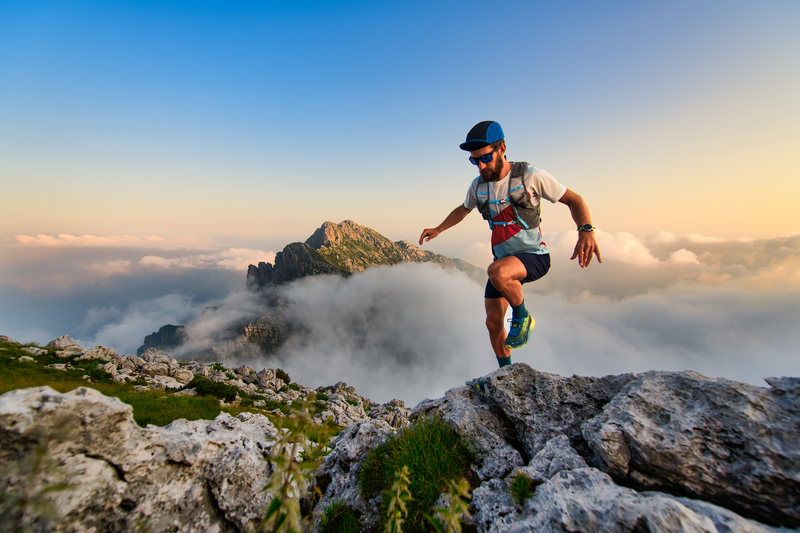
94% of researchers rate our articles as excellent or good
Learn more about the work of our research integrity team to safeguard the quality of each article we publish.
Find out more
REVIEW article
Front. Built Environ. , 18 November 2021
Sec. Construction Management
Volume 7 - 2021 | https://doi.org/10.3389/fbuil.2021.770496
This article is part of the Research Topic Construction project delivery: practices, challenges, and opportunities View all 5 articles
The 2030 agenda for sustainable development has embraced the importance of sustainable practices in the construction industry. Parallel to the Industry revolution 4.0, the construction industry needs to keep pace with technological advances in data management to keep pace with the revolution through the ability to process and extract value from data. This phenomenon attracts the requirement of Big Data (BD). The construction industry deals with large volumes of heterogeneous data, which is expected to increase exponentially following an intense use of modern technologies. This research presents a comprehensive study of the literature, investigating the potential application of BD integration in the construction industry. The adoption of such technologies in this industry remains at a nascent stage and lags broad uptake of these technologies in other fields. The Construction Industry is driving to boost its productivity through the implementation of data technologies; hence, significant research is needed in this area. Currently, there is a lack of deep comprehensive research on BD integration applications that provide insight for the construction industry. This research closes the gap and gives an overview of the literature. The discussion presented the current utilization, the issues, and ways for potential works along with the challenges companion with the implementation.
Data integration is a series of processes applied to retrieve and mix data from several sources into meaningful and useful information. An entire data integration solution delivers trusted data from a range of sources (IBM, 2020). Traditional data integration techniques mainly depended on the ETL (Extract, Transform, and Load) process to ingest and clean data, thereafter loading it into a data repository. Presently, a massive volume of data is collected from numerous heterogeneous data sources which are generating data in real time with different qualities which are considered Big Data (BD) (Konikov and Konikov, 2017). BD integration is extremely challenging especially after the normal data integration techniques are let down to handle it. BD integration varies from traditional data integration in many parameters—Volume, Velocity, Validity, Visualization, Variety, Value, and Veracity—which are the BD’s main characteristics. Construction project success is directly related to continuous access to accurate project data. A construction project generates an enormous amount of complex, specific, and professional data, although much effort has been applied to overcome these challenges (Kagan, 2019). This research is aiming to provide an overview of the big data integration technologies, opportunities, and challenges and to show some of the latest studies made in this domain.
The construction industry is dealing with significant data arising from diverse disciplines throughout the life cycle of a project. The main problem and questions are what is the definition of these data, from where to extract, how to transform, then how to visualize to integrate them to one platform. How can this data integration be measured? The capability to analyze big amounts of data and to extract beneficial insights from it has revolutionized the community and the innovation; hence, it has led to tremendous sustainable development around the globe. The construction industry is not new to data analytics application; the utilization of such application in the industry is left over at a budding stage and slows the wide usage of these applications from other industries (Bilal et al., 2016a).
Therefore, significant research is needed in this area. Consequently, this paper intends to establish a current overview of data technologies from the viewpoint of the construction sector with the aim of providing some possible directions for accelerating future works, presenting opportunities and barriers, and demonstrating some of the recent research in this area. These were accomplished by defining and evaluating the implementation of data technologies for integrating construction data to get the benefit from it, from research papers within the construction industry according to the context and the collection of keywords used for this analysis from 2015 till 2021. Based on the in-depth critical literature review of journal articles, thesis’, books, reports published, and conference proceedings and on content analysis, the main keywords on which the research was built were big data, construction digitalization, data integration and visualization, and data-driven construction project management. The flow map of the research are shown in Figure 1 alongside the objectives to determine the relative studies in English language that can be accessed from several databases such as Scopus, Web of Science, IEEE Xplore, ScienceDirect, and Google Scholar and analyze the potential applications of big data integration in the construction industry.
The research has analyzed the literature in depth to uncover many aspects, starting from the data revolution in the construction industry, and explore ways to digitize the construction industry. This is followed by an overview of data generation and how it is utilized with a summarization of the data collections, approaches, and systems. To finalize the research and form an integrated and comprehensive picture, all techniques, stages, preprocessing techniques, parameters, benefits of adoption, and recent challenges for adopting the integration of the analyzed data technologies were covered. In conclusion, a construction research context and detail of the research area has been analyzed and presented with highlighting the important keywords of the big data integration research from various literatures.
Data Revolution is improving the working dynamics of projects by expediting innovations in its aspects and refining decision making, boost productivity, and organizational abilities. The construction industry will also face these changes. The construction industry is currently suffering from a variety of problems, the most important of which is the severe inefficiency that leads to lower productivity, which in turn risks costing the global economy an estimated $1.6 trillion a year (Barbosa et al., 2017). Adopting technologies like BD integration sounds inevitable. Moreover, loss of human life due to poor safety and hazardous nature of work are due to the above problems. The construction industry is experiencing outstanding challenges like the shortage of digitalization, poor project management, ineffective design, poor worker safety, increased greenhouse gas emissions, and a volatile construction economy (Li et al., 2017c; Li et al., 2017d).
The incorporation or implementation of innovation and other mechanisms of change is one of the fundamentals of development, but the construction industry was lagging back in comparison to the manufacturing industry. Although the breadth of data may not be as “large” as the volume of data in retail or financial sectors, the construction sector deals with very heterogeneous data from various sources such as plans, bill of quantities, building specifications, rate of price, and day-to-day data project progress. Given this significant demand, there is an urgent need to analyze and integrate the above multivariate data (Moeini et al., 2017).
