuma et al., 2018). Compared to 600 ppm, Satish et al. (2012) showed that the mean score of nine decision-making tasks dropped 12 and 51% under CO2 levels of 1,000 and 2,500 ppm, respectively. These results are consistent with another study that found VOCs and CO2 to be independently associated with cognitive scores (Allen et al., 2016). The mechanism by which CO2 and VOCs affect cognitive performance remains unclear.
Lighting and access to views can affect cognitive performance. A previous study reported 26–62% higher cognitive function scores across the nine domains of the strategic management simulation test in an office with electrochromic glazing compared to roller blinds (Boubekri et al., 2020). These results are consistent with the results of another study that found improvements in working memory and inhibition in setting with electrochromic glazing or mesh shades, compared to blackout shades (Jamrozik et al., 2019). Using electric lighting only, Ru et al. (2019) found significant improvements in reaction speed at1000 lux compared to 100 lux at the eye.
It is important to note that most previous studies assessed cognitive performance using computer-based tasks. Some studies, outside the IEQ research domain, explored administering cognitive tests using wrist-mounted devices, smartphones, and tablets to overcome challenges related to administering cognitive tests in field studies and to allow for more frequent assessments of cognitive performance (Matsangas et al., 2017; Moore et al., 2017; Arsintescu et al., 2019; Koo and Vizer, 2019; Laurent et al., 2021). Currently, the use of wearables for cognitive tests limits the types of cognitive tests that can be administered, but this may change as more cognitive tests are developed for wearables.
Table 1 summarizes the studied relationships between IEQ factors and HPIs. This table does not show all possible relationships and does not suggest that a causation relationship was demonstrated, but it highlights objective HPIs that were collected using wrist or chest-mounted devices, as well as subjective HPIs that were assessed using EMAs from wearables or smartphones. Based on this table and the reviewed studies in Section 2.1.1 through Section 2.1.6, a few key points can be noted:
• Wearables were used in previous IEQ studies to collect several HPIs including sleep quality, heart rate, heart rate variability, and subjective responses.
• In several cases, the same HPI is affected by multiple IEQ factors. For example, sleep quality can be affected by lighting, CO2, noise level, and air temperature. This suggests that studies aiming to examine the effects of lighting on sleep quality should consider potential impacts of other IEQ factors as well as possible interaction effects between the IEQ factors.
• There is a need for a more holistic research model that addresses other factors, interactions, and combined effects (Bluyssen, 2020). Other stressors that need to be addressed include psychological, work-related factors, social stressors, and personal characteristics (Bluyssen et al., 2011).
• Because of the complexity in the relationships, it is important to explore how data from wearables can augment existing data collection tools, e.g., online questionnaires, to provide a comprehensive understanding of IEQ-HPI relationships.
• Lastly, most studies did not discuss the process and criteria used for the selection of wearable devices. This information is needed to help researchers select suitable devices and effectively utilize them.
TABLE 1. A summary of subjective and objective HPIs that were collected in at least one IEQ study using wearables. HPIs collected using smartphones are included for reference.
The spatial and temporal sampling resolution needed for IEQ measurements will vary based on the research questions and objectives. As discussed by Parkinson et al. (2019), spatio-temporal sampling is limited by the logistical challenges of characterizing different IEQ parameters with significant variability in time and space scales. Physical features of the space or activities of the occupants may also limit the sampling resolution of IEQ measurements as it is important to avoid any distraction or hindrance to participants. Previous studies measured IEQ data at different spatial resolutions ranging from localized measurements from wearables to point-in-time measurements using a handheld device or IEQ carts (Heinzerling et al., 2013).
Wearable devices offer the highest spatial resolution, continuously monitoring IEQ conditions experienced by individual participants as they move throughout multiple rooms or buildings (Figueiro et al., 2019; Boubekri et al., 2020; Cain et al., 2020; Peeters et al., 2020). The use of wearable devices to sense IEQ conditions provides advantages over fixed sensing stations in spaces with high spatial and/or temporal variability (Adamsson et al., 2019; Clements et al., 2019). Personal light exposure is one of the most common IEQ parameters collected via wearables, but it is also possible to measure and track temperature, relative humidity, CO2, and sound level. One study showed that thermal comfort sensors could be placed into a small enclosure and worn on the wrist; those sensors could then control an air conditioning system based on localized comfort estimates (Feldmeier and Paradiso, 2010). Ghahramani et al. (2018) utilized a wearable sensor worn on the chest to measure sound pressure level, CO2, illuminance, air temperature, relative humidity, and pressure. A large-scale study used sound measurements from a smart watch to examine personal sound exposures (Smith et al., 2020). Salamone et al. (2021) provided a comprehensive review of previous studies that used wearables for IEQ sensing.
Localized IEQ measurements using wearables can be directly related to other occupant-level data such as HPIs but may not be representative of ambient space-level conditions. For studies where participants spend the majority of their time in one location, like offices, a fixed monitoring station at each desk allows IEQ measurements to be captured and related to each participant (MacNaughton et al., 2016; Jamrozik et al., 2018; Clements et al., 2019). For environments where desktop monitoring stations are not feasible, a coarser approach is to use sensor stations on a mobile cart. These stations are often placed in the center of the room, capturing IEQ measurements overtime, or moved between workstations to capture point-in-time measurements of conditions participants may be experiencing (Chiang et al., 2001; Castaldo et al., 2018; Choi and Lee, 2018; Jin et al., 2018).
In addition to measuring IEQ factors and HPIs, previous studies suggested other data types that need to be collected for more holistic investigations (Figure 1). Personal and work-related factors can directly affect or mediate effects of IEQ on occupant well-being (Veitch, 2001; Boyce, 2003; Bluyssen et al., 2011; Bluyssen, 2020). The model proposed by Bluyssen et al. (2011) considered both physical and psychosocial stressors and their effects on well-being. Many personal factors can be considered including age, gender, ethnicity, life events, body mass index, consumption of stimulants, smoking, use of medications (allergy, anxiety, melatonin, sleep), chronotype, sleep disturbances, and responsibilities outside of work. Work-related factors include workload, job type, satisfaction with work, perceived productivity, workstation type, and level of education (Bluyssen et al., 2011). These factors can be used in the inclusion or exclusion process and can aid in data analysis to account for potential confounding effects. For example, Boubekri et al. (2020) collected information on the medical status of potential participants and excluded those that had sleep apnea, chronic depression, and other health issues that may affect sleep.
FIGURE 1. A diagram showing four main data types that are needed for a holistic evaluation of IEQ. Bold italic font denotes HPIs that can be collected from wearables.
In studies where the goal is to examine relationships between building system characteristics and occupant well-being, it is important to document general building attributes and system characteristics. Building system characteristics can be documented using existing checklists, such as the modified checklist from the Health Optimisation Protocol for Energy-efficient Buildings (HOPE) study (Cox, 2005; Bluyssen et al., 2016) and the Technical Attributes of Building Systems (TABS) survey (Aziz et al., 2010).
