- 1Departamento de Ingeniería Mecánica, Universidad de Santiago de Chile, USACH, Santiago de Chile, Chile
- 2Center for Research and Innovation in Bioengineering, Universitat Politècnica de València, València, Spain
- 3Departamento de Ingeniería Mecánica y Metalúrgica, Pontificia Universidad Católica de Chile, Santiago de Chile, Chile
The Constrained Mixture Model (CMM) is a novel approach to describe arterial wall mechanics, whose formulation is based on a referential physiological state. The CMM considers the arterial wall as a mixture of load-bearing constituents, each of them with characteristic mass fraction, material properties, and deposition stretch levels from its stress-free state to the in-vivo configuration. Although some reports of this model successfully assess its capabilities, they barely explore experimental approaches to model patient-specific scenarios. In this sense, we propose an iterative fitting procedure of numerical-experimental nature to determine material parameters and deposition stretch values. To this end, the model has been implemented in a finite element framework, and it is calibrated using reported experimental data of descending thoracic aorta. The main results obtained from the proposed procedure consist of a set of material parameters for each constituent. Moreover, a relationship between deposition stretches and residual strain measurements (opening angle and axial stretch) has been numerically proved, establishing a strong consistency between the model and experimental data.
1 Introduction
Numerous investigations have reported the presence of residual stresses on arteries (Chuong and Eason, 1986), whose effect is relevant to maintaining the homeostatic state in the cardiovascular system, preventing significant intramural stress gradients on the arterial wall (Rachev and Hayashi, 1999; Wang and Gleason, 2010; Peña et al., 2015; Sigaeva et al., 2019). Its presence may be explained by non-uniform growth and remodeling processes (Fung, 1991; Bellini et al., 2014), where the stress distribution and magnitude can be altered with respect to normal levels, in face of diseases, aging or injuries (Fung, 1991; Cardamone et al., 2009; Horný et al., 2017). The residual stresses on an artery are manifested when it is extracted from its physiological state and subsequently cut, evidencing geometrical changes concerning its initial shape. Specifically, a shortening is experienced in the axial direction and, when the arterial ring-shape in an ex-vivo state is cut along the thickness, an opening movement can be observed. These changes from the initial configuration of the artery proves the presence of residual stresses. In practice, experimental information about residual deformation is obtained via pre-stretching and ring-opening tests (Navarrete et al., 2020; Rivera et al., 2020) where, by assuming a stress-free geometry as referential configuration, the residual stresses can be determined by an indirect way through numerical modeling of the closure ring simulation (García-Herrera et al., 2016). The artery shortening and radial cut generate a nearly complete residual strain and stress release. However, different authors questioned this statement, adding alternative measures to characterize a complete stress-free state, by either measuring a longitudinal opening (Wang and Gleason, 2010), separating arterial layers (Peña et al., 2015) or formulating novel constitutive theories that do not require an experimental stress-free state due to the physical impracticality of generating a full release of residual stresses (Ciarletta et al., 2016).
The Constrained Mixture Model (CMM) appears as a phenomenological approach for soft tissue mechanics in which different aspects related to growth and remodeling (G&R) phenomena are considered. These aspects are mainly referred to the time-dependent production of arterial constituents to different stressed configurations, whose activity is driven by a mechanobiological response of cell activity (Humphrey and Rajagopal, 2002). Unlike classical approaches, which consider stress-free as a referential configuration (Maes et al., 2019), the CMM is based on an in-vivo referential geometry (pressurized and axially loaded). This reference configuration avoids the questioning mentioned above about the stress-free state and the lack of information about the compressive response of each specific constituent (Bellini et al., 2014). A fundamental feature of this model is the consideration that the artery wall is composed of different load-bearing constituents: elastin, collagen, and smooth muscle cells (Valentín et al., 2013; Mousavi and Avril, 2017); each one contributing to its overall behavior, using concepts related to the theory of mixtures and homogenization (Humphrey and Rajagopal, 2002). The CMM framework allows accounting for the weighted contribution of strain energy and stress for each constituent, according to kinetic considerations related to their mass evolution (production and removal). In this way, previous studies on arteries revealed a high rate of production of elastin in the perinatal period, followed by a low turnover rate during maturity (Humphrey and Rajagopal, 2002). In contrast, collagen is continuously produced and degraded (short half-life), which may be further reduced by arterial diseases, like hypertension or aneurysms (Cyron and Humphrey, 2017). On the other hand, the CMM takes into consideration potential structural changes of each component; for example, through degradation of their material properties (Latorre and Humphrey, 2020), or alteration of its stressed configuration over time (Laubrie et al., 2022). Particular attention is put on this last point since, as the in-vivo configuration is taken as a referential state, the definition of a characteristic deposition stretch (pre-strain) by each constituent is necessary, which quantifies its deformation from a stress-free (natural) configuration to the referential state. The theoretical basis of the CMM explains the presence of residual stresses as a result of the difference in stress states between constituents deposited to the material and those of the material itself (Humphrey and Rajagopal, 2002).
As stated by the overview work performed by (Morin and Avril, 2015), which is focused on inverse-nature problems applied to the arterial mechanical behavior, the proper calibration of the mechanobiological model parameters is a challenge to be addressed. One of the main tasks concerning models that consider an in-vivo reference state is the determination of its pre-stress field via pre-strain, due to the assumption of material elasticity (Maas et al., 2016). Therefore, it is necessary to conceive methods to determine the desired parameters, with the limitation associated to the lack of information about the stress-free state. Related to this point, different authors have developed computational frameworks to solve this problem (Weisbecker et al., 2014). utilized information about the in-vivo reference configuration (geometry and loads) to determine pre-stretch gradients present in this configuration, based on an iterative procedure that involves solving an inverse finite element problem. The work of (Maas et al., 2016) was focused on determining pre-stresses and pre-strains of biological tissues in a finite element context by an algorithm that verifies equilibrium in the face of potential incompatibilities concerning initial pre-strain values.
Many efforts have been put into the numerical implementation of the CMM and its application in G&R. The increasing need to characterize the material parameters to achieve practical use of this model applied to specific patients has contemporary significance. One of the main limitations is the difficulty at the time of determining material parameters and deposition stretch values directly from experimental data, because of the macroscopic nature of the most commonly used testing methods. In this regard (Latorre and Humphrey, 2018), developed a progressive nonlinear regression to determine elastic and G&R parameters of a CMM using experimental data from (Wu et al., 2014; Bersi et al., 2016) for a particular mouse model of hypertension for which information was available on the time course of changes in blood pressure, wall composition, material properties, and inflammation (Mousavi and Avril, 2017). developed a finite element implementation to determine the pre-strain tensor of elastin, which is subjected to high stretches due to its initial deposition in the perinatal period in conjunction with its long half-life. This implementation was applied to obtain residual stresses and to validate the opening angle on an idealized geometry, using experimental data of a murine artery segment. In addition, the determination of the residual stress field and material parameters using the CMM was evaluated on the patient-specific model of a human ascending thoracic aorta. Within the study performed by (Laubrie et al., 2022), an algorithm based on (Maas et al., 2016) was implemented to derive the spatial distribution of pre-strain, considering the combined effect of elastin and ground substances of extracellular matrix on a patient-specific aortic arch (Maes et al., 2019). introduced an iterative fitting method, determining a set of material parameters for the CMM under the assumption of fixed deposition stretch values.
In this context, taking previous studies as a basis, we propose in the present work a numerical approach to perform an integrated characterization on these values. To achieve this objective, the CMM has been implemented in the FEBio finite element framework (Maas et al., 2012). The model characterization has been performed using experimental mechanical data reported by (Rivera et al., 2021) on a newborn lamb-specific descending thoracic aorta. The CMM is fitted to fulfill and correlate with experimental data obtained from the following tests: ring-opening, pre-stretch, pressurization-stretch and uniaxial tensile using samples in circumferential and longitudinal directions.
