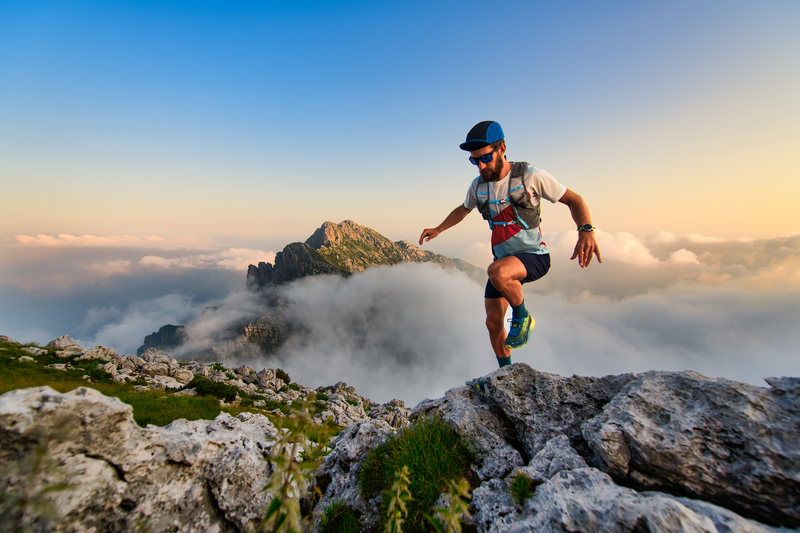
94% of researchers rate our articles as excellent or good
Learn more about the work of our research integrity team to safeguard the quality of each article we publish.
Find out more
ORIGINAL RESEARCH article
Front. Built Environ. , 12 April 2021
Sec. Transportation and Transit Systems
Volume 7 - 2021 | https://doi.org/10.3389/fbuil.2021.642295
This article is part of the Research Topic COVID-19 and the Role of Human Mobility and Transportation Systems View all 6 articles
This paper analyzes the impacts of COVID-19 pandemic on the United States air transportation network between March and August 2020. Despite dramatic reductions in flight and passenger volumes, the network remained robust and resilient against perturbation. Although 24% of airports closed, the reduction in network efficiency was only 5.1%, which means airlines continued to serve most destinations. A deeper analysis of airport closures reveals that 1) small peripheral airports were the most likely to be closed; 2) socio-economic and epidemiological factors characterizing the airport’s region such as income, income inequality, political leaning, and the number of observed COVID cases were not predictive of airport closure. Finally, we show that high network robustness has a downside: although emissions from United States air traffic in 2020 fell by 37.4% compared to 2019, mostly due to the drop in the number of flights, emissions per passenger doubled in the period April to August 2020 and increased eightfold in the week of April 5–11. This rise indicates inefficient use of resources by airlines.
The combined effects of social distancing, quarantining, travel restrictions, and apprehension to fly have led to a sharp decline in revenue, flight volume, and passenger-miles traveled in the United States air transportation network in 2020. The Air Transport Bureau. (2020) has predicted a worldwide decrease of approximately 2.89 billion passengers in the aviation system throughout 2020 compared to 2019. ACI. (2020) reported that by the end of the first quarter of 2020, the global year-over-year reduction in the supply and demand for commercial air travel was among the largest declines on record.
Similar trends can be observed in the United States. Figure 1A shows the precipitous drop in the number of domestic United States flights offered weekly beginning in March. The week ending Saturday, April 25 experienced the lowest number of domestic flights since the beginning of the pandemic. Additionally, approximately 400 United States airports that offer commercial flights have experienced at least short-term closure during the crisis up until the study’s termination. Most of these are small airports that typically operate fewer than 100 flights per week (Figure 1B).
FIGURE 1. General Network Observations. (A) Seven-day moving averages of number of domestic flights by day in 2019 vs. 2020 at 1,200 airports in the ASPM database. (B) Cumulative distribution of average number of domestic flights offered per week in 2019 by all United States airports in ASPM database vs. those United States airports that closed between May and August 2020. In total approximately 400 airports experienced closure for at least one week during the study period. Most of these airports are small airfields operating predominantly general aviation flights, the largest of which offers on average 60 ASPM-reported flights during a typical week. Those airports that closed during the study period were inactive for 11 weeks on average, though it should be noted that some of these airports remained closed at the study’s termination.
