- 1Department of Rheumatology, The Third Affiliated Hospital of Sun Yat-Sen University, Guangzhou, Guangdong, China
- 2Department of Nephrology, Kiang Wu Hospital, Macau, Macao SAR, China
- 3Department of Nephrology, GuangZhou Eighth People’s Hospital, GuangZhou Medical University, Guangzhou, Guangdong, China
- 4Department of Cardiovasology, The Third Affiliated Hospital of Sun Yat-Sen University, Guangzhou, Guangdong, China
- 5Department of Nephrology, The Third Affiliated Hospital of Sun Yat-Sen University, Guangzhou, Guangdong, China
Background: With the development of chronic kidney disease (CKD), there are various changes in metabolites. However, the effect of these metabolites on the etiology, progression and prognosis of CKD remains unclear.
Objective: We aimed to identify significant metabolic pathways in CKD progression by screening metabolites through metabolic profiling, thus identifying potential targets for CKD treatment.
Methods: Clinical data were collected from 145 CKD participants. GFR (mGFR) was measured by the iohexol method and participants were divided into four groups according to their mGFR. Untargeted metabolomics analysis was performed via UPLC-MS/MSUPLC–MSMS/MS assays. Metabolomic data were analyzed by MetaboAnalyst 5.0, one-way ANOVA, principal component analysis (PCA), and partial least squares discriminant analysis (PLS-DA) to identify differential metabolites for further analysis. The open database sources of MBRole2.0, including KEGG and HMDB, were used to identify significant metabolic pathways in CKD progression.
Results: Four metabolic pathways were classified as important in CKD progression, among which the most significant was caffeine metabolism. A total of 12 differential metabolites were enriched in caffeine metabolism, four of which decreased with the deterioration of the CKD stage, and two of which increased with the deterioration of the CKD stage. Of the four decreased metabolites, the most important was caffeine.
Conclusion: Caffeine metabolism appears to be the most important pathway in the progression of CKD as identified by metabolic profiling. Caffeine is the most important metabolite that decreases with the deterioration of the CKD stage.
1 Introduction
Chronic kidney disease (CKD) is a chronic disorder involving structural and functional organ changes. There are multiple underlying causes of this disorder which is characterized by its irreversibility and progressive development. CKD is a growing public health concern worldwide. As the disease progresses, the kidney’s ability to remove nitrogenous wastes, exogenous molecules, and metabolism of low molecular weight proteins decrease, resulting in multiple clinical sequelae. It is recognized that metabolites change with the development of kidney injury (Rysz et al., 2021). However, the effect of these metabolites on the etiology, progression, and prognosis of CKD remains unclear. The main clinical challenge is that current medical interventions aimed at delaying kidney decline are very limited. As such, the discovery of potential metabolic pathways could help us identify new therapeutic targets and address this serious problem (Chen et al., 2019a).
Several attempts have been made to identify new CKD-associated biomarkers and deepen our understanding of the pathological mechanisms underlying CKD (Dou et al., 2018; Xu et al., 2018). Metabolomics can be used to uncover metabolites associated with critical kidney functions such as the glomerular filtration rate (GFR), allowing for important clinical information to be obtained from biological samples (Earl et al., 2018; Lai et al., 2018) Rhee et al. reported that ten plasma metabolites are nominally associated with CKD progression (Rhee et al., 2016). In addition, Chen et al. found that five metabolites, including 5-methoxytryptophan (5-MTP), canavaninosuccinate (CSA), acetylcarnitine, tiglylcarnitine, and taurine, can be used to accurately identify the clinical stages of CKD, and that tryptophan hydroxylase-1 (TPH-1) presents a potential therapeutic target in CKD (Chen et al., 2019b). Feng et al. found that CKD rats can be differentiated from sham rats by metabolites involved in the pathways of gut microbial metabolism. They also found that improvement of gut dysbiosis retarded the progression of kidney disease in a rat model of CKD (Feng et al., 2019). Zhao et al. utilized an adenine-induced CKD model to identify perturbations in fatty acid metabolism, purine metabolism, and amino acid metabolism (Zhao et al., 2014). Brunetto et al. compared the serum metabolic profile of healthy and CKD dogs and found decreased urea, creatinine, creatine, citrate, lipids, lactate, branched-chain amino acids (BCAAs), and glutamine in CKD; a specific diet was able to maintain and retard the progression of CKD (Brunetto et al., 2021). Lanzon et al. analyzed the serum and urine of patients with severe obesity and CKD before and after undergoing bariatric surgery (BS) and found that isoleucine and tyrosine were increased in CKD patients compared to those without CKD (Lanzon et al., 2021). Gordin et al. (Gordin et al., 2019) used metabolic pathway analysis and reported that hexose, mitochondrial, amino acid, and purine pathways are associated with preserved kidney function.
