- 1Department of Biomedical Engineering, National Cheng Kung University, Tainan, Taiwan
- 2Department of Physical Medicine Rehabilitation, National Cheng Kung University Hospital, Tainan, Taiwan
- 3Medical Device Innovation Center, National Cheng Kung University, Tainan, Taiwan
There are several causes that can lead to functional weakness in the hands or upper extremities (UE), such as stroke, trauma, or aging. Therefore, evaluation and monitoring of UE rehabilitation have become essential. However, most traditional evaluation tools (TETs) and assessments require clinicians to assist or are limited to specific clinical settings. Several novel assessments might apply to wearable devices, yet those devices will still need clinicians or caretakers to help with further tests. Thus, a novel UE assessment device that is user-friendly and requires minimal assistance would be needed. The cylindrical grasp is one of the common UE movements performed in daily life. Therefore, a cylindrical sensor-embedded holding device (SEHD) for training and monitoring was developed for a usability test within this research. The SEHD has 14 force sensors with an array designed to fit holding positions and a six-axis inertial measurement unit (IMU) to monitor grip strength, hand dexterity, acceleration, and angular velocity. Six young adults, six healthy elderly participants, and three stroke survivors had participated in this study to see if the SEHD could be used as a reference to TETs. During result analyses, where the correlation coefficient analyses were applied, forearm rotation smoothness and the Purdue Pegboard Test (PPT) showed a moderate negative correlation [r (16) = −0.724, p < 0.01], and the finger independence showed a moderate negative correlation with the PPT [r (10) = −0.615, p < 0.05]. There was also a highly positive correlation between the maximum pressing task and Jamar dynamometer in maximum grip strength [r (16) = 0.821, p < 0.01]. These outcomes suggest that the SEHD with simple movements could be applied as a reference for users to monitor their UE ability.
Introduction
The elderly population is rapidly increasing thanks to better medical support globally (Nation, 2019). Several studies have suggested that normal aging might alter physical, mental, and social health (Onder et al., 2002). Physical changes include decreased strength and mobility (Chodock et al., 2020). In particular, upper extremity (UE) functions and strategies may affect activities of daily living (ADL) due to physical changes in normal aging (Reissner et al., 2019). However, aging is not the only cause of UE dysfunction; these physical problems are also seen in patients who have suffered stroke (Shi et al., 2011), trauma (Dowrick et al., 2005; Crowe et al., 2020), Parkinson’s disease (Quinn et al., 2013), and other UE musculoskeletal disorders (Huisstede et al., 2006) such as carpal tunnel syndrome (Yoshida et al., 2019). In addition, UE functions are important components of ADL capability and may impact the quality of life (QoL) (Kanti Majumdar, 2014). Therefore, assessing and monitoring UE function is critical for people with varying degrees of dysfunction.
Several clinical evaluation methods could be applied to evaluate one’s UE functions (Kim et al., 2016). However, most of them are not digitized and based on clinical observations. For example, the common assessment tool for UE are the Upper Extremity Functional Index (UEFI-20 and UEFI-15), which is a self-administered questionnaire consisting of activity lists. Users give a score to each activity based on its difficulty of completion. Healthy individuals can score 80 out of 80 in UEFI-20 or 100 out of 100 in UEFI-15 (13). Aside from the self-administered questionnaires, the Purdue Pegboard Test (PPT) is one of the most commonly used clinical evaluation tools for fine and gross motor dexterity and coordination. The participants perform tasks within the designed period and clinicians calculate their performances based on how many pieces (pegs) are placed in the desired locations (holes) during the tasks. It is a performance-based outcome measure (Sigirtmac and Oksuz, 2022). The normal range of the score can vary due to the different age groups and genders. For example, in the healthy male group aged 21 to 25 years, the average PPT is 15.44 ± 1.71 in the preferred hand task (Yeudall et al., 1986; Marvin, 2012). The PPT could evaluate one’s hand dexterity and UE gross movements. However, the outcome measure of the PPT presents the number of pieces that might not present one’s UE abilities on a different scale. It could be considered an overall evaluation tool instead of a segmented ability evaluation. Besides PPT, the Jamar dynamometer is a common clinical assessment instrument for upper extremities; it evaluates one’s grip-force. It is considered a gold standard tool with excellent validity and reliability in research and the clinic (Mathiowetz, 2002; Bohannon et al., 2006). However, similar to the PPT, the Jamar dynamometer requires at least one clinician to be on-site, which calls for additional human labor. Clinicians must be trained before collecting or grading subjects’ functions. An experienced clinician might grade differently from an inexperienced one when operating the same evaluation tool on the same patient. Therefore, some current clinical assessments might end up with more subjective results based on the clinician’s experience (Kim et al., 2013). Aside from that, several tests have ceiling effects, meaning healthy individuals might get total scores even though their hand functions may differ. Moreover, many people do not realize or deny functional changes in their UE with age, so they do not visit the clinic or hospital for further evaluations (Kim et al., 2016). Therefore, there is a need for an easily accessible device that can digitize the assessment results to provide a reference for clinicians, which will, in turn, also help the users.
Several wearable smart gloves have been invented to help people with UE functional impairments perform training or rehabilitation (Wang et al., 2014; Shin et al., 2016). However, such wearable smart gloves might not be suitable for all users. Researchers have developed data gloves embedded with different sensors, such as inertia measurement unit (IMU) sensor-embedded gloves (Hsiao et al., 2015; Lin et al., 2017; Lin et al., 2018), data gloves with force sensors (Tarchanidis and Lygouras, 2003; Hsiao et al., 2015), data gloves with flexible sensors (Tognetti et al., 2006), and gloves with flexible optic fiber bending transducers (Fujiwara et al., 2014). However, studies have shown that the elderly resist the adoption of assistive technology for reasons such as (A) the devices not being easy to set up; (B) the devices being so expensive that they are afraid of breaking or losing them; (C) or the caretakers not bothering to help them put these on (Yusif et al., 2016; Golant, 2017). Aside from that, although some of the data gloves use force sensors, many of the force sensors in the gloves do not provide actual force acquisition due to erroneous placement of the sensors. Most wearable devices use pseudo-augmented reality environments (screen projections) or virtual reality environments to integrate with the hardware. However, the lack of tactile feedback of the actual object might lead to inappropriate force application during training or rehabilitation. Although smart gloves are considered a potential developing technology and many companies and research groups are devoted to these in this field, most wearable smart gloves are still not widely used (Caeiro-Rodríguez et al., 2021).
Grabbing is one of the earliest reflexes in human development (Halverson, 1937), while gripping and grasping are intuitive movements in daily living (Yang et al., 2015). The three holding-related verbs might be confusing. The movements of gripping, grasping, and grabbing are all similar since they use the hands to hold and apply appropriate force to keep and move the object. These movements are also different in that they require different reaction times to make contact with the object, e.g., grabbing represents a quicker action than gripping. In other words, the grasping movement tends to be more planned, while gripping is more intuitive. In this article, we will use “grasping” to present the planned holding movement. Generally, there are two major types of grasping: power grasp and precision grasp. Power grasp, which means force application, is the key element in movements such as the large wrap, cylindrical grasp, and power sphere grasp. Precision grasp, which means the stability of the object or trajectory of the following movement, is more important than the force application of the movement, such as the writing tripod and precision sphere. In these two types of grasping, several subtypes are categorized by the involvement of the digits, grasping shapes, and purposes (Yang et al., 2015; Cai et al., 2016).