Peiffer (2016) declared that BD integration is on top of the considerable leading factors in configuring the way which would lead toward improving the efficiency of the industry. Also in the architecture, engineering, and construction industries, the adoption of data and information technologies enhances a deep transformation of the industry for modeling, designing, and managing intelligent construction (Parisi et al., 2021). However, construction digitalization in data science management is incompetent, which according to (Castagnino et al., 2016) was the outcome of the late and slow locomotion made by the industry to take over new technologies. This is confirmed by the MGI’s indicator that places the construction industry on the bottom of the list of the digitized industries in the world. Renz et al. (2016) added up to the deliberate modification made by the industry that is caused by inadequate data-driven decision-making.
Digitalizing the construction industry and integrating the data with suitable methods of visualization over the sector, deployment of such technologies and processes is essential to the required advancement of the construction industry. This advancement gives new opportunities over the entire value chain, through the project life-cycle phase. While having different characteristics to data analysis, the increasing volume of asset data and rising use of Building Information Modeling (BIM) is likely to create further demand for data analysts with roads agencies and local governments (Hart et al., 2018). Within 10 years, according to (Gerbert et al., 2016) estimation, full-scale digitalization in non-residential construction will lead to annual global cost savings of $1.2 trillion (21%) in the engineering and construction phases and $0.5 trillion (17%) in the operation phases. However, governmental projects like transportation and power plants will achieve a 15%–25% decrease in construction and engineering expenses. The operation of construction will face an 8%–13% possible reduction for all infrastructure projects (Gerbert et al., 2016).
Data analysis and integration make new contemplation from the big data collections generated from the project life cycle. New virtual reality (VR) and augmented reality (AR) methods help to distinguish interconnections and collisions during the design and construction phases. Through the use of mobile communication and AR, project management can engage and communicate in real time and provide engineers with additional information on site. The digital technologies currently available on the market are easily usable throughout the project (Gerbert et al., 2016; Renz et al., 2016). Figure 2 shows the application areas of digital technologies with the construction industry.
FIGURE 2. Digital technologies applied in the E&C value chain (Gerbert et al., 2016; Renz et al., 2016; Elagiry et al., 2019).
Data integration technologies and their usefulness in reducing costs and optimizing asset use and value through more effective asset and demand management will drive demand for technologist skills (to design, implement, manage, and secure data systems) as well as informers (data analysts, economists, planners) who can crunch the data, develop policies, and communicate strategies within agencies and across their stakeholders. Designers themselves (engineers, spatial scientists), via their skills incorrectly interpreting data, are likely to provide a crucial link between technologists and informers. Consequently, the engineer of the future will likely have a different range of skills beyond traditional civil functions in a more technological, data-infused future. The digitalized uprising has affected the construction industry highly to some extent as the industry is working with a diverse and big amount of data (Bilal et al., 2016b).
3D printing, BIM, real-time data, cloud computing, AR, VR, drone scanning, BD, and The Internet of Things (IoT) are not all the tools used in today’s world; the integration and deployment of these technologies is the future (You and Feng, 2020). Utilization of these technologies is the solution to breaking the challenges faced by the construction industry; also, it requires stakeholders who think fast and take smart decisions for the gross profits of their organizations and customers (Chaurasia and Verma, 2020). This adoption lets the construction sector players from the project manager to the workers practice the right and quick decisions, optimize design and sector automation, and decrease the risk of construction. Finally, the construction duration can be shortened by boosting productivity (Hafifi Che Wahid et al., 2019).
In North America and Europe, 40% or more of the companies analyze big data, and these companies currently benefit from these analyses (Bange et al., 2015). One of its strongest benefits is strategic decisions with an increase (69%), better control over the operational process (54%), greater customer absorption (52%), and lower costs (47%). Moreover, these companies increased their revenue from data analysis by 8% and a decrease in spending by 10% (Bange et al., 2015). Analyzing and combining big data has many benefits, and many challenges have to be faced, for example, data privacy and data security, which are the most important factors to be wary of in those companies that initiated the use of big data in place (Bange et al., 2015).
In the construction industry, the current operations in the construction data platform depend on the exchange of data between the structural, architectural, electrical, and mechanical divisions; however, they involve challenges of interconnection. Thus, to achieve various usage cases such as the comprehensive integration of data from BIM with material data, geographical information, machine sensors, and much more, this large-scale operation will allow the construction industry to realize the automation of its operations, which will improve the overall efficiency of the project. Integrated data that are available to users on the Internet have proven effective (Pauwels and Terkaj, 2016).
Technologies such as Social–BIM and BIMcloud are used in many industries; this would improve the adoption of BD (Bilal et al., 2016b). Plageras et al. (2018) proved the usability of IoT based on BD for smart buildings. For infrastructure and smart city planning, the Master Data Management has been used as a data-based application (Ng et al., 2017). Various studies have demonstrated the big opportunities engaging the BD-related technology for construction (Barima, 2017).
The construction sector has some barriers to adopt innovation at a larger scale because of the risks that can be associated with anything new that has not been well tested (Yousif et al., 2018; Qubole, 2019). Therefore, there is still more room to improve in developing an effective data integration platform that helps in storing and processing interconnected and diversified project data. This platform led to the foundation for creating efficient applications for smart buildings (Bilal et al., 2016b).
Since the project data are collected by different units, methods, and times and stored in various formats, it is necessary to integrate the data (Foote, 2019; Ramlia et al., 2019). The integration process converts data into useful information and therefore can improve the decision-making process for all levels of management (Ribeiro et al., 2015). The BD consolidation project is a difficult challenge as contractors must provide the required data in appropriate forms and in real time to benefit from them in decision-making (Vinitha and Ravichandran, 2018). Three categories describe the evolution of an effective data management process in terms of collecting project data, storing data in the system, and using approaches to produce information and improving business decisions (Woldesenbet et al., 2016). Figure 3 shows two generations of data and information development involving the three categories to be used in the construction industry.
Data integration over many application domains is required to fetch data from one environment to another, in another meaning from the source to the destination. Thus, therefore, to grasp the current status around big data integration, the key literature in this area has to be analyzed. The ETL (Extract, Transform, and Load) process has been utilized to achieve this in traditional data repository domains (Alshiekh, 2021). The ETL process combines three essential procedures required to bring the data from the source to the desired destination. The functions are first to extract and read data from the source, second to transform the form and data integration of the extracted data so that it fits the requirements of the targeted destination, and last to load the data to the final destination repository (Wang et al., 2019).
