- Department of Civil, Environmental, and Ocean Engineering, Stevens Institute of Technology, Hoboken, NJ, United States
Coastal areas of State of New Jersey in the Northeastern United States are exposed to extreme wind waves generated by tropical cyclones in the Atlantic Ocean. Past studies suggest that the frequency and intensity of major hurricanes in the Atlantic basin would increase under high greenhouse gas emission scenarios. Furthermore, sea level observations have revealed that the local mean sea level along the coast of New Jersey is rising at a rate higher than that of the global sea level rise. The objective of this study is to quantify the combined influence of sea level rise (SLR) and hurricane climatology change on wave heights induced by major hurricanes off the coast of New Jersey. To this end, a coupled hydrodynamic-wave model is utilized to simulate wind waves for synthetic hurricanes generated for the climate conditions in the historical period of 1980–2000 and future period of 2080–2100 under the RCP8.5 high emission scenario. The synthetic storms are generated by a hurricane model for the climate conditions obtained from four different global climate models. The projections of future wave heights show statistically significant increases in the wave heights induced by major hurricanes. Under the combined effects of hurricane climatology change and a SLR of 1.19 m, the increase in the extreme wave heights 15% in back-bays and shallow waters of the nearshore zone and up to 10% in deeper coastal waters. It is found that SLR alone would result in a significant increase in the hurricane-induced wave heights in the present-day surf zone.
Introduction
Tropical cyclones (TCs) are among the costliest and most destructive natural disasters worldwide (Smith and Katz, 2013). In addition to strong winds, heavy rainfall, and high storm surges, TCs generate destructive surface waves, which pose a significant threat to infrastructure, navigation, and communities. For example, the failure of a highway bridge on the Gulf of Mexico Coast during Hurricane Katrina in 2005 was caused by wave attack that accompanied by high water levels (Chen et al., 2009). In the absence of large storm surges, waves generated by offshore TCs still can cause dangerous surf conditions, rip currents, and severe coastal erosion as was observed along the U.S. East Coast during Hurricane Felix in 1995 (Lawrence et al., 1997).
Future TC-induced wave hazards could be influenced by climate change, as a result of sea level rise (SLR) and TC climatology change. SLR will increase the mean sea level, which will result in deeper water depths in the present-day surf zone. A deeper nearshore zone will, in turn, allow larger waves to reach the shoreline, potentially resulting in more damage. The sixth assessment report from the Intergovernmental Panel on Climate Change projects that the global mean sea level by the end of 21st century, under the high CO2 emissions scenario, will increase 0.63–1.02 m (likely range; at least a 66% chance) relative to the 1995–2014 (IPCC et al., 2021). The local relative SLR would vary regionally and differ from the global mean SLR (Stammer et al., 2013; Kopp et al., 2014). Under a high-emission scenario, projections of the relative sea level rise in New Jersey suggest an increase of 0.7–1.9 m (likely range) above the baseline mean sea level (1991–2009 average) by the end of 21st-century (Kopp et al., 2019). In addition to SLR, changes in the intensity of storms would influence wave hazards associated with TCs.
High-resolution modeling studies have projected an increase in the intensity of future TCs (e.g., Emanuel et al., 2008; Hill and Lackmann, 2011; Knutson et al., 2013; Gutmann et al., 2018). Knutson et al. (2020) compiled findings of past studies on TC climatology and found that most studies have projected, at least with medium-to-high confidence, an increase in the global average TC intensity. For example, using 10-member ensembles of nine different hurricanes, Patricola and Wehner (2018) showed that the intensity of hurricanes in the Atlantic basin would increase about 10 percent by the end of 21st-century under RCP 8.5 greenhouse gas concentration trajectory. While global warming would increase the intensity of TCs, it would also affect the TC frequency in various ocean basins.
Multiple studies have further projected that while the overall TC frequency would decrease in the future warmer climate, the frequency of intense TCs in most ocean basins would increase (e.g., Knutson et al., 2015; Walsh et al., 2016; Sugi et al., 2017). For instance, using an ensemble of 40 high-resolution global model experiments, Sugi et al. (2017) found an average increase of about 4 and 12%, respectively, in the frequency of very intense, and major hurricanes in the North Atlantic basin. Very intense and major TCs represent, respectively, category four to five and three to five hurricanes based on the Saffir–Simpson hurricane wind scale. Intense and major TCs are of great scientific and societal interest, given that they have historically caused significant economic losses. Based on the normalized economic damage of mainland U.S. hurricanes from 1900 to 2005, Pielke et al. (2008) showed that about 85% of the total damage is accounted for by the major hurricanes (Category 3+). Similarly, Weinkle et al. (2018) found that among continental U.S. hurricanes during the period of 1900–2017, major hurricanes account for more than 80% of total damage. Increasing frequency of intense hurricanes, together with SLR, could result in changes in wave hazards in coastal waters.
The combined effect of SLR and TC climatology change on the flood level at the coast has received attention since the past decade (e.g., Lin et al., 2016; Marsooli et al., 2019; Marsooli and Lin 2020). The effect of SLR and storm climatology on wind wave climate has also gained attention in the recent years. For instance, Shimura et al. (2017) analyzed wave climate projections from Atmosphere-Ocean global climate models to investigate the effects of climate change on TC-induced wind waves in the Western North Pacific. Wanders et al. (2017) investigated the wind wave climate in southwestern Australian coastal zone for different warmer climate scenarios. They found that the effect of SLR on the wave climate in their study area is substantial.
The combined effect of SLR and storm climatology change on TC-induced wave hazards in most coastal areas exposed to TCs is yet to be understood. It is of great interest to gain a quantitative understanding of changes in the future extreme wave hazards in both the offshore zone, which supports navigation and design and development of offshore structures (e.g., wind farms), and the nearshore zone, which informs safe recreational activities, coastal erosion studies, coastal development, among others.
To this end, the objective of the present study is to quantify the combined effects of sea level rise and future changes in the climatology of major hurricanes on the extreme waves in coastal waters of the State of New Jersey, located in the Northeastern United States. Long stretches of coastal areas in New Jersey are open coastlines facing the Atlantic Ocean. These coastal areas are exposed to destructive wind waves generated by local and offshore storms. Waves generated by TCs have historically caused significant damage to structures, coastal erosion, and flooding, e.g., during Hurricanes Donna in 1960, Gloria in 1985, Irene 2011, and Sandy 2012. Hurricane Irene in 2011, which made landfall near Atlantic City, New Jersey, generated large waves that contributed directly to major coastal erosion, and indirectly to landward flooding (Avila and Cangialosi 2011; Miller et al., 2012). Another example of a destructive TC event was Hurricane Sandy in 2012. Sandy made landfall near Brigantine, New Jersey, with an estimated wind speed near 36 ms−1. Its large storm surge and waves resulted in the inundation and breaching of barrier islands. Entire sand dunes in some areas were washed out due to the wave forcing while others suffered extreme erosion (Bilinski, et al., 2015; RSCNJ, 2012).
The objective of this study is achieved by simulating hurricane-induced waves, using the coupled hydrodynamic-wave model ADCIRC-SWAN, for a large number of synthetic major hurricanes. Simulations for the future climate are carried out with and without sea level rise, allowing us to separate the effect of hurricane climatology change from that of sea level rise. In the following sections, the study area is introduced in section 2, followed by data and methods in section 3. Section 4 evaluates the accuracy of historical synthetic wave simulations and presents the projected changes in the wave height. The results are discussed in section 5 and conclusions are summarized in section 6.
Study Area
The study area covers the Atlantic coast of the State of New Jersey in the northeast of the United States (Figure 1). The open coast extends approximately 205 km in length, and includes Cape May, Atlantic, Ocean, and Monmouth Counties (Hapke et al., 2011). The open coasts of Cape May, Atlantic, and Ocean Counties are separated from the mainland through barrier islands and back-bays. The back-bays are shallow and contain a large extent of tidal wetlands that serve as a nourishing environment for fish, shellfish, and birds.