Grasping is a complex movement that involves motor planning and the proper use of force application. Many muscles and the nervous system are involved in grasping (Castiello, 2005; Koester et al., 2016; Chen et al., 2022). Different people can employ different strategies when performing the same task and respond differently to different outcomes. Studies have shown that motor learning and motor control improve with more sensory inputs during movements (Chiu et al., 2009; Cano-De-La-Cuerda et al., 2015; Sani and Shanechi, 2021). The complexity of grasping can enhance brain activation by observing the environment (occipital cortex), understanding and planning movements (prefrontal cortex), and performing responsive movements (premotor cortex, primary motor cortex, and complementary motor cortex).
Overall, sensory feedback collected during grasping may help improve force application and motor performance. Therefore, the idea of innovating a grasping device for evaluation, monitoring, and rehabilitation was initiated and developed.
The purposes of this study are to (A) develop the novel sensor-embedded holding device (SEHD) for functional evaluation of grip-force and movements and (B) apply the SEHD to collect useful data that could further be used as references for clinicians to monitor UE functions.
The hypothesis of this study is that the SEHD could offer data, which after being processed, could provide results that can be considered as references to traditional evaluation tools (TETs) for users and clinicians to monitor the conditions of the users’ UE functions.
Design of upper extremities evaluation device
Design of hardware and its purposes
According to previous studies related to handgrips, holding devices could provide higher degrees of freedom to perform various movements, such as lifting, rotation, and horizontal position modification (Harwin and Barrow, 2013; Turella and Lingnau, 2014; Yang et al., 2015). In addition, studies related to grip force during movements suggest that data recorded from surface pressure or force sensors appear to facilitate further analysis to understand patterns of hand movement and hand function (Hsu et al., 2021a; Chen et al., 2022). Therefore, the design of the SEHD includes an inertial motor unit (IMU) that could provide speed and angular profiles. Based on this, we also encouraged the development of SEHD to collect sensory feedback.
Overall, the SEHD was designed and developed around several goals: 1) the SEHD should be capable of acquiring digitized data from different hand movements and UE movements; 2) it should be used and accepted by different users, such as the elderly, people with hand/UE disabilities, those who had survived a stroke, and children with developmental delays; 3) it should be affordable for the public and provide valuable analysis for monitoring, assessment, and even auxiliary training in the future. Therefore, designing and developing a proper SEHD appearance with useful functional details was very important and challenging.
Development of hardware and data collection
The cylindrical grasp is considered one of the common UE movements in daily living (Vergara et al., 2014). The cylindrical design of the SHED comes from a widely accepted everyday item—the soda can (diameter: 65 mm and height: 140 mm), which is a widely accepted daily object. Relative research supports the idea that the cylindrical shape for grasping was easy to use and could offer a nice grip feeling (Harih and Dolšak, 2014). A diameter of 65 mm was decided in accordance with the study on grip strength (Domalain et al., 2008) and the average hand length (Guerra et al., 2014) such that the SEHD could be wrapped comfortably by the participants. The initial design focused on the functionality of the device. The commercial circuit board and sensors were adapted for the SEHD.
We used 3D printing to build the shell, which consisted of two materials: polylactic acid (PLA) and thermoplastic elastomer (TPE). PLA was used for the hard shell of the case, while TPE was used for the elastic, flexible cover of the force sensors. Red and white were used for different materials because people tend to stick their palms to the colored areas intuitively. The weight of the object was 200 g, and the weight of the device linked to the IC board was 500 g.
A six-axis inertia measurement unit (IMU) and 14 force sensors were embedded in the SEHD (Figure 1). The IMU has been widely applied in many devices to evaluate or monitor different movement tasks (Hsu et al., 2021b; Nikkhoo et al., 2021). The embedded IMU was an MPU-6050 sensor (GY-521) from InvenSense, which has been applied in many studies related to motion control, wearable device innovation, and movement sensation inputs (Ngui and Lee, 2019; Karnik et al., 2020; Ares et al., 2022). The validity and reliability of this model of IMU have been demonstrated in many of the previous studies that have been accepted for clinical applications. The IMU was placed at the center of the device so as to measure movement parameters, and the force sensors were placed under the TPE cover. Wires linked the processing board with the device. The output of the processing board provides data transfer and power to a PC or laptop via micro USB (Figure 2, Figure 3).
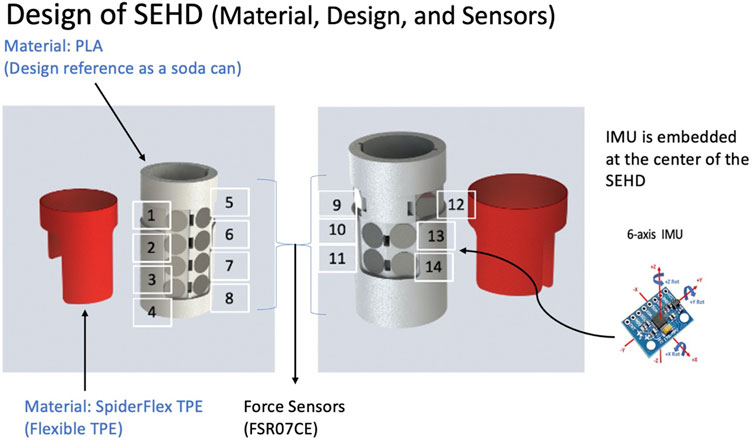
FIGURE 1. Design of the sensor-embedded holding device. Fourteen pressure sensors (FSR07CE, Ohmite) and the placement of the pressure sensors. Six-axis inertial measurement unit placed at the center of device. Inner part of structure made of PLA and outer part of the holding piece made of TPE material, and a 3D printer made both parts.
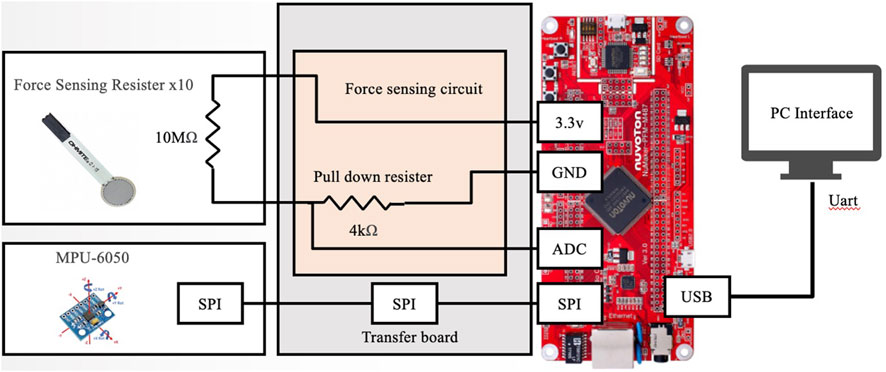
FIGURE 3. Circuit layout of SEHD: 14 force sensors linked to processing board (NuMaker-PFM-M487, Nuvoton, Taiwan) and IMU (MPU-6050, GY-521, InvenSense Inc., Taiwan) linked to SPI transfer board to transfer data to processing board. Processing board transfers data into digital output using micro-USB to the computer.