Conventionally, batch processing and ETL have been utilized together in data warehouse environments (Hohensinn, 2021). Data warehouses provide users with a route to integrate data and information over various sources to analyze and visualize relevant data to their specified work focus. The ETL process is used to modify the data into the layout desired by the data warehouse. The modification happens in an intermediate spot before the data are loaded to the data warehouse. Many application vendors, for example Talend, IBM, Informatica, Oracle, Pentaho, and Microsoft, provided traditional ETL software applications tools (Ahmed et al., 2017).
After the appearance of BD, cloud computing, the internet of thing, BIM, and artificial intelligence the conventional data repository systems insufficiency to, which raise the necessity for ameliorating and utilizing more efficient and effective technologies (Motawa, 2017; Lu et al., 2019; Marzouk and Enaba, 2019). As the number of construction projects that were built over time increased, an enormous amount of data had to be handled (Martínez-Rojas et al., 2015). One of the fastest-growing technologies in several industries is data integration and its applications. Data integration can be defined as a series of computer technologies that can store, process, combine, and manage much more data than were handled before. From this next-generation technology, data can be easily gathered and analyzed. The utilization of data integration in an optimum way could unquestionably be the new limit of innovation to the construction industry. Therefore, data integration technology needs to be applied (Foote, 2019). Thus, therefore it is a solution to handle effectively such data with the existing methodology for data storage and processing.
The components of the BD integration application administer and organize data in new ways in contrast to the classical relational database, consequent to the necessity for scalability and the high ability for administering all types of structured, semi-structured, and unstructured data. Moreover, conventional ETL tools are improving to leverage the new BD features; however, conventional types of integration carry on new signification in the era of BD, and the data integration technologies lack a mutual platform that supports data fineness and tuning. As abovementioned, conventional data integration was done by utilizing batch processing, while BD integration can be done in real time. That could make the ETL process be reordered to turn into ELT in some conditions, so the data are extracted then loaded into distributed file systems and then transformed before being used (Bansal and Kagemann, 2015).
Johnson and Sargunam (2020) defined BD as a massive scale of data that is complex in structure and has difficulties in storing and analyzing the data. Also, when there is a huge amount of data, it will be hard to handle and perform an effective analysis by using traditional techniques or methods. A data preprocessing technique can overcome the problem of data inconsistency, incompleteness, scalability, timeliness, and data security, as shown in Figure 4. Ridzuan et al. (2021) analyzed the issues and challenges of big data in three main areas which are storage issues, management issues, and processing issues. Data management insights require getting all the data collected and linking it in a way to extract important information. In response to that, Rawat and Yadav (2021) demonstrated a theoretical review on big data including its application, opportunities, challenges, techniques, and problems. However, the technology needs to be continually progressing to address the ever-changing issues and challenges in BD.
In the construction industry in parallel with all other sectors, BD refers to the huge quantities of information that have been stored in the past and that continue to be acquired today. The construction industry is familiar to work with a tremendous amount of data; the exploitation of these data would be the new limits for construction industry growth (Ismail et al., 2018). The determination of BD integration could vary from diverse literatures. The Vs family is typically used to characterize BD as volume, velocity, variety, veracity, etc., as shown in Figure 5 (Adluru et al., 2015; Hashem et al., 2015; Patgiri and Ahmed, 2016).
Figure 5 shows the data integration parameters and their meanings, as well as their ability to add a more detailed meaning to the main elements. First is Velocity; it refers to the amount of data sources growing and the ratio of data produced over time extremely increasing, particularly after the outcrop of social media and the Internet of things. Second is Variety, which refers to extra data sources that imply that we have a wide variety in how data are stored. There is a wide range of forms like photos, texts, tracks, documents, and spatial data. Third is Veracity, which refers to the features listed above, giving rise to that we own various data qualities, so we can find fuzzy or anonymous data, especially from social media, and authorize users to publish these types of data (Halaweh and Massry, 2015). Last is Volume; it is the premier parameter of big data (Gandomi and Haider, 2015; Alavi and Gandomi, 2017). Currently, the number of connected devices, machinery, and people are higher than before. In contrast, this connection highly affected the number of data providers and the size of data widespread (Shaw et al., 2021).
It was found that construction projects are directly proportional to the development of digital data and their analysis to improve and increase profitability. A lot of BD applications are under construction to do this. Thus, it has become easier to use these tools, especially by increasing previous practical experiences that can be followed. At the same time, customers are hoping that construction companies will turn more toward big data, to follow the lead of other industries (Veras et al., 2021). Extracting facility management information digitally from BIM rather than going deep into large amounts of documents and paper drawings and handing it over to a new customer as fast to use digital files is a distinct application. The way to continuously profitably use BD depends on the use of such applications (Zhang et al., 2017).
Achieving a more accurate vision in the future relies on a solid database to monitor changes, mainly dependent on the development of BD (Li L. et al., 2017; Li T. et al., 2017). Recently, studies have shown how big data integration contributes as a technological compliance for massive information analysis on the unsuspected risk to occur. The future will largely depend on the concept of “datafication” and technological progress in terms of its creation and participation, as machines will communicate with each other via data networks, thereby reducing human participation in the process (Hasan et al., 2019).
The increasing adoption of digital technology and the rapid proliferation of data have spurred the application of data analytics and BD to drive smart project and asset management (Aibinu et al., 2019). Data analytics is an enabler to risk prevention and immediate mitigation. Knowing its great benefits, the construction industry is left behind in integrating big data and adopting digital technologies (Bilal et al., 2016b). Cabrera-Sánchez and Villarejo-Ramos (2020) found that companies’ ability to collect and store valuable data greatly affects the adoption of BD integration in various industries, including construction (Raguseo, 2018). The adoption of BD integration enhances productivity and organizational capabilities and also enhances the skills of collecting and analyzing huge amounts of data. Examples of digital technology adoption benefits in the Architecture, Engineering and Construction (AEC) industry are shown in Figure 6.
Changes in administrative processes or methods lead to risks related to the process of data integration, and those risks affect many industries, including the construction industry. The different skills represent an important factor for the emergence of a strong enthusiasm to adopt this modern technology, and successful case studies are a supporter of adding value to it (Madanayake and Egbu, 2019). The reluctance of the risk that the sponsors take to the big changes in their companies constitutes an obstacle to the spread of this technology, which in turn may lead to losing it. The risks involved should be mentioned, such as data security and privacy, costs involved in the process, and finally weak analyses (Oudjehane and Moeini, 2017). Among the challenges in this area are as follows (Shamsuddin and Hasan, 2015):
1. Inefficient data scientists who are responsible for the collection, analysis, and presentation of corporate data. Experts extract knowledge of the deep analyses of data found in dynamic databases.
2. The high cost will be an obstacle for companies to adopt this technology, as an investment in these operations requires a large and sophisticated integration platform.
