- 1School of Built Environment, Engineering and Computing, Leeds Beckett University, Leeds, United Kingdom
- 2Centre for Sustainable Smart Cities, Central University of Technology, Bloemfontein, South Africa
- 3Department of Construction Economics and Management, University of Cape Town, Cape Town, South Africa
- 4Department of Quantity Surveying, Bells University of Technology, Ota, Nigeria
The contribution of smart campuses to smart cities’ development and vice versa has been elucidated in extant literature. The micro-transfer of smart technologies and probable procurement and contracting models remain critical for such contributions to take place. This study used a systems thinking approach to establish the interrelationships existing between smart campus technologies which can be scaled towards the development of smart cities and assess the critical failure factors negating the micro-transfer of these technologies to smart city development initiatives in developing countries. To achieve its objective, the study adopted a phenomenological research design wherein qualitative data was elicited from a purposively selected sample of seven interviewees in South African Universities. The next phase of the analysis involved a thematic approach for the production of a causal loop diagram (CLD) reflecting the interrelationships between the abovementioned facets and the associated impact on the transference of knowledge and technologies from smart campuses to smart cities. This CLD was subsequently validated by a cohort of five experts. Findings from the validation phase were incorporated in an improved CLD which provided different archetypes for engendering successful transference. This study holds salient implications for universities, cities and other stakeholders seeking to engage in a quadruple helix innovative arrangement for smart campus/city development. This study concluded that smart campuses could act as living labs for future smartness of cities globally. Government funding and willingness to produce smart cities from campuses is a fundamental feature of creating smart infrastructure in cities.
Introduction
Higher education institutions are expected to contribute their quota in achieving the UN Sustainable Development Goals. This responsibility of the university will be achieved by making concerted efforts directly and indirectly and paying adequate attention to protecting the environment, social inclusion and comprehensive economic growth. One of the many ways universities can add value to sustainable development goals is to establish smart campus solutions (Moraes et al., 2020). Studies have shown that smart campus solutions provide technologies for achieving the following SDGs: clean water and sanitation (Kuo et al., 2018), affordable and clean energy (Weng et al., 2019; Yuliansyah et al., 2019), sustainable cities and communities (Liu et al., 2017; Subbarao et al., 2019), responsible consumption and production (Lo, 2019) and climate action (Liu et al., 2017; Rodrigues et al., 2019). Muhamad et al. (2017) reckoned that several definitions of smart campus in literature are based on technology, smart city and business process. A smart campus is a result of the collaboration of technologies such as Big Data, cloud computing, Internet of Things (IoT), Internet and high-performance computing, virtualisation, mobile network and social network, sensors and common communication interfaces, 6LoWPAN (IPv6 over Low Power Wireless Personal Area Networks); and RFID (Radio-Frequency Identification) (Hu and Yan 2016; Wei 2016; Zhang and Jiang, 2018, Arshad et al., 2017; Guo and Zhang, 2015; Ivanovski, 2015; Majeed and Ali, 2018; Nie, 2013; Luo, 2018). However, a smart campus embodies the interactions of virtual and physical spaces to create new experiences by using intelligent devices on an IoT network to ensure operational efficiency (Petcovici and Stroulia, 2016). These innovative experiences arising from the utilisation of smart technologies improve students’ lives by automating workflows, conserving resources, eliminating waiting time and most importantly, improving student services within an academic environment.
Vasileva et al. (2018) reckoned that campuses are smaller versions of cities. The similarity is related to the facilities and infrastructure such as retail outlets, buildings, energy generation/distribution, waste treatment, transport and road infrastructure, medical outlets and sports centres. In some instances, tertiary institutions are bigger than cities. This assertion is confirmed by Villegas-Ch, Palacios-Pacheco and Luján-Mora (year) who argued that the enclosed academic environment for learning, such as universities, can be huge compared to cities. Either way, the two concepts are similar in the challenges faced by their users and the associated delivery of improved services and satisfaction. The use of digital technologies in campuses making it “smart” in a quest to make it more functional will aid the evolution of more smart cities. Popoola et al. (2018) posited that smart campuses have some interrelationships with the cities where they are located in terms of digital infrastructure and innovation. The increase in smart campuses with its associated infrastructures is the springboard for establishing more smart cities to attain sustainability goals, among others.
Research Aim and Novelty
The study sets out to develop a model which examines the interrelationship between infrastructure elements of smart campuses as a tool for smart cities in developing countries using a system thinking approach. The novelty of this study lies in the research analytical method of systems thinking archetypes that can be used to micro-transfer smart infrastructure from smart campuses to cities in developing countries.
The remaining part of this article is presented as follows: Literature Review presents the technology and IT infrastructure for the efficient smart campus and smart learning and teaching analytics. Methods and Materials reveals the research approach for carrying out the study; Analysis and Results presents the results and discussion of findings. The last part of the article is the implications and conclusion for the study.
Literature Review
Smart Campus Infrastructure
The concept of a smart city is based on the principle of smart infrastructure, where there is an interconnection of digital components to generate and use data intelligently to improve the performance of systems through the efficient use of resources (UN, 2016). The core infrastructural elements needed for smart city solutions include but are not limited to a sustainable environment, comprehensive IT digitalisation, efficient waste management, energy management, effective urban mobility, e-governance, telemedicine/education and constant electricity supply as identified by Omotayo et al. (2021) as presented the framework of Figure 1. Some of the smart city infrastructures within a city allow smart campus development within the same city. The smart city infrastructural systems become the platform for establishing mini-cities in the form of co-existence between the university and its communities.
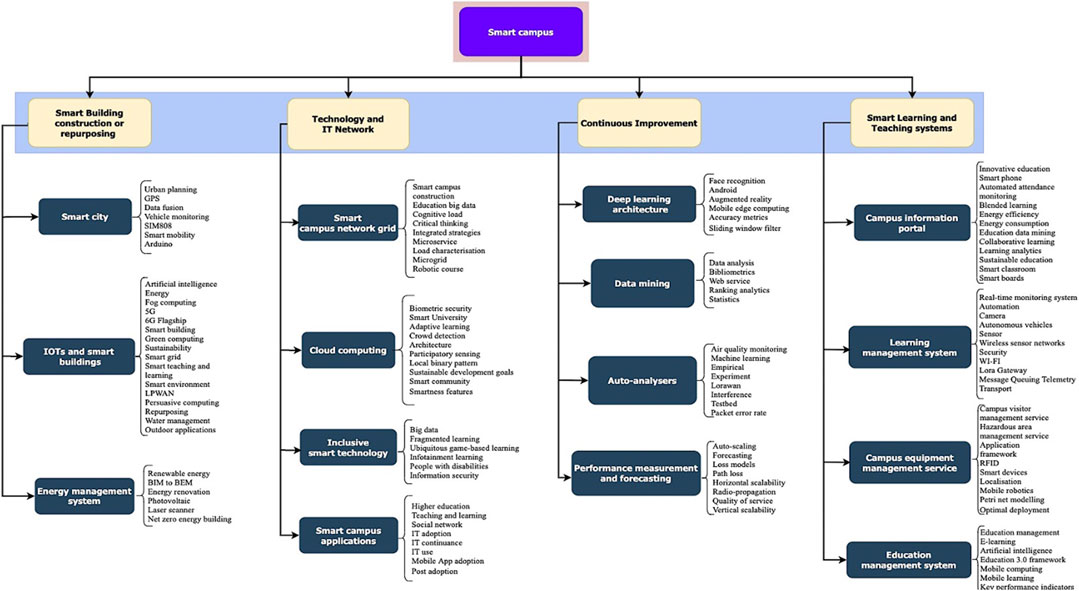
FIGURE 1. Elements of a smart campus infrastructure (Source: Omotayo et al., 2021).
Smart campus initiatives are based on the concept of IoT and its relative interaction with educational buildings through smart network grids (Omotayo et al., 2021), utilising and controlling a large volume of data remotely in the process. The initiative is premised on the construction of smart buildings or the repurposing of old campus buildings to create a sustainable environment essential for academic activities (Chen et al., 2017). The study of Omotayo et al. (2021) revealed that IoT-smart building interaction necessary for the smart campus is driven by 5G and 6G flagship, smart grid, artificial intelligence, fog computing, green computing, repurposing, energy management, smart teaching and learning. Using the technology of IoT and smart classrooms, social benefits arising from the management of resources within the university ecosystem as well as the efficient and effective citizen and e-governance services to the society emerge (Zhang L. et al., 2020).
Moura et al. (2021) argued that the use of large educational buildings and other facilities encourages high energy consumption costs within campuses, prompting the need for efficient energy management systems (EMS) through smart campus solutions. However, the integration of IoT provides monitoring and controlling platforms to evaluate the generation and demand of electricity supply, achieve energy use sustainability, and adopt smart devices to control loads through data. Cloud computing coupled with machine learning analysers is an essential component for the effective running of EMS within a smart campus network grid (Omotayo et al., 2021). The significant drivers are building information modelling to building energy model, renewable energy, photovoltaic, laser scanner and net-zero energy. Conversely, the most important driver in establishing an EMS for refurbishing an existing education building towards a smart campus is the application of BIM in BEM (Mazza, 2015; Michailidis et al., 2018).
