- 1Great Lakes Bioenergy Research Center, University of Wisconsin-Madison, Madison, WI, United States
- 2Wisconsin Energy Institute, University of Wisconsin-Madison, Madison, WI, United States
- 3Department of Civil and Environmental Engineering, University of Wisconsin-Madison, Madison, WI, United States
- 4Department of Civil and Environmental Engineering, University of Vermont, Burlington, VT, United States
- 5Department of Bacteriology, University of Wisconsin-Madison, Madison, WI, United States
The liquid residue resulting from various agroindustrial processes is both rich in organic material and an attractive source to produce a variety of chemicals. Using microbial communities to produce chemicals from these liquid residues is an active area of research, but it is unclear how to deploy microbial communities to produce specific products from the different agroindustrial residues. To address this, we fed anaerobic bioreactors one of several agroindustrial residues (carbohydrate-rich lignocellulosic fermentation conversion residue, xylose, dairy manure hydrolysate, ultra-filtered milk permeate, and thin stillage from a starch bioethanol plant) and inoculated them with a microbial community from an acid-phase digester operated at the wastewater treatment plant in Madison, WI, United States. The bioreactors were monitored over a period of months and sampled to assess microbial community composition and extracellular fermentation products. We obtained metagenome assembled genomes (MAGs) from the microbial communities in each bioreactor and performed comparative genomic analyses to identify common microorganisms, as well as any community members that were unique to each reactor. Collectively, we obtained a dataset of 217 non-redundant MAGs from these bioreactors. This metagenome assembled genome dataset was used to evaluate whether a specific microbial ecology model in which medium chain fatty acids (MCFAs) are simultaneously produced from intermediate products (e.g., lactic acid) and carbohydrates could be applicable to all fermentation systems, regardless of the feedstock. MAGs were classified using a multiclass classification machine learning algorithm into three groups, organisms fermenting the carbohydrates to intermediate products, organisms utilizing the intermediate products to produce MCFAs, and organisms producing MCFAs directly from carbohydrates. This analysis revealed common biological functions among the microbial communities in different bioreactors, and although different microorganisms were enriched depending on the agroindustrial residue tested, the results supported the conclusion that the microbial ecology model tested was appropriate to explain the MCFA production potential from all agricultural residues.
1 Introduction
Finding ways to generate chemicals and chemical precursors from renewable sources is an important step towards creating a sustainable circular economy that decreases society’s dependance on fossil fuels. Medium chain fatty acids (MCFAs) are one such class of product that can be microbially produced, have applications in lubricant synthesis, production of herbicides and antimicrobials, and can be further processing into additional chemicals (Sarria et al., 2017; Scarborough et al., 2018b). Microbes and microbial communities can produce MCFAs using a wide variety of carbohydrate-rich substrates, making biological MCFA production an attractive target due to the widespread availability of carbohydrate-rich organic wastes that can be used as substrates, such as undistilled corn beer (Ge et al., 2015), thin stillage (Fortney et al., 2021), lignocellulosic fermentation conversion residues (Scarborough et al., 2018a; Scarborough et al., 2018b), a soluble fraction of municipal solid waste (Grootscholten et al., 2013; Grootscholten et al., 2014) and winery residue (Kucek et al., 2016b). In addition to MCFAs, other fermentation products have been identified as coproduced by microbial communities that generate MCFAs from various substrates, including the accumulation of acetic, lactic, succinic, and butyric acids, as well as ethanol (Han et al., 2018; Fortney et al., 2021). Lactic, succinic, and butyric acids can be used as building blocks for materials such as bioplastics (Harmsen et al., 2014). Further, both lactic acid and ethanol have been shown to be intermediate metabolites during MCFA production by members of microbial communities that perform reverse ß-oxidation, also known as chain elongation (Agler et al., 2012; Zhu et al., 2015; Kucek et al., 2016a; Han et al., 2018). Although most MCFA production research has been conducted with microbial communities, it is not clear how to steer a community towards maximizing MCFA production without accumulation of other fermentation products, or how to harness the microbial community to produce primarily one fermentation product. Therefore, additional knowledge is needed to enable the engineering of microbial communities to produce the desired fermentation products. We are interested in generating models that can explain and possibly predict the relationship of microbial community structure with the type of carbohydrate-rich substrates and the type of fermentation products that accumulate.
An emerging microbial ecology model describes three main functions in a chain elongation microbiome; one group of microbes that can ferment carbohydrates to lactic acid but cannot perform chain elongation, other microbes that can perform chain elongation using lactic acid as an electron donor, and others that can perform chain elongation directly from carbohydrates (Scarborough et al., 2018a). This model, initially proposed based on experiments using xylose-rich organic residues from lignocellulosic ethanol production (Scarborough et al., 2018a), has been suggested for other substrates (Crognale et al., 2021; Fortney et al., 2021; Ingle et al., 2021), and there is emerging evidence of MCFA-producing microbes with the genomic capacity for producing MCFA from both lactic acid and carbohydrates (Kang et al., 2022; Wang et al., 2022). In other cases, it is proposed that ethanol can be used as an electron donor and act as an intermediate during MCFA production (Agler et al., 2012; Kucek et al., 2016a). To evaluate whether this microbial ecology model can be generalized to conceptually explain MCFA production from a variety of carbohydrate-rich organic residues, we evaluated the microbial communities that were enriched when the same inoculum was used in bioreactor experiments that fermented several agroindustrial residues, including thin stillage from starch ethanol production (Fortney et al., 2021; Fortney et al., 2022), thin stillage from cellulosic ethanol production (Scarborough et al., 2018a; Scarborough et al., 2020), xylose (Scarborough et al., 2022), dairy manure hydrolysate (Ingle et al., 2021; Ingle et al., 2022), and ultrafiltered milk permeate (Walters et al., 2022; Walters et al., 2023). In all cases, the inoculum was from an acid-phase anaerobic digester at the local wastewater treatment plant (Madison, WI, United States).
Here we present the comparison of metagenome assembled genomes (MAGs) from these bioreactors and examine the role of different microbial groups in the fermentation and chain elongation processes. For this analysis, we developed a script to identify genes encoding key metabolic enzymes in the MAGs and a machine learning algorithm to bin each MAG into relevant categories. This analysis revealed patterns showing that in fermentations in which MCFA is the primary product that accumulates, and the feedstock is a carbohydrate-rich substrate, the microbial ecology model that describes chain elongation occurring via utilization of intermediates or direct utilization of carbohydrates is applicable, even though different microorganisms were enriched depending on the agroindustrial residue tested.
2 Materials and methods
2.1 Metagenome assembled genome (MAG) sources
MAG data was obtained from previously published lab-scale bioreactor studies of microbial communities grown with various agroindustrial residues (Scarborough et al., 2018a; Scarborough et al., 2020; Fortney et al., 2021; Ingle et al., 2021; Fortney et al., 2022; Ingle et al., 2022; Scarborough et al., 2022; Walters et al., 2022). The operational conditions of the bioreactors are summarized in Table 1 and additional information on sample collection can be found in the respective publications. MAGs were obtained from the inoculum source (two samples, 10 MAGs) (Ingle et al., 2022) and bioreactors fed cellulosic ethanol thin stillage (six samples, 10 MAGs) (Scarborough et al., 2018a; Scarborough et al., 2020), synthetic medium containing xylose as the primary carbon source (three samples, 8 MAGs) (Scarborough et al., 2022), hydrolysate from dairy manure (four samples, 38 MAGs) (Ingle et al., 2021; Ingle et al., 2022), ultra-filtered milk permeate (34 samples, 123 MAGs) (Walters et al., 2022; Walters et al., 2023), and starch ethanol thin stillage (31 samples, 51 MAGs) (Fortney et al., 2021; Fortney et al., 2022). In all cases, only the best-quality representative MAGs determined in each study were used. In total, we used an initial dataset of 240 MAGs from 80 total samples (Table S1).
2.2 MAG dereplication and taxonomic classification
The program dRep (v3.2.2; dereplicate command) (Olm et al., 2017) was used to identify redundant MAGs using default settings, except -conW was set to 0.5 and -N50W was set to 5. This reduced the total MAG number from 240 to 217 non-redundant MAGs (Supplementary Table S2). CheckM (v1.0.11; lineage_wf and qa commands with default parameters) (Parks et al., 2015) was used to determine relevant quality parameters for each of the 217 MAGs (Supplementary Table S2). All 217 MAGs were taxonomically classified using GTDB-Tk (v1.5.1; database release 202; classify_wf command with default parameters) (Supplementary Table S3).
2.3 Alignment and relative abundance calculations
To predict the relative abundance of microorganisms represented by the 217-MAG dataset in samples from the different bioreactors, the genome FASTA files of all the MAGs were concatenated, and then Bowtie2 (v2.2.2 with default parameters) (Langmead and Salzberg, 2012) was used to align the FASTQ sequencing files. Resulting SAM files were converted into BAM files and sorted using samtools (v1.15.1; view and sort commands with default parameters) (Li et al., 2009). CoverM (v0.4.0; coverm genome command with default parameters) (https://github.com/wwood/CoverM) was used to generate relative abundance statistics of mapped reads in the sorted BAM files (Supplementary Table S2). We identified 131 MAGs with at least 1% relative abundance in at least one sample across all experiments, which we define as the high-abundance MAG dataset (Supplementary Table S4). A relative abundance of 1% has been used previously as an abundance threshold (Fitzgerald et al., 2015; Scarborough et al., 2018a; Scarborough et al., 2018b; Scarborough et al., 2020).
2.4 Phylogenetic analyses
Maximum likelihood phylogenetic trees were generated using RAxML-NG (v0.9.0; model LG + G8+F) (Kozlov et al., 2019) using 1,000 bootstraps. GTDB-Tk (v1.5.1; database release 202; ani_rep command with default parameters) (Chaumeil et al., 2019) was used to identify closest related genomes, which were downloaded from NCBI. The MAGs and closest genomes were compared using GTDB-Tk (identify and align commands with default parameters) using a set of 120 bacterial single-copy marker genes (Bac120) for all trees. Prevotella intermedia (GCF_001953955.1) was used as an outgroup to root the trees.
An additional analysis was performed to compare homologs of subunit B of the electron transfer flavoprotein (EtfB). For this, EtfB homologs were identified using known protein sequences (Walters et al., 2023) and tBLASTn (v2.8.1, default parameters) (Camacho et al., 2009) with “pident” (percent identity to the query sequence) > 25% and “qcovhsp” (coverage of the query sequence) > 70%. EtfB homologs were aligned using MUSCLE (v3.8.31, default parameters) and a phylogenetic tree was constructed using RAxML-NG using 500 boostraps. All files used in this analysis are available on GitHub (https://github.com/GLBRC/agroindustrial_residue_metagenomics).
2.5 Non-metric multidimensional scaling plots
Non-metric multidimensional scaling (NMDS) plots were generated from the relative abundance calculations for the 217 non-redundant MAGs using R (v4.1.0) (Core Team, 2018). Specifically, the vegdist command with the “bray” index (from the vegan package, v2.6-4) was used to determine the distance metrics and the metaMDS command (from the vegan package, v2.6-4) was used to generate the NMDS values. Plots were constructed using ggplot2 (Wickham, 2016) from the NMDS values and edited for clarity using Adobe Illustrator (v27.2). Statistical comparisons were performed using permutation-based multivariate analysis of variance (PerMANOVA) via the adonis command (from the vegan package, v2.6-4) with “euclidean” distance and the Benjamini-Hochberg adjustment (adjusted p-value <0.05 accepted as significant) (Benjamini and Hochberg, 1995; Anderson, 2017). The R script used to generate the NMDS plot is available on GitHub (GitHub page: https://github.com/GLBRC/agroindustrial_residue_metagenomics).