3. Fear of sharing data, there is a fear of governments and companies in sharing information that could be useful to the world. Therefore, policies relating to the protection of data and information collected about them must be formulated.
Currently is the perfect time to digitize data, as many different use cases have benefited from this technology. A kickstart should be carried out with a pilot project that includes different processes and data types. It is also necessary to give workers in the field to contribute to this by giving suggestions to help improve the process. Furthermore, senior management is the main driver for the success of the process (Bange et al., 2015). It should be borne in mind that change must not be from within, meaning that current employees are trained to counter this wave of technological advances in data management. Data privacy and security are a challenge by classifying data and developing appropriate guidelines and responsibilities to improve its security, give it realism, and reduce uncertainty (Bange et al., 2015).
The data revolution has started putting its feet in the construction industry in synchronizing with the other industries that have hugely profited from BD integration. In this matter, the construction industry would take advantage of BD integration similar to anticipation by the other industries and services. As mentioned before, this will include improving efficiency and decision-making. Bilal et al. (2016a) substantiated that the expectations on the usability of big data integration in construction would be aggrandized as the exciting constituents. Consequently, the impulse of these constituents and elements would be the indicators to push the industry to the new limits of data-driven actions.
Based on Ismail et al. (2018), the impractical direction gain from the processes of analysis would be summarized as risk and security, venture management, energy utilization, decision-making, design structure, and resource arrangement. Of the above mentioned, BD integration for construction management was specified as the zone in which the intensification of researchers around is observed. Undoubtedly, the construction industry is a data-subordinate industry; therefore, data should be administered efficiently with the proper application and system to assure the success of any project. Current data revolution studies and implementation extracted from diverse studies are abstracted in Table 1 highlighting the context of research, significant keywords specified, and detail of the research area from the overview which stand out.
A web-based application for the BD integration is one of the best solutions to provide a platform for monitoring from manual to automatic, to obtain the information required to evaluate the construction, and to help in speeding and smarter important decisions, improving the construction performance in terms of the sustainable and green approaches. Also, Sun and Zhang (2020) concluded that the integration of smart cities and big data technologies leads to the growth of smart city constructions and enhances green city developments. Above all, it provides an effective platform for competitors to be players in sustainable construction industry development. This innovation makes data analysis and management more user-friendly and efficient, reduce data complexity, have ease in data collaboration, and have data integrity.
Although there are few data integration technologies on construction, not all the tools are fully utilized due to certain issues (Chonpitakwong et al., 2021; Srinavin et al., 2021), such as lack of awareness and responsibility; the apparent lack of use of modern technologies to integrate data to obtain useful information in the fastest time, with minimal effort and cost; lack of proper training; and lack of advertising the tools and monetary allocation to manage the projects introduced. Encouragement from top management is very important to enhance the application of integration tools in the industry. Besides, stakeholders should also be responsible for their projects and maximize the sustainability of their projects for the good of the people in the future. The use of such technologies and making the best use of information and BD integration technologies are two of the ways to achieve a sustainable project.
Although the construction industry produces a lot of data during the life cycle of the projects, the utilization of such technology in this industry lags the advancement made in other industries. The importance of construction data integration and up-to-date monitoring of the well-integrated data in the construction process of projects is essential.
To improve the adequacy of the construction, the construction players need to leverage these technologies for processing, analyzing, and storing the data. This research has analyzed the range to which the industry has utilized this integration technology. To this end, we reviewed the latest research that has been published in which the new techniques have been established in many fields of application. The fundamentals of BD integration technologies are demonstrated to assist the readers in understanding the complex theme. Significant, previous applications and the adoption of such technologies by many divisions of the industry are discussed.
This technology has been set to leverage the industry; this research outcome is the catalyzer in making the required knowledge to back up the implementation of data integration in the industry. This can drive the industry to prepare in improving the abilities in employing this integration technology and to support the organizational development to catch the arriving surge of the data revolution. Collecting and analyzing the data and information to present in one phase or from a project can be utilized as data and/or information in another phase, or information generated from a project can be utilized by another project to manage active projects and/or plan future projects. This phenomenon creates data and information to be used by the construction industry at all times. Furthermore, future research is needed for examining the integrated data to be used for global commercialization. Lastly, continuous research on the applicability of development of a mobile application to be connected to the big data integration systems to present real-time data with low cost and efforts on the data collection process in the construction industry is needed.
All authors listed have made a substantial and intellectual contribution to the work and approved it for publication.
The authors declare that the research was conducted in the absence of any commercial or financial relationships that could be construed as a potential conflict of interest.
All claims expressed in this article are solely those of the authors and do not necessarily represent those of their affiliated organizations, or those of the publisher, the editors, and the reviewers. Any product that may be evaluated in this article, or claim that may be made by its manufacturer, is not guaranteed or endorsed by the publisher.
The authors would like to thank and show their appreciation for UTM and the research team for their inspirational support and guidance from the beginning to the end.
Adluru, P., Datla, S. S., and Zhang, X. (2015). “Hadoop Eco System for Big Data Security and Privacy,” in 2015 Long Island Systems, Applications and Technology, Farmingdale, NY, USA, 1-1 May 2015, 1–6. doi:10.1109/LISAT.2015.7160211
Ahmed, V., Tezel, A., Aziz, Z., and Sibley, M. (2017). The Future of Big Data in Facilities Management: Opportunities and Challenges. Facilities 35 (13/14), 725–745. doi:10.1108/F-06-2016-0064
Aibinu, A. A., Koch, F., and Ng, S. T. (2019). Data Analytics and Big Data in Construction Project and Asset Management. Bepam 9, 474–475. doi:10.1108/BEPAM-09-2019-139
Akbar, S. N. (2018). Is Big Data the Most Trending Thing in Construction Now. Available at: https://geniebelt.com/blog/big-data-in-construction (Accessed September 9, 2021).
Akhavian, R., and Behzadan, A. H. (2015). Construction Equipment Activity Recognition for Simulation Input Modeling Using mobile Sensors and Machine Learning Classifiers. Adv. Eng. Inform. 29 (4), 867–877. doi:10.1016/j.aei.2015.03.001
Alavi, A. H., and Gandomi, A. H. (2017). Big Data in Civil Engineering. Automation in Construction 79, 1–2. doi:10.1016/j.autcon.2016.12.008
Alshiekh, M. M. A. (2021). Development of a Data Warehousing for Pharmaceutical Companies: A Case Study of the National Fund for Medical Supplies. Gezira State, Sudan: University of Gezira. dissertation. Available at: http://repo.uofg.edu.sd/handle/123456789/3910.
Augur, H. (2016). Big Data Is Transforming Commercial Construction. Available at: http://dataconomy.com/2016/07/big-data-is-transforming-commercial-construction/ (Accessed September 9, 2021).
