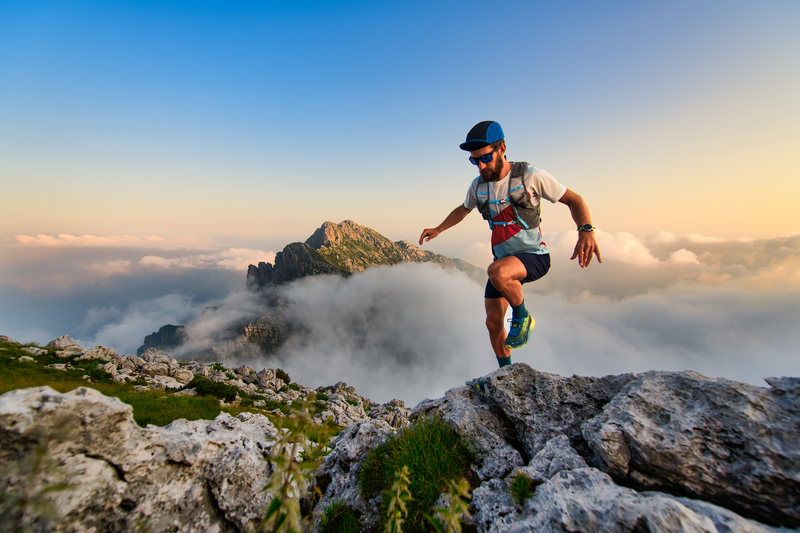
94% of researchers rate our articles as excellent or good
Learn more about the work of our research integrity team to safeguard the quality of each article we publish.
Find out more
ORIGINAL RESEARCH article
Front. Bioeng. Biotechnol. , 16 January 2024
Sec. Bioprocess Engineering
Volume 11 - 2023 | https://doi.org/10.3389/fbioe.2023.1325088
This article is part of the Research Topic Substrate-Enzyme Interactions in Lignocellulosic Biodegradation View all 6 articles
Daedaleopsis sinensis is a fungus that grows on wood and secretes a series of enzymes to degrade cellulose, hemicellulose, and lignin and cause wood rot decay. Wood-decaying fungi have ecological, economic, edible, and medicinal functions. Furthermore, the use of microorganisms to biodegrade lignocellulose has high application value. Genome sequencing has allowed microorganisms to be analyzed from the aspects of genome characteristics, genome function annotation, metabolic pathways, and comparative genomics. Subsequently, the relevant information regarding lignocellulosic degradation has been mined by bioinformatics. Here, we sequenced and analyzed the genome of D. sinensis for the first time. A 51.67-Mb genome sequence was assembled to 24 contigs, which led to the prediction of 12,153 protein-coding genes. Kyoto Encyclopedia of Genes and Genomes database analysis of the D. sinensis data revealed that 3,831 genes are involved in almost 120 metabolic pathways. According to the Carbohydrate-Active Enzyme database, 481 enzymes are found in D. sinensis, of which glycoside hydrolases are the most abundant. The genome sequence of D. sinensis provides insights into its lignocellulosic degradation and subsequent applications.
Most wood-decaying fungi are classed as basidiomycetes. Wood-decaying fungi can spread in wood through mycelia and secrete a series of enzymes to decompose the intertwined long-chain macromolecules cellulose, hemicellulose, and lignin in wood tissues (Si et al., 2013b; Zheng et al., 2016; Zheng et al., 2017; Ma et al., 2018; Si et al., 2021a; Si et al., 2021b). Simultaneously, the fungi can use the resulting starch and sugars as nourishment to meet their own growth and reproduction. Wood-decaying fungi have substantial ecological, economic, edible, and medicinal value. First, some small molecular substances degraded by wood-decaying fungi can be used for industrial production, such as bioethanol production. In addition, the enzyme system produced by wood-decaying fungi in the degradation process has a variety of applications; For example, laccase can be used to degrade pollutants, but also can synthesize antibiotics, amino acids, and other large molecular compounds. Second, many wood-decaying fungi are edible and can be used in medicine. Third, wood-decaying fungi are a vital part of the forest ecosystem and play an important role in degradation and reduction (Lonsdale et al., 2008; Krah et al., 2018; Li et al., 2022). The dead branches, fallen leaves, and decayed wood that are degraded by fungi can be returned to nature, thus participating in the material circulation and energy flow of the whole ecosystem, promoting metabolism, and maintaining dynamic balance. The wood residues degraded by brown rot fungi can remain in the soil for more than 3,000 years, which can increase soil ventilation and water retention. This process promotes the formation of ectomycorrhiza and the nitrogen-fixing ability of non-symbiotic microorganisms, as well as improving soil temperature, reducing soil pH, and increasing cation exchange in nutrients, all of which are crucial for seed germination and seedling development (Larsen et al., 1979; Larsen et al., 1982). In addition, wood-decaying fungi are closely related to other organisms that serve important ecological functions. For example, many insects and birds acquire the nutrients they need for their growth and development from wood-decaying fungi, and some of these insects also spread in spores. Therefore, wood-decaying fungi are an indispensable part of the forest ecosystem, and protecting and utilizing wood-decaying fungi is essential to protect the ecosystem and maintain ecosystem health.
Lignocellulose is composed of cellulose, hemicellulose, and lignin intertwined to form a dense network structure. Cellulose is a linear chain polysaccharide of glucose units connected by β-1,4 glucoside bonds (Liu et al., 2019; Kargar et al., 2021), and part of the linear long chain is connected by hydrogen bonds and van der Waals forces, showing a clear X-ray diffraction pattern that is called the crystallization zone. The other part of the molecular chain arrangement is an unordered, irregular, and relatively relaxed region termed the amorphous region (Zugenmaier, 2021). Cellulose is the most abundant natural polymer in the world. It is cheap, degradable, and environmentally friendly. Hemicellulose is the second type of polysaccharide in the plant fiber raw material contents. Hemicellulose is a low-molecular-weight branched polymer that is composed of two or more monosaccharides, mainly connected by β-1,4 glycosidic bonds, and has a high degree of branching and a certain degree of substitution (Sun et al., 2022; Rao et al., 2023). Lignin is a complex phenolic polymer that is renewable in nature and exists in the wood cell wall. The complex structure of lignin includes a rich variety of reactive groups, meaning this polymer can undergo various chemical reactions. Lignin can be converted into energy, chemicals, and functional materials, partially replacing fossil fuel-based products; hence, lignin is the third largest renewable biomass energy source after cellulose and hemicellulose (Liu et al., 2019; Ralph et al., 2019; Yang et al., 2020). The structural units of lignin are connected by ether and carbon-carbon bonds, forming a natural polymer with a three-dimensional structure (Sjöström, 1981; Linger et al., 2014; Sun et al., 2022). As the most abundant renewable resource on earth, lignocellulose is expected to replace fossil fuels as the main feedstock for biofuels. Prior to utilization, pretreatment is a critical step in the conversion of lignocellulose into fermentable sugars and biofuels (Wan and Li, 2012; Ponnusamy et al., 2019). Currently, white rot fungi are usually used as pretreated microorganisms, which can improve conversion efficiency through invading and destroying the protective barrier of lignocellulosic biomass feedstock with numerous advantages including high selectivity, low energy demand, nontoxic by-products, and sustainable development (Wan and Li, 2012). For pulping industry, the fungal pretreatment can significantly enhance paper strength, improve the dense energy input of mechanical pulping, and reduce the toxicity of pulping wastes (Kang et al., 2003; Rullifank et al., 2020). In conclusion, fungi play a very important role in the many industrial areas, and their genetic resources need to be further explored and developed.
Daedaleopsis sinensis is wood-decaying fungus that mainly infects the trunk of Betula and Alnus trees, is predominantly distributed in natural forests in Heilongjiang and Jilin, and causes white decay, which is common in nature (Dai, 2012). This species has coarse annual to biennial basidiocarps with large angular pores, pileate, and planar to triangular shapes (Núñez and Ryvarden, 2001; Dai and Penttilä, 2006; Li et al., 2016). Previously, several wood-decaying fungal strains with strong enzyme-secreting abilities were selected through guaiacol-containing solid plates and flask liquid cultivation, including D. sinensis used in the present study (Si et al., 2013a). Therefore, it was considered that this species has potential ability to degrade lignocellulosic materials.
Advances in DNA sequencing technologies have facilitated studies based on the genome sequences of macrofungi in addition to the continuing research on the metabolites themselves. Ganoderma lingzhi (Chen et al., 2012; Liu et al., 2012), Taiwanofungus camphoratus (Lu et al., 2014; Yang et al., 2018), Hericium erinaceus (Chen et al., 2017; Gong et al., 2020), Auricularia heimuer (Yuan et al., 2019), Russula griseocarnosa (Yu et al., 2020), and Phanerochaete chrysosporium (Martinez et al., 2004) have been used for whole genome sequencing. In this study, we sequenced the genome of D. sinensis and analyzed its functional annotation information to provide insights into secondary metabolism and carbohydrate metabolism.
The monokaryotic strain was isolated from a wild fruiting body collected from Changbai Mt., Antu County, Yanbian, Jilin Province, China. The mycelia of D. sinensis were harvested after growing on sterile cellophane covering potato dextrose agar (PDA) medium at 28°C for 10–14 days. The mycelia were collected, snap frozen in liquid nitrogen, and then stored at −80°C for further use. The enriched genomic DNA was extracted from the mycelia with a QIAGEN Genomic Kit and detected by agarose gel electrophoresis.
PacBio Sequel and MGISEQ2000 platforms were used for whole genome sequencing at Wuhan Nextomics Biosciences Co., Ltd. (Wuhan, China).
