- Department of Civil and Environmental Engineering, Rice University, Houston, TX, United States
The changing dynamics of coastal regions and climate pose severe challenges to coastal communities around the world. Effective planning of engineering projects and resilience strategies in coastal regions must not only address current conditions but also take into consideration the expected changes in the exposure and multi-hazard risk in these areas. However, existing performance-based engineering frameworks generally neglect time-varying factors and miss the opportunity to leverage related evidence as it becomes available. This paper proposes a Performance-Based Coastal Engineering (PBCE) framework that is flexible enough to accommodate uncertain time-varying factors, multi-hazard conditions, and cascading-effects. Furthermore, using a dynamic Bayesian network approach, the framework can incorporate observed evidence into the model to update the prior conditional distribution of the analyzed variables. As a proof of concept, two case studies—a typical elevated residential structure and a two-frame system—are presented, considering the effects of cascading failure, the incorporation of time-varying factors, and the influence of emerging evidence. Results show that neglecting cascading effects significantly underestimates the losses and that the incorporation of evidence reduces the uncertainty under the assumed distribution of evidence. The resulting PBCE framework can support data collection efforts, optimization of retrofitting strategies, integration of experts and community interests by facilitating interactions and knowledge sharing, as well as the identification of vulnerable regions and critical components in coastal multi-hazard regions.
Introduction
Coastal communities face severe socio-economic challenges posed by the disastrous impacts of natural hazards such as hurricanes. Such challenges are exacerbated by an increase in population over time coupled with the aging of existing infrastructure, rise in property value, and the expected shifts in the frequency and intensity of natural hazards due to climate change (Field et al., 2012; Camargo and Wing, 2021). For instance, the extreme levels of rainfall caused by Hurricane Harvey (2017) led to innumerous flood-related damages in the southeast Texas region, making it the second-costliest hurricane in United States history, preceded only by Hurricane Katrina (Blake and Zelinsky, 2018). Recent studies have linked the severe levels of rainfall observed during Harvey to climate change effects on the ocean’s heat content and surface temperature (Emanuel, 2017; Risser and Wehner, 2017; Trenberth et al., 2018; Wang et al., 2018). These complex conditions are expected with more frequency in the future (Hassanzadeh et al., 2020; Wang and Toumi, 2021) and raise important questions about the adequacy of our existing structural and infrastructure systems to withstand the upcoming hazard exposure. Therefore, to effectively design, manage and maintain structures and infrastructure systems in coastal regions, special consideration should be given to time-varying factors while conducting a performance evaluation.
In general, performance-based engineering offers methods to quantify the uncertain structural response when exposed to a stressor in terms of performance levels and incurred damage states, followed by estimating the probability of exceeding a defined value of a decision variable DV (e.g., economic losses, sustainability index such as waste generation or embodied energy) in a reference time of analysis. The framework for performance-based engineering, originally proposed in the earthquake engineering community (Porter, 2003; Moehle and Deierlein, 2004; Günay and Mosalam, 2013), has been recently extended to other types of natural hazards such as wind (PBWE) (Ciampoli et al., 2011), hurricanes (PBHE) (Barbato et al., 2013), and tsunamis (PBTE) (Attary et al., 2017; Attary et al., 2019), to perform probabilistic risk assessment and/or design of structures. These methodologies posed key advances in the performance assessment of coastal structures by accounting for: 1) the effects of the interaction between the structure and its surroundings (e.g. fluid-structure interaction, soil-structure interaction) (Ciampoli et al., 2011); 2) the intrinsic multi-hazard nature of coastal hazards (Barbato et al., 2013; Attary et al., 2019); and 3) successive damage to the structures (e.g. incoming and outcoming flows during tsunami events) (Attary et al., 2017). These frameworks employ the theorem of total probability, where the marginal probability distribution of the decision variable is computed by disaggregating the joint distributions into smaller components of analysis. Performance-based design methodologies have also been implemented for specific types of coastal structures (Van De Lindt and Dao, 2009; Van De Lindt and Taggart, 2009; Do et al., 2016; Attary et al., 2017; Cui and Caracoglia, 2018) by leveraging fragility functions and evaluation of mitigation strategies. However, existing performance-based engineering frameworks are focused on punctuated hazards and generally do not consider time-varying factors such as climate change and loss of performance over time, which are key in the analysis of coastal settings into the future.
Furthermore, past coastal hazard events, such as Hurricane Sandy (Blake et al., 2013) and Hurricane Katrina (Sills et al., 2008), have showcased the relevance of considering cascading effects while assessing the risk of coastal structures and infrastructure systems. Cascading effects are a sequence of events (i.e. effects) having a common source (i.e. cause) that result in system damages and disruptions as they propagate (Pescaroli and Alexander, 2015). These paths can either escalate over time to create independent chains (i.e. effects that become the cause of secondary chains of cascading effects) or follow a linear cause-effect path (i.e. having only one cause). Pescaroli and Alexander (2015) offer further details. The damage evaluation of the system without considering the impact of cascading effects can lead to the underestimation of losses and potentially hide vulnerable components in a system. For instance, the unforeseen failure mechanisms and damages inflicted to different levees and shore-protection systems on the Louisiana coast were the key drivers of the vast devastation caused in the city of New Orleans by Hurricane Katrina (Sills et al., 2008). During Hurricane Sandy, storm-induced damages to the natural gas pipeline network triggered several fires around New Jersey (Blake et al., 2013). Moreover, waterborne debris during storm surge events has also been observed to cause major disruption to transportation networks, interrupt port operations and augment the risk of damage to structures located further from the coast (FEMA, 2009; Gonzalez Duenas et al., 2019; Padgett et al., 2008). The performance-based hurricane engineering framework (Barbato et al., 2013) implements a simplified assumption of the windborne debris as an additional source of hazard to consider the cascading effect. However, such an assumption fails to explicitly model the coupling between one component’s failure and its subsequent effect on the remaining components. The coupled modeling of the cause and effect at the component level provides the necessary framework to evaluate the overall performance of the system.
In the literature, performance-based frameworks have focused on forward uncertainty propagation methodologies (mostly Monte Carlo simulation approaches) to compute the probability of exceedance of the decision variable in an analysis (Ciampoli et al., 2011; Li et al., 2012; Barbato et al., 2013; Günay and Mosalam, 2013; Cui and Caracoglia, 2018; Attary et al., 2019; Nofal et al., 2020; Zeng et al., 2020). However, these approaches are incapable of updating uncertainties in the decision variables once new information about the condition of the system emerges. In modern coastal settings, information is continuously harnessed from sensor data, satellite imagery, and camera images during regular monitoring, condition assessments, and reconnaissance surveys. Likewise, data from component test results (e.g. mechanical properties of materials, aging and corrosion of structural components under certain environmental actions) and higher resolution or refined models (e.g. climate change models, structural behavior), are constantly being updated as further research is conducted. Thus, the incorporation of emerging observation data is needed to enhance the probabilistic estimates of the system performance.
In order to address the above mentioned drawbacks, the proposed study aims to present a Performance-Based Coastal Engineering (PBCE) framework for design and risk assessment of coastal structures considering time-varying factors, which is flexible enough to accommodate the multi-hazard and cascading hazard effects, and a range of performance objectives of interest. Furthermore, the proposed PBCE framework is implemented using a dynamic Bayesian network that constructs a probabilistic model of coastal systems in the form of a graphical network, drawing dependencies among random variables according to engineering judgment. The prior belief of the constructed probabilistic graphical model can be updated in the presence of available evidence that integrates the data-oriented learning paradigm with expert domain knowledge. As a proof of concept, the performance assessment of an elevated coastal structure and two single-bay elastoplastic frames, are presented as case studies. The former corresponds to a typical Gulf Coast residential structure subjected to wave and surge loads while considering time-varying factors. The probabilities of failure and losses for different years (2030, 2050, and 2100), under different climate change scenarios are computed. The latter investigates cascading effects by considering the failure of one of the frames and its impact in the second one. In both case studies, the posterior probabilities of the decision variables are inferred by updating the corresponding dynamic Bayesian network.
The formulation of the PBCE framework is introduced in Proposed Performance-Based Coastal Engineering Framework. The individual components of the framework, as well as its time-varying and event-triggered factors that affect the performance of coastal systems, are described. The section also presents the implementation of the framework in the form of a probabilistic graphical model and inference using a dynamic Bayesian network. Illustrative Case Studies demonstrates the application of the proposed PBCE framework in the form of two case studies: 1) the performance assessment of a typical elevated residential structure subjected to wave and surge loads, and 2) the evaluation of cascading-effects in a system of two elastoplastic frames. The proposed methodology also infers the updated probabilities of the decision variables in the presence of available quantitative and qualitative evidence.
Proposed Performance-Based Coastal Engineering Framework
Factors Affecting de Performance of Coastal Systems
Coastal regions are complex environments shaped by the dynamics of physical, social, and economic processes. Structures and infrastructure systems in these regions are often exposed to multi-hazard environments and chronic hazards, which impose additional challenges to their design and overall assessment. Moreover, the impact of coastal hazard events usually escalates when cascading failures are triggered due to system interdependencies (Blake et al., 2013). Herein, factors affecting the performance of coastal systems are classified as 1) time-varying factors, and 2) event-triggered factors. The former accounts for factors that are time-dependent and therefore affect the system during its lifetime (e.g., climate change, stiffness degradation, market changes), while the latter considers factors that are conditioned on the occurrence of an event (e.g., cascading effects).
Time-Varying Factors
Erosion, loss of natural barriers (e.g. dunes, mangrove forests), sea-level rise, and overall changes in coastal hazards (e.g. intensity, frequency), have significantly affected the exposure and vulnerability of coastal systems, as observed in the landing and aftermath of tropical cyclones around the world in the last 2 decades (Pistrika and Jonkman, 2010; Stewart and Deng, 2015; Risser and Wehner, 2017; Toimil et al., 2017; Poddar et al., 2020). Furthermore, the degradation of structural capacity due to aging, previous load history, or exposure to harsh environmental conditions, leads to differences in the response of structural and infrastructure systems during their lifetime (Li et al., 2015; Sanchez-Silva et al., 2011; Stewart et al., 2011). Changes in the use of the structure (e.g. a residential to a commercial building) and the market (e.g. devaluation, discount rate) will in turn affect the measure of performance and the losses in the aftermath of a hurricane event (Baade et al., 2007). For instance, in recent years, methodologies to address the intergenerational risk transfer for socio-economic sustainability have been proposed to avoid intergenerational inequities (Ellingwood and Lee, 2016; Lee and Ellingwood, 2017).
Event-Triggered Factors
Coastal hazards can lead to cascading failures due to the interdependencies of infrastructure systems and urban planning considerations. For instance, the failure of one residence can lead to construction debris and jeopardize the structural integrity of neighboring houses (FEMA, 2009), vegetation debris or falling trees during a hurricane cause damages in infrastructure networks (e.g. power lines) and structural systems (e.g. residential houses), and can also lead to casualties (Blake et al., 2013; WTVD-TV, 2020). Power outages have been the cause of several losses due to business interruption and deaths (e.g. cold weather), especially in vulnerable populations such as elderly people (Blake et al., 2013). Moreover, aspects such as stakeholder risk aversion (de Boer et al., 2016), perspectives on non-acceptable losses, and specific requirements of the design (e.g. sustainability), can significantly impact and change the course of decision-making processes and as a result, modify the original design of the system.