Factors found to accelerate kidney decline have been explored in many studies. Most research involving metabolic profiling has focused on metabolites that increase with deteriorating renal function. However, in this study, we have focused on identifying the metabolic pathways associated with improved kidney function. By utilizing metabolic profiling, we attempted to identify metabolites that decrease as CKD deteriorates, ascertain the most relevant metabolic pathways, and identify potential therapeutic targets to improve kidney function.
2 Materials and methods
2.1 Participants and mGFR measurement
All participants provided informed consent before participating in the study according to a protocol approved by the Kiang Wu Hospitalethics committee. All participants were recruited in August 2019 from the Kiang Wu Hospital (Santo Antonio, Macau) and via outpatient clinics. The cohort included 145 patients who met the study inclusion criteria and were diagnosed with CKD based on the NKF-KDOI guidelines. Peripheral venous blood (4 mL) was collected from each patient; plasma samples were used for metabolomic analysis. Renal function was evaluated using GFR (mGFR) by utilizing the plasma clearance of iohexol (Shah et al., 2013). After blood was collected for the aforementioned tests, Iohexol was injected over 2 min (300 mg/mL, GE Healthcare, Shanghai, China) and plasma (6 mL) was collected from the contralateral upper extremity to detect the Iohexol concentration (by HPLC) at 120 and 240 min after Iohexol administration. For participants with eGFR<30 min/mL/1.73 m (Chen et al., 2019a), the blood collection times were changed to 120 and 300 min. All blood samples were centrifuged at 2000 g for 10 min at room temperature to extract plasma and stored at −80°C until analysis.
2.2 UPLC–MS/MS assays
All of the untargeted metabolomics analyses were conducted at the Dian Calibra-Metabolon Joint Metabolomics Laboratory (Hangzhou, China). Four different UPLC–MS/MS assays of small molecule metabolites were performed on each sample (Shen et al., 2020). Automatic liquid transfer during sample preparation was handled on a Hamilton automated MicroLab STAR® system (Hamilton, Switzerland). A methanol-based sample extraction solution was added to each sample and mixed using a GeneGrinder 2010 (Spex SamplePrep, United States of America) mixer. After 2 minutes of vigorous shaking and centrifugation to precipitate proteins and other debris, the extracted metabolites in the supernatant were collected and divided into four fractions: two fractions were analyzed by reversed-phase (RP) UPLC-MS/MS under positive electrospray ionization (ESI) mode. The two UPLC methods were slightly different using the same column (BEH C18 2.1 × 100 mm, 1.7 μm column, Waters). The mobile solutions for the two positive ESI UPLC-MS/MS were water and methanol containing 0.05% perfluoropentanoic acid (PFPA) and 0.1% formic acid (FA). The third fraction was used for reversed-phase UPLC-MS/MS in negative ion ESI mode (BEH C18 2.1 × 100 mm, 1.7 μm column, Waters), and the mobile solutions were methanol and water in 6.5 mM ammonium bicarbonate at pH 8. The last fraction was used for hydrophilic interaction liquid chromatography (HILIC)/UPLC-MS/MS in negative ESI mode (BEH Amide 2.1 × 150 mm, 1.7 μm column, Waters), and the mobile solutions consisted of water and acetonitrile with 10 mM ammonium formate at pH 10.8. Each fraction was dried under nitrogen gas flow and then dissolved in reconstitution solutions before being injected into each of the four UPLC-MS/MS systems. The QE mass spectrometer was alternated between full MS and data-dependent MS2 scans using dynamic exclusion for data collection. The scan range was 70–1,000 m/z. Processing, extraction, and peak identification of the raw mass spectrometry data were carried out using in-house developed software, and metabolites were identified by comparing the experimental ion characteristics to entries in an in-house library which was constructed using pure reference standards. The entries in the library included retention time/retention index (RI), mass to charge ratio (m/z), and MS/MS spectral data of each reference standard.
2.3 Metabolomics statistical analysis
All participants were divided into four groups according to mGFR: group A (mGFR<30 mL/min/1.73 m (Chen et al., 2019a)), group B (30 mL/min/1.73 m (Chen et al., 2019a)≤mGFR<60 mL/min/1.73 m (Chen et al., 2019a)), group C (60 mL/min/1.73 m (Chen et al., 2019a)≤mGFR <90 mL/min/1.73 m (Chen et al., 2019a)), and group D (mGFR ≥90 mL/min/1.73 m (Chen et al., 2019a)). Statistical analysis of patient data was carried out using SPSS 26.0. Statistical significance was determined using a threshold of p = 0.05. Metabolomic data analyses were carried out using MetaboAnalyst 5.0 (https://www.metaboanalyst.ca). The mass spectrometry data which were acquired by untargeted metabolomics analysis were uploaded as comma separated values (.csv). The uploaded data file contains a data matrix of 145 (samples) × 1,094 (compounds). Before data analysis, a data integrity check was performed to ensure that all the necessary information had been collected. To minimize bias associated with the omission of censored data, all missing and zero values were replaced by half of the minimum positive values across samples in the original data. Normalization was done via log transformation and Pareto scaling.