The quality of the DNA was examined using four methods: the DNA was inspected whether the appearance and shape of the sample contained foreign bodies; degradation and DNA fragment size of samples were detected by 0.75% agarose electrophoresis; DNA purity was measured by NanoDrop spectrophotometer; and precise quantification of the DNA was performed using a Qubit system.
After the samples passed the quality inspection, the genomic DNA was sheared with g-TUBEs (Covaris, United States) according to the size of the fragments built in the library, and magnetic beads were used to enrich and purify the targeted fragments of DNA. The fragmented DNA was then repaired for damage and terminal repair. Stem loop sequencing splices were connected at both ends of the DNA fragment, and exonuclease was used to remove the fragments that failed to connect. Next, BluePippin (Sage Science, United States) was used to screen the target fragments, and the library was purified. The library fragment size was then measured with an Agilent 2,100 Bioanalyzer (Agilent Technologies, United States). After construction of the library, DNA templates and enzyme complexes with a certain concentration and volume were transferred to the nanopore of the PacBio Sequel series sequencer for real-time single molecule sequencing.
Quality control was conducted on the raw sequencing data, and the low-quality areas and adapter sequences were removed to obtain high-quality DNA sequence data. Hifiasm software (parameter: −n5) was used for pure three-generation assembly to obtain the preliminary assembled genome sequence. Nextpolish software was used to calibrate the genome sequence four times with the second-generation data, and the final genome sequence was obtained.
For genome repeat sequence prediction, GMATA version 2.2 (Wang and Wang, 2016) was used to analyze the simple sequence repeats in the genome. TRF version 4.07b (Benson, 1999) was used to analyze tandem repeats in the genome with default parameters. The interspersed repetitive sequences were predicted using RepeatMasker version open-4.0.9 (Bedell et al., 2000). Three methods were employed in gene structure prediction, namely, transcriptome prediction, homologous protein prediction and de novo prediction. Based on transcriptome sequence data, genome gene prediction was performed by PASA version 2.3.3 (Haas et al., 2003). Homologous protein prediction used GeMoMa version 1.6.1 (Keilwagen et al., 2016) to compare the corresponding protein information with the genome. In addition, de novo prediction was performed using Augustus version 3.3.1 (Stanke et al., 2008). Subsequently, the gene prediction results obtained by the above three methods were integrated by using EVidenceModeler version 1.1.1 (Haas et al., 2008). Genome integrity was assessed using Benchmarking Universal Single-Copy Orthologs (BUSCO) version 3.0.1 (Simão et al., 2015).
Gene functions were predicted with references to nine databases: Kyoto Encyclopedia of Genes and Genomes (KEGG) database (https://www.kegg.jp/) to understand the higher functions and utility of cells, organisms, and ecosystems at the level of molecular information and to reveal the hidden features in biological data (Kanehisa et al., 2017), Gene Ontology (GO) database (http://www.geneontology.org) to understand the living organisms in three pathways: molecular function, cellular component, and biological process (Ashburner et al., 2000); Eukaryotic Orthologous Group of Protein (KOG) database (https://www.creative-proteomics.com/services/kog-annotation-analysis-service.htm), a eukaryote-specific version of the Clusters of Orthologous Groups tool for identifying the ortholog and paralog proteins (Galperin et al., 2015); Non-Redundant Protein (NR) database (https://www.ncbi.nlm.nih.gov/protein/), a non-redundant protein library for protein function annotation; Fungal Cytochrome P450 (CYP) database (http://p450.riceblast.snu.ac.kr/cyp.php) and CYP Engineering database (http://www.cyped.uni-stuttgart.de) to classify and analyze the CYP monooxygenase family for a better understanding of their biochemical characteristics and sequence-structure-function relationships (Sirim et al., 2009; Moktali et al., 2012); Swiss-Prot database (https://www.uniprot.org/), a protein sequence library designed to provide the high levels of annotation, minimal redundancy, and integration with other databases (Stanke and Waack, 2004; Stanke et al., 2006); Pfam database (http://pfam.xfam.org/), an aggregation of protein families including comparative sequences, species information, and hidden Markov models corresponding to special gene families (Mistry et al., 2021); Carbohydrate-Active Enzyme (CAZyme) database (http://www.cazy.org/) (Drula et al., 2022), a special database dedicated to presentation and analysis of genomic, structural, and biochemical information on CAZymes. CAZyme database classifies CAZymes into different protein families based on the similarity of amino acid sequences in the protein domain, which covers related enzymes required for lignocellulosic degradation. At present, the CAZyme database is an important reference for annotating CAZymes in most microbial genomes or metagenomes (Cantarel et al., 2009; Zhu et al., 2016; Busk et al., 2017; Helbert et al., 2019).
All predicted coding genes were aligned with these nine databases with E-value cut-offs ≤1 × 10−5, identity ≥40%, and coverage >30%.
The gene clusters of secondary metabolites were predicted using antiSMASH version 7.0.1 with default parameters (Blin et al., 2023).
To explore the evolutionary dynamics of D. sinensis, the genome sequences of 16 additional fungal species were downloaded from the National Center for Biotechnology Information (NCBI) (https://www.ncbi.nlm.nih.gov/genbank/) for phylogenomic analysis.
Single-copy orthologous genes from the 17 fungal species were inferred using OrthoFinder version 2.5.4 (Emms and Kelly, 2019) with the mafft option for subsequent multiple sequence alignment. On the basis of the resulting alignment, a maximum-likelihood tree was reconstructed using RAxML version 8.2.12 (Stamatakis et al., 2008). In addition, Timetree was used to calculate the divergence time among the 17 fungal species (Kumar et al., 2022).
Expansion and contraction of gene families were determined using CAFE version 4.2.1 (Han et al., 2013) with the following parameters: a cut-off p-value of 0.05; number of random samples = 1,000; the lambda value to calculate birth and death rates.
The internal transcribed spacer (ITS) sequences in Daedaleopsis species were aligned using ClustalX 1.83 (Chenna et al., 2003) and optimized manually using BioEdit 7.0.5.3 (Hall, 1999) prior to phylogenetic analysis. Thereafter, maximum parsimony (MP) bootstrap analysis was performed using PAUP* version 4.0 beta 10 to reveal the phylogenetics of Daedaleopsis species (Swofford, 2002). ITS sequence of D. sinensis was extracted as 559 bp from its genome data and then compared with other ten species in the same genus. At the same time, Earliella scabrosa was selected as an outgroup to construct the evolutionary tree (Li et al., 2016).
To explore the dynamics of speciation in D. sinensis, the genome sequences of D. sinensis and D. nitida were aligned in pairs using MCScanX (Wang et al., 2012) and NGenomeSyn (He et al., 2023).
In addition, the numbers of genes encoding various families of CAZymes in D. sinensis and D. nitida were clustered in a heatmap using TBtools version 1.127 (Chen et al., 2020) with the log-scale option.
Strain was identified as D. sinensis based on internal transcribed spacer-barcoding sequences. Figure 1 shows that the species used in the present study clustered with D. sinensis JX569732 and KU892444. Only one base difference from D. sinensis JX569732 and two base differences from D. sinensis KU892444, further confirming the strain used is D. sinensis based on phylogenetics.
FIGURE 1. Phylogeny of Daedaleopsis constructed by maximum parsimony analysis based on internal transcribed spacer sequences.
The 51.67 Mb genome sequence was assembled from 6,113 Mb raw data (106 × genome coverage) and to 24 contigs with a GC content of 56.46% (Table 1). Of the 24 contigs, the longest one was 5.41 Mb, whereas the N50 length was 4.40 Mb (Table 2). The GC skew did not present an obvious distribution pattern in the whole genome (Figure 2). A K-mer analysis with a depth of 34 showed a 1.60% heterozygous rate of the genome. Collectively, the above information indicates the high quality of the genome sequence assembly of D. sinensis.
FIGURE 2. Characteristics of the de novo assembly genomic features of Daedaleopsis sinensis. From outside to inside: (1) Contigs (>2 Mb in length); (2) Gene density, a heat map was used to show the relative number of bases contained in genes; (3) GC content, calculated as the percentage of G + C in 1 kb non-overlapping windows. The inward blue part represents the GC content that is lower than the average genome GC content, whereas the outward red part represents the opposite; (4) GC skew, calculated as the percentage of (G−C)/(G + C) in 1 kb non-overlapping windows. The inward orange part represents (G−C)/(G + C) < 0, and the outward green part represents (G−C)/(G + C) > 0; (5) Genome duplication, the regions where sequence similarity exists are connected by purple lines.
A total of 12,153 protein-coding genes were predicted with an average gene length of 2,420.31 bp and an average coding sequences (CDS) length of 1,476.57 bp, whereas 280 non-coding RNAs (ncRNAs) were predicted, accounting for 23.93% of the whole genome sequence. The total length of repetitive sequences was 7.62 Mb (Table 2) with the tandem repeat sequences and the interspersed repeated sequences accounting for 7.73% and 12.11%, respectively, of the whole genome sequence. The length of the transposable elements was 4.60 Mb with long terminal repeats (LTR) and non-LTR accounting for 7.71% and 1.19%, respectively, of the whole genome sequence. Assessment of genome integrity using BUSCO showed 97.36% of complete genome, indicating that the vast majority of conserved genes were predicted to be relatively complete and implying that there was high confidence in the genome assembly and prediction.
The annotation of genes using the KEGG pathway database can expand understanding of the biological functions and interactions of genes. A total of 3,831 annotated genes had matched in the KEGG database and were assigned into five levels. Of the five main levels, metabolism was the largest (2,400 genes, 48.40%), followed by genetic information processing (826 genes, 16.66%), organismal systems (737 genes, 14.86%), cellular processes (665 genes, 13.41%), and environmental information processing (331 genes, 6.67%). Thus, there were genes corresponding to significant metabolic processes. Many pathways related to lignocellulosic degradation and some key enzymes secreted by D. sinensis were identified in the KEGG database.