Bange, C., Grosser, T., and Janoschek, N. (2015). Big Data Use Cases: Getting Real on Data Monetization. Business Application Research Center (BARC). Available at: http://barc-research.com/research/big-data-use-cases-2015/ (Accessed September 9, 2021).
Bansal, S. K., and Kagemann, S. (2015). Integrating Big Data: A Semantic Extract-Transform-Load Framework. Computer 48 (3), 42–50. doi:10.1109/MC.2015.76
Barbosa, F., Woetzel, J., Mischke, J., Ribeirinho, M. J., Sridhar, M., Parsons, M., et al. (2017). Reinventing Construction through a Productivity Revolution. Washington, DC: McKinsey Global Institute. Available at: www.mckinsey.com/industries/capital-projects-and-infrastructure/our-insights/reinventing-construction-through-a-productivity-revolution (Accessed September 9, 2021).
Barima, O. (2017). “BIG Data and Construction Value Delivery”, in Construction Industry: Quality Management, Potential Challenges and Improvement Strategies. New York: Nova Science Publishers, 113–135. ISBN: 978-1-53610-742-5.
Bernstein, P. (2017). How Big Data and VR in Architecture Will Greatly Improve Design. Available at: https://www.autodesk.com/redshift/big-data-vr-in-architecture/ (Accessed September 9, 2021).
Bilal, M., Oyedele, L. O., Akinade, O. O., Ajayi, S. O., Alaka, H. A., Owolabi, H. A., et al. (2016a). Big Data Architecture for Construction Waste Analytics (CWA): A Conceptual Framework. J. Building Eng. 6, 144–156. doi:10.1016/j.jobe.2016.03.002
Bilal, M., Oyedele, L. O., Qadir, J., Munir, K., Ajayi, S. O., Akinade, O. O., et al. (2016b). Big Data in the Construction Industry: A Review of Present Status, Opportunities, and Future Trends. Adv. Eng. Inform. 30 (3), 500–521. doi:10.1016/j.aei.2016.07.001
Cabrera-sánchez, J.-P., and Villarejo-Ramos, Á. F. (2020). Factors Affecting the Adoption of Big Data Analytics in Companies. Rev. Adm. Empres. 59, 415–429. doi:10.1590/S0034-759020190607
Caron, F. (2015). Data Management in Project Planning and Control. Ijds 1 (1), 42–57. doi:10.1504/IJDS.2015.069050
Castagnino, S., Rothballer, C., and Gerbert, P. (2016). What’s the Future of the Construction Industry. Cologny, Switzerland: World Economic Forum. Available at: https://www.weforum.org/agenda/2016/04/building-in-the-fourth-industrial-revolution.
Çetin, S., De Wolf, C., and Bocken, N. (2021). Circular Digital Built Environment: An Emerging Framework. Sustainability 13 (11), 6348. doi:10.3390/su13116348
Chau, K. W., Chan, I. Y., Lu, W., and Webster, C. (2018). Proceedings of the 21st International Symposium on Advancement of Construction Management and Real Estate. Singapore: Springer. ISBN 978-981-10-6190-5. doi:10.1007/978-981-10-6190-5
Chaurasia, S. S., and Verma, S. (2020). Strategic Determinants of Big Data Analytics in the AEC Sector: A Multi-Perspective Framework. Ceb 20 (4), 63–81. doi:10.5130/AJCEB.v20i4.6649
Chonpitakwong, B., Kusonkhum, W., Kusonkhum, W., Chaitongrat, T., Srinavin, K., and Charnwasununth, P. (2021). Hindrance of Applying Big Data Technology for Construction Management in Thai Government. Jait 12, 159–163. doi:10.12720/jait.12.2.159-163
Demirdöğen, G., Diren, N. S., Aladağ, H., and Işık, Z. (2021). Lean Based Maturity Framework Integrating Value, BIM and Big Data Analytics: Evidence from AEC Industry. Sustainability 13 (18), 10029. doi:10.3390/su131810029
Elagiry, M., Marino, V., Lasarte, N., Elguezabal, P., and Messervey, T. (2019). BIM4Ren: Barriers to BIM Implementation in Renovation Processes in the Italian Market. Buildings 9 (9). doi:10.3390/buildings9090200
Foote, K. D. (2019). Big Data Integration, the Fundamentals of Data Integration. Available at: https://www.dataversity.net/the-fundamentals-of-data-integration (Accessed September 9, 2021).
Gandomi, A., and Haider, M. (2015). Beyond the Hype: Big Data Concepts, Methods, and Analytics. Int. J. Inf. Manag. 35 (2), 137–144. doi:10.1016/j.ijinfomgt.2014.10.007
Gerbert, P., Castagnino, S., Rothballer, C., Renz, A., and Filitz, R. (2016). Digital in Engineering and Construction: The Transformative Power of Building Information Modeling. Boston, MA: The Boston Consulting Group Inc.. Available at: https://www.bcg.com/publications/2016/engineered-products-infrastructure-digital-transformative-power-building-information-modeling.
Guo, S. Y., Ding, L. Y., Luo, H. B., and Jiang, X. Y. (2016). A Big-Data-Based Platform of Workers' Behavior: Observations from the Field. Accid. Anal. Prev. 93, 299–309. doi:10.1016/j.aap.2015.09.024
Hafifi Che Wahid, C. M. F., Aminudin, E., Abd Majid, M. Z., Hainin, M. R., Mohd Satar, M. K. I., Mohd Warid, M. N., et al. (2019). Carbon Footprints Calculator of Highway Pavement Rehabilitation: The Quantification of Carbon Emissions Per Unit Activity. IOP Conf. Ser. Mater. Sci. Eng. 512, 012010. doi:10.1088/1757-899x/512/1/012010
Hafiz, A., Lukumon, O., Muhammad, B., Olugbenga, A., Hakeem, O., and Saheed, A. (2015). “Bankruptcy Prediction of Construction Businesses: Towards a Big Data Analytics Approach,” in 2015 IEEE First International Conference on Big Data Computing Service and Applications, Redwood City, CA, USA, 30 March-2 April 2015, 347–352. doi:10.1109/BigDataService.2015.30
Halaweh, M., and Massry, A. E. (2015). Conceptual Model for Successful Implementation of Big Data in Organizations. J. Int. Techn. Inf. Manage. 24 (2), 2. Available at: https://scholarworks.lib.csusb.edu/jitim/.
Hampton, T. (2015). Big Data Boosts Safety in Brazil. Available at: https://www.enr.com/articles/9640-big-data-boosts-safety-in-brazil?v=preview (Accessed September 9, 2021).
