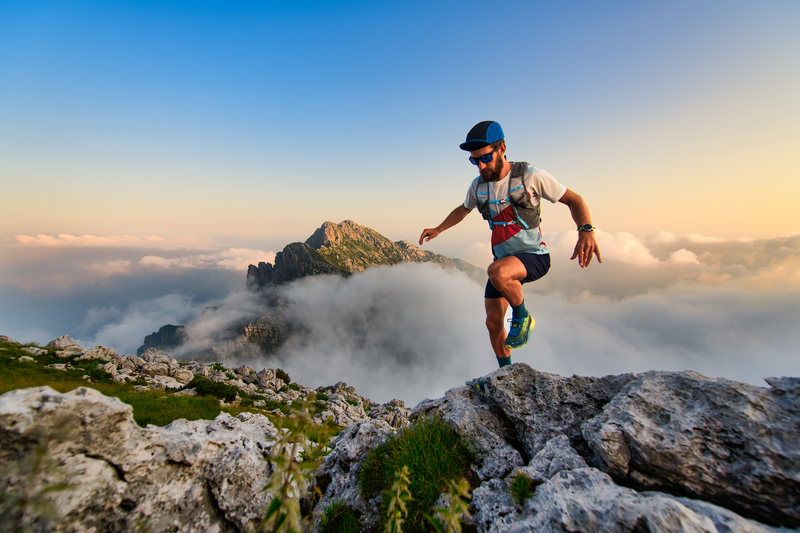
94% of researchers rate our articles as excellent or good
Learn more about the work of our research integrity team to safeguard the quality of each article we publish.
Find out more
ORIGINAL RESEARCH article
Front. Bioeng. Biotechnol. , 12 September 2022
Sec. Biomechanics
Volume 10 - 2022 | https://doi.org/10.3389/fbioe.2022.932469
This article is part of the Research Topic Cerebrospinal Fluid Dynamics and Intracranial Pressure Elevation – Novel Insights on Molecular and Physiological Mechanisms, and Implications for Neurological Disease View all 10 articles
In this paper, we used a computational model to estimate the clearance of a tracer driven by the circulation of cerebrospinal fluid (CSF) produced in the choroid plexus (CP) located within the lateral ventricles. CSF was assumed to exit the subarachnoid space (SAS) via different outflow routes such as the parasagittal dura, cribriform plate, and/or meningeal lymphatics. We also modelled a reverse case where fluid was produced within the spinal canal and absorbed in the choroid plexus in line with observations on certain iNPH patients. No directional interstitial fluid flow was assumed within the brain parenchyma. Tracers were injected into the foramen magnum. The models demonstrate that convection in the subarachnoid space yields rapid clearance from both the SAS and the brain interstitial fluid and can speed up intracranial clearance from years, as would be the case for purely diffusive transport, to days.
Cerebrospinal fluid (CSF) flow plays a fundamental role in the clearance of solutes from intracranial compartments (Abbott et al., 2018; Proulx, 2021). Current views postulate that CSF is primarily produced in the choroid plexus (Weed, 1922; Abbott et al., 2018), and flows through the ventricular system (Lindstrøm et al., 2018; Lindstrøm et al., 2019; Eide et al., 2020) and along the subarachnoid space (SAS) (Mestre et al., 2018a; Bedussi et al., 2018; Ma et al., 2019a). From there, CSF drains towards the venous system via arachnoid granulations (Vinje et al., 2020), towards lymph nodes via e.g., perineural routes across the cribriform plate (Ma et al., 2017; Ma et al., 2019a; Proulx, 2021), or the meningeal lymphatics (Louveau et al., 2017), or flows through the brain parenchyma itself via glymphatic (perivascular) pathways (Iliff et al., 2012). The relative importance of these pathways, their interplay, and role(s) in physiological as well as pathological solute transport remain unresolved (Hladky and Barrand, 2014; Louveau et al., 2017; Abbott et al., 2018; Bedussi et al., 2018; Ma et al., 2019a; Vinje et al., 2020; Proulx, 2021).
Importantly, CSF circulation characteristics change under physiological transitions, in neurological disorders, and with neurodegenerative disease. In patients diagnosed with idiopathic normal pressure hydrocephalus (iNPH), magnetic resonance imaging (MRI) reveals altered solute influx and clearance rates (Ringstad et al., 2018). In both Alzheimer’s and iNPH patients, CSF dynamics in the SAS are altered (Ringstad et al., 2018; Schubert et al., 2019), and CSF production within the choroid plexus may be reduced in iNPH (Eide et al., 2020). On the other hand, changes in glymphatic function may be associated with several types of dementia (Tarasoff-Conway et al., 2015). In Alzheimer’s disease, alterations in arterial pulsatility (Thal et al., 2008), aquaporin-4 function (Zeppenfeld et al., 2017) and sleep disturbances (Shokri-Kojori et al., 2018) have been proposed as causes of glymphatic impairment. Lastly, glymphatic transport has been reported to increase during sleep (Xie et al., 2013; Eide et al., 2021a).
