- 1Department of Biomedical Engineering, Faculty of Electrical Engineering and Communication, Brno University of Technology, Brno, Czechia
- 2Molecular Systems Biology (MOSYS), Department of Functional and Evolutionary Ecology, University of Vienna, Vienna, Austria
- 3Department of Microbiology and Antimicrobial Resistance, Veterinary Research Institute, Brno, Czechia
- 4Department of Biology, University of Oxford, Oxford, United Kingdom
The horizontal transfer of mobile gene elements between bacteria plays a crucial role in their evolutionary dynamics. Additionally, it enables the accumulation and dissemination of genes conferring antibiotic and heavy-metal resistance, and thus contributes to the worldwide emergence and spread of drug-resistance pathogens. This process is instrumental in maintaining genetic diversity within bacterial populations and facilitates their adaptation to novel environments. It allows bacteria to acquire genes responsible for the synthesis of enzymes that utilize alternative energy sources and substrates. Furthermore, bacteria can acquire genes associated with toxin production and increased virulence. Horizontal gene transfer serves as a pivotal mechanism in bacterial evolution, enabling the acquisition of novel genetic information and enhancing their capabilities. However, the proper detection and identification of horizontally transferred genes at the microbiome scale is challenging, whether using wet-lab experiments or bioinformatics approaches. In the paper, we summarize current bioinformatics tools for detecting Horizontal Gene Transfer and present the results of our bioinformatic analysis on a collection of genomes originating from chicken gut microbiota.
Introduction
Transfer of Mobile Gene Elements (MGEs) among bacteria is divided into two basic categories in the literature: Horizontal Gene Transfer (HGT) and Vertical Gene Transfer (VGT). VGT (Lawrence, 2005) is a process transmitting gene sequences from ancestor to descendant. This process is governed by Mendel’s laws of inheritance: Law of segregation, Law of independent assortment, and Law of dominance and recessiveness. From the evolutionary standpoint, VGT plays a crucial role in heredity, however, the HGT processes have established the groundwork for new evolutionary paradigms and are currently at the forefront of genomics research (Boto, 2010).
Through comparative genomic analysis, it has been revealed that HGT is prevalent among prokaryotes (Boto, 2010) and holds significant implications for adaptation and evolution, e.g., 17% of the Escherichia coli and Salmonella sp. genomes have been acquired by HGT over the last 100 million years (Lawrence and Ochman, 1998; Ho and Waldor, 2007). The ability of bacteria to adapt to new environments within the framework of evolution is often achieved through the acquisition of new genes via HGT, rather than mutations (Van Dijk et al., 2020). One notable illustration of this concept is the molecular arms race, which has significantly propelled the evolution of pathogenicity. Pathogens frequently possess multiple mechanisms or systems that perform similar functions, leading to redundancy in their capabilities. For example, they may have redundant sets of proteins, such as type III protein secretion effectors, which can manipulate host cells. These redundant systems provide a survival advantage to the pathogen, ensuring that even if one mechanism is compromised, others may still be functional. In contrast, hosts continually evolve and enhance their immune systems in response to these pathogenic challenges, leading to the development of increasingly sophisticated defence mechanisms (Jackson et al., 2011). On the contrary, some pathogens with narrow host range have adapted to the environment by gene deletion (Šmajs et al., 2011).
To gain a comprehensive understanding of HGT issues and scientific challenges in this field, it is important to introduce the fundamental terms used in HGT nomenclature. The bacterium that donates the gene is referred to the donor, while the bacterium that receives the gene is known as the recipient. The concept of HGT encompasses three fundamental mechanisms: conjugation, transduction, and natural transformation (Lapierre et al., 2014). Conjugation is a process of genetic recombination process in which DNA is transferred from the donor to the recipient through cell-to-cell pilus contact encoded by the F plasmid. Transduction is the transfer of DNA sequences from one bacterium to another by means of a bacteriophage or phage-like particle. Natural transformation is the uptake or integration of extracellular DNA, which can occur under the natural conditions of bacterial life (Blokesch, 2017; Winter et al., 2021).
Recent advances in technology have provided extensive opportunities for bacterial cultivation and sequencing of vast amounts of previously uncharacterized prokaryotes (Lagier et al., 2012; Medvecky et al., 2018). However, in the quest to identify horizontally transferred genes among diverse bacteria and accurately detect the mobility of MGEs, it is crucial to standardize tools while ensuring reproducible results. Although HGT has gained significant attention in research, its standardization through in-silico analysis, statistical methods, and data mining remains only partially explored. Therefore, this concise review aims to summarize the existing information on bioinformatic analysis and shed light on the identification and visualization of mobile genetic elements within the gut microbiome using a real dataset.