Han, K. K., and Golparvar-Fard, M. (2017). Potential of Big Visual Data and Building Information Modeling for Construction Performance Analytics: An Exploratory Study. Automation in Construction 73, 184–198. doi:10.1016/j.autcon.2016.11.004
Hart, A., Logie, R., and Jenkinson, T. (2018). Australia and New Zealand Roads Capability Analysis 2017-2027. Sydney, Australia: Austroads Ltd. Available at: https://www.onlinepublications.austroads.com.au/items/AP-R574-18.
Hasan, S. S., Zhang, Y., Chu, X., and Teng, Y. (2019). The Role of Big Data in China's Sustainable forest Management. Fer 1, 96–105. doi:10.1108/FER-04-2019-0013
Hashem, I. A. T., Yaqoob, I., Anuar, N. B., Mokhtar, S., Gani, A., and Ullah Khan, S. (2015). The Rise of “Big Data” on Cloud Computing: Review and Open Research Issues. Inf. Syst. 47, 98–115. doi:10.1016/j.is.2014.07.006
Hohensinn, D. (2021). Spooq: A Software Library for ETL Processes in Data Lakes. Linz, Austria: Universität Linz. Master's thesis. Available at: https://epub.jku.at/obvulihs/content/titleinfo/5763022.
Honarvar, A. R., and Sami, A. (2019). Towards Sustainable Smart City by Particulate Matter Prediction Using Urban Big Data, Excluding Expensive Air Pollution Infrastructures. Big Data Res. 17, 56–65. doi:10.1016/j.bdr.2018.05.006
IBM (2020). Data Integration. Available at: https://www.ibm.com/analytics/dataintegration (Accessed September 9, 2021).
Ibrahim, F. S., Esa, M. B., Esa, M., and A. Rahman, R. (2021). The Adoption of IOT in the Malaysian Construction Industry: Towards Construction 4.0. Ijscet 12 (1), 56–67. doi:10.30880/ijscet.2021.12.01.006
Ismail, S. A., Bandi, S., and Maaz, Z. N. (2018). An Appraisal into the Potential Application of Big Data in the Construction Industry. Int. J. BES 5 (2). doi:10.11113/ijbes.v5.n2.274
Janda, K. B., Patrick, J., Granell, R., Bright, S., Wallom, D., and Layberry, R. (2015). “A WICKED Approach to Retail Sector Energy Management,” in Proceedings of ECEEE Summer Study, France, May 15, 2015, 185–196. Available at: https://ora.ox.ac.uk/objects/uuid:180d450b109-a185a-143d186-189a193-ef180b70910ca70911.
Johnson, E., and Sargunam, S. S. (2020). Big Data: A New Way for Today’s Business Prospects and Challenges. Int. J. Conceptions Manage. Soc. Sci. 6 (2), 2357–2787. Available at: https://wairco.org/IJCMSS/November2020.html.
Kagan, P. (2019). Big Data Sets in Construction. E3s Web Conf. 110, 02007. doi:10.1051/e3sconf/201911002007
Kim, J.-S., and Kim, B.-S. (2018). Analysis of Fire-Accident Factors Using Big-Data Analysis Method for Construction Areas. KSCE J. Civ Eng. 22 (5), 1535–1543. doi:10.1007/s12205-017-0767-7
Knight, W. (2015). New Boss on Construction Sites Is a Drone. Cambridge, MA: MIT Technology Review, Massachusetts Institute of Technology. Available at: https://www.technologyreview.com/2015/08/26/10635/new-boss-on-construction-sites-is-a-drone.
Konikov, A., and Konikov, G. (2017). Big Data Is a Powerful Tool for Environmental Improvements in the Construction Business. IOP Conf. Ser. Earth Environ. Sci. 90 (1), 012184. doi:10.1088/1755-1315/90/1/012184
Koseleva, N., and Ropaite, G. (2017). Big Data in Building Energy Efficiency: Understanding of Big Data and Main Challenges. Proced. Eng. 172, 544–549. doi:10.1016/j.proeng.2017.02.064
Li, L., Hao, T., and Chi, T. (2017a). Evaluation on China's Forestry Resources Efficiency Based on Big Data. J. Clean. Prod. 142, 513–523. doi:10.1016/j.jclepro.2016.02.078
Li, T., Cui, Y., and Liu, A. (2017b). Spatiotemporal Dynamic Analysis of forest Ecosystem Services Using "big Data": A Case Study of Anhui Province, central-eastern China. J. Clean. Prod. 142, 589–599. doi:10.1016/j.jclepro.2016.09.118
Li, X., Wu, P., Shen, G. Q., Wang, X., and Teng, Y. (2017c). Mapping the Knowledge Domains of Building Information Modeling (BIM): A Bibliometric Approach. Automation in Construction 84, 195–206. doi:10.1016/j.autcon.2017.09.011
Li, X., Xu, J., and Zhang, Q. (2017d). Research on Construction Schedule Management Based on BIM Technology. Proced. Eng. 174, 657–667. doi:10.1016/j.proeng.2017.01.214
Lin, H., Liu, X., Wang, X., and Liu, Y. (2018). A Fuzzy Inference and Big Data Analysis Algorithm for the Prediction of forest Fire Based on Rechargeable Wireless Sensor Networks. Sustain. Comput. Inform. Syst. 18, 101–111. doi:10.1016/j.suscom.2017.05.004
Lu, W., Chen, X., Ho, D. C. W., and Wang, H. (2016). Analysis of the Construction Waste Management Performance in Hong Kong: the Public and Private Sectors Compared Using Big Data. J. Clean. Prod. 112, 521–531. doi:10.1016/j.jclepro.2015.06.106
Lu, W., Chen, X., Peng, Y., and Shen, L. (2015). Benchmarking Construction Waste Management Performance Using Big Data. Resour. Conservation Recycling 105, 49–58. doi:10.1016/j.resconrec.2015.10.013
Lu, W., Lai, C. C., and Tse, T. (2019). BIM and Big Data for Construction Cost Management. Oxfordshire: Routledge. doi:10.1201/9781351172325
Maaz, Z. N., Bandi, S., and Amirudin, R. (2018). A Contextual Parsing of Big Data Values to Quantity Surveyors. Int. J. BES 5 (3), 241–250. doi:10.11113/ijbes.v5.n3.311
Madanayake, U. H., and Egbu, C. (2019). Critical Analysis for Big Data Studies in Construction: Significant Gaps in Knowledge. Built Environment Project and Asset Management 9 (4), 530–547. doi:10.1108/BEPAM-04-2018-0074
Martínez-Rojas, M., Marín, N., and Vila, M. A. (2015). The Role of Information Technologies to Address Data Handling in Construction Project Management. J. Comput. Civil Eng. 30 (4), 04015064. doi:10.1061/(ASCE)CP.1943-5487.0000538
Marzouk, M., and Enaba, M. (2019). Analyzing Project Data in BIM with Descriptive Analytics to Improve Project Performance. Bepam 9, 476–488. doi:10.1108/BEPAM-04-2018-0069
Moeini, S., Oudjehane, A., Baker, T., and Hawkins, W. (2017). Application of an Interrelated UAS–BIM System for Construction Progress Monitoring, Inspection and Project Management. PM World J. VI (VIII), 1–13. Available at: https://pmworldlibrary.net/wp-content/uploads/2017/08/pmwj61-Aug2017-Moeini-Oudjehane-Baker-Hawkins-application-of-integrated-uas-bim-system.pdf.