Development of software
During initial design, the core functionality of the SHED software was to monitor device connectivity, monitor IMU and force sensor data in real time, and acquire data from the device. As shown in Figure 4, the interface design is dominated by simplicity and focuses on providing appropriate information. In particular, the data acquisition frequency was 100 Hz, and the IMU and force sensors were calibrated. The data were processed through the software, and the unit of each axis of the accelerometers is “G,” while that of each axis of the gyroscope is “degree/second.”
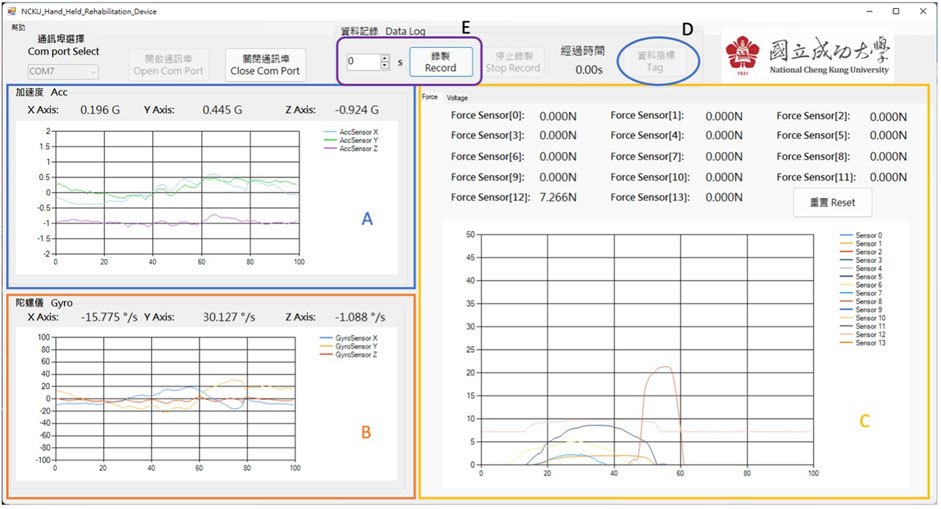
FIGURE 4. Software of SEHD: offers real-time monitoring during experiments. (A) Acceleration data; (B) angular velocity; (C) force sensor data: the reset button is for calibration; (D) “tag” button used as cues for different movement segments; (E) “record” button to preset the duration of each movement.
In addition, we had added some function buttons to improve user experience for the researchers. The a “calibration” button was used to zero the pressure from the elastic cover to the force sensors. A “Tag” button was designed for researchers to provide voice prompts to the participants during the experiment while also providing a “Tag” time point in the final output data. The adjustable duration could offer a fixed time for the researcher to conduct the tasks. After pressing the “record” button, the participants started performing the designed task. After the task was completed according to the preset duration, a pop-up window prompted that the data had been recorded and the storage path of the data.
Methods
Participants
Three groups of participants were recruited for this study: healthy young adults, healthy elderly, and stroke survivors. Fifteen participants (six healthy young adults, six healthy elderly, and three stroke survivors) were recruited based on the calculation of the sample size.
The calculation of the sample size was based on the textbook “Designing Clinical Research” by Hulley (2007). The ɑ of the two-tailed test was set as 0.05, β was set as 0.20, and r = 0.6 or 0.7. The results of the sample size would be N = 19 when r = 0.6 and N = 13 when r = 0.7. Therefore, 15 participants were recruited.
The inclusion criteria for healthy young adults were
1) aged between 20 and 30 years;
2) having no neurological or musculoskeletal disorders;
3) having had no UE surgery within past 6 months.
The inclusion criteria for the healthy elderly were
1) aged above 65 years;
2) having no neurological or musculoskeletal disorders;
3) having had no UE surgery within past 6 months;
4) being able to understand and follow instructions.
The inclusion criteria for the participants who had survived a stroke in the chronic stage were
1) survived more than 6 months after a stroke;
2) experienced only one stroke;
3) having no other neurological or musculoskeletal disorders;
4) having had no UE surgery within past 6 months;
5) capable of performing the Purdue Pegboard Test;
6) having no other cognitive impairment and being able to follow verbal instructions.
All participants were fully informed about the study and had signed the IRB-approved consent form of the National Cheng Kung University, Taiwan.
Procedure of study
The procedure was separated into two parts: 1) clinical traditional evaluation methods and 2) novel SEHD simple tasks. The traditional evaluation tools included 1) the Jamar dynamometer to evaluate maximum grip strength and 2) the Purdue Pegboard Test (PPT) to assess fine movement and hand dexterity.
The background information on each participant was collected before the traditional evaluation. This background information included name, gender, date of birth, and upper extremity dominance side. Three groups of participants were included in this study to increase the diversity of the users.
The Purdue Pegboard Test (PPT) was conducted according to the guidelines of the clinical tutorial. The PPT is a common clinical evaluation tool for UE and hand movement. The participants were asked to start the PPT assessment from the dominant side, the nondominant side, and with both hands and to do the assembly task. The first three parts of the PPT made the participants place pegs in the holes from the top to bottom. The researcher would offer verbal cues like “Start” and “Time is up” to the participants. Thirty seconds were given to the participants, and the researcher would count how many pegs had been placed within the 30-s period. The assembly task required the participants to assemble a set of pieces, which included a peg, washers, and a nut. The participants were asked to assemble the set by alternating their hands and placing the set from the top to bottom. The given time was 1 minute, and the researcher would count how many pieces had been placed and assembled correctly within this period. Three trials were given for each task. The participants could ask for rest during the PPT.
After the PPT assessments had been completed, the participants were asked to use the Jamar dynamometer to assess their grip-force, which is considered a clinical gold standard test for assessing grip-force. Three trials were collected for each participant with breaks of 1 minute between the trials. The purpose of the 1-min break was to avoid fatigue that might affect the results of the trials. The participants were asked to sit comfortably on a chair and hold the Jamar dynamometer in an upright position. When the researcher says “grasp,” the participants had to grasp as hard as they could until the researcher says “rest.”
Based on the task activities using the Jamar dynamometer and PPT that have been described above, we divided each activity and its characteristics into three task groups: forearm rotation, maximum grip strength, and sequential press (see Table 1).
All movement data were collected while the participants were sitting and holding the SEHD with their dominant hand. We verbally instructed and offered demo movement before collecting the data. There was no verbal instruction given during the experiments, but we provided sound cues for participants to understand and perform the related movements. The reasons for using sound cues (beep) during the experiment are that 1) sound cues are shorter and quicker for the participants to respond to, especially since the movement duration is relatively short; 2) it avoids additional cognitive burden, since sound cues are more intuitive than verbal cues that need more processing; and 3) it avoids any opportunity for researchers’ mistakes when offering the wrong verbal cues. The participants were asked to place their fingers at the desired places. Five trials were conducted for each task, and the average was calculated in post-processing as the performance ability of the participants.