Understanding the interactions between commercial air travel and the spread of COVID-19 is crucial to both control the transmission of the virus and to ensure the continued survival of the aviation industry. The former has been well studied in recent months. Chinazzi et al. (2020) employed a disease transmission model to predict COVID-19 spread and gauge the expected success of travel restrictions. Zhang et al. (2020) leveraged a network dynamics model to conclude that, to effectively limit transmission, travel restrictions must extend beyond hotspot areas. Finally, Kraemer et al. (2020) showed that voluntary quarantining and meticulous hygiene are better able to limit spread than travel restrictions alone.
Our work seeks to model the effects of the pandemic on the network characteristics of the United States aviation system as they have evolved during the COVID-19 pandemic through July 2020. More specifically, it 1) models the system’s efficiency and connectivity over time; 2) quantifies changes in its robustness and, using percolation theory, measures its resilience to airport closures and flight reductions; and 3) investigates the factors that led to the closure of airports in the network.
Despite the sharp decline in passengers and flight volume, our results indicate that the United States aviation network’s loss in efficiency was only 5.1%. Additionally, the size of the giant connected component (GCC), a measure of network connectivity, dropped 24.3% during these months. Regardless of week, a giant cluster persisted with few weakly connected components consisting of small, regional airports. The results imply that the United States air network is extremely robust and continues to maintain high connectivity despite significant reductions in passenger and flight volumes. In other words, demand has dropped, but airlines have continued to serve most destinations. Those airports that did close were typically small airfields on the periphery of the (mathematical) network. Additionally, our results indicate that the Coronavirus Aid, Relief, and Economic Security (CARES) Act distributed to airports through FAA grants is the second-best predictor of airport closure; however, the importance of FAA Grants in the model is dwarfed by the best predictor of closure–Number of Flights. Finally, we suspected that the robustness of the network creates negative externalities. Despite the reduction in annual emissions of 58.6 million metric tons of CO2 in 2020, which is more than a third of CO2 emissions produced by domestic air traffic in 2019, emissions per passenger increased up to eight times during the pandemic. The detailed results, methodology, and discussion of these effects are provided in the following sections.
Bonaccorsi et al. (2020), who analyzed passenger mobility in Italy, the first European nation to impose mobility restrictions during the pandemic, provided the motivation for our study. They used real-time mobility data to calculate the changing efficiency of the Italian mobility network and the connectivity of the country’s regions. They correlated these changes to each region’s socioeconomic attributes to determine which characteristics are common among those provinces that have experienced the sharpest declines in mobility. Similarly to Bonaccorsi et al. (2020), we start by analyzing network connectivity. Analysis of the network informed our next steps and signaled the need to both investigate the reasons behind airport closures and how the network’s evolution has impacted flight emissions. Both these analyses provide insights into ways the aviation system might effectively weather future disruption events.
Network efficiency is defined as the average of node efficiencies among the network’s nodes (Latora and Marchiori, 2001). It can be used to assess the cost-efficiency of a particular network or to investigate if the network is tolerant to the failures of nodes or links. The network efficiency E of the network G is calculated as:
where
The robustness of a network can be evaluated by calculating the size of its connected giant component when a random failure affects a fraction of its nodes (Buldyrev et al., 2010). A giant component is a segment of a network that contains a large majority of the network’s nodes. In a network with a giant component, almost every point is reachable from almost every other point in the network. By removing a node from the network, the size of the giant component is not reduced if the node is not situated in the giant component. If the removed node is located on the periphery of the giant component, the size of giant component drops by one. Most interestingly, there are cases where the removal of a node reduces the size of the giant component by more than one. In those cases, the removed node had a high centrality and served as a connection between a cluster of nodes and the rest of the network. In transportation, such nodes are called hubs.
The idea of removing nodes to test a network’s characteristics is the foundation of percolation theory (Albert et al., 2000). The theory uses the notion of progressive structural deterioration (Cohen et al., 2000), which tests the network’s robustness to failure or attack. A failure, as defined in percolation theory, is a removal of a randomly selected node, while an attack is the removal of a highly connected node. Hub-and-spoke transportation networks are particularly vulnerable to attacks.