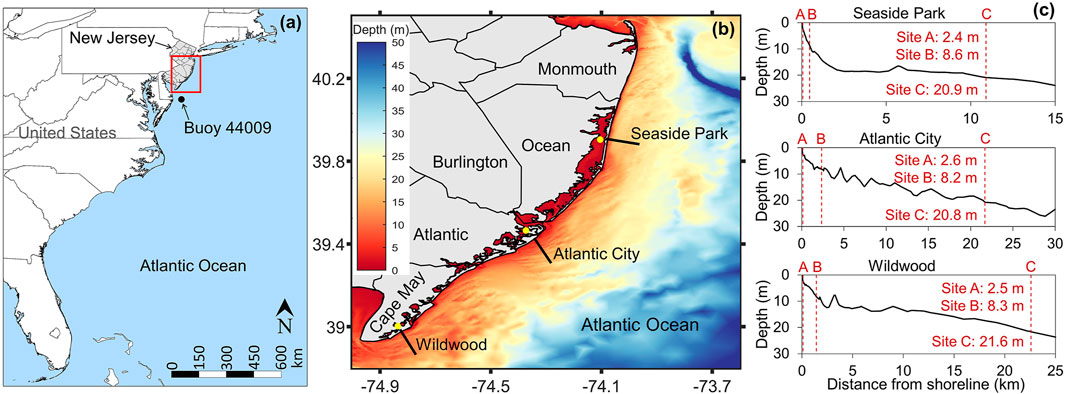
FIGURE 1. (A) Geographic location of the State of New Jersey in the Northeastern United States. (B) Bathymetry in coastal waters of the study area in New Jersey. The yellow circles show the location of three virtual sites in the back-bays. The black lines represent three cross-shore profiles named Seaside Park (normal to the coastline of Barnegat Bay Island, Ocean County), Atlantic City (Absecon Island, Atlantic County), and Wildwood (Five Mile Beach Island, Cape May). (C) Bathymetry along the three cross-shore profiles. Numbers represent the water depth at the location of virtual sites A, B, and C along the profiles.
Barrier islands in New Jersey extend 169 km in length and most of the islands are less than 1 km wide. The islands, which are primarily protected by beach-dune systems from storm surges and waves, are the main line of flood defense for New Jersey’s back-bays. The majority of New Jersey’s barrier islands are highly urbanized and populated. The islands are the second most densely populated barrier islands among states along the U.S. Atlantic and Gulf Coasts (Zhang and Leatherman 2011).
Sandy beach-dune systems are the predominant form of shoreline protection along the open coast of New Jersey. Dunes are usually confined to a narrow line between the berm and urban areas. They typically reach heights of between 2.4 and 4.8 m above the berm (Martínez and Psuty 2004), which is generally 2–3 m above mean sea level. Dunes are usually vegetated to slow the wind erosional effect. Most of the dunes are manmade, while some of them are natural with manmade maintenance and stabilization. Shoreline structures are also an important part of the New Jersey coastal protection system. Due to the continuous alongshore sediment transport, groins are the most common structures on the New Jersey shorelines (Baker, 2019).
The study area is exposed to storm surges and destructive waves generated by TCs in the Atlantic basin. Such TC events adversely affect the shorelines, causing infrastructure damage, and threatening the lives of coastal populations. For example, Hurricane Irene in 2011 produced a 1.2–1.8 m storm surge along the New Jersey coastline (Avila and Cangialosi 2011), and a maximum significant wave height of 6.36 m at a buoy located about 55 km southeast of Cape May County (buoy 44009 marked by a solid circle in Figure 1). The combined effect of storm surges and waves was moderate to severe tidal flooding with extensive beach erosion. An estimated nationwide total loss of $15.8 billion, and a statewide loss of $1 billion was attributed to TC Irene (Watson et al., 2013). Another extreme TC that affected New Jersey was Hurricane Sandy in 2012, with a peak significant wave height of 9.85 m at buoy 44025 located about 70 km east of Monmouth County. Sandy caused 37 deaths in New Jersey, and 125 deaths nationwide. Other statewide impacts included damage to 346,000 homes, the sinking of 1,400 vessels, impacts to 70 drinking water systems, and extreme erosion and dune washout, resulting in $30 billion damages statewide and $62 billion nationwide (Bilinski, et al., 2015).
Data and Methods
Hurricane Dataset
This study implements a dynamic wave model to simulate wave heights for synthetic major hurricanes generated for the climate conditions of a historical period in the late 20th century and a future period in the late 21st century. The synthetic hurricanes are based on the TC datasets from Marsooli and Lin (2020). Each dataset contains large numbers of TCs generated by the statistical/deterministic hurricane model of Emanuel et al. (2008) for the Atlantic basin. This hurricane model generates the synthetic TCs for a given large-scale atmospheric and oceanic environment that is estimated from observations or a climate model.
The observation-based historical TC dataset contains synthetic TCs that are generated for the observed climate of the 1980–2000 historical period based on the National Centers for Environmental Prediction (NCEP) reanalysis. The model-based historical and future TC datasets are, respectively, based on the modeled climates of the historical period of 1980–2000 and future period of 2080–2100, RCP 8.5 high emission scenario (Moss et al., 2008), from an ensemble of four different global climate models. The climate models include GFDL5 (Geophysical Fluid Dynamics Laboratory Climate Model, USA) (Donner et al., 2011); HadGEM5 (Hadley Centre Global Environment Model, United Kingdom. Meteorological Office) (Collins et al., 2011; Martin et al., 2011); MPI5 (Max–Planck–Institute for Meteorology, Germany) (Stevens et al., 2013); and MRI5 (Meteorological Research Institute, Japan) (Yukimoto et al., 2012).
From each dataset, we select and simulate major TCs, i.e., Category 3, 4, and 5 hurricanes. Only storms that pass within a 500 km radius from the southern tip of Cape May County, New Jersey (Figure 1 for the location of Cape May) are selected. The selected sub-datasets include 150 storms from the NCEP-based historical TC dataset; 399 storms from the ensemble model-based historical dataset (13 from GFDL, 267 from HadGEM, 56 from MPI, 63 from MRI); and 650 storms from the model-based future dataset (63 from GFDL, 397 from HadGEM, 107 from MPI, 83 from MRI) (Table 1). We compare the simulated significant wave heights (SWH) of the NCEP-based dataset with the simulated SWHs of the model-based historical dataset to evaluate the accuracy of the synthetic storms to reproduce the climate of hurricane wind waves in the study area (shown later in Figure 4).
Figure 2 shows the histograms of the characteristic parameters of the synthetic hurricanes when they are at their closest distance to the southern tip of Cape May County, New Jersey. The intensity of hurricanes, represented by the maximum wind speed, shows an increase from the historical period of 1980–2000 to the future period of 2080–2100. The histograms of the storm translation speed of the synthetic hurricanes show a decrease in the translation speed, implying that intense hurricanes would be slower under a warmer climate. There is also a projected decrease in the distance between the storm center and the southern tip of Cape May County, indicating that the intense hurricanes would pass within a closer distance to the study area. This is also observable in the histogram of the storm heading angle, which indicates a westward shift of the translational direction of storms when they are at their closest distance to Cape May.
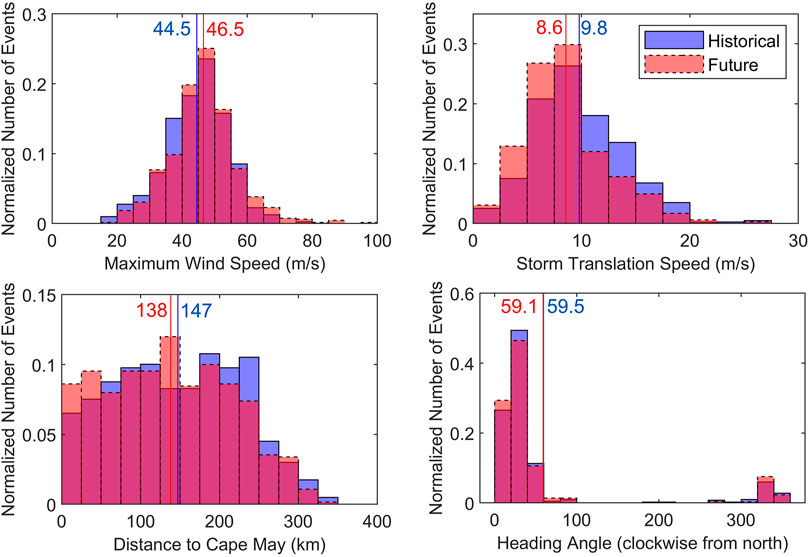
FIGURE 2. Characteristic parameters of the 399 historical and 650 future synthetic category three to five hurricanes that pass within 500 km of the southern tip of Cape May County, New Jersey when they are at their closest distance to this location. Vertical lines and their adjacent labels show the mean values (blue: historical period; red: future period).