Moreno, M. V., Dufour, L., Skarmeta, A. F., Jara, A. J., Genoud, D., Ladevie, B., et al. (2016). Big Data: the Key to Energy Efficiency in Smart Buildings. Soft Comput. 20 (5), 1749–1762. doi:10.1007/s00500-015-1679-4
Motawa, I. (2017). Spoken Dialogue BIM Systems - an Application of Big Data in Construction. Facilities 35 (13/14), 787–800. doi:10.1108/F-01-2016-0001
Moustafa, K. (2020). Make Good Use of Big Data: A home for Everyone. Cities 107, 102903. doi:10.1016/j.cities.2020.102903
Ng, S. T., Xu, F. J., Yang, Y., and Lu, M. (2017). A Master Data Management Solution to Unlock the Value of Big Infrastructure Data for Smart, Sustainable and Resilient City Planning. Proced. Eng. 196, 939–947. doi:10.1016/j.proeng.2017.08.034
Oudjehane, A., and Moeini, S. (2017). Big Data in Construction Projects Risk and Opportunity Management. Available at: http://pmsymposium.umd.edu/pm2017/wp-content/uploads/sites/3/2017/01/Big_Data_in_Construction_Projects_Risk_and_Opportunity_Management-Moeini.pdf (Accessed October 10, 2021).
Parisi, F., Fanti, M. P., and Mangini, A. M. (2021). Information and Communication Technologies Applied to Intelligent Buildings: a Review. ITcon 26 (25), 458–488. doi:10.36680/j.itcon.2021.025
Park, S.-W., Roh, M.-I., Oh, M.-J., and Kim, S.-H. (2019). Association Analysis of Piping Materials of an Offshore Structure Using Big Data Technology. J. Ship Prod. Des. 35, 220–230. doi:10.5957/JSPD.170058
Patgiri, R., and Ahmed, A. (2016). “Big Data: The V's of the Game Changer Paradigm,” in 2016 IEEE 18th International Conference on High Performance Computing and Communications; IEEE 14th International Conference on Smart City; IEEE 2nd International Conference on Data Science and Systems (HPCC/SmartCity/DSS), Sydney, NSW, Australia, 12-14 Dec. 2016, 17–24. doi:10.1109/HPCC-SmartCity-DSS.2016.0014
Pauwels, P., and Terkaj, W. (2016). EXPRESS to OWL for Construction Industry: Towards a Recommendable and Usable ifcOWL Ontology. Automation in Construction 63, 100–133. doi:10.1016/j.autcon.2015.12.003
Peiffer, E. (2016). 3 Concepts that Will Shape the Future of Construction. Available at: https://www.constructiondive.com/news/3-concepts-that-will-shape-the-future-of-construction/417049/ (Accessed October 10, 2021).
Plageras, A. P., Psannis, K. E., Stergiou, C., Wang, H., and Gupta, B. B. (2018). Efficient IoT-Based Sensor BIG Data Collection-Processing and Analysis in Smart Buildings. Future Generation Comput. Syst. 82, 349–357. doi:10.1016/j.future.2017.09.082
Qian, M., Yan, D., Liu, H., Berardi, U., and Liu, Y. (2020). Power Consumption and Energy Efficiency of VRF System Based on Large Scale Monitoring Virtual Sensors. Build. Simul. 13 (5), 1145–1156. doi:10.1007/s12273-020-0670-x
Qubole (2019). Big Data Analytics. Available at: https://www.qubole.com/big-data-analytics/ (Accessed October 10, 2021).
Raguseo, E. (2018). Big Data Technologies: An Empirical Investigation on Their Adoption, Benefits and Risks for Companies. Int. J. Inf. Manage. 38 (1), 187–195. doi:10.1016/j.ijinfomgt.2017.07.008
Ram, J., Afridi, N. K., and Khan, K. A. (2019). Adoption of Big Data Analytics in Construction: Development of a Conceptual Model. Bepam 9, 564–579. doi:10.1108/BEPAM-05-2018-0077
Ramlia, M. R., Noorb, Z. Z., Aminudina, E., Hainina, M. R., Zakariaa, R., Zina, R. M., et al. (2019). Carbon Footprint Assessment at Rest and Service Area of Malaysia Highway. Chem. Eng. 72, 73–78. doi:10.3303/CET1972013
Rawat, R., and Yadav, R. (2021). Big Data: Big Data Analysis, Issues and Challenges and Technologies. IOP Conf. Ser. Mater. Sci. Eng. 1022 (1), 012014. doi:10.1088/1757-899X/1022/1/012014
Redmond, A., El-Diraby, T., and Papagelis, M. (2015). “Employing an Exploratory Research Stage to Evaluate Green Building Technologies for Sustainable Systems,” in Proceedings of the International Conference on Civil, Structural and Transportation Engineering, Ottawa, Canada, May 4 – 5, 2015. Paper No. 291.
Renz, A., Solas, M., Almeida, P., Buhler, M., Gerbert, P., Castagnino, S., et al. (2016). Shaping the Future of Construction. A Breakthrough in Mindset and Technology. Geneva, Switzerland: World Economic Forum. Available at: https://www3.weforum.org/docs/WEF_Shaping_the_Future_of_Construction_report_020516.pdf.
Reyes Veras, P., Renukappa, S., and Suresh, S. (2021). Awareness of Big Data Concept in the Dominican Republic Construction Industry: an Empirical Study. Construction Innovation: Information, Process, Management. ahead-of-print, 1–19. doi:10.1108/CI-05-2021-0090
Ribeiro, A., Silva, A., and da Silva, A. R. (2015). Data Modeling and Data Analytics: a Survey from a Big Data Perspective. Jsea 08 (12), 617–634. doi:10.4236/jsea.2015.812058
Ridzuan, F., Wan Zainon, W. M. N., and Zairul, M. (2021). A Thematic Review on Data Quality Challenges and Dimension in the Era of Big Data. Proc. 12th Natl. Tech. Semin. Unmanned Syst. Techn. 2020, 725–737. doi:10.1007/978-981-16-2406-3_56
Sadhu, R. (2016). Big Data for Construction: How it Can Help You Deliver Your Project on Time. Available at: https://www.planacademy.com/big-data-construction/ (Accessed October 10, 2021).
