- 1Swiss BioMotion Lab, Department of Musculoskeletal Medicine, Lausanne University Hospital and University of Lausanne (CHUV-UNIL), Lausanne, Switzerland
- 2Department of Orthopaedic Surgery, Stanford University, Stanford, CA, United States
- 3Veterans Affairs Palo Alto Health Care System, Palo Alto, CA, United States
- 4Department of Mechanical Engineering, Stanford University, Stanford, CA, United States
- 5Department of Diagnostic and Interventional Radiology, Lausanne University Hospital and University of Lausanne (CHUV-UNIL), Lausanne, Switzerland
- 6Institute of Microengineering, Ecole Polytechnique Fédérale de Lausanne (EPFL), Lausanne, Switzerland
- 7The Sense Innovation and Research Center, Lausanne, Switzerland
Objective: To characterize ambulatory knee moments with respect to medial knee osteoarthritis (OA) severity comprehensively and to assess the possibility of developing a severity index combining knee moment parameters.
Methods: Nine parameters (peak amplitudes) commonly used to quantify three-dimensional knee moments during walking were analyzed for 98 individuals (58.7 ± 9.2 years old, 1.69 ± 0.09 m, 76.9 ± 14.5 kg, 56% female), corresponding to three medial knee osteoarthritis severity groups: non-osteoarthritis (n = 22), mild osteoarthritis (n = 38) and severe osteoarthritis (n = 38). Multinomial logistic regression was used to create a severity index. Comparison and regression analyses were performed with respect to disease severity.
Results: Six of the nine moment parameters differed statistically significantly among severity groups (p ≤ 0.039) and five reported statistically significant correlation with disease severity (0.23 ≤ |r| ≤ 0.59). The proposed severity index was highly reliable (ICC = 0.96) and statistically significantly different between the three groups (p < 0.001) as well as correlated with disease severity (r = 0.70).
Conclusion: While medial knee osteoarthritis research has mostly focused on a few knee moment parameters, this study showed that other parameters differ with disease severity. In particular, it shed light on three parameters frequently disregarded in prior works. Another important finding is the possibility of combining the parameters into a severity index, which opens promising perspectives based on a single figure assessing the knee moments in their entirety. Although the proposed index was shown to be reliable and associated with disease severity, further research will be necessary particularly to assess its validity.
1 Introduction
Knee osteoarthritis (OA) is a painful and disabling disease affecting hundreds of millions of people worldwide and this number is expected to grow in the decades to come, notably due to the aging of the population (Wallace et al., 2017; Safari et al., 2020). No cure exists for knee OA and the disease end-stage often leads to major surgery through total knee replacement (Ringdahl and Pandit, 2011), highlighting the need to better understand the pathogenesis of the disease and find ways to slow down its progression.
The repetitive mechanical loading at the knee associated with walking has been shown to play an important role in knee OA (Andriacchi et al., 2004). This contribution was particularly well highlighted in a recent report introducing the term “Mechanokine” to stress the unique property of mechanical signals to transcend scales from the external forces acting on the whole-body to the mechanical environment of the cell in a manner that can influence joint heath associated to knee OA (Andriacchi et al., 2020). For instance, the maximum values (peaks) of the knee adduction (KAMfirst) and flexion (KFMfirst) moments during the first half of stance have been related to medial knee OA severity and progression (Kean et al., 2012; Chehab et al., 2014; Erhart-Hledik et al., 2015) and gait modifications based on these parameters showed improvement in clinical outcomes (Cheung et al., 2018; Richards et al., 2018). However, the large majority of previous research on medial knee OA, the most frequent form of the disease (Ahlbäck, 1968), focused on these two parameters and little is known about the seven others usual parameters of knee moments during walking (Figure 1) (Benedetti et al., 1998; Chehab et al., 2017). While analyzing KAMfirst and KFMfirst was well motivated in prior works, the disregard of the other parameters was rarely justified. This is even more intriguing that there are evidences scattered across a few specific publications that the other parameters vary with medial knee OA (Thorp et al., 2006; Astephen et al., 2008; Huang et al., 2008; Baert et al., 2013; Mills et al., 2013). Given the possibility that each of the nine parameters illustrated in Figure 1 could influence joint health in different ways and at different stages of the disease, there is a need for comprehensive studies analyzing all nine parameters over the full range of medial knee OA severity.
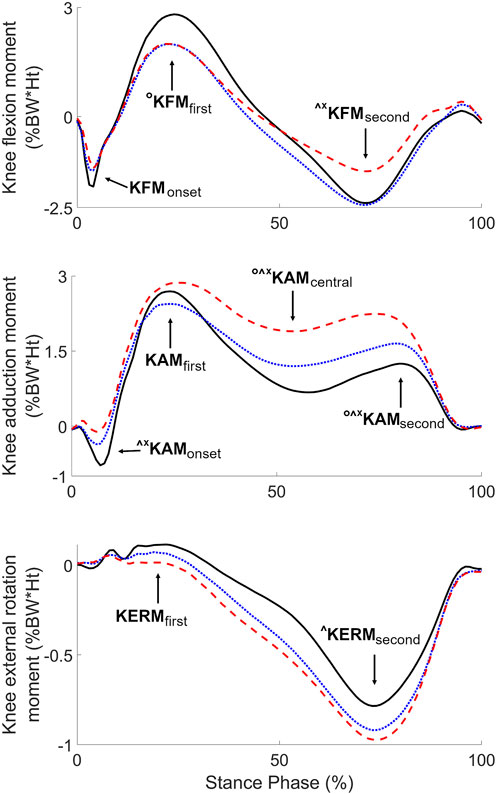
FIGURE 1. Average knee moments of the three severity groups (black solid lines: non-OA, blue dotted lines: mild OA, red dashed lines: severe OA), with indication of the nine usual parameters. Symbols indicate significant differences between groups (°: non-OA different from mild OA, ^: non-OA different from severe OA, *: mild OA different from severe OA) (p < 0.017).