Technology and IT Infrastructure
The conceptual framework of the infrastructural element of smart campus developed by Omotayo et al. (2021) highlighted the components of technology and IT infrastructure for a smart campus to include smart campus construction, micro-grid, robotic course, and cognitive course load, critical thinking, microservice, integrated service and load characterisation. According to Alrashed (2020), smart technologies are employed by educational institutions to address the problems related to the privacy of users, technology uptake and climate change through the development of smart network grids. Several authors including Chenoweth (2020), reckoned that the use of micro-grid in university campuses is on the rise as a recent development in the quest to achieving smart campus solutions.
The smart, inclusive technologies technology covers all students, especially students with disabilities, supports equality among students and the efficient management of teaching and learning within the academic environment. According to Omotayo et al. (2021), smart buildings in smart campuses reinforce big data principles, encourage infotainment learning and ubiquitous game-based learning for disabled students, and most notably, fragmented learning participatory involvement of students with diverse backgrounds. The concept of smart campus applications is made more effective with mobile apps by major stakeholders, academic and non-academic staff with and including students. Omotayo et al. (2021) hinted that IT continuance, IT adoption, social networks, and IT use are the significant drivers for smart campus applications. Other notable variables related to smart campus applications are higher education, teaching and learning, information systems and applications.
Continuous Improvement of the Smart Campus
The sub-elements of deep learning architecture in data mining processes in the smart campus are virtual reality, attendance systems, augmented reality, android, mobile edge computing, accuracy metrics, sliding window filters and face recognition (Omotayo et al., 2021). One of the major features of deep learning architecture is face recognition, which ensures campus security and safety. In the same vein, virtual and augmented reality in teaching and learning has many benefits, especially to ensure interaction and arouse study interest in different subject matters.
Data mining involves discovering valuable information, knowledge and pattern behind a large volume of data using machine learning to make decisions and measure performance (Qin and Chi, 2020; Vivi et al., 2015; Jiang et al., 2017). Most university campuses use data mining to assess student’s grades, evaluate student’s involvement in class activities, library usage, and campus applications. Similarly, smart campus data analytics are used to measure the presence of the staff activities and researches online. The drivers of data mining in smart campuses are web service, data analysis, statistics, random, ranking analytics, bibliometric and cite score. Auto-analysers are relevant to the effectiveness of smart buildings within a smart campus network infrastructure. Machine learning, experiment, testbed, interference, air quality monitoring and packet error rate are auto-analyser variables used to assess the continuous and active performance of the buildings within the territory (Omotayo et al., 2021). The concept of auto-analysers as an important component in smart campus studies is new, although advances in research in the area is on the increase.
Performance measurement and forecasting is an essential element of smart campus infrastructure as it involves understanding the smart grid network and other smart applications (Bilau et al., 2015). The large volume of data at the disposal of the university is processed and used for establishing the level of performance in academic-related activities. The key variables associated with performance measurement and forecasting in the smart campus include path loss, auto-scaling, loss models, forecasting, horizontal scaling, quality of service, radio propagation and vertical scalability (Omotayo et al., 2021). The state of the smart campus infrastructure can be measured with the quality of service, use of path loss and loss model. While performance measurement is suitable for assessing energy consumption in university buildings, forecasting seeks to evaluate energy future usage in those campus buildings and assess the efficiency of smart technologies in ensuring quality services and learning activities of the students. Putman et al. (2019) opined that horizontal scalability ensures more resources are added to existing smart campus network infrastructure. Vertical scalability is responsible for resizing servers and sensors to add more features for the efficiency of the smart network infrastructure.
Smart Learning and Teaching Analytics
Campus information portal (CIP) is an integrated platform in campus life that is set out to collect and release information to ensure efficiency, personalisation, security and other important campus resources (Zhicheng and Feng, 2018). Omotayo et al. (2021) enumerated that energy consumption and efficiency, blended learning, education data mining, smart classroom, collaborative learning, sustainable education, and learning analytics are key ingredients of CIP. One of the many features of CIP is the ability to oversee efficiency in energy consumption, considering the huge cost of energy worldwide. The data of the amount of energy used within campus should be available and ways to achieve energy efficiency. In addition, CIP should provide the platform for blended and hybrid learning for the teaching of students, which the current times allow (Zhang L. et al., 2020; Zhang Y. et al., 2020).
Learning management system (LMS) is a recent technology adopted in university education to create course content, its associated delivery and management (Mershad and Wakim, 2018). The centralised technology that incorporates instructional features involves student administration, evaluating attendances, and assessing student submissions. The major components of LMS in a smart campus are real-time monitoring, wireless fidelity (Wi-Fi), camera, automation, autonomous vehicle, sensor, wireless sensor network, and it has become an integral part of the smart campus. The technology of LMS assists in the monitoring of student’s attendance, hinged on good Wi-Fi systems and sensor networks to aid effective learning scenarios (Sun et al., 2018). Transportation for students in a big campus environment could be a problem that can be resolved by providing autonomous vehicles within the university premises. In addition, the provision of an effective Wi-Fi system is a bedrock for efficient IoT for any campus environment. The use of a close circuit camera will help ensure safety on smart campuses, which aid the security of students and staff.
Campus equipment management services (CEMS) include components in the smart campus such as mobile robotics, optimal deployment, application framework, radio frequency identification, smart devices, Petri net modelling, localisation and hazard area management service (Omotayo et al., 2020a). Zhou et al. (2020) reckon that RFIS is an important ingredient for establishing smart campus solutions. The hazard area management service and campus visitor management systems ensure on-site and online campus security (Luo, 2018; Liang, 2020).
The last element of smart learning and teaching analytics considered significant in smart campus infrastructure is the education management system (EMS). As reported in Omotayo et al. (2021), the related components of EMS are mobile computing, e-learning, mobile learning, and artificial intelligence. The adoption of mobile computing and learning is further enhanced with smart mobile gadgets and devices by lecturers and students (Li, 2020). The management team of smart campus are to provide enabling framework and environment powered by mobile computing and artificial intelligence to enhance smart learning by students.
Research Gaps and Scope of the Study
Recent studies by Min-Allah and Alrashed (2020); Omotayo et al. (2021); Zhicheng and Feng, (2018); Zhou et al. (2020); and Moura et al. (2021) reviewed and developed frameworks for smart campuses. The aforementioned studies did not consider the typologies of transferring smart cities to cities. The relationship between the attributes of smart campuses have only been identified and not studied. This study therefore intends to develop transferrable attributes of smart campuses to cities in developing countries with a focus on South African Universities. The causal relationships between smart campus features will be studied for the purpose of understanding what can be transferred to a larger environment.
Methods and Materials
The Surveys may be quantitative or qualitative (De Vaus, 2013). Qualitative data in the form of survey interviews aims to extract opinions about a subject topic, issue, or knowledge outside the social phenomenon (Folkestad, 2008; De Hoyos and Barnes, 2012). Hence, interviews aid the creation of new knowledge from a social constructivist paradigm. Figure 2 expresses the research protocol adopted for this investigation visually.
The first phase of this study reviewed the latest knowledge in smart campus research in a literature review and collected interview data. The outcome of the literature review informed the interview question design and sampling approach. Hence, the Theoretical sampling approach was used in this study. Theoretical sampling is a non-probabilistic sampling that targets the strategic individual with the right knowledge or opinion about the aim of a study out of a sample population (De Vaus, 2013). In this study, a theoretical sampling technique was used to collect interview data from two Universities in South Africa. Seven (7) respondents were interviewed, and the interview was discontinued at reaching the saturation point. Guest et al. (2006) and Weller et al. (2018) noted that saturation point is attained in an interview when the interviewees provide the same set of responses, and it will be redundant to have another interviewee further. The profile of the respondents is explained in Interviewee Demography. The data collected from the interviewees were then analysed qualitatively.
The second phase of this investigation analysed the interview with the aid of NVIVO content analysis. The themes were derived from the process of analysis and discussed descriptively. The categorisation of the themes was led to the third phase of this study.
In the third phase, after creating the themes through NVIVO content analysis, endogenous and exogenous variables were compiled for the causal loop diagramming with systems thinking. The development of a causal loop diagram for smart campuses to cities was validated in the next stage.
The validation of the causal loop diagram developed from the qualitative content analysis of responses provided by experts. The validation phase employed the opinions of five (5) experts from the United Kingdom, South Africa, and Pakistan, as explained in Smart Campus Infrastructure. The causal loop diagram was sent to the experts who provided their opinions for subsequent improvement of the final model.
The next sections addressed the first phase of this investigation as derived from the data collection process and analysis. A new causal loop diagram was produced in the fifth and final phases, and the archetypes extracted from the model were discussed. The implications of the archetypes on the realisation of smart cities in developing countries were further discussed with the aid of a force field diagram.
Interviewee Demography
All seven interviewees in this study are based in South African Universities as shown in Table 1. The respondents are in administrative positions in the university. One of the interviewees, SUA5, is the university’s deputy vice-chancellor and has been involved in strategy formulation. SUA6 is the director of estates and facilities management. All respondents have BSc and MSc degrees with over 2 years in the role they occupy in their various Universities.
Two (2) of the interviewees classified their university as a smart campus. However, the remaining five (5) find it difficult to categorically classify their campus as a smart campus even though they have some available technologies. Notwithstanding, the questions asked the interviewees were about:
• The applicability of smart technologies on campus.
• Procurement, contracting and construction of smart infrastructure
• How to micro-transferring smart campus technology to cities
The interview outcomes, which were partly conducted through computer-mediated interviews using MS Teams, were analysed using NVIVO and presented in the next section.