Databases and associated tools
The current study provides an overview and summary of the available databases and associated tools, encompassing information about MGEs and HGT. In Table 1, twenty-three distinct tools, pipeline and databases are outlined. Each of these software options has been categorized based on a typing that corresponds to specific data or functions.
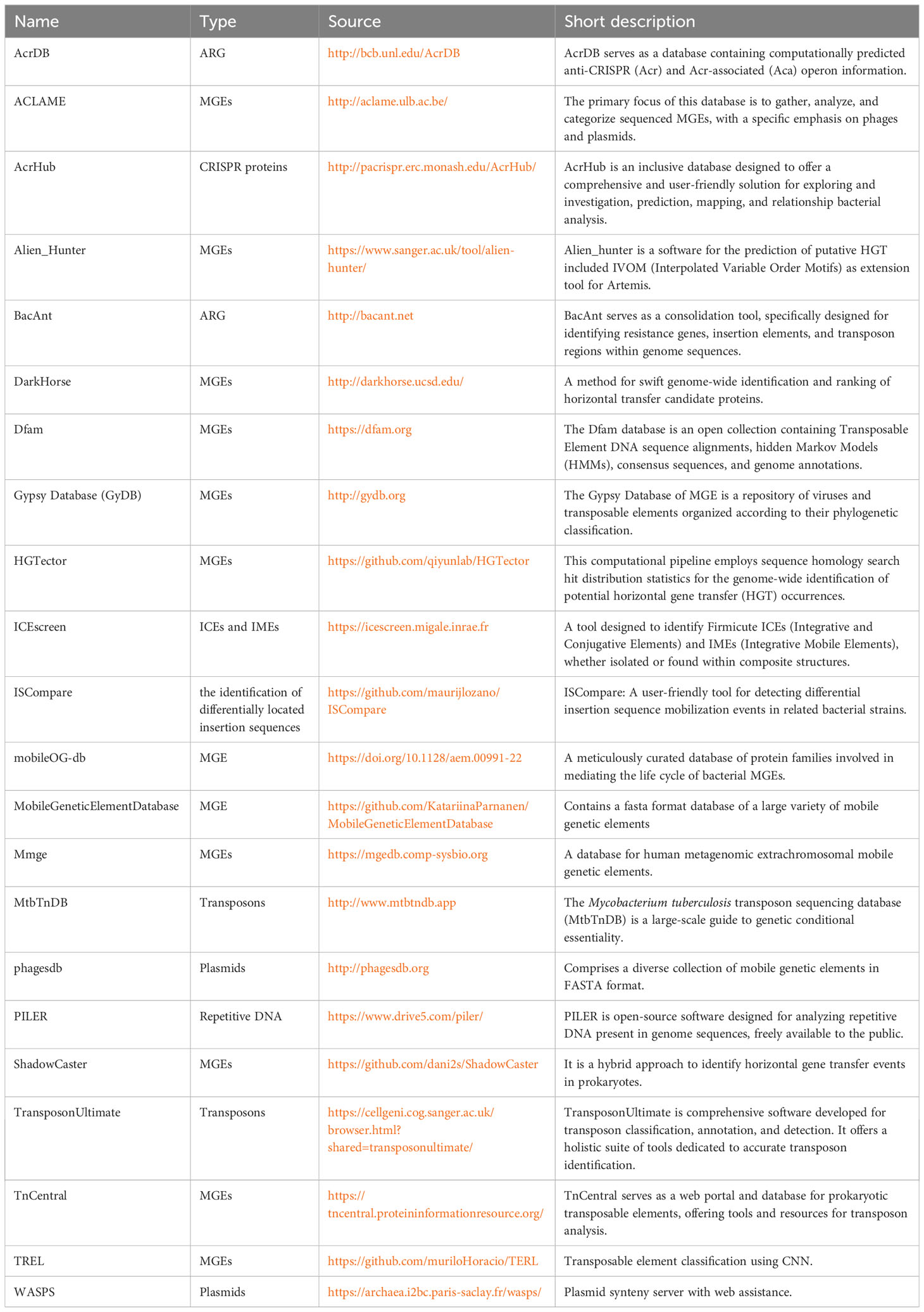
Table 1 Overview of approaches and databases for identifying MGEs in bacterial genomes and metagenomes.