While considering more parameters will enhance the description of knee moments, having a larger number of parameters to deal with could render the analysis and use of knee kinetics more complex. For example, assessing the effect of a treatment could become difficult when the results diverge among parameters. The situation could be even more arduous with personalized interventions, such as insoles or gait retraining (Reeves and Bowling, 2011), where it could be impossible to find solutions fulfilling modifications on several parameters (Edd et al., 2020; Ulrich et al., 2020). In fact, the increase in complexity when describing knee moments with a higher number of parameters could well be the main reason why most of prior works focused on KAMfirst and KFMfirst. Therefore, to benefit from a more complete characterization of knee moment without increasing the complexity-of-use, there is a need to combine the parameters into indices associated with specific features of the disease, such as severity. Prior works have already shown the relevance of combining knee moment parameters. For instance, the total joint moment (TJM) combination was introduced to assess the relative contributions of the KAM and KFM (Zabala et al., 2013) and the medial contact force (MCFfirst) parameter to estimate the peak force applied on the medial tibial plateau during the first half of stance (Walter et al., 2010; Manal et al., 2015).
This study first aimed at characterizing all nine usual parameters of knee moments during walking with respect to medial knee OA severity, through comparison and correlation analyses. A second objective was to assess the possibility of developing a severity index combining all nine parameters.
2 Methods
2.1 Study population
For this study, the database of the Stanford BioMotion lab was screened for individuals aged 40 years old or older, with a body mass index (BMI) lower than 35 kg/m2, and who got their gait analyzed following a standard procedure (see below) at the same time they were evaluated for symptoms and imaging signs of knee OA. From those, non-OA individuals, defined as individuals without self-reported pain or significant injury in the lower limb or lower back and without evidence of cartilage loss, osteophytes, subchondral bone marrow lesions, bone attrition, or meniscal pathology (subluxation, maceration, degeneration) in any knees, were selected for the present study (Hunter et al., 2011). Structural alterations of the knees were determined based on magnetic resonance imaging exams, including a three-dimensional fat-suppressed spoiled-gradient recalled echo sequence (3D SPGR; plane = sagittal, TR = 50 ms, TE = 7 ms, flip angle = 30°, field of view = 140 × 140 mm2, slice thickness = 1.5 mm, number of slices = 60, acquisition matrix = 256 × 256) and a fat-suppressed proton density fast spin echo sequence (PDFSE; plane = sagittal, TR = 4,000 ms, TE = 13 ms, flip angle = 90°, field of view = 140 × 140 mm2, slice thickness = 2.5 mm, number of slices = 33, acquisition matrix = 256 × 256), using a 1.5T machine (GE Medical Systems, Milwaukee, WI). Individuals with medial compartment knee OA were also selected for the present study. These persons were characterized by persistent self-reported pain and radiographic confirmation of the presence of primarily medial compartment OA in at least one knee, no primarily lateral or trochlea OA or arthroplasty in any knees, Kellgren and Lawrence (K/L) grading of both knees (Kellgren and Lawrence, 1957), no diagnosis or symptoms of OA in other lower extremity joints, no serious ankle, hip or back injury or surgery, no gout or recurrent pseudogout, and no use of ambulatory aids. All individuals selected for the present study got their data recorded in the framework of researches approved by the internal review board of Stanford University and gave their consent for further analysis of their data. Data from the most recent testing were used for individuals with multiple records in the database.
In total, 98 individuals (43 males) were available for this study. They were of mean (± standard deviation) age, height and weight of 58.7 ± 9.2 years old, 1.69 ± 0.09 m, and 76.9 ± 14.5 kg, respectively. One knee per individual was analyzed. For non-OA individuals, the study knee was randomly chosen, while the knee with the highest K/L grade was analyzed for OA individuals. In case of equal K/L grade for both knees, the study knee was randomly chosen. For comparison analyses, knees with K/L grade of I or II were considered mild OA and knees with K/L grade of III or IV severe OA, resulting in three severity groups of 22–38 knees each (Table 1). There was no statistically significant demographic difference among the severity groups, except for age, with younger individuals in the non-OA group compared to the two other groups (p < 0.001). A post hoc power analysis showed that effect sizes of at least 0.8 are detectable with groups of 22–38 knees each (Table 1) considering a power of 80% and a Bonferroni-corrected alpha level of 5% (G*Power, DE). These detectable effect sizes are appropriate, considering the large to strong effect sizes reported in prior studies comparing ambulatory knee moments with respect to OA severity (Cohen’s d between 0.8 and 1.2) (Astephen et al., 2008; Mills et al., 2013).
2.2 Gait analysis
All knees in this study were tested following the same standardized procedure including the recording of three 10 m-long straight-line trials at self-selected normal gait speed with personal walking shoes across a walkway instrumented with an optoelectronic motion capture system (Qualisys Medical, Gothenburg, SE) and a force plate (Bertec, Columbs, OH) operating synchronously at 120 Hz. Multiple operators from the same laboratory collected the data. Only trials with a clear step of the foot below the knee of interest on the force plate were recorded. Before recording the gait trials, clusters of reflective markers were fixed on the individuals and a calibration based on anatomical landmarks was performed, following a common protocol (Chehab et al., 2017). During the gait trials, the position and orientation of the lower-limb segments were calculated using the cluster marker trajectories and the calibration information (Andriacchi et al., 1998; Favre et al., 2009). The flexion, adduction and external rotation moments at the knee during the stance phases with the foot of interest on the force plate were calculated following a standard inverse dynamics approach (Zabala et al., 2013). The three moments were time-normalized to 0%–100% during stance and expressed as external moments in percentage of bodyweight and height (%BW × Ht). During each stance phase, the nine characteristic parameters of the moment curves were extracted for analysis (Table 2; Figure 1) (Benedetti et al., 1998; Chehab et al., 2017). Finally, each of the nine parameters was averaged over the three trials to have one value per parameter and knee. All biomechanical processing was done using the software application BioMove (Stanford, CA).