One-way analysis of variance (ANOVA), principal component analysis (PCA), and partial least squares-discriminant analysis (PLS-DA) were used to screen out the differential metabolites. MetaboAnalyst 5.0 provided one-way ANOVA test results to determine whether the overall comparison among each group was significant. Univariate analyses provided a preliminary overview of features that are potentially significant in discriminating the conditions under investigation. Statistical significance was determined using a threshold of p = 0.05.
PCA and PLS-DA were also performed using MetaboAnalyst 5.0. PCA is an unsupervised method aiming to find the directions that best explain the variance in a data set X) without referring to class labels Y). The data are summarized into much fewer variables called scores, which are weighted averages of the original variables. PLS is a supervised method that uses multivariate regression techniques to extract, via a linear combination of the original variables X), information that can predict class membership Y). To assess the significance of class discrimination, a permutation test was performed. In each permutation, a PLS-DA model was built between the data X) and the permuted class labels Y) using the optimal number of components determined by cross-validation for the model based on the original class assignment. MetaboAnalyst supports two types of test statistics for measuring class discrimination. The first one is based on prediction accuracy during training. The second is the separation distance based on the ratio of the between-group sum of the squares and the within-group sum of squares (B/Wratio). If the observed test statistic is part of the distribution based on the permuted class assignments, the class discrimination cannot be considered statistically significant. Variable Importance in Projection (VIP), which is an important variable in PLS-DA, is a weighted sum of squares of the PLS loadings taking into account the amount of explained Y-variation in each dimension. VIP scores are calculated for each component. When more components are used to calculate the feature importance, the average of the VIP scores is used. A VIP threshold >1.0 was considered statistically significant.
2.4 Pathway analysis
Metabolic pathways were identified by utilizing open database sources of MBRole2.0 (http://csbg.cnb.csic.es/mbrole2/), including KEGG and HMDB. Compound names of the differential metabolites were first converted to KEGG IDs using MetaboAnalyst 5.0 and the KEGG IDs were submitted to MBRole 2.0 for KEGG pathway analysis.
3 Results
3.1 Characteristics of the study populations
We recruited 145 individuals aged 20 to 96 years, 68 of whom were male. Based on mGFR, 22 were assigned to group A, 47 to group B, 39 to group C, and 37 to group D. Table 1 presents the summary statistics for each group. Age, weight, body mass index (BMI), systolic blood pressure, diastolic blood pressure, creatinine, eGFR, and mGFR were symmetrically distributed. Arithmetic means and standard deviations are provided. The p-values of one-way ANOVA tests are also presented. Creatinine, mGFR and eGFR were used a trend test, and the Ptrend values were presented. The height followed an asymmetric distribution, and thus median and interquartile ranges are shown. The p-values of the Kruskal–Wallis test are presented. Sex, current smoking, current drinking, diabetes, hypertension, coronary heart disease, stroke, hyperuricemia, use of antiplatelet drugs, antilipemic agents, antihypertensive agents, hypoglycemic agents, immunosuppressors, and uric acid reduction medicine were dichotomous variables. For these, quantities and frequencies are shown as appropriate. The p-values of Chi-square tests are presented. There were no cases of current drinking, stroke, and immunosuppressive drug use.
3.2 Univariate analysis
Before data analysis, a data integrity check was performed to make sure that all the necessary information had been collected. The data normalization result implemented by MetaboAnalyst5.0 provided in Figure 1 supplement. After one-way ANOVA for multigroup analysis, Table 2 supplement shows all significant metabolites selected by ANOVA with p-value threshold 0.05 and Table 2 details these findings for the top 50 metabolites. ANOVA only tells whether the overall comparison is significant or not, it is followed by post hoc analyses in order to identify which two levels are different (Table 2 supplement).
3.3 Principal component analysis (PCA)
Figure 1 shows the results of the PCA; the separation trend among each group is shown, indicating that each group had a unique metabolic spectrum.
3.4 Partial least squares-discriminant analysis (PLS-DA)
PLS-DA was used to better distinguish the overall difference in the metabolic spectrum among each group and determine the metabolites that were most characteristic of each group. Figure 2 shows the 2D score plot between selected components; Figure 2 supplement shows the classification performance with different number of components; and Figure 3 shows important features identified by PLS-DA. To assess the significance of class discrimination, a permutation test was performed. In each permutation, a PLS-DA model was built between the data and the permuted class labels using the optimal number of components determined by cross validation for the model based on the original class assignment (Figure 3 supplement). VIP scores are calculated for each component. When more than components are used to calculate the feature importance, the average of the VIP scores was used. The average VIP value of caffeine was the highest among the metabolites that decreased with mGFR deterioration.