Real tested dataset: a chicken gut microbiome collection
The minireview article presented herein also extends the analysis of our previous study (Schwarzerova et al., 2022). The methodology, including detailed processing steps, is provided in Supplement 1. This supplement contains a thorough description of each step, along with the specific parameters that were applied to analyze our real dataset from the microbiome collection. The genomes of 279 anaerobic chicken gut isolates (BioProject number PRJN377666) were analyzed, emphasizing the significant role of the chicken microbiota as a reservoir of MGEs (Yang et al., 2022). According to the current version of Genome Taxonomy Database: GTDB (Chaumeil et al., 2022), genome collection included eleven phyla: Actinobacteriota, Bacteroidota, Desulfobacterota, Elusimicrobiota, Firmicutes, Firmicutes_A, Firmicutes_C, Fusobacteriota, Proteobacteria, Synergistota and Verrucomicrobiota.
Methodological approaches to investigate portable mobile gene elements
Different approaches have been developed to detect HGT in genomic data. Based on the methodology basic approaches can be classified as follows:
(1) co-transfer between bacteria using gene-gene associations;
(2) combination of phylogenetic and best-match analysis;
(3) matrix-based homology search for co-transfer between bacteria;
(4) probabilistic search and genome composition analysis;
(5) isolation of easy-to-cultivate bacteria, such as lactobacilli, bifidobacterial, or members of Enterobacterales, followed by the characterization of their full genome sequences.
Co-transfer between bacteria using gene-gene associations
The analyzed genomes are screened against the curated databases (Table 1) for the presence of potential gene candidates associated with MGEs. The process can be referred to as MGE screening. In addition, several tools are accessible for detecting MGE insertions (Table 1), for example, Mauve, a whole-genome alignment tool that can effectively identify large insertions referred to as “genomic islands” (Durrant et al., 2020).
By applying this methodology, we detected in our real test dataset the tetQ (Shoemaker et al., 2001), mecA (Lambert, 2005), and mepA (Chizhevskaya et al., 2018) genes within our real dataset. These genes confer antibiotic resistance and were most prevalent in individuals of the Bacteroidota. Figure 1 (section A) provides an example of visualization of the mecA gene.
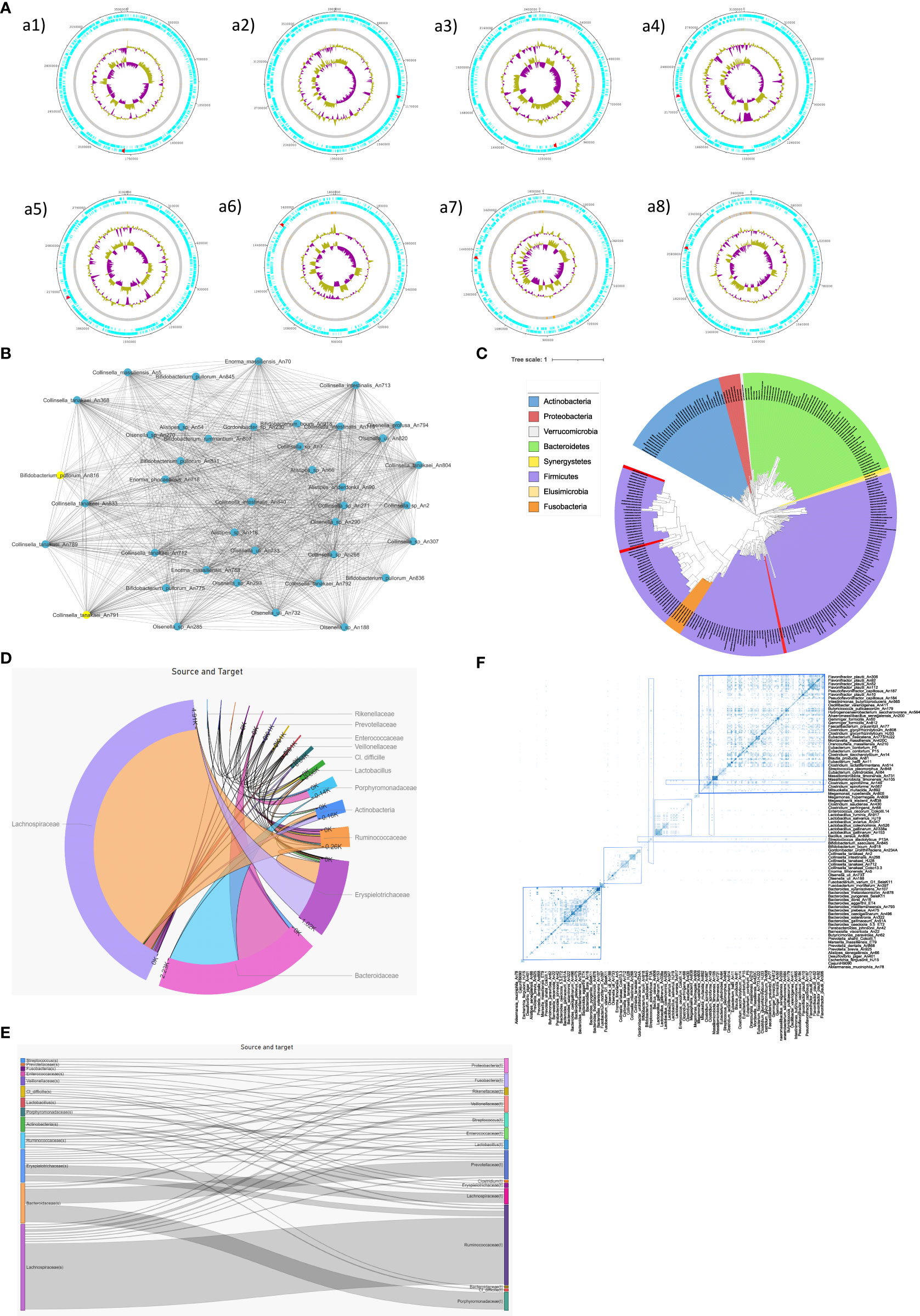