The contents of the manuscript are organized as follows: Section 2 presents the CMM background, details about experimental procedure and the information considered from it, along with the description of the iterative-fitting procedure proposed. Section 3 shows the main results obtained after applying this procedure on a patient-specific case, and a sensitivity analysis of the variation of relevant parameters. Section 4 includes an extensive discussion and analysis of numerical results and performance of the proposed procedure. Finally, further remarks and future research lines are identified.
2 Material and methods
In this section, the aspects related to the mathematical formulation of the Constrained Mixture Model (CMM) (Section 2.1) along with the procedure to perform the corresponding fitting (Section 2.3) are exhibited below. It is worth mentioning that experimental data used for this aim is totally obtained from previous studies (detailed in Section 2.2), and this work is particularly focused on the numerical analysis of this data.
2.1 Constrained mixture model (CMM)
According to Figure 1, the CMM considers that the arterial wall mechanics is influenced by a mixture of the major families of load-bearing constituents (α): elastin (e), collagen (c) and smooth muscle (m) (Wang et al., 2016), each one associated with its corresponding mass fraction (ϕα; α = e, c, m). Each constituent is deposited into the mixture, from its theoretical stress-free (natural) configuration to the in-vivo configuration (with pressurization and axial stretch at physiological levels). The deformation gradient experienced by each family of constituents α is, under this condition, named as deposition stretch (Gα). These constituents are constrained to deform with the mixture in the face of subsequent changes in the mechanical loads on the whole arterial wall, whose deformation gradient is denoted by F. Therefore, it is possible to define the deformation gradient of each specific constituent (Fα) when the arterial wall changes its configuration with reference to homeostatic conditions as:
The arterial wall is often considered an incompressible hyperelastic material (Chagnon et al., 2015). In this sense, the theory of hyperelasticity establishes that the stress-strain relationship is adequately modeled with a consistent strain energy function (W). To enforce incompressibility in the finite element context, the formulation of W is considered in its nearly-incompressible expression (Mousavi and Avril, 2017; Latorre and Humphrey, 2020). This condition is associated with an isochoric-volumetric decomposition of W, whose particularization to the CMM is stated by:
where the overall isochoric contribution corresponds to the sum of each specific-constituent strain energy function (left term of Eq. (2)), which in turn is dependent on the isochoric part of the right Cauchy-Green strain tensor
where κ is a penalty parameter related with the bulk modulus which, in a near incompressibility condition, is considered much greater than the shear modulus defined within the isochoric term of W.
Focusing on the definition of
where I is the identity tensor, ce represents the shear modulus, and
The strain energy function of the isochoric term of the collagen (c)
where
The strain energy function of the isochoric component of the smooth muscle (m)
where
The deposition stretch tensor of each constituent (Gα) is defined symmetrically and volume-preserving
The deposition stretch tensor of each family of collagen fibers (Gci, i = 0, 1) and smooth muscle (Gm) are defined in reference to their mean orientation (dci i = 0, 1 and dm, respectively) in cylindrical coordinates, according to (Maes et al., 2019):
where Gc and Gm correspond to the deposition stretch values along the respective fiber directions.
The definition of the different deformation gradients related to the CMM allows us to define the Second Piola-Kirchhoff stress tensor (S) as:
Finally, from the expression 10, the Cauchy-stress tensor can be written as:
2.2 Experimental procedure
The procedure described below considers the experimental data of a descending thoracic aorta of a 30-day-old newborn lamb (Ovis Aries) available in the works of (Rivera et al., 2020; Rivera et al., 2021). Further information about protocols and methods of measurement are detailed in both references. Figure 2 shows a scheme with the different mechanical loads and deformations to which an arterial specimen is subjected from its in-vivo state, to uniaxial stretch in longitudinal and circumferential directions. In this sense, the experimental procedure accounts for information of these states, via appropriate mechanical and residual deformation tests. The physiological arterial stretch λz related to in-vivo and zero-pressure states (steps (1) and (2), respectively) was obtained via the pre-stretching test, measuring the longitudinal shortening between the zero-pressure and ex-vivo states (steps (2) and (3)) (Navarrete et al., 2020). The outer diameters
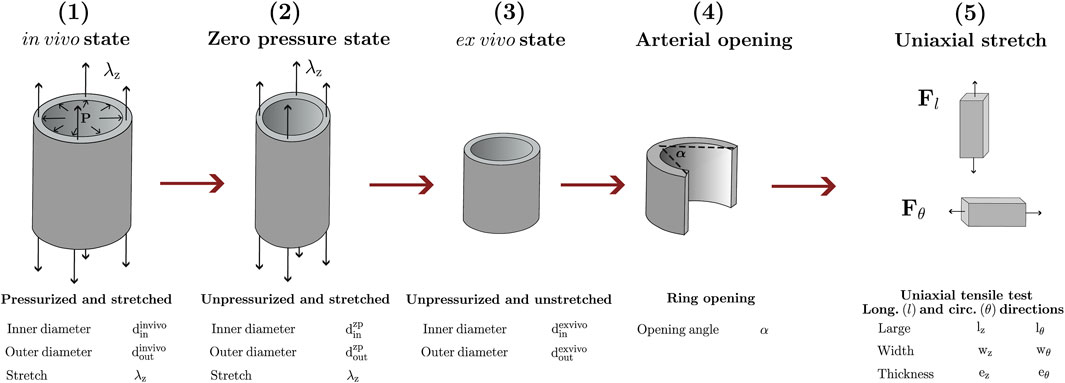
FIGURE 2. Mechanical loads and deformations to which the artery is subjected under different states: (1) in-vivo; (2) zero-pressure; (3) ex-vivo; (4) arterial opening; (5) uniaxial stretch.
2.3 Iterative numerical-experimental fitting
Taking as basis the iterative procedure followed by (Maes et al., 2019), the material parameters
To provide a clear description of the iterative procedure, we first distinguish two main stages (Figure 3), each of them aiming to the determination of: the set of materials parameters mp (Stage 1, Figure 3A) and the deposition stretch values ds (Stage 2, Figure 3B).
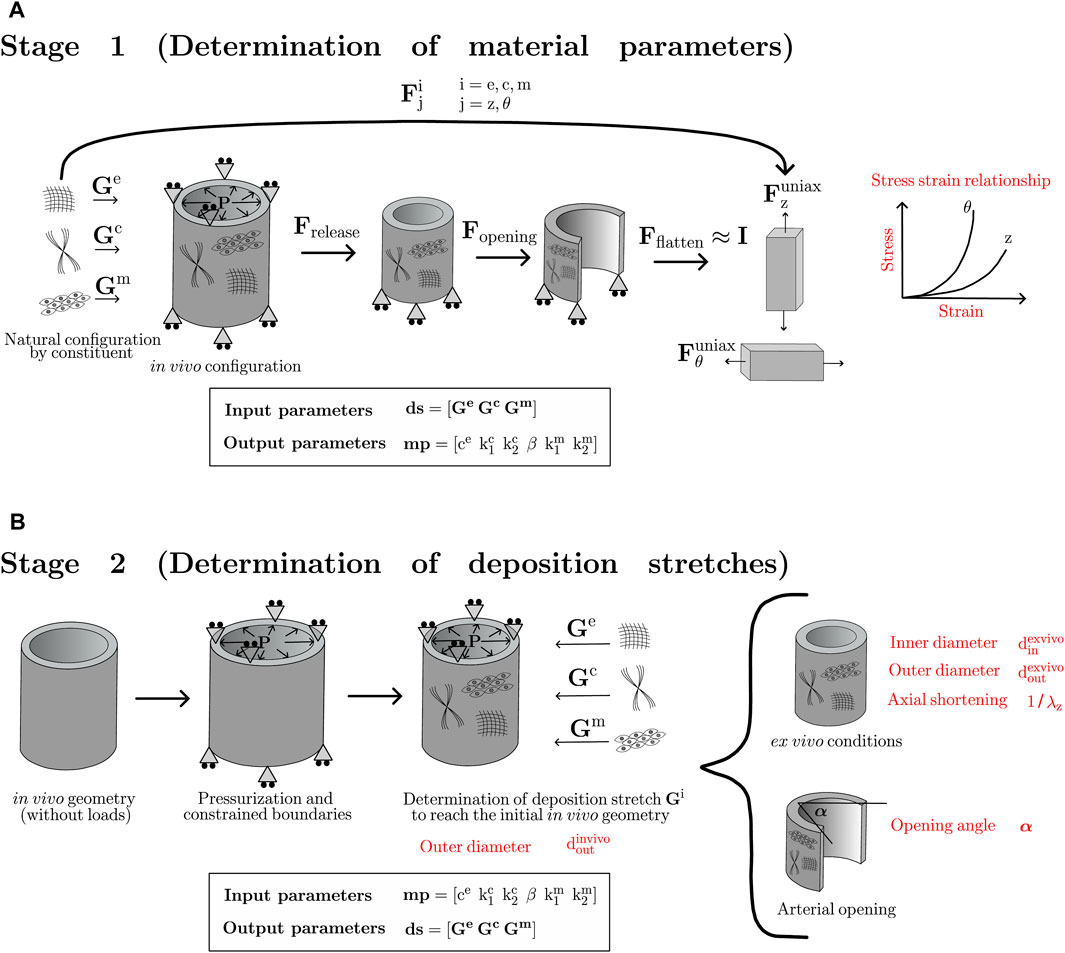
FIGURE 3. Numerical stages followed to determine: (A) material parameters; (B) deposition stretch values.