A key question is to what extent the CSF circulation induced by CSF production, vascular pulsatility, and CSF efflux contributes to the transport of solutes (both influx and outflux) in the SAS and brain parenchyma. While intraparenchymal transport and glymphatics have received substantial attention over the last decade (Iliff et al., 2012; Xie et al., 2013; Hladky and Barrand, 2014; Asgari et al., 2016; Abbott et al., 2018; Mestre et al., 2018b; Smith and Verkman, 2018; Croci et al., 2019; Ray et al., 2019; Valnes et al., 2020; Eide et al., 2021a; Ray et al., 2021), the clearance interplay between different regions within the intracranial compartment is less understood. To illustrate, while Xie et al. (2013) suggest that the sleep-wake cycle regulates the efficiency of glymphatic solute clearance via changes in the interstitial space volume, the findings of Ma et al. (2019a) offer an alternative interpretation in which increased CSF outflux during wakefulness effectively limits the availability of solutes at the surface and within parenchymal perivascular spaces (PVSs). As the intracranial CSF volume is only 10%–30% of that of the brain (Kohn et al., 1991; Yamada et al., 2016), rapid clearance of substances from the SAS is crucial to sustain diffusive transport from the brain parenchyma to the SAS.
Crucially, CSF flow velocities in the SAS, including in surface PVSs, are substantial. Pulsatile CSF velocities of at least 10–40 μm/s can be inferred from experimental measurements of microsphere movement in rodents (Mestre et al., 2018a; Bedussi et al., 2018). Furthermore, the resulting dispersion effects may dominate diffusion by a factor of 104 for the transport of smaller molecules such as the MRI contrast molecule Gadoteridol (Ray et al., 2021). In humans, CSF flow in the SAS varies significantly between patients and diseases (Eide et al., 2021b), with velocities at the foramen magnum induced by pulsatile flow on the order of 5 cm/s (Bradley et al., 2016). Interestingly, CSF bulk flow at a magnitude of μm/s can be induced in the ventricular system and surface PVSs by relatively small intracranial pressure gradients (<1–2 mmHg/m) (Vinje et al., 2019).
In this study, using biophysics-based finite element computational models created from T1-and T2-weighted MR images (Ringstad et al., 2017; Ringstad et al., 2018), we study CSF flow in the ventricular system and SAS and solute transport in these CSF-filled spaces and brain parenchyma. We first simulate flow patterns and magnitude induced by a production of 0.5L CSF per day (Pardridge, 2016) in the choroid plexus and different CSF efflux pathways: across the parasagittal dura, across the cribriform plate, and into meningeal lymphatics, as well as reversed flow scenarios. We next simulate solute transport in the SAS and brain parenchyma resulting from an intrathecal injection of gadobutrol. Our findings indicate that CSF flow in the SAS is a major player in brain clearance. However, no single outflow pathway alone is able to explain in vivo observations of brain-wide distribution of tracers combined with fast clearance from the SAS, and we thus propose that a combination of different outflow routes seems more likely.
In this computational study, we quantify and characterize CSF flow patterns and molecular transport in the SAS and parenchyma induced by different clearance pathways. We also consider a choroid plexus-based production of 0.5 L/day of CSF and efflux across the 1) parasagittal dura (Ringstad and Eide, 2020), 2) the cribriform plate (Ma et al., 2017), and 3) meningeal lymphatics (Louveau et al., 2017). We consider a scenario with retrograde flow in the aqueduct (Lindstrøm et al., 2018) by assuming that 0.5 L/day CSF production occurs within the spinal cord and, as such, that there is an influx through the foramen magnum, combined with an efflux route in the choroid plexus. An illustration of a slice of the computational domain is given in Figure 1. Also, for the ease of the reader, Table 1 summarizes all physical constants that will be used in this section.
TABLE 1. Overview of parameter values used in the model. Parameters with an asterisk are considered uncertain and were set to vary between different computational models (see Table 2).
FIGURE 1. (A) A cross section of our brain mesh shows the SAS (dark blue), white matter (orange), gray matter (light blue), and ventricles (red) (B) shows a zoom in on a part of the mesh with the edges of the mesh triangles. Note that for visualization purposes, the resolution shown here is coarser than the resolution used in the numerical simulations.