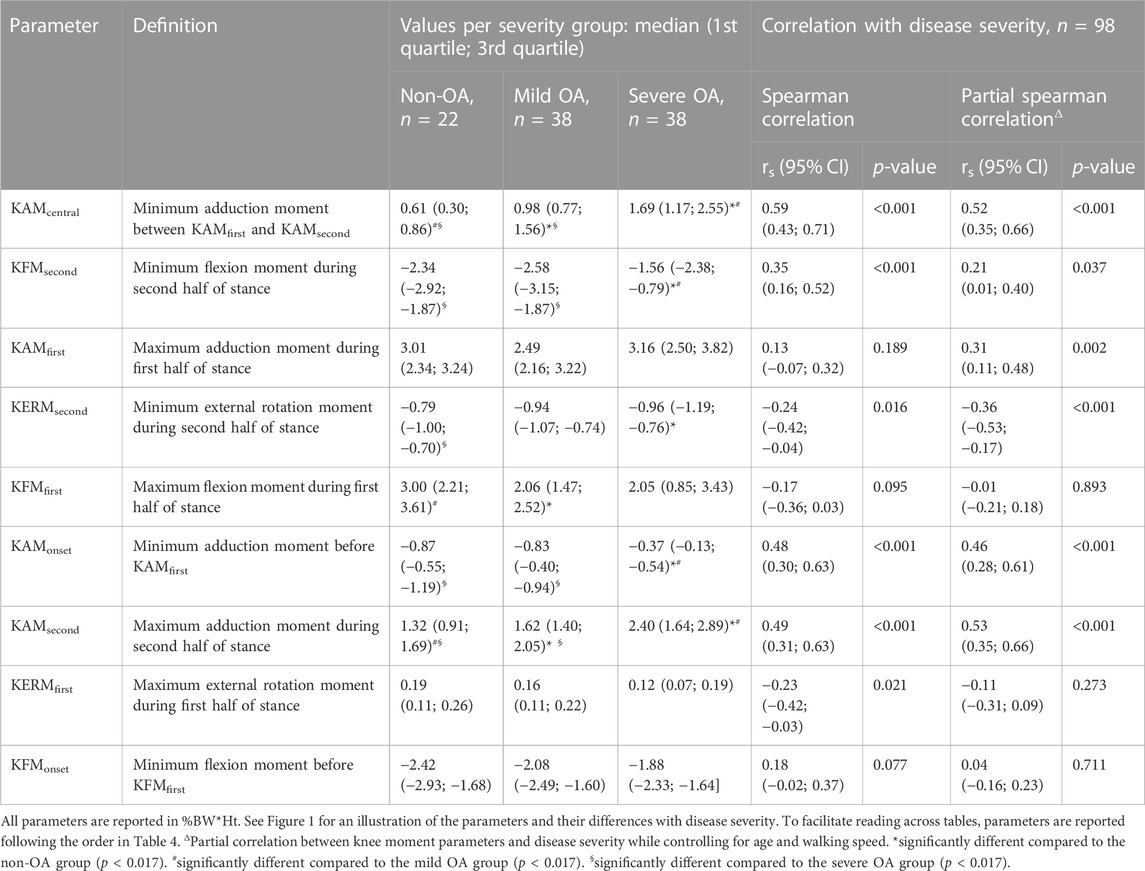
TABLE 2. Values of the knee moment parameters for the three severity groups, as well as Spearman correlation between the parameters and disease severity.
2.3 Severity index
The severity index was computed by multinomial logistic regression with the severity groups as nominal response and the nine knee moment parameters as predictors (McCullagh and Nelder, 2019). The parameters were standardized using a z-score transformation before performing the regression and a sigmoid transformation was applied to the regressed data to have indices ranging between 0 and 100. The regression was calculated by bootstrapping which allowed determining confidence intervals for the regression coefficients and assessing the reliability of the index (Efron and Tibshirani, 1994). Reliability was characterized using the intraclass correlation coefficient (ICC) and the standard error of measurement (SEM) (Weir, 2005).
For completeness with literature, two previously proposed combinations of knee moment parameters, the total knee joint moment (Zabala et al., 2013) and the medial contact force (Walter et al., 2010; Manal et al., 2015), were also computed. For the total knee joint moment, the square root of the sum of the squared knee flexion, adduction and external rotation moments was calculated for each time point of each stance (Zabala et al., 2013). Then, the maximal values during the first and second halves of each stance were extracted (TJMfirst and TJMsecond, respectively). Regarding the medial contact force, the maximum value during the first half of each stance (MCFfirst) was estimated based on KAMfirst and KFMfirst using a formula determined with instrumented knee prostheses (Walter et al., 2010; Manal et al., 2015; Uhlrich et al., 2018). Similar to the other moment parameters, TJMfirst, TJMsecond and MCFfirst were averaged over the three trials recoded for each knee.
2.4 Statistical analysis
Since the data were not normally distributed (Kolmogorov-Smirnov tests), they were analyzed using non-parametric statistics. Specifically, comparisons of the nine knee moment parameters, the proposed severity index, the three prior combination parameters and the walking speed among the severity groups were performed using Kruskal-Wallis tests with post hoc ranksum tests. Associations with disease severity, for the knee moment parameters, the severity index and the prior combination parameters, were assessed using Spearman correlations across severity groups. Since walking speed and age have been shown to influence knee moment parameters (Lelas et al., 2003; Chehab et al., 2017), partial Spearman correlations were also calculated to describe the relationship with disease severity while controlling for walking speed and age. Finally, Spearman correlations were performed to quantify the associations among parameters. Significance level was set a priori at p < 0.05, with Bonferroni corrections for multiple comparisons during post hoc analyses (effective p < 0.017).