The use of wearable devices in a research study can raise privacy concerns that may affect recruitment and collected responses. Hence, it is important to develop a strategy to address privacy during early stages of research planning. Safavi and Shukur (2014) proposed a conceptual framework that included ten principles for collecting and handling health data from wearables. The principles highlight the importance of transparency in communicating study purpose, the technology used to collect data, data collection procedures, and ownership of collected data.
Paul and Irvine (2014) tested four wearable devices and found that two had policies allowing them to collect information about the user from other sources. Other issues in privacy policies were related to data ownership, right to privacy of data, and whether device manufacturers can use collected data for commercial purposes. In a study that examined privacy concerns for wrist-mounted devices, participants were concerned about the Global Positioning System (GPS) sensor (Motti and Caine, 2015). Additionally, participants were generally concerned about these devices collecting information about them from a social network, the inability to recall and delete collected data, and having organizations or the government access the data without their awareness and consent. Fitbit’s approach of using social networks, such as Facebook, to log in can lead to concerns on data breaches or misuse of user profile data (Orlosky et al., 2019). To protect the subject’s privacy when publishing data, several techniques are often used such as anonymization, deidentification, and pseudonymization.
Data anonymization can be defined as an irreversible removal of the link between a subject and his/her record data (Kushida et al., 2012). This requires an a priori decision by researchers whether there would be any need in the future to link data to subjects, such as if there might be data removal requests when a subject opts out of a study. A less stringent technique is data de-identification, which is the removal or manipulation of direct and indirect identifiers such that reestablishing a link between a subject and his/her data would require a key that can be used to reverse the de-identification process (Garfinkel, 2015). De-identification can be achieved by replacing identifiers with pseudonyms or codes, or pseudonymization (Ren et al., 2021). For example, Kallio et al. (2020) assigned pseudonymization codes to participants that were used to log in and provide ratings of IEQ. Another study generated an ID for each participant using a cryptographic secure hash algorithm (SHA-1) using father and mother initials and months of birth (Bluyssen et al., 2016).
A complementary procedure that can be considered for certain data types from wearables is generalization, which transforms absolute data to ranges or categories. For example, age 30 can be transformed to age 25–35. Another example is location obfuscation, which is to deliberately reduce the precision of the position info. Like a circular area instead of exact geographical coordinates (Liu et al., 2018).
The previous section highlighted four main HPIs that were collected in prior studies using wearables including μEMAs of comfort ratings, sleep quality, heart rate, and stress response. To ensure success in a research study that uses wearables, researchers will likely need to examine several devices to select a device that fits the needs of the study. Device selection criteria may include data accessibility, types of data generated, accuracy and validation for the population of interest, sampling frequency, as well as system integration, and scalability. Other criteria that can be considered when selecting a wearable device are device reliability over time, device malfunction, firmware updates, and the use of proprietary algorithms (de Zambotti et al., 2019).
Arguably, a critical requirement for considering the use of a wearable device in a research study is data accessibility, which can be particularly challenging from consumer-grade devices that are typically intended for general tracking. Different devices may offer different access options that may require further effort or affect data resolution. Generally, previous IEQ studies that used wearables did not report device selection process and how data accessibility was verified prior to start of study. While most manufacturers clearly state data access options, some may require technical implementations such as the use of authentication (i.e., OAuth) and/or user/key management, and some may have reliability issues such as dropped data points.
Several wearable devices were used in previous studies, as shown in Table 1. Out of these devices, Fitbit Versa allowed for administering μEMAs. While this model is currently obsolete, its successor Fitbit Versa 2 can also be used with the Cozie clockface to prompt μEMAs (Jayathissa et al., 2020a). Additionally, Fitbit Versa 2 collects sleep quality, heart rate, physical activity, and the companion phone application calculates metrics such as heart rate variability, resting heart rate, and breathing rate. The advantages and drawbacks of other devices that we reviewed are shown in Table 2. Some devices did not collect heart rate, had a short battery life that makes it inadequate for IEQ field studies, or device cost was high limiting the ability to deploy it to a large number of participants in a field IEQ study. Research-grade devices tended to require a wired connection via to retrieve data which make them more appropriate for laboratory studies.
TABLE 2. A summary of wearable devices that were considered for the data-accessibility investigation.
The Fitbit Versa 2 collects the needed HPIs and may be appropriate for IEQ field studies. To explore data access options, accessible data types, and identify potential issues that might be encountered when using this device, a preliminary assessment of data accessibility from this device was conducted. Data accessibility was the only topic being explored; this study did not investigate any hypotheses related to the collected data.
Three Fitbit Versa 2 devices (Version 35.72.1.15; 9/2021) were acquired and worn by three of the authors for 2 weeks. During this period, these three participants volunteered to complete daily μEMAs using the Cozie clockface which included default questions about participant’s overall comfort, thermal comfort, satisfaction with lighting, noise, and location (indoor/outdoor). Figure 2 shows example questions from the Cozie question library as presented on the device (Jayathissa et al., 2020b). Each question could be answered by selecting one of two or three responses that were presented on the watch screen. The three authors that participated were working remotely during the 2-week test period. Each participant received a Fitbit Versa 2 a few weeks before the test period to become familiar with the device. The characteristics of the device are shown in Table 2. During the testing period, they were asked to complete as many μEMAs as possible while their phones were connected to internet via Wi-Fi or a cellular service.
Two aspects of data accessibility were evaluated: checking μEMAs for missing responses, and verifying the resolution of sleep, heart rate, and physical activity data. μEMA responses from each participant were assigned unique consecutive numbers, i.e., count. A missing count number indicates a missing response that was inaccessible. Fitbit data resolution was checked by directly querying the data using device API or web API (Fitbit, 2021).
All data from the three Fitbit devices were collected in a JavaScript Object Notation (JSON) file format and forwarded to a serverless function hosted on Azure cloud computing services. The serverless functions were Python scripts that checked incoming data for correct source, structure, and organization. Incoming data were passed to an Azure CosmoDB database, which could be accessed by the authors. The data were saved in a nested JSON format where higher levels have generic and wide-reaching descriptors while low levels are composed of specific and limited descriptors. The descriptors were formatted in a key-value paradigm structure (i.e., participant-name). The overall data collection structure allowed for each data source to collect their data independently and forward it to the same cloud service.