2.6 Homology-based gene identification
A homology-based analysis was performed to identify genes encoding enzymes of fermentation and central carbon metabolism in each MAG. The query protein sequences used were manually vetted through either EcoCyc (Keseler et al., 2011), MetaCyc (Caspi et al., 2020), SWISS-PROT via UniProtKB (Boutet et al., 2016), or other published datasets. Query protein amino acid sequences and metadata were downloaded from the UniProtKB database. tBLASTn (v2.8.1) (Camacho et al., 2009) was used to identify homologs using default parameters. Subject sequences that had an e-value less than 1 × 10−10, a “pident” (percent identity to the query sequence) value greater than 25%, and a “qcovhsp” (coverage of the query sequence) value greater than 70% were used to determine gene homologs (Supplementary Table S5). All files and scripts are available on GitHub (GitHub page: https://github.com/GLBRC/agroindustrial_residue_metagenomics).
2.7 Multiclass classification machine learning algorithm
MAGs were classified into four functional groups. The first group, “Ferment to Intermediates”, consists of MAGs that ferment carbohydrates into intermediate extracellular products, such as ethanol or lactic acid. The second group, “Intermediate Chain Elongators”, consists of MAGs that convert intermediate extracellular products (e.g., ethanol or lactic acid) into medium chain fatty acids (MCFAs) using reverse ß-oxidation. The third group, “Carbohydrate Chain Elongators”, consists of MAGs that ferment carbohydrates directly into MCFAs. A fourth group, “uninvolved”, was used to bin MAGs that could not be classified into the three functional groups.
Multiclass classification machine learning was utilized to categorize the MAGs based on gene homologs of key fermentation pathways that were detected. A training set was constructed using organisms known to fit into one of the four groups (Supplementary Table S6). Bifidobacterium species and lactic acid bacteria were used for the Ferment to Intermediates training set (Okada et al., 1979; Pokusaeva et al., 2011; Pruckler et al., 2015; Tanner et al., 2016; Eckel and Vogel, 2020; Ferrero et al., 2021; Kasmaei et al., 2022; Ksiezarek et al., 2022), Clostridium and Megasphaera species were used for the Intermediate Chain Elongators training set (Wallace et al., 2003; Seedorf et al., 2008; Jeon et al., 2017; Kobayashi et al., 2017; Tao et al., 2017; Yang et al., 2018; Yoshikawa et al., 2018; Litty and Muller, 2021), Caproicibacter and Roseburia species were used for the Carbohydrate Chain Elongators training set (Kim et al., 2015; Tamanai-Shacoori et al., 2017; Flaiz et al., 2020; Schoelmerich et al., 2020), and Acetobacter, Prevotella, and Sphaerochaeta species were used for the uninvolved training set.
Multiple multiclass classification machine learning algorithms were tested using the auto_ml module (v2.9.10) (https://github.com/ClimbsRocks/auto_ml). The algorithms tested against baseline were Decision Tree (Pedregosa et al., 2011), Random Forest (Pedregosa et al., 2011), Linear Regression (Pedregosa et al., 2011), XGBoost (https://xgboost.readthedocs.io/en/stable/index.html), Neural Network (Pedregosa et al., 2011), Nearest Neighbors (Pedregosa et al., 2011), Extra Trees (Pedregosa et al., 2011), CatBoost (Prokhorenkova et al., 2018), and LightGBM (Zhang et al., 2017). The machine learning algorithms were evaluated for correct classification of training set genomes into functional groups using multiple analyses: the logloss metric (-log(p), where p is the probability of correctly categorizing the training set) (Bian and Tao, 2011) for each algorithm compared to the baseline value of no algorithm, precision-recall (PR) curves for each algorithm and receiver operating characteristic (ROC) curves for each algorithm (Haibo and Garcia, 2009). These evaluations showed that using the LightGBM model provided the largest decrease in logloss metric (a 99.91% improvement compared to baseline alone) while maximizing true positives and minimizing false positives. The script, files used for the machine learning analysis, and the results of the multiclass classification machine learning analysis are available on GitHub (GitHub page: https://github.com/GLBRC/agroindustrial_residue_metagenomics).
2.9 Hierarchical clustering
MAGs were classified into predicted functional groups using hierarchical clustering based on the detected genes in metabolic pathways important in MCFA production (Walters et al., 2023). Hierarchical clustering was performed in R (v4.1.0) (Core Team, 2018) using the gplots R package (v3.1.3, heatmap.2 command with default parameters, https://github.com/talgalili/gplots/). MAGs were classified using the hierarchical clustering results in the Ferment to Intermediates group if they had high percentage of genes detected in the bifid shunt or phosphoketolase pathways and low percentage of genes detected in the lactic acid utilization and reverse ß-oxidation pathways, in the Intermediate Chain Elongators group if they had low percentage of genes detected in the bifid shunt or phosphoketolase pathways and high percentage of genes detected in the lactic acid utilization and reverse ß-oxidation pathways, and in the Carbohydrate Chain Elongators group if they had low percentage of genes detected in the bifid shunt, phosphoketolase, and lactic acid utilization pathways but high percentage of genes detected in the reverse ß-oxidation pathway (Supplementary Table S2). The script, files used, and results of this analysis are available on GitHub (GitHub page: https://github.com/GLBRC/agroindustrial_residue_metagenomics).
3 Results
3.1 Analysis of the non-redundant MAG dataset
For this study we used MAGs assembled from 10 different bioreactors that were fed various agroindustrial residues (Figure 1). The microbial communities that were enriched in these bioreactors originated from the same inoculum source, an acid-phase anaerobic digester used in the solids handling treatment train at the local wastewater treatment plant (Madison, WI, United States). In addition to the type of agroindustrial residue used as the feedstock, parameters such as temperature and pH were also different in some bioreactor experiments (Table 1). Bioreactor performance has been described elsewhere for a bioreactor fed xylose-rich thin stillage from cellulosic ethanol production (Scarborough et al., 2018b), one fed a carbohydrate-rich hydrolysate created from chemical pretreatment of dairy manure (Ingle et al., 2021), five bioreactors fed thin stillage from starch ethanol biorefining (Fortney et al., 2021), and one bioreactor fed lactose-rich ultra-filtered milk permeate (Walters et al., 2023). Two additional bioreactors complete the set of 10 bioreactors used in this study; one fed a xylose-rich synthetic medium and a second one operated with ultra-filtered milk permeate as the feedstock. The MAGs assembled from all of the bioreactors have been reported and are publicly available (Scarborough et al., 2018a; Fortney et al., 2022; Ingle et al., 2022; Scarborough et al., 2022; Walters et al., 2022). The main fermentation products that accumulated in the medium of these bioreactors include lactic and succinic acids, ethanol, as well as the short chain fatty acids (SCFAs) acetic and propionic acids and the MCFAs butyric, hexanoic, and octanoic acids (Figure 2).
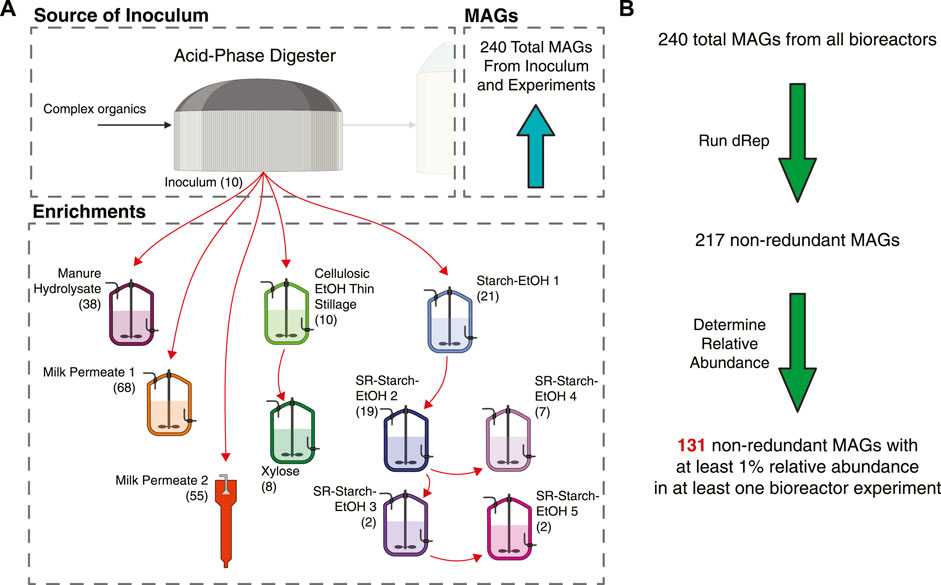
FIGURE 1. Overview of bioreactors operated with the different agroindustrial feedstocks and their contribution to the non-redundant MAG dataset. (A) Graphical overview of inoculum source and enrichments with different feedstocks, indicating the number of MAGs assembled from each source. All reactors were completely mixed flow-through reactors, except for Milk Permeate 2, which was an upflow sludge blanket reactor. See Table 1 for operational conditions. (B) Flow chart indicating how the MAGs were filtered for this work. From a total of 240 MAGs, dRep (Olm et al., 2017) was used to identify redundant MAGs and define a set of 217 non-redundant MAGs. Abundance was then used to define a set of 131 high-abundance and non-redundant MAGs.
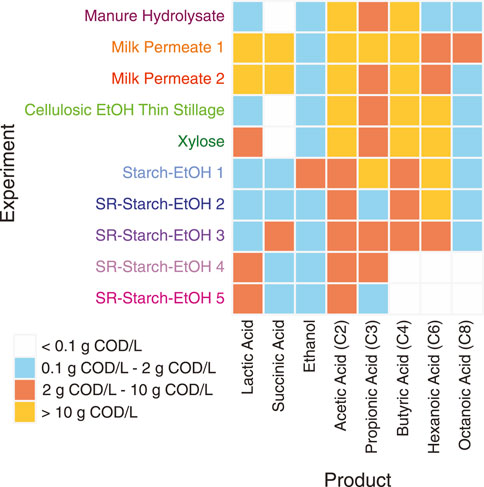
FIGURE 2. Summary of extracellular fermentation products that accumulated in the bioreactors. Product concentrations, measured in chemical oxygen demand (COD) per liter, are summarized into four groups, indicating maximum concentrations measured during the course of the experiments: <0.1 g COD/L (light grey), between 0.1 and 2 g COD/L (blue), between 2 g COD/L and 10 g COD/L (red), >10 g COD/L (yellow).
Combined, there are a total of 240 MAGs across these bioreactors (Figure 1B; Supplementary Table S1). Given the similarities in the inoculum source and in the accumulated fermentation products, we hypothesized that the MAGs assembled from these microbial communities would have a high degree of similarity. However, when the program dRep (Olm et al., 2017) was used to identify MAGs with at least 99% average nucleotide identity (ANI), only 23 MAGs were highly similar among the 240 MAGs (Figure 1B; Table S1). This dereplication analysis resulted in a library of 217 non-redundant MAGs that we used to further evaluate the microbial communities in the bioreactors (Supplementary Table S2).
This collection of 217 non-redundant MAGs represented median relative abundances ranging from 63.5% to 90.3% in the bioreactor samples, but a median relative abundance of only 11.6% for the inoculum (Table 2). The low percentage for the inoculum indicates that most of the 217 MAGs in the library represented microbial community members that were not abundant in the acid-phase digester inoculum, but were instead enriched during the operation of the bioreactors.