Components of the Performance-Based Coastal Engineering Framework
The proposed Performance-Based Coastal Engineering (PBCE) framework focuses on the assessment of coastal systems subjected to both chronic and punctuated natural hazards by incorporating time-varying and event-triggered factors. The PBCE framework consists of six basic components (Figure 1): 1) performance objectives, 2) hazard analysis, 3) structural characterization, 4) structural analysis, 5) damage analysis, and 6) performance analysis. This subdivision facilitates the identification and probabilistic characterization of the parameters pertinent to each component and its specific sources of uncertainties.
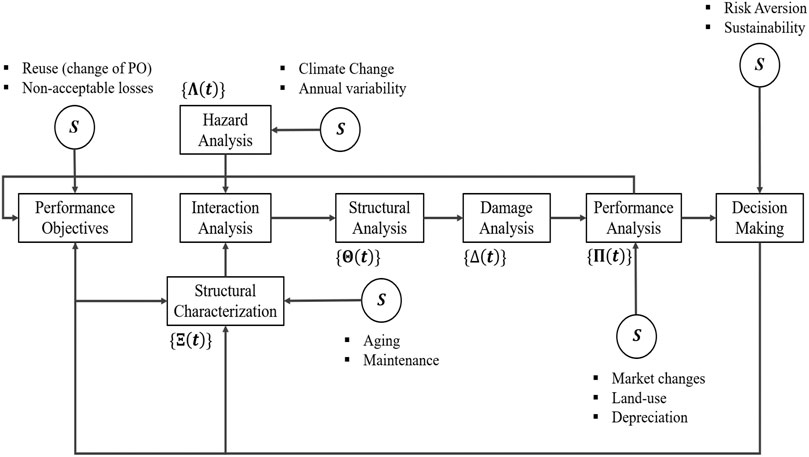
FIGURE 1. Proposed Performance-Based Coastal Engineering (PBCE) framework. The framework consists of six basic components: (1) performance objectives, (2) hazard analysis, (3) structural characterization, (4) structural analysis, (5) damage analysis, and (6) performance analysis.
Performance Objectives
Performance objectives (PO) are discrete qualitative measures of expected performance that are based on probable damages to the system given the occurrence of a hazard. Although similar nomenclature can be used across different civil engineering subfields to denote performance expectations, their definition and associated damage states vary significantly depending on the type of structure, construction material, and hazard. In the literature, performance objectives and damage classifications have been proposed for various types of coastal structures and hazards (Van De Lindt and Dao, 2009; Van De Lindt and Taggart, 2009; Ciampoli et al., 2011; Li et al., 2012; Massarra, 2012; Ataei and Padgett, 2013; Barbato et al., 2013; Attary et al., 2017; Emanuel, 2017; Park et al., 2017; Tomiczek et al., 2017; Baradaranshoraka et al., 2019; Xiong et al., 2019). PO are commonly associated with structural integrity, operational status, incurred losses, or serviceability requirements (Ciampoli et al., 2011; Barbato et al., 2013; American Society of Civil Engineers, 2017). Performance objectives can be evaluated by the damage of individual structural and nonstructural components (American Society of Civil Engineers, 2017) or the overall state of the structure in the aftermath of a hazard event (Barbato et al., 2013).
Hazard Analysis
The hazard analysis component starts with the probabilistic characterization of the vector of intensity parameters
Where
To select a suitable set of intensity parameters, consideration should be given to the hazard models selected to describe the event (Barbato et al., 2013), as well as their efficiency and sufficiency for the particular case study (i.e. hazard models might need information not available/very difficult to estimate at the site, or might be irrelevant for the overall performance of the structure) (Barbato et al., 2013). Considering an elevated residential coastal structure subjected to both significant surge and wave loads as an example, the vector of intensity parameters would be comprised of flood hazard intensity parameters (Barbato et al., 2013) such as surge depth
If wind, rainfall, or other types of hazards are seen or expected to cause significant loading to the structure during a hurricane or storm event, their respective
Structural Characterization
The uncertainty associated with the mechanical and geometrical properties of the system is described through the vector of structural parameters
for systems with more than one structure, and as
for single structures, where
Structural Analysis
The structural analysis provides the probabilistic characterization of the system response through the vector
where
Damage Analysis
The damage analysis allows the quantification of the probability of exceeding a certain damage level of a given structural element or the overall structure conditioned on their respective engineering demand parameters (EDPs). The damage function is discretized using damage states, which describe the amount of damage that the element undergoes until its complete loss of functionality or collapse when subjected to different levels of intensity (i.e. different hazard scenarios, increment in the intensity parameters). To estimate the element damage, a limit state function
where
This comparison allows defining if a certain component has failed using a Bernoulli random variable, which will state if the element has exceeded a certain damage level or not. Subsequently, the probabilistic characterization of the vector
where
To consider the possibility of complete collapse or loss of functionality of the structure, the last parameter of the damage vector is reserved to account for a global parameter that can define global collapse:
This allows checking the global stability of the system before performing computationally expensive element-wize calculations (Günay and Mosalam, 2013). Moreover, the limit state function is also used to develop fragility models that depict the probability of reaching or exceeding a damage state given intensity parameters and/or structural parameters. If fragility functions are already available for individual structural elements or the structural system itself, the component of structural analysis can be avoided, and the probability of exceeding a certain damage state is directly evaluated from the fragility model. Multiple fragility functions have been proposed in the literature for diverse types of coastal systems, with a recent shift toward parameterized fragility models, where fragility functions are conditioned on specific structural characteristics and load conditions (Ataei and Padgett, 2013; Tomiczek et al., 2014; Hatzikyriakou et al., 2016; Balomenos and Padgett, 2018; Saeidpour et al., 2018; Bernier and Padgett, 2019). In the case of ECRS under surge and wave load, most of the fragility models to date have been empirically derived in the aftermath of major hurricane events (Hatzikyriakou et al., 2016; Tomiczek et al., 2014, 2017); with some recent efforts to develop parameterized fragility functions using numerical simulations (Do, 2016; Do et al., 2020). To incorporate cascading effects, the damage parameter of an individual structure or system
Where
Performance Analysis
The component of performance analysis computes the marginal probability distribution of the decision variable DV. The selection of the decision variable implies an active communication with the stakeholders to adopt metrics that adequately portray the desired/required performance objectives of the system. A common choice of DV includes the annual economic loss or the life-cycle cost of the structure—the reason why the computation of the marginal probability distribution of the decision variable is typically known as “loss analysis” (Porter, 2003; Ciampoli et al., 2011; Barbato et al., 2013; Günay and Mosalam, 2013; Attary et al., 2017). Nevertheless, decision variables encompass various aspects of importance for stakeholders and communities, that cover a wide selection of metrics such as downtime, injuries and fatalities (Jonkman, 2007; Günay and Mosalam, 2013), carbon footprint (Dehghani and Shafieezadeh, 2019; Padgett and Tapia, 2013), environmental impact, and the threat to public health in the event of failure (Reible et al., 2006), loss of community service, and even aspects related to psychological trauma after a hazard event. Indirect costs (e.g. loss of productivity in the community, cost of power outages (Bjarnadottir et al., 2018), a decline in revenue (Becker et al., 2015), unemployment, evacuation efforts (Whitehead, 2003) and intangible consequences (e.g. ecosystem damages (Becker et al., 2015), individuals’ well-being (Berlemann, 2016)) should also be considered since they have a great effect on the total cost and impact of natural disasters (Dong and Li, 2016). Moreover, the DV can also be defined as a system metric, where the result of individual building responses is used to estimate the system-level performance, as in the case of community resilience metrics (Lounis and McAllister, 2016; Kameshwar et al., 2019) or sustainable neighborhoods (Ju et al., 2016; Wangel et al., 2016).
In the case of economic losses, the probability of exceeding a certain loss can be estimated by assigning costs to the actions needed to repair or replace the structural elements depending on the sustained damages computed in the damage analysis component. The loss associated with downtime can be estimated by computing the incurred costs due to loss of functionality of the system [e.g. business interruption, loss of household production (Rose et al., 2007)]. Moreover, different studies have proposed methodologies to assess the value of life (Landefeld and Seskin, 1982; Jonkman, 2007; Ditlevsen and Friis-Hansen, 2009; Luechinger and Raschky, 2009; Kousky, 2014; Daniell et al., 2015; Yu and Tang, 2017), however, serious ethical questions arise when estimating the cost of fatalities or injury in the aftermath of natural disasters. CO2 or other greenhouse gas emissions and embodied energy are typical DVs (Dehghani and Shafieezadeh, 2019; Lounis and McAllister, 2016; Müller et al., 2013; Padgett and Tapia, 2013) to measure the environmental impact of structures and infrastructure systems by associating costs to the emissions of the manufacturing process and transportation of construction materials (Lounis and McAllister, 2016), maintenance and repair (Dehghani and Shafieezadeh, 2019), and any detour of vehicles during the construction phase or in retrofitting, inspection, and repair actions (Lounis and McAllister, 2016; Padgett and Tapia, 2013). Furthermore, decision variables concerning public health, the individual’s well-being, and psychological impact can be associated with the expected number of failures of residences and special structures such as hospitals in a community in the aftermath of a hazard event. For instance, failure of residences during storm-surge events can release toxic substances into the environment in the form of construction debris, household-stored chemicals, fuel in flooded cars, and their improper disposal (Reible et al., 2006), jeopardizing the health of entire communities. Potentially more than one decision variable can be evaluated to analyze different consequences of system or element failure.
The vector
where
and
where the
Therefore, for the case of community metrics
where
Time-Dependent Probabilistic Graphical Model
With the main components of the PBCE defined, a mathematical abstraction of this framework is pursued, first with an overview of the adopted model—a dynamic Bayesian network, followed by an example of how to construct a dynamic Bayesian network from the PBCE framework representation. Probabilistic graphical models, such as Bayesian and dynamic Bayesian networks, provide a flexible approach for model updating using evidence and have been successfully implemented in the assessment of civil structures and infrastructure systems (Bensi et al., 2013; Schultz and Smith, 2016; Khakzad and Van Gelder, 2018). Moreover, the basic construction of probabilistic graphical models using nodes and links, allows direct consideration of dependencies (links) between different random variables (nodes) (Bensi et al., 2013), providing not only an efficient sampling strategy but also the easy incorporation of engineering judgment in the model while facilitating communication with stakeholders. This section provides a general overview of Bayesian networks followed by the case of dynamic Bayesian networks. Subsequently, their relevance and adaptation to modeling the PBCE problem are described.
Bayesian Network
Bayesian networks (BN) are directed acyclic probabilistic graphical models that represent the joint probability density function
The marginal probability density function (pdf) of any random variable is computed by performing integration of the joint pdf over the remaining random variables. For example
where
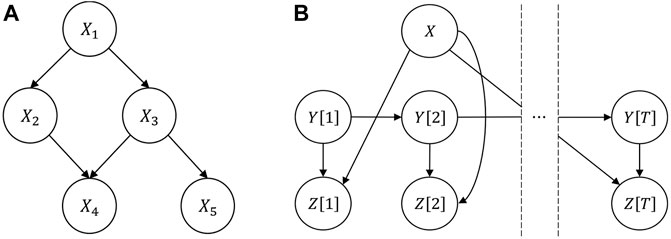
FIGURE 2. (A) Basic example of a Bayesian network. Nodes
Dynamic Bayesian Network
A Dynamic Bayesian Network (DBN) is an extension of classical BN which is capable of modeling the uncertainties of discrete time-varying systems (Murphy and Russell, 2002). Figure 2B shows an example of a DBN that models the probabilities of the set of random variables
where
For both BN and DBN, the child node originates from one or more parent nodes which are modeled through a directed arrow. The nodes that share a child node but are not children of that node are spouse nodes. The parent, child, and spouse nodes, together, form a Markov blanket. Apart from the nodes within the Markov blanket of a node, that random variable is independent of all other variables present in the network. The efficient sampling and inference of the joint pdf of the network are attributed to this concept of d-separation (Markov blanket) of a node in the network (Pearl, 2014). As new evidence of any node becomes available, the joint pdf of the network gets updated following the Bayes’ theorem, and the posterior distribution of the random variables that are inside the Markov blanket of the observed random variable gets updated. However, the marginal pdfs of those random variables that are d-separated from the observed node do not change. It is noteworthy to mention, that in the context of BNs and DBNs, evidence constitutes any measurement associated with one or more of the random variables in the network. New models or forecasts of the relations between the random variables (links) can be incorporated, but this would imply the modification of the network and a new model.