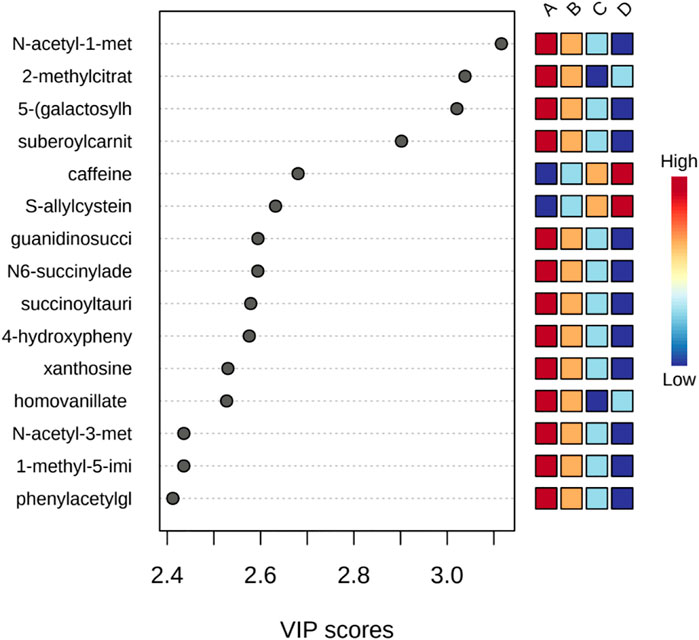
FIGURE 3. Important features identified by PLS-DA. The colored boxes on the right indicate the relative concentrations of the corresponding metabolite in each group under study.
3.5 Pathway analysis
Differential metabolites were selected by one-way ANOVA, PCA, and PLS-DA. The differential metabolites were p < 0.05 in one one-way ANOVA and VIP>1 in PLS-DA. The metabolites of VIP>1 are presented in Table 3 plsda_vip supplement. The KEGG ID of each differential metabolite was found through MetaboAnalyst 5.0. KEGG IDs of differential metabolites were uploaded to MBRole2.0 and the background was set as the full database for KEGG pathway analysis. MBRole performs an overrepresented (enrichment) analysis of categorical annotations for a set of compounds of interest. These categorical annotations correspond to biological and chemical information available in several public databases and software. Caffeine metabolism, metabolic pathways, pyrimidine metabolism, and histidine metabolism were classified as important in Table 3. Caffeine metabolism was classified as the most important pathway compared with the three other identified pathways. Metabolic pathways included the other three metabolic pathways, so further analysis was directed at the metabolites enriched in these three metabolic pathways (Figure 4).
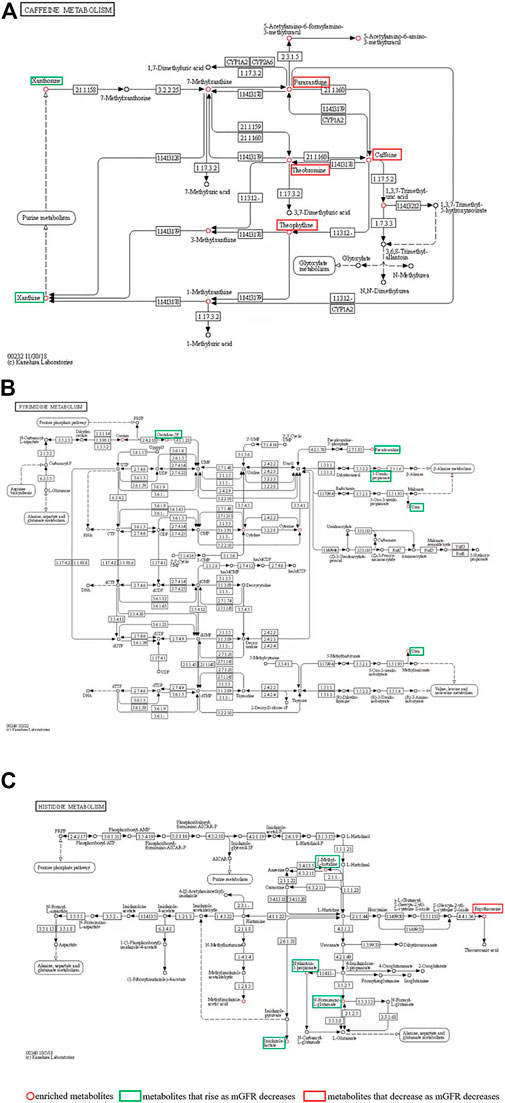
FIGURE 4. (A) Caffeine metabolism pathways; (B) Pyrimidine metabolism; (C) Histidine metabolism. The red circle represents the enriched metabolites; the green boxes are metabolites that rise as mGFR decreases; the red boxes are metabolites that decrease as mGFR decreases.