A non-metric multidimensional scaling (NMDS) analysis of the relative abundance of MAGs in the analyzed samples reveals divergence in the microorganisms that were enriched during growth in the different agroindustrial residues (Figure 3). The lack of overlap of the abundant MAGs among agroindustrial residue media used indicates that the media played a large role in shaping the microbial communities in these bioreactors. The dataset includes samples collected from bioreactors operated with the same agroindustrial residue but different operational conditions. In these cases, the NDMS plot suggests that agroindustrial residue used had a larger impact in shaping the microbial community compared to the operational condition. For example, several bioreactors were operated using starch ethanol thin stillage (Fortney et al., 2021), and in the NDMS plot (Figure 3) the samples from these bioreactors clustered together and separate from the samples from bioreactors that used other agroindustrial residues (adjusted p-value <0.05). The dataset also includes samples collected from bioreactors operated under identical conditions but receiving different agroindustrial residues. This is the case for the Milk Permeate 1, Xylose, and the Starch-EtOH 1 experiments (Figure 3). Although they were all operated under identical conditions, there is no overlap of the abundant MAGs from these reactors in the NDMS plot (adjusted p-value <0.05), supporting the argument that the agroindustrial residue used had a larger impact in the microbial communities than the operational conditions used.
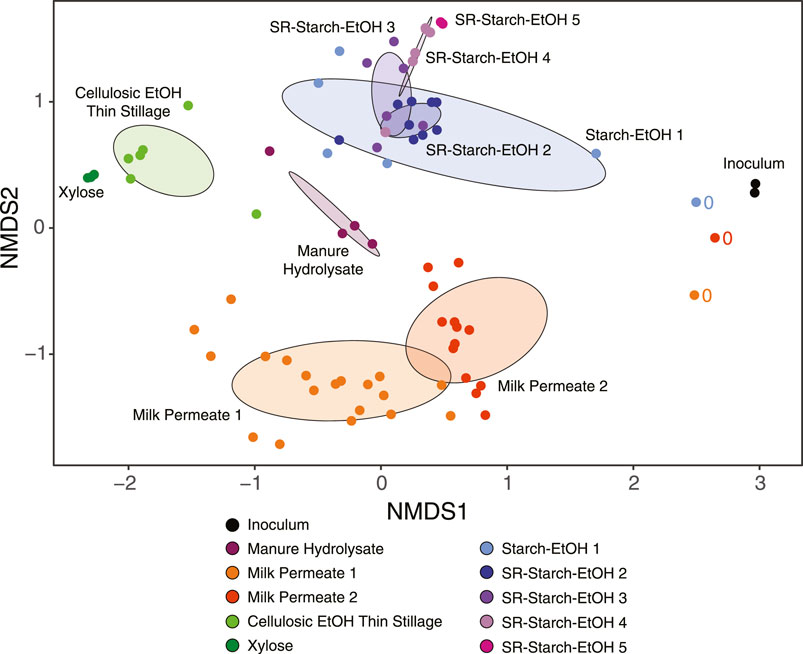
FIGURE 3. Non-metric multidimensional scaling (NMDS) plot of the relative abundances of the microbial communities using the 217 non-redundant MAGs across all experiments over all measured time points (stress value 0.17). Samples from different bioreactor experiments are color coded according to the key. Ovals represent the standard deviation of the average value for all samples from each bioreactor experiment and are color coded according to the key. Samples that were taken at the time of inoculation are marked with ‘0’. See Table 1 for description of bioreactor operational conditions and definition of experiment names.
The set of non-redundant MAGs has a diverse composition (Table 3; Supplementary Table S3), with MAGs belonging to eight phyla and 12 families within these phyla. 24 MAGs were classified to the genus level based on the coverage in the metagenomic data sets. In addition, this non-redundant set includes MAGs assembled with short-read Illumina (149 MAGs) and long-read PacBio technologies (68 MAGs). Estimates of completion and contamination in this dataset are greater than 75% and less than 7.5%, respectively. The MAGs resulting from Illumina sequencing had assemblies with 1–558 contigs, whereas the MAGs obtained from PacBio sequencing were assembled in 1–44 contigs (Table 3; Supplementary Table S2).
3.2 Enzymes in metabolic pathways identified in the non-redundant MAG dataset
We sought to make predictions on the role of different members of the microbial communities enriched in the bioreactors and to evaluate the microbial ecology model for MCFA production that hypothesizes the presence of some community members that produce MCFA directly from carbohydrates (Carbohydrate Chain Elongators), other community members that produce MCFA from lactic acid or ethanol as intermediate fermentation products (Intermediate Chain Elongators), and other community members that produce these intermediate products but do not perform chain elongation (Ferment to Intermediates) (Scarborough et al., 2018a). To this end, we queried the MAGs for the presence of homologs of individual proteins present in different fermentation pathways (Figure 4; Supplementary Table S5) (Walters et al., 2023). This allowed categorization of MAGs by association of similar patterns of the presence of homologous proteins from each metabolic pathway examined. Using the hierarchical clustering of the MAGs based on the percentage of homologs present per pathway, we categorized the MAGs into the functional groups. Based on this analysis, 79 MAGs are predicted to ferment carbohydrates to intermediate products (Ferment to Intermediates), 59 MAGs are predicted to produce MCFA from the intermediate products (Intermediate Chain Elongators), and 13 MAGs are predicted to produce MCFA from carbohydrates (Carbohydrate Chain Elongators, Figure 4; Supplementary Table S2).
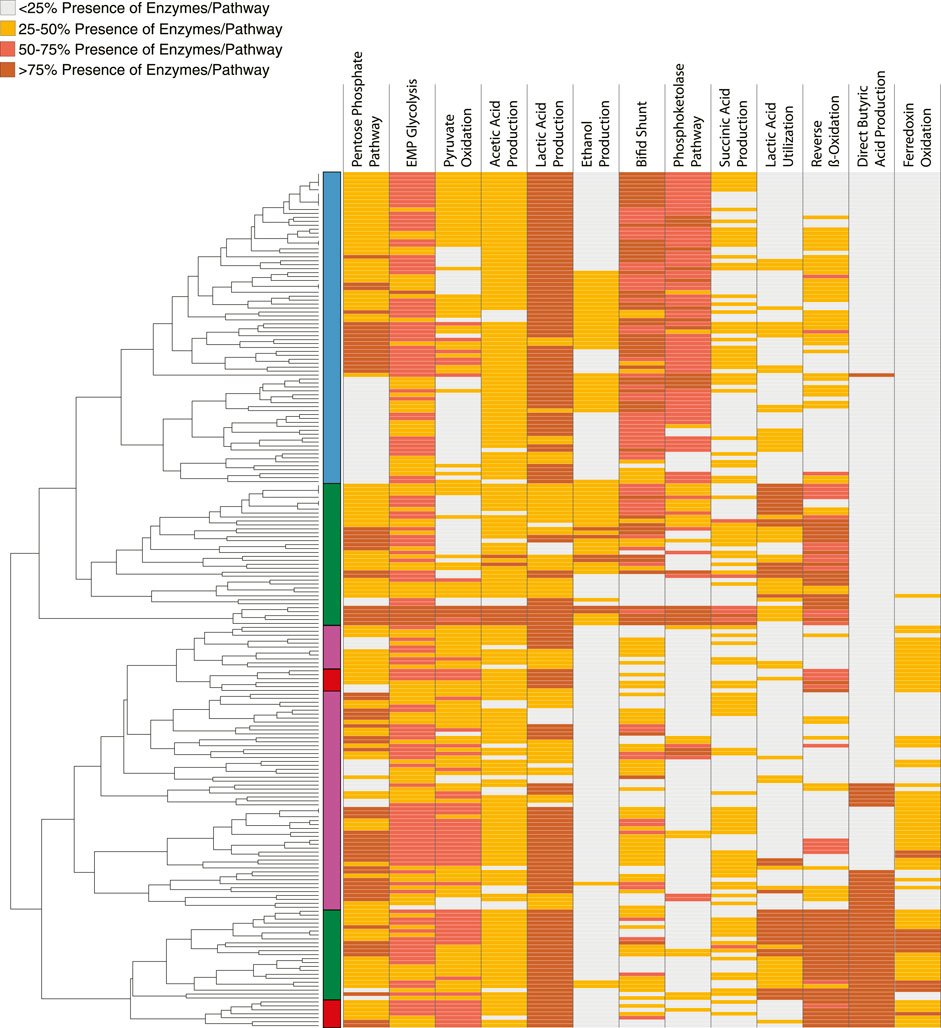
FIGURE 4. Clustering 217 MAGs using metabolic pathways. Identified homologous proteins in the indicated metabolic pathways (columns) for each of the 217 non-redundant MAGs (rows). Colors represent the percentage of protein homologs for each pathway for each MAG as indicated in the key. The MAGs were hierarchically clustered resulting the dendrogram on the left. Functional group assignments based on hierarchical clustering is indicated on the left, and color coded as Ferment to Intermediates (blue), Intermediate Chain Elongators (green), Carbohydrate Chain Elongators (red), and uninvolved in MCFA production (purple).
3.3 Machine learning-based classification
We also wanted to test if we could use multiclass classification machine learning to generate similar predictions, as a way to evaluate large MAG datasets quickly and to remove any bias in functional assignments based on enzyme assignments. For this evaluation, we constructed a training set of isolated organisms predicted to perform the three specific functions in the model, plus organisms not known or likely to participate in these activities (Supplementary Table S6). As input to the machine learning algorithm, we used the information gathered about detection of protein homologs in the metabolic pathways relevant to the ecological model (Supplementary Table S5). The training set was then used to investigate a number of possible multiclass classification machine learning algorithms, with the LightGBM algorithm (Zhang et al., 2017) producing the best results of binning the genomes into the correct functional groups based on multiple methods of evaluation (logloss comparison to baseline, PR curve, and ROC curve).
To evaluate the machine learning multiclass classifications, a subset of the most abundant MAGs was selected for further analysis. The 217 non-redundant MAGs across the experiments were filtered to include only MAGs with at least 1% relative abundance in at least one experiment sample (Figure 1B; Supplementary Table S4). The resultant 131 high-abundance MAGs include ones assembled from short read Illumina technology (74 MAGs) and long read PacBio technology (57 MAGs) and were categorized into one of four functional groups using the trained multiclass machine learning model. Overall, 63 MAGs were predicted as being able to ferment carbohydrates to intermediate products (Ferment to Intermediates), 17 MAGs were predicted as being able to convert intermediate products to MCFAs (Intermediate Chain Elongators), 12 MAGs were categorized as being able to ferment carbohydrates to MCFAs (Carbohydrate Chain Elongators), and 39 MAGs were predicted not to be involved in MCFA production (Figure 5A; Supplementary Table S4). The MAGs in each category were derived from several different agroindustrial residue experiments (Figure 5B), showing that similar functions occurred with the different agroindustrial residues.