Regarding the metabolism and biosynthesis of sugar-containing compounds, 22 enzymes encoded by 43 genes and involved in the biosynthesis of polysaccharides (starch and sucrose metabolism) were identified from D. sinensis (Supplementary Figure S1; Supplementary Table S1). Most of these enzymes were encoded by single- or double-copy genes, whereas β-glucosidase and cellulose 1,4-β-cellobiosidase were encoded by eight- and four-copy genes, respectively. In the glycolysis/gluconeogenesis pathway (Supplementary Figure S2; Supplementary Table S2), there were 24 enzymes encoded by 35 genes, some of which had double or multiple copies. Seventeen key enzymes involved in terpenoid backbone biosynthesis via the mevalonate pathway were identified from D. sinensis (Supplementary Figure S3). Of these 17 key enzymes, protein-S-isoprenylcysteine O-methyltransferase was encoded by two copies of the genes, whereas the remaining enzymes were each encoded by a single gene (Supplementary Table S3). Many genes involved in phenylalanine metabolism, benzoate degradation, toluene degradation, pyruvate metabolism, galactose metabolism, fatty acid degradation, and aminobenzoate degradation were identified in D. sinensis. For example, 24 key enzymes involved in the pyruvate metabolism pathway were identified (Supplementary Figure S4; Supplementary Table S4), with 37 genes encoding these enzymes, and 11 key enzymes involved in the phenylalanine metabolism pathway were identified, with 24 genes encoding these enzymes (Supplementary Figure S5; Supplementary Table S5).
The total number of genes encoding CAZymes among the two species of Daedaleopsis was more or less similar, as was the number of genes encoding each of the six classes of CAZymes (Table 2). Only a few differences could be concluded at the level of gene families encoding CAZymes (Figure 3). According to the number of genes belonging to different families of CAZymes, D. sinensis has a similar strategy to D. nitida for the utilization of woody substrates. From D. sinensis, 481 genes were assigned to the six classes of CAZymes, including 7 genes encoding carbohydrate-binding modules (CBMs), 33 carbohydrate esterases (CEs), 237 glycoside hydrolases (GHs), 73 glycosyltransferases (GTs), 18 polysaccharide lyases (PLs), and 113 auxiliary activities (AAs) (Table 2). Among the six classes, GHs were the dominant group (encoded by the most genes) and were mainly involved in the degradation of celluloses (GH1, GH3, GH5, GH6, GH7, GH9, GH12, GH51, and GH74), hemicelluloses (GH3, GH10, GH30, GH43, and GH51), pectins (GH28), chitins (GH18), and starches (GH31). The families each encoded by ten or more genes included AA2 (15), AA3 (29), AA5 (13), AA7 (20), AA9 (19), CE16 (13), GH16 (30), GH18 (21), GH28 (11), GH43 (10), GH5 (22), GH79 (12), and GT2 (20). The types and quantities of enzymes found in D. nitida were similar to those found in D. sinensis (Table 2; Supplementary Table S6).
FIGURE 3. Heatmap of CAZymes classification statistics in Daedaleopsis sinensis and D. nitida. The x and y-axes represent species and CAZyme families, respectively. Rectangular blocks are colored with the logarithmic value of the number of genes encoding the CAZyme families; the color from dark red to dark blue indicates the increase in gene numbers.
CYPs play a variety of roles in fungi, including detoxification, heterobiomass degradation, and biosynthesis of secondary metabolites. Genes encoding CYPs comprise 4.3% of the CDS of the genome. After comparison with the CYP database, 524 genes encoding 39 CYPs were identified in D. sinensis (Table 2; Figure 4). In D. nitida, 40 CYPs encoded by 992 genes were identified. The most abundant CYP families in D. sinensis were CYP51, CYP620, CYP53, and CYP4.
FIGURE 4. CYPs statistics in Daedaleopsis sinensis and D. nitida. The x and y-axes represent species and CYP families, respectively. The rounded rectangular blocks are colored with the logarithmic values of the number of genes encoding the CYPs; the color from red to yellow indicates the increases in gene numbers.
A total of 44 gene clusters were predicted from D. sinensis. Of them, 23 encode terpene synthases (TS), 3 encode iterative type I polyketide synthases (T1PKS), 2 encode nonribosomal peptide synthetases (NRPS), 11 encode NRPS-like fragment, and 5 encode fungal unspecified ribosomally synthesized and post-translationally modified peptide product (fungal-RiPP-like) (Table 2). In its congeneric species D. nitida, 44 secondary metabolite clusters were predicted, of which 21 were TS, 4 were T1PKS, and the rest were the same as D. sinensis.
A total of 2,260 orthologous groups, including 1,402 single-copy genes, were identified from all 17 studied fungal species. The phylogenomic tree inferred from an alignment of the 1,402 single-copy orthologous genes with 530,910 characters of amino acid residues delimited the phylogenetic relationships among the 17 species with full bootstrap support (Figure 5).
FIGURE 5. Maximum clade credibility tree inferred from 1,402 single-copy orthologous genes. All nodes received full bootstrap support. The divergence time is labeled as the mean crown age for each node, and the 95% highest posterior density is also given within the Daedaleopsis clade. The numbers of gene family expansions and contractions in each species are labeled after plus (red) and minus (blue) symbols, respectively.
Besides two species in Daedaleopsis, other white rot fungal species, viz. Trametes versicolor, Fomes fomentarius, Ganoderma sinense, Irpex rosettiformis, Pleurotus ostreatus, and P. chrysosporium, were also phylogenetically separated. Of the species in Daedaleopsis, D. sinensis and D. nitida, which occurred in a mean crown age of 28.77 Mya with a 95% highest posterior density of 21.22–36.67 Mya, had a close phylogenetic relationship. This is also the first time that the pairwise divergence time between these two species has been given. In the evolutionary process of the 17 sampled fungal species, gene family contraction occurred more commonly than gene family expansion (Figure 5). Regarding Daedaleopsis, 546 and 845 gene families had expanded in D. sinensis and D. nitida, respectively, whereas 1,348 and 573gene families had contracted in D. sinensis and D. nitida, respectively. Of these gene families, 46 (10 expanded and 36 contracted), and 59 (52 expanded and 7 contracted) in D. sinensis and D. nitida, respectively, experienced a rapid evolution.
Arrangement of the homologous genes or sequences between contigs >2.5 Mb in D. sinensis and contigs >0.6 Mb in D. nitida (Figure 6). Figure 6 shows only the connecting lines with similarity >5,000. Comparisons of homology allow for the study of evolutionary relationships between the two species.
FIGURE 6. Distribution and arrangement of homologous genes between Daedaleopsis sinensis and D. nitida.
Using the KOG database, 3,367 (27.71%) genes in D. sinensis were assigned to KOG categories (Figure 7). The most gene-rich KOG classifications were “general function prediction only,” “posttranslational modification, protein turnover, chaperones,” “secondary metabolites biosynthesis, transport, and catabolism,” and “signal transduction mechanisms.”
According to the GO database, 5,612 genes that accounted for 46.18% of the entire genome were distributed in the three functional categories of biological process, cellular components, and molecular function (Figure 8). Among biological processes, 17,835 genes were involved in “metabolic activities,” followed by “cellular process” (7,116 genes), “single-organism process” (2,627 genes), and “biological regulation” (1,617 genes) functions. Of the genes with products predicted to be involved in molecular function, 9,380, 2,407, and 329 were involved in binding, catalytic activity, and transporter activity, respectively. For cellular components, 3,224, 2,389, 1,996, and 1,029 genes were involved in the formation process of cell part, organelle, cell, and membrane, respectively.
In the two species of Daedaleopsis, the number of gene clusters involved in the synthesis of NRPS, NRPS-like, fungal-RiPP-like, and β-lactone was similar, and D. sinensis had the highest and the lowest numbers of gene clusters, which were involved in the syntheses of terpene and β-lactone, respectively (Table 2). In D. sinensis, there were 24 gene clusters encoding terpenes, which are key biosynthetic enzymes; 3 gene clusters encoding T1PKS, which are associated with the biosynthesis of polyketides; and 2 gene clusters encoding NRPSs, which are modular enzymes.
The genome sequencing and annotation of D. sinensis are crucial for its function and comparative genomics research. Following accurate species identification, this study presented the first whole genome sequencing of D. sinensis. Information on de novo genome assembly and characterization of D. sinensis is summarized in Table 1.
The most abundant CYP families in D. sinensis are CYP51, CYP620, CYP53, CYP4, CYP504, CYP505, CYP102, and CYP125. CYP51 is a structurally and functionally conserved fungal P450 family (Yu et al., 2020), and is widely distributed in different biological kingdoms, being found in animals, plants, fungi, yeast, lower eukaryotes, and bacteria (Yoshida et al., 2000; Lepesheva and Waterman, 2004). CYP51 is a key enzyme in biosterol synthesis (Yoshida et al., 2000) and can catalyze the 14α-methyl hydroxylation of the precursor of sterol (Waterman and Lepesheva, 2005; Lepesheva and Waterman, 2007). CYP51 has become an important target for cholesterol-lowering drugs, antifungal drugs, and herbicides (Lepesheva and Waterman, 2004). Genes in the CYP53 family are involved in degradation or detoxification of benzoate and its derivatives (Crešnar and Petrič, 2011; Yu et al., 2020). CYP505 genes are involved in ω-1 to ω-3 carbon hydroxylation of fatty acids (Nakayama et al., 1996; Bao et al., 2013). Search on biosynthesis of secondary metabolites showed that the CYP620 family is related to “carbohydrate metabolism-amino sugar and nucleotide sugar metabolism” and may play a role in terpenoid synthases (Yu et al., 2020). CYP504 family members are linked to the degradation of phenylacetate and its derivatives (Ferrer-Sevillano and Fernández-Cañón, 2007; Crešnar and Petrič, 2011).
