- 1Global Variations, Geneva, Switzerland
- 2Assemblée Nationale, Paris, France
Context: The third generation of cryptocurrencies gathers cryptocurrencies that are as diverse as the market is big (e.g., Dogecoin or Litecoin). While Dogecoin is seen as a memecoin, the other gathers a very different category of investors. To our knowledge, no study has independently assessed the crypto community’s economical impact on these cryptocurrencies. Furthermore, various methodological possibilities exist to forecast cryptocurrency price—mainly coming from online communities.
Method: Our study has retrospectively studied (from 01/01/2015 to 03/11/2021)—using open access data—the association strength (using normalized mutual information) and the linear correlation (using Pearson’s correlation) between Twitter activity and cryptocurrency economical attributes. In addition, we have computed different models (ADF, ARIMA, and Interpretable MultiVvariable Long Short-Term Memory recurrent neural network) that forecast past price values and assessed their precision.
Findings and conclusions: While the average Dogecoin transaction value is impacted by tweets, tweets are impacted by Litecoin transactions number and average Litecoin transaction value. Tweet number is impacted by Dogecoin whale behavior, but no significant relationship was found between Litecoin whales and tweets. The forecasting error resulting from our ARIMA (0,0,0) models was 0.08% (with Litecoin) and 0.22% (with Dogecoin). Therefore, those are just the beginning of scientific findings that may lead to building a trading robot based on these results. However, in itself, this study is only for academic discussion, and conclusions need to be drawn by further research. The authors cannot be liable if any financial investment is made based on its conclusions.
Introduction
Since Satoshi Nakamoto’s whitepaper in 2008, cryptocurrencies have grown to a huge market capitalization—currently over $2T (as of December 2021). This huge rise in cryptocurrency market capitalization seems, at first glance, deeply linked to the cryptocurrency community. Indeed, most coins have a strong community promoting them through social networks. One of the most relevant examples when talking about online advertising of a coin might be Elon Musk’s tweets. He seemed to have a huge impact on the cryptocurrency market as value seems to increase or decrease as he tweets, which could constitute an insider delay. However, according to a big data study (Tandon et al., 2021), it can clearly be stated that Elon Musk cannot control the utter volatile world of cryptocurrencies and especially Bitcoin and Dogecoin.
But the price of cryptocurrency could be more driven by the Lindy effect than anything else. This theory states that the future life expectancy of certain non-perishable goods—such as a technology or an idea—is proportional to their current age. Indeed, the longer something has been around, the more chances there are that it will survive longer, and among memes, competition for survival is fierce. In this jungle, the average lifespan is roughly four months. When compared to other memes, Doge is kind of a venerated elder. By surviving for eight years—The Doge meme first became popular in 2013—it has already proven to be one of the most resilient memes of the whole internet history. The Lindy effect suggests that for this reason alone, Dogecoin is more likely to persist into the future than any other meme. Just as the U.S. dollar is backed by America’s hegemonic power, Dogecoin is backed by some of the most powerful memes in existence—and the communities behind them. Dogecoin has a real fan base promoting its use through social networks. Part of what has made Dogecoin a successful cryptocurrency is the non-tribalism of its community.
Moreover, while being technically very similar (i.e., almost the same PoW and use cases), Litecoin has a less loud community. Despite being an older and more stable cryptocurrency, Litecoin does not have the same online popularity. Litecoin’s users are not that loud over social networks and do not mean (most of them) to organize coordinated buying in order to influence the currency’s value.
Litecoin and Dogecoin have been selected for this study because of their similarities. Indeed, Litecoin was an early Bitcoin spinoff (or altcoin), starting in October 2011 (Ex-Googler Gives the Worl, 2018), and Dogecoin’s protocol is based on the existing cryptocurrencies: Luckycoin and Litecoin (Gilbert, 2013). Dogecoin was launched on 6 December 2013 (Noyes, 2014). At first glance, these cryptocurrencies are different and might not be comparable.
As explained previously, the Lindy effect allowed Dogecoin—the “memecoin”—to free itself from being just a joke. Thus, after a period of time during which Dogecoin was ignored (Locke, 2021), it was pushed back by its community and became trendy again recently—both at the level of its adoption and at the level of its technical development (Dogecoin Foundation (2021, 2021) (reduction of transaction costs, bridge with ETH, first NFTs are being created on its network). Now, we can see a real decentralized approach coming from this cryptocurrency and its community. Litecoin, on the other hand, has a more centralized development—mainly because of its history—but does not prevent it from having one of the largest capitalizations. Therefore, Litecoin was one of the first altcoins, and Dogecoin was one of the first memecoins (if not the first). As with any cryptocurrency at launch, their future relies on their respective communities. We chose not to use other memecoins for comparison because of their speculative-driven mindset and have been so far removed from cypherpunk libertarian way of life (Hughes, 1993).
As it was broadly studied and well-documented, crypto-economy and the traditional financial economy behavior has about 20 well-known cognitive biases (Douziech, 2021). Here, we will be addressing the question: “how much of an impact does online activity—through Twitter in this study—have on the cryptocurrency market?” Questions like this one have already been raised and studied for Bitcoin.
- Temporal convolutional networks perform significantly better than both autoregressive and other deep learning–based models in the literature, and the tweet author meta-information, even detached from the tweet itself, is a better predictor than the semantic content and tweet volume statistics (Akbiyik et al., 2021);
- Statistical tests show that the simplest GARCH(1,1) has the best reaction to the addition of an external signal in order to model the volatility process on out-of-sample data (Barjašić and Antulov-Fantulin, 2020).
In this way, we will study two main cryptocurrencies (Litecoin and Dogecoin) here.
• Are cryptocurrency prices and tradeoffs affected by the fluctuation in cryptocurrency community tweets?
• Can the price of Litecoin and Dogecoin be forecasted (according to this assumption)? Which statistical forecasting model has the best performance?
• Can Dogecoin or any Memecoin actually become a currency of the future?
Methods
Our analysis is divided into two parts:
— The first one covers the correlation/causality analysis
— The second addresses the possible price forecasting for those cryptocurrencies based upon causality/correlation analysis.