Analysis and Results
NVIVO content analysis the interview data by following similar protocols adopted by Rapley (2001); Roulston (2014); and Omotayo et al. (2019) whereby:
a) Transcriptions were conducted, and coding of individual contexts was identified and associated with the aim of the study.
b) Themes were created after categorising each context based on the aim of the study.
c) The themes are broken down into nodes that are related to the aim of the study.
d) Cases are created from the nodes.
e) Interpretation of the cases is conducted descriptively to understand their implications for the study.
The themes produced from the analysis are smart campus infrastructure, procurement, contracting and construction, and Critical success factors for micro-transferring smart campus technologies to cities. Each of the themes under the categories was discussed in the sections below.
Smart Campus Infrastructure
The seven (7) themes in this section were drawn from the outcomes of the thematic analysis. Internet-of-things (IoT); Building management systems; Campus Energy management systems; Security systems with facial recognition technology; Performance measurement; Class instruction and engagement platforms; and Smart renewable energy grid were identified as the applicable technologies for the smart campus.
Theme 1- Internet-of-Things (IoT)
Wireless fidelity (WIFI) working with devices such as security sensors, scanners, facial recognition technology, renewable energy system, smart meters and other education devices on campus are considered as important in the concept of smart campus (Abuarqoub et al., 2017; Sari et al., 2017). Interviews SUA2, SUA6, SAU7 all agreed that IoT is essential to attaining a smart campus. However, interviewee SUA3 stated that “There is no, too little IoT installed on campus.” SUA4 also noted that “IoTs are marginally used for teaching and learning.” SUA3 and SUA4 made it clear that IoT is not just about WIFI but the devices used for teaching and learning, absent in South African Universities. IoT forms the basis of smart technology of smart campuses, and it must be available to meet the requirements of a smart campus.
Theme 2- Building Management Systems
SUA5 and SUA6 identified smart lighting, real-time occupancy systems, water leak detection with smart sensors, and self-service technologies as components of a building management system in a smart campus. Muhamad et al. (2017) and Prandi et al. (2020) identifies sensors as the basic smart building management system. Therefore, a smart campus must be fitted with sensors to monitor and manage the energy performance, facilities usage, and overall performance of buildings on campuses.
Theme 3- Campus Energy Management Systems
The energy management system of campuses must include sensors and smart devices for the monitoring and data storage of indoor air quality and parking spaces mapping (Aman et al., 2013; Talei et al., 2017). SUA5 noted that indoor air quality management systems and parking space mapping are essential technologies on campuses hoping to become smart.
Theme 4- Security System With Facial Recognition Technology
Mobile and contactless payment devices, smart IP video cameras, facial recognition technology, geofencing technologies and smart locks are attributes of a security system as identified by participant SUA6. Facial recognition and security applications may be sourced or developed by the IT service team on campus specifically for the University (Thorat et al., 2010; Devitsyna et al., 2019; Andrejevic and Selwyn, 2020). The security of campus users is essential, and there is a need to ensure that campuses adapt to the standards of using smart security systems.
Theme 5- Performance Measurement
Big data is an important component of smart campuses, and the application of data mining in evaluating the performance of the smart infrastructure is essential for continuous improvement (Omotayo et al., 2021). To support this theme, SAU1 noted that “…energy and water data for monitoring and optimising building performance….” However, SUA4 noted that “…discussions underway to plan the use for forecasting.” SAU1’s identification of energy and waste data monitoring and optimisation is geared towards improving building performance. SUA4’s opinion of performance measurement is applicable in forecasting energy usage and building performance. The existence of smart infrastructure on campus must be improved through constant performance measurement using data harnessed on campus.
Theme 6- Class Instruction and Engagement Platforms
Smartboards, campus information portals, equipment management, education and learning management system are essential attributes of a smart campus (Omotayo et al., 2021; Min-Allah and Alrashed, 2020). SAU5 stated that the aforementioned online platform ensures student engagement, especially when they are away from the university due to social restrictions. Classroom instruction and online engagement platforms for teaching and learning may be procured or developed by the IT service on campus.
Theme 7- Smart Renewable Energy Grid
Sustainable development is an important aspect of smart campus development (Althobaiti et al., 2020; Min-Allah and Alrashed, 2020; Moghayedi et al., 2021). SAU5 and SAU6 agreed that as part of sustainable building construction on campus, waste management, renewable energy such as solar photovoltaic panels and smart wastewater management systems are important attributes of the smart renewable energy grid and smart campuses.
Procurement, Contracting and Construction
All seven interviewees noted that contractors are required to supply and install smart campus infrastructure. The themes drawn from the procurement contracting and construction were analysed to produce the themes of competitive procurement, design and management, and contractual arrangement.
Theme 1- Competitive Procurement
SAU1, SAU2, SAU5 and SAU6 used traditional procurement and competitive design and build, to describe the strategic process of acquiring new smart campus infrastructure. Competitive tendering is also applied in this process to ensure transparency and open competition (Althobaiti et al., 2020; Min-Allah and Alrashed, 2020). SAU2 noted that “there is a good document procurement process with different tiers based on contract value.” SAU3 noted that invitation tenders are advertised in government gazettes to identify the most suitable contractors competitively. It is important to provide a structured and strategic arrangement for identifying and selecting specialist contractors in smart campus procurement.
Theme 2- Design and Manage
SAU1, SAU5 and SAU6, noted that the IT service might use manufacturer extended responsibilities to supply and install smart devices on campus. This arrangement is a design and manage procurement strategy that uses suppliers who are mostly manufacturers to not only have the ability to supply and install but also engage in the maintenance of smart campus infrastructure and the IT service.
Theme 3- Contractual Arrangement
The traditional contractual arrangements using the Joint Contract Tribunal (JCT), New Engineering Contract (NEC), and the International Federation of Consulting Engineers (FIDIC) contract suits are being used in the procurement and construction of smart campuses (Omotayo et al., 2021). SAU5 supports this theme by identifying JCT 2016, NEC4 and FIDIC as standard contract suits applied in smart campus procurement and contractual arrangement. Within the aforementioned standard forms of contracts and responses from the interview, lump sum and fixed-price contracts are mostly used in smart campus projects.
Barriers Negating Micro-Transferring Smart Campus Technologies to Cities
This final category of themes aimed to identify the challenges of smart campus knowledge transfer between Universities in South Africa and the government for the creation of smart cities. The themes which emerged from the interview analysis are ignorance, resistance to change, the university’s consultancy role and upskilling, source of capital for smart technologies, integration with existing technology, and the development of prototypes and stakeholder engagement and focus on transportation.
Theme 1- Ignorance
SAU5 identified ignorance as the leading barrier facing the development of smart cities in South Africa. SAU5 explained that government officials have limited knowledge about smart cities and the potential universities have to transfer their knowledge, provide training and support for a civil servant. This theme was further supported by resistance to change.
Theme 2- Resistance to Change
SAU3, SAU5 and SAU6 all identified resistance to change as the bane of knowledge transfer from universities to local government for smart campus development in South Africa. SAU3 specifically stated that “…resistance to change and status of institutional policies,” as the barrier facing smart cities development. As suggested by SAU3, resistance to change can support by Chourabi et al. (2012) and Khansari et al. (2014) studies of challenges affecting the development of smart cities not only in South Africa but in other developing countries. This theme can be linked to the next theme, the university’s consultancy role in facilitating the development of smart cities and up-skilling.
Theme 3- The University’s Consultancy Role and Up-Skilling
SAU5and SAU6 noted that universities in South Africa could play a role in transferring knowledge and training civil servants who are in charge of technology, procurement, and property management. SAU4 provided more clarity on this challenge by noting that “…lack of collaboration between a university and local government on these issues is the main challenge.” SAU1 opined that:
“There are several projects at a local and national level that university and government can closely collaborate and work together on. This depends on the type and nature of the project the funding, but majority funded either with SA government (local or national) or research funders (local and international).”
The university can provide consultancy services to enhance the capabilities of the government in procuring smart infrastructure. The smart campus procurement strategy used in universities may also be piloted in cities.
Theme 4- Source of Capital for Smart Technologies
The source of funding for developing smart cities is a major obstacle facing many developing economies. SUA6 suggested foreign financial agencies such as IMF and World Bank loans and grant applications as an opportunity for developing countries such as South Africa. In financing smart cities construction, Alok and Vashist (2016) and Hamilton and Zhu (2017) identified special purpose vehicles, fiscal policies, public-private partnership (PPP), performance-based revenue models and government investments strategic sources. In most developing countries, the option of loans and grants may be viewed as a quick source of revenue for capital projects. However, reorganising the fiscal policies for infrastructure development can repurpose the spending agenda towards smart infrastructure invites cities.
Theme 5- Integrating Local Technology With Existing Technology
SAU6 raised the challenge of integrating smart technology with existing ones in the cities. Hence, there is a need to up-skill the workers who engage in technology deployment and maintenance in the civil service. This may be achieved through knowledge transfer partnerships between the Universities with smart campus technologies and the local government.
Theme 6- Development of Prototypes and Stakeholder Engagement and Focus on Transportation
One of the suggestions raised by SAU1 was “focus on water, electricity and street lighting networks with online monitoring and control.” SAU5 also suggested that the government and the university can focus on the transportation section alone as a form of micro-scale transfer of knowledge. In attaining smart cities in developing countries, a micro-scale project can be used as a prototype to demonstrate the benefits, costs, and opportunities smart cities can provide.