The three participants completed 50, 56, and 57 μEMAs. The responses consisted of a numerical value for each response and did not require any post processing as shown in the example in Figure 3 and in Jayathissa et al. (2020b). The percentage of accessible EMAs were 88, 89, and 96.5%, respectively. In general, out of the 163 μEMAs that were completed using the Cozie clockface, 149 (91.4%) were accessible. This led to the conclusion that some of the μEMAs were being dropped. This issue could be due to the Fitbit not syncing the responses with the mobile device causing the dropped responses. One potential solution is to send reminders to participants asking them to maintain a connection between the wearable and mobile phone to regularly sync their responses. Given that responses were stored locally on the device then uploaded to the server, it is unlikely that the missing responses are due to the use of Wi-Fi versus cellular internet connection. GPS data were not accessible if a participant did not carry their phone at the time of completing a µEMA, this is because the Fitbit Versa 2 did not have a dedicated GPS sensor and used GPS data from the connected phone.
FIGURE 3. Example μEMA responses and ancillary data from the Cozie clockface (A), and sleep quality data (B).
The Fitbit Versa 2 provided sleep quality, heart rate, and physical activity data in their respective metrics and did not require any post processing (Fitbit, 2021). We found that the resolution of sleep and heart rate data was limited when accessed directly using the device API, compared to the web API. Using the device API, sleep data were binary indicating whether a person was asleep or not at the time of the query. Similarly, heart rate data were only for one point in time. The Web API provided sleep duration and time spent in each sleep stage in minutes as shown in Figure 3. Heart rate time-series data were collected at about 10-s intervals. Physical activity data from the Web API were daily summaries as well as minutes spent in each activity level (sedentary, light activity, fairly active, and very active). Physical activity data could not be accessed using the device API. Other metrics that were displayed in the Fitbit app like heart rate variability and breathing rate were not accessible from either access option.
Due to the nature of internet-capable devices and services, their software is subject to being updated partway through an experiment (Woolley et al., 2019; Chinoy et al., 2021; de Zambotti et al., 2019). This can cause unnoticeable to disruptive changes that can affect the consistency of measurements. This issue was not evaluated and warrants further investigation. The evaluation of data accessibility and resolution that we conducted focused on understanding device capability. A case study evaluation of wearables for a specific use case was outside the scope of the current investigation but important to explore in future studies.
The recommendations in this section are based on the literature review (Section 2) as well as the preliminary device assessment (Section 3). It is important to note that the recommendations are intended to be a starting point for researchers and are expected to be informed by specific research questions, research setting(s), study design, participant demographics, wearable device capabilities, along with other practical considerations for field studies.
To help evaluate effects of IEQ factors on occupant well-being, we recommend collecting subjective ratings of comfort via μEMAs, sleep quality parameters (sleep onset, sleep offset, sleep duration, and sleep efficiency), heart rate, and stress response as indicated by heart rate variability or skin conductance. Collecting these HPIs can help understand the mechanisms by which IEQ factors affect well-being. For example, Razjouyan et al. (2020) found indirect effects of relative humidity on sleep quality mediated by stress responses. It is important to note that each one of these HPIs can be influenced by several IEQ factors (Table 1). Therefore, we strongly recommend recording all related IEQ factors. This can help better quantify direct and indirect effects as well as interactions between different IEQ factors.
Administering μEMAs on comfort ratings on a smartwatch is recommended because it can help improve completion and compliance rates while reducing perceived distraction, compared to EMAs administered on a smartphone (Intille et al., 2016). It is important to note that standardized questionnaires such as Karolinska Sleepiness Scale will need to be adapted and validated for use on a wearable device. The frequency and timing of μEMA prompt are dependent on the research questions, design, and setting. For example, a study examining changes in reported comfort in a research setting with high temporal variability in IEQ conditions may have a higher number of prompts compared to another study conducted in a research setting with fairly stable conditions (Clements et al., 2019). It is important to note that there is no agreed-upon maximum number of μEMA prompts acceptable. Generally, we recommend up to five prompts a day to reduce participation burden (Burke et al., 2017), which may affect wearable device compliance over the course of a study (Mundnich et al., 2020).
Wearable sensors can be used to capture localized IEQ measurements. The location of the wearable sensor should be carefully considered as it may affect the performance of the device and usability of collected data. For example, to address the non-image-forming effects of light, vertical illuminance and spectrum are best measured using a wearable sensor placed close to the eye (Aarts et al., 2017; Peeters et al., 2021). Wearable light sensors should be placed on the front of the participant’s torso within approximately 20 cm from the chin (Cain et al., 2020), in a location that will not be blocked by clothing or hair. We do not recommend wrist-worn devices for measuring light exposure as they can be easily covered by clothing and may not provide an accurate representation of light at the eye of the occupant (Figueiro et al., 2013).
When wearables are used in an IEQ study, it is important to develop a strategy and implement techniques to protect the privacy of participants. A pseudonymization technique can be used for its flexibility, as used by Bluyssen et al. (2016). For example, it allows researchers to identify a participant to delete all or part of their data after data collection had ended. Another technique that is particularly useful for GPS data is generalization, which can help identify a participant’s general location, e.g., to determine if a participant was in or out of the office (Liu et al., 2018). These two techniques can facilitate recruitment by alleviating privacy concerns and reducing the ability to re-identify participants from IEQ sensors and wearable data.
While Table 2 shows several criteria that can be considered when selecting a wearable device, our assessment (Section 3) was limited to exploring data accessibility. Different platforms may have one or several options for accessing the data. If a system offers multiple data access options, it should be considered that the accuracy and precision of the data may differ depending on the access method. Some examples of the different ways data can be accessed include raw sensor values, web API, data forwards, bulk FTP downloads, web dashboards, and csv file download. Researchers and investigators should be aware that data points may be dropped or go missing throughout an experiment. For example, a device that is reliant on a smartphone connection for internet access may be susceptible to dropping data at multiple points in the chain of communicating data points to the investigator.
To verify data accessibility, we recommend first exploring the various access options the device provides. Manufacturer’s documentation and previous studies that used the same device can be a good place to start. While a literature review can aid in determining the specifications of a system, real-world implementations can reveal unforeseen issues. Therefore, we also recommend testing sample devices prior to the start of a study. This testing can help assess data accessibility by determining whether all data types were accessible at the desired resolution, and whether any data points were lost.
HPIs that were collected using wearables and are relevant to IEQ factors include comfort ratings, sleep quality parameters, heart rate, and stress response. Our review showed that each one of these HPIs can be affected by multiple IEQ factors. Hence, ideally, all relevant IEQ factors should be monitored to better quantify their direct and indirect effects as well as any potential interactions. Similarly, monitoring multiple HPIs is highly advantageous to help identify the mechanisms by which IEQ factors affect different HPIs. For example, to determine whether effects of relative humidity on sleep quality are mediated by stress response as explored by Razjouyan et al. (2020).
The evaluation of data accessibility from the Fitbit Versa 2 with Cozie clockface proved helpful as we found that some μEMA data were lost and that there were differences in data resolution depending on how the data were collected. Conducting a similar evaluation is recommended prior to research or operational deployment to identify potential issues and, if possible, mitigate them (e.g., choose a data collection option that provides necessary resolution) or develop methods for limiting their impact on data usage (e.g., collect more than the minimum data required for analysis to improve resiliency against data loss). Based on the results of this work, future IEQ research studies that utilize wearables are encouraged to report and discuss device selection process and data accessibility challenges, and propose best practices for human subject recruitment, human subject engagement and compensation, study duration, and data analysis.