Causality and Correlation Analysis
We used two methods to assess the association presence or absence between X and Y. These two methods were the classical Pearson’s correlation and the normalized Shannon mutual information.
Settings
We used historical data, spanning from 01/01/2015 to 03/11/2021, by extracting various economic trackers as detailed below.
Variables
With each method, we have studied the following variables: “date,” “top_100_percent” 100 first addresses with a large wallet on the studied crypto blockchain (i.e., «whales»), “median_transaction_value,” “market_cap,” “average_transaction_value,” “active_addresses” on Twitter (i.e., most important influencers), and “tweets”.
Data Sources
The data frame comes from three websites (1, 2, 3), but we use two dataframe versions here because of lacking figures for specific days. The first file is the original one which contains some “null” values. But, in order to work with our algorithm, they have been filled (in the second file) with the average value of the last existing value and the next one. This allows us to work with our files without introducing a new bias in our correlations.
Statistical Method
Obviously, correlation is not causation; but the lack of correlation implies absence of causality. Correlation (which might be negative or positive) is, therefore, a key component of the scientific process for it evinces collections of variables that may interact with each other, thereby warranting further study. Conversely, this methodology also accounts for the early dismissal of unwarranted hypotheses regarding such interplay between variables.
The first method we used is based on the standard Pearson’s correlation matrix (Caut et al., 2021), and the computation was performed using the Python Numpy library. Then, we controlled the results with two Pearson’s formulas for discrete series and continuous series. Specifically, we used the following function:
numpy.corrcoef (df [cols]. values.T).
where
- df is the dataframe of the data
- cols is the list of columns used for the matrix
First, let us talk about Pearson’s correlation: it is a commonly formulated criticism that one may not establish a linear correlation between a series of quantitative variables and another one of qualitative variables. However, it will help us identify those correlations as we are looking at them (Lev, 1949; Tate, 1954; Kornbrot, 2005). Pearson’s correlation evaluates the linear relationship between two continuous variables. A relationship is said to be linear when a modification of one of the variables is associated with a proportional modification of the other variable.
Spearman’s correlation evaluates the monotonic relationship between two continuous or ordinal variables. In a monotonic relationship, the variables tend to change together but not necessarily at a constant rate. This correlation coefficient is based on the ranked values of each variable rather than the raw data. Therefore, in view of the continuous variables that we will study, Pearson’s correlation seems more suitable to study the immediate impact of tweets on the economic parameters of Dogecoin and Litecoin.
The second method is based on mutual information entropy (Pébaÿ, 2021; Pébaÿ et al., 2021). This allows us to be free from the limitation assumption of monotony required by linear correlation. It measures the quantity of information (in the sense defined by Claude Shannon in 1948 (Shannon, 1948)) that two distributions share. In other words, it measures the association (“clustering”) between two variables: it is important to notice that his approach is not linear correlation but classical information entropy. Indeed, we computed a dimensionless quantity, generally expressed in units of bits (Thompson and Pebay, 2009), which may be thought of as the reduction in uncertainty about one random variable given the knowledge of another. For instance, high mutual information means a large reduction in uncertainty on one variable, given the other, whereas low mutual information indicates a small reduction in this uncertainty; eventually, zero mutual information between two random variables entails no association between the two distributions (McDaid et al., 2011). Furthermore, Shannon’s source coding theorem establishes strict bounds on what can be known about one data series and state might be compressed—which, in turn, explains how and to what extent one variable might be a proxy of another one without data loss. Shannon information entropy has been demonstrated to be especially efficient for algorithmic complexity evaluation when evaluated with the block decomposition method (Zenil et al., 2016; Zenil, 2020). Moreover, according to N. N. Taleb, entropy metrics solve practically all correlation paradoxes in the field of social sciences (or rather, pseudo-paradoxes) (Taleb, 2019). Another important example of the relevance of this technique is that of mother wavelet selection, where it demonstrated superior sensitivity to quantify the changes in signal structure than classical mean-squared error and correlation coefficient (Wijaya et al., 2017).
Other methods could be quoted: classic econometric models, as the wavelet-based exponential generalized autoregressive conditional heteroscedasticity model (Mohammed et al., 2020; Guasti Lima and Assaf Neto, 2022), or causal inference on time series datasets (and thus over stochastic processes) (Palachy, 2019; Shimoni et al., 2019).
Therefore, in order to compute reproducible results, we use the “muinther” R package available on GitHub, which uses these two statistical methods (Lansiaux et al., 2021).
Biases
The first important bias is community size. Indeed, that could impact Pearson’s method, more prone to these issues. However, a larger community will be able to reduce the extreme variations of the variables studied (number of tweets). Therefore, for the two methods, we will not be able to compare the raw data from the samples but only the coefficients (from Pearson’s or the normalized information theory) between these two cryptocurrencies.
The second bias is Pearson’s method in itself. Indeed, by its definition, Pearson’s correlation evaluates the linear relationship between two continuous variables. A relationship is said to be linear when a modification of one of the variables is associated with a proportional modification of the other variable. However, if one moves in a monotonic relationship, the variables tend to change together but not necessarily at a constant rate (de Winter et al., 2016). In this case, Spearman’s correlation would be better.
Price Prediction Model
Settings
We used historical data, spanning from 01/01/2015 to 03/11/2021, by extracting various economic trackers as detailed below.
Variables
Three variables were primarily used:
— “date”
— “tweets” (a continuous variable describing the number of tweets per day with a mention of the interest in cryptocurrency),
— “price” (a continuous variable describing adjusted closure price of the interested cryptocurrency).
If one of these values is missing, data were censored; in this way, we obtained 2,482 data for each variable.
Data Sources
Tweets were collected and extracted from the Twitter API, and prices were extracted on Yahoo Finance (3).
Statistical Method
Price Correlation/Causation With Tweet Number
The same method was used to explore the correlation and causation relationship between Dogecoin/Litecoin price and tweet number. In other words, we first used (all from “muinther” R package) Pearson’s correlation method and then Shannon mutual information entropy to assess that.
Application
Following our correlation/causation analysis, we will be able to determine two functions in order to establish a relationship between currency price and tweet number at some point “t”.