Dynamic Bayesian Network Modeling of the Performance-Based Coastal Engineering Framework
One of the key features of the proposed PBCE framework is its adaptability to model time-varying systems through the incorporation of time-varying parameters into the description of each of the components presented in Components of the Performance-Based Coastal Engineering Framework. DBNs offer the capability of modeling discrete-time or space varying systems and allow to represent stochastic processes by constructing a BN over each discrete point in time (Straub, 2009). To model the flow of information from one discrete-time index
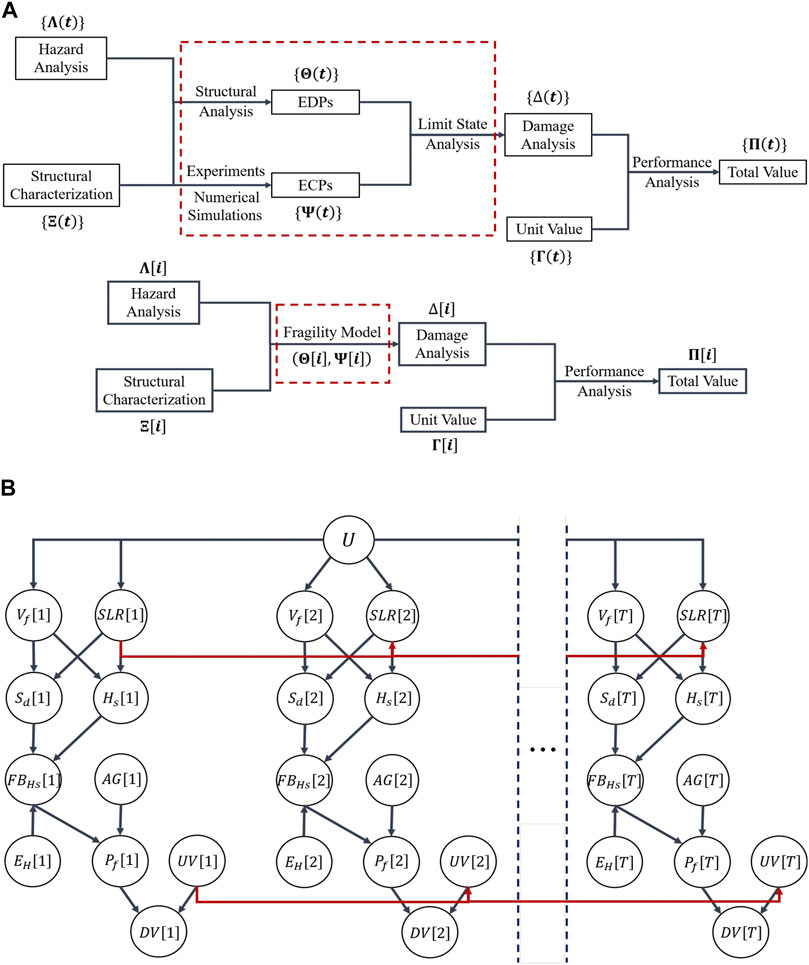
FIGURE 3. (A) Performance-Based Coastal Engineering framework representation of a typical Gulf Coast elevated residential structure subjected to storm surge and wave loading, defined both as continuous and discrete (i.e., at a time index
In this case study, the intensity parameters are selected as the significant wave height
With the nodes and their basic dependencies (links) defined, the DBN can be constructed by replicating the basic BN structure at each time step and connecting the nodes that have a time-variant relation. Figure 3B shows the DNB constructed for the house, where it is assumed that the time variation of the sea-level rise and the unit value of the replacement cost of the house are represented with the aid of a mathematical expression. For illustration, the probability distribution of the hazard parameters is assumed to be conditioned on a random influencing variable
To handle these situations, approximate inference algorithms such as Markov Chain Monte Carlo (MCMC) are used to avoid the exact computation of integrals by estimating the posterior distribution using ergodic averages (Yildirim, 2012). The Gibbs sampling algorithm is an MCMC technique that allows the efficient computation of large BNs, in which the Monte Carlo estimates of the marginal pdfs of each node are obtained by generating samples from a Markov chain. In this way, the explicit computation or inversion of the joint pdf is averted to generate samples from a complex marginal pdf. This is done by generating samples of the posterior distribution of a random variable in its conditional distribution form (as presented in Eq. 14 and Eq. 17) while maintaining the other random variables fixed until convergence. This is repeated for all the random variables in the BN. Nevertheless, convergence to the true joint probability distribution is only ensured for a large number of samples (i.e., when we reach the stationary state), which means that the early random samples might not be representative of it. Therefore, these initial samples are usually discarded, known as the tuning process or bur-in period (Yildirim, 2012). Details on the Gibbs sampling strategy can be found in (Yildirim, 2012). In this study, Markov chain Monte Carlo simulation with Gibbs sampling is applied. OpenBUGS (Spiegelhalter et al., 2007), an open-source Bayesian inference software, is used to implement the DBN model for the case studies discussed in the subsequent section. According to the best knowledge of the authors, this is the first instance of DBN adaptation to model a time-dependent PBE framework. Although this paper focuses on the PBCE framework, modeling concepts of DBN are easily transferable to other PBE frameworks.
Illustrative Case Studies
In this section, two case studies are introduced to illustrate the application of the PBCE framework and highlight two of its key features, the incorporation of time-varying parameters and cascading effects. First, the DBN constructed for the elevated residential structure in Dynamic Bayesian Network Modeling of the Performance-Based Coastal Engineering Framework (Figure 3B) is used to evaluate the probable economic loss associated with the replacement cost of the house (DV) under surge and wave loading. However, due to a lack of information on the year-to-year time variation of the parameters in the DBN model of the house, the marginal probability of the DV is computed independently for the years 2030, 2050, and 2100, by evaluating their respective BNs. For each year, the dependencies of the parameters and their mathematical description are elaborated to compute the marginal probability distribution of the economic loss given that a storm characteristic of the hazard conditions of that year occurs. The second case study corresponds to a system of two frames where the applicability of the framework to model cascading effects and time-varying systems is showcased. The year-to-year variation of relevant parameters in the model is included and the DBN evaluated for an illustrative period of analysis. In both examples, the evidence is incorporated into the model to demonstrate its effect in the estimates of the decision variable and highlight the flexibility of the framework to integrate new information in the model as it becomes available.
Realistic System: Existing Elevated Residential Structure
The residential building stock is one of the most vulnerable systems to hurricane hazard, and in a general sense, it dictates the overall impact of a storm in a community being a key component of the response before and after the hazard. Herein, a representative Gulf Coast wood-frame house is used to showcase the potential impact of storm surge and wave loading in the residential building stock when considering time-varying factors such as climate change and structural degradation. The house is selected randomly from a building inventory from Galveston Island, Texas (Fereshtehnejad et al., 2021). This database contains information on more than 15,000 structures and features such as geographical coordinates, year of construction, the elevation of the house with respect to the ground, and socio-demographical data (Fereshtehnejad et al., 2021). As mentioned in Dynamic Bayesian Network Modeling of the Performance-Based Coastal Engineering Framework, the house is elevated on piles, with a 4.572 m (15 ft) elevation above ground and was constructed in the year 2000. In general, coastal residential structures are designed to avoid the effects of surge and waves, which in turn makes the failure probability models highly sensitive to the free-board height. To better capture this effect, a lognormal distribution
Hazard Analysis
Galveston Island is located in a hurricane-prone region susceptible to the effects of storm surge and wave loading (FEMA, 2009; Liu and Irish, 2019). To consider the effect of a changing climate on the impact of future storms, the forward velocity of the storm
To parameterize the intensity parameters for the specific location of interest, a regression model is developed for both significant wave height and surge depth as a function of the hazard parameters, the storm forward velocity, and sea-level rise. The polynomial model with third-order in
where
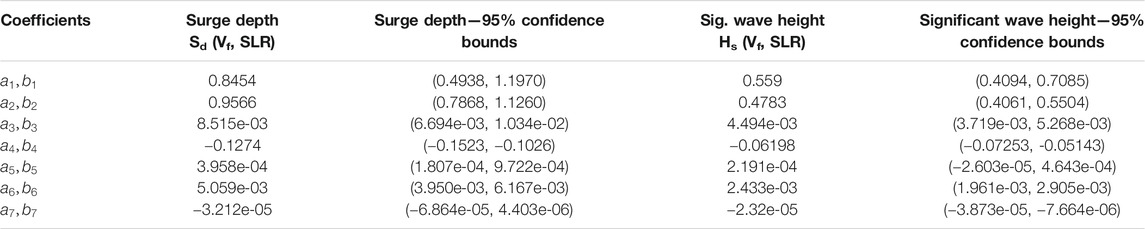
TABLE 1. Coefficients of the polynomial regression model and 95% confidence bounds for surge depth and significant wave height.
Sea-level rise projections and expected changes in the forward velocity of tropical cyclones are considered for three different years: 2030, 2050, and 2100. For each year, the local sea-level projections for Galveston proposed by Kopp et al. (2014) are used to define a normal distribution
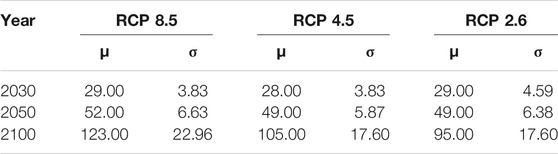
TABLE 2. Parameters of the normal distribution models for sea-level rise projections in Galveston, TX.
Structural Characterization, Structural Analysis, and Damage Analysis
The empirical fragility model proposed by Tomiczek et al. (2014) for wood-framed residences in the Galveston area following Hurricane Ike is used in this study to predict the probability of collapse (i.e. severe damage state) of the representative case study structure. Although the adopted fragility model has noted limitations, it also has the advantages of being representative of the construction practices of the region and developed for a tropical cyclone with predominant surge and wave loading effects (FEMA, 2009). The fragility model variant number five presented in (Tomiczek et al., 2014) is adopted in this study, which is a function of significant wave height
where
To capture the degradation of the structure over time, a reduction factor
Eq. 20 shows the modified fragility function. The strengthening of the structure is represented by considering positive values of R (Bjarnadottir et al., 2011).