Systems Thinking: Causal Loop Diagram
Ackoff (2003); Behl and Ferreira (2014); Loosemore and Cheung (2015); Prince, Zhen and Stephen (2016); and Awuzie et al. (2021) described systems thinking as an analytical tool of identifying deficiencies, observing new patterns and understanding interrelationships of cause and effect of a phenomenon. In this regard, systems thinking provides an in-depth understanding of a broad concept rather than the usual findings extracted from qualitative or quantitative data analysis. Systems thinking views social phenomena such as a smart city from an iceberg perspective.
The causal loop diagram represents the influence of one variable on another with an arrow starting from a “+” or “S” signs for positive impact or increase, or “–” or “O” sign for negative impact or decrease (Lezak and Thibodeau, 2016; Omotayo et al., 2020b; Awuzie et al., 2021). Therefore, the causal loop diagram will create looks that are usually reinforcing with the “R” sign or balancing with the “B” sign contained in a direction arrow. The reinforcing loop is created when there are even numbers of “+” or “S,” or “–” or “O” in a loop. When there is an odd number of “+” or “S,” or “–” or “O,” then a balancing loop will be indicated in the loop. Before the design of a causal loop, the exogenous and endogenous variables must be determined. In this study, the findings of the qualitative analysis formed the system of the exogenous and endogenous variables.
A summary of the interview findings was compiled in Figure 3. The findings from Smart Campus Infrastructure, Procurement, Contracting and Construction, to Barriers Negating Micro-Transferring Smart Campus Technologies to Cities were categorised as exogenous and endogenous variables to develop the causal loop diagram in Initial Causal Loop Model. Exogenous variables are external variables affecting a system, while endogenous variables are the internal variables in a system (Omotayo et al., 2020a; Iheukwumere et al., 2021). The system, in this instance, is the smart city and campus within the city. The exogenous variables in Figure 3 are mostly barriers and issues existing to limit the attainment of smart cities. The endogenous variables are themes that act as opportunities and strengthen the position of cities for smartness.
Initial Causal Loop Model
The initial causal loop diagram is designed from the constituents of Figure 3. The process of creating the causal loop diagram coloured smart campus infrastructure in red, smart campus procurement, contracting and construction in purple colour, and opportunities and challenges for micro-transferring smart campus infrastructures and knowledge to cities in blue colour. The new variables where were not identified in the analysis were indicated in black colour.
These new variables began with data mining from the left-hand side of Figure 4, and it has a positive impact on performance measurement and deep learning architecture. Cloud computing also influenced performance measurement. Smart building construction is central to the construction and energy management system as a positive variable. Education management systems and inclusive technology positively influence classroom instruction and engagement. Notably, in the top right corner, world bank and international grants, grant and loan applications positively influence the government’s desire to have a smart campus.
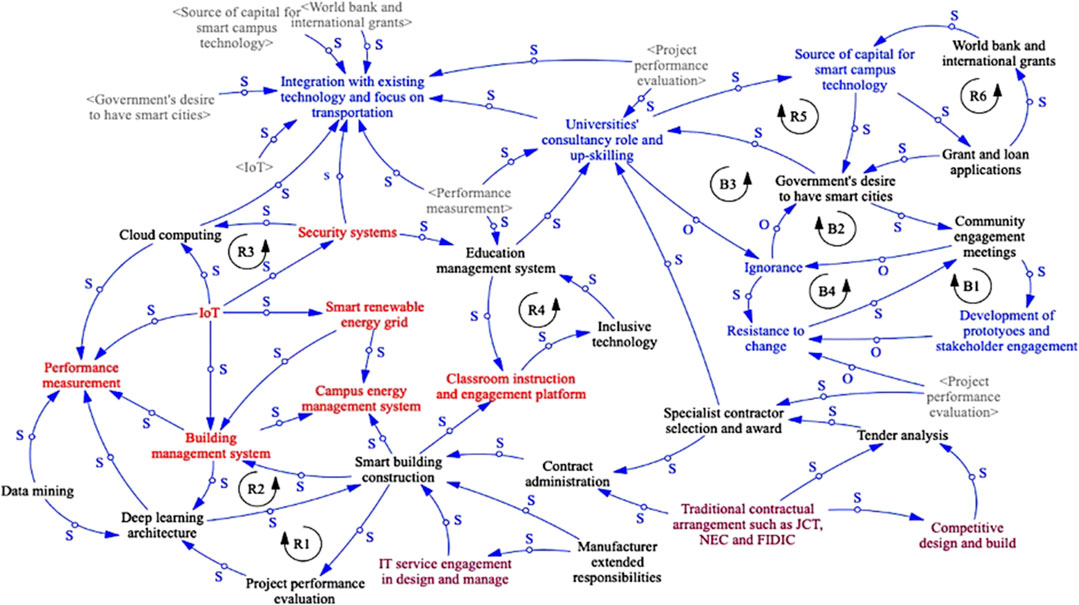
FIGURE 4. Causal loop diagram showing transference of knowledge and technologies in smart campuses to cities (Legend: Red variables = smart campus infrastructure; Purple variables = smart campus procurement, contracting and construction; Blue variables = opportunities and challenges for micro-transferring smart campus infrastructures and knowledge to cities; In black = new variables that emerged from the causal loop; S = Positive; O = Negative; R = Reinforcing loop; B = Balancing loop). Source: Authors.
Community engagement meetings balance the negativity of ignorance and resistance to change. Tender analysis and specialist contractor selection and award of the contract positively influence contract administration and procurement of smart buildings. The initial casual loop diagram of Figure 4 must be validated before relying on the system’s findings.
Validation of Findings
The purpose of the validation process is to investigate the applicability, practicality and ease of using the causal loop diagram in Figure 4, not only in developing countries, but around the world. The validation process was also to verify, investigate and identify any deficiencies in the variables included in the causal loop diagram. The causal loop diagram in Figure 4 was sent out by email to selected experts using the same purposive sampling approach adopted for the qualitative analysis. Five (5) experts in three continents of Europe, Asia and Africa provided dynamic viewpoints of the causal loop diagram for improvement and practical application as presented in Table 2. In all, two (2) experts from South Africa SA-EXP1 and SA-EXP2 with 27 and 10 years’ experience individually provided their African perspective on the model. An IT expert, PA-EX, from Pakistan with a year of experience, provided expert opinion on the model. Experts from the United Kingdom, UK-EXP1 and UK-EXP2, having 21 and 7 years of experience, respectively, responded to the validation process.

TABLE 2. Profile of the experts who provided their opinions in the validation phase. Source: Authors.
Table 3 summarises the expert opinions’ comments and the nature of changes effected in the model to produce a validated causal loop diagram. The validation questions were about the nature of variables, connectivity of the variables, and practicality. All five experts agreed that the model could be used in any country to transfer knowledge from smart campuses to cities. For improvement purposes, new variables were suggested by SA-EXP1, UK-EXP1, UK-EXP2, and SA-EXP2.
The changes effected as presented in Table 3 enhanced the quality of the causal loop model illustrated in Figure 5. The significant improvement included variables such as University community, building information management system, smart resource management, city information management system, facilities management, digital twins, big data and 5G technologies. The validation of the initial causal loop diagram was achieved effectively by enhancing the constituents of the model in Figure 5. The validated causal loop diagram can be used to generate archetypes of explaining the findings and further discussions.
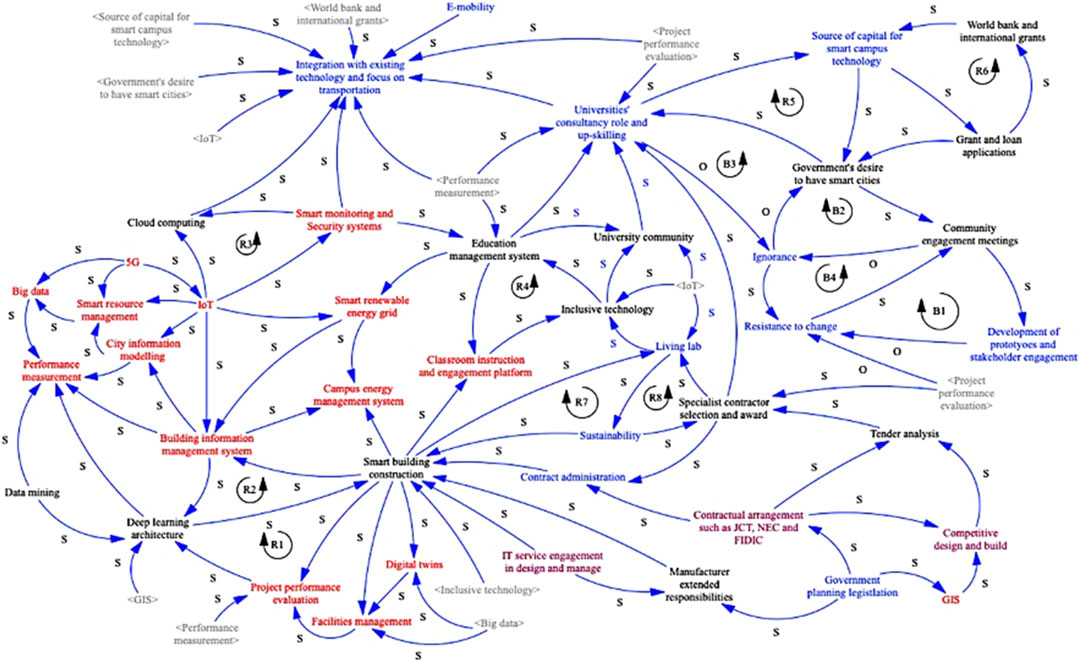
FIGURE 5. Validated smart campus to cities causal loop diagram (Legend: Red variables = smart campus infrastructure; Purple variables = smart campus procurement, contracting and construction; Blue variables = opportunities and challenges for micro-transferring smart campus infrastructures and knowledge to cities; In black = new variables that emerged from the causal loop; S = Positive; O = Negative; R = Reinforcing loop; B = Balancing loop). Source: Authors.