If data accessibility and other challenges can be overcome, the use of wearables might be extended beyond research studies to use in building system control. For example, subjective alertness response data might be used in control strategies that adjust light color and intensity to improve alertness. Such closed-loop systems could provide continuous refinements that are customized to the actual building occupants, rather than based on recommended practices or estimates of “average” occupant needs. As a result, these systems might deliver significant improvements to occupant well-being and reductions in energy use as compared to standard practices.
All authors contributed to the writing and reviewing of this manuscript. BA managed the study and contributed to study conceptualization. RD and MP contributed to study conceptualization and provided mentorship and supervision. All the authors have read and agreed to the published version of the manuscript.
This research was supported by the Energy Mission Seed Investment, under the Laboratory Directed Research and Development (LDRD) Program at Pacific Northwest National Laboratory (PNNL). PNNL is a multi-program national laboratory operated for the U.S. Department of Energy (DOE) by Battelle Memorial Institute under Contract No. DE-AC05-76RL01830.
The authors declare that the research was conducted in the absence of any commercial or financial relationships that could be construed as a potential conflict of interest.
All claims expressed in this article are solely those of the authors and do not necessarily represent those of their affiliated organizations, or those of the publisher, the editors, and the reviewers. Any product that may be evaluated in this article, or claim that may be made by its manufacturer, is not guaranteed or endorsed by the publisher.
We would like to thank Dan Corbriani for his help with data structures and Azure cloud services, Trevor Gudeman and Heather Orr for their help with OAuth implementation. We would also like to thank Michael Royer and Andrea Wilkerson for comments on earlier drafts of this article.
Aarts, M. P. J., van Duijnhoven, J., Aries, M. B. C., and Rosemann, A. L. P. (2017). Performance of Personally Worn Dosimeters to Study Non-image Forming Effects of Light: Assessment Methods. Building Environ. 117, 60–72. doi:10.1016/j.buildenv.2017.03.002
Adamsson, M., Laike, T., and Morita, T. (2019). Comparison of Static and Ambulatory Measurements of Illuminance and Spectral Composition that Can Be Used for Assessing Light Exposure in Real Working Environments. LEUKOS 15, 181–194. doi:10.1080/15502724.2017.1391101
Åkerstedt, T., and Gillberg, M. (1990). Subjective and Objective Sleepiness in the Active Individual. Int. J. Neurosci. 52, 29–37. doi:10.3109/00207459008994241
Akimoto, M., Sekhar, C., Bivolarova, M. P., Liao, C., Fan, X., Laverge, J., et al. (2021). Reviewing How Bedroom Ventilation Affects IAQ and Sleep Quality. ASHRAE J., 56–60.
Aletta, F., Oberman, T., and Kang, J. (2018). Associations between Positive Health-Related Effects and Soundscapes Perceptual Constructs: A Systematic Review. Ijerph 15, 2392. doi:10.3390/ijerph15112392
Allen, J. G., MacNaughton, P., Laurent, J. G. C., Flanigan, S. S., Eitland, E. S., and Spengler, J. D. (2015). Green Buildings and Health. Curr. Envir Health Rpt 2, 250–258. doi:10.1007/s40572-015-0063-y
Allen, J. G., MacNaughton, P., Satish, U., Santanam, S., Vallarino, J., and Spengler, J. D. (2016). Associations of Cognitive Function Scores with Carbon Dioxide, Ventilation, and Volatile Organic Compound Exposures in Office Workers: A Controlled Exposure Study of green and Conventional Office Environments. Environ. Health Perspect. 124, 805–812. doi:10.1289/ehp.1510037
Altomonte, S., Allen, J., Bluyssen, P. M., Brager, G., Heschong, L., Loder, A., et al. (2020). Ten Questions Concerning Well-Being in the Built Environment. Building Environ. 180, 106949. doi:10.1016/j.buildenv.2020.106949
Altomonte, S., and Schiavon, S. (2013). Occupant Satisfaction in LEED and Non-LEED Certified Buildings. Building Environ. 68, 66–76. doi:10.1016/j.buildenv.2013.06.008
Apte, M. G., Fisk, W. J., and Daisey, J. M. (2000). Associations between Indoor CO2 Concentrations and Sick Building Syndrome Symptoms in U.S. Office Buildings: An Analysis of the 1994-1996 BASE Study Data. Indoor Air 10, 246–257. doi:10.1034/j.1600-0668.2000.010004246.x
Arsintescu, L., Kato, K. H., Cravalho, P. F., Feick, N. H., Stone, L. S., and Flynn-Evans, E. E. (2019). Validation of a Touchscreen Psychomotor Vigilance Task. Accid. Anal. Prev. 126, 173–176. doi:10.1016/j.aap.2017.11.041
Arundel, A. V., Sterling, E. M., Biggin, J. H., and Sterling, T. D. (1986). Indirect Health Effects of Relative Humidity in Indoor Environments. Environ. Health Perspect. 65, 351–361. doi:10.1289/ehp.8665351
Aziz, A., Loftness, V., Park, J.-H., Cochran, E., Harmon, R., Vo, D., et al. (2010). National Environmental Assessment Toolkit. Available at: http://neat-cbpd.arc.cmu.edu/neat/manual/NEAT-Manual.pdf.