Performance Analysis
As mentioned in Dynamic Bayesian Network Modeling of the Performance-Based Coastal Engineering Framework, the unit value UV is defined as the replacement cost of the structure
where
The replacement cost of the structure in the present (2020) value dollars is estimated as
The decision variable DV is selected as the expected economic loss in case of failure given the occurrence of a hurricane in the year under analysis (2030, 2050, and 2100, in this case study). Therefore, for a given year,
where
Results and Discussion
OpenBUGS (Spiegelhalter et al., 2007) is used to implement the BN models of the 3 years of analysis. The convergence of Markov chain Monte Carlo (MCMC) simulation is ensured by computing the Gelman-Rubin statistic
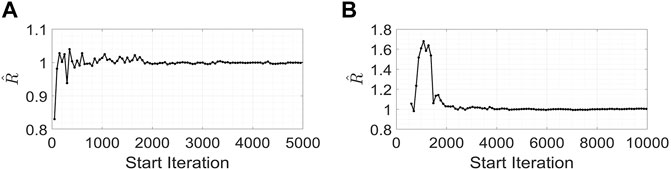
FIGURE 4. The convergence of MCMC simulation is ensured by computing the Gelman-Rubin statistic
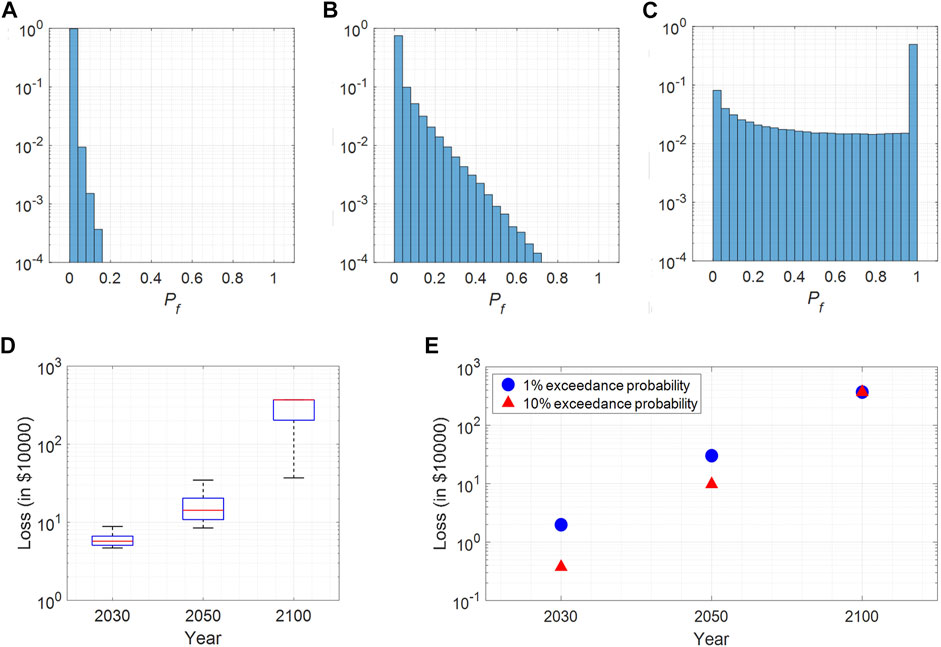
FIGURE 5. (A) The normalized histogram of failure probability of the house in the year 2030, (B) 2050, and (C) 2100, (D) the boxplot of the conditional distribution of loss given the probability of failure is more than 10%. The blue box limits the 25 and 75 percentile loss, the red line denotes the median loss, and the two end whiskers denote 5 and 95 percentile loss (E) the marginal loss corresponding to 1 and 10% exceedance probability for the years 2030, 2050 and 2100.
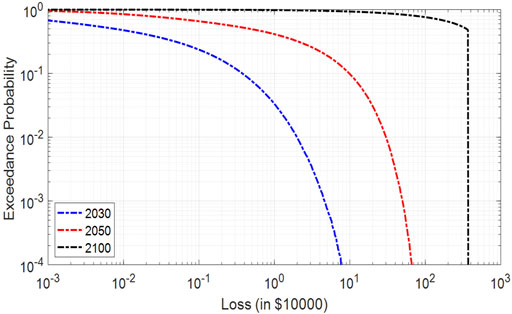
FIGURE 6. The variation of exceedance probability corresponding to the loss for the years 2030, 2050, and 2100.
Figure 5D shows the boxplot of the conditional distribution of loss
To illustrate the incorporation of evidence in the PBCE framework for the residential structure, the influence of new knowledge on the loss estimates is evaluated. Specifically, evidence related to measurements of the elevation of the house
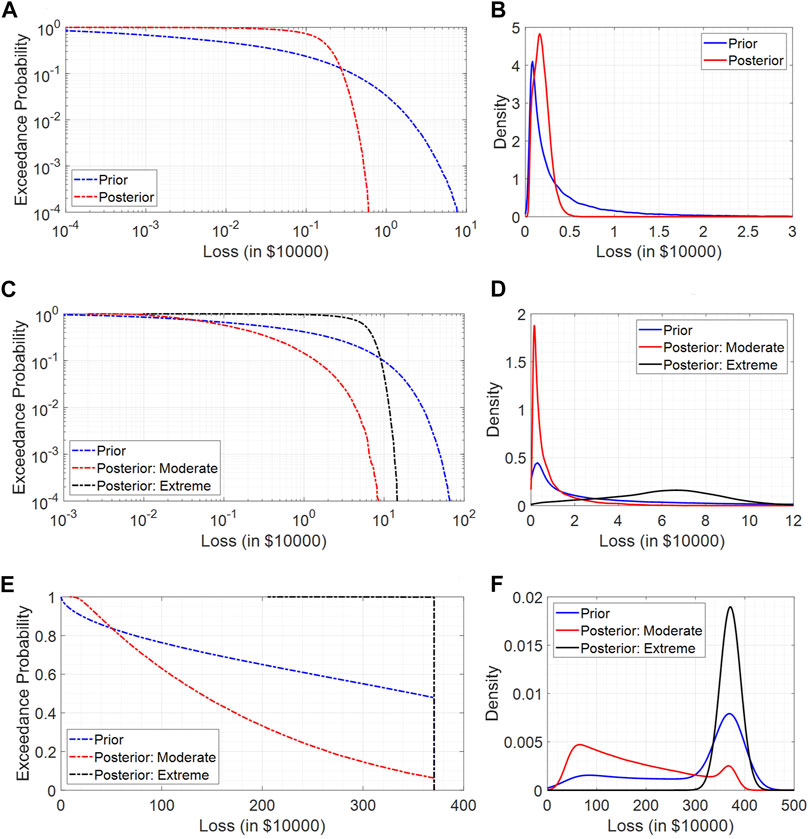
FIGURE 7. (A) The variation of the prior and the posterior exceedance probability corresponding to the loss for the year 2030 (B) the prior and the updated conditional posterior distribution of loss given probability of failure is more than 0.1% for the year 2030, (C) variation of the prior and the posterior exceedance probability corresponding to the loss under the observation of two different climate scenarios (moderate and extreme), for the year 2050, (D) the prior and the updated conditional posterior distribution of loss given probability of failure is more than 0.1% under the observation of two different climate scenarios (moderate and extreme), for the year 2050, (E) the variation of the prior and the posterior exceedance probability corresponding to the loss under the observation of two different climate scenarios (moderate and extreme), for the year 2100, (F) the prior and the updated conditional posterior distribution of loss given probability of failure is more than 10% under the observation of two different climate scenarios (moderate and extreme), for the year 2100.
For the years 2050 and 2100, we consider two climate scenarios, a moderate one where
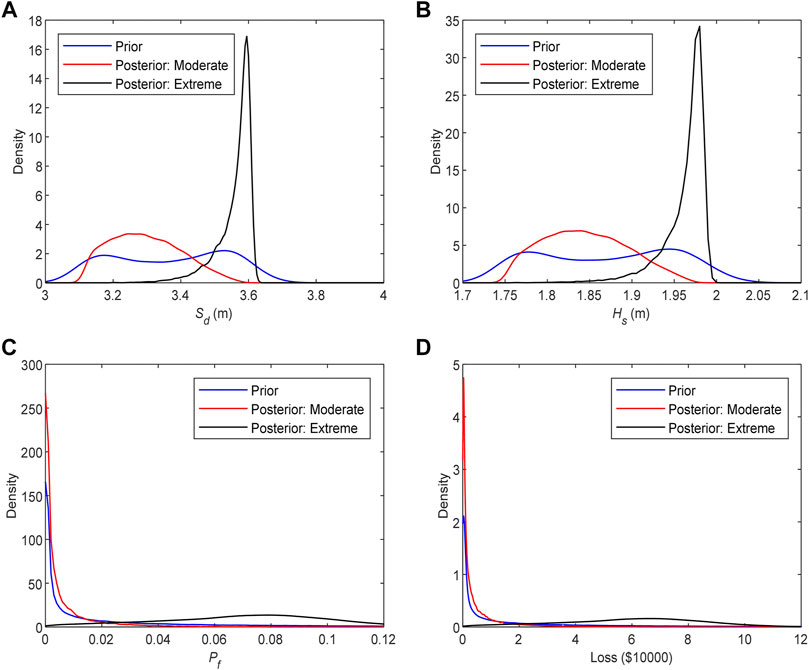
FIGURE 8. The effect of different sets of evidence (moderate vs. extreme climate scenario) in the marginal posterior distribution of the loss for the year 2050.
Finally, to illustrate how new evidence updates the posterior distribution of all the random variables that are inside the Markov blanket, Figure 9 shows the change in the posterior distribution of RCP under the two different climate scenarios. It is apparent that the parent node (
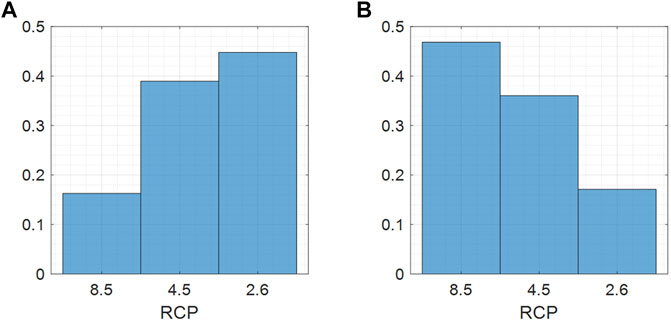
FIGURE 9. Variation in the posterior distribution of RCP under the two different climate scenarios: (A) moderate, and (B) extreme.
