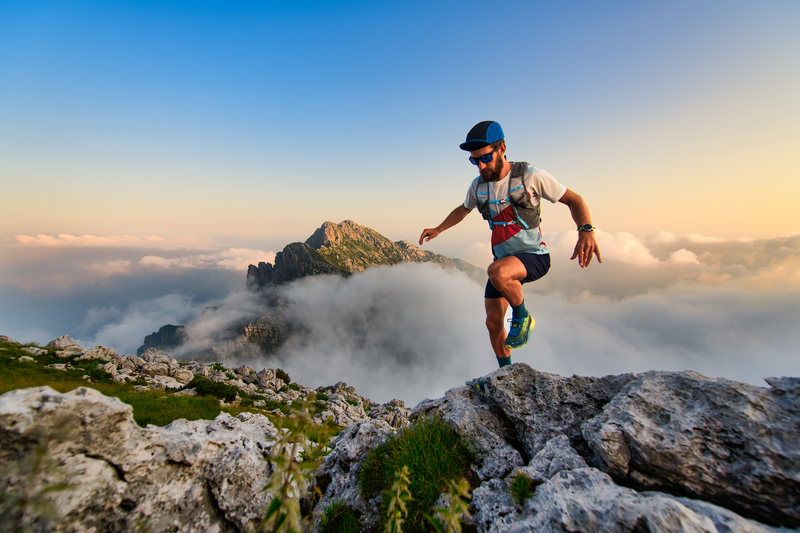
94% of researchers rate our articles as excellent or good
Learn more about the work of our research integrity team to safeguard the quality of each article we publish.
Find out more
EDITORIAL article
Front. Bioeng. Biotechnol. , 01 August 2023
Sec. Biosensors and Biomolecular Electronics
Volume 11 - 2023 | https://doi.org/10.3389/fbioe.2023.1239031
This article is part of the Research Topic Biomedical Engineering Technologies and Methods in Antenatal Medicine View all 5 articles
Editorial on the Research Topic
Biomedical engineering technologies and methods in antenatal medicine
Although pregnancy by itself should not be considered a pathological condition or a life-threatening event, the antepartum period is usually a very critical time window for both mothers and their fetuses. Pregnancy and consequently the complex interactions taking place at the level of the mother-fetus system are characterized by a peculiar trait: fast and continuous evolution in time. As a matter of fact the fetus passes from 2 cells to 3 kilos in about 9 months and the mother’s body must rapidly adapt. Therefore, careful monitoring of both subjects before delivery and during labor has been reported as an effective tool for preventing a set of possible unfavorable outcomes (Grivell et al., 2012).
In principles, a continuous tracking of pregnancy evolution in time would be the best way to proper address maternal and fetal wellbeing, however, even in the most developed countries, healthcare systems foresee an average of 3 monitoring sessions during pregnancy. Hence, these examinations are basically a series of snapshots of mother/fetus wellbeing, far apart one each other.
In this context, it is desirable to design medical devices and software tools capable of providing an effective check-up of pregnant women in several kinds of environment and daily life situations. The aim is to establish reliable health assessments in both acute (bleeding, severe nausea, abdominal pain), or non-acute situations of distress (preeclampsia, gestational diabetes, sporadic contraction, decline in fetus’ activity), for both mothers and fetuses and, eventually, to prevent them.
This Research Topic of Frontiers in Bioengineering and Biotechnology aimed at presenting new solutions for antenatal medicine from both the technological and methodological sides.
Four papers were accepted and published, covering several aspects of pre-natal monitoring.
Three of them deal with the most used fetal monitoring technology, the Cardiotocography (CTG), and try to provide new methodological tools for better identifying fetal critical conditions both in the antenatal period and during labour.
In particular, Ribeiro et al. present an exploratory work, which aims to develop intrapartum predictive models of perinatal asphyxia based on clinical parameters and fetal heart rate (fHR) indices. Perinatal asphyxia is one of the most frequent causes of neonatal mortality. Cardiotocographic signals (CTGs) in association with various clinical parameters were employed for the development of Binary logistic regression (BLR) and Naive-Bayes (NB) models. In the analyzed data (517 cases, with 15 pathological cases), he BLR model shows the best performance in predicting perinatal asphyxia.