System Archetypes of Smart Campus to Cities Model
The purpose of including system archetypes in this model is to clearly express the interconnectedness of important variables, reinforcing and balancing loops. Wolstenholme (2004) and Clancy (2018) explained that system archetypes express the commonalities and interactions in a causal loop diagram. System archetypes are extracted from their larger environment to show that they can stand alone in another environment. Systems archetypes are the important aspects of a causal loop diagram that can be used for practical implementation. Figures 5–10 consist of systems archetypes taken out from the causal loop diagram of Figure 5.
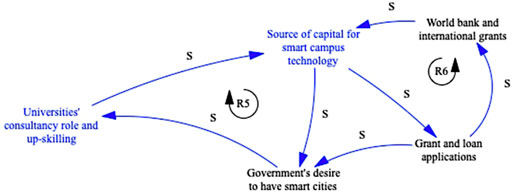
FIGURE 9. System archetype 4- The role of smart campus in smart cities development. Source: Authors.
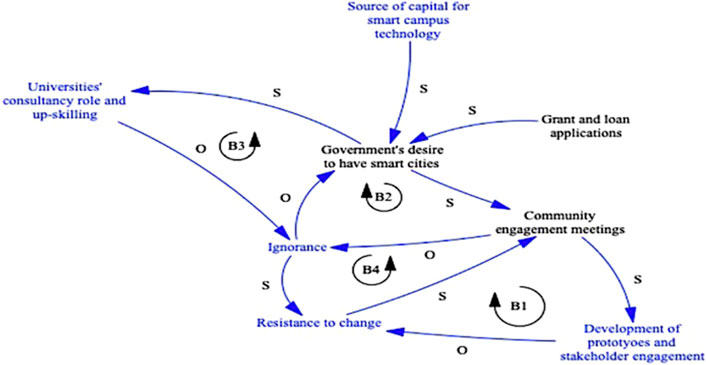
FIGURE 10. System archetype 5- Alleviating the challenges of developing smart cities in developing countries. Source: Authors.
Archetype 1- Smart Campus Technology and Management
The first system archetype, as illustrated in Figure 5, contains reinforcing loops R1 and R2. Reinforcing loop R1 shows how project performance evaluation of smart building construction can be improved with facilities management. Further analysis with data mining and deep learning architecture can inform the improvements of smart buildings. Building information management systems are connected with smart building construction and performance measurement through deep learning architecture in R2. IoT can be used to enhance the existing smart buildings through building information management systems.
System Archetype 2- Internet of Things and Data Management
Reinforcing loop R3 applies IoTs to improve city information modelling architecture, smart resource management, and smart monitoring and security system of Figure 6. IoT is dependent on 5G network, big data and performance management. Data management is an integral attribute of smart campuses and cities. Likewise, cloud computing is connected with big data and further improvements through performance measurement of smart campuses and cities.
System Archetype 3- Smart Campus Construction
Reinforcing loops R4, R7 and R8 are contained in the systems architecture of Figure 7. Inclusive technology consists of smart tools that help students with disabilities and learning difficulties. Inclusive technology in R4 positively impacts the university community and education management system. The education management system also aids classroom instruction and engagement platform, which in turn reinforces inclusive technology. IoT as a shadow variable is required for the benefit of the University community and inclusive technology. The living lab is a fundamental variable that connects the university community as a smart campus and the city through smart buildings and sustainability in R7. The living lab in R8 is reinforced by smart building construction through the specialist contractor. Construction activities through tender analysis and project performance evaluations are sacrosanct in R8.
System Archetype 4- The Role of Smart Campus in Smart Cities Development
R5 and R6 in systems archetype 4 of Figure 9 provide a typology of smart campuses for cities. The university’s role in providing consultancy and up-skilling is driven by the government’s desire to develop a smart city. Knowledge transfer of smart campus funding sources can be shared with the government in R5. This also aids loan and grant applications in R6.
System Archetype 5- Alleviating the Challenges of Developing Smart Cities in Developing Countries
Balancing loops B1, B2, B3, and B4, as presented in Figure 10, supports the systems archetypes for reducing the challenges of transferring knowledge from the university system archetypes with a smart campus to the cities in developing countries. B1 balances resistance to change by government officials and other stakeholders such as community heads and residents with the deployment of prototypes and stakeholder engagement. B2 and B3 balance the challenge of ignorance in governments of developing countries with the university’s role in consultancy and up-skilling, along with community engagement meetings. Balancing loop B4 may have resistance to change reinforced with ignorance, but community engagement meetings can reduce ignorance and resistance to change.
Discussion
This section will purposely compare the findings of the systems archetypes with the latest research in academia, highlight practical implications for smart campus and cities development.
Implications of Findings for Smart Campus Development
The practical application of the systems archetypes 1 to 3 for smart campus development considers the essence of smart technology, the internet of things with data management, and procurement. Min-Allah and Alrashed (2020) and Omotayo et al. (2021) sketch the infrastructure required for a smart campus. However, Min-Allah and Alrashed (2020) and Omotayo et al. (2021) did not include the living lab and smart resource management in their frameworks. Negreiros et al. (2020) noted that a smart campus could become a living lab for simulating how a smart city should look like.
The outcome of performance measures derived for continuous improvement of smart campuses can be shared with the government to benefit the city. Likewise, smart resource management provides a virtual library or database of all knowledge and data generated from smart technology and infrastructure (He et al., 2016). The living lab and smart resource management concept may not be viewed in many emerging smart campuses as opportunities for a city-wide transference of knowledge. The system archetypes 1 to 3 provides a practical approach for developing and enhancing existing smart campuses in developing countries as supported by Min-Allah and Alrashed (2020); Omotayo et al. (2021); Zhicheng and Feng, (2018); Zhou et al., 2020; and Moura et al. (2021). Procurement and tendering practices in smart campus construction are salient attributes of that can be shared with local governments in developing countries for smart city development. Information management systems of smart campuses infrastructure is another important aspect that can be facilitated with building and city information modelling. The purpose of understanding the nature of smart campus development in this study portends the nature of smart cities in developing countries. It is important to note that the systems thinking archetypes may also be valid for developed countries with existing smart campuses.
Implications of Findings for the Development of Smart Cities
Unlike other frameworks designed by AbuAlnaaj et al. (2020); Imbar et al. (2020) for smart campuses and cities where IoTs and cloud computing formed the main attributes, this study identified more with the end-users and management of smart infrastructure. The systems archetypes 4 and 5 in Figures 9, 10 support smart campus technology’s transference to cities. The funding sources for smart campuses, procurement, contractual arrangements, and construction approaches can be shared with the government. The concept of a smart campus has been embraced in different parts of the world with its many solutions to different aspects of an academic environment, primarily as a micro-scale city for smart cities. Lehman (2020) highlighted that Arizona state university, the University of Texas, the University of Michigan and the University of Wisconsin-Madison are a few US Universities that have adopted smart campus solutions. Some of the solutions include but are not limited to sensors and powerful cameras to ensure operational efficiency and improved entertainment scenes in a football stadium in Arizona State University. At the same time, the University of Texas Austin provides all of the electricity needed through the largest microgrids for 150 buildings within its premises. The both University of Michigan and the University of Wisconsin-Madison designed an autonomous vehicle trend that increases safety and reduces energy emissions. The aforementioned smart technologies are present in the city’s housing the universities. Developing smart campuses as a living lab for smart cities can be adopted in developing countries. In Brazil, Negreiros et al. (2020) campus smart grid and energy management are expected to be expanded to the large urban environment. Cloud computing and digital asset management provides a foundation for developing smart transportation systems in cities (Abuarqoub et al., 2017; Negreiros et al., 2020).
Smart campus development is premised on the concept of cloud computing as well as other recent technologies (Benltoufa et al., 2018; Flammini et al., 2018). Isolated systems such as office management, finance management, education management and other related systems are integrated into one system through cloud computing (Nie, 2013). Ikrissi and Mazri (2020) study listed the components relating to cloud computing in smart campus solutions, which has proven important for ease of operation within the campus ecosystem. They comprise embedded computing, biometrics, smart community and other smart applications. The technology adopts numerous devices and sensors connected to carry out simple tasks such as crowd detection, adaptive learning and local binary patterns. There are no differences in smart technologies on campuses and the concepts for commercial offices, residential accommodation, factory, transport, health and energy management systems in cities (Bates et al., 2015; Agarwal et al., 2020). Consequently, smart campuses have also been postulated as living labs for smart cities (Atif et al., 2015; Nina et al., 2016; Flammini et al., 2018). The concept of living labs in smart campuses can be used to understudy end-user interaction with smart technologies, identify what works well and propose continuous improvement through the transference of smart technologies.