Models
Augmented Dickey–Fuller Test
Since our Litecoin and Dogecoin data are time series datasets, it is important to check whether the data might be somehow stationary. In order to check this, we considered an ADF (an acronym for Augmented Dickey–Fuller) test. These unit roots are the reason for causing unpredictable results in time series data analysis. Thus, the ADF is a significance test, so a null and alternative hypothesis comes into play by that, test statistics are calculated, and the p-value is reported. Based on p-values, the stationarity of data is determined. Basically, ADF determines the trend of data and determines how strongly or weakly the time series is defined by a trend. However, we use three linear regression models to evaluate this.
1) The first type (type1) is a linear model with no drift and linear trend with respect to time:
where d is an operator of first order difference, that is, dx(t) = x(t) - x (t-1), and e(t) is an error term.
2) The second type (type2) is a linear model with drift but no linear trend:
3) The third type (type3) is a linear model with both drift and linear trend:
Stationary data means that data statistical properties do not depend on time. If the given data are non-stationary, we need to change it to being stationary by applying a natural log.
ARIMA
The model we choose to use is ARIMA (stands for AutoRegressive Integrated Moving Average). It is a part of linear regression models mainly used to predict future values based on past behavior of the target. It is said that history does not repeat itself, but it surely has and had its own rhythm. The beauty of ARIMA models is that these do not use any exogenous values imposed on them but rather are completely dependent on the past target values for prediction. ARIMA can be broken as AR, I, and MA. As mentioned previously, AR stands for Auto-Regressive, and it works on the idea of regressing the target on its past variable, which is nothing but lagging on itself. Eq. 1 indicates a value Y is a linear function of its past n values. These n values can be chosen and are beta values used during fitting of the model. This equation helps forecast future values by simply making the following changes as in Eq. 2.
The Integrated part of ARIMA deals with stationalizing data. Here, the differencing is applied on data as shown in Eq. 3. It indicates that the future values of Y are some linear function of its past changes. The reason for differencing is that the time series data are not stable and the values of Y should have mean variance stationary.
Moving average is all summarized in Eq. 4, somewhat similar to AN equation with lags. E indicates that the error in the data is nothing but the residual derivations between the model and target value.
This is the standard notation to represent ARIMA models. These parameters can be replaced with integer values to specify the type of the model used. Parameter “p” is referred to as the lag order of AR, that is, the number of lags in Y to be included in the model, “d” is the order of differencing required for making data stationary, and “q” is referred to as the order of MA, that is, the number of lagged forecast errors.
Criterions
There are several different models (i.e., based on the orders such as AR and ARMA models of certain order or different order) for building time series. The lower the value obtained using these criteria, the better suitable will be the model for our time series data. Parameters used in these criteria include—log-likelihood (L), signifying how strong the model is in fitting the data. Generally, it is considered that the more complicated the model is, the better it fits the data. It is true though, in fitting, but also leverages the concept of overfitting (i.e., the model fits the training data better but loses its ability to generalize on test data). To prevent that, the number of predictors k (i.e., number of lags (fixed amount of passing time)) plus a constant is incorporated. Another parameter to consider here is T, the number of samples or observations used for estimation.
Below are listed the criteria used in this experiment for selecting the best model.
• Akaike’s Information Criterion (AIC) – AIC is used to determine the order of an ARIMA model, and can also be used for selecting the predictors of the regression model. AIC can be calculated using the formula given below.
If T values are low, AIC may tend to predict too many predictors, so in order to prevent that bias, the corrected version of AIC, that is, AICc, will be considered.
where L is the likelihood value, p is the order of the AR model, q is the order of the MA model, k is the number of predictors, and T is the number of observations which is used for estimation as mentioned above. In order to obtain the best model, we need to consider the model with a low AIC value. That means, the value of k should be low, and the value of L should be at its maximum, illustrating that the model will be simple as k is low and fitting the data well with max l.
• Bayesian Information Criterion (BIC) – BIC, also known as Schwarz information criterion, is used for model selection based on the score obtained.
Here also, the minimum value should be taken into consideration. BIC with a small value illustrates that the model is simple, with a relatively low k number, which fits best the model and is trained on a few observations. In addition to that, other studies have revealed that the ARIMA model has strong potential for short-term prediction and can compete with existing techniques in stock price prediction (Mahan et al., 2015).
IMV-LSTM
Eventually, we will use and assess the precision of our SoA Deep Learning—especially the Interpretable Multivariable-Long Short-Term Memory neural networks (Guo et al., 2019). We use the implementation available in Pytorch4. Indeed, according to a previous study (Barić et al., 2021), it seems to be the only model with both a satisfying performance score and correct interpretability, capturing both autocorrelations and crosscorrelations between multiple time series. Interestingly, while evaluating IMV-LSTM on simulated data from statistical and mechanistic models, the correctness of interpretability increases with more complex datasets.
Other models are often used in this domain, including state-of-the-art model extensions to probabilistic forecasting such as CatBoostLSS (or Quantile Regression Forests) (Daniel, 2019) and Gaussian processes in a dynamic linear regression, as a replacement for the Kalman Filter5.
Data Availability
All data generated or analyzed during this study are included in this published article (and its repository 6).
Results
Correlation and Causation Analysis
Pearson’s Correlation Analysis
LITECOIN
All Pearson’s correlations studied with Litecoin (Supplementary File S1 and Figure 1A.) were significant with a p-value under 0.001, except the correlation between the Litecoin market cap and average Litecoin transaction value (p-value = 5.938*10^-3).