In the first movement task—the forearm rotation task—the participants were asked to sit comfortably in front of a desk such that their forearms could be placed on the desk at the resting position. The participants were asked to hold the SEHD restfully with their fingers placed on the red TPE area before the experiment started. They were asked to respond by raising the SEHD to an appropriate height when they heard the first sound cue (beep). When the second sound cue occurred, they had to respond by performing pronation, mimicking the action of pouring water from a cup parallel to the table. When the third sound cue occurred, they had to respond by performing supination back to the original position and wait for the last cue to complete the trial. After the trial, the participants were asked to place the SEHD back on the desk and wait for the next trial or the next task. The collection duration of each trial was 15 s. The participants could ask for a resting period between the trials.
In the second task—the maximum grip strength task—the participants were asked to sit restfully in front of the desk. The participants were asked to hold the SEHD restfully with their fingers placed on the red TPE area before the experiment started. The participants were asked to apply maximum grip when they heard the first beep and relax when they heard the second beep. Because the force application of the grip is a fast and quick movement that usually lasts less than 3 s (Kamimura and Ikuta, 2001), the duration of this task was designed to be 10 s and the duration of the compressions was to be less than 4 s to avoid additional fatigue. The participants were asked to take at least 1 minute of rest between each trial.
In the third task—the sequential pressing task—the participants were asked to perform sequential independent finger compressions from the index finger to little finger and from the little finger to index finger. A complete “sequential press trial” consisted of recording eight finger presses. A metronome offering 75 beats-per-minute (bpm) (0.8 s/cue) indicated that when the participants heard the first beep, they could start performing the task. They were asked to follow 75 bpm for each digit pressing and releasing (pressing for 0.8 s and releasing for 0.8 s). Once the full round of the sequential pressing trial (the index finger to little finger and the little finger to index finger) was completed, the participants were asked to hold their positions until the last sound cue occurred. The duration of each trial was 20 s.
The participants who had survived a stroke were only asked to collect three trials for the first two tasks, while the other participants were required to collect five trials for all three tasks. Fewer trials were collected due to the lower physical abilities of the participants who had suffered a stroke. The tasks were performed on both sides (affected and less affected) in order to gain a better understanding of the SEHD performance and TETs. The stroke survivors were not asked to perform the third task due to a lack of control of each digit and the poor understanding of the task.
Data collection and statistical analysis
The designed software collected task performance data in real time, and the default collection frequency was 100 Hz. After the collection process, the system automatically transferred the data to a text file for further processing.
The results of the first task (forearm rotation) were processed in order to identify the “movement phase” and “holding phase.” The “movement phase” is how we calculate the movement’s smoothness. First, we had to identify the duration of the movement such that we could further calculate movement smoothness. There are several methods to calculate the moving phase from the data of the IMU, and we used one of the common ways to calculate the movement by finding the peak of acceleration and then 5% of the acceleration as the initiation of the action. Similarly, to find the termination of the movement. The time between the initiation and termination of the movement was considered the duration of the movement. Movement smoothness was calculated during the movement phase so as to understand the quality of the movement. Log dimensionless jerk (LDLJ) (Eq. (1)) was applied to represent movement smoothness (Gulde and Hermsdörfer, 2018; Melendez-Calderon et al., 2021). The results of the parameters were analyzed using the Pearson correlation coefficient with the Purdue Pegboard Test in order to understand the correlation between the SEHD and traditional evaluation tools.
Log dimensionless jerk (angular velocity)
Pearson’s correlation coefficient was applied to compare the maximum grip strength of the device and results of the Jamar dynamometer.
The process of the sequential press was to calculate the percentage of total digit force application to the designed digit force application during the digit-pressing interval (Eq. 2). The results of the parameters were compared with the Purdue Pegboard Test so as to understand the correlation between the novel device and traditional tools by using Pearson’s correlation coefficient.
Finger independence
All data were processed using MATLAB software (MathWorks, Natick, Massachusetts, United States ) and analyzed by SPSS (SPSS Inc., Chicago, Illinois, United States ).
Results
Participants’ demographic information and traditional evaluation tools
There were three groups that participated in the study. The demographic information is listed in Table 2. The selection of the groups and the number of groups have been explained in the abovementioned Methods section. All the participants were right-side dominant.
The results from the traditional evaluation tools showed that stroke survivors performed the worst among the three groups across all assessments. Healthy young adults performed slightly better than healthy older adults on all outcomes assessed (Table 3).
All the stroke survivors had ischemic strokes on the left side of the brain. All of them had had a stroke during the past 6 months (time since stroke = 9.3 ± 3.5 years). The average Fugl-Meyer motor score for the affected side was 28.3, while the average Fugl-Meyer motor score for the less affected side was 36.3.
Forearm rotation (pronation and supination)
There were two movement phases in this task: wrist pronation and supination. After applying the Pearson’s correlation coefficient analysis, there was a medium negative correlation between the duration of supination with the dominant-side hand task in the PPT [r (Yeudall et al., 1986) = −0.493, p < 0.05], both-hands task in the PPT [r (Yeudall et al., 1986) = −0.469, p < 0.05], and the assembly task in the PPT [r (Yeudall et al., 1986) = −0.488, p < 0.05] (Table 4). There was a moderate-to-high negative correlation between the movement smoothness of pronation and the dominant-side hand task in the PPT [r (Yeudall et al., 1986) = −0.724, p < 0.01], medium-to-negative correlation between the smoothness of pronation with both-hands task in the PPT [r (Yeudall et al., 1986) = −0.479, p < 0.05], and the assembly task in the PPT [r (Yeudall et al., 1986) = −0.535, p < 0.05].
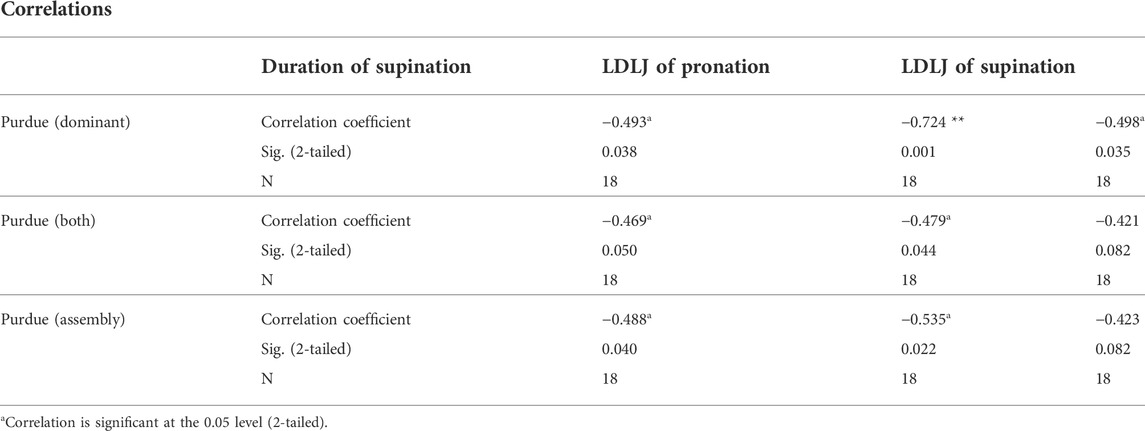
TABLE 4. Correlation between the Purdue Pegboard Test and SEHD data during forearm rotation movement task.