Representative System: Two Single-Bay Elastoplastic Bay
In this example, the impact of cascading effects and time-varying parameters in the marginal distribution of the DV are investigated. A system consisting of two single-bay elastoplastic frames is used to illustrate the effects of cascading failure in the form of debris load (Figures 10A,B). Moreover, the time-varying parameters of the system are incorporated into the analysis using a DBN representation of the PBCE framework. The single-bay frame structure is adapted from (Der Kiureghian, 2005; Straub and Der Kiureghian, 2010). Due to computational constraints, a service life of 15 years instead of the 20-years from the original example (Straub and Der Kiureghian, 2010), is selected for both the frames to illustrate the DBN modeling of the PBCE framework. Both frames are subjected to the same vertical
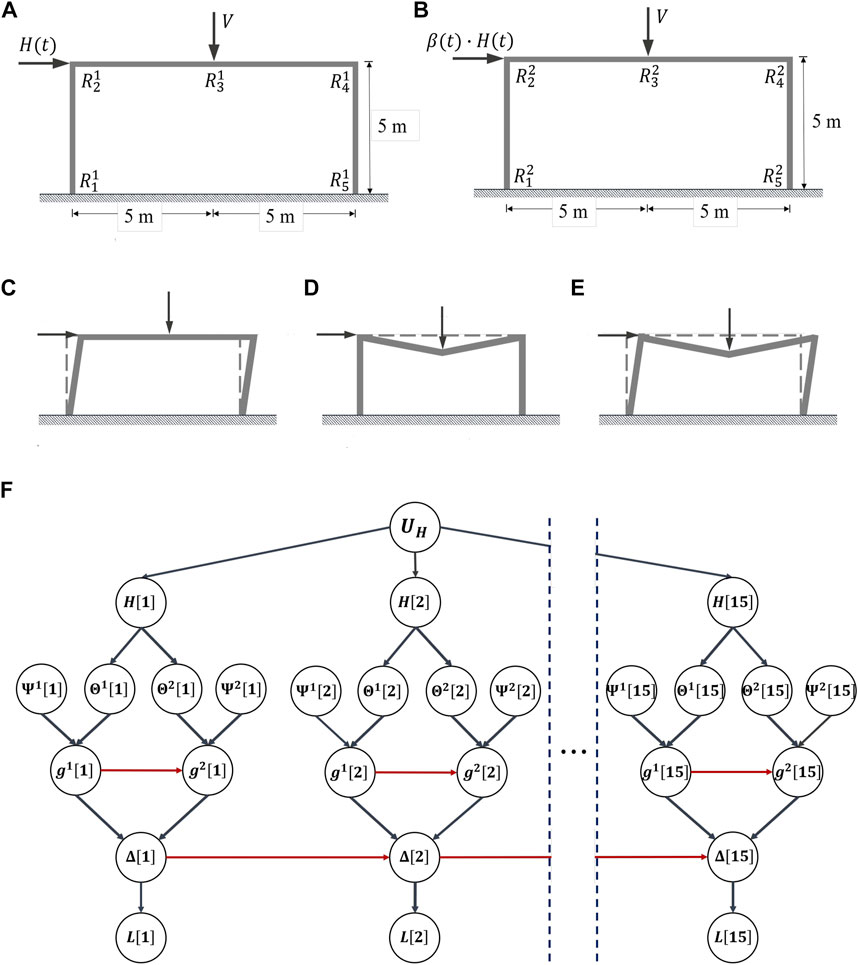
FIGURE 10. Typical single-bay elastoplastic frame model adapted from (Der Kiureghian, 2005; Straub and Der Kiureghian, 2010). In the two-frame system, each frame is subjected to vertical
Three different structural failure mechanisms (i.e. sway (Figure 10C), beam (Figure 10D), and combined (Figure 10E)) are defined for each frame by the limit-state functions presented in Eq. 24a, Eq. 24b, and Eq. 24c, where the capacity is given by the five plastic-moment capacities for both of the frames
where
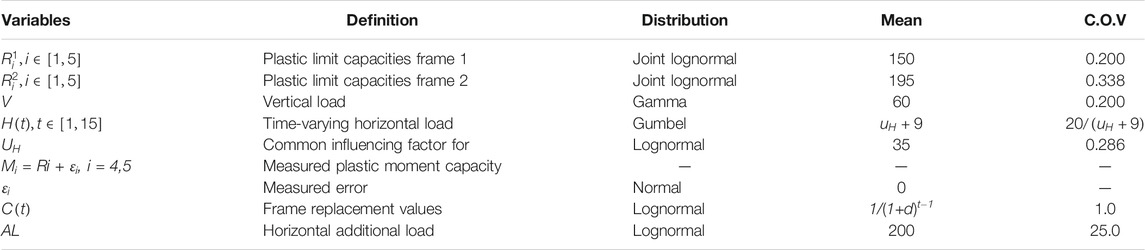
TABLE 3. A probabilistic model for the two single-bay elastoplastic frames system. Forces are in units of
Two decision variables associated with the expected economic loss in case of failure of the system are investigated in this case study, the 1) service-life loss
OpenBUGS (Spiegelhalter et al., 2007) is used to implement the DBN model of the two scenarios previously discussed for the cascading and no-cascading cases. The convergence of the MCMC simulation is ensured by computing the Gelman-Rubin statistic
Results and Discussion
Effect of Cascading Failure
The effect of cascading failure is analyzed by comparing the exceedance probability and density function of the normalized service-life loss
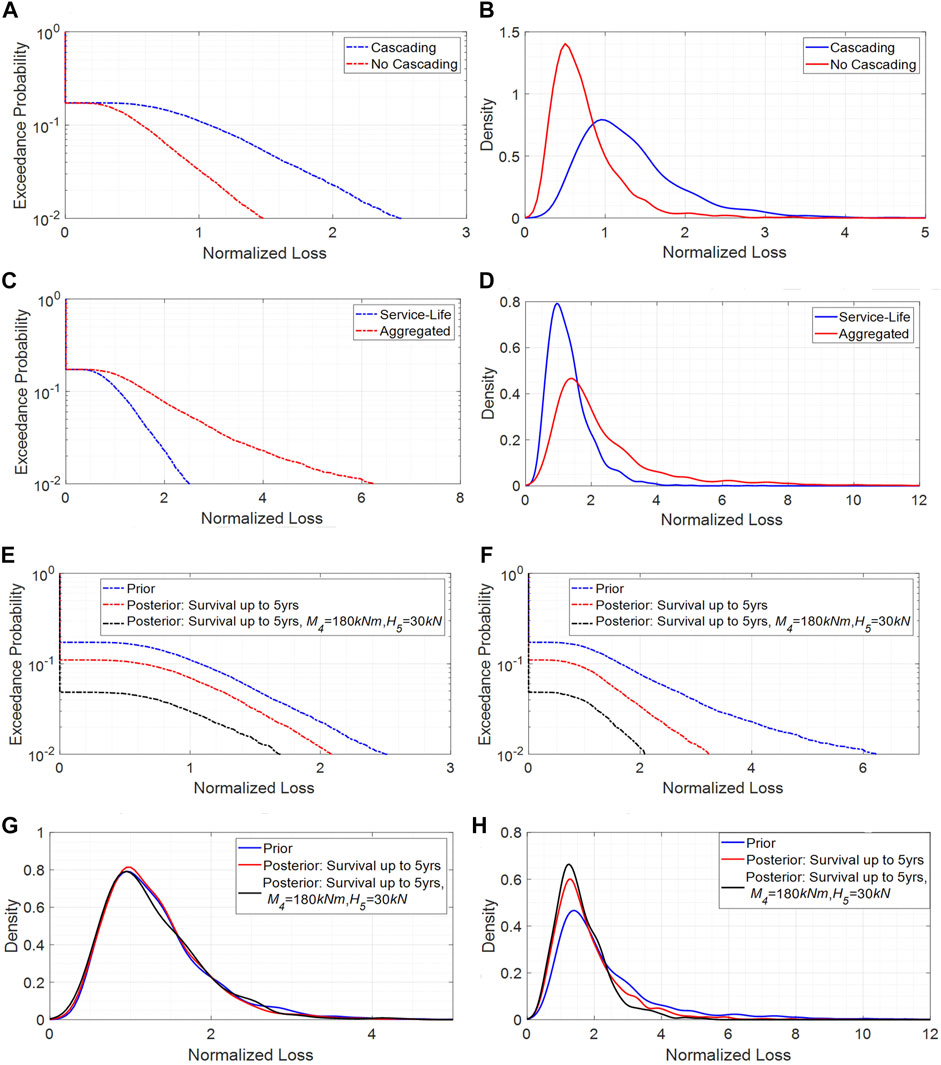
FIGURE 11. (A) Variation of exceedance probability corresponding to its normalized loss with and without the consideration of the effect of cascading failure, (B) conditional distribution of the normalized loss given at least one of the two frames have damaged, for both sets of analysis with and without considering cascading effect. (C) Comparison of the exceedance probability of normalized service-life loss and aggregated loss at the end of 15 years, (D) conditional distribution of the normalized service-life loss and aggregated loss given that at least one of the two frames have damage at the end of 15 years. The prior and posterior variation of exceedance probability corresponding to its (E) normalized service-life loss and (F) normalized aggregated loss at the end of 15 years. The conditional prior and posterior distributions of the (G) normalized service-life loss and (H) normalized aggregated loss given that at least one of the two frames have damage at the end of 15 years.
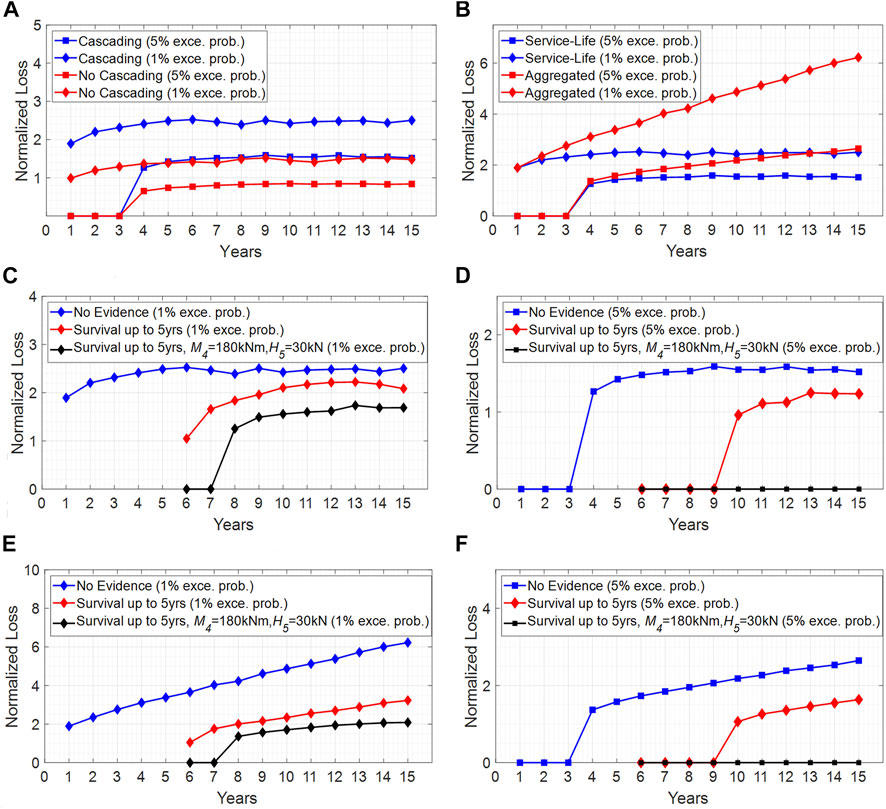
FIGURE 12. (A) Normalized loss corresponding to 1 and 5% exceedance probability for varying service-life, with and without the consideration of the effect of cascading failure, (B) normalized service-life loss and aggregated loss corresponding to 1 and 5% exceedance probability for varying service-life, (C) the prior and posterior normalized service-life loss corresponding to (C) 1% and (D) 5% exceedance probability for varying service-life, (E) the prior and posterior normalized aggregate loss corresponding to (E) 1% and (F) 5% exceedance probability for varying service-life 1 and 15 years.
Service-Life Loss Compared to Aggregated Loss
Having established the importance of considering cascading effects, the results from the present and following sections consider cascading failure in the analysis. Figure 11C presents a comparison of the exceedance probability for the normalized service-life loss and aggregated loss at the end of 15 years. As expected, the exceedance probability of aggregated loss is more than service-life loss due to recurrent repair of the system after each damage. Moreover, the assumption of repair after each damage scenario increases the uncertainty of aggregated loss compared to the service-life loss as observed in the conditional distribution of the normalized service-life loss and aggregated loss given that at least one of the two frames have damage at the end of 15 years, as shown in Figure 11D. The normalized service-life loss and aggregated loss corresponding to 1 and 5% exceedance probability for varying service-life between 1 and 15 years are depicted in Figure 12B. The assumption of repair after each damage scenario naturally increases the corresponding aggregated loss compared to the case of service-life loss over time. Moreover, when comparing the change of service-life and aggregated loss during the 15-years of analysis, it is seen that the difference between the 5 and 1% probability of exceedance of the service-life loss is significantly lower compared to the aggregated loss. This shows that the probability density function of the aggregated loss has a longer upper tail than the service-life loss distribution and that this difference increases in time, as seen by the growing gap between the 1 and 5% probability of exceedance (red curves in Figure 12B).