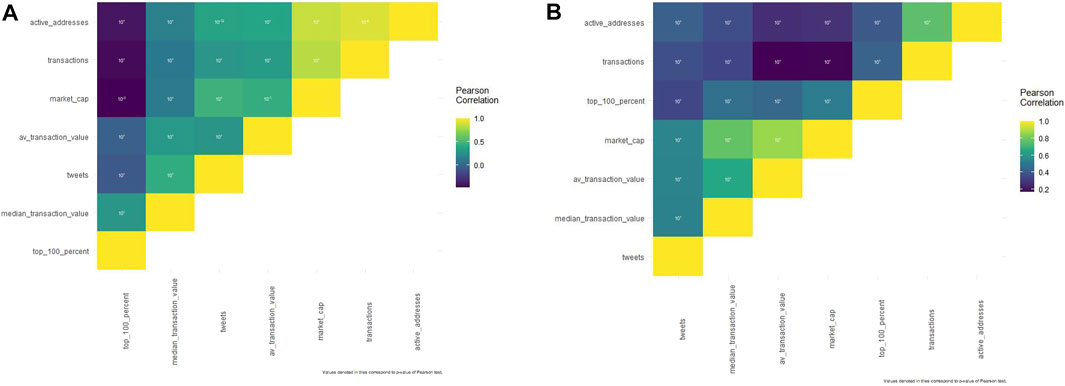
FIGURE 1. (A). Pearson’s correlation matrix concerning Dogecoin. (B). Pearson’s correlation matrix concerning Dogecoin.
Tweets have a small negative impact on Litecoin whale behavior (Pearson’s coefficient = -0.057). They are positively correlated with median Litecoin transaction value (0.449), average Litecoin transaction value (0.2944), Litecoin market cap (0.469), Litecoin transactions (0.296), and Litecoin active addresses (0.376).
Other results may seem surprising: Litecoin whales are negatively correlated with Litecoin active addresses (−0.398), transactions (−0.439), market cap (−0.466), and average Litecoin transaction value (−0.010) but not with median Litecoin transaction value (0.308).
DOGECOIN
All studied Pearson’s correlations for Dogecoin (Supplementary File S2. and Figure 1B.) were significant, with a p-value under 0.001.
Tweets are positively correlated to all economic variables with median Dogecoin transaction value (0.534), average Dogecoin transaction value (0.543), Dogecoin market cap (0.549), Dogecoin whales (0.343), Dogecoin transactions (0.376), and Dogecoin active addresses (0.430).
Dogecoin whales are positively correlated with Dogecoin active addresses (0.405), Dogecoin transactions (0.436), Dogecoin market cap (0.520), average Dogecoin transaction value (0.452), and median Dogecoin transaction value (0.476).
Mutual information theory analysis.
LITECOIN
“Association” Analysis
Community tweets are strongly (with a normalized mutual information coefficient of 0.9 at least) associated (Figure 2A and Supplementary Table S1) with all Litecoin variables but with fluctuant p-values.
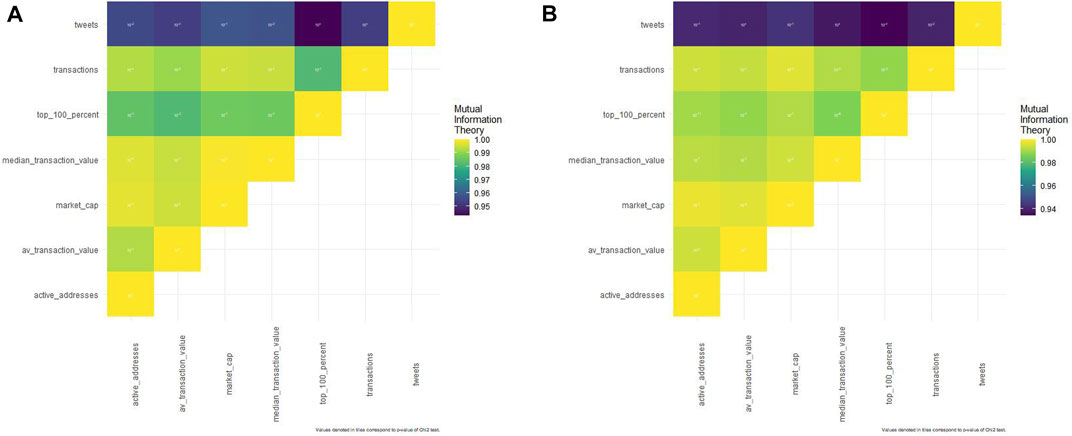
FIGURE 2. (A). Mutual information theory matrix concerning Litecoin. (B). Mutual information theory matrix concerning Dogecoin.
Indeed, only few association p-values are significant: Litecoin average transaction value with tweets (p-value = 0.0005), Litecoin average transaction value with whales (0.003), Litecoin active addresses with tweets (0.03), Litecoin transactions with tweets (0), Litecoin transactions with Litecoin active addresses (0.0005), and Litecoin transactions with Litecoin average transaction value (0.016).
“Causality” Analysis
We will explore and emphasize only significant association causality (previously described).
About tweet association with the economical trackers, tweet number was impacted by Litecoin transactions [conditional information entropy of tweets given Litecoin transactions (0.637) is higher than the conditional information entropy of Litecoin transactions given tweets (0.070) as Supplementary Table S1 shows] and tweet number was impacted by the Litecoin average transaction value too [conditional information entropy of tweets given Litecoin average transaction value (0.637) is higher than the conditional information entropy of Litecoin average transaction value given tweets (0.071)].
When looking at other associations, we can see that Litecoin active addresses are impacted by Litecoin transactions [conditional information entropy of Litecoin active addresses given Litecoin transactions (0.071) is higher than the conditional information entropy of Litecoin transactions given Litecoin active addresses (0.037)] and Litecoin transactions are impacted by Litecoin average transaction value [conditional information entropy of Litecoin transactions given Litecoin average transaction value (0.071) is higher than the conditional information entropy of Litecoin average transaction value given Litecoin transactions (0.070)].
DOGECOIN
“Association” Analysis
Community tweets are strongly (with a normalized mutual information coefficient of 0.9 at least) linked (Figure2B and Supplementary Table S2) to all Dogecoin variables but with fluctuant p-values.
There are also some significant p-values (under 0.05) but only with specific associations: Dogecoin transactions with median Dogecoin transaction value (0.03), Dogecoin transactions with Dogecoin whales (0.003), average Dogecoin transaction value with Dogecoin market cap (0.011), average Dogecoin transaction value with tweets (0), Dogecoin whales with Dogecoin active addresses (3.41*10^-11), and Dogecoin whales with tweets (3.22 * 10^-4).