The datasets used by Marsooli and Lin (2020) show that the annual frequencies of synthetic TCs in the historical and future periods are, respectively, 0.395 and 0.675 for the HadGEM dataset, 0.289 and 1.322 for the GFDL dataset, 0.399 and 0.638 for the MPI dataset, and 0.366 and 0.403 for the MRI dataset. We calculate the frequency of intense hurricanes in the selected sub-datasets based on the number of selected hurricanes in each sub-dataset and the total number of TCs in the datasets used by Marsooli and Lin (2020). This results in historical and future annual frequencies of intense hurricanes to be, respectively, 0.050 and 0.134 for the HadGEM sub-dataset, 0.002 and 0.040 for the GFDL sub-dataset, 0.011 and 0.033 for the MPI sub-dataset, and 0.011 and 0.016 for the MRI sub-dataset. Finally, an ensemble average frequency is calculated based on the number of storms in each dataset. The calculated ensemble average frequencies of intense hurricanes are 0.037 for the historical period and 0.093 for the future period, suggesting an increase by a factor of 2.5.
Sea Level Rise
The synthetic hurricanes in the model-based future TC dataset are simulated for two SLR scenarios: “No SLR” (in the absence of sea level rise) and “With-SLR” (in the presence of sea level rise). The same storms are simulated under these scenarios. These scenarios allow us to separate the effects of SLR on wave hazards from the effects of hurricane climatology change. Due to the high computational cost of wave modeling, only one value for SLR is considered. We use a SLR of 1.19 m, which is within the likely range of projected values for the state of New Jersey. This selected SLR scenario has a 50% chance to be equaled or exceeded by the end of 21st century under a high-emission climate scenario (Kopp et al., 2019).
Hydrodynamic-Wave Model
We utilize the coupled ADCIRC-SWAN model version 54.01 (Dietrich et al., 2011) to simulate wind waves generated by each synthetic hurricane. The SWAN wave model (Booij et al., 1999; Ris et al., 1999) is a spectral wave model that simulates the generation, propagation, and dissipation of surface gravity waves in the deep ocean and coastal waters. The model calculates the phase-averaged wave characteristics, e.g., significant wave height, by solving the depth-averaged two-dimensional wave action balance equation and accounting for important physical processes such as shoaling, nonlinear wave-wave interaction, depth- and current-induced refraction, and energy dissipation due to bottom friction and depth-induced breaking, among others. Coupling SWAN to the hydrodynamic model ADCIRC (Luettich et al., 1992; Westerink et al., 1994) allows the model to account for the effects of wave-current interactions and variations in the water depth.
The computational mesh, shown in Figure 3, is based on the one developed by the U.S. Federal Emergency Management Agency’s Region II coastal storm surge study, which was previously validated for both tropical and extratropical cyclones (FEMA, 2014a). This mesh was used by the FEMA study to map flood hazards for New Jersey and New York coastal communities. The computational mesh covers the western North Atlantic Ocean, i.e., the region with longitudes between 98°W and 60°W, and latitudes between 8°N and 46°N. The mesh has a high spatial resolution in our study area. The resolution is about 80 m in the shallow nearshore zone (depth smaller than 8 m) and gradually increases to 8 km at a water depth of 50 m. In the deep ocean, the mesh resolution reaches 100 km. The spectra space in the wave model consists of 24 equally distributed directional bins and 37 frequencies logarithmically distributed between 0.033 and 1 Hz.
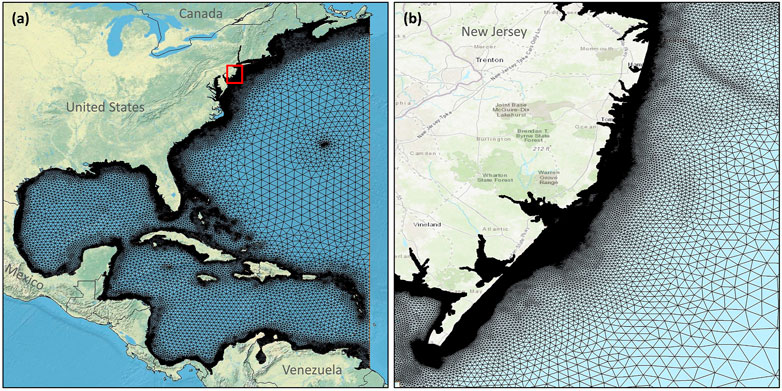
FIGURE 3. (A) Computational mesh of the ADCIRC-SWAN model used in the present study. (B) Closeup view of the computational mesh in the study area (the red box in panel a).
We use a computational time step of 3 s in the hydrodynamic model. The model is run in the depth-averaged mode with a constant eddy viscosity of 50 m2 s−1. Spatially varying bed roughness values (Manning’s n) are used in the model based on the land cover data (FEMA, 2014b). The same roughness values are used in the wave model. The wind drag coefficient is calculated using the method of Powell (2006) with a cap of 0.0025.
The computational time step in the SWAN wave model is 15 min, which is also the coupling interval to the hydrodynamic model. The SWAN model is run in its third-generation mode, which accounts for wave generation, dissipation in both deep and shallow waters, quadruplet wave-wave interactions in deep water and triad wave-wave interactions in shallower waters (SWAN, 2021). Wave growth by wind is calculated using the methods of Komen et al. (1984) and Cavaleri and Malanotte-Rizzoli (1981). Energy dissipation by whitecapping is included based on Komen et al. (1984) with its default parameters. Nonlinear quadruplet wave interactions and the triad wave-wave interactions are activated with their default parameters. Energy dissipations due to the bottom friction and limited water depth are calculated, respectively, based on the JONSWAP method (Hasselmann et al., 1973) and a breaker index of 0.73. The maximum limit for the wind drag coefficient is set to 0.0025. The atmospheric pressure and wind field at every computational time step are constructed from the hurricane parameters (e.g., maximum wind speed, minimum sea level pressure, and radius of maximum wind speed), using the asymmetric hurricane vortex formulation (Mattocks et al., 2006; Mattocks and Forbes 2008) based on the Holland (1980) gradient wind model.
Statistical Analysis
For each synthetic hurricane simulation, the temporal maximum SWH at each computational node are calculated from the hourly SWH time series simulated by the wave model. The simulated maximum SWHs for the storms in a specific dataset are utilized to calculate the mean and 95th-percentile of maximum SWH for that dataset. These metrics are used in the next sections to quantify and discuss the effects of climate change on hurricane-induced wave hazards. The non-parametric Wilcoxon rank-sum test (Hollander and Wolfe, 1999) is performed at a 5% significance level to determine if the projected changes in wave heights are statistically significant.
Together with the annual frequencies of intense hurricanes described earlier, the simulated maximum SWHs are also analyzed to estimate the return periods for the historical and future periods. Low return period SWHs are directly estimated using the empirical cumulative distribution function (CDF) of the simulated maximum SWHs. However, the high return-period SWHs, i.e., wave heights that represent the tail of the probability distribution, are estimated using the parametric CDF of the SWHs, given that the number of data points that represent the tail of distribution is small. We model the tail of the SWH CDF using the Peaks-Over-Threshold method (POT) with a Generalized Pareto Distribution (GPD) and maximum likelihood estimation (Coles, 2001). The threshold value used in the POT method is selected by trial and error so that the modeled CDF well represents the data points.
Results
Evaluation of Historical Synthetic Hurricanes
The accuracy of the climate model-based synthetic TCs to represent the historical climate of major hurricanes is evaluated by comparing the mean and 95th-percentile SWH metrics calculated for the NCEP- and climate model-based historical datasets (Figure 4). Overall, the correlation coefficient between the NCEP- and climate model-based SWH is 0.98 and the normalized bias (NBias) is about 5%. The normalized root-mean-square-error (NRMSE) is about 7% for the mean SWH and 10% for the 95th-percentile SWH. The bias and RMSE values are normalized using the NCEP-based SWH. A high correlation coefficient and low NBias and NRMSE indicate that the climate model-based synthetic hurricanes well reproduce the climate of hurricanes for the historical period of 1980–2000, even though the sample size in this dataset (399 storms) is different from the sample size in the NCEP-based dataset (150 storms). The ability of the dataset to reproduce the statistical characteristics of the NCEP-based wave height data is important, given that this study, similar to other climate change impact studies, utilizes statistical metrics to quantify the effects of climate change on hurricane-induced wind waves.