Maximum grip
The purpose of this movement was to understand whether to use affordable sensors to measure maximum gripping force. After the Pearson’s correlation coefficient analysis, there was a highly positive correlation between the maximum grip force and Jamar dynamometer [r (Yeudall et al., 1986) = 0.821, p < 0.01] (Table 5).
Sequential press
According to the designed task, the finger pressuring force could represent the independence of each digit if the participant could complete the task in sequence. After the Pearson’s correlation coefficient analysis of the finger independence, there was a moderate-to-negative correlation between the middle-finger independence and the right-hand subtask of the PPT [r (Yoshida et al., 2019) = −0.615, p < 0.05], medium-to-negative correlation between the middle-finger independence, and both-hand task of the PPT [r (Yoshida et al., 2019) = −0.565, p < 0.05] (Table 6).
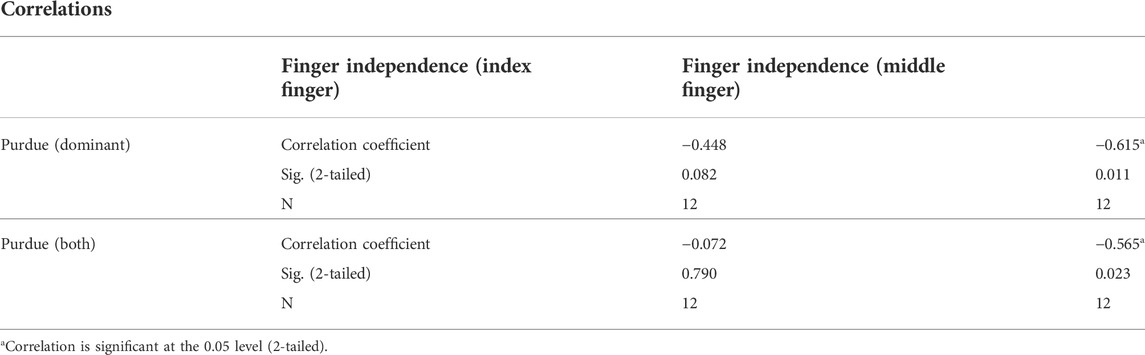
TABLE 6. Correlation between Purdue Pegboard Test and data from SEHD during sequential pressing task.
Discussion
Purdue Pegboard Test and device-based processed data
The Purdue Pegboard Test is a standardized and widely used evaluation tool of hand dexterity and upper extremity functions. The movement of the Purdue Pegboard Test could be segmented into the following phases: short lifting, the transition of the objects, picking up the object, and placing the object at the desired spot. If the designed tasks are based on these segmented movements, using the SEHD could offer proper correlations with the Purdue Pegboard Test and could be considered a proper reference for clinicians to monitor one’s upper extremity functions and/or hand dexterity.
The results showed a medium-to-moderate negative correlation between supination duration and moving forward the tasks. The duration of a movement is one of the indicators of movement ability; the shorter the movement duration, the better the movement ability. According to the calculation of movement smoothness (Balasubramanian et al., 2011), a larger LDLJ meant worse performance of movement smoothness. The results of the studies showed a moderate-to-negative correlation between the pronation and supination of the wrist movement and the traditional Purdue Pegboard Tests of the dominant side, both-hand side, and assembly.
However, the results do not appear to offer a high correlation, which might be due to the following reasons: (A) although there were three groups of participants, most of them were healthy individuals despite differences in age. In addition, the movements were designed according to activities of daily living, which means that these tasks were easy to perform for individuals without any upper extremity disability; (B) the Purdue Pegboard Test results were rendered, which meant that the results of the PPT could not offer details on each segment’s upper extremity abilities. Therefore, each individual might apply different strategies during the test, and the same individual might even apply different strategies in each object-picking process; (C) there were ceiling effects and learning effects in healthy individuals conducting the Purdue Pegboard Test (Tseng et al., 2017); (D) movement smoothness (LDLJ) was calculated using the period of the movement unit and the acceleration change during the movement unit. Therefore, LDLJ and the Purdue Pegboard Test might not correlate highly with each other.
Overall, correlation is one of the important indicators for measuring the quality of mobility movements. Although the results of the SEHD may not correlate well with the Purdue Pegboard Test, it can still be considered a useful monitoring device for assessing hand and upper extremity abilities.
Another task related to the Purdue Pegboard Test is using the SEHD for the sequential pressing tasks. Since finger independence is one of the indicators of hand dexterity, the correlation between calculated finger independence and the Purdue Pegboard Test might offer relative references in understanding one’s dexterity.
The results show that the middle finger offered a better correlation coefficient with the Purdue Pegboard Test. This may be due to the different roles of the index finger and middle finger. The index finger is the primary guide when performing precise movements, while the middle finger is more supportive and adjustive in the modification of the movement. Finger independence is presented as percentage, with a higher percentage representing poorer finger independence. Therefore, the negative correlations between the Purdue Pegboard Test and percentage of middle finger independence are consistent with our observations.
Jamar dynamometer and device-based processed data
The correlation results between the Jamar dynamometer and the data obtained from the SEHD during the maximum grip task are the most important results that can be derived from this study. This suggests that the application of affordable force sensors on this device can provide a reference for monitoring one’s power gripping ability and that this device can be used for the rehabilitation and monitoring of people with hand disabilities. Furthermore, the result also offers the reasonable belief that applying affordable force sensors to the device could provide references in monitoring one’s power gripping ability. Therefore, it is possible to use this device in the rehabilitation and monitoring of people with hand disabilities.
Novelty of sensor-embedded holding device
The idea of creating a holding device was to provide a possible solution for the clinical issues in evaluating the hand and UE functions. These issues included 1) undigitized data (Marvin, 2012; Chesworth et al., 2014; Kim et al., 2016), 2) subjective data collection (Marvin, 2012), 3) removal of additional human labor (Bohannon et al., 2006; Sigirtmac and Oksuz, 2022), 4) complicated newer technologies for most of the users (Yusif et al., 2016), and 5) newer devices that were not focused on the force (Tognetti et al., 2006; Lin et al., 2018).
The development of the SEHD was to solve the above problems. The data collected by the SEHD were digitized and could be applied as useful clinical relevant parameters after processing. The shape, appearance, and weight of the SEHD were designed to provide a more intuitive user experience, remove technical barriers for users, and shorten the learning time for users to operate the device. Force sensors that were used in the SEHD could collect appropriate data, and the integration of the IMU and force sensors could provide more parameters related to UE abilities that most of the existing devices could not achieve.