“Causality” Analysis
We will explore and emphasize only significant association causality (previously described).
When looking at associations between tweets and Dogecoin economical trackers, we notice that the average Dogecoin transaction value is impacted by tweets [conditional information entropy of average Dogecoin transaction value given tweets (0.861) is higher than the conditional information entropy of tweets given average Dogecoin transaction value (0.048)] and tweets are impacted by Dogecoin whales [conditional information entropy of tweets given Dogecoin whales (0.861) is higher than the conditional information entropy of Dogecoin whales given tweets (0.124)].
About other associations, mainly looking at whales; Dogecoin active addresses are impacted by Dogecoin whales [conditional information entropy of Dogecoin active addresses given Dogecoin whales (0.120) is higher than the conditional information entropy of Dogecoin whales given Dogecoin active addresses (0.029)]; Dogecoin whales are impacted by Dogecoin transactions [conditional information entropy of Dogecoin whales given Dogecoin transactions (0.124) is higher than the conditional information entropy of Dogecoin transactions given Dogecoin whales (0.049)].
As for other associations, Dogecoin transactions impact the median Dogecoin transaction value [conditional information entropy of median Dogecoin transaction value given Dogecoin transactions (0.078) is higher than the conditional information entropy of Dogecoin transactions given median Dogecoin transaction value (0.049)]; and average Dogecoin transaction value is impacted by Dogecoin market cap [conditional information entropy of average Dogecoin transaction value given Dogecoin market cap (0.047) is higher than the conditional information entropy of Dogecoin market cap given average Dogecoin transaction value (0.001)].
Price Prediction Model
Considering historical price data of Litecoin/Dogecoin, we first analyzed the price and volume evolution over the years. Since prices data are a time series dataset, it is necessary to check this stationarity for model building.
Stationary Testing
We used the ADF test to determine the property of our time series variable. To do so, considering null and alternative hypotheses as the representation of test data can be performed using unit root or is non-stationary.
ADF statistics and p-value of time series variables were calculated. The results (Table 1) were as follows:
- Dogecoin non-stationary data show no trend in both of the three models (without a significant p-value) (Figure 3A.).
- Litecoin non-stationary data show no trend in both of the three models but with a significant p-value only in the third one (Figure 4A.).
- Dogecoin stationary data show a significant trend absence in the first model, a nonsignificant trend absence in the third model, and a nonsignificant trend presence in the second model (Figure 3B).
- Litecoin stationary data show a nonsignificant trend presence in the first model and a nonsignificant trend absence in the second and third model (Figure 4B).
To make it stationary and remove the trend, we simply applied a natural logarithm on the price values of Litecoin and Dogecoin. The values of time series before and after removing trends can be found in the table. In this way, the third model (a linear model with both drift and linear trend) was the best one to study possible trends in those data. Figure 5 illustrates the non-stationary data (without and with a square scale transformation) and stationary data price v/s time line plot studied with the third model.
Price Correlation/Causation With Number of Tweets
About Pearson’s correlation between currency prices and tweets (Figure 6A and Table 2), Dogecoin prices are weakly correlated to Dogecoin community tweets (r = 0.29), while Litecoin ones are strongly correlated to Litecoin community tweets (r = 0.86). Those results are significant because our R algorithm computed a 0 rounded p-value.
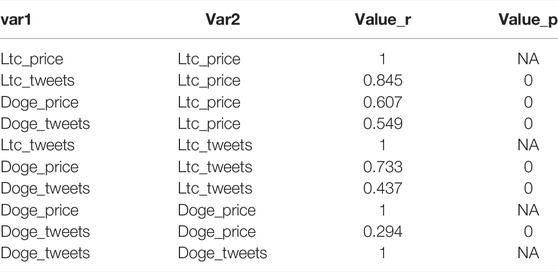
TABLE 2. Pearson’s correlation coefficients and p-value for tweets and cryptocurrency price relationship.
About the association explored with Shannon mutual information entropy (Figure 6B and Table 3), both Dogecoin and Litecoin links between their community tweet number and their price are strong (0.950 and 0.998, respectively). However, the first one is not as significant (p-value = 0.18) as the second one (p-value = 0.15).
About causality explored with Shannon mutual information entropy (Table 3):
- Dogecoin tweet number was impacting Dogecoin price. In fact, conditional information entropy of Dogecoin price given Dogecoin tweet number (0.724) is higher than conditional information entropy of Dogecoin tweet number given Dogecoin price (0.031).
- Litecoin tweet number is impacted by Litecoin price. In fact, conditional information entropy of Litecoin tweet number given Litecoin price (0.037) is higher than conditional information entropy of Litecoin price given Litecoin tweet number. (0.002).
Application
According to previous correlation/causation analysis, we could describe the relationship between currency price P and community tweet number C at a time t (Eq 8 and 9).
Then, in order to analyze this regression quality, for each cryptocurrency (Figure 7A, Figure 8A, Table 4.), we have performed ANOVA tests, compared residual data vs. fitted data, computed residual-scale location, performed normality verification by comparing the quantiles of the population with those of the normal law, and compared residual data vs. leverage data.
As the Pr (>F) is always under the alpha threshold (0.05), we reject the null hypothesis and therefore, non-null trends exist for both Litecoin and Dogecoin price. Furthermore, concerning residual data vs. fitted data studies, points are distributed randomly around the horizontal axis y = 0 and show no trend. “Scale-Location” charts show slight trends which, however, are not obvious. According to “QQ-Norm” charts, residues are normally distributed. The last graph, “Residuals vs. Leverage”, highlights the importance of each point in the regression; as we can see, there is only one point in each sample with a Cook distance greater than 1 (making the data suspect with that aberrant point).
We have also identified the suspect points, which are the points whose studentized residual is greater than 2 in absolute value and/or the Cook’s distance is greater than 1 (Figure 7B, Figure 8B). In the latter case, the point contributes very/too strongly to the determination of coefficients of the model compared to others. However, there is no one-size-fits-all method for dealing with these types of stitches. Thus, a machine learning modelization, as performed then, is required.