3.6 Caffeine metabolism accumulation of multiple metabolites decreased with the deterioration of CKD
The caffeine metabolism pathway mapped by MBRole2.0 enriched 12 metabolites (Table 3), including Xanthine, Xanthosine, Theophylline, Theobromine, Caffeine, Paraxanthine, 7-Methylxanthine, 3-Methylxanthine, 1-Methylxanthine, 1,3,7-Trimethyluric acid, 5-Acetylamino-6-formylamino-3-methyluracil, and 5-Acetylamino-6-amino-3-methyluracil, which were circled in red in Figure 4A. The levels of paraxanthine, theobromine, caffeine, and theophylline decreased with renal deterioration (metabolites in red boxes in Figure 4A). Xanthosine and xanthine increased with renal deterioration (metabolites in green boxes in Figure 4A).
4 Discussion
In this study, we enrolled 145 CKD individuals who were divided into four groups based on mGFR. Many differential metabolites were screened out by metabolic profiling. Through pathway analysis of differential metabolites, we report for the first that the caffeine metabolism pathway is critical in CKD. We showed that in this pathway, paraxanthine, theobromine, caffeine, and theophylline decreased with poorer renal function, while xanthosine and xanthine increased. Caffeine was the most important metabolite, decreasing with deteriorating renal function.
Previous studies have found that coffee consumption may reduce the risk of CKD (Li et al., 2021), showing a negative association between caffeine intake and all-cause mortality in patients with CKD (Bigotte et al., 2019). However, other studies have shown that caffeine exacerbates hypertension in rats with polycystic kidney disease (Tanner and Tanner, 2001), potentiates the development of more severe tubulointerstitial changes, and increases focal glomerulosclerosis (Tofovic et al., 2002). High caffeine-sugar content increases the incidence of cardiovascular disease and tissue inflammation by altering lipid profiles and blood glucose (Eltahir et al., 2020). Yu et al. (Yu et al., 2016) found that acute caffeine intake causes an acute increase in blood pressure, while chronic caffeine intake decreases blood pressure; the latter may be related to a diuretic effect. Chronic caffeine consumption also reduces sodium absorption, contributing to its antihypertensive effects in salt-sensitive rats (Wei et al., 2018). This study found that caffeine metabolism was the most important pathway and that caffeine was the most important metabolite.
In our study, paraxanthine, theobromine, and theophylline decreased with poorer renal function. It is known that theobromine activates sirtuin one to reduce extracellular matrix accumulation in the kidneys of diabetic rats (Papadimitriou et al., 2015); a single dose of prophylactic theophylline has been shown to prevent acute kidney injury (AKI)/severe kidney dysfunction in term neonates with severe birth asphyxia (Bhatt et al., 2019). This study corroborated that theobromine and theophylline improve the progression of CKD. The effect of paraxanthine on CKD is still unclear. In this study, paraxanthine decreased with decreasing mGFR, which may also improve the progression of CKD.
We also found that xanthosine and xanthine increased with poorer renal function. Chen et al. (Chen et al., 2020) demonstrated that xanthosine is associated with significantly greater risks of CKD progression. Xanthine is an intermediate metabolite of uric acid (UA), converted by xanthine oxidase (XO). Previous research suggests a pathogenic role of hyperuricemia in the development of CKD (Uedono et al., 2015; Mallat et al., 2016). XO inhibitors have been suggested to slow the progression of kidney disease (Pisano et al., 2017) but this remains controversial (Kimura et al., 2018). The present study supports that xanthosine and xanthine are associated with CKD progression.
We found that metabolites that are associated with CKD progression can be converted to more ‘desirable’ metabolites, as shown in Figure 4A. This conversion involves many enzymes that are shown in Figure 4A. Thus, the regulation of these enzymes offers a potential target for CKD treatment. In subsequent research, we plan to focus on these enzymes. One limitation was that we did not record the baseline caffeine ingestion in our participants; the influence of caffeine ingestion on renal function in CKD should be observed in subsequent studies.
In this study, we found that in pyrimidine metabolism and histidine metabolism (Figures 4B,C), orotidine-5P, pseudouridine, 3-Ureidopropionate, urea, 1-Methyl-L-histidine, Hydantoin-5-propionate, N-Formimino-L-glutamate and imidazole lactate rise as mGFR decreases. Consistent with previously reported results, pseudouridine is extremely correlated with mGFR and might be an ideal biomarker for CKD (Peng et al., 2022). Urea is an endogenous marker in CKD (Weiner et al., 2015). The significance of the accumulation of the other six metabolites in CKD has not been reported. These eight metabolites have limited significance in the search for targets to protect kidney function. Moreover, in histidine metabolism, ergothioneine which is absorbed from the intestine through food intake may function as a major antioxidant (Cheah et al., 2017), and the protective effect on the kidney can be further investigated.