Limitations and future research
The sample size of the study was small, yet the primary purpose of this study was to introduce a novel monitoring device and demonstrate its operability. Studies with larger sample sizes will be considered in future planning. Another limitation would be the choice of the traditional evaluation tools, as such tools might not be able to correlate comprehensively with the SEHD. The concept of the movement tasks for the SEHD was to mimic the partial movement segments of the Purdue Pegboard Test. The PPT is a much complicated movement test, and the score of the PPT is a rendered result that includes UE gross movement, hand dexterity, hand–eye coordination, and control of placement. Therefore, applying other UE movement–related evaluation tools or designing other complex movements for the SEHD might be required.
There are several studies that reveal that grasp is highly related to cognitive rehabilitation (Morganti, 2004; van Vliet et al., 2013) that could be applied to other groups for rehabilitation or training purposes, such as for stroke survivors in order to improve their external focus (Durham et al., 2014) and for people with mild cognitive impairments (Cosgrove, 2016).
Therefore, future research may focus on the following directions: (A) continue to develop the SEHD as a rehabilitation intervention for different populations, such as for people who have suffered stroke, people with mild cognitive impairment, people with carpal tunnel syndrome, and examining the outcomes after an intervention; (B) continue to improve the SEHD’s capabilities, such as changing the wired SEHD to a wireless one or changing the sensors to ones with better resolutions for researchers; (C) integrate the SEHD and gamification software to provide a better user experience in different monitoring and rehabilitation settings, such as in clinics, in institutes, or when performing home activities (Lin et al., 2021). With the programmed interface, users can operate the SEHD alone with minimum assistance, which could benefit the users and caregivers.
Conclusion
The purpose of the study was to develop a novel digitized assessment device that could acquire digitized data and process functional parameters from the designed movements in order to present upper extremity functional abilities, such as grip-force and movement abilities. By comparing the processed data with that of other traditional clinical tools, the quantitative functional outcome measurements suggest that the SEHD system can be used as a monitoring tool for users in need. The integrated hardware and software in the SEHD offer proper usability and feasibility for people who need UE rehabilitation, allowing them to understand their upper extremity ability at home or in hospitals.
Based on this research, SEHD processed data could be considered as references for users in understanding their UE functional abilities.
Data availability statement
The original contributions presented in the study are included in the article/Supplementary Material; further inquiries can be directed to the corresponding author.
Ethics statement
The studies involving human participants were reviewed and approved by the Institutional Review Board at the National Cheng Kung University Hospital. The patients/participants provided their written informed consent to participate in this study.
Author contributions
CM conducted this research and wrote the manuscript. FS instructed the study and offered valuable suggestions on the design of the device and study design. PM helped with the data processing and provided recommendations in discussions. Finally, HH provided ideas for study design and gave professional reviews on the clinical prospects. All authors contributed to manuscript revision, and read and approved the submitted version.
Funding
Grant support was provided by the Ministry of Science and Technology (Taiwan). The grant number is MOST 107-2221-E-006-233-MY3.
Acknowledgments
This research was conducted under the guidance of Hsiao-Feng Chieh, Chien-Ju Lin, and other members of the Motion Analysis Laboratory at the National Cheng Kung University. Clinical suggestions were provided by Li-Chieh Kuo. Technical support was provided by DASITEK Co., Taiwan.
Conflict of interest
The authors declare that this research was conducted in the absence of any commercial or financial relationships that could be construed as a potential conflict of interest.
Publisher’s note
All claims expressed in this article are solely those of the authors and do not necessarily represent those of their affiliated organizations, or those of the publisher, editors, and reviewers. Any product that may be evaluated in this article, or claim that may be made by its manufacturer, is not guaranteed or endorsed by the publisher.
Supplementary material
The Supplementary Material for this article can be found online at: https://www.frontiersin.org/articles/10.3389/fbioe.2022.976242/full#supplementary-material
References
Ares, F. A., Presso, M., and Aciti, C. (2022). “Prototype of a biomechanical MIDI controller for use in virtual synthesizers,” in Argentine Congress of Computer Science (Springer).
Balasubramanian, S., Melendez-Calderon, A., and Burdet, E. (2011). A robust and sensitive metric for quantifying movement smoothness. IEEE Trans. Biomed. Eng. 59 (8), 2126–2136. doi:10.1109/tbme.2011.2179545
Bohannon, R. W., Peolsson, A., Massy-Westropp, N., Desrosiers, J., and Bear-Lehman, J. (2006). Reference values for adult grip strength measured with a jamar dynamometer: A descriptive meta-analysis. Physiotherapy 92 (1), 11–15. doi:10.1016/j.physio.2005.05.003
Caeiro-Rodríguez, M., Otero-González, I., Mikic-Fonte, F. A., and Llamas-Nistal, M. (2021). A systematic review of commercial smart gloves: Current status and applications. Sensors 21 (8), 2667. doi:10.3390/s21082667
Cai M., Kitani K. M., and Sato Y. (2016). Understanding hand-object manipulation with grasp types and object attributes In Robotics: Science and Systems 3.
Cano-De-La-Cuerda, R., Molero-Sánchez, A., Carratalá-Tejada, M., Alguacil-Diego, I., Molina-Rueda, F., Miangolarra-Page, J., et al. (2015). Theories and control models and motor learning: Clinical applications in neurorehabilitation. Neurol. Engl. Ed. 30 (1), 32–41. doi:10.1016/j.nrleng.2011.12.012
Castiello, U. (2005). The neuroscience of grasping. Nat. Rev. Neurosci. 6 (9), 726–736. doi:10.1038/nrn1744
Chen, P-T., Hsu, H-Y., Su, Y-H., Lin, C-J., Chieh, H-F., Kuo, L-C., et al. (2022). Force control strategy of five-digit precision grasping with aligned and unaligned configurations. Hum. Factors, 00187208211040914. doi:10.1177/00187208211040914
Chesworth, B. M., Hamilton, C. B., Walton, D. M., Benoit, M., Blake, T. A., Bredy, H., et al. (2014). Reliability and validity of two versions of the upper extremity functional index. Physiother. Can. 66 (3), 243–253. doi:10.3138/ptc.2013-45
Chiu, H. Y., Hsu, H. Y., Kuo, L. C., Chang, J. H., and Su, F. C. (2009). Functional sensibility assessment. Part I: Develop a reliable apparatus to assess momentary pinch force control. J. Orthop. Res. 27 (8), 1116–1121. doi:10.1002/jor.20859
Chodock, E., Hahn, J., Setlock, C. A., and Lipps, D. B. (2020). Identifying predictors of upper extremity muscle elasticity with healthy aging. J. biomechanics 103, 109687. doi:10.1016/j.jbiomech.2020.109687
Cosgrove, J. (2016). Investigating reach and grasp in Parkinson's disease cognitive impairment. University of Hull and the University of York.