Santos, B., Soares, A., Nguyen, T.-A., Min, D.-K., Lee, J.-W., and Silva, F.-A. (2021). IoT Sensor Networks in Smart Buildings: A Performance Assessment Using Queuing Models. Sensors 21 (16), 5660. doi:10.3390/s21165660
Shahi, K., McCabe, B. Y., and Shahi, A. (2019). Framework for Automated Model-Based E-Permitting System for Municipal Jurisdictions. J. Manage. Eng. 35 (6), 04019025. doi:10.1061/(ASCE)ME.1943-5479.0000712
Shamsuddin, S. M., and Hasan, S. (2015). “Data Science vs Big Data @ UTM Big Data centre,” in 2015 International Conference on Science in Information Technology (ICSITech), Yogyakarta, Indonesia, October 27, 2015, 27–28. doi:10.1109/ICSITech.2015.7407766
Shaw, S., Rowland, Z., and Machova, V. (2021). Internet of Things Smart Devices, Sustainable Industrial Big Data, and Artificial Intelligence-Based Decision-Making Algorithms in Cyber-Physical System-Based Manufacturing. Econ. Manag. Financ. Mark. 16 (2), 106–116. doi:10.22381/emfm16220217
Skibniewski, M., and Golparvar-Fard, M. (2016). Toward a Science of Autonomy for Physical Systems: Construction. New York, NY: Cornell University. arXiv preprint arXiv:1604.03563.
Srinavin, K., Kusonkhum, W., Kusonkhum, W., Chonpitakwong, B., Chaitongrat, T., Leungbootnak, N., et al. (2021). Readiness of Applying Big Data Technology for Construction Management in Thai Public Sector. Jait 12, 1–5. doi:10.12720/jait.12.1.1-5
Sun, M., and Zhang, J. (2020). Research on the Application of Block Chain Big Data Platform in the Construction of New Smart City for Low Carbon Emission and green Environment. Comput. Commun. 149, 332–342. doi:10.1016/j.comcom.2019.10.031
Vinitha, A., and Ravichandran, P. (2018). Big Data Processing on Educational Data Mining Using Pyspark with Jupyter Notebook. Johor, Malaysia: Universiti Teknologi Malaysia. Master's thesis. Available at: http://eprints.utm.my/id/eprint/81375/1/VinithaRavichandranMFC2018.pdf.
Wang, Z., Xue, M., Wang, Y., Song, M., Li, S., Daziano, R. A., et al. (2019). Big Data: New Tend to Sustainable Consumption Research. J. Clean. Prod. 236, 117499. doi:10.1016/j.jclepro.2019.06.330
Woldesenbet, A., Jeong, H. D., and Park, H. (2016). Framework for Integrating and Assessing Highway Infrastructure Data. J. Manage. Eng. 32 (1), 04015028. doi:10.1061/(ASCE)ME.1943-5479.0000389
Wood, C. (2016). Betting on Big Data: How Construction Firms Are Leveraging Digitized Job Sites. Available at: https://www.constructiondive.com/news/betting-on-big-data-how-construction-firms-are-leveraging-digitized-job-si/431047/ (Accessed October 10, 2021).
Yang, J., Park, M.-W., Vela, P. A., and Golparvar-Fard, M. (2015). Construction Performance Monitoring via Still Images, Time-Lapse Photos, and Video Streams: Now, Tomorrow, and the Future. Adv. Eng. Inform. 29 (2), 211–224. doi:10.1016/j.aei.2015.01.011
You, Z., and Feng, L. (2020). Integration of Industry 4.0 Related Technologies in Construction Industry: a Framework of Cyber-Physical System. IEEE Access 8, 122908–122922. doi:10.1109/ACCESS.2020.3007206
Yousif, O. S., Majid, M. Z. A., Aminudin, E., Zakaria, R., Wahid, C. M. F. H. C., Neardey, M., et al. (2018). “Energy and Economic Benefits of LED Adoption in Malaysia Highway Lighting System,” in 4th International Conference on Low Carbon Asia (ICLCA 2018), Johor Bahru, Malaysia, October 24–26, 2018, 142. Available at: https://www.researchgate.net/profile/Omar-Yousif-4/publication/329505391_Energy_and_Economic_Benefits_of_LED_Adoption_in_Malaysia_Highway_Lighting_System/links/5c87889992851c831973e96e/Energy-and-Economic-Benefits-of-LED-Adoption-in-Malaysia-Highway-Lighting-System.pdf.
Zhang, Y., Luo, H., and He, Y. (2015). A System for Tender price Evaluation of Construction Project Based on Big Data. Proced. Eng. 123, 606–614. doi:10.1016/j.proeng.2015.10.114
Zhang, Y., Ren, S., Liu, Y., Sakao, T., and Huisingh, D. (2017). A Framework for Big Data Driven Product Lifecycle Management. J. Clean. Prod. 159, 229–240. doi:10.1016/j.jclepro.2017.04.172
Keywords: big data, construction digitalization, data integration, project management, technological innovation
Citation: Yousif OS, Zakaria RB, Aminudin E, Yahya K, Mohd Sam AR, Singaram L, Munikanan V, Yahya MA, Wahi N and Shamsuddin SM (2021) Review of Big Data Integration in Construction Industry Digitalization. Front. Built Environ. 7:770496. doi: 10.3389/fbuil.2021.770496
Received: 03 September 2021; Accepted: 25 October 2021;
Published: 18 November 2021.
Edited by:
João Carlos Gonçalves Lanzinha, University of Beira Interior, PortugalReviewed by:
Bruno Barzellay Ferreira Da Costa, Federal University of Rio de Janeiro, BrazilCopyright © 2021 Yousif, Zakaria, Aminudin, Yahya, Mohd Sam, Singaram, Munikanan, Yahya, Wahi and Shamsuddin. This is an open-access article distributed under the terms of the Creative Commons Attribution License (CC BY). The use, distribution or reproduction in other forums is permitted, provided the original author(s) and the copyright owner(s) are credited and that the original publication in this journal is cited, in accordance with accepted academic practice. No use, distribution or reproduction is permitted which does not comply with these terms.
*Correspondence: Omar Sedeeq Yousif, b21hci5hbWVlbjkzQGdtYWlsLmNvbQ==
Disclaimer: All claims expressed in this article are solely those of the authors and do not necessarily represent those of their affiliated organizations, or those of the publisher, the editors and the reviewers. Any product that may be evaluated in this article or claim that may be made by its manufacturer is not guaranteed or endorsed by the publisher.
Research integrity at Frontiers
Learn more about the work of our research integrity team to safeguard the quality of each article we publish.