Effect of Evidence in Service-Life Loss and Aggregated Loss
As discussed in Dynamic Bayesian Network Modeling of the Performance-Based Coastal Engineering Framework, the proposed dynamic Bayesian network model enables updating of the prior distribution of the network variables to obtain the posterior distributions when evidence about any variable emerges, both qualitative and quantitative. In this section, two sets of evidence are considered which are observed at the end of 5 years for exemplification purposes. The first one involves qualitative evidence about both of the frames where it is observed that both frames have survived until then (i.e. no observed damage to the frame at the end of 5 years). The second set includes both qualitative and quantitative evidence. In addition to the observation of the 5-years survival of both frames, quantitative evidence about the structure and the hazard are observed at the end of the fifth year. Specifically, the plastic moment capacity at
Figures 12C–D present the prior and posterior normalized service-life loss corresponding to 1% (Figure 12C) and 5% (Figure 12D) exceedance probability between 1 and 15 years. In both cases, the posterior marginal distribution of the loss is sensitive to observed evidence. Moreover, the incorporation of both qualitative and quantitative evidence significantly reduces the probability of incurred service-life loss, when compared to the prior and qualitative-only marginal distributions of the loss. Figure 12D shows that there is a 95% probability that the incurred service-life loss is zero when the two sets of evidence are observed. This behavior is also observed in the marginal distribution of the aggregated loss, as per Figures 12E,F.
Conclusion
The proposed performance-based coastal engineering framework provides a methodology for system performance assessment focused on coastal systems that incorporates new concepts such as time-varying and event-triggered factors into the analysis. The framework consists of six basic components: performance objectives, hazard analysis, structural characterization, structural analysis, damage analysis, and performance analysis. The framework is flexible enough to accommodate multi-hazard scenarios, systems with multiple structures, cascading-effects, and different performance metrics. To compute the marginal probability distribution of the decision variable, this study proposes the implementation of a probabilistic graphical model in the form of a dynamic Bayesian network. DBNs allow modeling the causal dependence among the variables, offering an efficient sampling strategy and facilitating communication with stakeholders and easy incorporation of expert knowledge. The dynamic Bayesian network is constructed based on the relevant parameters of the system, only needing their probabilistic description or estimates. In cases with no information of the probabilistic description of the parameters, non-informative priors can be used, such as a uniform distribution having limits based on engineering judgment or “best estimates”. Moreover, DBNs allow the incorporation of evidence, increasing the confidence of the parameter estimates through Bayesian updating. Two case studies showcase the application of the framework. The first one explored the effects of time-varying factors in the performance assessment of a typical elevated residential structure subjected to surge and wave loads for the years 2030, 2050, and 2100. Results showed the sensitivity of the probability of failure and probable loss of the house to the climate conditions, especially to the
Future studies will incorporate a fully probabilistic time-varying hazard model to probabilistically characterize the intensity parameters acting upon the structure for each year and its respective annual probability of exceedance. Moreover, real systems consisting of multiple structures or sub-components can be used as test beds of the framework to quantify the impact of cascading effects in a full probabilistic manner, as well as incorporating multi-hazard settings. Future directions of study can also include the consideration of feedback loops such as the change of performance objectives due to non-acceptable values of the decision variables or new inputs during the decision analysis component. Likewise, the framework can also be used to evaluate systems considering more than one decision variable and investigate optimization techniques to make decisions based on the analysis. Related opportunities in the field include the advancement of fragility functions specific to coastal structures and
Data Availability Statement
The raw data supporting the conclusion of this article will be made available by the authors, without undue reservation.
Author Contributions
CG-D: conceptualization, methodology, data collection, modeling, analysis, investigation, visualization, writing—original draft. JP: conceptualization, supervision, writing—review and editing, funding acquisition, project administration.
Conflict of Interest
The authors declare that the research was conducted in the absence of any commercial or financial relationships that could be construed as a potential conflict of interest.
Acknowledgments
The authors gratefully acknowledge the financial support of this research by the National Science Foundation under awards No. OISE-1545837 and CMMI-2002522.
References
Ataei, N., and Padgett, J. E. (2013). Limit State Capacities for Global Performance Assessment of Bridges Exposed to hurricane Surge and Wave. Struct. Saf. 41, 73–81. doi:10.1016/j.strusafe.2012.10.005
Attary, N., Unnikrishnan, V. U., van de Lindt, J. W., Cox, D. T., and Barbosa, A. R. (2017). Performance-Based Tsunami Engineering Methodology for Risk Assessment of Structures. Eng. Structures 141, 676–686. doi:10.1016/j.engstruct.2017.03.071
Attary, N., Van De Lindt, J. W., Barbosa, A. R., Cox, D. T., and Unnikrishnan, V. U. (2019). Performance-Based Tsunami Engineering for Risk Assessment of Structures Subjected to Multi-Hazards: Tsunami Following Earthquake. J. Earthquake Eng. 2019, 1–20. doi:10.1080/13632469.2019.1616335
Baade, R. A., Baumann, R., and Matheson, V. (2007). Estimating the Economic Impact of Natural and Social Disasters, with an Application to Hurricane Katrina. Urban Stud. 44 (11), 2061–2076. doi:10.1080/00420980701518917
Balomenos, G. P., and Padgett, J. E. (2018). Fragility Analysis of Pile-Supported Wharves and Piers Exposed to Storm Surge and Waves. J. Waterway, Port, Coastal Ocean Eng. 144 (2), 04017046. doi:10.1061/(ASCE)WW.1943-5460.0000436
Baradaranshoraka, M., Pinelli, J.-P., Gurley, K., Zhao, M., Peng, X., and Paleo-Torres, A. (2019). Characterization of Coastal Flood Damage States for Residential Buildings. Asce-asme J. Risk Uncertainty Eng. Syst. Part. A: Civ. Eng. 5 (1), 04019001. doi:10.1061/AJRUA6.0001006
Barbato, M., Petrini, F., Unnikrishnan, V. U., and Ciampoli, M. (2013). Performance-Based Hurricane Engineering (PBHE) Framework. Struct. Saf. 45, 24–35. doi:10.1016/j.strusafe.2013.07.002
Becker, A. H., Matson, P., Fischer, M., and Mastrandrea, M. D. (2015). Towards Seaport Resilience for Climate Change Adaptation: Stakeholder Perceptions of hurricane Impacts in Gulfport (MS) and Providence (RI). Prog. Plann. 99, 1–49. doi:10.1016/j.progress.2013.11.002
Bensi, M., Kiureghian, A. D., and Straub, D. (2013). Efficient Bayesian Network Modeling of Systems. Reliability Eng. Syst. Saf. 112, 200–213. doi:10.1016/j.ress.2012.11.017
Berlemann, M. (2016). Does hurricane Risk Affect Individual Well-Being? Empirical Evidence on the Indirect Effects of Natural Disasters. Ecol. Econ. 124, 99–113. doi:10.1016/j.ecolecon.2016.01.020
Bernier, C., and Padgett, J. E. (2019). Fragility and Risk Assessment of Aboveground Storage Tanks Subjected to Concurrent Surge, Wave, and Wind Loads. Reliability Eng. Syst. Saf. 191, 106571. doi:10.1016/j.ress.2019.106571
Bjarnadottir, S., Li, Y., Reynisson, O., and Stewart, M. G. (2018). Reliability-based Assessment of Climatic Adaptation for the Increased Resiliency of Power Distribution Systems Subjected to Hurricanes. Sustainable Resilient Infrastructure 3 (1), 36–48. doi:10.1080/23789689.2017.1345255
Bjarnadottir, S., Li, Y., and Stewart, M. G. (2011). A Probabilistic-Based Framework for Impact and Adaptation Assessment of Climate Change on hurricane Damage Risks and Costs. Struct. Saf. 33 (3), 173–185. doi:10.1016/j.strusafe.2011.02.003
Blake, E. S., Kimberlain, T. B., Berg, R. J., CangiaLosi, J. P., and Beven, J. L. (2013). Tropical Cyclone Report Hurricane Sandy (AL182012) 22–29. doi:10.1017/CBO9781107415324.004
Blake, E. S., and Zelinsky, D. A. (2018). National Hurricane Center Tropical Cyclone Report. Hurricane Harvey.
Briaud, J. L. (2015). Scour Depth at Bridges: Method Including Soil Properties. I: Maximum Scour Depth Prediction. J. Geotechnical Geoenvironmental Eng. 141 (2), 1–13. doi:10.1061/(ASCE)GT.1943-5606.0001222
Camargo, S. J., and Wing, A. A. (2021). Increased Tropical Cyclone Risk to Coasts. Science 371 (6528), 458–459. doi:10.1126/science.abg3651
Carther, S. (2020). Understanding the Time Value of Money. Investopedia. https://www.investopedia.com/articles/03/082703.asp.
Cavalli, A., Cibecchini, D., Togni, M., and Sousa, H. S. (2016). A Review on the Mechanical Properties of Aged wood and Salvaged Timber. Construction Building Mater. 114, 681–687. doi:10.1016/j.conbuildmat.2016.04.001
Chen, J. (2020). Future Value (FV). Investopedia. https://www.investopedia.com/terms/f/futurevalue.asp.
Choe, D.-E., Gardoni, P., Rosowsky, D., and Haukaas, T. (2008). Probabilistic Capacity Models and Seismic Fragility Estimates for RC Columns Subject to Corrosion. Reliability Eng. Syst. Saf. 93 (3), 383–393. doi:10.1016/j.ress.2006.12.015
Ciampoli, M., Petrini, F., and Augusti, G. (2011). Performance-Based Wind Engineering: Towards a General Procedure. Struct. Saf. 33 (6), 367–378. doi:10.1016/j.strusafe.2011.07.001
Crews, K. I., and MacKenzie, C. (2008). Development of Grading Rules for Re-cycled Timber Used in Structural Applications. World Conference on Timber Engineering. hdl.handle.net/10453/11172.
Cui, W., and Caracoglia, L. (2018). A Unified Framework for Performance-Based Wind Engineering of Tall Buildings in hurricane-prone Regions Based on Lifetime Intervention-Cost Estimation. Struct. Saf. 73, 75–86. doi:10.1016/j.strusafe.2018.02.003
Daniell, J. E., Schaefer, A. M., Wenzel, F., and Kunz-Plapp, T. (2015). The Value of Life in Earthquakes and Other Natural Disasters: Historical Costs and the Benefits of Investing in Life Safety. Proc. Tenth Pac. Conf. Earthquake Eng. 130.
de Boer, J., Botzen, W. J. W., and Terpstra, T. (2016). Flood Risk and Climate Change in the Rotterdam Area, The Netherlands: Enhancing Citizen's Climate Risk Perceptions and Prevention Responses Despite Skepticism. Reg. Environ. Change 16 (6), 1613–1622. doi:10.1007/s10113-015-0900-4
Dehghani, N. L., and Shafieezadeh, A. (2019). “Probabilistic Sustainability Assessment of Bridges Subjected to Multi-Occurrence Hazards,” in International Conference on Sustainable Infrastructure 2019: Leading Resilient Communities through the 21st Century, 555–565. doi:10.1061/9780784482650.059
Der Kiureghian, A. (2005). “First- and Second-Order Reliability Methods,” in Engineering Design Reliability Handbook, 1–24.