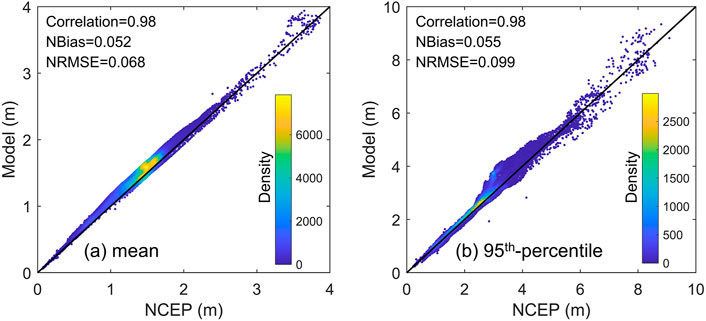
FIGURE 4. Historical mean and 95th-percentile SWH for the NCEP- and climate model-based major hurricane datasets. Each data point represents mean (or 95th-percentile) SWH at a specific computational node. Only nodes at a water depth of 20 m or shallower are considered. The scales of axes are different. The density refers to the number of data points in 0.05 m by 0.05 m cells in each plot.
To evaluate the accuracy of storm datasets beyond the mean and 95th-percentile SWH metrics, we further compare the cumulative distribution function (CDF) of NCEP- and model-based SWH at a series of virtual sites (Figure 5). These sites are located along three cross-shore profiles in the nearshore zone from the shoreline to a water depth of about 20 m (Figure 1 for the location of profiles). The profiles are hereafter named Seaside Park (Barnegat Bay Island, Ocean County), Atlantic City (Absecon Island, Atlantic County), and Wildwood (Five Mile Beach Island, Cape May). Sites A and B are in shallow areas of the nearshore zone at a water depth of about 2.4–2.6 m and 8.2–8.6 m, respectively, and site C is at a water depth of 20.8–21.6 m. For each profile, the CDF of SWHs is also shown for a site in the back-bays (at a water depth of about 1.5 m). Overall, the model-based CDFs of SWH agree with the NCEP-based CDFs at the location of virtual sites. In the back-bays, waves are small due to the limited water depth and wind fetch. The NCEP- and model-based CDFs agree well. In the open coast of barrier islands, the highest agreement is achieved at site A where nearly vertical CDFs indicate very small variability in the maximum SWH. This is because of shallow water depth (of about 2.5 m) at this site, which results in the depth-induced wave breaking to be the dominant factor controlling the wave height.
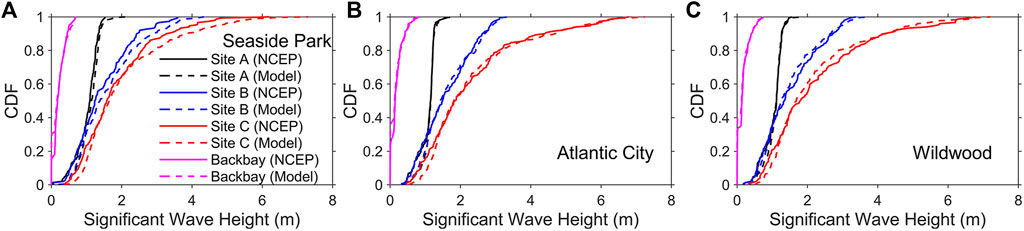
FIGURE 5. Cumulative distribution function (CDF) of historical SWH at the selected sites (Figure 1 for the location of sites).
We assumed that the NCEP dataset results in a realistic representation of the wave height climate for the historical period. The validity of the NCEP dataset may be evaluated against wave measurements. This will require wave measurements for historical hurricanes that impacted the study area during the full period of 1980–2000. However, such measurements are not available in the study area. Nevertheless, the validity of the NCEP dataset used in the present study was evaluated by Marsooli and Lin (2020) by comparing the NCEP-based and measured water levels at The Battery tide gauge station in New York Harbor, which is less than 30 km north of the northern tip of the study area. They found that the NCEP- and measurement-based storm tide return levels compare relatively well. Slight discrepancies were observed because the NCEP tracks are the statistical representations in the reanalysis climate of the historical TC tracks rather than the observed historical storms.
Future Projections
Our projections show a substantial increase, up to 0.27 m in the open coast, in hurricane-induced wave heights in coastal waters of New Jersey from the historical period of 1980–2000 to the future period of 2080–2100 (Figures 6, 7). Without SLR, the projected changes in the mean SWH metric, defined earlier, show an increase of between 0.1 and 0.22 m (5–10%) off the open coast and up to 0.04 m (15%) in the back-bays behind the barrier islands in Cape May, Atlantic, and Ocean Counties. Results for the With-SLR scenario, on the other hand, indicate that the combined effect of hurricane climatology change and a SLR of 1.19 m would be a statistically significant increase of the mean SWH in most areas. The projected increases reached 0.05–0.2 m in back-bays and 0.15–0.27 m in most areas of the open coast (percentage increase exceeds 15%). Similar patterns, with different magnitudes, are found for the 95th-percentile SWH metric (Figure 7).
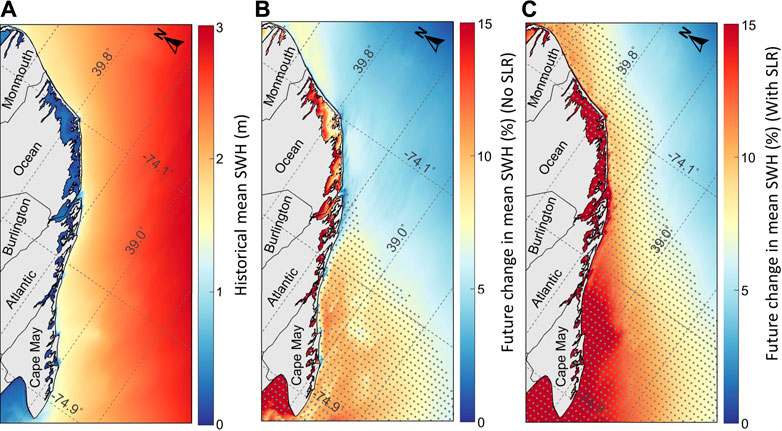
FIGURE 6. (A) Model-based historical mean SWH metric. (B) Projected changes by the end of 21st century under RCP8.5 scenario for the No-SLR scenario. (C) Same as (B) but for the With-SLR scenario. Stippling denotes areas where projected changes are statistically significant (with a confidence level of at least 95%). The scale bars in (B,C) are different from that in (A).
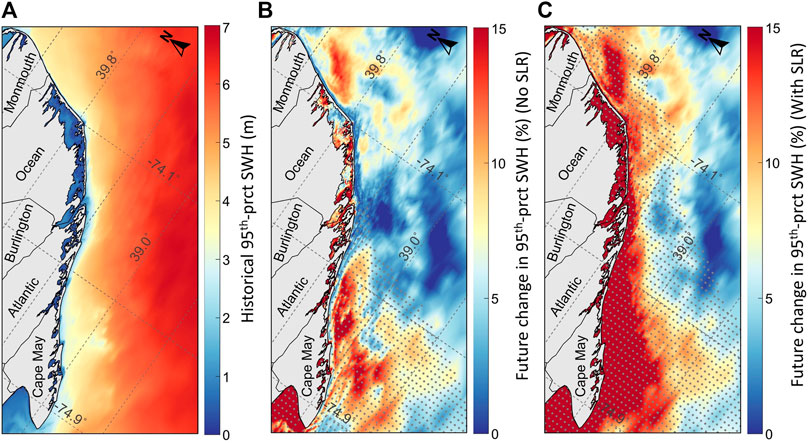
FIGURE 7. (A) Model-based historical 95th-percentile SWH metric. (B) Projected changes by the end of 21st century under RCP8.5 scenario for the No-SLR scenario. (C) Same as (B) but for the With-SLR scenario. Stippling denotes areas where projected changes are statistically significant (with a confidence level of at least 95%). The scale bars in (B,C) are different from that in (A).
To better illustrate the effect of SLR on wave hazards, Figure 8 shows the mean and 95th-percentile SWH profiles along three cross-shore profiles (Figure 1 for the location of profiles). It is evident that under the No-SLR scenario, the effects of future hurricanes on the wave height are more pronounced in the deep water while the effects on waves in the surf zone are small. For example, in the absence of SLR, negligible changes in the mean SWH are obtained for shallow areas with a water depth of smaller than 3 m. However, there are substantial increases in the SWH metrics for the same areas when SLR is considered. This is because the wave height in the shallow nearshore zone is mainly controlled by the water depth and wave energy dissipation due to depth-induced wave breaking. Regardless of how large offshore waves are, the wave height in the surf zone is limited, given the extensive depth-induced wave breaking (Thornton and Guza, 1982). However, SLR will increase the water depth in the present-day surf zone, allowing the larger waves to reach the shoreline, assuming that the present-day shorelines will not migrate landward. This is an acceptable assumption because New Jersey’s barrier islands are highly developed, and the state policy has been towards protecting beaches and shorelines to remain in the same location, e.g., through beach nourishment and re-nourishment projects. Furthermore, the development restricts the ability of beaches and shorelines to naturally adapt to the new sea level by migrating upland.