This study has potential limitations. Based on the metabolomic approach, we identified the significance of caffeine metabolism in CKD, but the mechanisms linking caffeine metabolism to CKD have yet to be clarified, and the exact intricate mechanism needs additional animal experiments and prospective studies. Future investigations will need to include more animal experiments and prospective studies to elucidate the mechanism and the significance of caffeine metabolism in CKD identified in this study.
5 Conclusion
In conclusion, metabolic profiling identified caffeine metabolism as the most important pathway in CKD progression. Decreased renal function was associated with decreased paraxanthine, theobromine, caffeine, and theophylline, and with increased xanthosine and xanthine. Caffeine was the most important metabolite associated with CKD deterioration.
6 Summary at a glance
Metabolic profiling was used to identify significant metabolic pathways in CKD progression. Four metabolic pathways were classified as important in the progression of CKD. Caffeine metabolism appears to be the most important pathway. Caffeine is the most important metabolite that decreases with the deterioration of CKD stage.
Data availability statement
The original contributions presented in the study are included in the article/supplementary material, further inquiries can be directed to the corresponding authors.
Ethics statement
The studies involving human participants were reviewed and approved by the Institutional Review Board of Ethics Commission of Kiang Wu Hospital (KWH 2018-001). The patients/participants provided their written informed consent to participate in this study. Written informed consent was obtained from the individual(s) for the publication of any potentially identifiable images or data included in this article.
Author contributions
All authors have read and approved the manuscript. XG and HP: Conceptualization, methodology, and writing - original draft preparation. HP: Funding acquisition. PL: Visualization. LT: Investigation. JF: Software and Validation. CA: Supervision. TT and TsT: Data curation. HP and XL: Writing-review, funding and editing.
Funding
This study was supported by the Science and Technology Development Fund, Macau SAR (File no. 0032/2018/A1), the National Natural Science Foundation of China (Grant No.81873631, 81370866, 81070612) and the Guangzhou Science and technology planning project (Grant No.202002020047), NSFC-Guangdong United Fund (Grant No. 2020B1515120037).
Acknowledgments
The authors would like to express their gratitude to EditSprings (https://www.editsprings.cn) for the expert linguistic services provided.
Conflict of interest
The authors declare that the research was conducted in the absence of any commercial or financial relationships that could be construed as a potential conflict of interest.
Publisher’s note
All claims expressed in this article are solely those of the authors and do not necessarily represent those of their affiliated organizations, or those of the publisher, the editors and the reviewers. Any product that may be evaluated in this article, or claim that may be made by its manufacturer, is not guaranteed or endorsed by the publisher.
References
Bhatt, G. C., Gogia, P., Bitzan, M., and Das, R. R. (2019). Theophylline and aminophylline for prevention of acute kidney injury in neonates and children: A systematic review. Arch. Dis. Child. 104 (7), 670–679. doi:10.1136/archdischild-2018-315805
Bigotte, V. M., Magrico, R., Viegas, D. C., Leitao, L., and Neves, J. S. (2019). Caffeine consumption and mortality in chronic kidney disease: A nationally representative analysis. Nephrol. Dial. Transpl. 34 (6), 974–980. doi:10.1093/ndt/gfy234
Brunetto, M. A., Ruberti, B., Halfen, D. P., Caragelasco, D. S., Vendramini, T. H. A., Pedrinelli, V., et al. (2021). Healthy and chronic kidney disease (CKD) dogs have differences in serum metabolomics and renal diet may have slowed disease progression. Metabolites 11 (11), 782. doi:10.3390/metabo11110782