We consider baseline T1-and T2-weighted MR images (resolution 1 mm) from an iNPH patient collected in a previous clinical study. This patient then underwent a (0.5 ml, 1 mmol/ml) intrathecal injection of the gadolininium-based tracer gadobutrol, and follow-up MR images were taken at several time points post injection. LookLocker images were also obtained with the T1-weighted MR images. The clinical study was approved by the Regional Committee for Medical and Health Research Ethics (REK) of Health Region South-East, Norway (2015/96), the Institutional Review Board of Oslo University Hospital (2015/1868), and the National Medicines Agency (15/04932-7), and conducted in accordance with the ethical standards of the Declaration of Helsinki of 1975 (and as revised in 1983). All study participants were included after written and oral informed consent.
The baseline MR images were post-processed using FreeSurfer v6.0 (Fischl, 2012) to obtain a segmentation of the brain. To define a choroid plexus (CP) completely enclosed by the lateral ventricles, a CP domain was manually marked in the images. Next, the left and right pial membranes, white matter interface, cerebellum, ventricles, and aqueduct were represented via triangulated surfaces. The segmentation of the SAS was performed by thresholding a registered T2-weighted image, and any clusters not connected to the FreeSurfer segmentation were removed. Subsequently, a surface bounding the SAS was constructed, and expanded by 1 mm in the surface normal direction to ensure that the SAS was represented as a continuous compartment between the pia and dura around the whole brain. The CSF volume before and after expansion was 457 and 602 ml, respectively. The spinal cord was not segmented and was represented as CSF for simplicity. The parenchymal volume was 1,266 ml. Both the CSF and parenchymal volumes are slightly above average values in iNPH patients (Yamada et al., 2016).
The generated surfaces were further post-processed using SVMTK (2021), and finally used to generate a volumetric mesh Ω of the parenchyma ΩP and surrounding CSF-spaces ΩF combined (Figure 1). We label the boundary separating ΩP and ΩF by ∂ΩP. The choroid plexus ΩCP ⊂ ΩF is located within the lateral ventricles and we denote its surface (in contact with the CSF) by ∂ΩCP. The outer boundary of the SAS is split into three parts: ∂ΩS, ∂ΩFM, and ∂Ωout, representing the arachnoid membrane, foramen magnum, and a chosen efflux route, respectively. We consider and define three different regions Ωout for efflux of CSF: locally across the parasagittal dura (Figure 2A), locally across the cribriform plate (Figure 2B), or into the meningeal lymphatics distributed over the outer (arachnoid) boundary (Figure 2C). Finally, to simulate retrograde net aquaductal flow, we consider flow into the choroid plexus (Figure 2E) from the foramen magnum (Figure 2D).
FIGURE 2. Red markers highlight important subregions and boundaries in the computational domain: the (A) parasagittal dura, (B) cribriform plate, (C) meningeal lymphatics, (D) foramen magnum, and (E) choroid plexus.
We model the flow of CSF in ΩF by the incompressible Stokes equations: find the CSF velocity field u and pressure p such that
where μ = 0.7 × 10–3 Pas (Daversin-Catty et al., 2020) is the CSF viscosity and g is a given source of fluid. With the low Reynolds numbers (0.001) reported for flow in PVS (Mestre et al., 2018a; Daversin-Catty et al., 2020), we find steady Stokes flow to be a reasonable assumption for the present study. To represent CSF production in the choroid plexus, we let g be a given positive constant in ΩCP and zero elsewhere in ΩF. Specifically, by default, we set g such that approximately 0.5L of CSF is produced every 24 h. We also consider a scenario with increased CSF production. In humans, CSF production has been reported to increase during sleep (Nilsson et al., 1992), while high CSF turnover through lymphatics has been reported in awake mice (Ma et al., 2019a). We set the parenchymal CSF/brain interstitial fluid (ISF) velocity to be zero (in ΩP).
We set the CSF velocity at the outer boundary (representing the arachnoid membrane) to be zero, except at specific efflux/absorption sites ∂Ωout to be further specified. At these, we set a traction condition:
where R0 ≥ 0 represents an efflux resistance acting to moderate CSF outflow in these regions, and n denotes the outward-pointing boundary normal. The fluid source, in combination with the zero or low resistance efflux routes, induces a flow of CSF from the CP through the ventricular system, through the SAS, and out across either the parasagittal dura, cribriform plate, or meningeal lymphatics.
We also consider a reversed flow scenario, in which g is set negative with a value corresponding to a sink of 0.5 L/day, a zero traction condition is imposed at the foramen magnum ∂ΩFM, and zero velocity (no slip) is imposed on the remainder of the boundary.