According to the CAZyme database, GH genes involved in the degradation of glucoside bonds between sugars and sugars or between sugars and non-sugar groups were the most abundant CAZyme groups in D. sinensis, accounting for 49.27% of the total CAZyme gene sequences (Table 2). The second largest group with a proportion of 23.49% was the coenzyme family enzymes (AAs), which are involved in other CAZymes (some families are also involved in the degradation of lignin). Genes encoding GTs, which catalyze the biosynthesis of monosaccharides, disaccharides, oligosaccharides, and polysaccharides, accounted for 15.18% of the total CAZyme gene sequences in D. sinensis. CEs, which are responsible for sugar modification, and CBMs, which promote anchoring of lignocellulose-degrading enzymes to the corresponding substrate, accounted for 6.86% and 1.46%, respectively. The proportion of PLs, which are involved in the cleavage of polysaccharide aldehyde polyglycan chains, was 3.74%. In summary, the cumulative proportion of GH and PL genes responsible for the degradation of carbohydrates in D. sinensis was as high as 53.01%, and this reflects, to some extent, the ability of D. sinensis to degrade lignocellulose.
Cellulose is a linear polymer composed of 500–15,000 glucose molecules linked via β-1,4-glucoside bonds. Due to it comprising the largest proportion of lignocellulosic biomass (approximately 45% of dry weight), cellulose is considered the most valuable part of lignocellulosic biomass. The complete hydrolysis of cellulose is accomplished by the synergistic action of three different types of cellulases: endo-1,4-β-glucanases (EC 3.2.1.4), exo-1,4-β-glucanases (EC 3.3.1.91), and β-glucosidases (EC 3.2.1.21) (Zhou et al., 2017). Cellulose-degrading enzymes typically contain one or more CBMs (Arfi et al., 2014). In the CAZyme database, the most of these enzymes belong to three families: GH3, GH5, and GH9. Endoglucanase hydrolyzes the internal glycosidic bonds in the cellulose chain, releasing glucose, cellobiose, oligosaccharides, and other products, while exobiose hydrolase attacks the highly ordered crystalline and amorphous regions of the fiber, and subsequently releases cellobiose. The cellobiose and oligosaccharides released by these two enzymes are further hydrolyzed to glucose by β-glycosidases from the GH1, GH3, GH5, GH9, and GH116 families. Enzymes from the GH3 family can remove glycosylated residues from the non-reducing end of carbohydrates and the side chain of hemicellulose and convert them into small substrates, releasing oligosaccharides and monosaccharides that can provide energy for the growth of host microorganisms (Harvey et al., 2000; Lacerda Júnior et al., 2017). The number of the genes of GH5 and GH12 families in D. sinensis was higher in the GHs families (Figure 3), therefore the activity of endoglucanase may be stronger, along with its ability to degrade the amorphous region of cellulose. GH5 family is the most conserved cellulase family, also known as “cellulase family A” (Henrissat et al., 1989), and can hydrolyze glycosidic bonds through an acid/base retention mechanism. Its tertiary structure presents a a (β/α)8 barrel-folded conformation. The members in GH5 family have a wide range of substrate specificity, conferring their extensive industrial potential including alteration of the taste of fruits and vegetables (Marlatt et al., 1992), improvement of the aroma of wine (Caldini et al., 1994), and enhancement the ability of animals to digest and absorb feeds (Bedford and Classen, 1992). GH12 has the smallest molecular weight in the GH family, with catalytic domain but without CBM region. Amino acid triplet structures are found in most enzymes of the GH12 family, which make up for the absence of CBM region to some extent (Prates et al., 2013). Many glycoside hydrolases in GH12 family have been used in transglycosylation for enzymatic synthesis of macromolecular materials as well as bio-polishment and decolorization to improve the softness and surface appearance of cotton fabrics (Gusakov et al., 2001; Kobayashi et al., 2010; Tanaka et al., 2010). Obviously, the large numbers of GH5 and GH12 genes in D. sinensis also suggested potential industrial values of this species. The number of GH1 and GH3 genes was relatively higher among the genes encoding CAZymes in D. sinensis (Figure 3). Consequently, the activity of β-1,4-glucosidase in D. sinensis is relatively strong, and the decomposition ability of the cellulose crystallization region is fascinating. Similar to other wood-decaying fungi, the degradation of cellulose by D. sinensis mainly depends on the synergistic interaction between different enzyme systems. In general, D. sinensis has a strong ability to degrade cellulose.
Hemicellulose has a complex structure, among which xylan and mannan are the most abundant constituents (Dhawan and Kaur, 2007). The enzymes involved in xylan and mannan hydrolysis mainly include endo-β-1,4-xylanases, β-1,4-xylosidases, and exo-β-1,4-glucanases. The complex structure of hemicellulose means that the synergistic participation of GHs and CEs is required for complete degradation of the plant polysaccharide. GH43, GH10, and GH78 families are the main families containing hemicellulases, among which GH43 family is the most abundant. The enzymes of this family usually have a variety of hemicellulolytic activities and can generally degrade xylan and araban, which could be applied in starch processing, bioenergy manufacturing, feed enzyme preparation, wine production, and pharmaceutical synthesis (Grootaert et al., 2007; Ferrer et al., 2012). In D. sinensis, the number of genes encoding GH43 family enzymes was also relatively high. β-xylosidase, which is a key enzyme in xylan degradation, has a crucial role in the conversion of hemicellulose substrates and important application potential in substrate recognition and lignocellulosic resource conversion (Lagaert et al., 2014; Rohman et al., 2019). The reported β-xylosidases from fungi are predominantly concentrated in the GH3 and GH43 families, with those from filamentous fungi mainly found in the GH3 family (Lagaert et al., 2014; Rohman et al., 2019). Notably, there were more genes encoding GH3 family enzymes in D. sinensis. In addition, CEs can degrade hemicellulose by catalyzing the hydrolysis of acetyl groups on xylans (Armendáriz-Ruiz et al., 2018). Two genes encoding glucuronyl esterase (CE15) were identified in D. sinensis. A complex cross-linking structure exists between lignin and hemicellulose through ester and ether bonds (Balakshin et al., 2011). Glucuronic acid (GlcA) is methylated to 4-O-methyl-D-glucuronic acid (MeGlcA). MeGlcA is linked to xylan via 1–2 on one side, whereas on the other side its carboxyl group forms an ester bond with the alcohol hydroxyl group of lignin, which in turn forms a skeleton structure in which the cell wall becomes hardened (Spániková and Biely, 2006). CE15 can hydrolyze the ester bond between MeGlcA and lignin (Monrad et al., 2018). Thus, CE15 is instrumental in the dissociation of lignin from cellulose and hemicellulose. Collectively, these related enzymes provide some evidence of the ability of D. sinensis to degrade hemicellulose.
Lignin is a highly heterogeneous and resistant aromatic polymer that accounts for 10%–30% of the total lignocellulosic biomass (Wang et al., 2015). White rot fungi catalyze the initial depolymerization of lignin by secreting an array of oxidases and peroxidases that generate highly reactive and nonspecific free radicals, which in turn undergo a complex series of spontaneous cleavage reactions (Kirk and Farrell, 1987; Martinez et al., 2004). The complete degradation of lignin requires two groups of enzymes: lignin-modifying enzymes (LMEs) and lignin-degrading auxiliary enzymes (LDAs) (Janusz et al., 2017). LMEs mainly belong to the AA1 family and AA2 family in the CAZyme database. LDAs themselves cannot degrade lignin, but they can use O2 to produce H2O2, accompanied by the oxidation of aromatic alcohols and glyoxals or reduction of carbohydrates. The H2O2 produced can support LMEs or trigger non-enzymatic Fenton reactions that degrade lignin (Sweeney and Xu, 2012). AA1 enzymes are multicopper oxidases that use diphenols and related substances as donors with oxygen as the acceptor. The AA1 family is currently divided into three subfamilies: laccases, ferroxidases, and laccase-like multicopper oxidases. The AA2 family contains class II lignin-modifying peroxidases. AA2 enzymes are secreted heme-containing enzymes that use H2O2 or organic peroxides as electron acceptors to catalyze numerous oxidative reactions. In addition, AA3 enzymes are flavoproteins containing a flavin-adenine dinucleotide-binding domain. The AA3 family is one of the main families that contain lignin-degrading enzymes and belongs to the glucose-methanol-choline oxidoreductases family, which could be widely used in waste-to energy regenerating and biosensor manufacturing (Henske et al., 2018; Sützl et al., 2018; Sugano et al., 2021). AA3 is a powerful family of redox enzymes, all of whose members have similar structural characteristics, and can assist other AA family enzymes in catalysis through its reaction products or participate in the role of GHs in lignocellulose degradation. Besides, AA4, AA5, AA6, AA7, and AA9 families all have a certain amount of lignin degradation related enzymes. The abundance of enzymes encoding AA2, AA3, and AA7 families in D. sinensis is high. The above situation indicates that D. sinensis contains a large number of genes encoding lignin-degrading enzymes, reflecting the lignin-degrading ability of D. sinensis.