Crowe, C. S., Massenburg, B. B., Morrison, S. D., Chang, J., Friedrich, J. B., Abady, G. G., et al. (2020). Global trends of hand and wrist trauma: A systematic analysis of fracture and digit amputation using the global burden of disease 2017 study. Inj. Prev. 26 (2), i115–i124. doi:10.1136/injuryprev-2019-043495
Domalain, M., Vigouroux, L., Danion, F., Sevrez, V., and Berton, E. (2008). Effect of object width on precision grip force and finger posture. Ergonomics 51 (9), 1441–1453. doi:10.1080/00140130802130225
Dowrick, A. S., Gabbe, B. J., Williamson, O. D., and Cameron, P. A. (2005). Outcome instruments for the assessment of the upper extremity following trauma: A review. Injury 36 (4), 468–476. doi:10.1016/j.injury.2004.06.014
Durham, K., Sackley, C., Wright, C., Wing, A., Edwards, M., and Van Vliet, P. (2014). Attentional focus of feedback for improving performance of reach-to-grasp after stroke: A randomised crossover study. Physiotherapy 100 (2), 108–115. doi:10.1016/j.physio.2013.03.004
Fujiwara, E., dos Santos, M. F. M., and Suzuki, C. K. (2014). Flexible optical fiber bending transducer for application in glove-based sensors. IEEE Sens. J. 14 (10), 3631–3636. doi:10.1109/jsen.2014.2330998
Golant, S. M. (2017). A theoretical model to explain the smart technology adoption behaviors of elder consumers (Elderadopt). J. Aging Stud. 42, 56–73. doi:10.1016/j.jaging.2017.07.003
Guerra, R., Fonseca, I., Pichel, F., Restivo, M., and Amaral, T. (2014). Hand length as an alternative measurement of height. Eur. J. Clin. Nutr. 68 (2), 229–233. doi:10.1038/ejcn.2013.220
Gulde, P., and Hermsdörfer, J. (2018). Smoothness metrics in complex movement tasks. Front. Neurol. 9, 615. doi:10.3389/fneur.2018.00615
Halverson, H. M. (1937). Studies of the grasping responses of early infancy: I. Pedagogical Seminary J. Genet. Psychol. 51 (2), 371–392. doi:10.1080/08856559.1937.10532507
Harih, G., and Dolšak, B. (2014). Comparison of subjective comfort ratings between anatomically shaped and cylindrical handles. Appl. Ergon. 45 (4), 943–954. doi:10.1016/j.apergo.2013.11.011
Harwin, W., and Barrow, A. (2013). Multi-finger grasps in a dynamic environment. Springer, 5–30. Multi-finger Haptic Interaction.
Hsiao, P-C., Yang, S-Y., Lin, B-S., Lee, I-J., and Chou, W. (2015). “Data glove embedded with 9-axis IMU and force sensing sensors for evaluation of hand function,” in 2015 37th annual international conference of the IEEE Engineering in Medicine and Biology Society (EMBC), Milan, Italy, 25-29 August 2015 (IEEE). doi:10.1109/EMBC.2015.7319426
Hsu, C-H., Lin, Y-C., Hsu, H-Y., Chieh, H-F., Lin, C-J., Ling, S-F., et al. (2021). A novel and clinically feasible instrument for quantifying upper Limb muscle tone and motor function via indirect measure methods. IEEE J. Transl. Eng. Health Med. 10, 1–8. doi:10.1109/jtehm.2021.3136754
Hsu, H-Y., Kuan, T-S., Tsai, C-L., Wu, P-T., Kuo, Y-L., Su, F-C., et al. (2021). Effect of a novel perturbation-based pinch task training on sensorimotor performance of upper extremity for patients with chronic stroke: A pilot randomized controlled trial. Archives Phys. Med. Rehabilitation 102 (5), 811–818. doi:10.1016/j.apmr.2020.11.004
Huisstede, B. M., Bierma-Zeinstra, S. M., Koes, B. W., and Verhaar, J. A. (2006). Incidence and prevalence of upper-extremity musculoskeletal disorders. A systematic appraisal of the literature. BMC Musculoskelet. Disord. 7 (1), 7. doi:10.1186/1471-2474-7-7
Kamimura, T., and Ikuta, Y. (2001). Evaluation of grip strength with a sustained maximal isometric contraction for 6 and 10 seconds. J. Rehabilitation Med. 33 (5), 225–229. doi:10.1080/165019701750419626
Kanti Majumdar, K. (2014). Relationship of activity of daily living with quality of life. British Medical Bulletin, 757–764. BBB[2][4][2014].
Karnik, A., Adke, D., and Sathe, P. (2020). “Low-cost compact theft-detection system using MPU-6050 and blynk IoT platform,” in 2020 IEEE Bombay Section Signature Conference (IBSSC), Mumbai, India, 04-06 December 2020 (IEEE). doi:10.1109/IBSSC51096.2020.9332214
Kim, J-N., Ryu, M-H., Choi, H-R., Yang, Y-S., and Kim, T-K. (2013). Development and functional evaluation of an upper extremity rehabilitation system based on inertial sensors and virtual reality. Int. J. Distributed Sens. Netw. 9 (8), 168078. doi:10.1155/2013/168078
Kim, W-S., Cho, S., Baek, D., Bang, H., and Paik, N-J. (2016). Upper extremity functional evaluation by Fugl-Meyer assessment scoring using depth-sensing camera in hemiplegic stroke patients. PloS one 11 (7), e0158640. doi:10.1371/journal.pone.0158640
Koester, D., Schack, T., and Westerholz, J. (2016). Neurophysiology of grasping actions: Evidence from ERPs. Front. Psychol. 7, 1996. doi:10.3389/fpsyg.2016.01996
Lin, B-S., Hsiao, P-C., Yang, S-Y., Su, C-S., and Lee, I-J. (2017). Data glove system embedded with inertial measurement units for hand function evaluation in stroke patients. IEEE Trans. Neural Syst. Rehabil. Eng. 25 (11), 2204–2213. doi:10.1109/tnsre.2017.2720727
Lin, B-S., Lee, I-J., Yang, S-Y., Lo, Y-C., Lee, J., and Chen, J-L. (2018). Design of an inertial-sensor-based data glove for hand function evaluation. Sensors 18 (5), 1545. doi:10.3390/s18051545
Lin, C-C., Lin, Y-C., Hsu, H-Y., Lin, Y-S., Su, F-C., and Kuo, L-C. (2021). “Home-based tele-rehabilitation system provides task-oriented training courses based on the stages of stroke,” in 2021 IEEE Region 10 Symposium (TENSYMP), Jeju, Korea, 23-25 August 2021 (IEEE). doi:10.1109/TENSYMP52854.2021.9550884
Marvin, K. (2012). Purdue pegboard test (PPT). Dostupno na. Available at: https://strokengineca/en/assessments/purdue-pegboard-test-ppt.
Mathiowetz, V. (2002). Comparison of Rolyan and Jamar dynamometers for measuring grip strength. Occup. Ther. Int. 9 (3), 201–209. doi:10.1002/oti.165
Melendez-Calderon, A., Shirota, C., and Balasubramanian, S. (2021). Estimating movement smoothness from inertial measurement units. Front. Bioeng. Biotechnol. 8, 558771. doi:10.3389/fbioe.2020.558771
Morganti, F. (2004). Virtual interaction in cognitive neuropsychology. Cybertherapy: Ios Press, 55–70.
