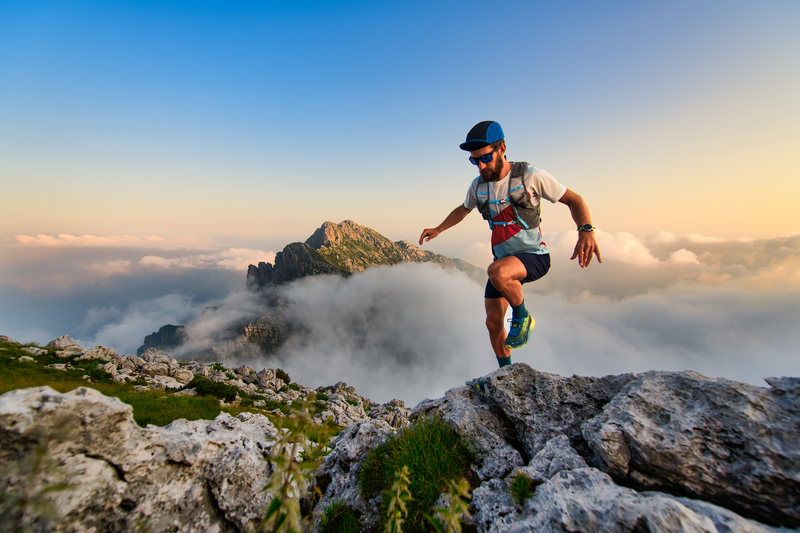
95% of researchers rate our articles as excellent or good
Learn more about the work of our research integrity team to safeguard the quality of each article we publish.
Find out more
EDITORIAL article
Front. Bioeng. Biotechnol. , 28 March 2023
Sec. Nanobiotechnology
Volume 11 - 2023 | https://doi.org/10.3389/fbioe.2023.1189769
This article is part of the Research Topic Bioinformatics Analysis Tools for Nanopore Sequencing and Applications View all 4 articles
Editorial on the Research Topic
Bioinformatics analysis tools for nanopore sequencing and applications
Nanopore sequencing technology (NST), also known as single molecule real-time sequencing technology, allows deciphering single DNA and RNA molecules without polymerase chain reaction (PCR). Currently, NST has undergone rapid development in scientific research and clinical practice, with substantial improvements made in the read length and sequencing throughput. These breakthroughs have required extensive development of experiments and bioinformatics methods to take full advantage of nanopore long-read sequencing in areas such as genomics, transcriptomics, epigenomics and epitranscriptomics (Amarasinghe et al., 2020; Wang et al., 2021). NST is currently being applied to genome assembly, full-length transcript sequencing and base-modification detection, and other specialized fields, such as rapid clinical diagnosis of pathogenic infections (Charalampous et al., 2019) and detection of pathogenic variants (Goenka et al., 2022).
However, due to the designed principle and data characteristics of NST (Magi et al., 2017), bioinformatics methods and tools developed based on NST have not been entirely satisfactory in terms of their adaptability, accuracy, and robustness. Analytical tools for next-generation sequencing (NGS) cannot be directly used to analyze NST data, so there is an urgent need for specially designed and efficient NST bioinformatics analysis methods and toolkits to provide solutions for scientific research and clinical practice. This Research Topic includes four papers that explain the application of NST and related bioinformatics analysis tools from different perspectives, such as the Android App (KARGAMobile) for real-time portable analysis of antibiotic resistance genes (ARGs) Barquero et al., evaluation of KIF20A as a prognostic biomarker and therapeutic target for lung adenocarcinoma (LUAD) Sun et al., the detection method (NanoSTR) of target short tandem repeats (STRs) Lang et al. and improving the base-calling accuracy with data augmentation (Chen et al.).
Barquero et al. developed a mobile Android app, named KARGAMobile, which can be used for portable, real-time, and easy-to-interpret analysis of antibiotic resistance genes sequenced by NST. KARGAMobile uses a compressed ARG reference database and different internal data structures to save RAM usage, and has a user-friendly graphical interface that guides file browsing, loading, parameterization, and process execution. The output files are post-processed to create visual, printable, and shareable reports that help users interpret the results.
Sun et al. used TCGA and GTEx data to identifyKIF20A as a hub gene for lung adenocarcinoma with comprehensive bioinformatics analysis. They found a negative correlation between KIF20A expression and overall survival, progression-free survival, and disease-free survival. They also found that KIF20A knockdown inhibited cell proliferation, induced G2/M phase arrest, and promoted apoptosis. Moreover, they speculated that KIF20Amay be used as a prognostic biomarker and therapeutic target for LUAD.