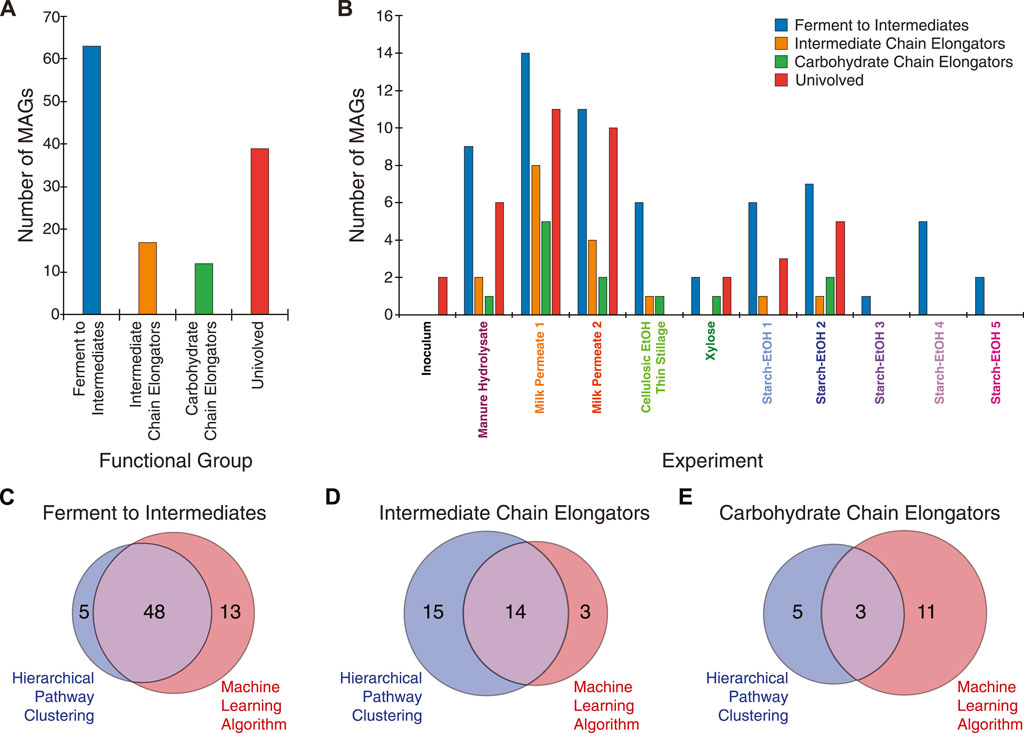
FIGURE 5. Summary of machine learning categorization. (A) Distribution of the 131 MAGs in the different functional groups used for machine learning classification. (B) Distribution of the 131 MAGs according to the experiment from which each was identified in according to how they were classified in by machine learning. Venn diagrams show comparison of the hierarchical pathway clustering classification and the machine learning classification for Ferment to Intermediates group (C), the Intermediate Chain Elongators group (D), and the Carbohydrate Chain Elongators group (E). Only MAGs present with at least 1% relative abundance in at least one sample across all experiments are included in the comparison analysis.
Comparison of the MAGs classified into the functional groups by the machine learning algorithm to classification by hierarchical pathway clustering reveals differences based on the approaches (Figures 5C–E). The Ferment to Intermediates group shows a large amount of overlap between the two methods (Figure 5C). The hierarchical pathway clustering method identified more MAGs than the machine learning algorithm for the Intermediate Chain Elongators group while there was little overlap among the methods for the Carbohydrate Chain Elongators group (Figures 5D, E).
Focusing on the machine learning classification, and to further investigate the MAGs present in functional groups responsible MCFA production, phylogenetic trees were constructed comparing the genomes used in the training set and the MAGs classified into each functional group (Figures 6–8). The MAGs were taxonomically classified using GTDB-Tk (Chaumeil et al., 2019). For each functional group examined, we found multiple taxonomic groups across taxonomic levels, ranging from phyla to family (Figures 6–8). Indeed, a subset of the MAGs in groups share no overlap at the class or family level with genomes in the training set, suggesting the machine learning algorithm is identifying new taxonomic groups that may perform the specific biological function.
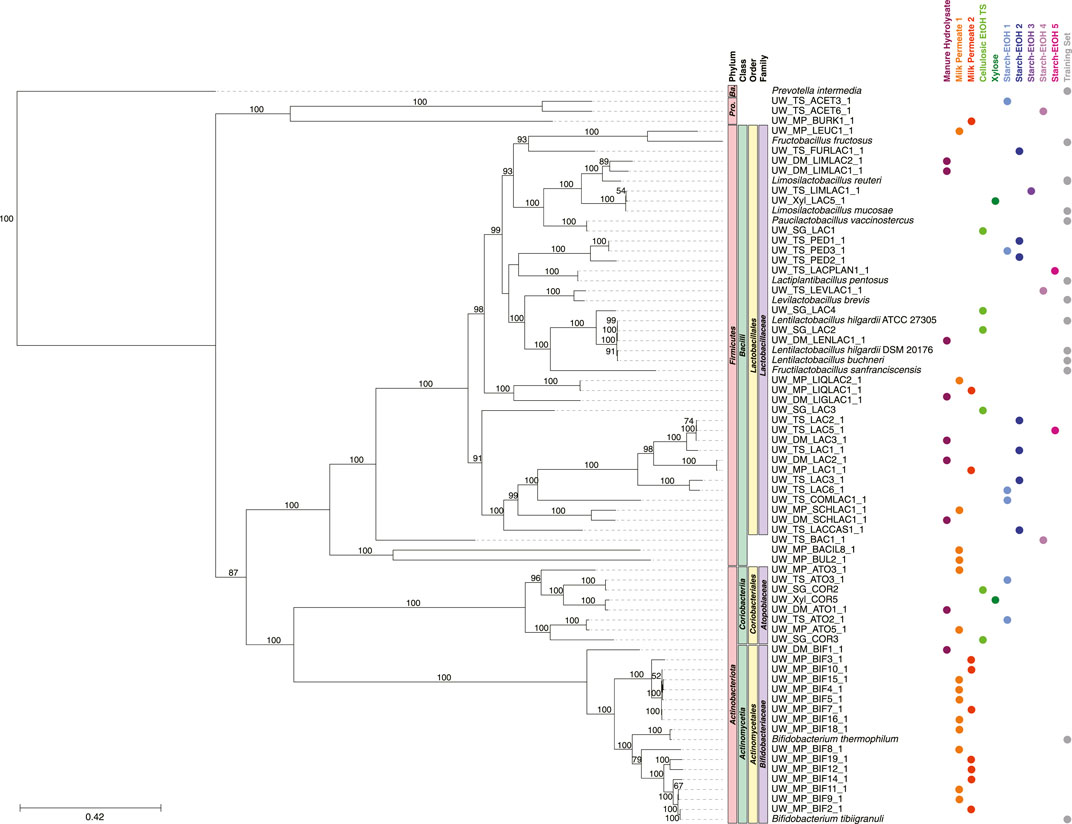
FIGURE 6. Phylogenetic tree of MAGs classified in the Ferment to Intermediates group and the genomes used in the training set. A maximum-likelihood phylogenetic tree constructed using RAxML-NG (Kozlov et al., 2019) with 1,000 bootstraps (values >50 shown) and using the 120 bacterial housekeeping gene concatenations generated by GTDB-Tk (Chaumeil et al., 2019). Taxonomic classification performed using GTDB-Tk (database version 202) (Chaumeil et al., 2019). The scale bar indicates the number of nucleotide substitutions per sequence site. Genomes used in the training set are shown (labeled Training Set) and NCBI Accession Numbers are found in Supplementary Table S6. Color dots indicate experiment the MAG was identified in (experiments with no MAGs present in the tree are not shown). Ba., Bacteroidota; Pro., Proteobacteria.
3.4 MAGs predicted to participate in fermentation to intermediate products
The majority of the MAGs predicted in the Ferment to Intermediates group belonged to the Lactobacillaceae, Bifidobacteriaceae, and Atopobiaceae families (Figure 6). In general, the MAGs in Bifidobacteriaceae and Lactobacillaceae clustered with the genomes from the same taxonomic group used in the training set. Further, the machine learning algorithm classified MAGs of the Atopobiaceae family into this group, despite no member of this family being present in the training set. A small subset of the MAGs in this functional group belonged to other taxonomic groups: class Bacilli (3 MAGs) and phylum Proteobacteria (3 MAGs) (Figure 6).
3.5 MAGs predicted to participate in chain elongation from intermediate products
The majority of the MAGs in the Intermediate Chain Elongators group, predicted to convert fermentation intermediates into MCFAs, were predicted to belong to five families: Megasphaeraceae, Acidaminococcaceae, Clostridiaceae, Anaerovoracaceae, and Eubacteriaceae (Figure 7). This included a MAG (UW_SG_EUB1, Ca. Pseudoramibacter fermentans) that was studied at the transcriptomic level and predicted to ferment intermediates into MCFAs (Scarborough et al., 2020). The MAGs in four of the five families were clustered with genomes in the same families used in the training set. However, there were no genomes in the training set that belonged to the family Acidaminococcaceae, Lachnospiraceae, or Oscillospiraceae. Two MAGs belonged to phylum Bacteroidota (order Bacteroidales).
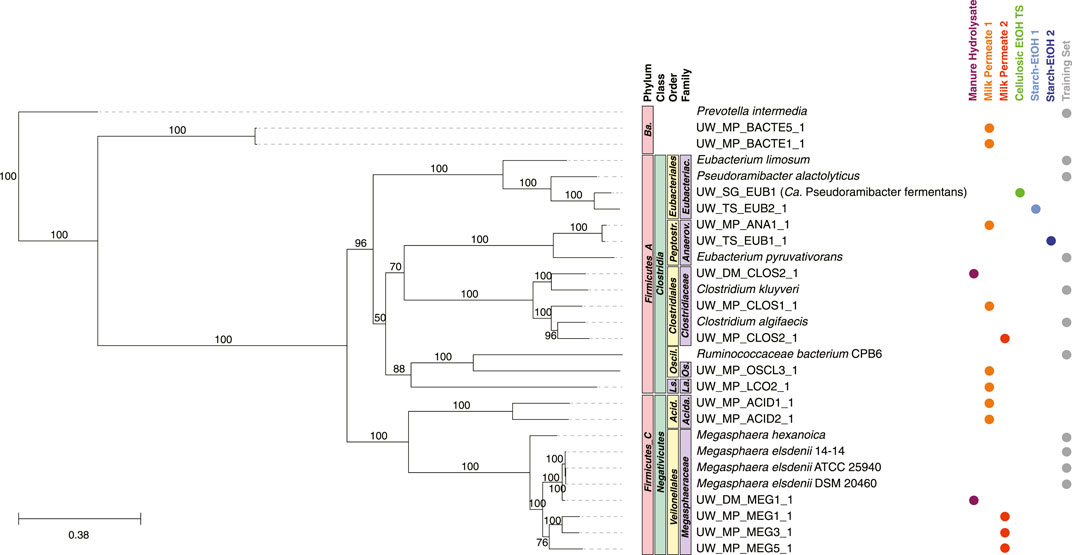
FIGURE 7. Phylogenetic tree of MAGs classified in the Intermediate Chain Elongators group and genomes used in the training set. A maximum-likelihood phylogenetic tree constructed using RAxML-NG (Kozlov et al., 2019) with 1,000 bootstraps (values >50 shown) and using the 120 bacterial housekeeping gene concatenations generated by GTDB-Tk (Chaumeil et al., 2019). Taxonomic classification performed using GTDB-Tk (database version 202) (Chaumeil et al., 2019). The scale bar indicates the number of nucleotide substitutions per sequence site. Genomes used in the training set for this group are shown (labeled Training Set) and NCBI Accession Numbers are found in Supplementary Table S6. Color dots indicate experiment the MAG was identified in (experiments with no MAGs present in the tree are not shown). Ba., Bacteroidota; Acida., Acidaminococcales; Acida., Acidaminococcaceae; Peptostr., Peptostreptococcales; Anaerov., Anaerovoracaceae; Eubacteriac., Eubacteriaceae; Ls., Lachnospirales; La., Lachnospiraceae; Oscil., Oscillospirales; Os. Oscillospiraceae.
3.6 MAGs predicted to participate in chain elongation from carbohydrates
The MAGs predicted to belong to the Carbohydrate Chain Elongators group, ones which convert carbohydrates directly to MCFAs, belonged primarily to two families: Lachnospiraceae and Acutalibacteraceae (Figure 8). Included in this group is a MAG (UW_SG_LCO1, Ca. Weimeria bifida) previously studied in-depth and suggested to perform chain elongation from carbohydrate substrates (Scarborough et al., 2020). Seven of the MAGs present in the Carbohydrate Chain Elongators group belonged to other taxonomic groups: class Bacilli, class Clostridia as well as phyla Proteobacteria and Spirochaetota (Figure 8).