Irrespective of the resistant force of transitioning smart campuses to cities, as indicated in Figure 11, developing countries can leverage any existing smart campus as a living lab to gradually include smartness in their cities’ infrastructures. The key driving forces of transitioning smart campuses to cities are the capabilities of IoTs and data management and the university’s knowledge of smart campus construction. With the abovesaid driving forces, the challenge of integration with existing technology and community engagement issues can be resolved.
Conclusion and Limitations of the Study
This study aimed to develop a model that examines the interrelationship between infrastructure elements of smart campuses as a tool for smart cities in developing countries using the system thinking approach. The findings identified the key attributes of smart campuses in the form of systems archetypes from a validated causal loop diagram. Smart campus technology and management; IoTs and data management; smart campus construction; the role of smart campus in smart cities development; the reduction of challenges of developing smart cities in developing countries are the key findings of this study. Financial opportunities from foreign financial lending agencies must be the last resort after considering repurposing budgetary allocation for infrastructure, PPP arrangement, investment, and internal funding sources. Universities with smart campuses can also be used as consultants and training agents for the property and infrastructure department.
The present approach of acquiring smart campuses must be based on a framework that can be transferred to the environment outside the campus. In this regard, smart campus construction framework as identified in archetypes 1 to 3, must feed into archetype 4 whereby the governance of smart campuses act as champions of smart cities. Likewise, archetype 5 can be used in developing and developed countries to enhance the smartness infrastructure of cities. The transference of knowledge from smart campuses to cities around the world can focus on the smart mobilities for transportation, management of smart assets, big data storage and analytics, safety and security, energy management, building and city information modelling. The procurement and contractual arrangements adopted for smart campus construction can also be shared with local governments in charge of smart city development.
The desire to attain smartness is cities around the world, depends on the willingness of the government, technological capabilities and availability of funds. Furthermore, the developed countries with smart cities can study campuses within their cities as living labs for continuous improvement of their smart infrastructure. Smart campuses as living labs are inclusive of students with disabilities as part of the community of campus users, their reaction and application to smart technologies and lifestyle changes can be extracted as a form of big data for the purpose of analytics. The idea of smart campuses acting as living labs for new technologies is a major concept worth exploring by governments around the world.
The limitations of this study were a source of data from a single geographical location, South Africa. Additional data from multiple countries and case studies of campuses in developing countries may be used in future studies. Although the study was also qualitative, quantitative data may be collected and analysed to understand the most important smart campus technologies or preferred procurement strategies. Notwithstanding, the aim of this study was resolved using the validation of the causal loop diagram and importation tool in systems thinking.
Data Availability Statement
The raw data supporting the conclusions of this article will be made available by the authors, without undue reservation.
Ethics Statement
The studies involving human participants were reviewed and approved by The research ethics committee of the School of Built Environment Engineering and Computing of the Leeds Beckett University approved this research under research ethics Application Ref: 83397. The patients/participants provided their written informed consent to participate in this study.
Author Contributions
OO Contributed to the literature review. TO contributed to the methodology and analysis. BA and AM contributed to the data collection, curation and proofreading. BA contributed the abstract.
Funding
This study was funded by the School of Built Environment, Engineering and Computing of the Leeds Beckett University, United Kingdom.
Conflict of Interest
The authors declare that the research was conducted in the absence of any commercial or financial relationships that could be construed as a potential conflict of interest.
Publisher’s Note
All claims expressed in this article are solely those of the authors and do not necessarily represent those of their affiliated organizations, or those of the publisher, the editors and the reviewers. Any product that may be evaluated in this article, or claim that may be made by its manufacturer, is not guaranteed or endorsed by the publisher.
References
AbuAlnaaj, K., Ahmed, V., and Saboor, S. (2020). “A Strategic Framework for Smart Campus,” in Proceedings of the International Conference on Industrial Engineering and Operations Management, 0(March), 790–798.
Abuarqoub, A., Abusaimeh, H., Hammoudeh, M., Uliyan, D., Abu-Hashem, M. A., Murad, S., Al-Jarrah, M., and Al-Fayez, F. (2017). “A Survey on Internet of Things Enabled Smart Campus Applications,” in Proceedings of the International Conference on Future Networks and Distributed Systems, 1–7. doi:10.1145/3102304.3109810
Agarwal, P., Kumar G.V.V., R., and Agarwal, P. (2020). “IoT Based Framework for Smart Campus: COVID-19 Readiness,” in Proceedings of the World Conference on Smart Trends in Systems, Security and Sustainability (WS4), 2020, 539–542. doi:10.1109/WorldS450073.2020.9210382
Alok, V. N., and Vashist, A. (2016). “Financing Smart Cities in India,” in Indian Journal of Public Administration. (New Delhi, India: SAGE Publications Sage India), 62, 791–804. doi:10.1177/00195561201604054
Alrashed, S. (2020). Key Performance Indicators for Smart Campus and Microgrid. Sust. Cities Soc. 60, 102264–102269. doi:10.1016/j.scs.2020.102264
Althobaiti, M. M., Technology, I., and Arabia, S. (2020). Toward a Smart Campus Based on Smart Technologies and Best, 11(10), 1385–1394. doi:10.34218/IJARET.11.10.2020.132
Aman, S., Simmhan, Y., and Prasanna, V. K. (2013). Energy Management Systems: State of the Art and Emerging Trends. IEEE Commun. Mag. 51 (1), 114–119. doi:10.1109/mcom.2013.6400447
Andrejevic, M., and Selwyn, N. (2020). “Facial Recognition Technology in Schools: Critical Questions and Concerns,” in Facial Recognition Technology in Schools: Critical Questions and Concerns’, Learning, Media and Technology (Taylor & Francis), 45(2), 115–128. doi:10.1080/17439884.2020.1686014
Arshad, S., Azam, M. A., Ahmed, S. H., and Loo, J. (2017). “Towards Information-Centric Networking (Icn) Naming for Internet of Things (Iot): The Case of Smart Campus,” in Proceedings of the International Conference on Future Networks and Distributed Systems, 1–6.
Atif, Y., Mathew, S. S., and Lakas, A. (2015). Building a Smart Campus to Support Ubiquitous Learning. J. Ambient Intell. Hum. Comput. 6 (2), 223–238. doi:10.1007/s12652-014-0226-y
Awuzie, B., Ngowi, A. B., Omotayo, T., Obi, L., and Akotia, J. (2021). Facilitating Successful Smart Campus Transitions: A Systems Thinking-SWOT Analysis Approach. Appl. Sci. 11 (5), 2044. doi:10.3390/app11052044
Bates, O., Friday, A., and Hoster, H. E. (2015) ‘A Living Lab Campus: A Unique Opportunity to Not Be a" Smart City", EnviroInfo/ICT4S (2). 7903. Available at: http://enviroinfo.eu/sites/default/files/pdfs/vol9073/0206.pdf, (Accessed 4 July 2014).
Behl, D. V., and Ferreira, S. (2014). Systems Thinking: An Analysis of Key Factors and Relationships. Proced. Comp. Sci. 36, 104–109. doi:10.1016/j.procs.2014.09.045
Benltoufa, A. N. H. S., Jaafar, F., Maraoui, M., Said, L., Zili, M., Hedfi, H., Labidi, M., Bouzidi, A., Jrad, B. B., and Belhadj Salah, H. (2017). “From Smart Campus to Smart City: Monastir Living Lab,” in Proceedings of 2017 International Conference on Engineering and Technology, ICET 2017, 2018-January, 1–6. doi:10.1109/ICEngTechnol.2017.8308196
Bilau, A. B., Ajagbe, A. S., Kigbu, H. H., and Sholanke, A. B. (2015). Review of Shortage of Skilled Craftsmen in Small and Medium Construction Firms in Nigeria. J. Environ. Earth Sci. 5 (15), 98–103.
Chen, H., Jiang, W., Yang, Y., Yang, Y., and Man, X. (2017). State of the Art on Food Waste Research: a Bibliometrics Study from 1997 to 2014. J. Clean. Prod. 140, 840–846. doi:10.1016/j.jclepro.2015.11.085
Chenoweth, H. (2020). The Rise of university Microgrids. Available online: https://info.higheredfacilitiesforum.com/blog/the-rise-of-university-microgrids.pdf (Accessed June 20, 2020).
Chourabi, H., Nam, T., Walker, S., Gil-Garcia, J. R., Mellouli, S., Nahon, K., Pardo, T. A., and Scholl, H. J. (2012). “Understanding Smart Cities: An Integrative Framework,” in 2012 45th Hawaii international conference on system sciences (IEEE), 2289–2297.
Clancy, T. (2018). Systems Thinking: Three System Archetypes Every Manager Should Know. IEEE Eng. Manag. Rev. 46 (2), 32–41. doi:10.1109/emr.2018.2844377
De Hoyos, M., and Barnes, S. A. (2012). Analysing Interview Data. Warwick, United Kingdom: Warwick Institute for employment research.
De Vaus, D., and de Vaus, D. (2013). Surveys in Social Research. London: Routledge. doi:10.4324/9780203519196
Devitsyna, S., Eletskaya, T., and Meshkov, A. (2019). “Developing Facial Recognition Software to Control Access to Campus Facilities,” in CEUR Workshop Proceedings, 68–76.