To test these characteristics, we collected individual flight records for approximately 1,200 United States airports from the FAA Aviation System Performance Metrics (ASPM) database (Federal Aviation Administration, 2020a). These counts were aggregated to find the total number of weekly flights between all United States airport pairs from March 1 to August 7, 2020. In total, data for 22 weeks were used to create 22 networks.
To better understand patterns among those airports that closed during the pandemic, a random forest model was implemented to predict closure based on nine independent variables categorized in three groups. The first group of variables includes network characteristics such as airport size (measured in the number of weekly flights), airport degree, and length of the shortest path to the closest hub. These metrics are calculated based on traffic data from ASPM. The second group of variables includes socio-economic measures associated with the county where an airport is located. These include household income, income inequality, political leaning, the number of COVID-19 cases per capita, and whether the airport received a Federal Aviation Administration grant to bolster operations (Federal Aviation Administration, 2020b). We selected income and income inequality as socioeconomic factors in reference to Bonaccorsi et al. (2020), who correlated changes in regional network efficiency with economic variables. We used data collected in the American Community Survey (ACS) (U.S. Census Bureau, 2019) to estimate county-level household income as well as income inequality. We included political leanings in our model because, in a survey of more than 5,000 individuals, de Bruin et al. (2020) found that self-identified Democrats were more likely to cancel planned air travel in response to concerns of COVID-19. This behavior suggests that personal politics could indicate changes in air travel patterns. Political leaning for each county was estimated based on the average percent differentials of votes won in the last four presidential elections (MIT Election Data and Science Lab, 2018). The differences were transformed into categorical values:
We decided to code the variable instead of using continuous numbers because a small change in the election percentage does not add information to the model. The data on COVID-19 infections was downloaded from The Center for Systems Science and Engineering (CSSE) at Johns Hopkins University (Dong et al., 2020). The descriptions of variables and the sources of the data can be found in Table 1 and Supplementary Material.
The presented variables were used to train a random forest model, a method of machine learning which constructs multiple independent decision trees. In each iteration a subset of samples is selected to create a tree. The samples that are not selected for that particular tree are called Out-Of-Bag (OOB). Since OOB samples are independent of those used to build the tree, error rate calculated based on the OOB sample is sufficient to train the model without the need to use a cross-validation or testing sample (Breiman, 2001). An advantage of using OOB error for training the model is that the complete original data set is used both for constructing the random forest classifier and for error estimation, which results in better performance of the classifier (Janitza and Hornung, 2018). Random forest is used for many applications because it is computationally efficient, operates quickly over large data sets, and is versatile enough to deal with both classification and regression (Oshiro et al., 2012). In addition, the model provides a ranking of variables that reflects their relative importance in predicting the target variable. Variable importance is the reason we use random forest–to identify the most relevant factors of airport closure.
Random forest is defined by a set of hyper-parameters, which define the structure of the trees included in the model (see Biau and Scornet. (2016) for a theoretical background). The values of these hyper-parameters influence the performance and accuracy of the model. The most frequently used method for choosing the parameters is minimization of the OOB error (Goldstein et al., 2010). We consider the following hyper-parameters: the number of trees in the model, the number of features, the maximum depth of the tree, and the size of the node. To maximize the accuracy of the model, we created a grid, looped through each hyper-parameter combination, and evaluated the model. The results are presented in the next section.