3 Results
Six of the nine knee moment parameters showed statistically significant effect of disease severity (KFMfirst, p = 0.034; KERMsecond, p = 0.039; KAMcentral, KFMsecond, KAMonset, and KAMsecond, all p < 0.001). Post-hoc testing indicated significant incremental differences in KAMcentral and KAMsecond from non-OA to mild OA, to severe OA, with larger values in more severely affected knees (Table 2; Figure 1). KAMonset was significantly larger in the severe OA group compared to both the non-OA and the mild OA groups. KFMsecond was significantly larger in severe OA knees compared to both the non-OA and the mild OA knees, while KFMfirst was significantly smaller in mild OA than in non-OA knees. Finally, KERMsecond was significantly smaller in the severe OA than in the non-OA group.
Statistically significant correlations with disease severity were found for five of the nine moment parameters: KAMcentral (rs = 0.59, p < 0.001), KAMsecond (rs = 0.49, p < 0.001), KAMonset (rs = 0.48, p < 0.001), KFMsecond (rs = 0.35, p < 0.001), KERMsecond (rs = −0.24, p = 0.016) and KERMfirst (rs = −0.23, p = 0.021) (Table 2). Controlling for age and walking speed resulted in the same statistically significant correlations, except for KAMfirst which became significant (rs = 0.31, p = 0.002) and KERMfirst which exceeded the significance level (rs = −0.11, p = 0.273). Correlations among the nine moment parameters are reported in Supplementary Table S1.
The proposed regression method allowed compiling a severity index showing an excellent reliability, with ICC of 0.96 and SEM of 6.78 units (Koo and Li, 2016). Moreover, Kruskal-Wallis test showed statistically significant differences among severity groups (p < 0.001), with post hoc analyses indicating significant difference between the three groups. Indeed, the non-OA group had significantly lower severity indices than the mild OA and severe OA groups, and the mild OA group had significantly lower severity indices than the severe OA groups (Table 3). Additionally, a significant correlation was found between the severity index and disease severity (rs = 0.70, p < 0.001). The correlation remained significant when controlling for age and walking speed (rs = 0.63, p < 0.001).
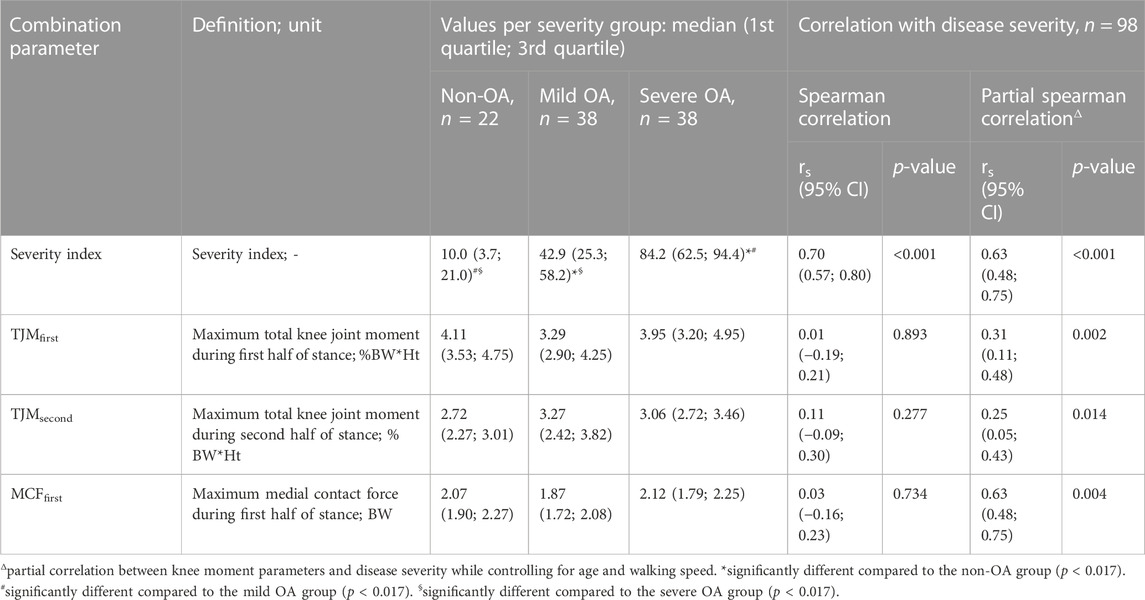
TABLE 3. Values of the severity index and of three prior moment combination parameters for the three severity groups, as well as Spearman correlation between these measures and disease severity.
Since the moment parameters were standardized before calculating the severity index, the coefficients of the regression leading to the severity index can be analyzed to compare the contribution of the nine moment parameters to the severity index. Doing so, indicated that KAMcentral had the biggest effect on the index with a coefficient of −1.52, contributing 27.0% to the severity index, followed by KFMsecond and KAMfirst with coefficients of −1.00 and 0.98 and contributing for 17.7% and 17.4% of the severity index, respectively (Table 4; Figure 2). On the opposite, KFMonset, KERMfirst and KAMsecond had the least impact on the index, with coefficients of 0.095, −0.178, and −0.304 and contributions of 1.7%, 3.2% and 5.4% to the severity index, respectively.
Additionally, Kruskal-Wallis tests showed no statistically significant difference among severity groups for any of the three prior combinations parameters (TJMfirst, p = 0.079; TJMsecond, p = 0.168; MCFfirst, p = 0.061). When controlling for age and walking speed, statistically significant correlations with disease severity were observed for all three combinations: TJMfirst (rs = 0.31, p = 0.002), TJMsecond (rs = 0.25, p = 0.014), and MCFfirst (rs = 0.29, p = 0.004). The correlations were non-significant when no control for age and walking speed was applied (rs ≤ 0.13, p ≥ 0.188).