Figure 1 (A) Individual visualization of 8 selected genomes where red color represents mecA gene across different genomes; a1] Enterococcus gallinarum An774; a2] Anaerobutyricum sp002161065 An11; a3] Faecalicatena concorta An936; a4] Faecalicatena concorta An846; a5] Faecalitalea cylindroides An733; a6] Ligilactobacilluss salivarius An63; a7] Ligilactobacilluss salivarius An453; a8] Streptococcus gallolyticus An908; (B) Network visualization of HGT events among strains of Actinobacteriota using the Cytoscape platform; (C) Tree visualization of MGE using ITOL (Letunic & Bork, 2007); the red lines highlight detected mecA genes with the same sequence identity in different species; (D) Chord diagram applied on tested dataset; (E) Sankey diagram - an alternative visualization of chord diagram. Both are used to represent the flow/connection and the corresponding frequency depicted by the thickness/width of the bars and edges; (F) Matrix visualization of the abundance of genes co-transferred between bacterial genomes from the examined bacterial collection; blue frames indicated MGEs co-shared by phylogenetically closely related individuals, whereas red frames depicted MGEs co-shared by taxonomically more distant bacterial genomes.
Utilizing genomic databases and computational tools for HGT detection enhances efficiency and facilitates access to extensive genetic data. MGE screening plays a pivotal role in identifying crucial genetic elements like plasmids, transposons, and phages (Table 1), shedding light on their roles in gene transfer and adaptation. This screening is especially critical for monitoring the dissemination of antibiotic resistance genes, which holds significant implications for public health and also provides valuable insights into genome evolution and adaptive mechanisms within changing environments. MGE screening, however, is susceptible to both false-positive and false-negative results, which depend on the completeness and accuracy of genomic databases. It also poses challenges in elucidating the functional roles of MGEs, including the potential oversight of unexplored genes involved in HGT, such as hypothetical proteins. As a result, confirming the presence of MGEs often requires labor-intensive and resource-intensive experimental validation.
Integrating phylogenetic and best-match approaches in HGT detection
An alternative way for MGE detection centers on diverse phylogenetic clustering approaches, as demonstrated in various studies (Arredondo-Alonso et al., 2023; Nakao et al., 2016; Schwarzerova et al., 2022). The UBCG (Up-to-date bacterial core gene) (Na et al., 2018) seems to serve as a fitting gold standard approach to construct correct phylogenetic tree across different phyla. It comprises a suite of tools for fundamental analysis, enabling the generation and assessment of phylogenetic trees for bacterial sequences.