The Intergovernmental Panel on Climate Change (IPCC) provides guidelines for the calculation of greenhouse gas inventories, which include airline emissions (IPCC, 2006). The guide defines three methods of varying complexity for calculating the emissions in two stages of flight: the landing and take-off (LTO) cycle and cruise. The LTO cycle includes all activities below the altitude of 3,000 feet: taxi-in and out, take-off, climb-out, approach, and landing. Cruise is the portion of flight at altitudes above 3,000 feet. The first method of calculation, Tier 1, estimates emissions by multiplying an emission factor (the amount of pollutants created by burning a unit of fuel) with the total quantity of fuel sold for aviation purposes. Tier two calculations are performed when there is data on the number of LTO cycles per type of aircraft, but there is no information available on the distances flown in the cruise stage. The Tier three methodology, used in this paper, is based on the actual flight trajectory data. It estimates LTO emissions based on the number of flights and type of aircraft, while cruise emissions are estimated based on flying distances. The accuracy of the method depends on the research question and the level of detail of available data. The most complex methods calculate fuel consumption based on the aircraft engine performance, engine thrust, weather, and time spent in different flying modes. The goal of this paper is to calculate the change in annual emissions of CO2 and trends in emissions per passenger in the period of March to August 2020, which does not require such a level of detail. We use a modified Tier three calculation for LTO and cruise emissions; instead of using engine performance characteristics for each individual flight, we group all flights in five categories based on flying distance, assign a “reference aircraft” to each category, and assume that all aircraft have engine characteristics of one of these five reference aircraft. The aircraft model most frequently used in a flight category is designated as a reference aircraft. The emissions are calculated using the following equations:
where
TABLE 2. Flight categories, reference aircraft and their performance. Flights are divided into five categories based on flying distance. An aircraft most frequently used in each flight category is called a reference aircraft. All flights within the same flight category are assumed to be operated by the reference aircraft.
It is assumed that all flights in the same flight class are conducted by the reference aircraft, and that the fuel consumption and emission production of the reference aircraft accurately represent all aircraft within the group. Categorization into five distance-based groups–commuter, regional, short-haul, medium-haul, and long-haul flights–is regularly used by airlines. However, different airlines use different ranges to define these categories. The ranges presented in Table 2 are an attempt to reconcile these differences.
Finally, the total amount of LTO CO2 is calculated according to Eq. 3 by multiplying the number of operations within the flight group and the estimated CO2 emission per LTO of the reference aircraft. Cruise emissions (Eq. 4) are calculated by finding the total fuel consumed in all flights and multiplying it by the average emission index
The ability of a network to maintain its structure when experiencing local failures is a function of the degree of connectivity of its nodes. It is well known that the global air transportation network exhibits the characteristics of a homogeneous, scale-free network, whereby a few highly connected nodes are statistically significant and ensure the network maintains its form (Verma et al., 2014).
The domestic United States network’s global efficiency and connectivity are depicted in Figure 2. Global efficiency, which considers the distance between nodes and the numbers and weights of edges among them, quantifies how readily information or individuals can travel through the network. It takes on a normalized value in [0,1]. Although the United States aviation network’s efficiency drops from March through April, the loss in efficiency is only 5.1%.
FIGURE 2. The Continued Robustness of the Domestic Aviation Network. (A) The evolution of network connectivity illustrated by the size of the giant connected component by week. The trend is declining until April 18 and increasing through July. (B) Global network efficiency as calculated by Latora and Marchiori. (2001). The trend is decreasing until May 16 and increasing through July.
To further validate the network’s strong connectivity, the evolution of the size and number of clusters/communities over time was calculated. Regardless of week, a giant cluster persists with few weakly connected components consisting of small, regional airports.
Albert et al. (2000) established that scale-free networks are surprisingly resilient against random error, but vulnerable to targeted attack. To quantify the aviation network’s ability to withstand failure, (i.e. airport closure and flight volume reduction), node percolation was performed.
Figure 3 demonstrates the network’s response to node removal via three procedures: (a), (d) removing nodes randomly, (b), (e) removing nodes in order of decreasing unweighted node degree, and (c), (f) removing and adding node airports in the sequence in which they closed and reopened during the pandemic. 1) and 2) show the size of the largest community
FIGURE 3. Network Response to Failure and Attack. (A), (B), (D), (E) illustrate the size of the largest component and characteristic cluster size as percolation is conducted on the network of flights on the week ending April 25, the week with the lowest observed number of flights. (A) and (D) correspond to 10,000 trials of the random removal of nodes, i.e., random error. (B) and (E) correspond to the removal of nodes by decreasing unweighted degree. (A),(B),(D),(E) are plotted against parameter f, the fraction of nodes that have been removed. (C) and (F) correspond to the case in which nodes are removed and added as observed during the pandemic. (A)–(C) show the relative size of the largest component
The size of the giant component was reduced by 24.3% in the worst week of April. Since all airports are a node in the giant component, the reduction of GC of 24.3% corresponds to the removal of the same fraction of the total nodes in the network. In other words, the airports that closed minimally impacted the connectivity of other nodes in the network. When 24.3% of nodes are randomly removed from the same network, the size of the giant component drops by 32.5% (Figure 3A). The size of the observed giant component is 8.5 percentage points larger that expected in the case of random removal. This result indicates that closed airports were less connected than the average node in the network and that their closures were not random.