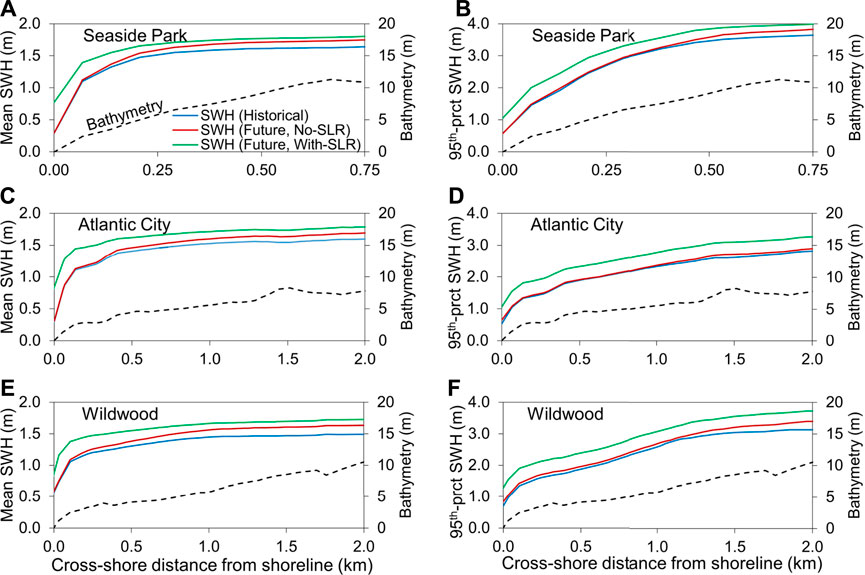
FIGURE 8. Cross-shore profiles of (A–C) mean and (D–F) 95th-percentile SWH along the three profiles shown in Figure 1. The black dashed lines represent the bathymetry. The origin of distance in the x axis is the shoreline (mean sea level vertical datum) along the open coast of barrier islands.
The combined effect of future hurricanes and SLR is to increase the wave height in the future surf zone. Figure 9 illustrates the 95th-percentile SWH along the shore of the three selected barrier islands. The wave heights are from computational nodes at a water depth of about 2.5 m for both with and without SLR scenarios. Overall, the combined effects of SLR and hurricane climatology change would result in larger waves compared to the wave height increase solely due to the hurricane climatology change. For instance, spatially averaged along the Five Mile Beach Island in Cape May County, the calculated 95th-percentile SWH increases from 1.42 m for the historical period to 1.52 and 1.64 m, i.e., 7 and 15% increases, for the future periods without and with SLR, respectively.
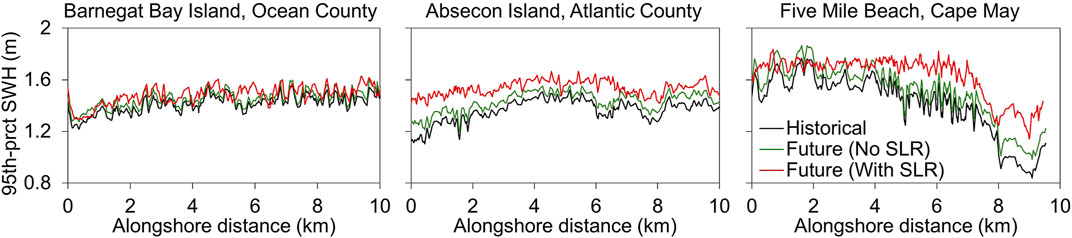
FIGURE 9. 95th-percentile SWH at a water depth of about 2.5 m along the shore of three selected barrier islands. Distances are measured from south to north along each barrier island (the origin of distance is the southmost point of each island).
The full percentiles of significant wave heights, shown in Figure 10, suggest that future hurricane climatology change (i.e., No-SLR scenario) would be the main cause of increases in the deep-water wave heights (i.e., at site C). For instance, averaged over the CDF of SWH at site C of the Atlantic City profile (red long dashed line in Figure 10), the increase is 6.8% under hurricane climatology change while it is 8.4% under both hurricane climatology change and SLR. Because of deep waters at this site, the effect of SLR on the water depth and, in turn, the wave height is negligible. On the other hand, in shallow-water sites (i.e., at site A), SLR is the main driver of projected increases in the wave height in the present-day surf zone. At these sites, while the percentage increase in the SWH remains below 10% for the No-SLR scenario, it reaches 40% for the With-SLR scenario.
Discussion
Findings of the present study suggest that, under a high emission scenario, climate change from the historical period of 1980–2000 (baseline) to the future period of 2080–2100 would significantly increase the extreme wave heights generated by major hurricanes off the coast of New Jersey. The projected increase in the extreme wave heights contrasts with projections obtained from previous wave climate change studies based on global climate models. These previous studies found a future decrease in the mean and extreme wave heights in the North Atlantic Ocean (Hemer et al., 2013; Erikson et al., 2016; Meucci et al., 2020). For example, Erikson et al. (2016) simulated wind waves derived by wind fields from four CMIP5 (Coupled Model Intercomparison Project Phase 5) global climate models (GCMs) and found that, under the RCP8.5 emission scenario, the wave heights by the end of 21st century would decrease along much of the mainland U.S. coast including the East Coast. Meucci et al. (2020) quantified the changes in global extreme wave events over the 21st century using surface winds from an ensemble of seven CMIP5 global climate models. For low-to mid-latitudes of the North Atlantic, they found statistically significant decreases (5–15%) in the 100-years return period SWH by the end of the 21st century (under RCP8.5 scenario) relative to the 1979–2005 period.
The difference between the projected changes in extreme wave heights from the present study and the previous global/basin-scale studies based on global wave climate models is because the latter studies were based on the wind field from GCMs, which are unable to adequately resolve extreme wind events, e.g., major hurricanes, due to their coarse resolution (Timmermans et al., 2017). Casas-Prat et al. (2018) simulated waves using atmospheric forcing from five GCMs under a RCP8.5 emission scenario and found a decrease in the annual mean SWH in the North Atlantic Ocean, which was attributed to the statistically significant decrease in the mean 10-m wind speed in the western North Atlantic Ocean and the Atlantic tropical cyclone formation region. In contrast to the projected decrease in the future mean wind speed climate, several studies have shown that the intensity and frequency of major hurricanes in the Atlantic basin would increase under a high emission scenario (e.g., Walsh et al., 2016; Sugi et al., 2017; Knutson et al., 2020). Unlike these (and other) global wave climate studies based on GCMs with a coarse resolution (in terms of hurricane modeling), the present study was based on dynamic downscaling and synthetic TCs generated by a hurricane model (Emanuel et al., 2008).
Our projections showed that hurricane climatology change, i.e., No-SLR scenario, would increase wave heights in the offshore zone of New Jersey. The increase is statistically significant in low-latitude regions of New Jersey including the Cape May and Atlantic Counties. In the present-day surf zone, e.g., shallower than 3–5 m, SLR would result in a significant increase in the wave height because the wave height in the surf zone is mainly controlled by the depth-induced wave breaking process. SLR will make the present-day surf zone deeper and, in turn, less depth-induced wave energy dissipation. Thus, larger waves in the nearshore zone would lead to changes in the distribution of breaking vs. unbroken waves, which would influence the surf zone dynamics and processes driven by breaking waves, e.g., sediment transport, wave setup, and turbulence (Feddersen and Trowbridge 2005; Ruessink 2010). When the influence of SLR was combined with that of hurricane climatology change, i.e., With-SLR scenario, we found statistically significant increases in the SWH in the entire nearshore zone, including in the present-day surf zone. As a result of deeper water due to SLR, the projected increase in the wave height exceeded 15%.