Within the examined dataset, different bacteria shared an identical mecA gene sequence despite significant genomic differences and their presence in separate branches of the phylogenetic tree. Therefore, based on the results of our phylogenetic analysis (Figure 1C), we suggest that the mecA gene is highly likely to have undergone HGT. The mecA gene is often associated with resistance to methicillin (Ito et al., 2012). This gene encodes a low-affinity penicillin-binding protein (PBP), designated PBP2a or PBP2′. In most staphylococci, mecA is part of a chromosomally integrated mobile genetic element called the staphylococcal cassette chromosome mec (SCCmec) (Ito et al., 2012). A study conducted by Juhas et al. (Juhas et al., 2009) further corroborated that mecA falls within the category of horizontally transmitted MGEs. This may be a confirmation that this gene belongs to the group of MGEs that are transmitted horizontally, also within our tested dataset.
Best-match approaches in HGT detection offer simplicity and efficiency for rapid screening. However, they are limited in resolution, prone to generating false positives based solely on sequence similarity, and often unable to determine the direction of gene transfers. On the other hand, phylogenetic approaches provide an invaluable evolutionary context, statistical support, and the ability to infer gene lineages and potential donors and recipients. Nevertheless, these methods can be computationally intensive, reliant on data quality, and sensitive to parameter choices. Integrating both approaches can enhance HGT detection, combining efficiency with evolutionary context and statistical rigor to yield more reliable results.
Matrix-based homology search for co-transfer between bacteria
Matrix-based homology is a representation using heatmaps of the abundance of genes co-shared by different genomes. Detection can be achieved based on prior phylogenetic clustering and finding homology, e.g., a pipeline, from the study of (Schwarzerova et al., 2022).
The heatmap (Figure 1, section F) was created in R v. 4.1.3 using R package RColorBrewer (Neuwirth, 2022). MGEs can be easily detected in the visualization of the matrix.
Probabilistic search and genome composition analysis
Probabilistic search methods have become one of the indispensable methods in investigating HGT across different organisms. These methods use statistical models and algorithms to assess the likelihood of gene transfer between species based on sequence homology and evolutionary patterns (Vernikos and Parkhill, 2006; Sánchez-Soto et al., 2020). Using probabilistic approaches, researchers can identify potential HGT candidates and distinguish them from vertically inherited genes. These methods take into account various factors, such as codon usage and sequence composition, to estimate the probability of gene transfer events. Probabilistic search techniques enable the identification of HGT events even in cases where sequence similarities are low, thus enhancing our understanding of the complex evolutionary processes involving horizontal gene transfer. On the contrary, to identify HGT events between closely related organisms, the loss of synteny theory can be employed (Sevillya et al., 2020). As the field of bioinformatics continues to advance, probabilistic searching of HGT remains an essential tool for unraveling the complex dynamics of genetic exchange and its impact on the evolution of diverse organisms.
In summary, genome composition analysis offers a comprehensive view of genomic features but may require expert interpretation and can be computationally demanding.
Isolation of easy-to-cultivate organisms, such as members of Enterobacteriaceae, followed by their full genome sequence characterization
Isolating members of the Enterobacteriaceae family and characterizing their full genome sequences regarding HGT is an important step in understanding the dynamics of genetic exchange among these bacteria (Stecher et al., 2012; Tamilmaran et al., 2021). By obtaining pure cultures of Enterobacteriaceae strains and performing whole-genome sequencing, researchers can explore the presence of MGEs and identify potential HGT events. Various bioinformatics tools and approaches, including comparative genomics and phylogenetic analyses, are employed to identify regions with distinct evolutionary histories, indicating potential HGT events (Sato and Miyazaki, 2017). The characterization of these HGT events can shed light on the transfer of beneficial traits, such as antibiotic resistance genes or virulence factors, among Enterobacteriaceae members. Understanding the patterns and mechanisms of HGT within this family is crucial in managing antimicrobial resistance and controlling the spread of pathogenic traits among bacterial populations. Overall, the full genome sequence characterization of Enterobacteriaceae members regarding HGT provides valuable insights into the genetic plasticity and adaptability of these bacteria, aiding in the development of strategies to combat emerging health threats.
On the other hand, this method primarily targets cultivable organisms, potentially overlooking the diversity of HGT events involving non-cultivable or less easily cultivable microorganisms, which can be prevalent in specific environments. Consequently, HGT detection tends to favor well-studied organisms, potentially leading to the oversight of new HGT events. Additionally, this approach may introduce bias in the selection of isolates chosen for sequencing, further affecting the comprehensiveness of HGT detection.