ARIMA
As given in Methods, the ARIMA model predicts future values based on past behavior. We considered certain criteria to know the condition of our model after training and the loss of information during training and to select the best model. Minimum loss indicates better training. Choices were automatically made using the “auto.arima” function of the R forecast package (Guasti Lima and Assaf Neto, 2022).
We chose about 0.99% of total Litecoin/Dogecoin price data for training 24,482 samples that we considered to predict all past values of Litecoin/Dogecoin pricing (Figure 9). Then, we calculated the error (Eq. 10) for each predicted past value based on forecasted and actual values.
Average errors of prediction were:
-375.761% for Dogecoin non-stationary ARIMA (2,1,2)
-0.222% for Dogecoin stationary ARIMA (0,0,0)
-375.761% for Litecoin non-stationary ARIMA (1,1,2)
-0.085% for Litecoin stationary ARIMA (0,0,0)
IMV-LSTM
About the IMV-LTSM method application on the Dogecoin data (Figure 10), we have found a very weak match between forecasting and reality (e.g., the price forecasted blue curve is very distant from the real price curve in green). The tweet volume value only participated at a 45% level in the neural network price forecasting.
About its application to Litecoin data (Figure 11), this method seems very interesting. After only 166 iterations, we can capture the main trends (e.g., the price forecasted blue curve is very close to the real price curve in green). The tweet volume participated at a level of 29% in the neural network price forecasting.
Discussion
While the average Dogecoin transaction value is impacted by tweets, Litecoin’s transaction number and tweets are impacted by the average Litecoin transaction value. About whales, tweets are impacted by Dogecoin whales, but no significant relationship was found between Litecoin whales and tweets. Furthermore, the lack of association was clearly observed using one fundamental approach (mutual information theory), resting on wholly different assumptions and principles: one with classical Pearson’s correlation, the other Shannon mutual information (often used in this study field (Piškorec et al., 2014; Keskin and Aste, 2020)). Furthermore, we saw that these two approaches contradicted slightly but mainly, rather nicely, complemented each other, in that Pearson’s correlation made it possible to study the sign of the correlation (positive vs negative), while normalized mutual information made it possible to assess the association strength in a finer way, independent from the assumption of monotony required by linear correlation.
About our price study and prediction based on community tweet volume, the most accurate ARIMA models are non-seasonal and are white noise (Mahan et al., 2015). In this way, those nonstationary (e.g., with a log transformation) ARIMA (0,0,0) models are the most accurate to predict cryptocurrencies prices based on their community tweet volume. White noise is a random signal with equal intensities at every frequency and is often defined in statistics as a signal whose samples are a sequence of unrelated, random variables with no mean and limited variance. In this way, in our study, this means that prices are independent between them (confirmed by prices correlation studies). At last, we have performed further analysis based upon Interpretable Multivariable-Long Short-Term Memory neural network application. IMV-LSTM seems interesting for crypto with less volatility such as Litecoin, but ARIMA (0,0,0) seems more interesting for cryptos more volatile such as Dogecoin.
We surmised that the main limitations of our work would most likely be grounded in community size. Indeed, a larger and more active community such as that of a “memecoin” could have a greater impact than a weaker community. In addition, a qualitative impact of some specific tweets by very well-known people could be studied (in a further study) in order to better understand how Twitter may impact cryptocurrency values. In addition, we need to complete our results in the near future with a real comparison between the different causality assessment techniques and a comparison between the various financial prediction techniques (based on the same variables).
Very few studies addressing the behavioral impact on cryptocurrencies were made (Akbiyik et al., 2021; Barjašić and Antulov-Fantulin, 2020; Lansiaux et al., 2021;6; de Winter et al., 2016). Most of those look exclusively at Bitcoin (Akbiyik et al., 2021; Barjašić and Antulov-Fantulin, 2020;7; Piškorec et al., 2014; Kraaijeveld and De Smedt, 2020; Xu and Livshits, 2019; Ante, 2021; Abraham et al., 2018; Li et al., 2019; Beck et al., 2019). The last one is a comparison between Bitcoin and Dogecoin (Tandon et al., 2021); despite their main limitation, Dogecoin and Bitcoin are from different cryptocurrency generations. This study results in the unpredictability of prices when looking at tweets from a community. Therefore, we carried out the first study about behavioral impact analysis between cryptocurrencies.
Our study has, thus, proven the interest of behavioral economics applied to the cryptocurrency world like others before it (Tandon et al., 2021; Akbiyik et al., 2021; Barjašić and Antulov-Fantulin, 2020; Lansiaux et al., 2021; 4; de Winter et al., 2016; 5; Ariyo et al., 2014; Mahan et al., 2015; Piškorec et al., 2014; Kraaijeveld and De Smedt, 2020; Xu and Livshits, 2019; Ante, 2021; Abraham et al., 2018; Li et al., 2019; Beck et al., 2019). However, many things remain to be improved: the application of those models to cryptocurrencies operating under other consensus mechanisms [Proof of Stake (PoS), Delegated Proof of Stake (DPoS), Practical Byzantine Fault Tolerance (PBFT)]. The application to cryptocurrencies with a voting consensus (for such consensus, the formulation of the problem would be more meaningful, and from the point of view of applications to commodity-backed cryptocurrency, their study would be more meaningful) and the creation of an online bot to predict cryptocurrencies future prices. However, before that, we still have to carry out a sensitivity study on all causal inference models on time series data (including our methods) and on all statistical prediction models (both regressive and based on neural networks) and on various cryptocurrencies in order to choose the most accurate. This will be our next study on cryptocurrency price prediction topic.
Data Availability Statement
The datasets presented in this study can be found in online repositories. The names of the repository/repositories and accession number(s) can be found in the article/Supplementary Material.
Author Contributions
EL and NT designed and conducted the research. EL performed the statistical analysis and created the normalized information software. EL and JF wrote the first draft of the manuscript. EL and JF contributed to the writing of the manuscript. All the authors contributed to the data interpretation, revised each draft for important intellectual content, and read and approved the final manuscript.
Conflict of Interest
The authors declare that the research was conducted in the absence of any commercial or financial relationships that could be construed as a potential conflict of interest.