Cheah, I. K., Tang, R. M., Yew, T. S., Lim, K. H., and Halliwell, B. (2017). Administration of pure ergothioneine to healthy human subjects: Uptake, metabolism, and effects on biomarkers of oxidative damage and inflammation. Antioxid. Redox Signal 26 (5), 193–206. doi:10.1089/ars.2016.6778
Chen, D. Q., Cao, G., Chen, H., Argyopoulos, C. P., Yu, H., Su, W., et al. (2019). Identification of serum metabolites associating with chronic kidney disease progression and anti-fibrotic effect of 5-methoxytryptophan. Nat. Commun. 10 (1), 1476. doi:10.1038/s41467-019-09329-0
Chen, Y. Y., Chen, D. Q., Chen, L., Liu, J. R., Vaziri, N. D., Guo, Y., et al. (2019). Microbiome-metabolome reveals the contribution of gut-kidney axis on kidney disease. J. Transl. Med. 17 (1), 5. doi:10.1186/s12967-018-1756-4
Chen, Y., Zelnick, L. R., Wang, K., Hoofnagle, A. N., Becker, J. O., Hsu, C. y., et al. (2020). Kidney clearance of secretory solutes is associated with progression of CKD: The CRIC study. J. Am. Soc. Nephrol. 31 (4), 817–827. doi:10.1681/asn.2019080811
Dou, F., Miao, H., Wang, J. W., Chen, L., Wang, M., Chen, H., et al. (2018). An integrated lipidomics and phenotype study reveals protective effect and biochemical mechanism of traditionally used alisma orientale juzepzuk in chronic kidney disease. Front. Pharmacol. 9, 53. doi:10.3389/fphar.2018.00053
Earl, D. C., Ferrell, P. J., Leelatian, N., Froese, J. T., Reisman, B. J., Irish, J. M., et al. (2018). Discovery of human cell selective effector molecules using single cell multiplexed activity metabolomics. Nat. Commun. 9 (1), 39. doi:10.1038/s41467-017-02470-8
Eltahir, H. M., Alamri, G., Alamri, A., Aloufi, A., Nazmy, M., Elbadawy, H., et al. (2020). The metabolic disorders associated with chronic consumption of soft and energy drinks in rats. Acta Biochim. Pol. 67 (1), 79–84. doi:10.18388/abp.2020_2914
Feng, Y. L., Cao, G., Chen, D. Q., Vaziri, N. D., Chen, L., Zhang, J., et al. (2019). Microbiome-metabolomics reveals gut microbiota associated with glycine-conjugated metabolites and polyamine metabolism in chronic kidney disease. Cell Mol. Life Sci. 76 (24), 4961–4978. doi:10.1007/s00018-019-03155-9
Gordin, D., Shah, H., Shinjo, T., St-Louis, R., Qi, W., Park, K., et al. (2019). Characterization of glycolytic enzymes and pyruvate kinase M2 in type 1 and 2 diabetic nephropathy. Diabetes Care 42 (7), 1263–1273. doi:10.2337/dc18-2585
Kimura, K., Hosoya, T., Uchida, S., Inaba, M., Makino, H., Maruyama, S., et al. (2018). Febuxostat therapy for patients with stage 3 CKD and asymptomatic hyperuricemia: A randomized trial. Am. J. Kidney Dis. 72 (6), 798–810. doi:10.1053/j.ajkd.2018.06.028
Lai, Z., Tsugawa, H., Wohlgemuth, G., Mehta, S., Mueller, M., Zheng, Y., et al. (2018). Identifying metabolites by integrating metabolome databases with mass spectrometry cheminformatics. Nat. Methods 15 (1), 53–56. doi:10.1038/nmeth.4512
Lanzon, B., Martin-Taboada, M., Castro-Alves, V., Vila-Bedmar, R., Gonzalez de Pablos, I., Duberg, D., et al. (2021). Lipidomic and metabolomic signature of progression of chronic kidney disease in patients with severe obesity. Metabolites 11 (12), 836. doi:10.3390/metabo11120836
Li, Y., Li, W., Lu, Y., and Zhang, J. (2021). Coffee consumption is associated with a decreased risk of incident chronic kidney disease: A protocol for systematic review and meta-analysis. Med. Baltim. 100 (37), e27149. doi:10.1097/md.0000000000027149
Mallat, S. G., Al, K. S., Tanios, B. Y., and Jurjus, A. (2016). Hyperuricemia, hypertension, and chronic kidney disease: An emerging association. Curr. Hypertens. Rep. 18 (10), 74. doi:10.1007/s11906-016-0684-z
Papadimitriou, A., Silva, K. C., Peixoto, E. B., Borges, C. M., Lopes de Faria, J. M., and Lopes de Faria, J. B. (2015). Theobromine increases NAD(+)/Sirt-1 activity and protects the kidney under diabetic conditions. Am. J. Physiol. Ren. Physiol. 308 (3), F209–F225. doi:10.1152/ajprenal.00252.2014
Peng, H., Liu, X., Aoieong, C., Tou, T., Tsai, T., Ngai, K., et al. (2022). Identification of metabolite markers associated with kidney function. J. Immunol. Res. 2022, 1–9. doi:10.1155/2022/6190333