In addition to sea level rise, storm surge and astronomical tides could significantly contribute to the water depth in the surf zone and, thus, the wave climate along the coast. The effects of storm surge are included in this study, as the SWAN wave model is coupled with the hydrodynamic ADCIRC model. The effects of astronomical tides are not considered, due to the randomness of the storm arrival time and timing of tides, i.e., the storm arrival time could coincide with any tidal phase (e.g., low water, neap tide compared to highwater, spring tide). Including the effects from tides will require modeling each storm for different tidal timing, which will increase the number of simulations and computational cost by several factors. In regions with a large tidal range, the effects of tides on the water depth in shallow areas and, thus, the wave height in the surf zone could be significant and should be studied in future studies. Given by the U.S. National Oceanic and Atmospheric Administration (NOAA) for the tide gauge Atlantic City NJ, the mean range of tides along the open coast of New Jersey is about 1.2 m, which is nearly the same as the SLR scenario considered in the present study. For a storm impacting the coast at high water during a spring tide, we would expect the effect to be an additional increase in the wave height (which could be comparable to the magnitude of increase caused by SLR), whereas for a neap tide we would expect the wave height increase due to SLR be partly diminished. Therefore, the influence of SLR on wave heights could be more profound if the peak waves occur during high tides. Wave hazard assessment studies for use in coastal planning and development should account for the influence of tides and the random arrival time of storms.
The projected future increase in the wave heights induced by major hurricanes could lead to an increase in the vulnerability of coastal communities and ecosystems to extreme wave hazards in the future. Many coastal communities worldwide are protected against flooding using engineering structures such as seawalls and levees. Depending on their crest height, these structures are best to prevent flooding due to storm surge but could be overtopped by surface waves during extreme events. An increase in extreme wave heights would increase the risks associated with wave overtopping. Like the barrier islands and open coasts in New Jersey, many other coastal areas exposed to TCs are protected from extreme sea levels by sandy beaches and dunes as well as coastal structures. Larger waves could increase the vulnerability to coastal flooding because of a higher potential for exposure of these protective systems to wave runup, overtopping, and erosion of beach-dune systems.
Design waves in coastal and ocean engineering projects are decided based on the statistical characteristics of waves with certain return periods. The increase in the future annual frequency of intense hurricanes and the projected increases in the wave heights would shorten the return period of extreme waves and, in turn, the design wave heights. Figure 11 shows the return period curves for the historical and future SWHs at sites along the Atlantic City cross-shore profile. The effects of hurricane climatology change on return periods of SWHs at the three sites is to substantially shorten the return period of low-probability wave heights. For example, the historical 1000-years event (SWH of 1.41 m at site A, 3.03 m at site B, and 5.91 m at site C) would become about a 280-years event by the end of 21st century. When the effects of SLR is included, the return periods become even shorter in the nearshore zone. For example, at site B, the return period of the historical 1000-years SWH would become 85-years.
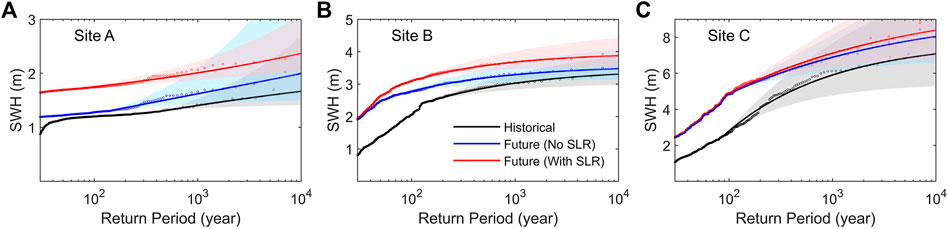
FIGURE 11. Return periods of SWHs for the three sites along the Atlantic City cross-shore profile (Figure 1 for the site location). Shaded areas cover the 90% statistical confidence interval (5th to 95th percentiles). Dots are data points.
The present study focused on the combined effects of SLR and hurricane climatology change on wave hazards. Given that our results showed that SLR would significantly increase wave heights in shallow waters, a higher mean sea level alone, i.e., with no changes in the climate of surface winds, would also have a considerable effect on the wave climate in shallow waters. This is of great concern for New Jersey because large areas in its back-bays are covered by salt marshes. Previous studies have shown that salt marshes are a natural source of wave energy dissipation (Marsooli and Wu 2014; Smith et al., 2016; Marsooli et al., 2017). However, the effectiveness of salt marshes for wave attenuation is proportional to their submergence ratio. Deeper waters due to SLR would not only result in larger waves but could lead to more submergence during storms and, thus, less wave energy dissipation by vegetation. More research studies should be carried out to investigate the impact of extreme wave climate change on coastal communities and infrastructure.
Summary and Conclusion
The objective of this study was to quantify the influence of SLR and TC climatology change on future wind waves generated by major hurricanes (category 3-5 hurricanes) off the coast of New Jersey. To achieve this objective, we utilized a high-resolution hydrodynamic-wave model to simulate synthetic hurricane-induced waves for the historical period of 1980–2000 and the future period of 2080–2100 under the RCP8.5 high emission scenario. Simulations of the future period were performed both with and without the contribution of SLR.
The projected future wave heights showed that hurricane climatology change would alone increase the wave heights up to 15% in the open coastal waters as well as back-bays of the study area. Projected wave heights in deeper waters showed a larger increase than in shallow waters, given that the wave climate in shallow waters is mainly influenced by the water depth. Hurricane climatology change together with a SLR of 1.19 m would result in a statistically significant increase in the projected future wave heights. In present-day shallow areas of the nearshore zone, the influence of SLR on the wave height overwhelmingly dominates the influence of hurricane climatology change. This suggests that, even with no changes in future hurricane characteristics, SLR will result in more energetic waves to reach the present-day shorelines.
The results of the present study are particularly valuable as coastal communities grapple with the challenges posed by a changing climate. Coastal communities in New Jersey and throughout the Northeast of the United States are typically economic engines that drive the regional economy. At the same time, the waters provide critical habitat for a range of species including several threatened and endangered species. The changes identified in the offshore extreme wave climate poses a threat to not only existing industries such as maritime commerce, but also developing industries such as offshore wind. Closer to shore, increasing extreme wave heights pose a threat to both the built and natural environment in the form of beach and marsh erosion, increased overtopping, and structural damage. Potential next steps would be to identify the most significant impacts associated with the projected changes and to develop long and short-term strategies for mitigating these impacts. Ultimately, these strategies should be included in local, state, and regional resilience plans, such as the one currently being developed in New Jersey.
Data Availability Statement
The raw data supporting the conclusion of this article will be available from the authors upon request.
Author Contributions
RM designed the study and conducted the numerical simulations. RM wrote the first draft of the manuscript. MJ and JM wrote sections of the manuscript. All authors discussed the results.
Funding
This article is the result of research work sponsored by the New Jersey Sea Grant Consortium (NJSGC) with funds from the National Oceanic and Atmospheric Administration (NOAA) Office of Sea Grant, U.S. Department of Commerce, under NOAA grant number NA18OAR4170087 and the NJSGC. The statements, findings, conclusions, and recommendations are those of the authors and do not necessarily reflect the views of the NJSGC or the U.S. Department of Commerce. NJSG-21-978.
Conflict of Interest
The authors declare that the research was conducted in the absence of any commercial or financial relationships that could be construed as a potential conflict of interest.
Publisher’s Note
All claims expressed in this article are solely those of the authors and do not necessarily represent those of their affiliated organizations, or those of the publisher, the editors and the reviewers. Any product that may be evaluated in this article, or claim that may be made by its manufacturer, is not guaranteed or endorsed by the publisher.
Acknowledgments
We would like to thank the three reviewers for their constructive comments and suggestions.
References
Avila, L. A., and Cangialosi, J. (2011). Hurricane Irene Tropical Cyclone Report. Miami, US: National Hurricane Center. NOAA.
Baker, M. (2019). New Jersey State Hazard Mitigation Plan. Rochester, US: Michael Baker International Inc.
Bilinski, J., Buchanan, G., Frizzera, D., Hazen, R., Lippincott, R., Procopio, N., et al. (2015). Damage Assessment Report on the Effects of Hurricane Sandy on the State of New Jersey’s Natural Resources: Final Report. New Jersey, US: Office of Science, New Jersey Department of Environmental Protection.