4 Discussion
This study confirmed that diverse knee moment parameters differ with respect to the severity of medial knee OA. Compared to prior works, the present study, testing all usual parameters over the entire spectrum of disease severity, provided a basis to assemble the pieces disseminated in literature. Various factors, including participants’ characteristics or analysis protocols, could influence knee moments and lead to diverging results among studies (Messier et al., 2005; Chehab et al., 2017; Schrijvers et al., 2021). Nevertheless, even with such possible methodological variations among studies, strong consensuses could be identified for four parameters. These included smaller KFMfirst in mild OA compared to non-OA (asymptomatic) knees and in severe OA compared to mild OA knees, although the severe-mild difference was not observed in the present study (Weidow et al., 2006; Astephen et al., 2008; Huang et al., 2008). Consistent observations also existed for larger KFMsecond in severe OA than in non-OA (asymptomatic) and mild OA knees (Astephen et al., 2008; Baert et al., 2013), as well as larger KAMcentral and KAMsecond in mild OA than in non-OA (asymptomatic) and in severe OA than in mild OA (Thorp et al., 2006; Astephen et al., 2008; Huang et al., 2008). No consensus existed for KAMfirst, which was already shown to have highly inconsistent results among studies (Mills et al., 2013), and no consolidation could be attempted for the other parameters due to lacking data in literature. Altogether, the present study shed light on three parameters, KAMcentral, KAMsecond, and KFMsecond, which were frequently disregarded in prior works. This suggests that future research should not limit the analysis to KAMfirst and KFMfirst. This suggestion is particularly well supported by two recent studies relating KAMcentral with disease progression and symptoms (Astephen Wilson et al., 2017; Costello et al., 2020).
With the consideration of more than two parameters appearing wise for the characterization of the knee moments, the possibility to combine the parameters into an index reflecting disease severity constitutes another important finding of the present study. Indeed, while considering a larger number of parameters will contribute to better descriptions, having a larger number of parameters to manage could increase study design complexity and make gait interventions more complex (Edd et al., 2020). Therefore, the possibility of combining the parameters, as demonstrated in this study, is interesting practically. However, beyond practical considerations, indices could be especially relevant for the global assessments of the knee moments they allow. For example, in personalized interventions, such as gait retraining (Cheung et al., 2018; Richards et al., 2018; Ulrich et al., 2020), it could become possible to aim for a global change, instead of aiming for changes in one or two moment parameters, without consideration for the others.
The second objective of assessing the possibility of developing a severity index was fully achieved, with the design of an index reliable, significantly different among the three severity groups and showing a large correlation with disease severity. Further research will now be necessary to assess the validity of the proposed index. The techniques to record and calculate knee moments differ among institutions (Benedetti et al., 2013; Schrijvers et al., 2021). Therefore, the sensitivity of the severity index to variations in gait analysis protocols will need to be determined. It is well possible that the index will be little sensitive to such methodological differences, as it is an aggregate of standardized parameters. It will also be necessary evaluating the index longitudinally and characterizing its relationships with key features of knee OA, such as pain or disease progression (Felson, 2009).
It is interesting to note that KAMfirst accounted for 17% of the severity index (third most important contributor to the index), although it was not significantly different among severity groups. While such an important role in the severity index could appear peculiar in view of its relationship with disease severity, this role well agrees with medial knee OA literature, where KAMfirst is a prevalent parameter and the primary focus of gait interventions (Reeves and Bowling, 2011; Mills et al., 2013; Favre and Jolles, 2016). Thus, it is possible that the severity index actually captured the global essence of disease severity. Three combination parameters were already proposed in literature, TJMfirst, TJMsecond, and MCFfirst (Walter et al., 2010; Zabala et al., 2013; Manal et al., 2015), but the severity index in this study is the first to have been designed to reflect disease severity.
The present study brought new insights into the relationship between knee moment parameters and disease severity that could reveal particularly useful in the evaluation and rehabilitation of medial knee OA gait (Favre and Jolles, 2016). Nevertheless, further research will be necessary to determine the mechanisms behind these relationships. Walking speed and age certainly play a role in the relationships between knee moment parameters and disease severity, but, as confirmed in this study, the causes are more complex than simply variations in walking speed or in age (Landry et al., 2007). Consequently, the role of other factors, including motor control, muscle strength and soft-tissue properties (Lewek et al., 2004; Hubley-Kozey et al., 2006; Rudolph et al., 2007; Adouni and Shirazi-Adi, 2014; Stanahan et al., 2015) as well as pain (Boyer, 2018), will need to be clarified in future studies. Further works should also assess the relevance of the severity index in pre-OA both for early disease detection and gait modification (Reeves and Bowling, 2011; Ulrich et al., 2020).
This study has some limitations, including the use of a single cross-sectional dataset, as discussed above. Multiple operators contributed to the gait data collection, which could have led to increased inter-individual variability and limited the detection of differences and correlations with disease severity. Nevertheless, obtaining conclusive results based on data collected by multiple operators remained a strength in view of future large-scale applications where gait recording will likely be performed by different operators. Another point worth mentioning is the multinomial logistic regression used to determine the severity index. While it is a common method, which successfully combined the moment parameters, one cannot exclude that there could be other ways to combine the parameters. This is particularly supported by the fact that some parameters were correlated to each other. It is important to note that the possible existence of alternative combinations does not affect the main findings but requires caution to not over interpret the combination obtained in this study as unique or being the best. Depending on the results of the validity studies to follow, in the future, it might be necessary comparing different combination methods. Additionally, in line with literature, this study focused on discrete knee moment parameters. Nevertheless, analyzing the knee moment curves could also reveal interesting, for example, using one-dimensional statistical parametric mapping (Friston et al., 1994; Pataky, 2012). Finally, since the severity groups differed in age and walking speed, it is possible that a fraction of the severity index reflects the variations in moment parameters with respect to age or walking speed.