Flammini, A., Pasetti, M., Rinaldi, S., Bellagente, P., Ciribini, A. C., Tagliabue, L. C., Zavanella, L. E., Zanoni, S., Oggioni, G., and Pedrazzi, G. (2018). “A Living Lab and Testing Infrastructure for the Development of Innovative Smart Energy Solutions: the eLUX Laboratory of the University of Brescia,” in 2018 110th AEIT International Annual Conference (Bari, Italy: The eLUX Laboratory of the University of Brescia’AEIT 2018AEIT), 15–20. doi:10.23919/AEIT.2018.8577329
Folkestad, B. (2008). Analysing Interview Data: Possibilities and Challenges. Eurosphere Working Paper Series. Online Working Paper. Available from: https://ideas.repec.org/p/erp/ewpxxx/p0037.html (Accessed July 20, 2021).
Guest, G., Bunce, A., and Johnson, L. (2006). How Many Interviews Are Enough. Field Methods 18 (1), 59–82. doi:10.1177/1525822X05279903
Guo, M., and Zhang, Y. (2015). “The Research of Smart Campus Based on Internet of Things Amp; Cloud Computing,” in Proc. 11th WiCOM, 1–6.
Hamilton, S., and Zhu, X. (2017). “Funding and Financing Smart Cities,” in The Journal of Government Financial Management (London, United Kingdom: Association of Government Accountants), 66(1), 26–33.
He, J., Atabekov, A., and Haddad, H. M. (2016). “Internet-of-things Based Smart Resource Management System: a Case Study Intelligent Chair System,” in 2016 25th International Conference on Computer Communication and Networks (ICCCN) (IEEE), 1–6. doi:10.1109/icccn.2016.7568533
Hu, H., and Yan, H. (2016). “A Study on Discovery Method of Hot Topics Based on Smart Campus Big Data Platform,” in International Conference on Intelligent Transportation, Big Data & Smart City (ICITBS) (IEEE), 176–179. doi:10.1109/icitbs.2016.10
Iheukwumere, O., Moore, D., and Omotayo, T. (2021). “Analysis of Multi-Factors Affecting the Performance of Nigeria's Refineries: a Systems Thinking Approach, Ijppm, Ahead-Of-Print,” in International Journal of Productivity and Performance Management (Emerald Publishing Limited). ahead-of-p(ahead-of-print). doi:10.1108/IJPPM-11-2020-0585
Ikrissi, G., and Mazri, T. (2020). A Study of Smart Campus Environment and its Security Attacks. Int. Arch. Photogramm. Remote Sens. Spat. Inf. Sci. XLIV-4/W3-2020, 255–261. doi:10.5194/isprs-archives-XLIV-4-W3-2020-255-2020
Imbar, R. V., Supangkat, S. H., and Langi, A. Z. R. (2020). “Smart Campus Model: A Literature Review,” ‘in Smart Campus Model: A Literature Review’, 7th International Conference on ICT for Smart Society (Bandung City, Indonesia: AIoT for Smart Society, ICISS 2020 - Proceeding), 15–21. doi:10.1109/ICISS50791.2020.9307570
Ivanovski, D. (2015). “The “green” in Internet of Things: Case Study of Faculty Environment,” inInternational Conference on Knowledge Management in Organizations. Editors L. Uden, M. Heriˇcko, and I.-H. Ting (Cham: Springer), 561–571. doi:10.1007/978-3-319-21009-4_42
Jiang, T., Cao, J., Su, D., and Yang, X. (2017). “Analysis and Data Mining of Students’ Consumption Behavior Based on a Campus Card System,” in Proceedings of the 2017 International Conference on Smart City and Systems Engineering (ICSCSE) Changsha, China. doi:10.1109/icscse.2017.22
Khansari, N., Mostashari, A., and Mansouri, M. (2014). Conceptual Modeling of the Impact of Smart Cities on Household Energy Consumption. Proced. Comp. Sci. 28, 81–86. Elsevier. doi:10.1016/j.procs.2014.03.011
Kuo, W., Pan, C., and Lin, L. (2018). “Wastewater Treatment and Reuse on Campus: A Case Study in Kun Shan University,” in 2018 4th International Conference on Green Technology and Sustainable Development (GTSD) (IEEE), 583–586. doi:10.1109/GTSD.2018.8595652
Lehman, N. (2020). The Future of Higher Education: Smart Campuses. Available online: https://spaces4learning.com/articles/2019/03/01/smart-campuses.aspx.pdf (Accessed June 20, 2020).
Lezak, S. B., and Thibodeau, P. H. (2016). Systems Thinking and Environmental Concern. J. Environ. Psychol. 46, 143–153. doi:10.1016/j.jenvp.2016.04.005
Li, M. (2020).Based on the Construction of Financial Management Information Platform of Colleges and Universities in 5G Environment--Take Guangzhou Nanyang Polytechnic Vocational College as an Example J. Phys. Conf. Ser., 1616, 012011. doi:10.1088/1742-6596/1616/1/012011
Li, Y. (2021). “Application of Big Data Technology in Campus Security Management under the Background of Information Age,” in Journal of Physics: Conference Series J. Phys. Conf. Ser., 022097. 1881 (2) doi:10.1088/1742-6596/1881/2/022097
Liang, W. (2020). “Analysis of the Application of Artificial Intelligence Technology in the Construction of Smart Campus,” in Proceedings of the 2020 International Wireless Communications and Mobile Computing (IWCMC) Limassol, Cyprus. doi:10.1109/iwcmc48107.2020.9148200
Liu, D., Huang, R., and Wosinski, M. (2017). “Smart Learning in Digital Campus,” in Smart Learning in Smart Cities; Lecture Notes in Educational Technology (Singapore: Springer), 51–90. doi:10.4028/www.scientific.net/AMM.543-547.3213
Lo, Y. Y. (2019). “Creating a Sustainable Campus through Digitalization : Exploring Workplace Practices to Increase Employee Engagement,” in Master’s thesis, KTH, School of Electrical Engineering and Computer Science (EECS)
Loosemore, M., and Cheung, E. (2015). Implementing Systems Thinking to Manage Risk in Public Private Partnership Projects. Int. J. Project Manage. 33 (6), 1325–1334. doi:10.1016/j.ijproman.2015.02.005
Luo, L. (2018). Data Acquisition and Analysis of Smart Campus Based on Wireless Sensor. Wireless Pers Commun. 102, 2897–2911. doi:10.1007/s11277-018-5314-4
Majeed, M., and Ali, M. (2018). “How Internet-Of-Things (Iot) Making the university Campuses Smart? Qa Higher Education (Qahe) Perspective,” in IEEE 8th Annual Computing and Communication Workshop and Conference (CCWC) (IEEE), 646–648.
Mazza, I. F. S. (2015). “Strategic Management of Resources and Technologies for a Smart and Green Space Campus,” in Proceedings of the International Astronautical Congress Jerusalem, Israel, 9476–9480.