The modification and disassembly of pectin are executed by various hydrolytic enzymes (Mahmood et al., 2021). In the genome of D. sinensis, the number of GH28 (polygalacturonase, PG) genes encoding pectin decomposing enzyme was as high as 11, and the number of CE8 (pectin methylesterase, PME) encoding gene was only 2. Among the hydrolases, PGs are major pectin hydrolytic enzymes that catalyze the hydrolysis of the α-(1→4) and D-GlcA linkage in homogalacturonan, leading to cell separation (Ke et al., 2018). PME can hydrolyze methyl ester group in pectin, release methanol, and reduce the degree of methylation of pectin. PME is an important pectin enzyme and has a wide application prospect in food processing and paper production (Wang et al., 2020). PME and PG together can significantly enhance the clarifying effect of fruit juice (Tu et al., 2013). Other pectin decomposing enzyme genes (CE12, GH53, GH78, GH88, GH105, and PL4) were present in D. sinensis. This indicates that D. sinensis tends to hydrolyze all components in pectin.
According to the KEGG database analysis of D. sinensis data, 3,831 genes are involved in nearly 120 metabolic pathways. These pathways mainly include carbon metabolism, fatty acid biosynthesis and degradation, amino and nucleotide sugar metabolism, terpenoid metabolism, starch and sucrose metabolism, metabolism of cofactors and vitamins, methane metabolism, polycyclic aromatic hydrocarbon degradation, pyruvate metabolism, phenylalanine metabolism, and carbohydrate metabolism. Among them, the metabolism of sugar-containing compounds includes the tricarboxylic acid cycle and the classic pentose phosphate pathway. In addition, fructose, mannose, and galactose metabolism pathways are predicted. Studies have shown that the degradation of lignin by white rot fungi generally belongs to secondary metabolism, whereas the degradation of cellulose and hemicellulose exists in both primary and secondary metabolic stages (Zhao et al., 2019). The KEGG results indicate that substrate and energy metabolism and trophic growth are among the major biological processes carried out by D. sinensis, which is consistent with the reality that catabolism and trophic growth are the predominant life activities of D. sinensis itself as a degrader. The genome data suggested that D. sinensis possesses several carbon metabolic pathways and glucose metabolic pathways related to the degradation of cellulose and hemicellulose. There are 35 genes in the genome involved in glycolysis or gluconeogenesis pathways, and 43 genes involved in starch and sucrose metabolism pathways. The starch and sucrose metabolic pathways are closely related to the degradation of cellulose. In the pathways, a total of 11 genes encoding cellulase were identified. Among them, eight genes encode β-glucosidase, two genes encode exoglucanase, and one gene encodes endoglucanase. These annotated cellulase genes are involved in the transformation of cellulose into D-glucose (Supplementary Figure S1). Cellulose can be converted to cellodextrin by the action of endoglucanase, and then to cellobiose with the participation of β-glucosidase (or a combination of endoglucanase and exglucanase), which is hydrolyzed to D-glucose by the action of β-glucosidase. The benzoic acid metabolism pathway is the main metabolic pathway in the process of lignin degradation, but this has been less studied in fungi and mainly reported in bacteria (Egland et al., 1997). In D. sinensis, the pathways associated with lignin degradation include those associated with the degradation of aromatic compounds, such as phenylalanine metabolism, benzoate degradation, toluene degradation, pyruvate metabolism, galactose metabolism, fatty acid degradation, and aminobenzoate degradation.
The whole genome sequence of D. sinensis was obtained for the first time. Genomic analysis shows that potential ability of D. sinensis to degrade lignocellulosic materials. The analysis of the lignocellulose degradation capacity of D. sinensis is vital for future wide-ranging applications of this fungus. Further studies on D. sinensis should focus on its metabolic pathways as well as enzymatic analysis. In addition, subsequent transcriptome and metabolome data will further facilitate the application of D. sinensis. A comprehensive understanding of the D. sinensis genome is expected to pave the way for applications of this fungus in industrial production.
The original contributions presented in the study are publicly available. This data can be found here: https://www.ncbi.nlm.nih.gov/bioproject/PRJNA1030494/.
J-XM: Data curation, Formal Analysis, Investigation, Methodology, Writing–original draft, Writing–review and editing, Software, Visualization. HW: Investigation, Methodology, Visualization, Writing–original draft. CJ: Formal Analysis, Methodology, Software, Visualization, Writing–original draft. Y-FY: Formal Analysis, Methodology, Visualization, Writing–original draft. L-XT: Formal Analysis, Methodology, Writing–original draft. JnS: Conceptualization, Data curation, Formal Analysis, Funding acquisition, Investigation, Methodology, Project administration, Resources, Supervision, Validation, Writing–original draft, Writing–review and editing. JeS: Conceptualization, Formal Analysis, Investigation, Methodology, Software, Supervision, Validation, Writing–review and editing.
The author(s) declare financial support was received for the research, authorship, and/or publication of this article. This study was supported by the National Natural Science Foundation of China (32070016 and 32270016) and the Beijing Nova Program (20230484322).
We express our gratitude to Dr. Hai-Jiao Li (National Institute of Occupational Health and Poison Control, Chinese Center for Disease Control and Prevention, China) for sample collection and data analysis.
The authors declare that the research was conducted in the absence of any commercial or financial relationships that could be construed as a potential conflict of interest.
All claims expressed in this article are solely those of the authors and do not necessarily represent those of their affiliated organizations, or those of the publisher, the editors and the reviewers. Any product that may be evaluated in this article, or claim that may be made by its manufacturer, is not guaranteed or endorsed by the publisher.
The Supplementary Material for this article can be found online at: https://www.frontiersin.org/articles/10.3389/fbioe.2023.1325088/full#supplementary-material.
Arfi, Y., Shamshoum, M., Rogachev, I., Peleg, Y., and Bayer, E. A. (2014). Integration of bacterial lytic polysaccharide monooxygenases into designer cellulosomes promotes enhanced cellulose degradation. Proc. Natl. Acad. Sci. U. S. A. 111 (25), 9109–9114. doi:10.1073/pnas.1404148111
Armendáriz-Ruiz, M., Rodríguez-González, J. A., Camacho-Ruíz, R. M., and Mateos-Díaz, J. C. (2018). Carbohydrate esterases: an overview. Methods Mol. Biol. 1835, 39–68. doi:10.1007/978-1-4939-8672-9_2
Ashburner, M., Ball, C. A., Blake, J. A., Botstein, D., Butler, H., Cherry, J. M., et al. (2000). Gene Ontology: tool for the unification of biology. Nat. Genet. 25 (1), 25–29. doi:10.1038/75556
Balakshin, M., Capanema, E., Gracz, H., Chang, H. M., and Jameel, H. (2011). Quantification of lignin-carbohydrate linkages with high-resolution NMR spectroscopy. Planta 233 (6), 1097–1110. doi:10.1007/s00425-011-1359-2
Bao, D. P., Gong, M., Zheng, H. J., Chen, M. J., Zhang, L., Wang, H., et al. (2013). Sequencing and comparative analysis of the straw mushroom (Volvariella volvacea) genome. PLoS ONE 8 (3), e58294. doi:10.1371/journal.pone.0058294
Bedell, J. A., Korf, I., and Gish, W. (2000). MaskerAid: a performance enhancement to RepeatMasker. Bioinformatics 16 (11), 1040–1041. doi:10.1093/bioinformatics/16.11.1040
Bedford, M. R., and Classen, H. L. (1992). Reduction of intestinal viscosity through manipulation of dietary rye and pentosanase concentration is effected through changes in the carbohydrate composition of the intestinal aqueous phase and results in improved growth rate and food conversion efficiency of broiler chicks. J. Nutr. 122 (3), 560–569. doi:10.1093/jn/122.3.560
Benson, G. (1999). Tandem repeats finder: a program to analyze DNA sequences. Nucleic Acids Res. 27 (2), 573–580. doi:10.1093/nar/27.2.573
Blin, K., Shaw, S., Augustijn, H. E., Reitz, Z. L., Biermann, F., Alanjary, M., et al. (2023). antiSMASH 7.0: new and improved predictions for detection, regulation, chemical structures, and visualisation. Nucleic Acids Res. 51 (W1), W46–W50. doi:10.1093/nar/gkad344
Busk, P. K., Pilgaard, B., Lezyk, M. J., Meyer, A. S., and Lange, L. (2017). Homology to peptide pattern for annotation of carbohydrate-active enzymes and prediction of function. BMC Bioinforma. 18 (1), 214. doi:10.1186/s12859-017-1625-9
Caldini, C., Bonomi, F., Pifferi, P. G., Lanzarini, G., and Galante, Y. M. (1994). Kinetic and immobilization studies on fungal glycosidases for aroma enhancement in wine. Enzyme Microb. Technol. 16 (4), 286–291. doi:10.1016/0141-0229(94)90168-6
Cantarel, B. L., Coutinho, P. M., Rancurel, C., Bernard, T., Lombard, V., and Henrissat, B. (2009). The carbohydrate-active enZymes database (CAZy): an expert resource for glycogenomics. Nucleic Acids Res. 37, D233–D238. doi:10.1093/nar/gkn663
Chen, C. J., Chen, H., Zhang, Y., Thomas, H. R., Frank, M. H., He, Y. H., et al. (2020). TBtools: an integrative toolkit developed for interactive analyses of big biological data. Mol. Plant 13 (8), 1194–1202. doi:10.1016/j.molp.2020.06.009
Chen, J., Zeng, X., Yang, Y. L., Xing, Y. M., Zhang, Q., Li, J. M., et al. (2017). Genomic and transcriptomic analyses reveal differential regulation of diverse terpenoid and polyketides secondary metabolites in Hericium erinaceus. Sci. Rep. 7 (1), 10151. doi:10.1038/s41598-017-10376-0
Chen, S. L., Xu, J., Liu, C., Zhu, Y. J., Nelson, D. R., Zhou, S. G., et al. (2012). Genome sequence of the model medicinal mushroom Ganoderma lucidum. Nat. Commun. 3, 913. doi:10.1038/ncomms1923
Chenna, R., Sugawara, H., Koike, T., Lopez, R., Gibson, T. J., Higgins, D. G., et al. (2003). Multiple sequence alignment with the Clustal series of programs. Nucleic Acids Res. 31 (13), 3497–3500. doi:10.1093/nar/gkg500
Crešnar, B., and Petrič, S. (2011). Cytochrome P450 enzymes in the fungal kingdom. Biochim. Biophys. Acta. 1814 (1), 29–35. doi:10.1016/j.bbapap.2010.06.020
Dai, Y. C. (2012). Pathogenic wood-decaying fungi on woody plants in China. Mycosystema 31 (4), 493–509. doi:10.13346/j.mycosystema.2012.04.014
Dai, Y. C., and Penttilä, R. (2006). Polypore diversity of fenglin nature reserve, northeastern China. Ann. Bot. Fenn. 43 (2), 81–96.