To investigate the factors engendering these closures, we trained a random forest model. The calculation was performed with the package random Forest in the R software. Nine variables, presented in Figure 4A, were used to create a model that predicts whether an airport closes. While a random forest algorithm does not require testing against overfitting since the OOB sample is independent, optimization of hyperparameters requires a validation set to get an unbiased estimate. The sample containing 1,192 airports was split into a training set and validation set (80/20 split). Due to the imbalance of classes in the data, the training set was undersampled to achieve 1-to−1 balance. The overall performance of our prediction method was evaluated by OOB error defined as the fraction of the number of incorrect classifications over the number of out-of-bag samples. The OOB error for the optimal combination of hyper-parameters is 12.3% (number of trees, ntree = 500; number of variables at each split, mtry = 3, minimum size of terminal node, node size = 1). The training accuracy of the model, defined as the percent of accurately classified instances, is 87.7%. The prediction accuracy of the developed random forest on the validation set is 82.4%. The accuracy exceeds the accuracy of a model that predicts according to random chance, quantified by proportional chance criterion proposed by Morrison. (1969) of 57.4%.
FIGURE 4. Random Forest results. (A) Correlation matrix with significant correlations
The most frequently used measures of importance in random forests–Mean decrease in Accuracy and Gini Index are prone to overestimation bias when the variables are highly correlated (Strobl et al., 2008). This is the case with some of the variables in our model, as shown in Figure 4A. As a result, instead of traditional measures of importance, we use conditional variable importance (Strobl et al., 2008), which is more reliable in reflecting the impact of each predictor variable in the case of high correlations. Figure 4B presents the importance plot. The results show that airport size is by far the most important predictor of closure. FAA grants are the second best predictor in the model, indicating that financial aid distributed to airports in some cases made a difference between their closing and remaining open. The importance measures of other variables, including network-related, socio-economic, and pandemic measures are inconsequential compared to Number of flights. The lack of connection between socio-economic characteristics of a county and the operations of its airports indicates that airlines and the FAA are the main decision-makers, rather than the local governing bodies.
Finally, the aircraft emissions analysis presented in Figure 5A indicates that total CO2 emissions produced by the United States domestic air traffic dropped in 2020 compared to 2019 by 37.4%, from 156.9 – 98.3 million metric tons of CO2. However, per-passenger emissions spiked eightfold in the week of April 5–11 and stabilized in the late summer at double the rate of the pre-COVID-19 level. These inefficiencies can be explained by several factors. First, airlines establish long-term lease agreements with airports, specifying the ways terminal facilities and airfields are used and funded. Under such long-term agreements airlines are obliged to operate leased facilities at a certain percent of the time. Due to the pandemic, certain amendments to the existing airport-airline agreements had to be made, which required time. Second, airlines submit their official schedules in advance, which prevented them from rapidly changing their operations due to low demand. Finally, some airlines were hesitant to cancel flights and adjust the schedules as they feared that they would lose landing slots. According to the FAA’s slot usage requirement, airlines are required to use allocated slots at least 80% of the time at slot-controlled airports such as John F. Kennedy International Airport (JFK), LaGuardia Airport (LGA), and Ronald Reagan Washington National Airport (DCA). Although the FAA eventually waived minimum slot-use requirements at slot-controlled airports, it took some time for these adjustments to be implemented. In the end, the highly structured and regulated nature of the network proved to be both a virtue and a drawback in the pandemic. The robustness of the network enabled high connectivity even in times of low traffic, but also created a lack of flexibility hindering adjustment to new conditions and more efficient allocation of resources.
FIGURE 5. United States air traffic CO2 emissions produced during the pandemic. Domestic air traffic (A), and Emissions per passenger (B). Although total CO2 emissions in 2020 dropped by 37.4% (58.6 Mton) compared to 2019, emissions per passenger have dramatically increased. The air traffic network remains robust because airlines continue flying aircraft with lower load factors. The cost of robustness is an increase in per-passenger emissions.