2.3.1 Stage 1
In Stage 1, the individual constituents are deposited from its natural configuration to the artery in its in-vivo state through a given set of ds (input parameters). Since the uniaxial test configuration is a flat strip of tissue, a series of deformation gradients, that allow to account for the changes occurred between the in-vivo and uniaxial flatten-strips, are applied to the uniaxial problem. Those gradients account for the loads release (Frelease), radial opening (Fopening) and strip flattening (Fflatten), where this last step is considered to produce near-zero strains on the sample strip (Fflatten ≈ I), due to its negligible value with respect to the deformation experienced in the previously mentioned steps (Maes et al., 2019). During the first iteration, both deformation gradients Frelease and Fopening are considered to be equal to an identity tensor (I), as well as the deposition stretch parameters ds. However, in the consecutive iterations, those tensors and parameters are updated from the second stage of the proposed algorithm. The mentioned tensors, defined in cylindrical coordinates (r, θ, z), are given by the following expressions:
Finally, the deformation gradient of each constituent from its natural configuration to the stretching applied in the uniaxial tensile test
Through this procedure, a set of final material parameters mp can be computed (output parameters). To this end, the experimental longitudinal and circumferential stress-strain relationships are taken as target curves, where the optimal parameters mp are determined by solving the inverse finite element problem associated with the numerical uniaxial tensile test in both directions.
2.3.2 Stage 2
As shown in Figure 3B, Stage 2 considers fixed material parameters (mp) obtained as an output from Stage 1, and the in-vivo arterial geometry as the referential configuration, according to the fact that the constituents are deposited from its stress-free state to the mentioned state. Thus, the procedure of Stage 2 considers the reference geometry in the diastolic pressure stage, experimentally obtained by the inflation-extension test. Then, the artery is pressurized and axially constrained to reproduce in-vivo loads. However, geometrical dimensions reached in this step are not compatible with any real configuration. Then, the next step consists of applying the corresponding set of deposition stretches (ds), such that these values enable us to obtain the initial dimensions at which the analysis of Stage 2 began, ideally producing a near-zero dimensional change in the reference configuration. Therefore, at the end of this step, the artery has reached the referential geometry with its corresponding in-vivo stress field. Stage 2 ends with the release of the in-vivo loads, assumed to be a numerical ex-vivo configuration. The determination of parameters ds is performed via a finite element inverse approach, in which
2.3.3 Integrated procedure
The two stages described above (Section 2.3.1 and Section 2.3.2) are part of the iterative procedure proposed in this work, whose goal consists of simultaneously determining material parameters (mp) and deposition stretch values (ds). As Figure 4 states, consecutive iterations from both stages are computed in a custom inverse implementation of Python’s LMFit library (Newville and Stensitzki, 2018) using FEBio results together with the standard Levenberg-Marquardt algorithm of this implementation. An experimental-numerical residual minimization approach was used to characterize both material parameters and the deposition stretches. A stop criterion on the material parameters is set to a 5% variation between the optimization iterations. The objective function f(x) used to optimize the material parameters in Stage 1 is defined as follows:
, where J is the standardization function for the stress-strain residual, n represents the number of discrete points in each curve, y is the experimental value, and
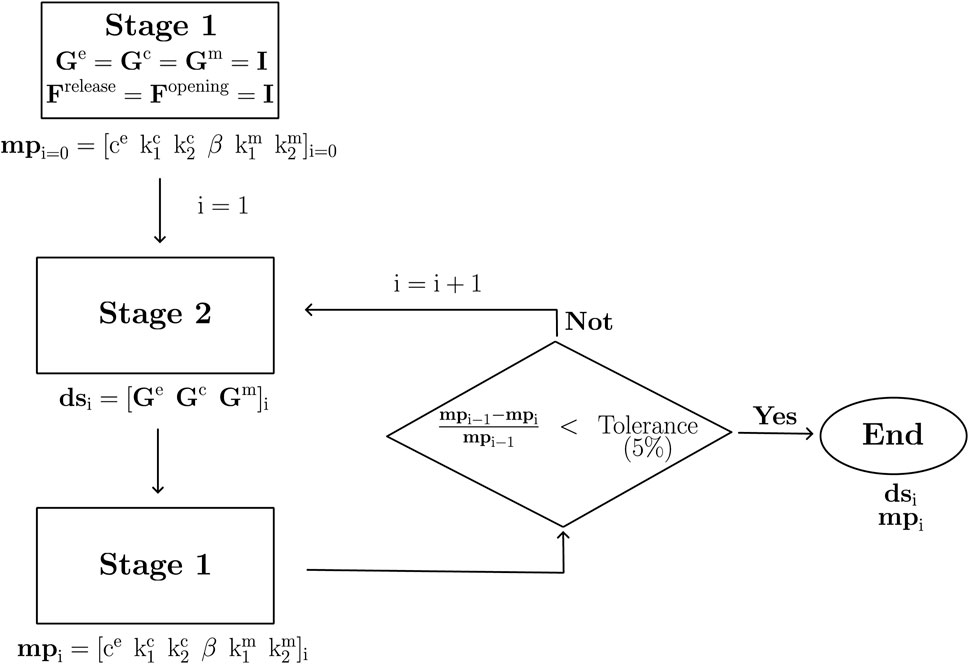
FIGURE 4. Algorithm proposed to determine the set of material parameters (mp) and deposition stretches (ds).
By another side, the objectve function g(x) utilized to optimize the desposition stretch values in Stage 2 is defined as:
, where the K function defines the quadratic error used to calculate the in vivo and ex-vivo geometry error between experimental and numerical values.
The ring-shaped finite element mesh used in the numerical simulations is composed of 8-node hexahedra, with a total of 900 elements and 1952 nodes (15 radial elements, 60 circumferential elements, and one element in the axial direction). The formulation considers a three-field implementation in order to avoid numerical locking of nearly-incompressible materials (Maas et al., 2012). Moreover, the numerical simulation of the uniaxial tensile test is performed, due to the development of homogeneous stress and strain fields, by considering a single 8-noded hexahedral element.
3 Results
3.1 Patient-specific study on newborn lamb aorta
Taking the experimental results of the in-vivo geometry, whose values are detailed in Section 2.2, the descending thoracic aorta is modeled considering a straight cylindrical shape (Karšaj et al., 2010; Valentín et al., 2011). From the output parameters of the fitting procedure (detailed in Section 2.3), a set of material parameters mp and deposition stretch values ds were obtained. Table 1 shows the parameters used as input and those obtained from the numerical procedure (output values).