Nation, U. (2019). World population ageing 2019: Key messages. United Nation: World Population Ageing, 1–2.
Ngui, M-H., and Lee, W-K. (2019). “Low power wearable device with GPS and indoor positioning system,” in 2019 International Conference on Green and Human Information Technology (ICGHIT), Kuala Lumpur, Malaysia, 5-17 January 2019 (IEEE). doi:10.1109/ICGHIT.2019.00037
Nikkhoo, M., Niu, C-C., Fu, C-J., Lu, M-L., Chen, W-C., Lin, Y-H., et al. (2021). Reliability and validity of a mobile device for assessing head control ability. J. Med. Biol. Eng. 41 (1), 45–52. doi:10.1007/s40846-020-00577-w
Onder, G., Penninx, B. W., Lapuerta, P., Fried, L. P., Ostir, G. V., Guralnik, J. M., et al. (2002). Change in physical performance over time in older women: The women's health and aging study. Journals Gerontology Ser. A Biol. Sci. Med. Sci. 57 (5), M289–M293. doi:10.1093/gerona/57.5.m289
Quinn, L., Busse, M., and Dal Bello-Haas, V. (2013). Management of upper extremity dysfunction in people with Parkinson disease and huntington disease: Facilitating outcomes across the disease lifespan. J. Hand Ther. 26 (2), 148–155. doi:10.1016/j.jht.2012.11.001
Reissner, L., Fischer, G., List, R., Giovanoli, P., and Calcagni, M. (2019). Assessment of hand function during activities of daily living using motion tracking cameras: A systematic review. Proc. Inst. Mech. Eng. H. 233 (8), 764–783. doi:10.1177/0954411919851302
Sani, O. G., and Shanechi, M. M. (2021). Motor Control: Sensory feedback can give rise to neural rotations. Elife 10, e75469. doi:10.7554/elife.75469
Shi, Y. X., Tian, J. H., Yang, K. H., and Zhao, Y. (2011). Modified constraint-induced movement therapy versus traditional rehabilitation in patients with upper-extremity dysfunction after stroke: A systematic review and meta-analysis. Archives Phys. Med. rehabilitation 92 (6), 972–982. doi:10.1016/j.apmr.2010.12.036
Shin, J-H., Kim, M-Y., Lee, J-Y., Jeon, Y-J., Kim, S., Lee, S., et al. (2016). Effects of virtual reality-based rehabilitation on distal upper extremity function and health-related quality of life: A single-blinded, randomized controlled trial. J. Neuroeng. Rehabil. 13 (1), 17–10. doi:10.1186/s12984-016-0125-x
Sigirtmac, I. C., and Oksuz, C. (2022). Determination of the optimal cutoff values and validity of the Purdue Pegboard Test. Br. J. Occup. Ther. 85 (1), 62–67. doi:10.1177/03080226211008046
Tarchanidis, K. N., and Lygouras, J. N. (2003). Data glove with a force sensor. IEEE Trans. Instrum. Meas. 52 (3), 984–989. doi:10.1109/tim.2003.809484
Tognetti, A., Carbonaro, N., Zupone, G., and De Rossi, D. (2006). “Characterization of a novel data glove based on textile integrated sensors,” in 2006 International Conference of the IEEE Engineering in Medicine and Biology Society, New York, NY, USA, 30 August 2006 - 03 September 2006 (IEEE). doi:10.1109/IEMBS.2006.260574
Tseng, Y-C., Chang, K-Y., Liu, P-L., and Chang, C-C. (2017). “Applying the purdue pegboard to evaluate precision assembly performance,” in 2017 IEEE International Conference on Industrial Engineering and Engineering Management (IEEM), Singapore, 10-13 December 2017 (IEEE). doi:10.1109/IEEM.2017.8290078
Turella, L., and Lingnau, A. (2014). Neural correlates of grasping. Front. Hum. Neurosci. 8, 686. doi:10.3389/fnhum.2014.00686
van Vliet, P., Pelton, T. A., Hollands, K. L., Carey, L., and Wing, A. M. (2013). Neuroscience findings on coordination of reaching to grasp an object: Implications for research. Neurorehabil. Neural Repair 27 (7), 622–635. doi:10.1177/1545968313483578
Vergara, M., Sancho-Bru, J. L., Gracia-Ibáñez, V., and Pérez-González, A. (2014). An introductory study of common grasps used by adults during performance of activities of daily living. J. Hand Ther. 27 (3), 225–234. doi:10.1016/j.jht.2014.04.002
Wang, Q., Chen, W., and Markopoulos, P. (2014). “Literature review on wearable systems in upper extremity rehabilitation,” in IEEE-EMBS International Conference on Biomedical and Health Informatics (BHI), Valencia, Spain, 01-04 June 2014 (IEEE). doi:10.1109/BHI.2014.6864424
Yang, Y., Fermuller, C., Li, Y., and Aloimonos, Y. (2015). “Grasp type revisited: A modern perspective on a classical feature for vision,” in Proceedings of the IEEE Conference on Computer Vision and Pattern Recognition, Boston, MA, USA, 07-12 June 2015. doi:10.1109/CVPR.2015.7298637
Yeudall, L. T., Fromm, D., Reddon, J. R., and Stefanyk, W. O. (1986). Normative data stratified by age and sex for 12 neuropsychological tests. J. Clin. Psychol. 42 (6), 918–946. doi:10.1002/1097-4679(198611)42:6<918::aid-jclp2270420617>3.0.co;2-y
Yoshida, A., Kurimoto, S., Iwatsuki, K., Saeki, M., Nishizuka, T., Nakano, T., et al. (2019). Upper extremity disability is associated with pain intensity and grip strength in women with bilateral idiopathic carpal tunnel syndrome. NeuroRehabilitation 44 (2), 199–205. doi:10.3233/nre-182589
Keywords: biomechanics, rehabilitation, aging, upper extremities, robotic
Citation: Ma CC, Mo P-C, Hsu H-Y and Su F-C (2022) A novel sensor-embedded holding device for monitoring upper extremity functions. Front. Bioeng. Biotechnol. 10:976242. doi: 10.3389/fbioe.2022.976242
Received: 23 June 2022; Accepted: 19 October 2022;
Published: 03 November 2022.
Edited by:
Kwong Ming Tse, Swinburne University of Technology, AustraliaReviewed by:
Daihua Yu, University of Pittsburgh, United StatesGhorban Taghizadeh, Iran University of Medical Sciences, Iran
Copyright © 2022 Ma, Mo, Hsu and Su. This is an open-access article distributed under the terms of the Creative Commons Attribution License (CC BY). The use, distribution or reproduction in other forums is permitted, provided the original author(s) and the copyright owner(s) are credited and that the original publication in this journal is cited, in accordance with accepted academic practice. No use, distribution or reproduction is permitted which does not comply with these terms.
*Correspondence: Fong-Chin Su, ZmNzdUBuY2t1LmVkdS50dw==