5 Conclusion
This study confirmed that diverse knee moment parameters differ with disease severity. In particular, differences among severity groups were found to be consistent across studies for four parameters, including three that were frequently disregarded in prior works (KAMcentral, KAMsecond, KFMsecond). Future studies are therefore recommended to not limit the analysis to KAMfirst and KFMfirst. Another important finding of this study was the possibility to combine the parameters into a severity index, which opens promising perspectives based on a single figure assessing the knee moments in their entirety. While the proposed index was shown to be reliable and correlated with disease severity, further research will be necessary to assess its validity.
Data availability statement
The data analyzed in this study is subject to the following licenses/restrictions: The data are not publicly available due to regulatory provisions. Requests to access these datasets should be directed to YmFwdGlzdGUudWxyaWNoQGNodXYuY2g=.
Ethics statement
The studies involving human participants were reviewed and approved by the Commission cantonale d'éthique de la recherche sur l'être humain CER-VD. The patients/participants provided their written informed consent to participate in this study.
Author contributions
Study conception and design were done by BU, TA, BJ, and JF. Data were collected and assembled by BU, JE-H, and JA. Analysis and interpretation of the data were performed by BU, PO, TA, and JF. The article was drafted by BU, TA, and JF, and critically revised for important intellectual content by all authors. Funding was obtained by TA, BJ, and JF. The study was supervised by TA, BJ, and JF, who should be considered as last authors. All authors contributed to the article and approved the submitted version.
Funding
This study was partially funded by the Swiss National Science Foundation (SNSF grant #32003B-166433), the Lausanne Orthopedic Research Foundation and the Profectus Foundation.
Acknowledgments
The present study is part of the PhD thesis of BU (Ulrich 2021).
Conflict of interest
The authors declare that the research was conducted in the absence of any commercial or financial relationships that could be construed as a potential conflict of interest.
Publisher’s note
All claims expressed in this article are solely those of the authors and do not necessarily represent those of their affiliated organizations, or those of the publisher, the editors and the reviewers. Any product that may be evaluated in this article, or claim that may be made by its manufacturer, is not guaranteed or endorsed by the publisher.
Supplementary material
The Supplementary Material for this article can be found online at: https://www.frontiersin.org/articles/10.3389/fbioe.2023.1176471/full#supplementary-material
References
Adouni, M., and Shirazi-Adl, A. (2014). Evaluation of knee joint muscle forces and tissue stresses-strains during gait in severe OA versus normal subjects. J. Orthop. Res. 32 (1), 69–78. doi:10.1002/jor.22472
Ahlbäck, S. (1968). Osteoarthrosis of the knee. A radiographic investigation. Acta. Radiol. diagn. 227, 277:7-72–72.
Andriacchi, T. P., Alexander, E. J., Toney, M. K., Dyrby, C., and Sum, J. A. (1998). A point cluster method for in vivo motion analysis: Applied to a study of knee kinematics. J. Biomech. Eng. 120 (6), 743–749. doi:10.1115/1.2834888
Andriacchi, T. P., Griffin, T. M., Loeser, R. F., Chu, C. R., Roos, E. M., Hawker, G. A., et al. (2020). Bridging disciplines as a pathway to finding new solutions for osteoarthritis a collaborative program presented at the 2019 orthopaedic research society and the osteoarthritis research society international. Osteoarthr. Cartil. Open 2 (1), e100026. doi:10.1016/j.ocarto.2020.100026
Andriacchi, T. P., Mündermann, A., Smith, R. L., Alexander, E. J., Dyrby, C. O., and Koo, S. (2004). A framework for the in vivo pathomechanics of osteoarthritis at the knee. Ann. Biomed. Eng. 32 (3), 447–457. doi:10.1023/b:abme.0000017541.82498.37
Astephen, J. L., Deluzio, K. J., Caldwell, G. E., and Dunbar, M. J. (2008). Biomechanical changes at the hip, knee, and ankle joints during gait are associated with knee osteoarthritis severity. J. Orthop. Res. 26 (3), 332–341. doi:10.1002/jor.20496
Astephen Wilson, J. L., Stanish, W. D., and Hubley-Kozey, C. L. (2017). Asymptomatic and symptomatic individuals with the same radiographic evidence of knee osteoarthritis walk with different knee moments and muscle activity. J. Orthop. Res. 35 (8), 1661–1670. doi:10.1002/jor.23465
Baert, I. A., Jonkers, I., Staes, F., Luyten, F. P., Truijen, S., and Verschueren, S. M. (2013). Gait characteristics and lower limb muscle strength in women with early and established knee osteoarthritis. Clin. Biomech. 28 (1), 40–47. doi:10.1016/j.clinbiomech.2012.10.007
Benedetti, M. G., Catani, F., Leardini, A., Pignotti, E., and Giannini, S. (1998). Data management in gait analysis for clinical applications. Clin. Biomech. 13 (3), 204–215. doi:10.1016/S0268-0033(97)00041-7
Benedetti, M. G., Merlo, A., and Leardini, A. (2013). Inter-laboratory consistency of gait analysis measurements. Gait Posture 38 (4), 934–939. doi:10.1016/j.gaitpost.2013.04.022
Boyer, K. A. (2018). Biomechanical response to osteoarthritis pain treatment may impair long-term efficacy. Exerc. Sport Sci. Rev. 46 (2), 121–128. doi:10.1249/JES.0000000000000141
Chehab, E. F., Andriacchi, T. P., and Favre, J. (2017). Speed, age, sex, and body mass index provide a rigorous basis for comparing the kinematic and kinetic profiles of the lower extremity during walking. J. Biomech. 58, 11–20. doi:10.1016/j.jbiomech.2017.04.014
Chehab, E. F., Favre, J., Erhart-Hledik, J. C., and Andriacchi, T. P. (2014). Baseline knee adduction and flexion moments during walking are both associated with 5 year cartilage changes in patients with medial knee osteoarthritis. Osteoarthr. Cartil. 22 (11), 1833–1839. doi:10.1016/j.joca.2014.08.009