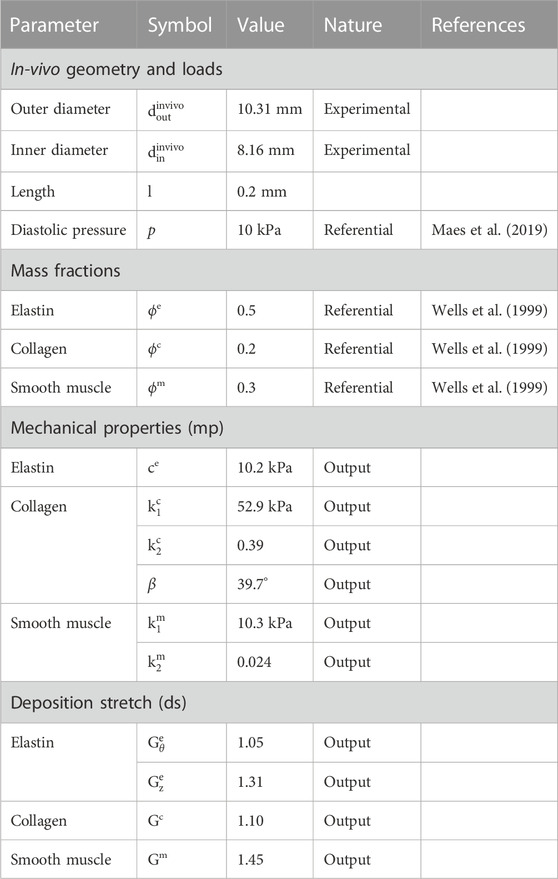
TABLE 1. CM model parameters, specifying those determined experimentally, by reference and as output from iterative procedure.
Focusing on the characterization procedure, Figure 5 denotes the percentage difference of each parameter, concerning the values of the previous iteration. Figure 5A, refers to the material parameters mp that quickly approach to the 0% variation level (red line). On this basis, a remarkable case is visualized for
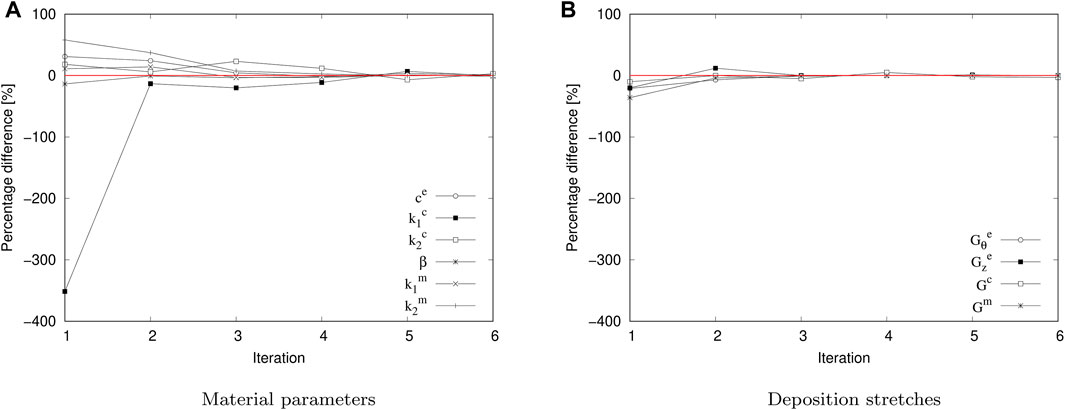
FIGURE 5. Percentage difference regarding to the successive iteration steps in the iterative fitting procedure. (A) material parameter relative variation (mp). (B) deposition stretch relative variation (ds).
As stated in the description of Stage 1 (Section 2.3.1), a release and opening deformation gradient is obtained and utilized for the uniaxial extension. After the sixth iteration, the average values (average ±SEM)) across the thickness of the tissue are:
Concerning to the target information used in Stages 1 and 2 of the iterative procedure, Figure 6A shows experimental and numerical Cauchy stress-stretch curves in circumferential and longitudinal directions, which are used in Stage 1 (Figure 3A). The numerical results are obtained from the set of material parameters (mp) after the last iteration performed. A good fit between both curves is reflected by the R-squared values:
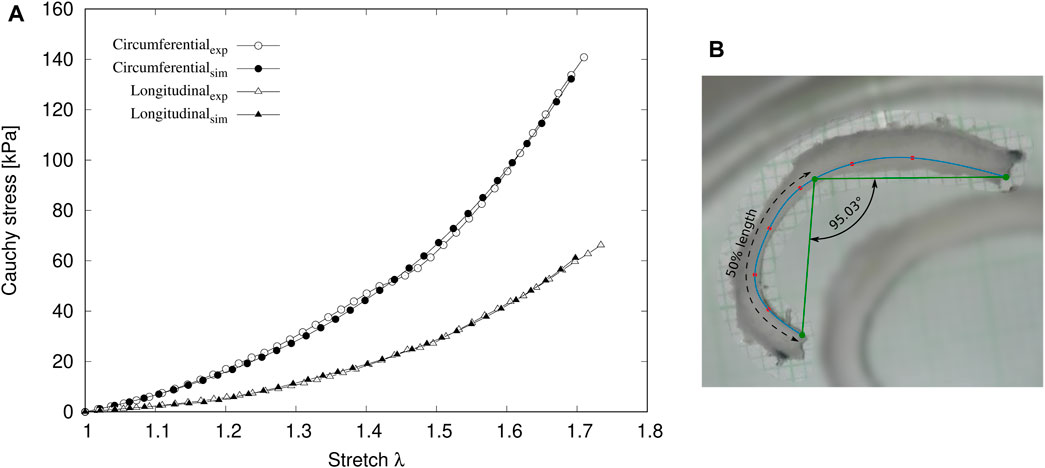
FIGURE 6. (A) Experimental and numerical Cauchy stress-stretch curves in circumferential and longitudinal directions. (B) experimental opening angle α.

TABLE 2. Experimental and numerical ratios between in-vivo and ex-vivo dimensions, along with its corresponding percentage difference.
It is important to emphasize that the results shown in Table 2 are obtained from the last iteration performed. The main results denote that the difference between the numerical and experimental results, both for the variation of the outer diameter, thickness, and length of the artery, show differences that do not exceed 10%. Unreported results of this work indicate that once the pressurization and deposition stretches are applied on the arterial wall (see Figure 3), the geometrical variation respecting the initial in-vivo geometry is practically negligible (radial node displacement is in the order of 10–2 mm), meaning that the equilibrium between the in-vivo loads and the deposition process is successfully achieved.
The opening angle α is considered as an external parameter to check the consistency of the obtained values in the procedure followed in this work. Figure 6B exhibits the measurement of the experimental opening angle of 95.03°. According to the set of mp and ds values obtained via the iterative fitting procedure, the numerical ring opening simulation (Figure 3B) results in an opening angle of 91.39°, corresponding to an error of 3.83%.
3.2 Sensitivity analysis
Once the consistency of the experimental-numerical procedure has been proved (Section 3.1), a sensitivity study is performed to explore the effect of the deposition stretch and mass fraction variations in the resulting residual strains. For this analysis, the numerical results extracted from the longitudinal arterial shortening and opening angle have been considered. The variation range of the considered parameters, along with their respective referential values, are shown in Table 3. These baseline values and the material parameters mp have been taken from the final iteration of the patient-specific study of the lamb aorta, according to the values shown in Table 1. It needs to be noted that separated studies about the impact of mass fractions and deposition stretches are performed, meaning that the mass fraction sensitivity is done under a constant deposition stretch parameter set (from the last characterization iteration), and vice versa for the deposition stretch study (under constant mass fraction distribution, from the fixed reference values). It is essential to highlight that the mass fraction of smooth muscle ϕm is dependent on the remaining mass fractions, and Gc has been maintained in its baseline value (1.10), due to its negligible variation during the iterative procedure (Figure 5).