Pisano, A., Cernaro, V., Gembillo, G., D’Arrigo, G., Buemi, M., and Bolignano, D. (2017). Xanthine oxidase inhibitors for improving renal function in chronic kidney disease patients: An updated systematic review and meta-analysis. Int. J. Mol. Sci. 18 (11), 2283. doi:10.3390/ijms18112283
Rhee, E. P., Clish, C. B., Wenger, J., Roy, J., Elmariah, S., Pierce, K. A., et al. (2016). Metabolomics of chronic kidney disease progression: A case-control analysis in the chronic renal insufficiency cohort study. Am. J. Nephrol. 43 (5), 366–374. doi:10.1159/000446484
Rysz, J., Franczyk, B., Lawinski, J., Olszewski, R., Cialkowska-Rysz, A., and Gluba-Brzozka, A. (2021). The impact of CKD on uremic toxins and gut microbiota. Toxins (Basel). 13 (4), 252. doi:10.3390/toxins13040252
Shah, V. O., Townsend, R. R., Feldman, H. I., Pappan, K. L., Kensicki, E., and Vander Jagt, D. L. (2013). Plasma metabolomic profiles in different stages of CKD. Clin. J. Am. Soc. Nephrol. 8 (3), 363–370. doi:10.2215/cjn.05540512
Shen, B., Yi, X., Sun, Y., Bi, X., Du, J., Zhang, C., et al. (2020). Proteomic and metabolomic characterization of COVID-19 patient sera. Cell 182 (1), 59–72.e15. doi:10.1016/j.cell.2020.05.032
Tanner, G. A., and Tanner, J. A. (2001). Chronic caffeine consumption exacerbates hypertension in rats with polycystic kidney disease. Am. J. Kidney Dis. 38 (5), 1089–1095. doi:10.1053/ajkd.2001.28614
Tofovic, S. P., Kost, C. J., Jackson, E. K., and Bastacky, S. I. (2002). Long-term caffeine consumption exacerbates renal failure in obese, diabetic, ZSF1 (fa-fa(cp)) rats. Kidney Int. 61 (4), 1433–1444. doi:10.1046/j.1523-1755.2002.00278.x
Uedono, H., Tsuda, A., Ishimura, E., Yasumoto, M., Ichii, M., Ochi, A., et al. (2015). Relationship between serum uric acid levels and intrarenal hemodynamic parameters. Kidney Blood Press Res. 40 (3), 315–322. doi:10.1159/000368507
Wei, X., Lu, Z., Yang, T., Gao, P., Chen, S., Liu, D., et al. (2018). Stimulation of intestinal Cl- secretion through CFTR by caffeine intake in salt-sensitive hypertensive rats. Kidney Blood Press Res. 43 (2), 439–448. doi:10.1159/000488256
Weiner, I. D., Mitch, W. E., and Sands, J. M. (2015). Urea and ammonia metabolism and the control of renal nitrogen excretion. Clin. J. Am. Soc. Nephrol. 10 (8), 1444–1458. doi:10.2215/cjn.10311013
Xu, X., Eales, J. M., Akbarov, A., Guo, H., Becker, L., Talavera, D., et al. (2018). Molecular insights into genome-wide association studies of chronic kidney disease-defining traits. Nat. Commun. 9 (1), 4800. doi:10.1038/s41467-018-07260-4
Yu, H., Yang, T., Gao, P., Wei, X., Zhang, H., Xiong, S., et al. (2016). Caffeine intake antagonizes salt sensitive hypertension through improvement of renal sodium handling. Sci. Rep. 6, 25746. doi:10.1038/srep25746
Keywords: metabolomics, chronic kidney disease, glomerular filtration rate, caffeine metabolism, caffeine
Citation: Guo X, Peng H, Liu P, Tang L, Fang J, Aoieong C, Tou T, Tsai T and Liu X (2023) Metabolic profiling identifies the significance of caffeine metabolism in CKD. Front. Bioeng. Biotechnol. 11:1006246. doi: 10.3389/fbioe.2023.1006246
Received: 29 July 2022; Accepted: 06 February 2023;
Published: 17 February 2023.
Edited by:
Di Li, First Hospital of Jilin University, ChinaReviewed by:
Karen De Jesus Nicácio, Federal University of Mato Grosso, BrazilTieci Yi, First Hospital, Peking University, China
Copyright © 2023 Guo, Peng, Liu, Tang, Fang, Aoieong, Tou, Tsai and Liu. This is an open-access article distributed under the terms of the Creative Commons Attribution License (CC BY). The use, distribution or reproduction in other forums is permitted, provided the original author(s) and the copyright owner(s) are credited and that the original publication in this journal is cited, in accordance with accepted academic practice. No use, distribution or reproduction is permitted which does not comply with these terms.
*Correspondence: Hongquan Peng, hpeng93170@gmail.com; Xun Liu, naturestyle@163.com
†ORCID: Xinghua Guo, orcid.org/0000-0003-0774-5817; Hongquan Peng, orcid.org/0000-0003-4893-7903; Xun Liu, orcid.org/0000-0002-2360-1429