Publisher’s Note
All claims expressed in this article are solely those of the authors and do not necessarily represent those of their affiliated organizations, or those of the publisher, the editors, and the reviewers. Any product that may be evaluated in this article, or claim that may be made by its manufacturer, is not guaranteed or endorsed by the publisher.
Supplementary Material
The Supplementary Material for this article can be found online at: https://www.frontiersin.org/articles/10.3389/fbloc.2022.829865/full#supplementary-material
Footnotes
4https://github.com/KurochkinAlexey/IMV_LSTM.
5https://github.com/CharlesNaylor/gp_regression.
6https://github.com/edlansiaux/Behavorial-Cryptos-Study.
7https://www.rdocumentation.org/packages/forecast/versions/8.15/topics/auto.arima.
References
Abraham, J., Higdon, D., Nelson, J., and Ibarra, J. (2018). Cryptocurrency Price Prediction Using Tweet Volumes and Sentiment Analysis. SMU Data Sci. Rev. 1 (No. 3), 1.
Akbiyik, M. E., Erkul, M., Kaempf, K., Vasiliauskaite, V., and Antulov-Fantulin, N. (2021). Ask" Who", Not" what": Bitcoin Volatility Forecasting with Twitter Data. arXiv preprint arXiv:2110.14317.
Ante, L. (2021). How Elon Musk’s Twitter Activity Moves Cryptocurrency Markets. SSRN. doi:10.2139/ssrn.3778844
Ariyo, A. A., Adewumi, A. O., and Ayo, C. K. (2014). Stock Price Prediction Using the ARIMA Model. 2014 UKSim-AMSS 16th Int. Conf. Computer Model. Simulation 2014, 106–112. doi:10.1109/UKSim.2014.67
Barić, D., Fumić, P., Horvatić, D., and Lipic, T. (2021). Benchmarking Attention-Based Interpretability of Deep Learning in Multivariate Time Series Predictions. Entropy (Basel) 23, 143. doi:10.3390/e23020143
Barjašić, I., and Antulov-Fantulin, N. (2020). Time-varying Volatility in Bitcoin Market and Information Flow at Minute-Level Frequency. arXiv preprint arXiv:2004.00550.
Beck, J., Huang, R., Lindner, D., Guo, T., Ce, Z., Helbing, D., et al. (2019). “Sensing Social media Signals for Cryptocurrency News,” in Companion proceedings of the 2019 World Wide Web conference, San Francisco, USA, May 13-17, 2019 (New York, NY, US: Association for Computing Machinery), 1051–1054. doi:10.1145/3308560.3316706
Caut, J-L., Pébaÿ, P., Lansiaux, E., and Forget, J. (2021). COVID_Dept [Internet]. Available from: https://gitlab.com/covid-data-2/lockdown-and-curfew/-/blob/master/code/COVID_Dept.py (Accessed December 5, 2021).
Daniel, F. (2019). Financial Time Series Data Processing for Machine Learning. arXiv preprint arXiv:1907.03010.
de Winter, J. C. F., Gosling, S. D., and Potter, J. (2016). Comparing the Pearson and Spearman Correlation Coefficients across Distributions and Sample Sizes: A Tutorial Using Simulations and Empirical Data. Psychol. Methods 21 (3), 273–290. doi:10.1037/met0000079
Dogecoin Foundation (2021). Announcement: Re-establishing the Dogecoin Foundation. Dogecoin Foundation. Retrieved September 30, 2021.
Douziech, T. Les mécaniques du consensus [Internet]. zonebourse. 2021 [cited 2021 Dec 4]. Available from: https://www.zonebourse.com/actualite-bourse/Les-mecaniques-du-consensus–36769932/?countview=0&fbclid=IwAR2Iy174eDAU6Y6mTxO6ZP4agG5QlSfDUOzh0J4CwD77BHiOaYQiuvdsoU4 (Accessed December 5, 2021).
Ex-Googler Gives the World a Better Bitcoin (2018). Ex-Googler Gives the World a Better Bitcoin. WIRED. Archived from the original on 2018. Retrieved 2017-10-25.
Gilbert, D. (2013). "What Is Dogecoin? the Meme that Became the Hot New Virtual Currency. Int. Business Times. . Archived from the original on September 6, 2015. Retrieved December 20, 2013.
Guasti Lima, F., and Assaf Neto, A. (2022). Combining Wavelet and Kalman Filters for Financial Time Series Forecasting. J. Int. Finance Econ. 12.
Guo, T., Lin, T., and Antulov-Fantulin, N. (2019). “Exploring Interpretable Lstm Neural Networks over Multi-Variable Data,” in International conference on machine learning, Long Beach, California, 9-15 June 2019 (PMLR), 2494–2504.
Hughes, E. (1993). A Cypherpunk's Manifesto,. San Francisco, California, US: Electronic Frontier Foundation.
Keskin, Z., and Aste, T. (2020). Information-theoretic Measures for Nonlinear Causality Detection: Application to Social media Sentiment and Cryptocurrency Prices. R. Soc. Open Sci. 7, 200863. doi:10.1098/rsos.200863
Kornbrot, D. (2005). “Point Biserial Correlation,” in Encyclopedia of Statistics in Behavioral Science [Internet] (American Cancer Society). doi:10.1002/0470013192.bsa485
Kraaijeveld, O., and De Smedt, J. (2020). The Predictive Power of Public Twitter Sentiment for Forecasting Cryptocurrency Prices. J. Int. Financial Markets, Institutions Money 65, 101188. doi:10.1016/j.intfin.2020.101188
Lansiaux, E., Caut, J. L., Forget, J., and Pébaÿ, P. P. (2021): Muinther. Figshare. Software. doi:10.6084/m9.figshare.17161871.v1
Lev, J. (1949). The Point Biserial Coefficient of Correlation. Ann. Math. Stat., 20. doi:10.1214/aoms/1177730103
Li, T. R., Chamrajnagar, A. S., Fong, X. R., Rizik, N. R., and Fu, F. (2019). Sentiment-based Prediction of Alternative Cryptocurrency price Fluctuations Using Gradient Boosting Tree Model. Front. Phys. 7, 98. doi:10.3389/fphy.2019.00098
Locke, T. (2021). The Co-creator of Dogecoin Explains Why He Doesn't Plan to Return to Crypto: It's 'controlled by a Powerful Cartel of Wealthy Figures'. CNBC. Retrieved July 20, 2021.