Azuma, K., Kagi, N., Yanagi, U., and Osawa, H. (2018). Effects of Low-Level Inhalation Exposure to Carbon Dioxide in Indoor Environments: A Short Review on Human Health and Psychomotor Performance. Environ. Int. 121, 51–56. doi:10.1016/j.envint.2018.08.059
Bjørnstad, S., Patil, G. G., and Raanaas, R. K. (2015). Nature Contact and Organizational Support during Office Working Hours: Benefits Relating to Stress Reduction, Subjective Health Complaints, and Sick Leave. Wor 53, 9–20. doi:10.3233/WOR-152211
Bluyssen, P. M., Janssen, S., van den Brink, L. H., and de Kluizenaar, Y. (2011). Assessment of Wellbeing in an Indoor Office Environment. Building Environ. 46, 2632–2640. doi:10.1016/j.buildenv.2011.06.026
Bluyssen, P. M., Roda, C., Mandin, C., Fossati, S., Carrer, P., de Kluizenaar, Y., et al. (2016). Self-reported Health and comfort in 'modern' Office Buildings: First Results from the European OFFICAIR Study. Indoor Air 26, 298–317. doi:10.1111/ina.12196
Bluyssen, P. M. (2013). The Healthy Indoor Environment: How to Assess Occupants’ Wellbeing in Buildings. doi:10.4324/9781315887296
Bluyssen, P. M. (2020). Towards an Integrated Analysis of the Indoor Environmental Factors and its Effects on Occupants. Intell. Buildings Int. 12, 199–207. doi:10.1080/17508975.2019.1599318
Boubekri, M., Cheung, I. N., Reid, K. J., Wang, C.-H., and Zee, P. C. (2014). Impact of Windows and Daylight Exposure on Overall Health and Sleep Quality of Office Workers: A Case-Control Pilot Study. J. Clin. Sleep Med. 10, 603–611. doi:10.5664/jcsm.3780
Boubekri, M., Lee, J., Macnaughton, P., Woo, M., Schuyler, L., Tinianov, B., et al. (2020). The Impact of Optimized Daylight and Views on the Sleep Duration and Cognitive Performance of Office Workers. Ijerph 17, 3219. doi:10.3390/ijerph17093219
Burke, L. E., Shiffman, S., Music, E., Styn, M. A., Kriska, A., Smailagic, A., et al. (2017). Ecological Momentary Assessment in Behavioral Research: Addressing Technological and Human Participant Challenges. J. Med. Internet Res. 19, e77. doi:10.2196/jmir.7138
Caddick, Z. A., Gregory, K., Arsintescu, L., and Flynn-Evans, E. E. (2018). A Review of the Environmental Parameters Necessary for an Optimal Sleep Environment. Building Environ. 132, 11–20. doi:10.1016/j.buildenv.2018.01.020
Cain, S. W., McGlashan, E. M., Vidafar, P., Mustafovska, J., Curran, S. P. N., Wang, X., et al. (2020). Evening home Lighting Adversely Impacts the Circadian System and Sleep. Sci. Rep. 10, 19110. doi:10.1038/s41598-020-75622-4
Castaldo, V. L., Pigliautile, I., Rosso, F., Cotana, F., De Giorgio, F., and Pisello, A. L. (2018). How Subjective and Non-physical Parameters Affect Occupants' Environmental comfort Perception. Energy and Buildings 178, 107–129. doi:10.1016/j.enbuild.2018.08.020
Cedeño Laurent, J. G., MacNaughton, P., Jones, E., Young, A. S., Bliss, M., Flanigan, S., et al. (2021). Associations between Acute Exposures to PM2.5 and Carbon Dioxide Indoors and Cognitive Function in Office Workers: a Multicountry Longitudinal Prospective Observational Study. Environ. Res. Lett. 16, 094047. doi:10.1088/1748-9326/ac1bd8
Chiang, C. M., Chou, P. C., Lai, C. M., and Li, Y. Y. (2001). A Methodology to Assess the Indoor Environment in Care Centers for Senior Citizens. Building Environ. 36, 561–568. doi:10.1016/S0360-1323(00)00024-X
Chinoy, E. D., Cuellar, J. A., Huwa, K. E., Jameson, J. T., Watson, C. H., Bessman, S. C., et al. (2021). Performance Of Seven Consumer Sleep-Tracking Devices Compared With Polysomnography. Sleep 44. doi:10.1093/sleep/zsaa291
Choi, J.-H., and Lee, K. (2018). Investigation of the Feasibility of POE Methodology for a Modern Commercial Office Building. Building Environ. 143, 591–604. doi:10.1016/j.buildenv.2018.07.049
Choi, J.-H., Loftness, V., and Aziz, A. (2012a). Post-occupancy Evaluation of 20 Office Buildings as Basis for Future IEQ Standards and Guidelines. Energy and Buildings 46, 167–175. doi:10.1016/j.enbuild.2011.08.009
Choi, J.-H., Loftness, V., and Lee, D.-W. (2012b). Investigation of the Possibility of the Use of Heart Rate as a Human Factor for thermal Sensation Models. Building Environ. 50, 165–175. doi:10.1016/j.buildenv.2011.10.009
Clements, N., Zhang, R., Jamrozik, A., Campanella, C., and Bauer, B. (2019). The Spatial and Temporal Variability of the Indoor Environmental Quality during Three Simulated Office Studies at a Living Lab. Buildings 9, 62. doi:10.3390/buildings9030062
Colenberg, S., Jylhä, T., and Arkesteijn, M. (2020). The Relationship between interior Office Space and Employee Health and Well-Being - a Literature Review. Building Res. Inf. 49, 352–366. doi:10.1080/09613218.2019.1710098
Colton, M. D., Macnaughton, P., Vallarino, J., Kane, J., Bennett-Fripp, M., Spengler, J. D., et al. (2014). Indoor Air Quality in green vs Conventional Multifamily Low-Income Housing. Environ. Sci. Technol. 48, 7833–7841. doi:10.1021/es501489u
Coulby, G., Clear, A., Jones, O., and Godfrey, A. (2020). A Scoping Review of Technological Approaches to Environmental Monitoring. Ijerph 17, 3995. doi:10.3390/ijerph17113995
Cox, C. (2005). Health Optimisation Protocol for Energy-Efficient Buildings. Available at: https://hope.epfl.ch/results/results-intro.htm.
de la Iglesia, H. O., Fernández-Duque, E., Golombek, D. A., Lanza, N., Duffy, J. F., Czeisler, C. A., et al. (2015). Access to Electric Light Is Associated with Shorter Sleep Duration in a Traditionally Hunter-Gatherer Community. J. Biol. Rhythms 30, 342–350. doi:10.1177/0748730415590702
de Vries, L. P., Baselmans, B. M. L., and Bartels, M. (2021). Smartphone-Based Ecological Momentary Assessment of Well-Being: A Systematic Review and Recommendations for Future Studies. J. Happiness Stud. 22, 2361–2408. doi:10.1007/s10902-020-00324-7
de Zambotti, M., Cellini, N., Goldstone, A., Colrain, I. M., and Baker, F. C. (2019). Wearable Sleep Technology in Clinical and Research Settings. Med. Sci. Sports Exerc. 51, 1538–1557. doi:10.1249/MSS.0000000000001947
Du, B., Tandoc, M. C., Mack, M. L., and Siegel, J. A. (2020). Indoor CO 2 Concentrations and Cognitive Function: A Critical Review. Indoor Air 30, 1067–1082. doi:10.1111/ina.12706
Elzeyadi, I., Gatland, S., and Abboushi, B. (2017). “Dynamic Daylight: Measuring the Impacts of a High Performance Daylighting System on Occupants Health, Satisfaction, and Productivity,” in IES Annual Conference 2017 (Portland.
Erdmann, C. A., Steiner, K. C., and Apte, M. G. (2002). “Indoor Carbon Dioxide Concentrations and Sick Building Syndrome Symptoms in the Base Study Revisited: Analyses of the 100 Building Dataset,” in Indoor Air 2002: The 9th International Conference on Indoor Air Quality and Climate, Monterey, CA, United States.