Dhawan, S., and Kaur, J. (2007). Microbial mannanases: an overview of production and applications. Crit. Rev. Biotechnol. 27 (4), 197–216. doi:10.1080/07388550701775919
Drula, E., Garron, M. L., Dogan, S., Lombard, V., Henrissat, B., and Terrapon, N. (2022). The carbohydrate-active enzyme database: functions and literature. Nucleic Acids Res. 50 (D1), D571–D577. doi:10.1093/nar/gkab1045
Egland, P. G., Pelletier, D. A., Dispensa, M., Gibson, J., and Harwood, C. S. (1997). A cluster of bacterial genes for anaerobic benzene ring biodegradation. Proc. Natl. Acad. Sci. U. S. A. 94 (12), 6484–6489. doi:10.1073/pnas.94.12.6484
Emms, D. M., and Kelly, S. (2019). OrthoFinder: phylogenetic orthology inference for comparative genomics. Genome Biol. 20 (1), 238. doi:10.1186/s13059-019-1832-y
Ferrer, M., Ghazi, A., Beloqui, A., Vieites, J. M., López-Cortés, N., Marín-Navarro, J., et al. (2012). Functional metagenomics unveils a multifunctional glycosyl hydrolase from the family 43 catalysing the breakdown of plant polymers in the calf rumen. PLoS ONE 7 (6), e38134. doi:10.1371/journal.pone.0038134
Ferrer-Sevillano, F., and Fernández-Cañón, J. M. (2007). Novel phacB-encoded cytochrome P450 monooxygenase from Aspergillus nidulans with 3-hydroxyphenylacetate 6-hydroxylase and 3,4-dihydroxyphenylacetate 6-hydroxylase activities. Eukaryot. Cell 6 (3), 514–520. doi:10.1128/EC.00226-06
Galperin, M. Y., Makarova, K. S., Wolf, Y. I., and Koonin, E. V. (2015). Expanded microbial genome coverage and improved protein family annotation in the COG database. Nucleic Acids Res. 43, D261–D269. doi:10.1093/nar/gku1223
Gong, W. B., Wang, Y. H., Xie, C. L., Zhou, Y. J., Zhu, Z. H., and Peng, Y. D. (2020). Whole genome sequence of an edible and medicinal mushroom, Hericium erinaceus (Basidiomycota, Fungi). Genomics 112 (3), 2393–2399. doi:10.1016/j.ygeno.2020.01.011
Grootaert, C., Delcour, J. A., Courtin, C. M., Broekaert, W. F., Verstraete, W., and Wiele, T. V. (2007). Microbial metabolism and prebiotic potency of arabinoxylan oligosaccharides in the human intestine. Trends Food Sci. Technol. 18 (2), 64–71. doi:10.1016/j.tifs.2006.08.004
Gusakov, A. V., Sinitsyn, A. P., Markov, A. V., Sinitsyna, O. A., Ankudimova, N. V., and Berlin, A. G. (2001). Study of protein adsorption on indigo particles confirms the existence of enzyme-indigo interaction sites in cellulase molecules. J. Biotechnol. 87 (1), 83–90. doi:10.1016/s0168-1656(01)00234-6
Haas, B. J., Delcher, A. L., Mount, S. M., Wortman, J. R., Smith, R. K., Hannick, L. I., et al. (2003). Improving the Arabidopsis genome annotation using maximal transcript alignment assemblies. Nucleic Acids Res. 31 (19), 5654–5666. doi:10.1093/nar/gkg770
Haas, B. J., Salzberg, S. L., Zhu, W., Pertea, M., Allen, J. E., Orvis, J., et al. (2008). Automated eukaryotic gene structure annotation using EVidenceModeler and the program to assemble spliced alignments. Genome Biol. 9 (1), R7. doi:10.1186/gb-2008-9-1-r7
Hall, T. A. (1999). BioEdit: a user-friendly biological sequence alignment editor and analysis program for Windows 95/98/NT. Nucleic Acids Symp. Ser. 41, 95–98. doi:10.14601/Phytopathol_Mediterr-14998u1.29
Han, M. V., Thomas, G. W. C., Lugo-Martinez, J., and Hahn, M. W. (2013). Estimating gene gain and loss rates in the presence of error in genome assembly and annotation using CAFE 3. Mol. Biol. Evol. 30 (8), 1987–1997. doi:10.1093/molbev/mst100
Harvey, A. J., Hrmova, M., De Gori, R., Varghese, J. N., and Fincher, G. B. (2000). Comparative modeling of the three-dimensional structures of family 3 glycoside hydrolases. Proteins 41 (2), 257–269. doi:10.1002/1097-0134(20001101)41:2<257::aid-prot100>3.0.co;2-c
He, W. M., Yang, J., Jing, Y., Xu, L., Yu, K., and Fang, X. D. (2023). NGenomeSyn: an easy-to-use and flexible tool for publication-ready visualization of syntenic relationships across multiple genomes. Bioinformatics 39 (3), btad121. doi:10.1093/bioinformatics/btad121
Helbert, W., Poulet, L., Drouillard, S., Mathieu, S., Loiodice, M., Couturier, M., et al. (2019). Discovery of novel carbohydrate-active enzymes through the rational exploration of the protein sequences space. Proc. Natl. Acad. Sci. U. S. A. 116 (13), 6063–6068. doi:10.1073/pnas.1815791116
Henrissat, B., Claeyssens, M., Tomme, P., Lemesle, L., and Mornon, J. P. (1989). Cellulase families revealed by hydrophobic cluster analysi. Gene 81 (1), 83–95. doi:10.1016/0378-1119(89)90339-9
Henske, J. K., Springer, S. D., O'Malley, M. A., and Butler, A. (2018). Substrate-based differential expression analysis reveals control of biomass degrading enzymes in Pycnoporus cinnabarinus. Biochem. Eng. J. 130, 83–89. doi:10.1016/j.bej.2017.11.015
Janusz, G., Pawlik, A., Sulej, J., Swiderska-Burek, U., Jarosz-Wilkolazka, A., and Paszczynski, A. (2017). Lignin degradation: microorganisms, enzymes involved, genomes analysis and evolution. FEMS Microbiol. Rev. 41 (6), 941–962. doi:10.1093/femsre/fux049
Kanehisa, M., Furumichi, M., Tanabe, M., Sato, Y., and Morishima, K. (2017). KEGG: new perspectives on genomes, pathways, diseases and drugs. Nucleic Acids Res. 45 (D1), D353–D361. doi:10.1093/nar/gkw1092
Kang, K. Y., Jo, B. M., Oh, J. S., and Mansfield, S. D. (2003). The effects of biopulping on chemical and energy consumption during kraft pulping of hybrid poplar. Wood Fiber Sci. 35 (4), 594–600. doi:10.1007/s00107-003-0406-5
Kargar, F., Mortazavi, M., Maleki, M., Mahani, M. T., Ghasemi, Y., and Savardashtaki, A. (2021). Isolation, identification and in silico study of native cellulase producing bacteria. Curr. Proteomics 18 (1), 3–11. doi:10.2174/1570164617666191127142035
Ke, X. B., Wang, H. S., Li, Y., Zhu, B., Zang, Y. X., He, Y., et al. (2018). Genome-wide identification and analysis of polygalacturonase genes in Solanum lycopersicum. Int. J. Mol. Sci. 19 (8), 2290. doi:10.3390/ijms19082290
Keilwagen, J., Wenk, M., Erickson, J. L., Schattat, M. H., Grau, J., and Hartung, F. (2016). Using intron position conservation for homology-based gene prediction. Nucleic Acids Res. 44 (9), e89. doi:10.1093/nar/gkw092
Kirk, T. K., and Farrell, R. L. (1987). Enzymatic "combustion": the microbial degradation of lignin. Annu. Rev. Microbiol. 41, 465–501. doi:10.1146/annurev.mi.41.100187.002341
Kobayashi, A., Tanaka, T., Watanabe, K., Ishihara, M., Noguchi, M., Okada, H., et al. (2010). 4,6-dimethoxy-1,3,5-triazine oligoxyloglucans: novel one-step preparable substrates for studying action of endo-beta-1,4-glucanase III from Trichoderma reesei. Bioorg. Med. Chem. Lett. 20 (12), 3588–3591. doi:10.1016/j.bmcl.2010.04.122
Krah, F. S., Seibold, S., Brandl, R., Baldrian, P., Müller, J., and Bässler, C. (2018). Independent effects of host and environment on the diversity of wood-inhabiting fungi. J. Ecol. 106, 1428–1442. doi:10.1111/1365-2745.12939
Kumar, S., Suleski, M., Craig, J. M., Kasprowicz, A. E., Sanderford, M., Li, M., et al. (2022). TimeTree 5: an expanded resource for species divergence times. Mol. Biol. Evol. 39 (8), msac174. doi:10.1093/molbev/msac174
Lacerda Júnior, G. V., Noronha, M. F., de Sousa, S. T., Cabral, L., Domingos, D. F., Sáber, M. L., et al. (2017). Potential of semiarid soil from Caatinga biome as a novel source for mining lignocellulose-degrading enzymes. FEMS Microbiol. Ecol. 93 (2), fiw248. doi:10.1093/femsec/fiw248
Lagaert, S., Pollet, A., Courtin, C. M., and Volckaert, G. (2014). β-Xylosidases and α-L-arabinofuranosidases: accessory enzymes for arabinoxylan degradation. Biotechnol. Adv. 32 (2), 316–332. doi:10.1016/j.biotechadv.2013.11.005