Cheung, R. T., Ho, K. K. W., Au, I. P. H., An, W. W., Zhang, J. H., Chan, Z. Y. S., et al. (2018). Immediate and short-term effects of gait retraining on the knee joint moments and symptoms in patients with early tibiofemoral joint osteoarthritis: A randomized controlled trial. Osteoarthr. Cartil. 6 (11), 1479–1486. doi:10.1016/j.joca.2018.07.011
Costello, K. W., Wilson, J. L. A., Stanish, W. D., Urquhart, N., and Hubley-Kozey, C. L. (2020). Differences in baseline joint moments and muscle activation patterns associated with knee osteoarthritis progression when defined using a clinical versus a structural outcome. J. Appl. Biomech. 36 (1), 39–51. doi:10.1123/jab.2019-0127
Edd, S. N., Bennour, S., Ulrich, B., Jolles, B. M., and Favre, J. (2020). Modifying stride length in isolation and in combination with foot progression angle and step width can improve knee kinetics related to osteoarthritis; A preliminary study in healthy subjects. J. Biomech. Eng. 142 (7), e074505. doi:10.1115/1.4046713
Erhart-Hledik, J. C., Favre, J., and Andriacchi, T. P. (2015). New insight in the relationship between regional patterns of knee cartilage thickness, osteoarthritis disease severity, and gait mechanics. J. Biomech. 48 (14), 3868–3875. doi:10.1016/j.jbiomech.2015.09.033
Favre, J., Aissaoui, R., Jolles, B. M., de Guise, J. A., and Aminian, K. (2009). Functional calibration procedure for 3D knee joint angle description using inertial sensors. J. Biomech. 42 (14), 2330–2335. doi:10.1016/j.jbiomech.2009.06.025
Favre, J., and Jolles, B. M. (2016). Gait analysis of patients with knee osteoarthritis highlights a pathological mechanical pathway and provides a basis for therapeutic interventions. EFORT Open Rev. 1 (10), 368–374. doi:10.1302/2058-5241.1.000051
Felson, D. T. (2009). Developments in the clinical understanding of osteoarthritis. Arthritis Res. Ther. 11 (1), 203–211. doi:10.1186/ar2531
Friston, K. J., Holmes, A. P., Worsley, K. J., Poline, J.-P., Frith, C. D., and Frackowiak, R. S. J. (1994). Statistical parametric maps in functional imaging: A general linear approach. Hum. Brain Mapp. 2 (4), 189–210. doi:10.1002/hbm.460020402
Huang, S. C., Wei, I. P., Chien, H. L., Wang, T. M., Liu, Y. H., Chen, H. L., et al. (2008). Effects of severity of degeneration on gait patterns in patients with medial knee osteoarthritis. Med. Eng. Phys. 30 (8), 997–1003. doi:10.1016/j.medengphy.2008.02.006
Hubley-Kozey, C. L., Deluzio, K. F., Landry, S. C., McNutt, J. S., and Stanish, W. D. (2006). Neuromuscular alterations during walking in persons with moderate knee osteoarthritis. J. Electromyogr. Kinesiol. 16 (4), 365–378. doi:10.1016/j.jelekin.2005.07.014
Hunter, D. J., Arden, N., Conaghan, P. G., Eckstein, F., Gold, G., Grainger, A., et al. (2011). Definition of osteoarthritis on MRI: Results of a delphi exercise. Osteoarthr. Cartil. 19 (8), 963–969. doi:10.1016/j.joca.2011.04.017
Kean, C. O., Hinman, R. S., Bowles, K. A., Cicuttini, F., Davies-Tuck, M., and Bennell, K. L. (2012). Comparison of peak knee adduction moment and knee adduction moment impulse in distinguishing between severities of knee osteoarthritis. Clin. Biomech. 27 (5), 520–523. doi:10.1016/j.clinbiomech.2011.12.007
Kellgren, J. H., and Lawrence, J. (1957). Radiological assessment of osteo-arthrosis. Ann. Rheum. Dis. 16 (4), 494–502. doi:10.1136/ard.16.4.494
Koo, T. K., and Li, M. Y. (2016). A guideline of selecting and reporting intraclass correlation coefficients for reliability research. J. Chiropr. Med. 15 (2), 155–163. doi:10.1016/j.jcm.2016.02.012
Landry, S. C., McKean, K. A., Hubley-Kozey, C. L., Stanish, W. D., and Deluzio, K. J. (2007). Knee biomechanics of moderate OA patients measured during gait at a self-selected and fast walking speed. J. Biomech. 40 (8), 1754–1761. doi:10.1016/j.jbiomech.2006.08.010
Lelas, J. L., Merriman, G. J., Riley, R. P., and Kerrigan, D. C. (2003). Predicting peak kinematic and kinetic parameters from gait speed. Gait Posture 17 (2), 106–112. doi:10.1016/S0966-6362(02)00060-7
Lewek, M. D., Rudolph, K. S., and Snyder-Mackler, L. (2004). Quadriceps femoris muscle weakness and activation failure in patients with symptomatic knee osteoarthritis. J. Orthop. Res. 22 (1), 110–115. doi:10.1016/S0736-0266(03)00154-2
Manal, K., Gardinier, E., Buchanan, T. S., and Snyder-Mackler, L. (2015). A more informed evaluation of medial compartment loading: The combined use of the knee adduction and flexor moments. Osteoarthr. Cartil. 23 (7), 1107–1111. doi:10.1016/j.joca.2015.02.779
McCullagh, P., and Nelder, J. A. (2019). Generalized linear models. Routledge. doi:10.1201/9780203753736
Messier, S. P., Gutekunst, D. J., Davis, C., and DeVita, P. (2005). Weight loss reduces knee-joint loads in overweight and obese older adults with knee osteoarthritis. Arthritis Rheum. 52 (7), 2026–2032. doi:10.1002/art.21139
Mills, K., Hunt, M. A., and Ferber, R. (2013). Biomechanical deviations during level walking associated with knee osteoarthritis: A systematic review and meta-analysis. Arthritis Care Res. 65 (10), 1643–1665. doi:10.1002/acr.22015
Pataky, T. C. (2012). One-dimensional statistical parametric mapping in Python. Comput. Methods Biomech. Biomed. Engin. 15 (3), 295–301. doi:10.1080/10255842.2010.527837
Reeves, N. D., and Bowling, F. L. (2011). Conservative biomechanical strategies for knee osteoarthritis. Nat. Rev. Rheumatol. 7, 113–122. doi:10.1038/nrrheum.2010.212
Richards, R., Van de Noort, J. C., Van der Esch, M., Booik, M. J., and Harlaar, J. (2018). Gait retraining using real-time feedback in patients with medial knee osteoarthritis: Feasibility and effects of a six-week gait training program. Knee 25 (5), 814–824. doi:10.1016/j.knee.2018.05.014
Ringdahl, E. N., and Pandit, S. (2011). Treatment of knee osteoarthritis. Am. Fam. Physician 83 (11), 1287–1292.