Feng et al. propose to model intrapartum CTG from a dynamical system perspective. Empirical dynamic modeling with Gaussian processes is used, which is a Bayesian nonparametric approach for function estimation. The capacity of Gaussian processes allows for revealing causal relationships between time series. Results on real CTG recordings show that fetal heart rate (FHR) and uterine activity (UA) signals are causally related. The authors emphasize that this causal relationship and the estimated attractor manifolds can be exploited for applications in the computerized analysis of CTG records.
The contribution by Spairani et al. proposes a hybrid Machine Learning approach based on a neural architecture that receives heterogeneous data in input (quantitative parameters and images built from fHR signal) for classifying healthy and pathological fetuses. Improvements in the classification performance are achieved by setting a supervised network owing two connected branches, consisting respectively of a Multi-Layer Perceptron (MLP) and a Convolutional Neural Network (CNN). The classification system was trained with a considerable number of fHR tracings, 7,000 healthy and 7,000 pathological, recorded during prenatal ambulatory non stress tests. The classification network reaches an overall promising accuracy of 80.1%.
The fourth paper considers multi-channel fetal monitoring by means of abdominal ECG. This technology, with respect to classical US CTG fetal monitoring, can be used for long term monitoring during everyday life of the pregnant woman through wearable systems. However, fECG should be extracted from signals containing also maternal ECG, and this process implies both to select the best leads for fECG and to apply robust and reliable algorithms for its separation from maternal ECG. Baldazzi et al. propose a machine learning approach for the Signal Quality Assesment that drives channel selection to feed fully featured fECG extraction algorithms. This approach can enhance fetal QRS detection performance by identifying the most-informative channels in high-density recordings, or the best electrode positioning from repeated measurements with a low number of channels.
The research and development work presented in the papers of this special section demonstrates that advanced signal processing and wearable technologies are making big strides toward the assessment of a quantitative approach in the field of antenatal medicine. Despite a qualitative evaluation of fetal monitoring is still the most used approach in the clinical practice, we have recently witnessed growing interest and significant developments in methods focused on extracting quantitative features from fECG and/or CTG signals. We trust that the readers of Frontiers in Bioengineering and Biotechnology will find the material presented in this Research Topic to be stimulating and illustrating a good picture of the state of the art in the field.
All authors listed have made a substantial, direct, and intellectual contribution to the work and approved it for publication.
The authors declare that the research was conducted in the absence of any commercial or financial relationships that could be construed as a potential conflict of interest.
All claims expressed in this article are solely those of the authors and do not necessarily represent those of their affiliated organizations, or those of the publisher, the editors and the reviewers. Any product that may be evaluated in this article, or claim that may be made by its manufacturer, is not guaranteed or endorsed by the publisher.
Keywords: fetal monitoring, fetal heart rate analysis, cardiotocography (CTG), signal processing, machine learning methods
Citation: Magenes G and Signorini MG (2023) Editorial: Biomedical engineering technologies and methods in antenatal medicine. Front. Bioeng. Biotechnol. 11:1239031. doi: 10.3389/fbioe.2023.1239031
Received: 12 June 2023; Accepted: 19 July 2023;
Published: 01 August 2023.
Edited by:
Shi Yuan, Children’s Hospital of Chongqing Medical University, ChinaReviewed by:
Micaela Morettini, Marche Polytechnic University, ItalyCopyright © 2023 Magenes and Signorini. This is an open-access article distributed under the terms of the Creative Commons Attribution License (CC BY). The use, distribution or reproduction in other forums is permitted, provided the original author(s) and the copyright owner(s) are credited and that the original publication in this journal is cited, in accordance with accepted academic practice. No use, distribution or reproduction is permitted which does not comply with these terms.
*Correspondence: Giovanni Magenes, Z2lvdmFubmkubWFnZW5lc0B1bmlwdi5pdA==
†These authors have contributed equally to this work
Disclaimer: All claims expressed in this article are solely those of the authors and do not necessarily represent those of their affiliated organizations, or those of the publisher, the editors and the reviewers. Any product that may be evaluated in this article or claim that may be made by its manufacturer is not guaranteed or endorsed by the publisher.
Research integrity at Frontiers
Learn more about the work of our research integrity team to safeguard the quality of each article we publish.