This paper analyzes the network characteristics of the United States domestic air transportation system as they have evolved during the COVID-19 pandemic. Despite the precipitous fall in passengers and flight volumes, our results indicate that the United States aviation network’s loss in efficiency was only 5.1% and that a giant cluster persisted with few weakly connected components consisting of small, regional airports, which means that the United States air network is extremely robust and continues to operate effectively. The percolation analysis shows that the random removal of nodes from the network would shrink the size of the giant component by 8% more than was actually observed. This result indicates that closed airports were less connected than the average node in the network and that their closures were not random. Indeed, we find that closed airports were small airports on the periphery of the (mathematical) network. Finally, as suspected, the robustness of the network creates negative externalities. We find that emissions per passenger in the domestic United States air transportation network increased eightfold during the pandemic.
There are two main avenues of research we would like to explore in the future. The first includes the analysis of the resilience of air networks during the pandemic in different geographies. The United States government provided financial support to ensure the continued operation of some airports and airlines. These funds prevented major disruptions in United States air traffic and led us to conclude that the United States air network was robust. Are other air traffic networks equally robust, even without financial support? The analysis of airline markets outside the United States might provide insight. The second research theme includes airline emissions. The airlines in the United States increased per-passenger emissions by using their resources inefficiently. Did the government miss an opportunity to demand reductions in emissions in exchange for financial support? Several European countries imposed such conditions. The analysis of those policies and their effects could provide creative solutions for curbing ever-growing airline emissions.
The original contributions presented in the study are included in the article/Supplementary Material, further inquiries can be directed to the corresponding author.
AB and SP designed the models, AB, SP, and XJ collected and analyzed the data. AB and SP wrote the manuscript with input from all authors. JR and MG conceived the study and oversaw overall direction and planning. All authors provided critical feedback and helped shape the research, analysis, and manuscript.
The authors declare that the research was conducted in the absence of any commercial or financial relationships that could be construed as a potential conflict of interest.
The Supplementary Material for this article can be found online at: https://www.frontiersin.org/articles/10.3389/fbuil.2021.642295/full#supplementary-material.
ACI (2020). The impact of COVID-19 on the airport business: two-fifths of passenger traffic and almost half of revenues wiped out in 2020, Montreal, QC: Tech. Rep.
Air Transport Bureau (2020). Effects of novel coronavirus (COVID-19) on civil aviation: economic impact analysis, Montréal, QC, Canada: International civil aviation organization (ICAO).
Albert, R., Jeong, H., and Barabási, A.-L. (2000). Error and attack tolerance of complex networks. Nature 406, 378–382. doi:10.1038/35019019
Biau, G., and Scornet, E. (2016). A random forest guided tour. Test 25, 197–227. doi:10.1007/s11749-016-0481-7
Bonaccorsi, G., Pierri, F., Cinelli, M., Flori, A., Galeazzi, A., Porcelli, F., et al. (2020). Economic and social consequences of human mobility restrictions under covid-19. Proc. Natl. Acad. Sci. USA 117, 15530–15535. doi:10.1073/pnas.2007658117
Buldyrev, S. V., Parshani, R., Paul, G., Stanley, H. E., and Havlin, S. (2010). Catastrophic cascade of failures in interdependent networks. Nature 464, 1025–1028. doi:10.1038/nature08932
Chinazzi, M., Davis, J. T., Ajelli, M., Gioannini, C., Litvinova, M., Merler, S., et al. (2020). The effect of travel restrictions on the spread of the 2019 novel coronavirus (COVID-19) outbreak. Science 368, 395–400. doi:10.1126/science.aba9757
Cohen, R., Erez, K., Ben-Avraham, D., and Havlin, S. (2000). Resilience of the internet to random breakdowns. Phys. Rev. Lett. 85, 4626. doi:10.1103/PhysRevLett.85.4626
de Bruin, W. B., Saw, H.-W., and Goldman, D. P. (2020). Political polarization in us residents’ covid-19 risk perceptions, policy preferences, and protective behaviors. J. Risk uncertainty 61, 1–18. doi:10.1007/s11166-020-09336-3
Dong, E., Du, H., and Gardner, L. (2020). An interactive web-based dashboard to track COVID-19 in real time. Lancet Infect. Dis. doi:10.1016/S1473-3099(20)30120-1
Federal Aviation Administration (2020a). Aviation system performance metrics (ASPM), Washington, DC: Federal Aviation Administration.