Booij, N., Ris, R. C., and Holthuijsen, L. H. (1999). A Third-Generation Wave Model for Coastal Regions: 1. Model Description and Validation. J. Geophys. Res. 104 (C4), 7649–7666. doi:10.1029/98JC02622
Casas-Prat, M., Wang, X. L., and Swart, N. (2018). CMIP5-based Global Wave Climate Projections Including the Entire Arctic Ocean. Ocean Model. 123, 66–85. doi:10.1016/j.ocemod.2017.12.003
Cavaleri, L., and Malanotte-Rizzoli, P. (1981). Wind Wave Prediction in Shallow Water: Theory and Applications. J. Geophys. Res. 86 (C11), 10,961–10,973. doi:10.1029/jc086ic11p10961
Chen, Q., Wang, L., and Zhao, H. (2009). Hydrodynamic Investigation of Coastal Bridge Collapse during Hurricane Katrina. J. Hydraulic Eng. 135 (3). doi:10.1061/(asce)0733-9429(2009)135:3(175)
Collins, W. J., Bellouin, N., Doutriaux-Boucher, M., Gedney, N., Halloran, P., Hinton, T., et al. (2011). Development and Evaluation of an Earth-System Model - HadGEM2. Geosci. Model. Dev. 4, 1051–1075. doi:10.5194/gmd-4-1051-2011
Dietrich, J. C., Zijlema, M., Westerink, J. J., Holthuijsen, L. H., Dawson, C., Luettich, R. A., et al. (2011). Modeling hurricane Waves and Storm Surge Using Integrally-Coupled, Scalable Computations. Coastal Eng. 58 (1), 45–65. doi:10.1016/j.coastaleng.2010.08.001
Donner, L. J., Wyman, B. L., Hemler, R. S., Horowitz, L. W., Ming, Y., Zhao, M., et al. (2011). The Dynamical Core, Physical Parameterizations, and Basic Simulation Characteristics of the Atmospheric Component AM3 of the GFDL Global Coupled Model CM3. J. Clim. 24, 3484–3519. doi:10.1175/2011jcli3955.1
Emanuel, K., Sundararajan, R., and Williams, J. (2008). Hurricanes and Global Warming: Results from Downscaling IPCC AR4 Simulations. Bull. Amer. Meteorol. Soc. 89 (3), 347–368. doi:10.1175/bams-89-3-347
Erikson, L. H., Hegermiller, C. E., Barnard, P. L., and Storlazzi, C. (2016). Wave Projections for United States mainland Coasts. Virginia, US: U.S. Geological Survey summary of methods to accompany data release. doi:10.5066/F7D798GR
Feddersen, F., and Trowbridge, J. H. (2005). The Effect of Wave Breaking on Surf-Zone Turbulence and Alongshore Currents: A Modeling Study*. J. Phys. Oceanography 35, 2187–2203. doi:10.1175/jpo2800.1
FEMA (2014a). Region II Storm Surge Project - Mesh Development. Washington DC, USA: Federal Emergency Management Agency, Department of Homeland Security.
FEMA (2014b). Region II Storm Surge Project – Spatially Varying Nodal Attribute Parameters. Washington DC, USA: Federal Emergency Management Agency, Department of Homeland Security.
Gutmann, E. D., Rasmussen, R. M., Liu, C., Ikeda, K., Bruyere, C. L., Done, J. M., et al. (2018). Changes in Hurricanes from a 13-yr Convection-Permitting Pseudo-global Warming Simulation. J. Clim. 31, 3643–3657. doi:10.1175/jcli-d-17-0391.1
Hapke, C. J., Himmelstoss, E. A., Kratzmann, M. G., List, J. H., and Thieler, E. R. (2011). National Assessment of Shoreline Change; Historical Shoreline Change along the New England and Mid-Atlantic Coasts: U.S. Geological Survey Open-File Report 2010-1118. Virginia, US: U.S. Geological Survey, 57.
Hasselmann, K., Barnett, T. P., Bouws, E., Carlson, H., Cartwright, D. E., Enke, K., et al. (1973). Measurements of Wind−wave Growth and Swell Decay during the Joint North Sea Wave Project (JONSWAP). Dtsch. Hydrogr. Z. Suppl. 12, A8.
Hemer, M. A., Fan, Y., Mori, N., Semedo, A., and Wang, X. L. (2013). Projected Changes in Wave Climate from a Multi-Model Ensemble. Nat. Clim Change 3, 471–476. doi:10.1038/nclimate1791
Hill, K. A., and Lackmann, G. M. (2011). The Impact of Future Climate Change on TC Intensity and Structure: A Downscaling Approach. J. Clim. 24, 4644–4661. doi:10.1175/2011jcli3761.1
Holland, G. J. (1980). An Analytic Model of the Wind and Pressure Profiles in Hurricanes. Mon. Wea. Rev. 108 (8), 1212–1218. doi:10.1175/1520-0493(1980)108<1212:aamotw>2.0.co;2
Hollander, M., and Wolfe, D. A. (1999). Nonparametric Statistical Methods. Hoboken, NJ: John Wiley & Sons.
Knutson, T., Camargo, S. J., Chan, J. C. L., Emanuel, K., Ho, C.-H., Kossin, J., et al. (2020). Tropical Cyclones and Climate Change Assessment: Part II: Projected Response to Anthropogenic Warming. Bull. Am. Meteorol. Soc. 101 (3), E303–E322. doi:10.1175/bams-d-18-0194.1
Knutson, T. R., Sirutis, J. J., Vecchi, G. A., Garner, S., Zhao, M., Kim, H.-S., et al. (2013). Dynamical Downscaling Projections of Twenty-First-Century Atlantic Hurricane Activity: CMIP3 and CMIP5 Model-Based Scenarios. J. Clim. 26, 6591–6617. doi:10.1175/jcli-d-12-00539.1
Knutson, T. R., Sirutis, J. J., Zhao, M., Tuleya, R. E., Bender, M., Vecchi, G. A., et al. (2015). Global Projections of Intense Tropical Cyclone Activity for the Late Twenty-First century from Dynamical Downscaling of CMIP5/RCP4.5 Scenarios. J. Clim. 28, 7203–7224. doi:10.1175/jcli-d-15-0129.1
Komen, G. J., Hasselmann, K., and Hasselmann, K. (1984). On the Existence of a Fully Developed Wind-Sea Spectrum. J. Phys. Oceanogr. 14, 1271–1285. doi:10.1175/1520-0485(1984)014<1271:oteoaf>2.0.co;2
Kopp, R. E., Andrews, C., Broccoli, A., Garner, A., Kreeger, D., Leichenko, R., et al. (2019). New Jersey’s Rising Seas and Changing Coastal Storms: Report of the 2019 Science and Technical Advisory Panel. Trenton, New Jersey: Rutgers, The State University of New Jersey, Prepared for the New Jersey Department of Environmental Protection.
Kopp, R. E., Horton, R. M., Little, C. M., Mitrovica, J. X., Oppenheimer, M., Rasmussen, D. J., et al. (2014). Probabilistic 21st and 22nd century Sea‐level Projections at a Global Network of Tide‐gauge Sites. Earth's Future 2 (8), 383–406. doi:10.1002/2014ef000239
Lawrence, M. B., Mayfield, B. M., Avila, L. A., Pasch, R. J., and Rappaport, E. N. (1997). Atlantic Hurricane Season of 1995. Monthly Weather Rev. 126, 1124–1151.
Lin, N., Kopp, R. E., Horton, B. P., and Donnelly, J. P. (2016). Hurricane Sandy's Flood Frequency Increasing from Year 1800 to 2100. Proc. Natl. Acad. Sci. USA 113 (43), 12071–12075. doi:10.1073/pnas.1604386113
Luettich, R. A., Westerink, J. J., and Scheffner, N. W. (1992). ADCIRC: An Advanced Three-Dimensional Circulation Model for Shelves, Coasts and Estuaries. U.S. Army Corps of Engineers, Report 1. Theory and methodology ofADCIRC-2DDI and ADCIRC-3DL (Tech. Rep. DRP-92-6).