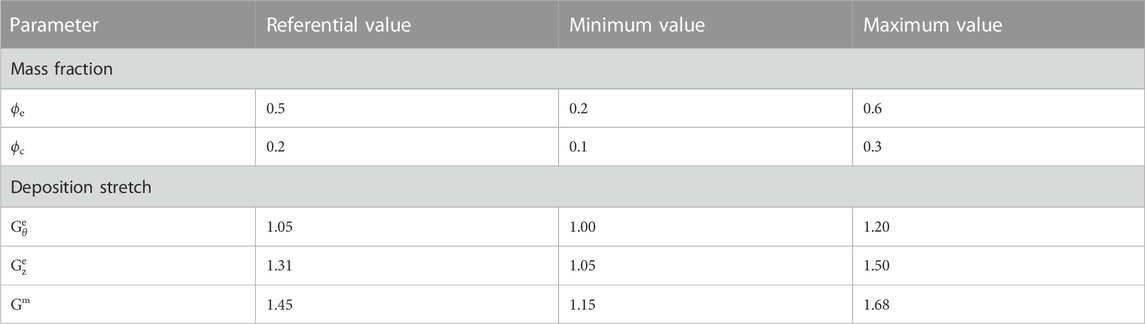
TABLE 3. Range of each parameter considered in sensitivity analysis and their respective referential values.
Figure 7 displays all the results from this study, according to the different cases considered. In particular, Figure 7A shows the opening angle α by varying the ϕe and ϕc values. According to the range of the variation of these mass fractions, there is an inverse relationship between them and the opening angle reached. Specifically, the highest values of ϕe and ϕc considered in this study have resulted in an opening angle of
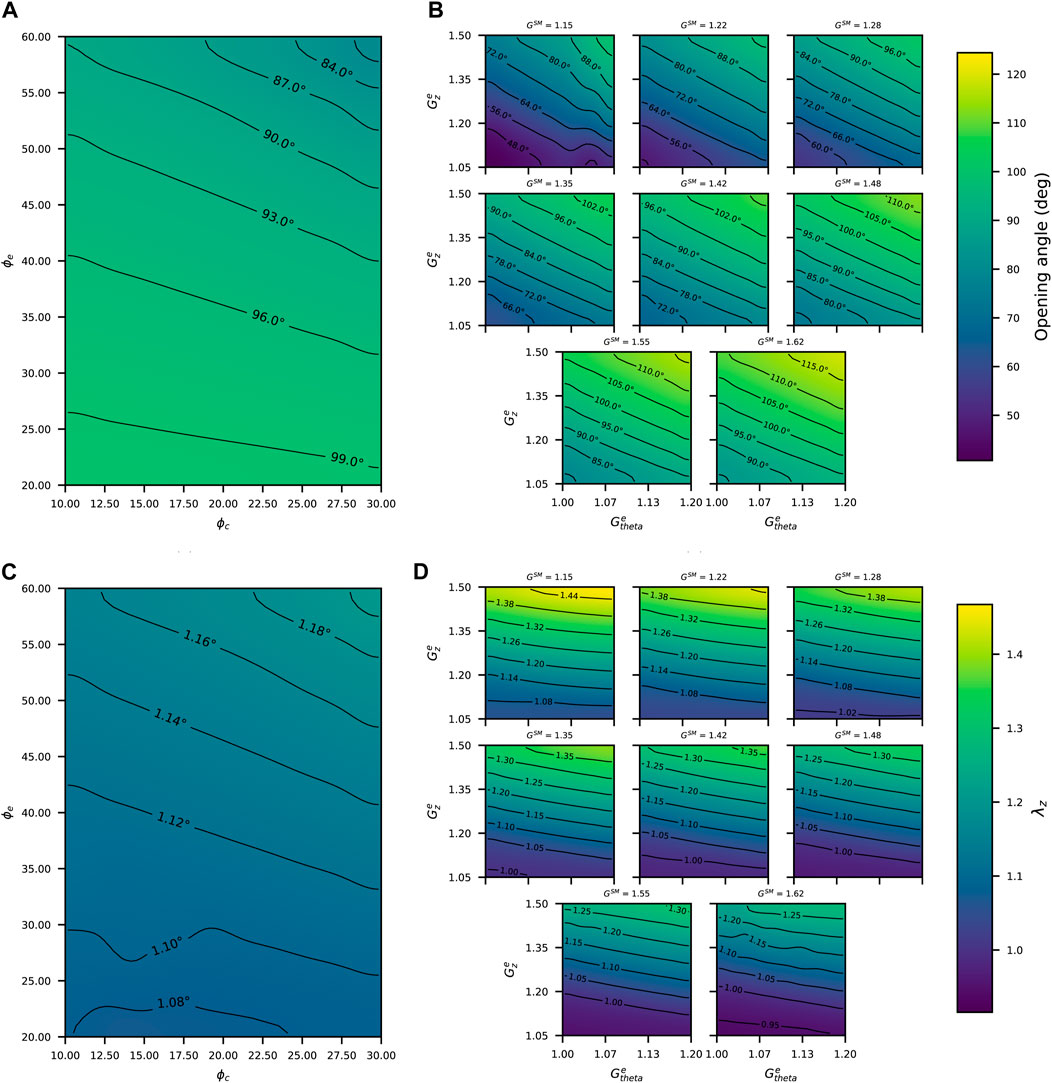
FIGURE 7. Opening angle in function of: (A) mass fractions; (B) deposition stretches. Longitudinal stretches λz in function of (C) mass fractions; (D) deposition stretches.
4 Discussion
A relevant aspect related to the CMM, particularly referred to the consideration of the in-vivo state as the referential configuration, is that it allows its applicability in a clinical context, where non-invasive techniques, such as Computed Tomography (CT) and Magnetic Resonance Imaging (MRI), are capable of measuring the in-vivo arterial geometry (Di Cesare et al., 2016). In these circumstances, its implementation in the FE context arises as an essential tool, allowing to predict the mechanical stress field on the arterial wall, under different configurations (for instance in-vivo, ex-vivo and zero pressure) (Mousavi and Avril, 2017). Regarding the features of CMM, one of the main problems is the lack of information, due to experimental limitations, about the mechanical properties and residual deformation at a constituent level. As proposed in this work, numerical procedures are a feasible way to approximate these unknown parameters based on the available experimental information. Consequently, we have applied it to a patient-specific case, and consistency between the obtained parameters with experimental information has been successfully demonstrated.
Focusing the analysis on the specific aspects related to this study, the results displayed in Figure 5 show a fast convergence of both material parameters (mp) and deposition stretches (ds), where after the sixth iteration, the percentage error in all cases is less than the tolerance defined. Concerning the same analysis performed by Maes et al., 2019 (Maes et al., 2019), the convergence to the set of their material parameters is reached after the seventh iteration, with the consideration of five material parameters (in this study, there were six), and known deposition stretch values.
The parameter fitting procedure described in Section 2.3.3 involves a number of challenges to be addressed, aiming to overcome difficulties in the iterative process and reach consistent results, such as is obtained in this study (see Figure 5). A critical issue to be discussed is referred to the suitable choice of parameters, mainly because of the localized optimization nature of the gradient-based optimization algorithms. The starting parameters have been chosen based on those reported in the existing literature to avoid possible non-realistic solutions. Even when the experimental measurement of the ring-opening angle (α) does not form part of the optimization process itself, it has been proved that the material parameters along with the deposition stretches naturally affect this residual strain, serving as a consistency verification measurement of the stress-release process experienced in this test. A remark regarding this measurement is the consistency of the curvature across the opened tissue, suggesting possible bias regarding the idealized geometry considered in this work. Nevertheless, considering that the obtained error percentage between the experimental and numerical opening angle for the final iteration is under 5%, we consider this as a successful verification of the fitted parameters.
A physical interpretation of the determined deposition stretch parameters needs to be analyzed. Relating to elastin (e) fibers, the circumferential and longitudinal components of the deposition stretch tensor determine the values reached by the radial direction, given by the incompressibility constraint considered in this formulation. As such, the higher the values of
Given the scarce information of the parameters that have been established in this work and the results obtained in this context, the deposition stretch value of the smooth muscle Gm is outstanding, with reference to those typically reported in the literature. Most of the works in this topic take this value in the range of 1.1–1.2 (Karšaj et al., 2010; Bellini et al., 2014; Laubrie et al., 2022), noting that the half-life of this component within the arterial wall is relatively low, and it is in continuous renewal, so it is not subjected to a great extent to the effects of growth and remodeling. However, no concrete experimental study determines a reference in the magnitude of this value, so the value determined in this work further motivates the study of the mechanisms by which the deposition stretch is physically established (Humphrey et al., 2014).