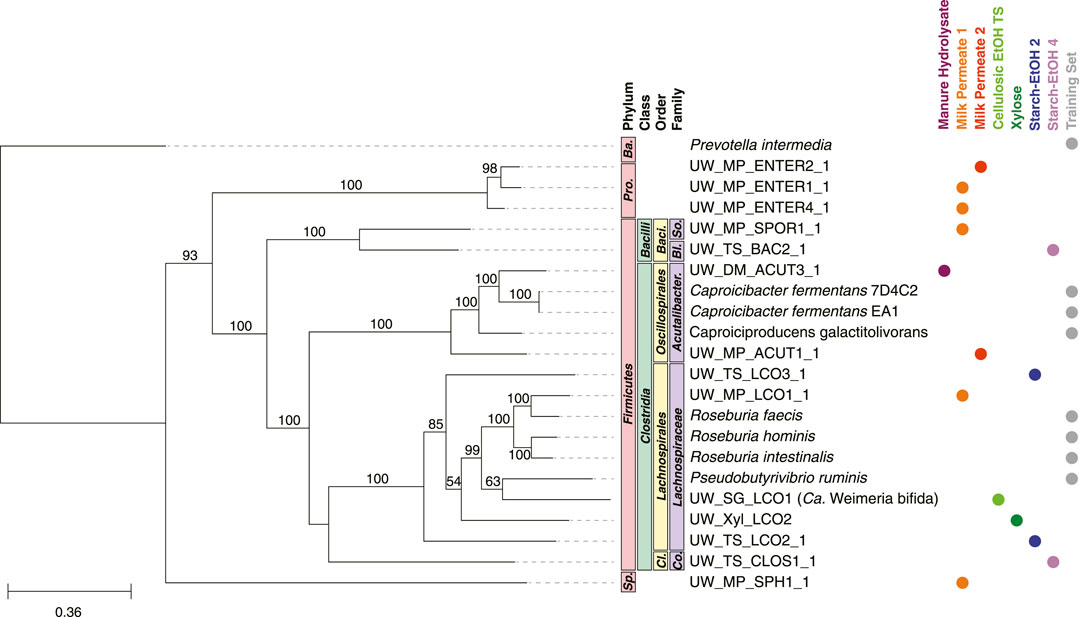
FIGURE 8. Phylogenetic tree of MAGs classified in the Carbohydrate Chain Elongators group and genomes used in the training set. A maximum-likelihood phylogenetic tree constructed using RAxML-NG (Kozlov et al., 2019) with 1,000 bootstraps (values >50 shown) and using the 120 bacterial housekeeping gene concatenations generated by GTDB-Tk (Chaumeil et al., 2019). Taxonomic classification performed using GTDB-Tk (database version 202) (Chaumeil et al., 2019). The scale bar indicates the number of nucleotide substitutions per sequence site. Genomes used in the training set for this group are shown (labeled Training Set) and NCBI Accession Numbers are found in Supplementary Table S6. Color dots indicate experiment the MAG was identified in (experiments with no MAGs present in the tree are not shown). Ba., Bacteroidota; Pro., Proteobacteria; Baci., Bacillales; So., Sporolactobacillaceae; Bl., Bacillaceae; Acutalibacter., Acutalibacteraceae; Cl., Clostridiales; Co., Clostridiaceae; Sp., Spirochaetota.
4 Discussion
We have used a dataset of over 200 MAGs from 10 previously published bioreactor experiments to evaluate the prevalence of the emerging microbial ecological model for chain elongation microbiomes. In this model, MCFAs can be produced either from intermediates, such as lactic acid, or directly from carbohydrates. Using machine learning and protein homology predictions, we find that this ecology model is conserved across various microbial communities from bioreactors fed various carbohydrate rich agroindustrial residues. While the MAGs assembled from each microbial community were not found to be identical in terms of sequence similarity, the biological functions of the microbial communities are predicted to be maintained in MAGs from various taxonomic groups with different relative abundances (Figure 9). Below we discuss observations about the organisms classified into each group.
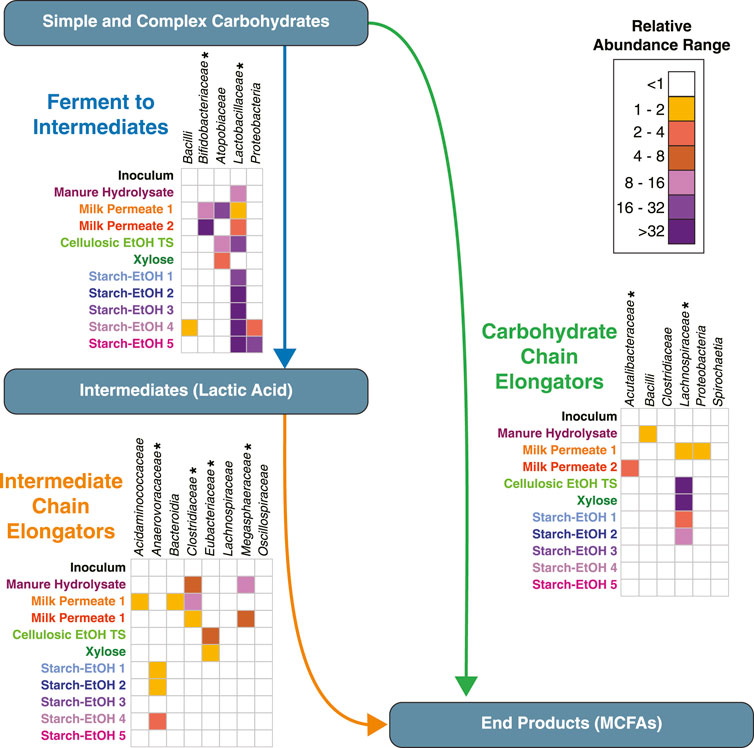
FIGURE 9. Cumulative relative abundances for taxa within each group reveal common biological functions across agroindustrial residues. Cumulative relative abundances for each taxa across the 10 experiments for MAGs classified in the Ferment to Intermediates group, the Intermediate Chain Elongators group, and the Carbohydrate Chain Elongators group as labeled. For each panel, the heat map represents the cumulative relative abundance, with white indicating a cumulative relative abundance <1. Asterisks indicate taxa present in the machine learning training set. MCFA, Medium Chain Fatty Acid.
4.1 A taxonomically diverse set of MAGs is predicted to ferment carbohydrates to intermediates
The Ferment to Intermediates functional group was comprised of many MAGs classified in the phylum Firmicutes, specifically lactic acid bacteria, which are associated with carbohydrate fermentation to lactic acid and other intermediates (Garde et al., 2002; Ganzle and Follador, 2012; Gänzle, 2015; Zhang and Vadlani, 2015). Indeed, Firmicutes, specifically those in the family Lactobacillaceae, make up a large portion of the microbial community in most of the bioreactors analyzed when using cumulative relative genomic abundance as a measure (Figure 9), suggesting MAGs in this phylum may play a key role in fermentation to intermediates across the agroindustrial residues examined. There were other taxonomic groups classified in this group. MAGs from both family Atopobiaceae and family Bifidobacteriaceae (phylum Actinobacteriota) were found to be fairly abundant in a subset of the experiments, specifically Milk Permeate 1 and 2, as well as Cellulosic Ethanol Thin Stillage and Xylose (Figure 9), which supports previous observations of the relationship between these two families (Scarborough et al., 2018a; Carvajal-Arroyo et al., 2019; Walters et al., 2023). Three MAGs in the class Bacilli but not part of the Lactobacillaceae family as well as three MAGs in the phylum Proteobacteria were both categorized as being in this functional group (Figure 9) and were found to be of high abundance in two Starch-EtOH experiments that were conducted at a higher temperature and did not result in accumulation of MCFA chain elongation products (Figure 2; Table 1) (Fortney et al., 2021).
From a metabolic potential perspective, fermentation to intermediates can be accomplished as homolactic fermentation wherein only lactic acid is produced, or heterolactic fermentation, either by the phosphoketolase pathway or the bifid shunt pathway, wherein lactic acid and other products (ethanol or acetate) are produced (Pokusaeva et al., 2011; Gänzle, 2015). The percentage of detected gene homologs that encode enzymes unique to each fermentative pathway can be used to evaluate which fermentative pathways may be present in each MAG (Supplementary Figure S1A). In the majority of MAGs, greater than 60% of the unique proteins in the homolactic and the heterolactic bifid shunt pathways were detected, suggesting these are the primary sources of lactic acid across the microbial communities. This included the MAGs in the phylum Proteobacteria and the non-Lactobacillaceae MAGs in the class Bacilli, suggesting this is a key reason these MAGs from unexpected taxonomic groups were categorized into this functional group (Supplementary Figure S1A). No MAGs contained more than 60% of the unique proteins in the heterolactic phosphoketolase fermentation pathway, with the majority containing less than 40% of the unique enzymes (Supplementary Figure S1A), suggesting this is a not a key pathway in abundant members of the communities that are found when using these agroindustrial residues. Nearly all the MAGs in the family Bifidobacteriaceae have over 80% of the unique enzymes in the heterolactic bifid shunt fermentative pathway, which is to be expected for members of this family (Supplementary Figure S1A) (Pokusaeva et al., 2011). Future research can explore the proposal that these MAGs that perform lactic acid fermentation and do so using the homolactic fermentation pathway or heterolactic bifid shunt fermentation pathway.
4.2 MAGs from several taxonomic groups are predicted to use intermediates for chain elongation
The Intermediate Chain Elongators functional group was comprised of MAGs from a variety of taxonomic classifications (Figure 7). While nearly all the MAGs were part of the phyla Firmicutes_A or Firmicutes_C, the lower taxonomic levels were more differentiated (Figures 7, 9), suggesting a variety of microorganisms capable of performing this transformation in these microbial communities. Several of these MAGs belonged to families included in the training set, supporting the functional classification—Anaerovoracaceae, Clostridiaceae, Eubacteriaceae, and Megasphaeraceae—and were the MAGs with the highest relative level of genomic abundance in the experimental microbial communities (Figure 9). This suggests that these MAGs may play a key role in converting intermediates to MCFAs. Interestingly, the machine learning approach predicted MAGs from other families may also perform this biological function. These included MAGs from the phylum Bacteroidia and the families Acidaminococcaceae, Lachnospiraceae, and Oscillospiraceae (Figure 7). A member of the family Oscillospiraceae, Caproicibacterium lactatifermentans, was shown to utilize lactic acid, a function unique from other members of this family (Wang et al., 2022), and the Oscillospiraceae MAG has homologs of the key proteins for conversion of lactic acid to MCFAs (Supplementary Figure S1B). MAGs that belong to family Lachnospiraceae have been shown to convert carbohydrates directly to MCFAs (Scarborough et al., 2018a; Scarborough et al., 2020), but our analysis suggests they may also convert fermentation intermediates into these products. Indeed, UW_MP_LCO2_1 contains all three proteins key for conversion of lactic acid to MCFAs, supporting a possible alternative role of the MAG from this family (Supplementary Figure S1B).
However, neither the Lachnospiraceae MAG nor the Oscillospiraceae MAG were highly abundant in any of the datasets analyzed (Figure 9), suggesting they may not play a large role, even if they do generate MCFAs from intermediates. Interestingly, the Acidaminococcaceae and Bacteroidia MAGs have relatively high abundance in the Milk Permeate 1 experiment (Figure 9), raising the possibility that the unique conditions of that experiment (Walters et al., 2023) may lead to the enrichment of these MAGs to convert fermentation intermediates to MCFAs. However, the two MAGs belonging to phylum Bacteroidota are the only two MAGs for which a majority of genes encoding for lactic acid utilization and reverse ß-oxidation were not detected (Supplementary Figure S1B). This raises the possibility that these MAGs were misclassified, but their metabolic potential deserves future exploration since phylogenetically related organisms have recently been associated with SCFA production in microbial communities (Watanabe et al., 2021; Ho et al., 2021; Liu et al., 2022).