Marsooli, R., Lin, N., Emanuel, K., and Feng, K. (2019). Climate Change Exacerbates hurricane Flood Hazards along US Atlantic and Gulf Coasts in Spatially Varying Patterns. Nat. Commun. 10 (3785), 3785. doi:10.1038/s41467-019-11755-z
Marsooli, R., and Lin, N. (2020). Impacts of Climate Change on hurricane Flood Hazards in Jamaica Bay, New York. Clim. Change. 163, 2153–2171. doi:10.1007/s10584-020-02932-x
Marsooli, R., Orton, P. M., and Mellor, G. (2017). Modeling Wave Attenuation by Salt Marshes in J Amaica B Ay, N Ew Y Ork, Using a New Rapid Wave Model. J. Geophys. Res. Oceans 122, 5689–5707. doi:10.1002/2016jc012546
Marsooli, R., and Wu, W. (2014). Numerical Investigation of Wave Attenuation by Vegetation Using a 3D RANS Model. Adv. Water Resour. 74, 245–257. doi:10.1016/j.advwatres.2014.09.012
Martin, G. M., Bellouin, N., Collins, W. J., Culverwell, D., Halloran, P. R., Hardiman, S. C., et al. (2011). The HadGEM2 Family of Met Office Unified Model Climate Configurations. Geophys. Model. Dev. 4, 723–757. doi:10.5194/gmd-4-723-2011
Mattocks, C., and Forbes, C. (2008). A Real-Time, Event-Triggered Storm Surge Forecasting System for the State of North Carolina. Ocean Model. 25 (3-4), 95–119. doi:10.1016/j.ocemod.2008.06.008
Mattocks, C., Forbes, C., and Ran, L. (2006). Design and Implementation of Real-Time Storm Surge and Flood Forecasting Capability for the State of North Carolina.UNC, UNC-CEP Technical Report. Available at: https://www.dropbox.com/s/ld5j1gvsst0je0s/2006_Mattocks.pdf?dl=0.
Meucci, A., Young, I. R., Hemer, M., Kirezci, E., and Ranasinghe, R. (2020). Projected 21st century Changes in Extreme Wind-Wave Events. Sci. Adv. 6 (24), eaaz7295. doi:10.1126/sciadv.aaz7295
Miller, J. K., Lopez, O., Wehof, J., and Bak, S. (2012). A Comparison of the Impacts of Hurricane Irene and the “Friday the 13th” Northeaster in New Jersey. Shore and Beach 80 (2).
Moss, R., Babiker, M., Brinkman, S., Calvo, E., Carter, T., Edmonds, J., et al. (2008). Towards New Scenarios for Analysis of Emissions, Climate Change, Impacts, and Response Strategies. Geneva: Intergovernmental Panel on Climate Change, 132.
Patricola, C. M., and Wehner, M. F. (2018). Anthropogenic Influences on Major Tropical Cyclone Events. Nature 563, 339–346. doi:10.1038/s41586-018-0673-2
Pielke, R. A., Gratz, J., Landsea, C. W., Collins, D., Saunders, M. A., and Musulin, R. (2008). Normalized Hurricane Damage in the United States:1900–2005. Nat. Hazards Rev. 9 (1). doi:10.1061/(asce)1527-6988(2008)9:1(29)
Powell, M. D. (2006). Drag Coefficient Distribution and Wind Speed Dependence in Tropical Cyclones. Final Report to the National Oceanic and Atmospheric Administration (NOAA) Joint Hurricane Testbed (JHT) Program. Available at: https://www.nhc.noaa.gov/jht/05-07reports/final_Powell_JHT07.pdf.
Ris, R. C., Holthuijsen, L. H., and Booij, N. (1999). A Third-Generation Wave Model for Coastal Regions: 2. Verification. J. Geophys. Res. 104 (C4), 7667–7681. doi:10.1029/1998JC900123
RSCNJ (2012). Hurricane Sandy: Beach-Dune Performance Assessment of New Jersey Beach Profile Network Sites. Annual Report, Hurricane Sandy: NJ’s Beach-Dune Responses. Available at: https://stockton.edu/coastal-research-center/njbpn/reports.html.
Ruessink, B. G. (2010). Observations of Turbulence within a Natural Surf Zone. J. Phys. Oceanography 40 (12), 2696–2712. doi:10.1175/2010jpo4466.1
Smith, A. B., and Katz, R. W. (2013). US Billion-Dollar Weather and Climate Disasters: Data Sources, Trends, Accuracy and Biases. Nat. Hazards 67, 387–410. doi:10.1007/s11069-013-0566-5
Smith, J. M., Bryant, M. A., and Wamsley, T. V. (2016). Wetland Buffers: Numerical Modeling of Wave Dissipation by Vegetation. Earth Surf. Process. Landforms 41, 847–854. doi:10.1002/esp.3904
Stammer, D., Cazenave, A., Ponte, R. M., and Tamisiea, M. E. (2013). Causes for Contemporary Regional Sea Level Changes. Annu. Rev. Mar. Sci. 5 (1), 21–46. doi:10.1146/annurev-marine-121211-172406
Stevens, B., Giorgetta, M., Esch, M., Mauritsen, T., Crueger, T., Rast, S., et al. (2013). Atmospheric Component of the MPI‐M Earth System Model: ECHAM6. J. Adv. Model. Earth Syst. 5 (2), 146–172. doi:10.1002/jame.20015
Sugi, M., Murakami, H., and Yoshida, K. (2017). Projection of Future Changes in the Frequency of Intense Tropical Cyclones. Clim. Dyn. 49, 619–632. doi:10.1007/s00382-016-3361-7
SWAN, (2021). Scientific and Technical Documentation. Delft, The Netherlands: Delft University of Technology. Available at: https://swanmodel.sourceforge.io/download/zip/swantech.pdf.
Thornton, E. B., and Guza, R. T. (1982). Energy Saturation and Phase Speeds Measured on a Natural beach. J. Geophys. Res. 87, C12. doi:10.1029/jc087ic12p09499
Timmermans, B., Stone, D., Wehner, M., and Krishnan, H. (2017). Impact of Tropical Cyclones on Modeled Extreme Wind‐wave Climate. Geophys. Res. Lett. 44, 1393–1401. doi:10.1002/2016gl071681
IPCC (2021). in Climate Change 2021: The Physical Science Basis. Contribution of Working Group I to the Sixth Assessment Report of the Intergovernmental Panel on Climate Change [Masson-Delmotte. Editors V. P. Zhai, A. Pirani, S. L. Connors, C. Péan, S. Bergeret al. (Cambridge, UK: Cambridge University Press). In Press.
Walsh, K. J. E., McBride, J. L., Klotzbach, P. J., Balachandran, S., Camargo, S. J., Holland, G., et al. (2016). Tropical Cyclones and Climate Change. Wires Clim. Change 7 (1), 65–89. doi:10.1002/wcc.371
Watson, K. M., Collenburg, J. V., and Reiser, R. G. (2013). Hurricane Irene and Associated Floods of August 27-30, 2011. in New Jersey (No. 2013-5234) (Virginia, US: US Geological Survey).
Weinkle, J., Landsea, C., Collins, D., Musulin, R., Crompton, R. P., Klotzbach, P. J., et al. (2018). Normalized hurricane Damage in the continental United States 1900-2017. Nat. Sustain. 1, 808–813. doi:10.1038/s41893-018-0165-2
Westerink, J. J., Luettich, R. A., Blain, C. A., and Scheffner, N. W. (1994). ADCIRC: An Advanced Three-Dimensional Circulation Model for Shelves, Coasts and Estuaries. U.S. Army Corps of Engineers, Report 2: Users’ manual for ADCIRC-2DDI (Tech. Rep. DRP-92-6).
Yukimoto, S., Adachi, Y., Hosaka, M., Sakami, T., Yoshimura, H., Hirabara, M., et al. (2012). A New Global Climate Model of the Meteorological Research Institute: MRI-CGCM3 -Model Description and Basic Performance-. J. Meteorol. Soc. Jpn. 90A, 23–64. doi:10.2151/jmsj.2012-a02
Keywords: wind waves, tropical cyclone, hurricane, climate change, sea level rise, New Jersey
Citation: Marsooli R, Jamous M and Miller JK (2021) Climate Change Impacts on Wind Waves Generated by Major Tropical Cyclones off the Coast of New Jersey, USA. Front. Built Environ. 7:774084. doi: 10.3389/fbuil.2021.774084
Received: 10 September 2021; Accepted: 08 November 2021;
Published: 02 December 2021.
Edited by:
Nobuhito Mori, Kyoto University, JapanReviewed by:
Tomoya Shimura, Kyoto University, JapanTakayuki Suzuki, Yokohama National University, Japan
Christine Angelini, University of Florida, United States
Copyright © 2021 Marsooli, Jamous and Miller. This is an open-access article distributed under the terms of the Creative Commons Attribution License (CC BY). The use, distribution or reproduction in other forums is permitted, provided the original author(s) and the copyright owner(s) are credited and that the original publication in this journal is cited, in accordance with accepted academic practice. No use, distribution or reproduction is permitted which does not comply with these terms.
*Correspondence: Reza Marsooli, cm1hcnNvb2xAc3RldmVucy5lZHU=