The sensitivity analysis (Section 3.2) is performed to get a better overview of the relative influence of the mass fractions and deposition stretches of each constituent on residual strains (opening angle α and axial stretch λz), which are easily measurable experimentally. Figure 7, being formulated based on the same geometrical and material parameters, allows linking strain measurements with residual stresses in the circumferential and axial directions. An important aspect to emphasize from these results is that both α and λz are more sensitive to the variation in the deposition stretch values than to the mass fractions. That point supports the procedure established in Section 2.3 of this work, regarding the consideration of referential values of mass fraction, while the deposition stretch values were those to be determined within the fitting procedure due to the importance of a correct choice of their values. This aspect reinforces the effectiveness of the iterative fitting procedure, by applying it to a patient-specific case.
In addition, some works related to CMM consider the arterial wall as a two-layer material, according to the fact that the media and adventitia have different composition and material properties (Karšaj and Humphrey, 2012; Mousavi et al., 2019). However, in most animal models taken as reference (including those used in this work), it is not possible to separate both layers experimentally to perform individual mechanical tests. In many cases, the parameters considered are fictitious, and the analysis is focused on numerical purposes. In Latorre and Humphrey, 2018 (Latorre and Humphrey, 2018), however, this distinction let account for differences in medial versus adventitial fibrosis that resulted in marked aortic maladaptation in hypertension. In this particular case, as the experimental information available refers to the whole tissue, the CMM considers homogenized parameters along the thickness, avoiding the incorporation of different hypotheses about the characteristic behavior by each specific layer.
The consideration about the behavior of fibers under compressive loads, named in literature as tension-compression switch (Latorre et al., 2016; Horgan and Murphy, 2020), has been implemented in this study, according to the seminal works of Holzapfel et al., 2000 and Gasser et al., 2006 (Holzapfel et al., 2000; Gasser et al., 2006). In this regard, different objections have been raised about this hypothesis, mainly due to the lack of experimental evidence to back it up (Horgan and Murphy, 2020). suggested physical contradictions concerning isotropic response predicted by the constitutive models under a compression state, according to the experimental results provided by Holzapfel 2006 (Holzapfel, 2006). However, there is no clear evidence on the underlying mechanism of the fibers under compression. Some studies, related to the CMM, incorporate the distinction of tension and compression material properties on collagen fibers and smooth muscle (Bellini et al., 2014; Mousavi and Avril, 2017). However, there is no clear experimental evidence to support the values used.
The strain energy function derived within the CMM context (Expression 2) represents a phenomenological approach of arterial structure, through the consideration of isotropic and anisotropic behavior in separated terms (the former representing the integrated influence of elastin (e) and ground substances, and the latter those of collagen (c) and smooth muscle (m)). According to the material parameters (mp) determination (Holzapfel, 2006), identified a critical issue, referred to the impossibility of determining a unique set of values from the information provided only by uniaxial tensile tests, due to the fact that strain state of this mechanical test does not represent the physiological biaxial condition. To overcome this problem, it is necessary to obtain additional information about the microstructural arrangement of the arterial wall via histological analysis in order to characterize a physically-consistent set of material parameters as well as to include more experimental data from uniaxial or other experimental tests (Latorre et al., 2016). Recent investigations have focused on determining and evaluating non-destructive techniques for measuring arterial wall constituents, related directly to the determination of collagen fiber orientation and its reorientation in the face of external loads. Several methods have been used by different authors, as for instance, the second harmonic generation (SHG) (Deniset-Besseau et al., 2010; Bancelin et al., 2012; Bancelin et al., 2014; Golaraei et al., 2019; Cavinato et al., 2020), polarized spatial frequency domain imaging (pSFDI) (Jett et al., 2020) and quantitative-polarized light microscopy (Q-PLM) (Greiner et al., 2021), establishing an interesting possibility to switch from a phenomenological approach to a microstructural-consistent one.
In general, the incompressibility of the arterial wall is commonly accepted, and it has been widely taken as a hypothesis in multiple numerical formulations. However, some authors questioned this consideration, reporting volume changes in the physiological pressure range (Yosibash et al., 2014; Yossef et al., 2017). The work of Nolan et al., 2014 (Nolan and McGarry, 2016) performed on an ovine aorta revealed a degree of compressibility, given by a Poisson’s ratio value of 0.44. Therefore, constitutive models of compressible nature could affect the accuracy of stress prediction (Skacel and Bursa, 2019).
5 Conclusion
In order to assess the practical applicability of the CM model, through the determination of consistent parameters describing the mechanical response of the arterial wall, a numerical-experimental framework based on the finite element model has been established and applied to a patient-specific case. A fitting algorithm has been implemented to achieve this goal, considering experimental information of the in-vivo and ex-vivo geometries, along with data from uniaxial tensile tests in two directions. This information is set as a target, and by the application of an inverse finite element problem procedure, the material parameters (mp) and deposition stretch (ds) values are determined. The convergence of the procedure is evident, and the consistency of these parameters is reflected in the good relationship between experimental and numerical data. On the other hand, a parameter sensitivity study has established the preponderance of the deposition stretch values concerning the residual deformation levels. It should be noted that the CM model is formulated to model G&R phenomena, so the parameter determination through the procedure established in this work is contextualized as a starting point for the incorporation of these effects in the different numerical models to be developed.
Data availability statement
The raw data supporting the conclusion of this article will be made available by the authors, without undue reservation.
Author contributions
AN: Data curation, Formal Analysis, Investigation, Methodology, Visualization, Writing–original draft, Writing–review and editing. AU: Data curation, Formal Analysis, Funding acquisition, Investigation, Methodology, Software, Validation, Visualization, Writing–original draft. ER: Conceptualization, Data curation, Formal Analysis, Funding acquisition, Investigation, Methodology, Visualization, Writing–review and editing. ML: Conceptualization, Formal Analysis, Investigation, Methodology, Visualization, Writing–review and editing. DC: Conceptualization, Formal Analysis, Funding acquisition, Methodology, Software, Supervision, Writing–review and editing. CG-H: Conceptualization, Formal Analysis, Funding acquisition, Investigation, Project administration, Supervision, Writing–review and editing.
Funding
The author(s) declare financial support was received for the research, authorship, and/or publication of this article. The authors are grateful for the support of the Chilean National Agency of Research and Development (ANID) through project FONDECYT No. 1220956, the Faculty of Engineering (FING) USACH and the European Union’s research and innovation programme “Horizon Europe” through the (ERC) grant No. 101039349.
Acknowledgments
AN and AU thank ANID PFCHA/DOCTORADO NACIONAL BECAS CHILE (2019–21190623 and 2023-21231932, respectively).
Conflict of interest
The authors declare that the research was conducted in the absence of any commercial or financial relationships that could be construed as a potential conflict of interest.
Publisher’s note
All claims expressed in this article are solely those of the authors and do not necessarily represent those of their affiliated organizations, or those of the publisher, the editors and the reviewers. Any product that may be evaluated in this article, or claim that may be made by its manufacturer, is not guaranteed or endorsed by the publisher.