4.3 MAGs from various taxonomic groups are predicted to use carbohydrates for chain elongation
A majority of the MAGs classified in the Carbohydrate Chain Elongators group by the machine learning algorithm we used belong to the phylum Firmicutes and specifically five families: Lachnospiraceae, Acutalibacteraceae, Bacillaceae, Sporolactobacillaceae, and Clostridiaceae (Figure 9). Of these MAGs, Lachnospiraceae has been shown to produce MCFAs from carbohydrates in other microbial communities (Scarborough et al., 2018a; Scarborough et al., 2020). Indeed, the Lachnospiraceae MAGs are the most abundant across the largest number of reactor experiments, suggesting they are key players in MCFA synthesis from carbohydrate (Figure 9). Interestingly, for two of these MAGs we were not able to identify homologs to three of the four enzymes involved in chain elongation (Supplementary Figure S1C). While this may indicate mis-classification, it also raises the possibility that other enzymes may perform these processes in these organisms or that the enzymes have diverged enough in these MAGs so the homologs were below our thresholds. Additional research into these MAGs will be required to examine these hypotheses.
Most of the MAGs in this group contain homologs for the chain elongation genes, although many of them outside the Lachnospiraceae family also contain at least one homolog of the lactic acid utilization genes (Supplementary Figure S1C). These results suggest that these MAGs may be able to convert both carbohydrates as well as lactic acid into MCFAs. This has been observed in other microbes including Caproicibacterium lactatifermentans (family Acutalibacteraceae) (Wang et al., 2022) and Megasphaera hexanoica (family Megasphaeraceae) (Jeon et al., 2017; Kang et al., 2022). Interestingly, MAGs within the same family (Acutalibacteraceae) differ in the presence of lactic acid utilization homologs (Supplementary Figure S1C), suggesting this difference may be on the genus or species level. Recent results suggest members of this family can produce MCFAs from lactic acid (Wang et al., 2022) as well as carbohydrates (Van Nguyen et al., 2023). Further research into these MAGs and related isolated organisms will be valuable to evaluate this new hypothesis.
Of the two MAGs in the class Bacilli that are classified as Carbohydrate Chain Elongators, UW_MP_SPOR1_1 (family Sporolactobacillaceae) lacked homologs to the electron bifurcating acyl-CoA dehydrogenase and the acetyl-CoA C-acetyltransfase enzymes while UW_TS_BAC2_1 (family Bacillaceae) contained homologs for all examined enzymes (Supplementary Figure S1C). Members of the family Sporolactobacillaceae are known to produce lactic acid (Chang et al., 2008; Tolieng et al., 2017), so our findings raise the possibility that some members of class Bacilli may be able to produce MCFAs as well. Similarly, the MAG in the family Clostridiaceae contained homologs for all enzymes examined, including the lactic acid utilization proteins, suggesting that this MAG may produce MCFAs from lactic acid as well as carbohydrates. Members of the phyla Spirochaetota and Proteobacteria are not known to perform chain elongation, but the MAGs contain at least some of the genes encoding enzymes important for chain elongation, raising the possibility of an expanded functional role of MAGs from these taxonomic groups (Supplementary Figure S1C). Taken together, the results from the machine learning analysis both support previous research and suggest potential new groups of organisms that may be able to perform the specific biological function.
4.4 Phylogenetic analysis of EtfB homologs can differentiate between lactic acid utilization and chain elongation
The electron flavoprotein (EtfAB) can form a complex with both electron confurcating lactate dehydrogenase (ecLDH, involved in lactic acid utilization) and acyl-CoA dehydrogenase (ACD, involved in chain elongation) (Garcia Costas et al., 2017; Detman et al., 2019) and phylogenetic analysis of the beta subunit (EtfB) can be used to differentiate between the ability to use lactic acid and to perform chain elongation (Walters et al., 2023). This analysis suggests that three MAGs in the Intermediate Chain Elongators group contain multiple copies of EtfB, one associated with ecLDH and one associated with ACD (Figure 10; Supplementary Figure S2), supporting the functional classification that these MAGs use lactic acid to perform chain elongation. Three MAGs in the Carbohydrate Chain Elongators group contain a single copy of EtfB associated with ACD (Figure 10; Supplementary Figure S2), supporting the classification that these MAGs can produce MCFAs but not utilize lactic acid. However, a majority of the MAGs in both functional groups contain EtfB homologs for which the phylogenetic analysis cannot predict a metabolic function. Additional research into the metabolism of microorganisms represented by these MAGs will be required to elucidate the function of these EtfB homologs.
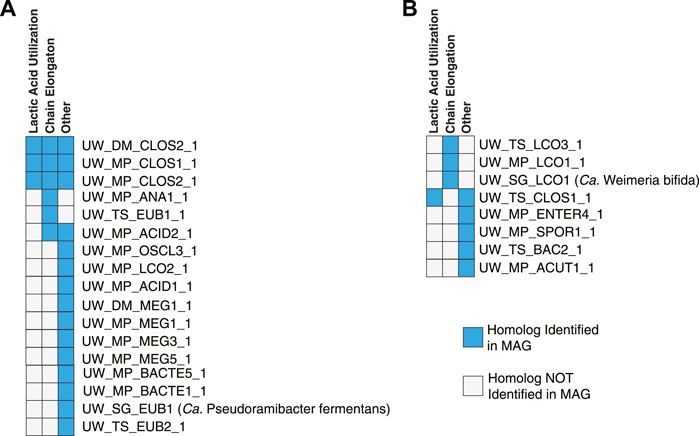
FIGURE 10. Association of EtfB homologs with lactic acid utilization, chain elongation, or other functions. Summary of the phylogenetic analysis (Supplementary Figure S2) examining EtfB homologs in the MAGs from the Intermediate Chain Elongators group (A) and the Carbohydrate Chain Elongators group (B). MAGs with an EtfB homolog that the phylogenetic analysis suggests is associated with lactic acid utilization have a blue box in the first column while MAGs with an EtfB homolog that the phylogenetic analysis suggests is associated with chain elongation have a blue box in the second column. A blue box in the Other column indicates that a MAG has an EtfB homolog for which the phylogenetic analysis cannot indicate a clear function.
4.5 Additional data needed to better understand and predict operation of these microbial communities
All of the analyses in this study were performed using metagenomic data for the MAGs across the 10 experiments. Importantly, metagenomics data can inform what genes are present in a microbial community, and thus we can use this presence to classify MAGs using machine learning. However, presence of a gene does not indicate how much that gene is expressed and thus how important the protein is to the microbial community. Previous work has shown a dramatic disconnect in MAG abundance when calculated using metagenomics (DNA) data or metatranscriptomics (RNA) data (Jewell et al., 2016; Lawson et al., 2017; Beach et al., 2021; Watanabe et al., 2021). The addition of metatranscriptomics to study this ecological microbial model would not only indicate the expression level of the genes in each MAG, but would also provide more information about the functional abundance of each MAG within each functional group.
For the machine learning analysis, we selected isolated bacteria that had been shown to perform the biological function for each group. This meant we were limited in how many organisms were available to use to build our training set. One key example is the lack of isolated organisms shown to convert ethanol to MCFAs. The only isolated organism we were able to find supported evidence for this biological process was the well-studied species Clostridium kluyveri (Seedorf et al., 2008; Han et al., 2018). Due to the limited available genomes that represent isolated organisms known to produce MCFA from ethanol by chain elongation, we did not attempt to predict this as a separate functional group. As more bacteria are isolated and studied for this biological process, it is likely the machine learning model can be updated to distinguish between MAGs that using ethanol and those that use lactic acid to produce MCFAs, adding more value to this type of classification procedure.
This study suggests that the ecological microbial model of different functional groups (Ferment to Intermediates, Intermediate Chain Elongators, and Carbohydrate Chain Elongators) is common among microbial communities enriched in carbohydrate-rich agroindustrial residues seeded with anaerobic digester sludge from the wastewater treatment plant. Examination of a microbial community enriched in food waste, a carbohydrate-rich liquid medium, and an inoculum of anaerobic digester sludge from a wastewater treatment plant suggested a similar ecological model (Crognale et al., 2021). A key question that remains is how widespread this ecological model is when applied to other microbial communities, especially in terms of different inocula and feedstock used. Additional research into the composition and genomic make up of other microbial communities would be fascinating and reveal how universal this model is among microbial communities performing chain elongation to produce MCFAs.
4.6 Concluding remarks
Examining the 240 MAGs across 10 experiments provided us an opportunity to develop new tools to better understand the microbial communities present across the bioreactors. Specifically, the large data set enabled the use of multiclass classification machine learning to categorize the MAGs into distinct functional groups in an unbiased manner. These tools can be adapted to evaluate other microbial ecology models by changing or expanding the functional groups included in the models. Thus, this analysis not only further explained the core functional groups for MCFA production in carbohydrate rich agroindustrial residues but also demonstrated a new way to quickly examine and explore microbial communities. Such knowledge will help generate hypotheses about microbial community members that could be experimentally tested, helping in the development of better strategies to manage microbiomes to produce desired products, as well as to better characterize microbial functions in a wide variety of microbiomes.
Data availability statement
The datasets presented in this study can be found in online repositories. The names of the repository/repositories and accession number(s) can be found below: https://www.ncbi.nlm.nih.gov/, PRJNA768492, https://www.ncbi.nlm.nih.gov/, PRJNA418244, https://www.ncbi.nlm.nih.gov/, PRJNA535528, https://www.ncbi.nlm.nih.gov/, PRJNA518398, https://www.ncbi.nlm.nih.gov/, PRJNA518399, https://www.ncbi.nlm.nih.gov/, PRJNA518400.
Author contributions
AI, KW, NF, MS, TD, and DN designed the bioreactor experiments. AI, KW, NF, and MS performed the bioreactor experiments. KM and AI performed all computational analyses. KM, TD, and DN wrote the manuscript. All authors listed have made a substantial, direct, and intellectual contribution to the work and approved it for publication.
Funding
This material is based upon work supported by the Great Lakes Bioenergy Research Center, U.S. Department of Energy, Office of Science, Office of Biological and Environmental Research under Award Number DE-SC0018409, the National Dairy Council under project MSN214606 (AAG8952 and AAK8347), and the National Science Foundation under project CBET-1803055.
Acknowledgments
We thank members of the TD and DN labs for helpful discussion in preparation of this manuscript.
Conflict of interest
The authors declare that the research was conducted in the absence of any commercial or financial relationships that could be construed as a potential conflict of interest.
Publisher’s note
All claims expressed in this article are solely those of the authors and do not necessarily represent those of their affiliated organizations, or those of the publisher, the editors and the reviewers. Any product that may be evaluated in this article, or claim that may be made by its manufacturer, is not guaranteed or endorsed by the publisher.
Supplementary material
The Supplementary Material for this article can be found online at: https://www.frontiersin.org/articles/10.3389/fbioe.2023.1197175/full#supplementary-material
References
Agler, M. T., Spirito, C. M., Usack, J. G., Werner, J. J., and Angenent, L. T. (2012). Chain elongation with reactor microbiomes: Upgrading dilute ethanol to medium-chain carboxylates. Energy & Environ. Sci. 5 (8), 8189. doi:10.1039/c2ee22101b
Anderson, M. J. (2017). Wiley StatsRef: Statistics reference online. New Jersey, United States: Wiley, 1–15.