Larsen, M. J., Jurgenson, M. F., and Harvey, A. E. (1979). N2 fixation associated with wood decayed by some common fungi in western Montana. Can. J. For. Res. 8, 341–345. doi:10.1139/x78-050
Larsen, M. J., Jurgenson, M. F., and Harvey, A. E. (1982). N2 fixation in brown-rotted soil wood in an intermountain cedar-hemlock ecosystem. For. Sci. 28, 292–296. doi:10.1093/forestscience/28.2.292
Lepesheva, G. I., and Waterman, M. R. (2004). CYP51−the omnipotent P450. Mol. Cell. Endocrinol. 215 (1-2), 165–170. doi:10.1016/j.mce.2003.11.016
Lepesheva, G. I., and Waterman, M. R. (2007). Sterol 14α-demethylase cytochrome P450 (CYP51), a P450 in all biological kingdoms. Biochim. Biophys. Acta 1770 (3), 467–477. doi:10.1016/j.bbagen.2006.07.018
Li, H. J., Si, J., and He, S. H. (2016). Daedaleopsis hainanensis sp. nov. (Polyporaceae, Basidiomycota) from tropical China based on morphological and molecular evidence. Phytotaxa 275, 294–300. doi:10.11646/phytotaxa.275.3.7
Li, T., Cui, L. Z., Song, X. F., Cui, X. Y., Wei, Y. L., Tang, L., et al. (2022). Wood decay fungi: an analysis of worldwide research. J. Soils Sediment. 22, 1688–1702. doi:10.1007/s11368-022-03225-9
Linger, J. G., Vardon, D. R., Guarnieri, M. T., Karp, E. M., Hunsinger, G. B., Franden, M. A., et al. (2014). Lignin valorization through integrated biological funneling and chemical catalysis. Proc. Natl. Acad. Sci. U. S. A. 111 (33), 12013–12018. doi:10.1073/pnas.1410657111
Liu, C., Wu, S. L., Zhang, H. Y., and Xiao, R. (2019). Catalytic oxidation of lignin to valuable biomass-based platform chemicals: a review. Fuel Process. Technol. 191, 181–201. doi:10.1016/j.fuproc.2019.04.007
Liu, D. B., Gong, J., Dai, W. K., Kang, X. C., Huang, Z., Zhang, H. M., et al. (2012). The genome of Ganoderma lucidum provide insights into triterpense biosynthesis and wood degradation. PLoS ONE 7 (5), e36146. doi:10.1371/journal.pone.0036146
Lonsdale, D., Pautasso, M., and Holdenrieder, O. (2008). Wood-decaying fungi in the forest: conservation needs and management options. Eur. J. For. Res. 127, 1–22. doi:10.1007/s10342-007-0182-6
Lu, M. Y. J., Fan, W. L., Wang, W. F., Chen, T. C., Tang, Y. C., Chu, F. H., et al. (2014). Genomic and transcriptomic analyses of the medicinal fungus Antrodia cinnamomea for its metabolite biosynthesis and sexual development. Proc. Natl. Acad. Sci. U. S. A. 111 (44), E4743–E4752. doi:10.1073/pnas.1417570111
Ma, H. F., Meng, G., Cui, B. K., Si, J., and Dai, Y. C. (2018). Chitosan crosslinked with genipin as supporting matrix for biodegradation of synthetic dyes: laccase immobilization and characterization. Chem. Eng. Res. Des. 132, 664–676. doi:10.1016/j.cherd.2018.02.008
Mahmood, U., Fan, Y. H., Wei, S. Y., Niu, Y., Li, Y. H., Huang, H. L., et al. (2021). Comprehensive analysis of polygalacturonase genes offers new insights into their origin and functional evolution in land plants. Genomics 113, 1096–1108. doi:10.1016/j.ygeno.2020.11.006
Marlatt, C., Ho, C. T., and Chien, M. (1992). Studies of aroma constituents bound as glycosides in tomato. J. Agr. Food Chem. 40 (2), 249–252. doi:10.1021/jf00014a016
Martinez, D., Larrondo, L. F., Putnam, N., Gelpke, M. D. S., Huang, K., Chapman, J., et al. (2004). Genome sequence of the lignocellulose degrading fungus Phanerochaete chrysosporium strain RP78. Nat. Biotechnol. 22, 695–700. doi:10.1038/nbt967
Mistry, J., Chuguransky, S., Williams, L., Qureshi, M., Salazar, G. A., Sonnhammer, E. L. L., et al. (2021). Pfam: the protein families database in 2021. Nucleic Acids Res. 49 (D1), D412–D419. doi:10.1093/nar/gkaa913
Moktali, V., Park, J., Fedorova-Abrams, N. D., Park, B., Choi, J., Lee, Y. H., et al. (2012). Systematic and searchable classification of cytochrome P450 proteins encoded by fungal and oomycete genomes. BMC Genomics 13, 525. doi:10.1186/1471-2164-13-525
Monrad, R. N., Eklöf, J., Krogh, K. B. R. M., and Biely, P. (2018). Glucuronoyl esterases: diversity, properties and biotechnological potential. A review. Crit. Rev. Biotechnol. 38 (7), 1121–1136. doi:10.1080/07388551.2018.1468316
Nakayama, N., Takemae, A., and Shoun, H. (1996). Cytochrome P450foxy, a catalytically self-sufficient fatty acid hydroxylase of the fungus Fusarium oxysporum. J. Biochem. 119 (3), 435–440. doi:10.1093/oxfordjournals.jbchem.a021260
Núñez, M., and Ryvarden, L. (2001). East Asian polypores 2. Polyporaceae s. lato. Synop. Fungorum 14, 170–522.
Ponnusamy, V. K., Nguyen, D. D., Dharmaraja, J., Shobana, S., Banu, J. R., Saratale, R. G., et al. (2019). A review on lignin structure, pretreatments, fermentation reactions and biorefinery potential. Bioresour. Technol. 271, 462–472. doi:10.1016/j.biortech.2018.09.070
Prates, E. T., Stanković, I. M., Silveira, R. L., Liberato, M. V., Henrique-Silva, F., Pereira, N., et al. (2013). X-ray structure and molecular dynamics simulations of endoglucanase 3 from Trichoderma harzianum: structural organization and substrate recognition by endoglucanases that lack cellulose binding module. PLoS ONE 8 (3), e59069. doi:10.1371/journal.pone.0059069
Ralph, J., Lapierre, C., and Boerjan, W. (2019). Lignin structure and its engineering. Curr. Opin. Biotechnol. 56, 240–249. doi:10.1016/j.copbio.2019.02.019
Rao, J., Lv, Z. W., Chen, G. G., and Peng, F. (2023). Hemicellulose: structure, chemical modification, and application. Prog. Polym. Sci. 140, 101675. doi:10.1016/j.progpolymsci.2023.101675
Rohman, A., Dijkstra, B. W., and Puspaningsih, N. N. T. (2019). β-Xylosidases: structural diversity, catalytic mechanism, and inhibition by monosaccharides. Int. J. Mol. Sci. 20 (22), 5524. doi:10.3390/ijms20225524
Rullifank, K. F., Roefinal, M. E., Kostanti, M., Sartika, L., and Evelyn, E. (2020). Pulp and paper industry: an overview on pulping technologies, factors, and challenges. IOP Conf. Ser. Mater. Sci. Eng. 845, 012005. doi:10.1088/1757-899x/845/1/012005
Si, J., Cui, B. K., and Dai, Y. C. (2013a). Decolorization of chemically different dyes by white-rot fungi in submerged cultures. Ann. Microbiol. 63 (3), 1099–1108. doi:10.1007/s13213-012-0567-8
Si, J., Ma, H. F., Cao, Y. J., Cui, B. K., and Dai, Y. C. (2021a). Introducing a thermo-alkali-stable, metallic ion-tolerant laccase purified from white rot fungus Trametes hirsuta. Front. Microbiol. 12, 670163. doi:10.3389/fmicb.2021.670163
Si, J., Peng, F., and Cui, B. K. (2013b). Purification, biochemical characterization and dye decolorization capacity of an alkali-resistant and metal-tolerant laccase from Trametes pubescens. Bioresour. Technol. 128, 49–57. doi:10.1016/j.biortech.2012.10.085
Si, J., Wu, Y., Ma, H. F., Cao, Y. J., Sun, Y. F., and Cui, B. K. (2021b). Selection of a pH- and temperature-stable laccase from Ganoderma australe and its application for bioremediation of textile dyes. J. Environ. Manage. 299, 113619. doi:10.1016/j.jenvman.2021.113619
Simão, F. A., Waterhouse, R. M., Ioannidis, P., Kriventseva, E. V., and Zdobnov, E. M. (2015). BUSCO: assessing genome assembly and annotation completeness with single-copy orthologs. Bioinformatics 31 (19), 3210–3212. doi:10.1093/bioinformatics/btv351
Sirim, D., Wagner, F., Lisitsa, A., and Pleiss, J. (2009). The cytochrome P450 engineering database: integration of biochemical properties. BMC Biochem. 10, 27. doi:10.1186/1471-2091-10-27
Sjöström, E. (1981). Wood chemistry: fundamentals and applications. Salt Lake: Academic Press, 68–82.