Ditlevsen, O., and Friis-Hansen, P. (2009). Cost and Benefit Including Value of Life, Health and Environmental Damage Measured in Time Units. Struct. Saf. 31 (2), 136–142. doi:10.1016/j.strusafe.2008.06.010
Do, T. Q., van de Lindt, J. W., and Cox, D. T. (2020). Hurricane Surge-Wave Building Fragility Methodology for Use in Damage, Loss, and Resilience Analysis. J. Struct. Eng. 146 (1), 04019177. doi:10.1061/(asce)st.1943-541x.0002472
Do, T. Q. (2016). Fragility Approach for Performance-Based Design in Fluid-Structure Interaction Problems, Part I: Wind and Wind Turbines, Part II: Waves and Elevated Coastal Structures. Colorado State University.
Do, T. Q., van de Lindt, J. W., and Cox, D. T. (2016). Performance-based Design Methodology for Inundated Elevated Coastal Structures Subjected to Wave Load. Eng. Structures 117, 250–262. doi:10.1016/j.engstruct.2016.02.046
Dong, Y., and Li, Y. (2016). Risk-based Assessment of wood Residential Construction Subjected to hurricane Events Considering Indirect and Environmental Loss. Sust. Resilient Infrastructure 1 (1–2), 46–62. doi:10.1080/23789689.2016.1179051
Ebad Sichani, M., Anarde, K. A., Capshaw, K. M., Padgett, J. E., Meidl, R. A., Hassanzadeh, P., et al. (2020). Hurricane Risk Assessment of Petroleum Infrastructure in a Changing Climate. Front. Built Environ. 6, 104. doi:10.3389/fbuil.2020.00104
Ebersole, B. A., Massey, T. C., Melby, J. A., Nadal-Caraballo, N. C., Hendon, D. L., Richardson, T. W., et al. (2017). Interim Report—IKE dike Concept for Reducing hurricane Storm Surge in the Houston-Galveston Region. Jackson: MS.
Ellingwood, B. R., and Lee, J. Y. (2016). Life Cycle Performance Goals for Civil Infrastructure: Intergenerational Risk-Informed Decisions. Struct. Infrastructure Eng. 12 (7), 822–829. doi:10.1080/15732479.2015.1064966
Emanuel, K. (2017). Assessing the Present and Future Probability of Hurricane Harvey's Rainfall. Proc. Natl. Acad. Sci. USA 114 (48), 12681–12684. doi:10.1073/pnas.1716222114
Federal Emergency Management Agency (FEMA) and U.S. Army Corps of Engineers (USACE) (2011). Flood Insurance Study: Coastal Counties. Texas.
FEMA (2009). Hurricane Ike in Texas and Louisiana: Mitigation Assessment Team Report, Building Performance Observations, Recommendations, and Technical Guidance. FEMA P-757. https://www.fema.gov/media-library-data/20130726-1648-20490-9826/fema757.pdf.
Fereshtehnejad, E., Gidaris, I., Rosenheim, N., Tomiczek, T., Padgett, J. E., Cox, D. T., et al. (2021). Probabilistic Risk Assessment of Coupled Natural-Physical-Social Systems: Cascading Impact of Hurricane-Induced Damages to Civil Infrastructure in Galveston, Texas. Nat. Hazards Rev. 22 (3), 04021013. doi:10.1061/(ASCE)NH.1527-6996.0000459
Field, C. B., Barros, V., Stocker, T. F., and Dahe, Q. (2012). Managing the Risks of Extreme Events and Disasters to advance Climate Change Adaptation. Cambridge University Press.
Gardoni, P., Der Kiureghian, A., and Mosalam, K. M. (2002). Probabilistic Capacity Models and Fragility Estimates for Reinforced concrete Columns Based on Experimental Observations. J. Eng. Mech. 128 (10), 1024–1038. doi:10.1061/(asce)0733-9399(2002)128:10(1024)
Gelman, A., and Rubin, D. B. (1992). Inference from Iterative Simulation Using Multiple Sequences. Statist. Sci. 7 (4), 457–472. doi:10.1214/ss/1177011136
Ghahramani, Z. (1998). Learning Dynamic Bayesian Networks. International School on Neural Networks, Initiated by IIASS and EMFCSC, 168–197. doi:10.1007/BFb0053999
Gonzalez Duenas, C., Bernier, C., and Padgett, J. (2019). Probabilistic Assessment of Bridges Subjected to Waterborne Debris. Coastal Structures 2019, 356–365. doi:10.18451/978-3-939230-64-9_036
Günay, S., and Mosalam, K. M. (2013). PEER Performance-Based Earthquake Engineering Methodology, Revisited. J. Earthquake Eng. 17 (6), 829–858. doi:10.1080/13632469.2013.787377
Hallowell, S. T., Myers, A. T., Arwade, S. R., Pang, W., Rawal, P., Hines, E. M., et al. (2018). Hurricane Risk Assessment of Offshore Wind Turbines. Renew. Energ. 125, 234–249. doi:10.1016/j.renene.2018.02.090
Hassanzadeh, P., Lee, C.-Y., Nabizadeh, E., Camargo, S. J., Ma, D., and Yeung, L. Y. (2020). Effects of Climate Change on the Movement of Future Landfalling Texas Tropical Cyclones. Nat. Commun. 11 (1), 1–9. doi:10.1038/s41467-020-17130-7
Hatzikyriakou, A., Lin, N., Gong, J., Xian, S., Hu, X., and Kennedy, A. (2016). Component-based Vulnerability Analysis for Residential Structures Subjected to Storm Surge Impact from Hurricane Sandy. Nat. Hazards Rev. 17 (1), 05015005. doi:10.1061/(ASCE)NH.1527-6996.0000205
Hu, X., Liu, B., Wu, Z. Y., and Gong, J. (2016). Analysis of Dominant Factors Associated with Hurricane Damages to Residential Structures Using the Rough Set Theory. Nat. Hazards Rev. 17 (3), 1–10. doi:10.1061/(ASCE)NH.1527-6996.0000218
Jia, G., and Taflanidis, A. A. (2013). Kriging Metamodeling for Approximation of High-Dimensional Wave and Surge Responses in Real-Time Storm/hurricane Risk Assessment. Comp. Methods Appl. Mech. Eng. 261-262, 24–38. doi:10.1016/j.cma.2013.03.012
Jonkman, S. N. (2007). Loss of Life Estimation in Flood Risk Assessment; Theory and Applications. doi:10.1142/9789812709554_0118
Ju, C., Ning, Y., and Pan, W. (2016). A Review of Interdependence of Sustainable Building. Environ. Impact Assess. Rev. 56, 120–127. doi:10.1016/j.eiar.2015.09.006
Kameshwar, S., Cox, D. T., Barbosa, A. R., Farokhnia, K., Park, H., Alam, M. S., et al. (2019). Probabilistic Decision-Support Framework for Community Resilience: Incorporating Multi-Hazards, Infrastructure Interdependencies, and Resilience Goals in a Bayesian Network. Reliability Eng. Syst. Saf. 191, 106568. doi:10.1016/j.ress.2019.106568
Kameshwar, S., and Padgett, J. E. (2018). Parameterized Fragility Assessment of Bridges Subjected to Pier Scour and Vehicular Loads. J. Bridge Eng. 23 (7), 1–11. doi:10.1061/(ASCE)BE.1943-5592.0001240
Khakzad, N., and Van Gelder, P. (2018). Vulnerability of Industrial Plants to Flood-Induced Natechs: A Bayesian Network Approach. Reliability Eng. Syst. Saf. 169, 403–411. doi:10.1016/j.ress.2017.09.016
Kopp, R. E., Horton, R. M., Little, C. M., Mitrovica, J. X., Oppenheimer, M., Rasmussen, D. J., et al. (2014). Probabilistic 21st and 22nd century Sea‐level Projections at a Global Network of Tide‐gauge Sites. Earth's Future 2 (8), 383–406. doi:10.1002/2014ef000239
Kousky, C. (2014). Informing Climate Adaptation: A Review of the Economic Costs of Natural Disasters. Energ. Econ. 46, 576–592. doi:10.1016/j.eneco.2013.09.029
Landefeld, J. S., and Seskin, E. P. (1982). The Economic Value of Life: Linking Theory to Practice. Am. J. Public Health 72 (6), 555–566. doi:10.2105/AJPH.72.6.555
Lee, J. Y., and Ellingwood, B. R. (2017). A Decision Model for Intergenerational Life-Cycle Risk Assessment of Civil Infrastructure Exposed to Hurricanes under Climate Change. Reliability Eng. Syst. Saf. 159 (10), 100–107. doi:10.1016/j.ress.2016.10.022
Lerner, U., Segal, E., and Koller, D. (2013). Exact Inference in Networks with Discrete Children of Continuous Parents. ArXiv Preprint ArXiv:1301.2289.
Li, Q., Wang, C., and Ellingwood, B. R. (2015). Time-dependent Reliability of Aging Structures in the Presence of Non-stationary Loads and Degradation. Struct. Saf. 52, 132–141. doi:10.1016/j.strusafe.2014.10.003
Li, Y., and Ellingwood, B. R. (2009). Risk-based Decision-Making for Multi-hazard Mitigation for wood-frame Residential Construction. Aust. J. Struct. Eng. 9 (1), 17–26. doi:10.1080/13287982.2009.11465006
Li, Y., Van De Lindt, J. W., Dao, T., Bjarnadottir, S., and Ahuja, A. (2012). Loss Analysis for Combined Wind and Surge in Hurricanes. Nat. Hazards Rev. 13 (1), 1–10. doi:10.1061/(ASCE)NH.1527-6996.0000058
Liu, Y., and Irish, J. L. (2019). Characterization and Prediction of Tropical Cyclone Forerunner Surge. Coastal Eng. 147, 34–42. doi:10.1016/j.coastaleng.2019.01.005
Lounis, Z., and McAllister, T. P. (2016). Risk-Based Decision Making for Sustainable and Resilient Infrastructure Systems. J. Struct. Eng. 142 (9), 1–14. doi:10.1061/(asce)st.1943-541x.0001545
Luechinger, S., and Raschky, P. A. (2009). Valuing Flood Disasters Using the Life Satisfaction Approach. J. Public Econ. 93 (3–4), 620–633. doi:10.1016/j.jpubeco.2008.10.003
Massarra, C. C. (2012). Hurricane Damage Assessment Process for Residential Buildings. Louisiana State University. http://etd.lsu.edu/docs/available/etd-07092012-145208/.
Meinshausen, M., Smith, S. J., Calvin, K., Daniel, J. S., Kainuma, M. L. T., Lamarque, J.-F., et al. (2011). The RCP Greenhouse Gas Concentrations and Their Extensions from 1765 to 2300. Climatic Change 109 (1–2), 213–241. doi:10.1007/s10584-011-0156-z
Melby, J. A., Nadal-Caraballo, N., Ratcliff, J. J., Massey, T. C., and Jensen, R. E. (2017). Sabine Pass to Galveston Bay Wave and Water Level Modeling. Vicksburg, Mississippi: US Army Engineer Research and Development Center, 287.
Moehle, J., and Deierlein, G. G. (2004). “A Framework Methodology for Performance-Based Earthquake Engineering,” in 13th World Conference on Earthquake Engineering, 679.
Moon, I.-J., Kim, S.-H., and Chan, J. C. L. (2019). Climate Change and Tropical Cyclone Trend. Nature 570 (7759), E3–E5. doi:10.1038/s41586-019-1222-3
Müller, D. B., Liu, G., Løvik, A. N., Modaresi, R., Pauliuk, S., Steinhoff, F. S., et al. (2013). Carbon Emissions of Infrastructure Development. Environ. Sci. Technol. 47 (20), 11739–11746. doi:10.1021/es402618m
Murphy, K. P., and Russell, S. (2002). Dynamic Bayesian Networks: Representation, Inference and Learning.