Beach, N. K., Myers, K. S., Owen, B. R., Seib, M., Donohue, T. J., and Noguera, D. R. (2021). Exploring the meta-regulon of the CRP/FNR family of global transcriptional regulators in a partial-nitritation anammox microbiome. mSystems 6 (5), e0090621. doi:10.1128/msystems.00906-21
Benjamini, Y., and Hochberg, Y. (1995). Controlling the false discovery rate: A practical and powerful approach to multiple testing. J. R. Stat. Soc. Ser. B Methodol. 57 (1), 289–300. doi:10.1111/j.2517-6161.1995.tb02031.x
Bian, W., and Tao, D. (2011). in Learning a distance metric by empirical loss minimization. Editor T. Walsh (Barcelona, Catalonia, Spain: AAAI Press), 1186–1191.
Boutet, E., Lieberherr, D., Tognolli, M., Schneider, M., Bansal, P., Bridge, A. J., et al. (2016). UniProtKB/Swiss-Prot, the manually annotated section of the UniProt KnowledgeBase: How to use the entry view. Methods Mol. Biol. 1374, 23–54. doi:10.1007/978-1-4939-3167-5_2
Camacho, C., Coulouris, G., Avagyan, V., Ma, N., Papadopoulos, J., Bealer, K., et al. (2009). BLAST+: Architecture and applications. BMC Bioinforma. 10 (1), 421–429. doi:10.1186/1471-2105-10-421
Carvajal-Arroyo, J. M., Candry, P., Andersen, S. J., Props, R., Seviour, T., Ganigué, R., et al. (2019). Granular fermentation enables high rate caproic acid production from solid-free thin stillage. Green Chem. 21 (6), 1330–1339. doi:10.1039/c8gc03648a
Caspi, R., Billington, R., Keseler, I. M., Kothari, A., Krummenacker, M., Midford, P. E., et al. (2020). The MetaCyc database of metabolic pathways and enzymes - a 2019 update. Nucleic Acids Res. 48, D445–D453. doi:10.1093/nar/gkz862
Chang, Y. H., Jung, M. Y., Park, I. S., and Oh, H. M. (2008). Sporolactobacillus vineae sp. nov., a spore-forming lactic acid bacterium isolated from vineyard soil. Int. J. Syst. Evol. Microbiol. 58, 2316–2320. doi:10.1099/ijs.0.65608-0
Chaumeil, P. A., Mussig, A. J., Hugenholtz, P., and Parks, D. H. (2019). GTDB-tk: A toolkit to classify genomes with the genome taxonomy database. Bioinformatics 36 (6), 1925–1927. doi:10.1093/bioinformatics/btz848
Crognale, S., Braguglia, C. M., Gallipoli, A., Gianico, A., Rossetti, S., and Montecchio, D. (2021). Direct conversion of food waste extract into caproate: Metagenomics assessment of chain elongation process. Microorganisms 9 (2), 327. doi:10.3390/microorganisms9020327
Detman, A., Mielecki, D., Chojnacka, A., Salamon, A., Blaszczyk, M. K., and Sikora, A. (2019). Cell factories converting lactate and acetate to butyrate: Clostridium butyricum and microbial communities from dark fermentation bioreactors. Microb. Cell Fact. 18 (1), 36. doi:10.1186/s12934-019-1085-1
Eckel, V. P. L., and Vogel, R. F. (2020). Genomic and physiological insights into the lifestyle of Bifidobacterium species from water kefir. Arch. Microbiol. 202 (7), 1627–1637. doi:10.1007/s00203-020-01870-7
Ferrero, F., Tabacco, E., and Borreani, G. (2021). Lentilactobacillus hilgardii inoculum, dry matter contents at harvest and length of conservation affect fermentation characteristics and aerobic stability of corn silage. Front. Microbiol. 12, 675563. doi:10.3389/fmicb.2021.675563
Fitzgerald, C. M., Camejo, P., Oshlag, J. Z., and Noguera, D. R. (2015). Ammonia-oxidizing microbial communities in reactors with efficient nitrification at low-dissolved oxygen. Water Res. 70, 38–51. doi:10.1016/j.watres.2014.11.041
Flaiz, M., Baur, T., Brahner, S., Poehlein, A., Daniel, R., and Bengelsdorf, F. R. (2020). Caproicibacter fermentans gen. nov., sp. nov., a new caproate-producing bacterium and emended description of the genus Caproiciproducens. Int. J. Syst. Evol. Microbiol. 70 (7), 4269–4279. doi:10.1099/ijsem.0.004283
Fortney, N. W., Hanson, N. J., Rosa, P. R. F., Donohue, T. J., and Noguera, D. R. (2021). Diverse profile of fermentation byproducts from thin stillage. Front. Bioeng. Biotechnol. 9, 695306. doi:10.3389/fbioe.2021.695306
Fortney, N. W., Myers, K. S., Ingle, A. T., Walters, K. A., Scarborough, M. J., Donohue, T. J., et al. (2022). Metagenomes and metagenome-assembled genomes from microbiomes metabolizing thin stillage from an ethanol biorefinery. Microbiol. Resour. Announc 11 (8), e0029022. doi:10.1128/mra.00290-22
Ganzle, M. G., and Follador, R. (2012). Metabolism of oligosaccharides and starch in lactobacilli: A review. Front. Microbiol. 3, 340. doi:10.3389/fmicb.2012.00340
Gänzle, M. G. (2015). Lactic metabolism revisited: Metabolism of lactic acid bacteria in food fermentations and food spoilage. Curr. Opin. Food Sci. 2, 106–117. doi:10.1016/j.cofs.2015.03.001
Garcia Costas, A. M., Poudel, S., Miller, A. F., Schut, G. J., Ledbetter, R. N., Fixen, K. R., et al. (2017). Defining electron bifurcation in the electron-transferring flavoprotein family. J. Bacteriol. 199 (21), 004400–e517. doi:10.1128/jb.00440-17
Garde, A., Jonsson, G., Schmidt, A. S., and Ahring, B. K. (2002). Lactic acid production from wheat straw hemicellulose hydrolysate by Lactobacillus pentosus and Lactobacillus brevis. Bioresour. Technol. 81 (3), 217–223. doi:10.1016/s0960-8524(01)00135-3
Ge, S., Usack, J. G., Spirito, C. M., and Angenent, L. T. (2015). Long-term n-caproic acid production from yeast-fermentation beer in an anaerobic bioreactor with continuous product extraction. Environ. Sci. Technol. 49 (13), 8012–8021. doi:10.1021/acs.est.5b00238
Grootscholten, T. I. M., Kinsky Dal Borgo, F., Hamelers, H. V. M., and Buisman, C. J. N. (2013). Promoting chain elongation in mixed culture acidification reactors by addition of ethanol. Biomass Bioenergy 48, 10–16. doi:10.1016/j.biombioe.2012.11.019
Grootscholten, T. I. M., Strik, D. P. B. T. B., Steinbusch, K. J. J., Buisman, C. J. N., and Hamelers, H. V. M. (2014). Two-stage medium chain fatty acid (MCFA) production from municipal solid waste and ethanol. Appl. Energy 116, 223–229. doi:10.1016/j.apenergy.2013.11.061
Haibo, H., and Garcia, E. A. (2009). Learning from imbalanced data. IEEE Trans. Knowl. Data Eng. 21 (9), 1263–1284. doi:10.1109/tkde.2008.239
Han, W., He, P., Shao, L., and Lu, F. (2018). Metabolic interactions of a chain elongation microbiome. Appl. Environ. Microbiol. 84 (22), e01614–e01618. doi:10.1128/aem.01614-18
Harmsen, P. F. H., Hackmann, M. M., and Bos, H. L. (2014). Green building blocks for bio-based plastics. Biofuels, Bioprod. Biorefining 8 (3), 306–324. doi:10.1002/bbb.1468
Ho, H. E., Chun, Y., Jeong, S., Jumreornvong, O., Sicherer, S. H., and Bunyavanich, S. (2021). Multidimensional study of the oral microbiome, metabolite, and immunologic environment in peanut allergy. J. Allergy Clin. Immunol. 148 (2), 627–632.e3. doi:10.1016/j.jaci.2021.03.028
Ingle, A. T., Fortney, N. W., Myers, K. S., Walters, K. A., Scarborough, M. J., Donohue, T. J., et al. (2022). Metagenome-assembled genomes from a microbiome grown in dairy manure hydrolysate. Microbiol. Resour. Announc 11 (8), e0029222. doi:10.1128/mra.00292-22
Ingle, A. T., Fortney, N. W., Walters, K. A., Donohue, T. J., and Noguera, D. R. (2021). Mixed acid fermentation of carbohydrate-rich dairy manure hydrolysate. Front. Bioeng. Biotechnol. 9, 724304. doi:10.3389/fbioe.2021.724304
Jeon, B. S., Kim, S., and Sang, B. I. (2017). Megasphaera hexanoica sp. nov., a medium-chain carboxylic acid-producing bacterium isolated from a cow rumen. Int. J. Syst. Evol. Microbiol. 67 (7), 2114–2120. doi:10.1099/ijsem.0.001888
Jewell, T. N., Karaoz, U., Brodie, E. L., Williams, K. H., and Beller, H. R. (2016). Metatranscriptomic evidence of pervasive and diverse chemolithoautotrophy relevant to C, S, N and Fe cycling in a shallow alluvial aquifer. ISME J. 10 (9), 2106–2117. doi:10.1038/ismej.2016.25
Kang, S., Kim, H., Jeon, B. S., Choi, O., and Sang, B. I. (2022). Chain elongation process for caproate production using lactate as electron donor in Megasphaera hexanoica. Bioresour. Technol. 346, 126660. doi:10.1016/j.biortech.2021.126660
Kasmaei, K. M., Kalyani, D. C., Reichenbach, T., Jimenez-Quero, A., Vilaplana, F., and Divne, C. (2022). Crystal structure of the feruloyl esterase from Lentilactobacillus buchneri reveals a novel homodimeric state. Front. Microbiol. 13, 1050160. doi:10.3389/fmicb.2022.1050160
Keseler, I. M., Collado-Vides, J., Santos-Zavaleta, A., Peralta-Gil, M., Gama-Castro, S., Muniz-Rascado, L., et al. (2011). EcoCyc: A comprehensive database of Escherichia coli biology. Nucleic Acids Res. 39, D583–D590. doi:10.1093/nar/gkq1143
Kim, B. C., Seung Jeon, B., Kim, S., Kim, H., Um, Y., and Sang, B. I. (2015). Caproiciproducens galactitolivorans gen. nov., sp. nov., a bacterium capable of producing caproic acid from galactitol, isolated from a wastewater treatment plant. Int. J. Syst. Evol. Microbiol. 65 (12), 4902–4908. doi:10.1099/ijsem.0.000665
Kobayashi, H., Nakasato, T., Sakamoto, M., Ohtani, Y., Terada, F., Sakai, K., et al. (2017). Clostridium pabulibutyricum sp. nov., a butyric-acid-producing organism isolated from high-moisture grass silage. Int. J. Syst. Evol. Microbiol. 67 (12), 4974–4978. doi:10.1099/ijsem.0.002387
Kozlov, A. M., Darriba, D., Flouri, T., Morel, B., and Stamatakis, A. (2019). RAxML-NG: A fast, scalable and user-friendly tool for maximum likelihood phylogenetic inference. Bioinformatics 35 (21), 4453–4455. doi:10.1093/bioinformatics/btz305
Ksiezarek, M., Grosso, F., Ribeiro, T. G., and Peixe, L. (2022). Genomic diversity of genus Limosilactobacillus. Microb. Genom 8 (7), mgen000847. doi:10.1099/mgen.0.000847
Kucek, L. A., Spirito, C. M., and Angenent, L. T. (2016a). High n-caprylate productivities and specificities from dilute ethanol and acetate: Chain elongation with microbiomes to upgrade products from syngas fermentation. Energy & Environ. Sci. 9 (11), 3482–3494. doi:10.1039/c6ee01487a
Kucek, L. A., Xu, J., Nguyen, M., and Angenent, L. T. (2016b). Waste conversion into n-caprylate and n-caproate: Resource recovery from wine lees using anaerobic reactor microbiomes and in-line extraction. Front. Microbiol. 7, 1892. doi:10.3389/fmicb.2016.01892
Langmead, B., and Salzberg, S. L. (2012). Fast gapped-read alignment with Bowtie 2. Nat. Methods 9 (4), 357–359. doi:10.1038/nmeth.1923
Lawson, C. E., Wu, S., Bhattacharjee, A. S., Hamilton, J. J., Mcmahon, K. D., Goel, R., et al. (2017). Metabolic network analysis reveals microbial community interactions in anammox granules. Nat. Commun. 8 (1), 15416. doi:10.1038/ncomms15416
Li, H., Handsaker, B., Wysoker, A., Fennell, T., Ruan, J., Homer, N., et al. (2009). The sequence alignment/map format and SAMtools. Bioinformatics, 25(16): 2078–2079. doi:10.1093/bioinformatics/btp352
Litty, D., and Muller, V. (2021). Butyrate production in the acetogen Eubacterium limosum is dependent on the carbon and energy source. Microb. Biotechnol. 14 (6), 2686–2692. doi:10.1111/1751-7915.13779
Liu, J., Bai, Y., Liu, F., Kohn, R. A., Tadesse, D. A., Sarria, S., et al. (2022). Rumen microbial predictors for short-chain fatty acid levels and the grass-fed regimen in angus cattle. Anim. (Basel) 12 (21), 2995. doi:10.3390/ani12212995
Okada, S., Suzuki, Y., and Kozaki, M. (1979). A new heterofermentative lactobacillus species with meso-diaminopimelic acid in peptidoglycan, Lactobacillus vaccinostercus kozaki and okada sp. Nov. J. General Appl. Microbiol. 25 (4), 215–221. doi:10.2323/jgam.25.215
Olm, M. R., Brown, C. T., Brooks, B., and Banfield, J. F. (2017). dRep: a tool for fast and accurate genomic comparisons that enables improved genome recovery from metagenomes through de-replication. ISME J. 11 (12), 2864–2868. doi:10.1038/ismej.2017.126
Parks, D. H., Imelfort, M., Skennerton, C. T., Hugenholtz, P., and Tyson, G. W. (2015). CheckM: Assessing the quality of microbial genomes recovered from isolates, single cells, and metagenomes. Genome Res. 25 (7), 1043–1055. doi:10.1101/gr.186072.114
Pedregosa, F., Varoquaux, G., Gramfort, A., Michel, V., Thirion, B., Grisel, O., et al. (2011). Scikit-learn: Machine learning in Python. J. Mach. Learn. Res. 12 (85), 2825–2830.