Spániková, S., and Biely, P. (2006). Glucuronoyl esterase-novel carbohydrate esterase produced by Schizophyllum commune. FEBS Lett. 580 (19), 4597–4601. doi:10.1016/j.febslet.2006.07.033
Stamatakis, A., Hoover, P., and Rougemont, J. (2008). A rapid bootstrap algorithm for the RAxML web servers. Syst. Biol. 57 (5), 758–771. doi:10.1080/10635150802429642
Stanke, M., Diekhans, M., Baertsch, R., and Haussler, D. (2008). Using native and syntenically mapped cDNA alignments to improve de novo gene finding. Bioinformatics 24 (5), 637–644. doi:10.1093/bioinformatics/btn013
Stanke, M., Schöffmann, O., Morgenstern, B., and Waack, S. (2006). Gene prediction in eukaryotes with a generalized hidden Markov model that uses hints from external sources. BMC Bioinforma. 7, 62. doi:10.1186/1471-2105-7-62
Stanke, M., and Waack, S. (2004). Gene prediction with a hidden Markov model and a new intron submodel. Bioinformatics 19, 215–225. doi:10.1093/bioinformatics/btg1080
Sugano, J., Maina, N., Wallenius, J., and Hildén, K. (2021). Enhanced lignocellulolytic enzyme activities on hardwood and softwood during interspecific interactions of white- and brown-rot fungi. J. Fungi 7, 265. doi:10.3390/jof7040265
Sun, D., Lv, Z. W., Rao, J., Tian, R., Sun, S. N., and Peng, F. (2022). Effects of hydrothermal pretreatment on the dissolution and structural evolution of hemicelluloses and lignin: a review. Carbohyd. Polym. 281, 119050. doi:10.1016/j.carbpol.2021.119050
Sützl, L., Laurent, C. V. F. P., Abrera, A. T., Schütz, G., Ludwig, R., and Haltrich, D. (2018). Multiplicity of enzymatic functions in the CAZy AA3 family. Appl. Microbiol. Biotechnol. 102, 2477–2492. doi:10.1007/s00253-018-8784-0
Sweeney, M. D., and Xu, F. (2012). Biomass converting enzymes as industrial biocatalysts for fuels and chemicals: recent developments. Catalysts 2 (2), 244–263. doi:10.3390/catal2020244
Swofford, D. L. (2002). PAUP*: Phylogenetic analysis using parsimony (*and other methods). version 4.0 beta 10. Sunderland: Sinauer Associates. doi:10.1002/0471650129.dob0522
Tanaka, T., Noguchi, M., Watanabe, K., Misawa, T., Ishihara, M., Kobayashi, A., et al. (2010). Novel dialkoxytriazine-type glycosyl donors for cellulase-catalysed lactosylation. Org. Biomol. Chem. 8 (22), 5126–5132. doi:10.1039/c0ob00190b
Tu, T., Meng, K., Bai, Y. G., Shi, P. J., Luo, H. Y., Wang, Y. R., et al. (2013). High-yield production of a low-temperature-active polygalacturonase for papaya juice clarification. Food Chem. 141 (3), 2974–2981. doi:10.1016/j.foodchem.2013.05.132
Wan, C. X., and Li, Y. B. (2012). Fungal pretreatment of lignocellulosic biomass. Biotechnol. Adv. 30 (6), 1447–1457. doi:10.1016/j.biotechadv.2012.03.003
Wang, J. H., Feng, J. J., Jia, W. T., Chang, S., Li, S. Z., and Li, Y. X. (2015). Lignin engineering through laccase modification: a promising field for energy plant improvement. Biotechnol. Biofuels 8, 145. doi:10.1186/s13068-015-0331-y
Wang, S., Meng, K., Luo, H. Y., Yao, B., and Tu, T. (2020). Research progress in structure and function of pectin methylesterase. Chin. J. Biotechnol. 36 (6), 1021–1030. doi:10.13345/j.cjb.190418
Wang, X. W., and Wang, L. (2016). GMATA: an integrated software package for genome-scale SSR mining, marker development and viewing. Front. Plant Sci. 7, 1350. doi:10.3389/fpls.2016.01350
Wang, Y. P., Tang, H. B., DeBarry, J. D., Tan, X., Li, J. P., Wang, X. Y., et al. (2012). MCScanX: a toolkit for detection and evolutionary analysis of gene synteny and collinearity. Nucleic Acids Res. 40 (7), e49. doi:10.1093/nar/gkr1293
Waterman, M. R., and Lepesheva, G. I. (2005). Sterol 14α-demethylase, an abundant and essential mixed-function oxidase. Biochem. Biophys. Res. Commun. 338 (1), 418–422. doi:10.1016/j.bbrc.2005.08.118
Yang, H. T., Yu, B., Xu, X. D., Bourbigot, S., Wang, H., and Song, P. A. (2020). Lignin-derived bio-based flame retardants toward high-performance sustainable polymeric materials. Green Chem. 22, 2129–2161. doi:10.1039/d0gc00449a
Yang, L. Y., Guan, R. L., Shi, Y. X., Ding, J. M., Dai, R. H., Ye, W. X., et al. (2018). Comparative genome and transcriptome analysis reveal the medicinal basis and environmental adaptation of artificially cultivated Taiwanofungus camphoratus. Mycol. Prog. 17, 871–883. doi:10.1007/s11557-018-1391-8
Yoshida, Y., Aoyama, Y., Noshiro, M., and Gotoh, O. (2000). Sterol 14-demethylase P450 (CYP51) provides a breakthrough for the discussion on the evolution of cytochrome P450 gene superfamily. Biochem. Biophys. Res. Commun. 273 (3), 799–804. doi:10.1006/bbrc.2000.3030
Yu, F., Song, J., Liang, J. F., Wang, S. K., and Lu, J. K. (2020). Whole genome sequencing and genome annotation of the wild edible mushroom, Russula griseocarnosa. Genomics 112 (1), 603–614. doi:10.1016/j.ygeno.2019.04.012
Yuan, Y., Wu, F., Si, J., Zhao, Y. F., and Dai, Y. C. (2019). Whole genome sequence of Auricularia heimuer (Basidiomycota, Fungi), the third most important cultivated mushroom worldwide. Genomics 111 (1), 50–58. doi:10.1016/j.ygeno.2017.12.013
Zhao, Q. Q., Chi, Y. J., Zhang, J., and Feng, L. R. (2019). Transcriptome construction and related gene expression analysis of Lenzites gibbosa in woody environment. Sci. Silvae Sin. 55 (8), 95–105. doi:10.11707/j.1001-7488.20190811
Zheng, F., An, Q., Meng, G., Wu, X. J., Dai, Y. C., Si, J., et al. (2017). A novel laccase from white rot fungus Trametes orientalis: purification, characterization, and application. Int. J. Biol. Macromol. 102, 758–770. doi:10.1016/j.ijbiomac.2017.04.089
Zheng, F., Cui, B. K., Wu, X. J., Meng, G., Liu, H. X., and Si, J. (2016). Immobilization of laccase onto chitosan beads to enhance its capability to degrade synthetic dyes. Int. Biodeterior. Biodegr. 110, 69–78. doi:10.1016/j.ibiod.2016.03.004
Zhou, M., Guo, P., Wang, T., Gao, L. N., Yin, H. J., Cai, C., et al. (2017). Metagenomic mining pectinolytic microbes and enzymes from an apple pomace-adapted compost microbial community. Biotechnol. Biofuels 10, 198. doi:10.1186/s13068-017-0885-y
Zhu, N., Yang, J. S., Ji, L., Liu, J. W., Yang, Y., and Yuan, H. L. (2016). Metagenomic and metaproteomic analyses of a corn stover-adapted microbial consortium EMSD5 reveal its taxonomic and enzymatic basis for degrading lignocellulose. Biotechnol. Biofuels 9, 243. doi:10.1186/s13068-016-0658-z
Keywords: wood-decaying fungi, whole genome, lignocellulose degradation, secondary metabolism, gene annotation
Citation: Ma J-X, Wang H, Jin C, Ye Y-F, Tang L-X, Si J and Song J (2024) Whole genome sequencing and annotation of Daedaleopsis sinensis, a wood-decaying fungus significantly degrading lignocellulose. Front. Bioeng. Biotechnol. 11:1325088. doi: 10.3389/fbioe.2023.1325088
Received: 20 October 2023; Accepted: 15 December 2023;
Published: 16 January 2024.
Edited by:
Wenlong Xiong, Zhengzhou University, ChinaReviewed by:
Cheng Cai, South China Agricultural University, ChinaCopyright © 2024 Ma, Wang, Jin, Ye, Tang, Si and Song. This is an open-access article distributed under the terms of the Creative Commons Attribution License (CC BY). The use, distribution or reproduction in other forums is permitted, provided the original author(s) and the copyright owner(s) are credited and that the original publication in this journal is cited, in accordance with accepted academic practice. No use, distribution or reproduction is permitted which does not comply with these terms.
*Correspondence: Jing Si, amluZ3NpMTc4OEAxMjYuY29t; Jie Song, amllc29uZzEyMkBnbWFpbC5jb20=
Disclaimer: All claims expressed in this article are solely those of the authors and do not necessarily represent those of their affiliated organizations, or those of the publisher, the editors and the reviewers. Any product that may be evaluated in this article or claim that may be made by its manufacturer is not guaranteed or endorsed by the publisher.
Research integrity at Frontiers
Learn more about the work of our research integrity team to safeguard the quality of each article we publish.