Fang, L., Wyon, D. P., Clausen, G., and Fanger, P. O. (2004). Impact of Indoor Air Temperature and Humidity in an Office on Perceived Air Quality, SBS Symptoms and Performance. Indoor Air 14, 74–81. doi:10.1111/j.1600-0668.2004.00276.x
Feldmeier, M., and Paradiso, J. A. (2010). Personalized HVAC Control System. Internet of Things (IOT) 2010, 1–8. doi:10.1109/IOT.2010.5678444
Figueiro, M., Hamner, R., Bierman, A., and Rea, M. (2013). Comparisons of Three Practical Field Devices Used to Measure Personal Light Exposures and Activity Levels. Lighting Res. Tech. 45, 421–434. doi:10.1177/1477153512450453
Figueiro, M., Steverson, B., Heerwagen, J., Yucel, R., Roohan, C., Sahin, L., et al. (2019). Light, Entrainment and Alertness: A Case Study in Offices. Lighting Res. Tech. 52, 736–750. doi:10.1177/1477153519885157
Fisk, W. J. (2019). Do Indoor CO2 Levels Directly Affect Perceived Air Quality, Health, or Work Performance? ASHRAE J.
Fitbit (2021). Fitbit Reference. Available at: https://dev.fitbit.com/build/reference/(Accessed November 12, 2021).
Garfinkel, S. (2015). De-identification of Personal Information Gaithersburg, MD, United States: National Institute of Standards and Technology.
Ghahramani, A., Pantelic, J., Lindberg, C., Mehl, M., Srinivasan, K., Gilligan, B., et al. (2018). Learning Occupants' Workplace Interactions from Wearable and Stationary Ambient Sensing Systems. Appl. Energ. 230, 42–51. doi:10.1016/j.apenergy.2018.08.096
Graham, L. t., Parkinson, T., and Schiavon, S. (2021). Lessons Learned from 20 Years of CBE's Occupant Surveys. Build. Cities 2, 166–184. doi:10.5334/bc.76
Heinzerling, D., Schiavon, S., Webster, T., and Arens, E. (2013). Indoor Environmental Quality Assessment Models: A Literature Review and a Proposed Weighting and Classification Scheme. Building Environ. 70, 210–222. doi:10.1016/j.buildenv.2013.08.027
Intille, S., Haynes, C., Maniar, D., Ponnada, A., and Manjourides, J. (20162016). μEMA. Proc. ACM Int. Conf. Ubiquitous Comput. Ubicomp, 1124–1128. doi:10.1145/2971648.2971717
Jahncke, H., Hygge, S., Halin, N., Green, A. M., and Dimberg, K. (2011). Open-plan Office Noise: Cognitive Performance and Restoration. J. Environ. Psychol. 31, 373–382. doi:10.1016/j.jenvp.2011.07.002
Jamrozik, A., Clements, N., Hasan, S. S., Zhao, J., Zhang, R., Campanella, C., et al. (2019). Access to Daylight and View in an Office Improves Cognitive Performance and Satisfaction and Reduces Eyestrain: A Controlled Crossover Study. Building Environ. 165, 106379. doi:10.1016/j.buildenv.2019.106379
Jamrozik, A., Ramos, C., Zhao, J., Bernau, J., Clements, N., Vetting Wolf, T., et al. (2018). A Novel Methodology to Realistically Monitor Office Occupant Reactions and Environmental Conditions Using a Living Lab. Building Environ. 130, 190–199. doi:10.1016/j.buildenv.2017.12.024
Jayathissa, P., Quintana, M., Abdelrahman, M., and Miller, C. (2020a). Humans-as-a-Sensor for Buildings-Intensive Longitudinal Indoor Comfort Models. Buildings 10, 174. doi:10.3390/buildings10100174
Jayathissa, P., Quintana, M., Sood, T., Nazarian, N., and Miller, C. (2019). Is Your Clock-Face Cozie? A Smartwatch Methodology for the In-Situ Collection of Occupant comfort Data. J. Phys. Conf. Ser. 1343, 012145. doi:10.1088/1742-6596/1343/1/012145
Jayathissa, P., Quintana, M., Sood, T., Nazarian, N., and Miller, C. (2020b). Project Cozie. Available at: https://github.com/cozie-app/cozie.
Jin, M., Liu, S., Schiavon, S., and Spanos, C. (2018). Automated mobile Sensing: Towards High-Granularity Agile Indoor Environmental Quality Monitoring. Building Environ. 127, 268–276. doi:10.1016/j.buildenv.2017.11.003
Kallio, J., Vildjiounaite, E., Koivusaari, J., Räsänen, P., Similä, H., Kyllönen, V., et al. (2020). Assessment of Perceived Indoor Environmental Quality, Stress and Productivity Based on Environmental Sensor Data and Personality Categorization. Building Environ. 175, 106787. doi:10.1016/j.buildenv.2020.106787
Kaplan, S. (1995). The Restorative Benefits of Nature: Toward an Integrative Framework. J. Environ. Psychol. 15, 169–182. doi:10.1016/0272-4944(95)90001-2
Kjellberg, A., and Landström, U. (1994). Noise in the Office: Part II - the Scientific Basis (Knowledge Base) for the Guide. Int. J. Ind. Ergon. 14, 93–118. doi:10.1016/0169-8141(94)90008-6
Konis, K., and Annavaram, M. (2017). The Occupant Mobile Gateway: A Participatory Sensing and Machine-Learning Approach for Occupant-Aware Energy Management. Building Environ. 118, 1–13. doi:10.1016/j.buildenv.2017.03.025
Koo, B. M., and Vizer, L. M. (2019). Mobile Technology for Cognitive Assessment of Older Adults: A Scoping Review. Innov. Aging 3, igy038. doi:10.1093/geroni/igy038
Kushida, C. A., Nichols, D. A., Jadrnicek, R., Miller, R., Walsh, J. K., and Griffin, K. (2012). Strategies for De-identification and Anonymization of Electronic Health Record Data for Use in Multicenter Research Studies. Med. Care 50 (Suppl. l), S82–S101. doi:10.1097/MLR.0b013e3182585355
Li, D., Menassa, C. C., and Kamat, V. R. (2017). Personalized Human comfort in Indoor Building Environments under Diverse Conditioning Modes. Building Environ. 126, 304–317. doi:10.1016/j.buildenv.2017.10.004
Lindberg, C. M., Srinivasan, K., Gilligan, B., Razjouyan, J., Lee, H., Najafi, B., et al. (2018). Effects of Office Workstation Type on Physical Activity and Stress. Occup. Environ. Med. 75, 689–695. doi:10.1136/oemed-2018-105077
Liu, B., Zhou, W., Zhu, T., Gao, L., and Xiang, Y. (2018). Location Privacy and its Applications: A Systematic Study. IEEE Access 6, 17606–17624. doi:10.1109/ACCESS.2018.2822260