Mahan, M. Y., Chorn, C. R., and Georgopoulos, A. P. (2015). “White Noise Test: Detecting Autocorrelation and Nonstationarities in Long Time Series after ARIMA Modeling,” in Proceedings 14th Python in Science Conference (Scipy 2015), Austin, TX, July 6-12, 2015. doi:10.25080/majora-7b98e3ed-00f
McDaid, A., Greene, D., and Hurley, N. (2011). Normalized Mutual Information to Evaluate Overlapping Community Finding Algorithms. CoRR.
Mohammed, S. A., Abu Bakar, M. A., Ariff, N. M., and Ariff, N. M. (2020). Volatility Forecasting of Financial Time Series Using Wavelet Based Exponential Generalized Autoregressive Conditional Heteroscedasticity Model. Commun. Stat. - Theor. Methods 49, 178–188. doi:10.1080/03610926.2018.1535073
Noyes, J. (2014). An Interview with the Creator of Dogecoin: The Internet's Favourite New Currency. Junkee. Junkee Media. Retrieved January 12, 2021. Jackson Palmer accidentally invented Dogecoin in early December. within the first 30 days there were over a million visitors to Dogecoin.com.
Palachy, S. (2019). Inferring Causality in Time Series Data: a Concise Review of the Major Approaches. Medium. https://towardsdatascience.com/inferring-causality-in-time-series-data-b8b75fe52c46 (Accessed December 5, 2021).
Pébaÿ, P. (2021). Bivariate-cardinalities [Internet]. NexGen Analytics. Available from: https://gitlab.com/covid-data-2/lockdown-and-curfew/-/blob/master/code/src_bivariate-cardinalities.py (Accessed December 5, 2021).
Pébaÿ, P., Caut, J-L., Lansiaux, E., and Forget, J. (2021). CF_Dept_entopy [Internet]. Available from: https://gitlab.com/covid-data-2/lockdown-and-curfew/-/blob/master/code/COVID_Dept_entropy.py (Accessed December 5, 2021).
Piškorec, M., Antulov-Fantulin, N., Novak, P. K., Mozetič, I., Grčar, M., Vodenska, I., et al. (2014). Cohesiveness in Financial News and its Relation to Market Volatility. Sci. Rep. 4, 5038. doi:10.1038/srep05038
Shannon, C. E. (1948). A Mathematical Theory of Communication. Bell Labs Tech. J. 27, 379–423. doi:10.1002/j.1538-7305.1948.tb01338.x
Shimoni, Y., Karavani, E., Ravid, S., Bak, P., Ng, T. H., and AlfordGoldschmidt, S. H. Y. (2019). An Evaluation Toolkit to Guide Model Selection and Cohort Definition in Causal Inference. arXiv preprint arXiv:1906.00442.
Taleb, N. N. (2019). Fooled by Correlation: Common Misinterpretations Social ‘Science’. Available from: https://www.academia.edu/39797871/Fooled_by_Correlation_Common_Misinterpretations_in_Social_Science_ (Accessed December 5, 2021).
Tandon, C., Revankar, S., Palivela, H., and Parihar, S. S. (2021). How Can We Predict the Impact of the Social media Messages on the Value of Cryptocurrency? Insights from Big Data Analytics. Int. J. Inf. Management Data Insights 1 (2), 100035. doi:10.1016/j.jjimei.2021.100035
Tate, R. (1954). Correlation between a Discrete and a Continuous Variable. Point-Biserial Correlation. Ann. Math. Stat., 25. doi:10.1214/aoms/1177728730
Thompson, D. C., and Pebay, P. P. (2009). “Parallel Contingency Statistics with Titan,”. SAND2013-3435 463505. Available from: https://www.osti.gov/biblio/993626 (Accessed December 5, 2021).
Wijaya, D. R., Sarno, R., and Zulaika, E. (2017). Information Quality Ratio as a Novel Metric for Mother Wavelet Selection. Chemometrics Intell. Lab. Syst. 160, 59–71. doi:10.1016/j.chemolab.2016.11.012
Xu, J., and Livshits, B. (2019). The Anatomy of a Cryptocurrency Pump-And-Dump Scheme. 28th USENIX Security Symp. (USENIX Security 19, 1609–1625. doi:10.48550/arXiv.1811.10109
Zenil, H., Soler-Toscano, F., Kiani, N., Hernandez-Orozco, S., and Rueda-Toicen, A. (2016). A Decomposition Method for Global Evaluation of Shannon Entropy and Local Estimations of Algorithmic Complexity. Entropy, 20. doi:10.3390/e20080605
Keywords: Shannon information entropy, conditional probabilities, Pearson’s correlation coefficient, Dogecoin, Litecoin, community impact, Twitter
Citation: Lansiaux E, Tchagaspanian N and Forget J (2022) Community Impact on a Cryptocurrency: Twitter Comparison Example Between Dogecoin and Litecoin. Front. Blockchain 5:829865. doi: 10.3389/fbloc.2022.829865
Received: 06 December 2021; Accepted: 17 March 2022;
Published: 19 April 2022.
Edited by:
Tomislav Lipic, Rudjer Boskovic Institute, CroatiaReviewed by:
Alexander Vladimirovich Bogdanov, Saint Petersburg State University, RussiaJiahua Xu, University College London, United Kingdom
Copyright © 2022 Lansiaux, Tchagaspanian and Forget. This is an open-access article distributed under the terms of the Creative Commons Attribution License (CC BY). The use, distribution or reproduction in other forums is permitted, provided the original author(s) and the copyright owner(s) are credited and that the original publication in this journal is cited, in accordance with accepted academic practice. No use, distribution or reproduction is permitted which does not comply with these terms.
*Correspondence: Edouard Lansiaux, edouard.lansiaux@globalvariations.com