Nakajima, S., and Murakami, T. (2010). “Comparison of Two Structural Reuse Options of Two-By-Four Salvaged Lumbers,” in Proceedings of the WCTE 2010-World Conference on Timber Engineering (Italy: Riva Del Garda), 20–24.
Nofal, O. M., van de Lindt, J. W., and Do, T. Q. (2020). Multi-variate and Single-Variable Flood Fragility and Loss Approaches for Buildings. Reliability Engineering and System Safety, 106971. doi:10.1016/j.ress.2020.106971
Padgett, J., Desroches, R., Nielson, B., Yashinsky, M., Kwon, O.-S., Burdette, N., et al. (2008). Bridge Damage and Repair Costs from Hurricane Katrina. J. Bridge Eng. 13 (1), 6–14. doi:10.1061/(asce)1084-0702(2008)13:1(6)
Padgett, J. E., and Tapia, C. (2013). Sustainability of Natural Hazard Risk Mitigation: Life Cycle Analysis of Environmental Indicators for Bridge Infrastructure. J. Infrastruct. Syst. 19 (4), 395–408. doi:10.1061/(asce)is.1943-555x.0000138
Park, H., Cox, D. T., and Barbosa, A. R. (2017). Comparison of Inundation Depth and Momentum Flux Based Fragilities for Probabilistic Tsunami Damage Assessment and Uncertainty Analysis. Coastal Eng. 122, 10–26. doi:10.1016/j.coastaleng.2017.01.008
Pearl, J. (2014). Probabilistic Reasoning in Intelligent Systems: Networks of Plausible Inference. Elsevier.
Pescaroli, G., and Alexander, D. (2015). A Definition of Cascading Disasters and Cascading Effects: Going beyond the “Toppling Dominos” Metaphor. GRF Davos Planet@Risk 3 (1), 58–67.
Pistrika, A. K., and Jonkman, S. N. (2010). Damage to Residential Buildings Due to Flooding of New Orleans after hurricane Katrina. Nat. Hazards 54 (2), 413–434. doi:10.1007/s11069-009-9476-y
Poddar, S., Mondal, M., and Ghosh, S. (2020). A Survey on Disaster: Understanding the After-Effects of Super-cyclone Amphan and Helping Hand of Social Media. ArXiv Preprint ArXiv:2007.14910.
Porter, K. A. (2003). “An Overview of PEER’s Performance-Based Earthquake Engineering Methodology,” in Proceedings of Ninth International Conference on Applications of Statistics and Probability in Civil Engineering, 1–8.
Reible, D. D., Haas, C. N., Pardue, J. H., and Walsh, W. J. (2006). Toxic and Contaminant Concerns Generated by Hurricane Katrina. J. Environ. Eng. 132 (6), 565–566. doi:10.1061/(ASCE)0733-9372(2006)132
Risser, M. D., and Wehner, M. F. (2017). Attributable Human‐Induced Changes in the Likelihood and Magnitude of the Observed Extreme Precipitation during Hurricane Harvey. Geophys. Res. Lett. 44 (24), 457–464. doi:10.1002/2017GL075888
Rose, A., Porter, K., Dash, N., Bouabid, J., Huyck, C., Whitehead, J., et al. (2007). Benefit-cost Analysis of FEMA hazard Mitigation grants. Nat. Hazards Rev. 8 (4), 97–111. doi:10.1061/(asce)1527-6988(2007)8:4(97)
Saeidpour, A., Chorzepa, M. G., Christian, J., and Durham, S. (2018). Parameterized Fragility Assessment of Bridges Subjected to hurricane Events Using Metamodels and Multiple Environmental Parameters. J. Infrastructure Syst. 24 (4), 1–14. doi:10.1061/(ASCE)IS.1943-555X.0000442
Sanchez-Silva, M., Klutke, G.-A., and Rosowsky, D. V. (2011). Life-cycle Performance of Structures Subject to Multiple Deterioration Mechanisms. Struct. Saf. 33 (3), 206–217. doi:10.1016/j.strusafe.2011.03.003
Schultz, M. T., and Smith, E. R. (2016). Assessing the Resilience of Coastal Systems: A Probabilistic Approach. J. Coastal Res. 321 (5), 1032–1050. doi:10.2112/jcoastres-d-15-00170.1
Sharma, H., Gardoni, P., and Hurlebaus, S. (2015). Performance-Based Probabilistic Capacity Models and Fragility Estimates for RC Columns Subject to Vehicle Collision. Computer-Aided Civil Infrastructure Eng. 30 (7), 555–569. doi:10.1111/mice.12135
Shenoy, P. P. (2012). Inference in Hybrid Bayesian Networks Using Mixtures of Gaussians. ArXiv Preprint ArXiv:1206.6877.
Sills, G. L., Vroman, N. D., Wahl, R. E., and Schwanz, N. T. (2008). Overview of New Orleans Levee Failures: Lessons Learned and Their Impact on National Levee Design and Assessment. J. Geotech. Geoenviron. Eng. 134 (5), 556–565. doi:10.1061/(asce)1090-0241(2008)134:5(556)
Spiegelhalter, D., Thomas, A., Best, N., and Lunn, D. (2007). OpenBUGS User Manual, version 3.0.2. Cambridge: MRC Biostatistics Unit.
Stewart, M. G., and Deng, X. (2015). Climate Impact Risks and Climate Adaptation Engineering for Built Infrastructure. Asce-asme J. Risk Uncertainty Eng. Syst. Part. A: Civ. Eng. 1 (1), 04014001–04014012. doi:10.1061/AJRUA6.0000809
Stewart, M. G., Wang, X., and Nguyen, M. N. (2011). Climate Change Impact and Risks of concrete Infrastructure Deterioration. Eng. Structures 33 (4), 1326–1337. doi:10.1016/j.engstruct.2011.01.010
Straub, D., and Der Kiureghian, A. (2010). Bayesian Network Enhanced with Structural Reliability Methods: Application. J. Eng. Mech. 136 (10), 1259–1270. doi:10.1061/(ASCE)EM.1943-7889.000017310.1061/(asce)em.1943-7889.0000170
Straub, D. (2009). Stochastic Modeling of Deterioration Processes through Dynamic Bayesian Networks. J. Eng. Mech. 135 (10), 1089–1099. doi:10.1061/(ASCE)EM.1943-7889.0000024
Toimil, A., Losada, I. J., Camus, P., and Díaz-Simal, P. (2017). Managing Coastal Erosion under Climate Change at the Regional Scale. Coastal Eng. 128, 106–122. doi:10.1016/j.coastaleng.2017.08.004
Tomiczek, T., Kennedy, A., and Rogers, S. (2014). Collapse Limit State Fragilities of wood-framed Residences from Storm Surge and Waves during Hurricane Ike. J. Waterway, Port, Coastal, Ocean Eng. 140 (1), 43–55. doi:10.1061/(ASCE)WW.1943-5460.0000212
Tomiczek, T., Kennedy, A., Zhang, Y., Owensby, M., Hope, M. E., Lin, N., et al. (2017). Hurricane Damage Classification Methodology and Fragility Functions Derived from Hurricane Sandy’s Effects in Coastal New Jersey. J. Waterway, Port, Coastal Ocean Eng. 143 (5), 04017027. doi:10.1061/(ASCE)WW.1943-5460.0000409
Tomiczek, T., Prasetyo, A., Mori, N., Yasuda, T., and Kennedy, A. (2016). Physical Modelling of Tsunami Onshore Propagation, Peak Pressures, and Shielding Effects in an Urban Building Array. Coastal Eng. 117, 97–112. doi:10.1016/j.coastaleng.2016.07.003
Trenberth, K. E., Cheng, L., Jacobs, P., Zhang, Y., and Fasullo, J. (2018). Hurricane Harvey Links to Ocean Heat Content and Climate Change Adaptation. Earth's Future 6 (5), 730–744. doi:10.1029/2018EF000825
Van De Lindt, J. W., and Dao, T. N. (2009). Performance-Based Wind Engineering for Wood-Frame Buildings. J. Struct. Eng. 135 (2), 169–177. doi:10.1061/(asce)0733-9445(2009)135:2(169)
Van De Lindt, J. W., and Taggart, M. (2009). Fragility Analysis Methodology for Performance-Based Analysis of wood-frame Buildings for Flood. Nat. Hazards Rev. 10 (3), 113–123. doi:10.1061/(asce)1527-6988(2009)10:3(113)
Wang, S.-Y. S., Zhao, L., Yoon, J.-H., Klotzbach, P., and Gillies, R. R. (2018). Quantitative Attribution of Climate Effects on Hurricane Harvey's Extreme Rainfall in Texas. Environ. Res. Lett. 13 (5), 054014. doi:10.1088/1748-9326/aabb85
Wang, S., and Toumi, R. (2021). Recent Migration of Tropical Cyclones toward Coasts. Science 371 (6528), 514–517. doi:10.1126/science.abb9038
Wangel, J., Wallhagen, M., Malmqvist, T., and Finnveden, G. (2016). Certification Systems for Sustainable Neighbourhoods: What Do They Really Certify? Environ. Impact Assess. Rev. 56, 200–213. doi:10.1016/j.eiar.2015.10.003
Whitehead, J. C. (2003). One Million Dollars Per Mile? the Opportunity Costs of hurricane Evacuation. Ocean Coastal Manag. 46 (11–12), 1069–1083. doi:10.1016/j.ocecoaman.2003.11.001
Winter, A. O. (2019). Effects of Flow Shielding and Channeling on Tsunami-Induced Loading of Coastal Structures. University of Washington.
Work, P. A., Rogers, S. M., and Osborne, R. (1999). Flood Retrofit of Coastal Residential Structures: Outer banks, North Carolina. J. Water Resour. Plann. Manag. 125 (2), 88–93. doi:10.1061/(asce)0733-9496(1999)125:2(88)
WTVD-TV (2020). 14-year-old Louisiana Girl Among 6 Killed by Falling Trees during Hurricane Laura. Louisiana Gov. https://abc11.com/hurricane-laura-update-categories-damage/6391611/#:∼:text=At least six people in.
Xiong, Y., Liang, Q., Park, H., Cox, D., and Wang, G. (2019). A Deterministic Approach for Assessing Tsunami-Induced Building Damage through Quantification of Hydrodynamic Forces. Coastal Eng. 144, 1–14. doi:10.1016/j.coastaleng.2018.11.002
Yu, X., and Tang, Y. (2017). A Critical Review on the Economics of Disasters. Jracr 7 (1), 27. doi:10.2991/jrarc.2017.7.1.4
Keywords: performance-based engineering, coastal engineering, time-varying factors, cascading effects, dynamic Bayesian network, climate change, elevated coastal residential structure
Citation: González-Dueñas C and Padgett JE (2021) Performance-Based Coastal Engineering Framework. Front. Built Environ. 7:690715. doi: 10.3389/fbuil.2021.690715
Received: 03 April 2021; Accepted: 14 June 2021;
Published: 25 June 2021.
Edited by:
Rodolfo Silva, National Autonomous University of Mexico, MexicoReviewed by:
Mireille Escudero, National Autonomous University of Mexico, MexicoManuel Gerardo Verduzco-Zapata, University of Colima, Mexico
Copyright © 2021 González-Dueñas and Padgett. This is an open-access article distributed under the terms of the Creative Commons Attribution License (CC BY). The use, distribution or reproduction in other forums is permitted, provided the original author(s) and the copyright owner(s) are credited and that the original publication in this journal is cited, in accordance with accepted academic practice. No use, distribution or reproduction is permitted which does not comply with these terms.
*Correspondence: Jamie E. Padgett, jamie.padgett@rice.edu