Supplementary material
The Supplementary Material for this article can be found online at: https://www.frontiersin.org/articles/10.3389/fbioe.2023.1301988/full#supplementary-material
References
Ahmadzadeh, H., Rausch, M. K., and Humphrey, J. D. (2019). Modeling lamellar disruption within the aortic wall using a particle-based approach. Sci. Rep. 9, 15320. doi:10.1038/s41598-019-51558-2
Bancelin, S., Aimé, C., Coradin, T., and Schanne-Klein, M.-C. (2012). In situ three-dimensional monitoring of collagen fibrillogenesis using SHG microscopy. Biomed. Opt. Express 3 (6), 1446. doi:10.1364/boe.3.001446
Bancelin, S., Nazac, A., Ibrahim, B. H., Dokládal, P., Decencière, E., Teig, B., et al. (2014). Determination of collagen fiber orientation in histological slides using Mueller microscopy and validation by second harmonic generation imaging. Opt. Express 22 (19), 22561. doi:10.1364/oe.22.022561
Bellini, C., Ferruzzi, J., Roccabianca, S., Di Martino, E. S., and Humphrey, J. D. (2014). A microstructurally motivated model of arterial wall mechanics with mechanobiological implications. Ann. Biomed. Eng. 42 (3), 488–502. doi:10.1007/s10439-013-0928-x
Bersi, M. R., Bellini, C., Wu, J., Montaniel, K. R., Harrison, D. G., and Humphrey, J. D. (2016). Excessive adventitial remodeling leads to early aortic maladaptation in angiotensin-induced hypertension. Hypertension 67, 890–896. doi:10.1161/HYPERTENSIONAHA.115.06262
Cañas, D., García-Herrera, C. M., Herrera, E. A., Celentano, D. J., and Krause, B. J. (2018). Mechanical characterization of arteries affected by fetal growth restriction in Guinea pigs (Cavia porcellus). J. Mech. Behav. Biomed. Mater. 88, 92–101. doi:10.1016/j.jmbbm.2018.08.010
Cardamone, L., Valentín, A., Eberth, J. F., and Humphrey, J. D. (2009). Origin of axial prestretch and residual stress in arteries. Biomechanics Model. Mechanobiol. 8 (6), 431–446. doi:10.1007/s10237-008-0146-x
Carew, T. E., Vaishnav, R. N., and Patel, D. J. (1968). Compressibility of the arterial wall. Circulation Res. 23 (1), 61–68. doi:10.1161/01.RES.23.1.61
Cavinato, C., Badel, P., Krasny, W., Avril, S., and Morin, C. (2020). Experimental characterization of adventitial collagen fiber kinematics using second-harmonic generation imaging microscopy: similarities and differences across arteries, species and testing conditions. Multi-scale Extracell. Matrix Mech. Mechanobiol. 23, 123–164. doi:10.1007/978-3-030-20182-1_5
Chagnon, G., Rebouah, M., and Favier, D. (2015). Hyperelastic energy densities for soft biological tissues: a review. J. Elast. 120, 129–160. doi:10.1007/s10659-014-9508-z
Chuong, C. J., and Eason, G. (1986). On residual stresses in arteries. J. Biomechanical Eng. 108 (2), 189–192. doi:10.1115/1.3138600
Ciarletta, P., Destrade, M., and Gower, A. L. (2016). On residual stresses and homeostasis: an elastic theory of functional adaptation in living matter. Sci. Rep. 6 (1), 24390–24398. doi:10.1038/srep24390
Cyron, C. J., and Humphrey, J. D. (2017). Growth and remodeling of load-bearing biological soft tissues. Meccanica 52 (3), 645–664. doi:10.1007/s11012-016-0472-5
Deniset-Besseau, A., De Sa Peixoto, P., Mosser, G., and Schanne-Klein, M.-C. (2010). Nonlinear optical imaging of lyotropic cholesteric liquid crystals. Opt. Express 18 (2), 1113. doi:10.1364/oe.18.001113
Di Cesare, E., Splendiani, A., Barile, A., Squillaci, E., Di Cesare, A., Brunese, L., et al. (2016). CT and MR imaging of the thoracic aorta. Open Med. (Wars) 11, 143–151. doi:10.1515/med-2016-0028
Fung, Y. C. (1991). What are the residual stresses doing in our blood vessels? Ann. Biomed. Eng. 19 (3), 237–249. doi:10.1007/BF02584301
García-Herrera, C. M., Bustosa, C. A., Celentano, D. J., and Ortega, R. (2016). Mechanical analysis of the ring opening test applied to human ascending aortas. Comput. Methods Biomechanics Biomed. Eng. 19 (16), 1738–1748. doi:10.1080/10255842.2016.1183125
Gasser, T. C., Ogden, R. W., and Holzapfel, G. A. (2006). Hyperelastic modelling of arterial layers with distributed collagen fibre orientations. J. R. Soc. Interface 3, 15–35. doi:10.1098/rsif.2005.0073
Golaraei, A., Mirsanaye, K., Ro, Y., Krouglov, S., Akens, M. K., Wilson, B. C., et al. (2019). Collagen chirality and three-dimensional orientation studied with polarimetric second-harmonic generation microscopy. J. Biophot. 12 (1), e201800241. doi:10.1002/jbio.201800241
Greiner, C., Grainger, S., Farrow, S., Davis, A., Su, J. L., Saybolt, M. D., et al. (2021). Robust quantitative assessment of collagen fibers with picrosirius red stain and linearly polarized light as demonstrated on atherosclerotic plaque samples. PLoS ONE 16 (3), e0248068. doi:10.1371/journal.pone.0248068
Holzapfel, G. A. (2006). Determination of material models for arterial walls from uniaxial extension tests and histological structure. J. Theor. Biol. 238 (2), 290–302. doi:10.1016/j.jtbi.2005.05.006
Holzapfel, G. A., Gasser, T. C., and Ogden, R. W. (2000). A new constitutive framework for arterial wall mechanics and a comparative study of material models. J. Elast. 61 (1-3), 1–48. doi:10.1023/A:1010835316564
Horgan, C. O., and Murphy, J. G. (2020). On the tension-compression switch hypothesis in arterial mechanics. J. Mech. Behav. Biomed. Mater. 103, 103558. doi:10.1016/j.jmbbm.2019.103558
Horný, L., Adámek, T., and Kulvajtová, M. (2017). A comparison of age-related changes in axial prestretch in human carotid arteries and in human abdominal aorta. Biomechanics Model. Mechanobiol. 16 (1), 375–383. doi:10.1007/s10237-016-0797-y
Humphrey, J. D., Dufresne, E. R., and Schwartz, M. A. (2014). Mechanotransduction and extracellular matrix homeostasis. Nat. Rev. Mol. Cell Biol. 15, 802–812. doi:10.1038/NRM3896
Humphrey, J. D., and Rajagopal, K. R. (2002). A constrained mixture model for growth and remodeling of soft tissues. Math. Models Methods Appl. Sci. 12 (3), 407–430. doi:10.1142/S0218202502001714
Jett, S. V., Hudson, L. T., Baumwart, R., Bohnstedt, B. N., Mir, A., Burkhart, H. M., et al. (2020). Integration of polarized spatial frequency domain imaging (pSFDI) with a biaxial mechanical testing system for quantification of load-dependent collagen architecture in soft collagenous tissues. Acta Biomater. 102, 149–168. doi:10.1016/j.actbio.2019.11.028
Karšaj, I., and Humphrey, J. D. (2012). A multilayered wall model of arterial growth and remodeling. Mech. Mater. 44, 110–119. doi:10.1016/j.mechmat.2011.05.006
Karšaj, I., Sorić, J., and Humphrey, J. D. (2010). A 3-D framework for arterial growth and remodeling in response to altered hemodynamics. Int. J. Eng. Sci. 48 (11), 1357–1372. doi:10.1016/j.ijengsci.2010.06.033
Latorre, M., and Humphrey, J. D. (2018). Modeling mechano-driven and immuno-mediated aortic maladaptation in hypertension. Biomechanics Model. Mechanobiol. 17, 1497–1511. doi:10.1007/S10237-018-1041-8
Latorre, M., and Humphrey, J. D. (2020). Fast, rate-independent, finite element implementation of a 3D constrained mixture model of soft tissue growth and remodeling. Comput. Methods Appl. Mech. Eng. 368, 113156. doi:10.1016/j.cma.2020.113156