From data to insight: a survey of MGE visualization methods
Various approaches exist for visualizing MGEs, each offering unique insights into their distribution, organization, and potential functional significance. The summary of some possible visualization is given in Figure 1. Linear representations provide a detailed sequential display of the host genome, enabling precise annotation and assessment of MGE positions (Figure 1A). One commonly employed method is tree visualization (Figure 1C). This approach allows for an overview of MGE locations in relation to other genetic elements. Network-based visualizations, such as gene interaction networks, capture the intricate relationships between MGEs and surrounding genetic elements (Figure 1B). Chord (Figure 1D) and Sankey (Figure 1E) show the flow/connection and their associated frequency. In addition, heatmaps and density plots offer a quantitative depiction of MGE distribution across genomes, aiding in the identification of hotspots or regions of frequent insertion (Figure 1F). Each visualization technique contributes to a deeper understanding of MGE dynamics, facilitating the exploration of their roles in evolution, adaptation, and genetic diversity across different organisms.
Conclusion and future perspectives
MGEs play a crucial role in maintaining genetic diversity among bacterial populations and enable them to adapt to new environments by acquiring genes for alternative energy utilization, toxin production, and increased virulence. The establishment of standardized tools and pipelines is essential to ensure reproducible and reliable results in the study of HGT. Furthermore, comprehending of MGEs is crucial for addressing the spread of pathogens and the emergence of bacteria with diverse resistance genes, which pose challenges to conventional therapeutic interventions.
This brief overview highlights the importance of bioinformatic analysis in the understanding mobile genetic elements in the microbiome research. The use of real datasets enhances our grasp of HGT dynamics and provides valuable insights into bacterial evolution. Additionally, we offer an overview of databases and tools that contribute to MGE and HGT research. We also present various detection methodologies for MGEs and HGT, exemplified with our microbiome dataset.
The future of HGT detection holds great promise but also presents significant challenges. Machine learning and artificial intelligence will play a crucial role in enhancing the accuracy of HGT detection by uncovering subtle patterns in extensive datasets. Long-read sequencing technologies, particularly their increasing availability and decreasing costs, will significantly enhance our capacity to explore intricate genomic regions, encompassing mobile genetic elements and transferred genes. Emerging experimental technologies (Saak et al., 2020), offer the potential to comprehensively understand the network of potential DNA transfers in diverse microbial communities. However, several challenges, such as distinguishing ancient and recent HGT events, will persist. In summary, HGT detection is progressing, providing valuable insights into gene transfer across various fields.
Author contributions
JS: Conceptualization, Formal Analysis, Visualization, Writing – original draft. AL: Formal Analysis, Investigation, Writing – original draft. MV: Visualization, Writing – review & editing. IR: Conceptualization, Formal Analysis, Methodology, Writing – review & editing. DC: Conceptualization, Writing – review & editing, Funding acquisition.
Funding
The author(s) declare financial support was received for the research, authorship, and/or publication of this article. This work has been funded by the Czech Science Foundation (22-16786S) to DC. Computational resources were provided by the e-INFRA CZ project (ID:90140), supported by the Ministry of Education, Youth and Sports of the Czech Republic.
Conflict of interest
The authors declare that the research was conducted in the absence of any commercial or financial relationships that could be construed as a potential conflict of interest.
The author DC declared that they were an editorial board member of Frontiers, at the time of submission. This had no impact on the peer review process and the final decision.
Publisher’s note
All claims expressed in this article are solely those of the authors and do not necessarily represent those of their affiliated organizations, or those of the publisher, the editors and the reviewers. Any product that may be evaluated in this article, or claim that may be made by its manufacturer, is not guaranteed or endorsed by the publisher.