Pokusaeva, K., Fitzgerald, G. F., and Van Sinderen, D. (2011). Carbohydrate metabolism in bifidobacteria. Genes Nutr. 6 (3), 285–306. doi:10.1007/s12263-010-0206-6
Prokhorenkova, L., Gusev, G., Vorobev, A., Dorogush, A. V., and Gulin, A. (2018). in CatBoost: Unbiased boosting with categorical features. Editors S. Bengio, H. Wallach, H. Larochelle, K. Grauman, N. Cesa-Bianchi, and R. Garnett.
Pruckler, M., Lorenz, C., Endo, A., Kraler, M., Durrschmid, K., Hendriks, K., et al. (2015). Comparison of homo- and heterofermentative lactic acid bacteria for implementation of fermented wheat bran in bread. Food Microbiol. 49, 211–219. doi:10.1016/j.fm.2015.02.014
Sarria, S., Kruyer, N. S., and Peralta-Yahya, P. (2017). Microbial synthesis of medium-chain chemicals from renewables. Nat. Biotechnol. 35 (12), 1158–1166. doi:10.1038/nbt.4022
Scarborough, M. J., Lawson, C. E., Hamilton, J. J., Donohue, T. J., and Noguera, D. R. (2018a). Metatranscriptomic and thermodynamic insights into medium-chain fatty acid production using an anaerobic microbiome. mSystems 3 (6), 002211–e318. doi:10.1128/msystems.00221-18
Scarborough, M. J., Lynch, G., Dickson, M., Mcgee, M., Donohue, T. J., and Noguera, D. R. (2018b). Increasing the economic value of lignocellulosic stillage through medium-chain fatty acid production. Biotechnol. Biofuels 11, 200. doi:10.1186/s13068-018-1193-x
Scarborough, M. J., Myers, K. S., Donohue, T. J., and Noguera, D. R. (2020). Medium-chain fatty acid synthesis by "candidatus weimeria bifida" gen. Nov., sp. nov., and "candidatus pseudoramibacter fermentans" sp. nov. Appl. Environ. Microbiol. 86 (3), 022422–e2319. doi:10.1128/aem.02242-19
Scarborough, M. J., Myers, K. S., Fortney, N. W., Ingle, A. T., Donohue, T. J., and Noguera, D. R. (2022). Metagenome-assembled genomes from a microbiome converting xylose to medium-chain carboxylic acids. Microbiol. Resour. Announc 11 (4), e0115121. doi:10.1128/mra.01151-21
Schoelmerich, M. C., Katsyv, A., Donig, J., Hackmann, T. J., and Muller, V. (2020). Energy conservation involving 2 respiratory circuits. Proc. Natl. Acad. Sci. U. S. A. 117 (2), 1167–1173. doi:10.1073/pnas.1914939117
Seedorf, H., Fricke, W. F., Veith, B., Bruggemann, H., Liesegang, H., Strittmatter, A., et al. (2008). The genome of Clostridium kluyveri, a strict anaerobe with unique metabolic features. Proc. Natl. Acad. Sci. U. S. A. 105 (6), 2128–2133. doi:10.1073/pnas.0711093105
Tamanai-Shacoori, Z., Smida, I., Bousarghin, L., Loreal, O., Meuric, V., Fong, S. B., et al. (2017). Roseburia spp.: A marker of health? Future Microbiol. 12 (2), 157–170. doi:10.2217/fmb-2016-0130
Tanner, S. A., Chassard, C., Rigozzi, E., Lacroix, C., and Stevens, M. J. (2016). Bifidobacterium thermophilum RBL67 impacts on growth and virulence gene expression of Salmonella enterica subsp. enterica serovar Typhimurium. BMC Microbiol. 16, 46. doi:10.1186/s12866-016-0659-x
Tao, Y., Zhu, X., Wang, H., Wang, Y., Li, X., Jin, H., et al. (2017). Complete genome sequence of Ruminococcaceae bacterium CPB6: A newly isolated culture for efficient n-caproic acid production from lactate. J. Biotechnol. 259, 91–94. doi:10.1016/j.jbiotec.2017.07.036
Tolieng, V., Prasirtsak, B., Miyashita, M., Shibata, C., Tanaka, N., Thongchul, N., et al. (2017). Sporolactobacillus shoreicorticis sp.nov., a lactic acid-producing bacterium isolated from tree bark. Int. J. Syst. Evol. Microbiol. 67 (7), 2363–2369. doi:10.1099/ijsem.0.001959
Van Nguyen, T., Viver, T., Mortier, J., Liu, B., Smets, I., Bernaerts, K., et al. (2023). Isolation and characterization of a thermophilic chain elongating bacterium that produces the high commodity chemical n-caproate from polymeric carbohydrates. Bioresour. Technol. 367–128170. doi:10.1016/j.biortech.2022.128170
Wallace, R. J., Mckain, N., Mcewan, N. R., Miyagawa, E., Chaudhary, L. C., King, T. P., et al. (2003). Eubacterium pyruvativorans sp. nov., a novel non-saccharolytic anaerobe from the rumen that ferments pyruvate and amino acids, forms caproate and utilizes acetate and propionate. Int. J. Syst. Evol. Microbiol. 53, 965–970. doi:10.1099/ijs.0.02110-0
Walters, K. A., Mohan, G., Myers, K. S., Ingle, A. T., Donohue, T. J., and Noguera, D. R. (2023). A metagenome-level analysis of a microbial community fermenting ultra-filtered milk permeate. Front. Bioeng. Biotechnol. 11. doi:10.3389/fbioe.2023.1173656
Walters, K. A., Myers, K. S., Wang, H., Fortney, N. W., Ingle, A. T., Scarborough, M. J., et al. (2022). Metagenomes and metagenome-assembled genomes from microbial communities fermenting ultrafiltered milk permeate. Microbiol. Resour. Announc 11 (7), e0029322. doi:10.1128/mra.00293-22
Wang, H., Zhou, W., Gao, J., Ren, C., and Xu, Y. (2022). Revealing the characteristics of glucose- and lactate-based chain elongation for caproate production by Caproicibacterium lactatifermentans through transcriptomic, bioenergetic, and regulatory analyses. mSystems 7 (5), e0053422. doi:10.1128/msystems.00534-22
Watanabe, K., Yamano, M., Masujima, Y., Ohue-Kitano, R., and Kimura, I. (2021). Curdlan intake changes gut microbial composition, short-chain fatty acid production, and bile acid transformation in mice. Biochem Biophys Rep 27, 101095. doi:10.1016/j.bbrep.2021.101095
Yang, P., Leng, L., Tan, G-Y. A., Dong, C., Leu, S-Y., Chen, W-H., et al. (2018). Upgrading lignocellulosic ethanol for caproate production via chain elongation fermentation. Int. Biodeterior. Biodegrad. 135, 103–109. doi:10.1016/j.ibiod.2018.09.011
Yoshikawa, S., Araoka, R., Kajihara, Y., Ito, T., Miyamoto, H., and Kodama, H. (2018). Valerate production by Megasphaera elsdenii isolated from pig feces. J. Biosci. Bioeng. 125 (5), 519–524. doi:10.1016/j.jbiosc.2017.12.016
Zhang, Y., and Vadlani, P. V. (2015). Lactic acid production from biomass derived sugars via co-fermentation of Lactobacillus brevis and Lactobacillus plantarum. J. Biosci. Bioeng. 119 (6), 694–699. doi:10.1016/j.jbiosc.2014.10.027
Zhang, H., Si, S., and Hsieh, C-J. (2017). GPU-acceleration for Large-scale Tree Boosting. Machine Learning (stat.ML). arXiv: 1706.08359. doi:10.48550/arXiv.1706.08359
Keywords: microbiome, fermentation, chain elongation, agroindustrial residue, metagenomics
Citation: Myers KS, Ingle AT, Walters KA, Fortney NW, Scarborough MJ, Donohue TJ and Noguera DR (2023) Comparison of metagenomes from fermentation of various agroindustrial residues suggests a common model of community organization. Front. Bioeng. Biotechnol. 11:1197175. doi: 10.3389/fbioe.2023.1197175
Received: 30 March 2023; Accepted: 27 April 2023;
Published: 10 May 2023.
Edited by:
David Strik, Wageningen University and Research, NetherlandsReviewed by:
Anna Christine Trego, University of Galway, IrelandAlessandra Fontana, Catholic University of the Sacred Heart, Piacenza, Italy
Copyright © 2023 Myers, Ingle, Walters, Fortney, Scarborough, Donohue and Noguera. This is an open-access article distributed under the terms of the Creative Commons Attribution License (CC BY). The use, distribution or reproduction in other forums is permitted, provided the original author(s) and the copyright owner(s) are credited and that the original publication in this journal is cited, in accordance with accepted academic practice. No use, distribution or reproduction is permitted which does not comply with these terms.
*Correspondence: Daniel R. Noguera, dnoguera@wisc.edu
†Present address: Nathaniel W. Fortney, Thermo Fisher Scientific, Middleton, WI, United States