Latorre, M., Romero, X., and Montáns, F. J. (2016). The relevance of transverse deformation effects in modeling soft biological tissues. Int. J. Solids Struct. 99, 57–70. doi:10.1016/J.IJSOLSTR.2016.08.006
Laubrie, J. D., Mousavi, S. J., and Avril, S. (2022). About prestretch in homogenized constrained mixture models simulating growth and remodeling in patient-specific aortic geometries. Biomechanics Model. Mechanobiol. 2 (21), 455–469. doi:10.1007/s10237-021-01544-3
Maas, S. A., Ellis, B. J., Ateshian, G. A., and Weiss, J. A. (2012). FEBio: finite elements for biomechanics. J. Biomechanical Eng. 134 (1), 011005–011010. doi:10.1115/1.4005694
Maas, S. A., Erdemir, A., Halloran, J. P., and Weiss, J. A. (2016). A general framework for application of prestrain to computational models of biological materials. J. Mech. Behav. Biomed. Mater. 61, 499–510. doi:10.1016/j.jmbbm.2016.04.012
Maes, L., Fehervary, H., Vastmans, J., Mousavi, S. J., Avril, S., and Famaey, N. (2019). Constrained mixture modeling affects material parameter identification from planar biaxial tests. J. Mech. Behav. Biomed. Mater. 95, 124–135. doi:10.1016/j.jmbbm.2019.03.029
Morin, C., and Avril, S. (2015). Inverse problems in the mechanical characterization of elastic arteries. MRS Bull. 40, 317–323. doi:10.1557/mrs.2015.63
Mousavi, S. J., and Avril, S. (2017). Patient-specific stress analyses in the ascending thoracic aorta using a finite-element implementation of the constrained mixture theory. Biomechanics Model. Mechanobiol. 16 (5), 1765–1777. doi:10.1007/s10237-017-0918-2
Mousavi, S. J., Farzaneh, S., and Avril, S. (2019). Patient-specific predictions of aneurysm growth and remodeling in the ascending thoracic aorta using the homogenized constrained mixture model. Biomechanics Model. Mechanobiol. 18 (6), 1895–1913. doi:10.1007/s10237-019-01184-8
Navarrete, A., Chen, Z., Aranda, P., Poblete, D., Utrera, A., García-Herrera, C. M., et al. (2020). Study of the effect of treatment with atrial natriuretic peptide (ANP) and cinaciguat in chronic hypoxic neonatal lambs on residual strain and microstructure of the arteries. Front. Bioeng. Biotechnol. 8, 590488. doi:10.3389/fbioe.2020.590488
Newville, M., and Stensitzki, T. (2018). Non-linear least-squares minimization and curve-fitting for Python, non-linear least-squares minimization and curve-fitting for Python, 65.
Nolan, D. R., and McGarry, J. P. (2016). On the compressibility of arterial tissue. Ann. Biomed. Eng. 44 (4), 993–1007. doi:10.1007/s10439-015-1417-1
Peña, J. A., Martínez, M. A., and Peña, E. (2015). Layer-specific residual deformations and uniaxial and biaxial mechanical properties of thoracic porcine aorta. J. Mech. Behav. Biomed. Mater. 50, 55–69. doi:10.1016/j.jmbbm.2015.05.024
Rachev, A., and Hayashi, K. (1999). Theoretical study of the effects of vascular smooth muscle contraction on strain and stress distributions in arteries. Ann. Biomed. Eng. 27 (4), 459–468. doi:10.1114/1.191
Rivera, E., Canales, C., Pacheco, M., García-Herrera, C., Macías, D., Celentano, D. J., et al. (2021). Biomechanical characterization of the passive response of the thoracic aorta in chronic hypoxic newborn lambs using an evolutionary strategy. Sci. Rep. 11 (1), 13875. doi:10.1038/s41598-021-93267-9
Rivera, E., García-Herrera, C., González-Candia, A., Celentano, D. J., and Herrera, E. A. (2020). Effects of melatonin on the passive mechanical response of arteries in chronic hypoxic newborn lambs. J. Mech. Behav. Biomed. Mater. 112 (12), 104013. doi:10.1016/j.jmbbm.2020.104013
Sigaeva, T., Sommer, G., Holzapfel, G. A., and Di Martino, E. S. (2019). Anisotropic residual stresses in arteries. J. R. Soc. Interface 16 (151), 20190029. doi:10.1098/rsif.2019.0029
Skacel, P., and Bursa, J. (2019). Compressibility of arterial wall – direct measurement and predictions of compressible constitutive models. J. Mech. Behav. Biomed. Mater. 90, 538–546. doi:10.1016/j.jmbbm.2018.11.004
Valentín, A., Humphrey, J. D., and Holzapfel, G. A. (2011). A multi-layered computational model of coupled elastin degradation, vasoactive dysfunction, and collagenous stiffening in aortic aging. Ann. Biomed. Eng. 39 (7), 2027–2045. doi:10.1007/s10439-011-0287-4
Valentín, A., Humphrey, J. D., and Holzapfel, G. A. (2013). A finite element-based constrained mixture implementation for arterial growth, remodeling, and adaptation: theory and numerical verification. Int. J. Numer. Methods Biomed. Eng. 29 (8), 822–849. doi:10.1002/cnm.2555
Wang, R., and Gleason, R. L. (2010). A mechanical analysis of conduit arteries accounting for longitudinal residual strains. Ann. Biomed. Eng. 38 (4), 1377–1387. doi:10.1007/s10439-010-9916-6
Wang, Y., Zeinali-Davarani, S., and Zhang, Y. (2016). Arterial mechanics considering the structural and mechanical contributions of ECM constituents. J. Biomechanics 49 (12), 2358–2365. doi:10.1016/j.jbiomech.2016.02.027
Weisbecker, H., Pierce, D. M., and Holzapfel, G. A. (2014). A generalized prestressing algorithm for finite element simulations of preloaded geometries with application to the aorta. Int. J. Numer. Methods Biomed. Eng. 30 (9), 857–872. doi:10.1002/cnm.2632
Wells, S. M., Langille, B. L., Lee, J. M., and Adamson, S. L. (1999). Determinants of mechanical properties in the developing ovine thoracic aorta. Am. J. Physiology - Heart Circulatory Physiology 277 (4 46-4), H1385–H1391. doi:10.1152/ajpheart.1999.277.4.h1385
Wu, J., Thabet, S. R., Kirabo, A., Trott, D. W., Saleh, M. A., Xiao, L., et al. (2014). Inflammation and mechanical stretch promote aortic stiffening in hypertension through activation of p38 mitogen-activated protein kinase. Circulation Res. 114, 616–625. doi:10.1161/CIRCRESAHA.114.302157
Yosibash, Z., Manor, I., Gilad, I., and Willentz, U. (2014). Experimental evidence of the compressibility of arteries. J. Mech. Behav. Biomed. Mater. 39, 339–354. doi:10.1016/j.jmbbm.2014.07.030
Keywords: constrained mixture, arterial wall, deposition stretch, material parameters, finite element method
Citation: Navarrete Á, Utrera A, Rivera E, Latorre M, Celentano DJ and García-Herrera CM (2023) An inverse fitting strategy to determine the constrained mixture model parameters: application in patient-specific aorta. Front. Bioeng. Biotechnol. 11:1301988. doi: 10.3389/fbioe.2023.1301988
Received: 25 September 2023; Accepted: 06 November 2023;
Published: 20 November 2023.
Edited by:
Hao Gao, University of Glasgow, United KingdomReviewed by:
Daniela Valdez-Jasso, University of California, San Diego, United StatesMIljan Milosevic, University of Kragujevac, Serbia
Copyright © 2023 Navarrete, Utrera, Rivera, Latorre, Celentano and García-Herrera. This is an open-access article distributed under the terms of the Creative Commons Attribution License (CC BY). The use, distribution or reproduction in other forums is permitted, provided the original author(s) and the copyright owner(s) are credited and that the original publication in this journal is cited, in accordance with accepted academic practice. No use, distribution or reproduction is permitted which does not comply with these terms.
*Correspondence: Claudio M. García-Herrera, Y2xhdWRpby5nYXJjaWFAdXNhY2guY2w=