Federal Aviation Administration (2020b). CARES Act—airport grants, Washington, DC: Federal Aviation Administration.
Goldstein, B. A., Hubbard, A. E., Cutler, A., and Barcellos, L. F. (2010). An application of random forests to a genome-wide association dataset: methodological considerations & new findings. BMC Genet. 11, 49. doi:10.1186/1471-2156-11-49
ICAO (2016). Airport air quality manual, attachment B to appendix 1—simplified aircraft emission indices, ICAO: Montreal, QC. 1, 98.
IPCC (2006). IPCC guidelines for National greenhouse gas inventories, intergovernmental panel on climate change. Energy Chap. 3: Mob. Combust. 2, 3.56–3.74.
Janitza, S., and Hornung, R. (2018). On the overestimation of random forest's out-of-bag error. PLoS One 13, e0201904. doi:10.1371/journal.pone.0201904
Kraemer, M. U. G., Yang, C.-H., Gutierrez, B., Wu, C.-H., Klein, B., Pigott, D. M., et al. (2020). The effect of human mobility and control measures on the COVID-19 epidemic in China. Science 368, 493–497. doi:10.1126/science.abb4218
Latora, V., and Marchiori, M. (2001). Efficient behavior of small-world networks. Phys. Rev. Lett. 87, 198701. doi:10.1103/physrevlett.87.198701
MIT Election Data and Science Lab (2018). County presidential election returns 2000-2016. doi:10.7910/DVN/VOQCHQ
Morrison, D. (1969). On the interpretation of discriminant analysis. J. Marketing Res. 6. doi:10.2307/3149666
Onnela, J.-P., Saramäki, J., Hyvönen, J., Szabó, G., Lazer, D., Kaski, K., et al. (2007). Structure and tie strengths in mobile communication networks. Pnas 104, 7332–7336. doi:10.1073/pnas.0610245104
Oshiro, T., Perez, P., and Baranauskas, J. A. (2012). How many trees in a random forest? Berlin, Germany: Springer, 154–168.
Strobl, C., Boulesteix, A.-L., Kneib, T., Augustin, T., and Zeileis, A. (2008). Conditional variable importance for random forests. BMC bioinformatics 9, 1–11. doi:10.1186/1471-2105-9-307
Verma, S. K., Bhadauria, S. S., and Akhtar, S. (2014). Monitoring corrosion of steel bars in reinforced concrete structures. Scientific World J. 2014, 1–9. doi:10.1155/2014/957904
Keywords: emissions, resilience, network science, aviation, covid-19
Citation: Bauranov A, Parks S, Jiang X, Rakas J and González MC (2021) Quantifying the Resilience of the U.S. Domestic Aviation Network During the COVID-19 Pandemic. Front. Built Environ. 7:642295. doi: 10.3389/fbuil.2021.642295
Received: 15 December 2020; Accepted: 16 February 2021;
Published: 12 April 2021.
Edited by:
Erika Fille Legara, Asian Institute of Management, PhilippinesReviewed by:
Jesus Felix Bayta Valenzuela, Asian Institute of Management, PhilippinesCopyright © 2021 Bauranov, Parks, Jiang, Rakas and González. This is an open-access article distributed under the terms of the Creative Commons Attribution License (CC BY). The use, distribution or reproduction in other forums is permitted, provided the original author(s) and the copyright owner(s) are credited and that the original publication in this journal is cited, in accordance with accepted academic practice. No use, distribution or reproduction is permitted which does not comply with these terms.
*Correspondence: Aleksandar Bauranov, YmF1cmFub3ZAZ3NkLmhhcnZhcmQuZWR1
Disclaimer: All claims expressed in this article are solely those of the authors and do not necessarily represent those of their affiliated organizations, or those of the publisher, the editors and the reviewers. Any product that may be evaluated in this article or claim that may be made by its manufacturer is not guaranteed or endorsed by the publisher.
Research integrity at Frontiers
Learn more about the work of our research integrity team to safeguard the quality of each article we publish.