Supplementary material
The Supplementary Material for this article can be found online at: https://www.frontiersin.org/articles/10.3389/fbrio.2023.1275910/full#supplementary-material
References
Arredondo-Alonso S., Gladstone R. A., Pöntinen A. K., Gama J. A., Schürch A. C., Lanza V. F., et al. Consistent typing of plasmids with the mge-cluster pipeline. (2023). NAR Genom Bioinform. 5 (3), lqad066. doi: 10.1101/2022.12.16.520696
Blokesch M. (2017). In and out—contribution of natural transformation to the shuffling of large genomic regions. Curr. Opin. Microbiol. 38, 22–29. doi: 10.1016/j.mib.2017.04.001
Boto L. (2010). Horizontal gene transfer in evolution: Facts and challenges. Proc. R. Soc. B: Biol. Sci. 277 (1683), 819–827. doi: 10.1098/rspb.2009.1679
Chaumeil P. A., Mussig A. J., Hugenholtz P., Parks D. H. (2022). GTDB-Tk v2: Memory friendly classification with the genome taxonomy database. Bioinf. (Oxford England) 38 (23), 5315–5316. doi: 10.1093/bioinformatics/btac672
Chizhevskaya E. P., Naidenova E. A., Onishchuk O. P., Andronov E. E., Simarov B. V. (2018). The melanin biosynthesis gene from the CA15-1 strain of alfalfa nodule bacteria: Molecular analysis and phylogeny. Russian J. Genet. 54 (8), 925–932. doi: 10.1134/S1022795418080045
Durrant M. G., Li M. M., Siranosian B. A., Montgomery S. B., Bhatt A. S. (2020). A bioinformatic analysis of integrative mobile genetic elements highlights their role in bacterial adaptation. Cell Host Microbe 27 (1), 140–153.e9. doi: 10.1016/j.chom.2019.10.022
Ho T. D., Waldor M. K. (2007). Enterohemorrhagic Escherichia coli O157:H7 gal mutants are sensitive to bacteriophage P1 and defective in intestinal colonization. Infection Immun. 75 (4), 1661–1666. doi: 10.1128/IAI.01342-06
Ito T., Hiramatsu K., Tomasz A., De Lencastre H., Perreten V., Holden M. T. G., et al. (2012). Guidelines for reporting novel mecA gene homologues. Antimicrobial Agents Chemotherapy 56 (10), 4997–4999. doi: 10.1128/AAC.01199-12
Jackson R. W., Vinatzer B., Arnold D. L., Dorus S., Murillo J. (2011). The influence of the accessory genome on bacterial pathogen evolution. Mobile Genet. Elements 1 (1), 55–65. doi: 10.4161/mge.1.1.16432
Juhas M., van der Meer J. R., Gaillard M., Harding R. M., Hood D. W., Crook D. W. (2009). Genomic islands: Tools of bacterial horizontal gene transfer and evolution. FEMS Microbiol. Rev. 33 (2), 376–393. doi: 10.1111/j.1574-6976.2008.00136.x
Lagier J. C., Armougom F., Million M., Hugon P., Pagnier I., Robert C., et al. (2012). Microbial culturomics: Paradigm shift in the human gut microbiome study. Clin. Microbiol. Infection 18 (12), 1185–1193. doi: 10.1111/1469-0691.12023
Lambert P. A. (2005). Bacterial resistance to antibiotics: Modified target sites. Advanced Drug Delivery Rev. 57 (10), 1471–1485. doi: 10.1016/j.addr.2005.04.003
Lapierre P., Lasek-Nesselquist E., Gogarten J. P. (2014). The impact of HGT on phylogenomic reconstruction methods. Briefings Bioinf. 15 (1), 79–90. doi: 10.1093/bib/bbs050
Lawrence J. G. (2005). Horizontal and vertical gene transfer: the life history of pathogens. Contributions to Microbiol. 12, 255–271. doi: 10.1159/000081699
Lawrence J. G., Ochman H. (1998). Molecular archaeology of the Escherichia coli genome Proc. Natl. Acad. Sci. USA 95(16), 9413–9417. doi: 10.1073/pnas.95.16.9413
Letunic I., Bork P. (2007). Interactive Tree Of Life (iTOL): An online tool for phylogenetic tree display and annotation. Bioinformatics 23 (1), 127–128. doi: 10.1093/bioinformatics/btl529
Medvecky M., Cejkova D., Polansky O., Karasova D., Kubasova T., Cizek A., et al. (2018). Whole genome sequencing and function prediction of 133 gut anaerobes isolated from chicken caecum in pure cultures. BMC Genomics 19 (1), 561. doi: 10.1186/s12864-018-4959-4
Na S. I., Kim Y. O., Yoon S. H., Ha S., Baek I., Chun J. (2018). UBCG: Up-to-date bacterial core gene set and pipeline for phylogenomic tree reconstruction. J. Microbiol. 56 (4), 281–285. doi: 10.1007/s12275-018-8014-6
Nakao R., Abe T., Funayama S., Sugimoto C. (2016). Horizontally transferred genetic elements in the tsetse fly genome: an alignment-free clustering approach using batch learning self-organising map (BLSOM). BioMed. Res. Int. 2016. doi: 10.1155/2016/3164624
Neuwirth E. (2022) Title colorBrewer palettes. Available at: http://colorbrewer2.org.