MacNaughton, P., Spengler, J., Vallarino, J., Santanam, S., Satish, U., and Allen, J. (2016). Environmental Perceptions and Health before and after Relocation to a green Building. Building Environ. 104, 138–144. doi:10.1016/j.buildenv.2016.05.011
Matsangas, P., Shattuck, N. L., and Brown, S. (2017). Preliminary Validation Study of the 3-min Wrist-Worn Psychomotor Vigilance Test. Behav. Res. 49, 1792–1801. doi:10.3758/s13428-016-0821-2
Medvedev, O., Shepherd, D., and Hautus, M. J. (2015). The Restorative Potential of Soundscapes: A Physiological Investigation. Appl. Acoust. 96, 20–26. doi:10.1016/j.apacoust.2015.03.004
Menzies, D., Popa, J., Hanley, J. A., Rand, T., and Milton, D. K. (2003). Effect of Ultraviolet Germicidal Lights Installed in Office Ventilation Systems on Workers' Health and Wellbeing: Double-Blind Multiple Crossover Trial. The Lancet 362, 1785–1791. doi:10.1016/S0140-6736(03)14897-0
Mitchell, C. S., Zhang, J., Sigsgaard, T., Jantunen, M., Lioy, P. J., Samson, R., et al. (2007). Current State of the Science: Health Effects and Indoor Environmental Quality. Environ. Health Perspect. 115, 958–964. doi:10.1289/ehp.8987
Moore, R. C., Swendsen, J., and Depp, C. A. (2017). Applications for Self-Administered mobile Cognitive Assessments in Clinical Research: A Systematic Review. Int. J. Methods Psychiatr. Res. 26, e1562. doi:10.1002/mpr.1562
Motti, V. G., and Caine, K. (2015). “Users' Privacy Concerns about Wearables,” in Financial Cryptography and Data Security. Editors M. Brenner, N. Christin, B. Johnson, and K. Rohloff (Berlin, Heidelberg: Springer Berlin Heidelberg), 231–244. doi:10.1007/978-3-662-48051-9_17
Mundnich, K., Booth, B. M., L’Hommedieu, M., Feng, T., Girault, B., L’Hommedieu, J., et al. (2020). TILES-2018, a Longitudinal Physiologic and Behavioral Data Set of Hospital Workers. Sci. Data 7, 354. doi:10.1038/s41597-020-00655-3
Orlosky, J., Ezenwoye, O., Yates, H., and Besenyi, G. (2019). “A Look at the Security and Privacy of Fitbit as a Health Activity Tracker,” in Proceedings of the 2019 ACM Southeast Conference ACM SE ’19 (New York, NY, USA: Association for Computing Machinery), 241–244. doi:10.1145/3299815.3314468
Pan, L., Lian, Z., and Lan, L. (2012). Investigation of Sleep Quality under Different Temperatures Based on Subjective and Physiological Measurements. Hvac\\&r Res. 18, 1030–1043. doi:10.1080/10789669.2012.667037
Park, J., Loftness, V., Aziz, A., and Wang, T.-H. (2019). Critical Factors and Thresholds for User Satisfaction on Air Quality in Office Environments. Building Environ. 164, 106310. doi:10.1016/j.buildenv.2019.106310
Parkinson, T., Parkinson, A., and de Dear, R. (2019). Continuous IEQ Monitoring System: Context and Development. Building Environ. 149, 15–25. doi:10.1016/j.buildenv.2018.12.010
Paul, G., and Irvine, J. (2014). Privacy Implications of Wearable Health Devices, in Proceedings of the 7th International Conference on Security of Information and Networks-SIN’14 New York, NY, United States. ACM Press, 117–121. doi:10.1145/2659651.2659683
Peeters, S. T., Smolders, K. C. H. J., and de Kort, Y. A. W. (2020). What You Set Is (Not) what You Get: How a Light Intervention in the Field Translates to Personal Light Exposure. Building Environ. 185, 107288. doi:10.1016/j.buildenv.2020.107288
Peeters, S. T., Smolders, K. C. H. J., Vogels, I. M. L. C., and de Kort, Y. A. W. (2021). Less Is More? Effects of More vs. Less Electric Light on Alertness, Mood, Sleep and Appraisals of Light in an Operational Office. J. Environ. Psychol. 74, 101583. doi:10.1016/j.jenvp.2021.101583
Pigliautile, I., Casaccia, S., Morresi, N., Arnesano, M., Pisello, A. L., and Revel, G. M. (2020). Assessing Occupants' Personal Attributes in Relation to Human Perception of Environmental comfort: Measurement Procedure and Data Analysis. Building Environ. 177, 106901. doi:10.1016/j.buildenv.2020.106901
Piwek, L., Ellis, D. A., Andrews, S., and Joinson, A. (2016). The Rise of Consumer Health Wearables: Promises and Barriers. Plos Med. 13, e1001953–9. doi:10.1371/journal.pmed.1001953
Razjouyan, J., Lee, H., Gilligan, B., Lindberg, C., Nguyen, H., Canada, K., et al. (2020). Wellbuilt for Wellbeing: Controlling Relative Humidity in the Workplace Matters for Our Health. Indoor Air 30, 167–179. doi:10.1111/ina.12618
Ren, W., Tong, X., Du, J., Wang, N., Li, S., Min, G., et al. (2021). Privacy Enhancing Techniques in the Internet of Things Using Data Anonymisation. Inf. Syst. Front.. doi:10.1007/s10796-021-10116-w
Ru, T., de Kort, Y. A. W., Smolders, K. C. H. J., Chen, Q., and Zhou, G. (2019). Non-image Forming Effects of Illuminance and Correlated Color Temperature of Office Light on Alertness, Mood, and Performance across Cognitive Domains. Building Environ. 149, 253–263. doi:10.1016/j.buildenv.2018.12.002
Safavi, S., and Shukur, Z. (2014). Conceptual Privacy Framework for Health Information on Wearable Device. PLoS One 9, e114306. doi:10.1371/journal.pone.0114306
Sakellaris, I., Saraga, D., Mandin, C., Kluizenaar, Y., Fossati, S., Spinazzè, A., et al. (2020). Association of Subjective Health Symptoms with Indoor Air Quality in European Office Buildings: The OFFICAIR Project. Indoor Air 31, 426–439. 14. doi:10.1111/ina.12749
Salamone, F., Masullo, M., and Sibilio, S. (2021). Wearable Devices for Environmental Monitoring in the Built Environment: A Systematic Review. Sensors 21, 4727. doi:10.3390/s21144727
Sanguinetti, A., Pritoni, M., Salmon, K., and Morejohn, J. (2016). TherMOOstat : Occupant Feedback to Improve Comfort and Efficiency on a University CampusACEEE Summer Study 2016.