Rudolph, K. S., Schmitt, L. C., and Lewek, M. D. (2007). Age-related changes in strength, joint laxity, and walking patterns: Are they related to knee osteoarthritis? Phys. Ther. 87 (11), 1422–1432. doi:10.2522/ptj.20060137
Safiri, S., Kolahi, A.-A., Smith, E., Hill, C., Bettampadi, D., Mansournia, M. A., et al. (2020). Global, regional and national burden of osteoarthritis 1990-2017: A systematic analysis of the global burden of disease study 2017. Ann. Rheum. Dis. 79 (6), 819–828. doi:10.1136/annrheumdis-2019-216515
Schrijvers, J. C., Rutherford, D., Richards, R., Van den Noort, J. C., Van der Esch, M., and Harlaar, J. (2021). Inter-laboratory comparison of knee biomechanics and muscle activation patterns during gait in patients with knee osteoarthritis. Knee 29, 500–509. doi:10.1016/j.knee.2021.03.001
Stanahan, C. J., Hodges, P. W., Wrigley, T. V., Bennell, K. L., and Farrell, M. J. (2015). Organisation of the motor cortex differs between people with and without knee osteoarthritis. Arthritis Res. Ther. 17 (1), 1–11. doi:10.1186/s13075-015-0676-4
Thorp, L. E., Sumner, D. R., Block, J. A., Moisio, K. C., Shott, S., and Wimmer, M. A. (2006). Knee joint loading differs in individuals with mild compared with moderate medial knee osteoarthritis. Arthritis Rheum. 54 (12), 3842–3849. doi:10.1002/art.22247
Uhlrich, S. D., Silder, A., Beaupre, G. S., Shull, P. B., and Delp, S. L. (2018). Subject-specific toe-in or toe-out gait modifications reduce the larger knee adduction moment peak more than a non-personalized approach. J. Biomech. 66, 103–110. doi:10.1016/j.jbiomech.2017.11.003
Ulrich, B., Cosendey, K., Jolles, B. M., and Favre, J. (2020). Decreasing the ambulatory knee adduction moment without increasing the knee flexion moment individually through modifications in footprint parameters: A feasibility study for a dual kinetic change in healthy subjects. J. Biomech. 111, e110004. doi:10.1016/j.jbiomech.2020.110004
Ulrich, B. (2021). Gait modifications for medial knee osteoarthritis. [Lausanne, Switzerland]: University of Lausanne. [Doctoral thesis].
Wallace, I. J., Worthington, S., Felson, D. T., Jurmain, R. D., Wren, K. T., Maijanen, H., et al. (2017). Knee osteoarthritis has doubled in prevalence since the mid-20th century. Proc. Natl. Acad. Sci. U. S. A. 114 (35), 9332–9336. doi:10.1073/pnas.1703856114
Walter, J. P., D’Lima, D. D., Colwell, C. W., and Fregly, B. J. (2010). Decreased knee adduction moment does not guarantee decreased medial contact force during gait. J. Orthop. Res. 28 (10), 1348–1354. doi:10.1002/jor.21142
Weidow, J., Tranberg, R., Saari, T., and Kärrholm, J. (2006). Hip and knee joint rotations differ between patients with medial and lateral knee osteoarthritis: Gait analysis of 30 patients and 15 controls. J. Orthop. Res. 24 (9), 1890–1899. doi:10.1002/jor.20194
Weir, J. P. (2005). Quantifying test-retest reliability using the intraclass correlation coefficient and the SEM. J. Strength Cond. Res. 19 (1), 231–240. doi:10.1519/15184.1
Keywords: gait analysis, kinetics, knee adduction moment, knee flexion moment, biomechanics, machine learning
Citation: Ulrich B, Erhart-Hledik JC, Asay JL, Omoumi P, Andriacchi TP, Jolles BM and Favre J (2023) Diverse parameters of ambulatory knee moments differ with medial knee osteoarthritis severity and are combinable into a severity index. Front. Bioeng. Biotechnol. 11:1176471. doi: 10.3389/fbioe.2023.1176471
Received: 28 February 2023; Accepted: 31 May 2023;
Published: 13 June 2023.
Edited by:
Simone Tassani, Pompeu Fabra University, SpainReviewed by:
Jonathan Rylander, Baylor University, United StatesXiaolong Zeng, Second Affiliated Hospital of Guangzhou Medical University, China
Copyright © 2023 Ulrich, Erhart-Hledik, Asay, Omoumi, Andriacchi, Jolles and Favre. This is an open-access article distributed under the terms of the Creative Commons Attribution License (CC BY). The use, distribution or reproduction in other forums is permitted, provided the original author(s) and the copyright owner(s) are credited and that the original publication in this journal is cited, in accordance with accepted academic practice. No use, distribution or reproduction is permitted which does not comply with these terms.
*Correspondence: Baptiste Ulrich, YmFwdGlzdGUudWxyaWNoQGNodXYuY2g=
†These authors share last authorship