Saak C. C., Dinh C. B., Dutton R. J. (2020). Experimental approaches to tracking mobile genetic elements in microbial communities. FEMS Microbiol. Rev. 44 (5), 606–630. doi: 10.1093/femsre/fuaa025
Sato M., Miyazaki K. (2017). Phylogenetic network analysis revealed the occurrence of horizontal gene transfer of 16S rRNA in the genus Enterobacter. Front. Microbiol. 8 (NOV). doi: 10.3389/fmicb.2017.02225
Sánchez-Soto D., Agüero-Chapin G., Armijos-Jaramillo V., Perez-Castillo Y., Tejera E., Antunes A., et al. (2020). Shadowcaster: compositional methods under the shadow of phylogenetic models to detect horizontal gene transfers in prokaryotes. Genes 11 (7), 1–12. doi: 10.3390/genes11070756
Schwarzerova J., Zeman M., Rychlik I., Weckwerth W., Provaznik I., Dolejska M., et al. (2022). “Systems biology approach for analysis of mobile genetic elements in chicken gut microbiome,” in Proceedings - 2022 IEEE International Conference on Bioinformatics and Biomedicine, BIBM 2022. New York, USA: IEEE 2865–2870. doi: 10.1109/BIBM55620.2022.9995414
Sevillya G., Adato O., Snir S. (2020). Detecting horizontal gene transfer: a probabilistic approach. BMC Genomics 21. (Suppl 1), 106doi: 10.1186/s12864-019-6395-5
Shoemaker N. B., Vlamakis H., Hayes K., Salyers A. A. (2001). Evidence for extensive resistance gene transfer among Bacteroides spp. and among Bacteroides and other genera in the human colon. Appl. Environ. Microbiol. 67 (2), 561–568. doi: 10.1128/AEM.67.2.561-568.2001
Šmajs D., Zobaníková M., Strouhal M., Čejková D., Dugan-Rocha S., Pospíšilová P., et al. (2011). Complete genome sequence of Treponema paraluiscuniculi, strain Cuniculi A: the loss of infectivity to humans is associated with genome decay. PLoS One 6 (5), e20415. doi: 10.1371/journal.pone.0020415
Stecher B., Denzler R., Maier L., Bernet F., Sanders M. J., Pickard D. J., et al. (2012). Gut inflammation can boost horizontal gene transfer between pathogenic and commensal Enterobacteriaceae. Proc. Natl. Acad. Sci. United States America 109 (4), 1269–1274. doi: 10.1073/pnas.1113246109
Tamilmaran N., Sankaranarayanan R., Selvakumar A.S P., Munavar M. H. (2021). Horizontal transfer of domains in ssrA gene among Enterobacteriaceae. Genes to Cells 26 (7), 541–550. doi: 10.1111/gtc.12869
Van Dijk B., Hogeweg P., Doekes H. M., Takeuchi N. (2020). Slightly bene1cial genes are retained by bacteria evolving DNA uptake despite sel1sh elements. ELife 9, 1–36. doi: 10.7554/eLife.56801
Vernikos G. S., Parkhill J. (2006). Interpolated variable order motifs for identification of horizontally acquired DNA: Revisiting the Salmonella pathogenicity islands. Bioinformatics 22 (18), 2196–2203. doi: 10.1093/bioinformatics/btl369
Winter M., Buckling A., Harms K., Johnsen P. J., Vos M. (2021). Antimicrobial resistance acquisition via natural transformation: Context is everything. Curr. Opin. Microbiol. 64, 133–138. doi: 10.1016/j.mib.2021.09.009
Keywords: horizontal gene transfer, bacterial genomics, mobilome, microbiome, genome analysis
Citation: Schwarzerova J, Labanava A, Rychlik I, Varga M and Cejkova D (2023) A minireview on the bioinformatics analysis of mobile gene elements in microbiome research. Front. Bacteriol. 2:1275910. doi: 10.3389/fbrio.2023.1275910
Received: 10 August 2023; Accepted: 22 September 2023;
Published: 11 October 2023.
Edited by:
Yanjiao Zhou, UCONN Health, United StatesReviewed by:
Han Mei, Stanford University, United StatesCopyright © 2023 Schwarzerova, Labanava, Rychlik, Varga and Cejkova. This is an open-access article distributed under the terms of the Creative Commons Attribution License (CC BY). The use, distribution or reproduction in other forums is permitted, provided the original author(s) and the copyright owner(s) are credited and that the original publication in this journal is cited, in accordance with accepted academic practice. No use, distribution or reproduction is permitted which does not comply with these terms.
*Correspondence: Darina Cejkova, cejkovad@vut.cz