Satish, U., Mendell, M. J., Shekhar, K., Hotchi, T., Sullivan, D., Streufert, S., et al. (2012). Is CO2an Indoor Pollutant? Direct Effects of Low-To-Moderate CO2Concentrations on Human Decision-Making Performance. Environ. Health Perspect. 120, 1671–1677. doi:10.1289/ehp.1104789
Seddigh, A., Berntson, E., Jönsson, F., Danielson, C. B., and Westerlund, H. (2015). The Effect of Noise Absorption Variation in Open-Plan Offices: A Field Study with a Cross-Over Design. J. Environ. Psychol. 44, 34–44. doi:10.1016/j.jenvp.2015.08.004
Seppänen, O., and Fisk, W. (2006). Some Quantitative Relations between Indoor Environmental Quality and Work Performance or Health. Hvac&r Res. 12, 957–973. doi:10.1080/10789669.2006.10391446
Shafiee Motlagh, M., Golmohammadi, R., Aliabadi, M., Faradmal, J., and Ranjbar, A. (2018). Empirical Study of Room Acoustic Conditions and Neurophysiologic Strain in Staff Working in Special Open-Plan Bank Offices. Acoust. Aust. 46, 329–338. doi:10.1007/s40857-018-0143-x
Smith, L. M., Wang, L., Mazur, K., Carchia, M., DePalma, G., Azimi, R., et al. (2020). Impacts of COVID-19-Related Social Distancing Measures on Personal Environmental Sound Exposures. Environ. Res. Lett. 15, 104094. doi:10.1088/1748-9326/abb494
Smith-Jackson, T. L., and Klein, K. W. (2009). Open-plan Offices: Task Performance and Mental Workload. J. Environ. Psychol. 29, 279–289. doi:10.1016/j.jenvp.2008.09.002
Taub, M., Lockhart, V., and Clements-Croome, D. (2016). WEARABLES IN THE WORKPLACE. London. Available at: https://www.bco.org.uk/Research/Publications/Wearables_in_the_Workplace.aspx.
Tham, K. W., and Willem, H. C. (2010). Room Air Temperature Affects Occupants' Physiology, Perceptions and Mental Alertness. Building Environ. 45, 40–44. doi:10.1016/j.buildenv.2009.04.002
Thayer, J. F., Verkuil, B., Brosschotj, J. F., Kevin, K., West, A., Sterling, C., et al. (2010). Effects of the Physical Work Environment on Physiological Measures of Stress. Eur. J. Cardiovasc. Prev. Rehabil. 17, 431–439. doi:10.1097/HJR.0b013e328336923a
USGBC (2021). LEED Credit Library. Available at: https://www.usgbc.org/credits.
van Bommel, W. J. M. (2006). Non-visual Biological Effect of Lighting and the Practical Meaning for Lighting for Work. Appl. Ergon. 37, 461–466. doi:10.1016/j.apergo.2006.04.009
van Bommel, W. (2005). Visual, Biological and Emotional Aspects of Lighting: Recent New Findings and Their Meaning for Lighting Practice. Leukos 2, 7–11. doi:10.1582/LEUKOS.02.01.001
Veitch, J. A. (2001). Lighting Quality Contributions from Biopsychological Processes. J. Illuminating Eng. Soc. 30, 3–16. doi:10.1080/00994480.2001.10748329
Veitch, J., Newsham, G., Boyce, P., and Jones, C. (2008). Lighting Appraisal, Well-Being and Performance in Open-Plan Offices: A Linked Mechanisms Approach. Lighting Res. Tech. 40, 133–151. doi:10.1177/1477153507086279
Vetter, C., Pattison, P. M., Houser, K., Herf, M., Phillips, A. J. K., Wright, K. P., et al. (2021). A Review of Human Physiological Responses to Light: Implications for the Development of Integrative Lighting Solutions. Leukos, 1–28. doi:10.1080/15502724.2021.1872383
Wams, E. J., Woelders, T., Marring, I., van Rosmalen, L., Beersma, D. G. M., Gordijn, M. C. M., et al. (2017). Linking Light Exposure and Subsequent Sleep: A Field Polysomnography Study in Humans. Sleep 40. doi:10.1093/sleep/zsx165
Wei, M., Houser, K. W., Orland, B., Lang, D. H., Ram, N., Sliwinski, M. J., et al. (2014). Field Study of Office Worker Responses to Fluorescent Lighting of Different CCT and Lumen Output. J. Environ. Psychol. 39, 62–76. doi:10.1016/j.jenvp.2014.04.009
Wolkoff, P. (2017). External Eye Symptoms in Indoor Environments. Indoor Air 27, 246–260. doi:10.1111/ina.12322
Woolley, S. I., Collins, T., Mitchell, J., and Fredericks, D. (2019). Investigation of Wearable Health Tracker Version Updates. BMJ Heal. Care Informat. 26. doi:10.1136/bmjhci-2019-100083
Zhang, R., Campanella, C., Aristizabal, S., Jamrozik, A., Zhao, J., Porter, P., et al. (2020). Impacts of Dynamic Led Lighting on the Well-Being and Experience of Office Occupants. Ijerph 17, 7217–7227. doi:10.3390/ijerph17197217
API application programming interface
FTP file transfer protocol
GPS global positioning system
HPIs health performance indicators
IEQ indoor environmental quality
EMAs ecological momentary assessments
JSON javascript object notation
LEED leadership in energy and environmental design
PM particulate matter
SBS sick building symptoms
VOCs volatile organic compounds
Keywords: wearables, indoor environmental quality, occupant well-being, health performance indicators, data accessibility
Citation: Abboushi B, Safranek S, Rodriguez-Feo Bermudez E, Pratoomratana S, Chen Y, Poplawski M and Davis R (2022) A Review of the Use of Wearables in Indoor Environmental Quality Studies and an Evaluation of Data Accessibility from a Wearable Device. Front. Built Environ. 8:787289. doi: 10.3389/fbuil.2022.787289
Received: 30 September 2021; Accepted: 01 February 2022;
Published: 18 February 2022.
Edited by:
Francesco Salamone, National Research Council of Italy, ItalyReviewed by:
Michele Rocca, University of Pisa, ItalyCopyright © 2022 Abboushi, Safranek, Rodriguez-Feo Bermudez, Pratoomratana, Chen, Poplawski and Davis. This is an open-access article distributed under the terms of the Creative Commons Attribution License (CC BY). The use, distribution or reproduction in other forums is permitted, provided the original author(s) and the copyright owner(s) are credited and that the original publication in this journal is cited, in accordance with accepted academic practice. No use, distribution or reproduction is permitted which does not comply with these terms.
*Correspondence: Belal Abboushi, QmVsYWwuYWJib3VzaGlAZ21haWwuY29t
Disclaimer: All claims expressed in this article are solely those of the authors and do not necessarily represent those of their affiliated organizations, or those of the publisher, the editors and the reviewers. Any product that may be evaluated in this article or claim that may be made by its manufacturer is not guaranteed or endorsed by the publisher.
Research integrity at Frontiers
Learn more about the work of our research integrity team to safeguard the quality of each article we publish.