Lang et al. developed NanoSTR, a method for target short tandem repeats detection based on NST. NanoSTR uses statistical methods such as “multi-sampling” and Length-Number-Rank (LNR) information with sequencing data to detect the target STR locus and genotyping. Compared with existing STR detection tools, NanoSTR showed higher accuracy, better performance, and certain robustness. NanoSTR not only avoided the problem of genotyping errors or inability caused by the NST data characteristics, but also eliminated the requirement of a genome background database construction or reference genome alignment, which could reduce the consumption of computing resources, and did not require secondary processing.
Chen et al. developed several data augmentation strategies for NST to reduce the size requirements of the dataset while improving the robustness, accuracy, and other performance of the basecalling algorithm. These data augmentation strategies could improve the accuracy of basecalling by more than 1% without doubling the size of the training dataset. This work not only reduced the time and computational costs consumed during development but also provided new insights into the new basecalling algorithm.
In conclusion, although there are not many papers in our Research Topic, we believe that these papers can provide help and reference to similar research fields, and we hope to promote the application of NST in more scientific research and practical scenarios.
The idea of and concept of this Research Topic came out from JL. And he drafted the initial version of this editorial. All authors contributed to the writing process of the editorial and approved the final version.
We thank the authors for submitting their work to this Research Topic and the reviewers who agreed to review individual contributions.
JL is currently employed by Qitan Technology (Beijing) Co., Ltd. JY is currently employed by Geneis Beijing Co., Ltd.
The remaining author declares that the research was conducted in the absence of any commercial or financial relationships that could be construed as a potential conflict of interest.
All claims expressed in this article are solely those of the authors and do not necessarily represent those of their affiliated organizations, or those of the publisher, the editors and the reviewers. Any product that may be evaluated in this article, or claim that may be made by its manufacturer, is not guaranteed or endorsed by the publisher.
Amarasinghe, S. L., Su, S., Dong, X., Zappia, L., Ritchie, M. E., and Gouil, Q. (2020). Opportunities and challenges in long-read sequencing data analysis. Genome Biol. 21, 30. doi:10.1186/s13059-020-1935-5
Charalampous, T., Kay, G. L., Richardson, H., Aydin, A., Baldan, R., Jeanes, C., et al. (2019). Nanopore metagenomics enables rapid clinical diagnosis of bacterial lower respiratory infection. Nat. Biotechnol. 37, 783–792. doi:10.1038/s41587-019-0156-5
Goenka, S. D., Gorzynski, J. E., Shafin, K., Fisk, D. G., Pesout, T., Jensen, T. D., et al. (2022). Accelerated identification of disease-causing variants with ultra-rapid nanopore genome sequencing. Nat. Biotechnol. 40, 1035–1041. doi:10.1038/s41587-022-01221-5
Magi, A., Giusti, B., and Tattini, L. (2017). Characterization of MinION nanopore data for resequencing analyses. Brief. Bioinform 18, 940–953. doi:10.1093/bib/bbw077
Keywords: nanopore sequencing technology, application analysis tools, bioinformatics, statistical analysis, deep learning methods
Citation: Lang J, Yang J and Xu M (2023) Editorial: Bioinformatics analysis tools for nanopore sequencing and applications. Front. Bioeng. Biotechnol. 11:1189769. doi: 10.3389/fbioe.2023.1189769
Received: 20 March 2023; Accepted: 22 March 2023;
Published: 28 March 2023.
Edited and reviewed by:
Gianni Ciofani, Italian Institute of Technology (IIT), ItalyCopyright © 2023 Lang, Yang and Xu. This is an open-access article distributed under the terms of the Creative Commons Attribution License (CC BY). The use, distribution or reproduction in other forums is permitted, provided the original author(s) and the copyright owner(s) are credited and that the original publication in this journal is cited, in accordance with accepted academic practice. No use, distribution or reproduction is permitted which does not comply with these terms.
*Correspondence: Jidong Lang, bGFuZ2ppZG9uZ0Bob3RtYWlsLmNvbQ==
Disclaimer: All claims expressed in this article are solely those of the authors and do not necessarily represent those of their affiliated organizations, or those of the publisher, the editors and the reviewers. Any product that may be evaluated in this article or claim that may be made by its manufacturer is not guaranteed or endorsed by the publisher.
Research integrity at Frontiers
Learn more about the work of our research integrity team to safeguard the quality of each article we publish.