Mershad, K. P., and Wakim, P. (2018). A Learning Management System Enhanced with Internet of Things Applications. Jel 7 (3), 23–40. doi:10.5539/jel.v7n3p23
Michailidis, I. T., Schild, T., Sangi, R., Michailidis, P., Korkas, C., Fütterer, J., et al. (2018). Energy-efficient HVAC Management Using Cooperative, Self-Trained, Control Agents: A Real-Life German Building Case Study. Appl. Energ. 211, 113–125. doi:10.1016/j.apenergy.2017.11.046
Min-Allah, N., and Alrashed, S. (2020). Smart Campus-A Sketch. Sust. Cities Soc. 59 (December2019), 102231. Elsevier. doi:10.1016/j.scs.2020.102231
Moghayedi, A., Awuzie, B., Omotayo, T., Le Jeune, K., Massyn, M., Ekpo, C. O., et al. (2021). A Critical Success Factor Framework for Implementing Sustainable Innovative and Affordable Housing: A Systematic Review and Bibliometric Analysis. Buildings 11 (8), 317. doi:10.3390/buildings11080317
Moraes, P., Pisani, F., and Borin, J. (2020). “Towards a Simulator for Green Smart Campus Systems,” in Workshop De Computacao Aplicada A Gestao Do Meio Ambiente E Recursos Naturais (WCAMA) (Sociedade Brasileira de Computação), 111–120. doi:10.5753/wcama.2020.11025
Moura, P., Moreno, J. I., López López, G., and Alvarez-Campana, M. (2021). IoT Platform for Energy Sustainability in University Campuses. Sensors 21, 357. doi:10.3390/s21020357
Muhamad, W., Kurniawan, N. B., and Yazid, S. (2017). “Smart Campus Features, Technologies, and Applications: A Systematic Literature Review,” in 2017 International conference on information technology systems and innovation (ICITSI) (IEEE), 384–391. doi:10.1109/icitsi.2017.8267975
Negreiros, I., Francisco, A. C. C., Fengler, F. H., Faria, G., Pinto, L. G. P., Tolotto, M., Rogoschewski, R. B., Romano, R. R., and Netto, R. S. (2020). “Smart Campus as a Living Lab on Sustainability Indicators Monitoring,” in 2020 IEEE International Smart Cities Conference (ISC2) (IEEE), 1–5. doi:10.1109/isc251055.2020.9239017
Nie, X. (2013). “Constructing Smart Campus Based on the Cloud Computing Platform and the Internet of Things,” in Proceedings of the 2nd International Conference on Computer Science and Electronics Engineering (ICCSEE 2013) Hangzhou, China, 1576–1578. doi:10.2991/iccsee.2013.395
Nina, M., Oliveira, Á., and Medina, J. (2014). SMART CAMPUS - Building-User Interaction towards Energy Efficiency through ICT-Based Intelligent Energy Management Systems. Eur. Project Space Inf. Commun. Syst., 11–30. doi:10.5220/0006182900110030
Omotayo, T., Awuzie, B., Egbelakin, T., Obi, L., and Ogunnusi, M. (2020b). AHP-systems Thinking Analyses for Kaizen Costing Implementation in the Construction Industry. Buildings 10 (12), 230. doi:10.3390/buildings10120230
Omotayo, T., Moghayedi, A., Awuzie, B., and Ajayi, S. (2021). Infrastructure Elements for Smart Campuses: A Bibliometric Analysis. Sustainability 13 (14), 7960. doi:10.3390/su13147960
Omotayo, T., Olanipekun, A., Obi, L., and Boateng, P. (2020a). A Systems Thinking Approach for Incremental Reduction of Non-physical Waste. Bepam 10, 509–528. Built Environment Project and Asset Management, ahead-of-p. doi:10.1108/BEPAM-10-2019-0100
Omotayo, T. S., Boateng, P., Osobajo, O., Oke, A., and Obi, L. I. (2019). Systems Thinking and CMM for Continuous Improvement in the Construction Industry. Ijppm 69 (2), 271–296. doi:10.1108/IJPPM-11-2018-0417
Petcovici, A., and Stroulia, E. (2016). “Location-based Services on a Smart Campus: A System and a Study,” in Proc. 3rd IEEE WF-IoT, 94–99. doi:10.1109/wf-iot.2016.7845406
Popoola, S. I., Atayero, A. A., Badejo, J. A., John, T. M., Odukoya, J. A., and Omole, D. O. (2018). Learning Analytics for Smart Campus: Data on Academic Performances of Engineering Undergraduates in Nigerian Private university. Data in brief 17, 76–94. doi:10.1016/j.dib.2017.12.059
Prandi, C., Monti, L., Ceccarini, C., and Salomoni, P. (2020). Smart Campus: Fostering the Community Awareness through an Intelligent Environment. Mobile Netw. Appl. 25 (3), 945–952. Springer. doi:10.1007/s11036-019-01238-2
Prince, B., Zhen, C., and Stephen, O. O. (2016). A Dynamic Framework for Managing the Complexities of Risks in Megaprojects Scott Sutherland School of Architecture and Built Environment, 5. Aberdeen , Scotland , UK’: Robert Gordon University, 1–13.
Putman, K., Boekhout, H. D., and Takes, F. W. (2019). “Fast Incremental Computation of Harmonic Closeness Centrality in Directed Weighted Networks,” in Proceedings of the 2019 IEEE/ACM International Conference on Advances in Social Networks Analysis and Mining (New York, USA: ACM). doi:10.1145/3341161.3344829
Rapley, T. J. (2001). The Art(fulness) of Open-Ended Interviewing: Some Considerations on Analysing Interviews. Qual. Res. 1 (3), 303–323. Sage Publications Sage CA: Thousand Oaks, CA. doi:10.1177/146879410100100303
Qin, Y., and Chi, M. (2020). Rsimagenet: a Universal Deep Semantic Segmentation Lifecycle for Remote Sensing Images. IEEE Access 8, 68254–68267. doi:10.1109/ACCESS.2020.2986514
Rodrigues, P. L., dos Santos Rabello, R., and Cervi, C. R. (2019). “An Application to Generate Air Quality Recommendations and Alerts on a Smart Campus,” in HCI International - Posters. Editor C. Stephanidis, 507–514. doi:10.1007/978-3-030-23528-4_69
Sari, M. W., Ciptadi, P. W., and Hardyanto, R. H. (2017). “Study of Smart Campus Development Using Internet of Things Technology,” in IOP Conference Series: Materials Science and Engineering (Semarang, Indonesia: IOP Publishing), 12032. doi:10.1088/1757-899x/190/1/012032
Subbarao, V., Srinivas, K., and Pavithr, R. S. (2019). “A Survey on Internet of Things Based Smart, Digital green and Intelligent Campus,” in Proc. 4th IoT-SIU (IEEE), 1–6. doi:10.1109/iot-siu.2019.8777476
Sun, R., Xi, J., Yin, C., Wang, J., and Kim, G.-j. (2018). Location Privacy protection Research Based on Querying Anonymous Region Construction for Smart Campus. Mobile Inf. Syst. 2018, 1–11. doi:10.1155/2018/3682382
Talei, H., Essaaidi, M., and Benhaddou, D. (2017). “Smart Campus Energy Management System: Advantages, Architectures, and the Impact of Using Cloud Computing,” in Proceedings of the 2017 International Conference on Smart Digital Environment, 1–7.
Thorat, S. B., Nayak, S. K., and Dandale, J. P. (2010). Facial Recognition Technology: An Analysis with Scope in India. arXiv preprint arXiv:1005.4263.
Vasileva, R., Rodrigues, L., Hughes, N., Greenhalgh, C., Goulden, M., and Tennison, J. (2018). What Smart Campuses Can Teach Us about Smart Cities: User Experiences and Open Data. Information 9, 251. doi:10.3390/info9100251
Villegas-Ch, W., Palacios-Pacheco, X., and Luján-Mora, S. (2019). Application of a Smart City Model to a Traditional university Campus with a Big Data Architecture: A Sustainable Smart Campus. Sustainability 11, 2857. doi:10.3390/su11102857
Vivi, Q. L., Parlikad, A. K., Woodall, P., Ranasinghe, G. D., and Heaton, J. (2019). “Developing a Dynamic Digital Twin at a Building Level: Using Cambridge Campus as Case Study,” in International Conference on Smart Infrastructure and Construction 2019 (ICSIC) (Cambridge, UK: ICE Publishing). doi:10.1680/icsic.64669.067
Wei, L. (2016). “Campus Management Strategy Research under the Environment of Big Data,” in Proc. ICITBS (IEEE), 195–199. doi:10.1109/icitbs.2016.56
Weller, S. C., Vickers, B., Bernard, H. R., Blackburn, A. M., Borgatti, S., Gravlee, C. C., et al. (2018). Open-ended Interview Questions and Saturation. PLoS ONE 13 (6), e0198606–18. Public Library of Science. doi:10.1371/journal.pone.0198606
Weng, Y., Zhang, N., and Xia, C. (2019). Multi-agent-based Unsupervised Detection of Energy Consumption Anomalies on Smart Campus. IEEE Access 7, 2169–2178. doi:10.1109/access.2018.2886583
Wolstenholme, E. (2004). Using Generic System Archetypes to Support Thinking and Modelling. Syst. Dyn. Rev. 20 (4), 341–356. Wiley Online Library. doi:10.1002/sdr.302
Yuliansyah, H., Corio, D., Yunmar, R. A., and Aziz, M. R. K. (2019). Energy Monitoring System Based on Internet of Things toward Smart Campus in Institut Teknologi Sumatera. IOP Conf. Ser. Earth Environ. Sci. 258, 012008. doi:10.1088/1755-1315/258/1/012008
Zhang, L., Yuan, H., Chang, S.-H., and Lam, A. (2020). Research on the Overall Architecture of Internet of Things Middleware for Intelligent Industrial parks. Int. J. Adv. Manuf. Technol. 107, 1081–1089. doi:10.1007/s00170-019-04310-z
Zhang, W., and Jiang, L. (2018). Algorithm Analysis for Big Data in Education Based on Depth Learning. Wireless Pers. Commun. 102 (4), 3111–3119. doi:10.1007/s11277-018-5331-3
Zhang, Y., Dong, Z. Y., Yip, C., and Swift, S. (2020). Smart Campus: A User Case Study in Hong Kong. IET Smart Cities 2, 146–154. doi:10.1049/iet-smc.2020.0047
Zhicheng, D., and Feng, L. (2018). “Evaluation of the Smart Campus Information Portal,” in Proceedings of the 2018 2nd International Conference on Education and E-Learning, New York, NY, USA (New York, United States: ACM). doi:10.1145/3291078.3291083
Keywords: causal loop diagram, developing countries, smart campus, smart cities, systems thinking
Citation: Omotayo T, Awuzie B, Ajayi S, Moghayedi A and Oyeyipo O (2021) A Systems Thinking Model for Transitioning Smart Campuses to Cities. Front. Built Environ. 7:755424. doi: 10.3389/fbuil.2021.755424
Received: 08 August 2021; Accepted: 22 September 2021;
Published: 14 October 2021.
Edited by:
Timothy O. Olawumi, Edinburgh Napier University, United KingdomReviewed by:
Alessio Ricci, Eindhoven University of Technology, NetherlandsAssed N. Haddad, Federal University of Rio de Janeiro, Brazil
Copyright © 2021 Omotayo, Awuzie, Ajayi, Moghayedi and Oyeyipo. This is an open-access article distributed under the terms of the Creative Commons Attribution License (CC BY). The use, distribution or reproduction in other forums is permitted, provided the original author(s) and the copyright owner(s) are credited and that the original publication in this journal is cited, in accordance with accepted academic practice. No use, distribution or reproduction is permitted which does not comply with these terms.
*Correspondence: Temitope Omotayo, t.s.omotayo@leedsbeckett.ac.uk