We also model molecular transport within the CSF-spaces and parenchyma resulting from an influx of gadobutrol at the foramen magnum (resulting, e.g., from an intrathecal injection). We model transport of a concentration c in the entire domain Ω via the diffusion-convection equation.
where u is a convective velocity field, D denotes an apparent diffusion coefficient, and α is a dispersion factor. We set the apparent diffusion coefficients DF = 3.8 ⋅ 10–4 mm2/s in ΩF and
To represent an influx of gadobutrol at the foramen magnum, we set
Based on tracer enhancement as reported by Eide et al. (2020), F(t) is modeled as a linearly decreasing function until T0 ≈ 2.24 h (8,064 s) and zero thereafter, i.e.,
The solute influx F(t) (given in mmol/(s mm2) is chosen such that the total amount of gadobutrol injected is approximately 0.5 mmol. At the efflux sites ∂Ωout, we let the solute be absorbed via the relation.
where β is a given membrane permeability. The case β = 0 corresponds to no absorption, β = ∞ corresponds to free movement of solutes across the boundary, while 0 < β < ∞ represents a diffusive resistance to molecular outflow. On the remainder of the boundary, we do not allow for solute efflux, by setting D∇c ⋅ n − cu ⋅ n = 0. Moreover, we let the initial concentration be c (x, 0) = 0. Note that to model transport associated with the reversed flow scenario, we let ∂ΩCP take the role of ∂Ωout.
At the interface between ΩF and ΩP we conserve mass (enforce conservation of molecules) by setting ϕDP∇cP ⋅ n = DF∇cF ⋅ n. Here, DP and DF denote D restricted to ΩP and ΩF; respectively, n is the normal vector on the interface, pointing from ΩP to ΩF and ϕ denotes the extracellular space (ECS) porosity.
CSF and solutes may have several simultaneous and possibly partially independent outflow routes (Proulx, 2021). We here consider six different flow and transport models separately (Table 2), each with different dispersion factors. This design allows us to systematically examine different pathways and evaluate whether each or combinations thereof could describe in-vivo observations of Gadobutrol transport. Model I and II describe flow induced by CSF production in the CP and CSF efflux across the parasagittal dura and cribriform plate, respectively. For these models, we assume free molecular efflux at the absorption sites. Model III is a variant of Model I with a finite molecular efflux permeability at the parasagittal dura absorption site. Model IV reflects a different efflux pathway with CSF production in the CP, CSF efflux in the meningeal lymphatics, and a finite molecular efflux permeability. Model V represents a reversed flow scenario with absorption of CSF in the CP region (and CSF influx at the foramen magnum). Model VI represents a variant of Model II with increased CSF production. CSF production has been reported to vary between subjects and different central nervous system disorders (Eide et al., 2020; Eide et al., 2021b). Furthermore, CSF production has been reported to increase by a factor of up to three during the nighttime compared to the daytime (Nilsson et al., 1992), and increased CSF efflux to the lymphatic system has been reported to limit tracer entry to the brain (Ma et al., 2019b). In this study, we set the CSF production for model VI to be 1.0 L/day (i.e., twice that of all other models).
TABLE 2. Overview of computational models. Production and absorption sites refer to the production sites for CSF and efflux/absorption sites of CSF and the solute concentration, respectively. R0 is a CSF efflux resistance parameter cf. (2), while β represents a diffusive resistance to molecular efflux cf. (6). The values for R0 and β were estimated by numerical experimentation.
The Stokes equations are solved using a finite element method with Taylor-Hood (continuous piecewise quadratic and continuous piecewise linear) elements for the velocity and pressure. The diffusion-convection equation with boundary conditions is solved numerically using the finite element method with continuous linear finite elements for the concentration in space and the backward Euler method in time; all using the FEniCS finite element software (Logg et al., 2012; Alnæs et al., 2015). The brain mesh has 6 691 432 cells and 1 088 640 vertices. The degree of freedom for the diffusion equation is equal to the number of vertices. For the Taylor-Hood case, the number of degrees of freedom is 27 858 018. Moreover, the largest cell size is 2.4 mm and the smallest is 0.07 mm. The largest cells are in the middle of the white matter where there is no stokes flow or sharp gradients.
A time resolution study was performed to ensure that our simulation results were independent of the choice of time step (Supplementary Figure S1). As mesh refinements of the entire geometry are too expensive, a mesh resolution study was performed on a partition of the mesh containing both CSF and brain tissue (Supplementary Figure S2). Refinements from a cell size comparable to the resolution in the full geometry did not change key quantities like flow velocity in the SAS or tracer concentration in the brain (Supplementary Table S2). Including testing and validation, a total of ≈30,000 CPU hours were used to run the simulations on big memory nodes. All simulations were run on the high-performance computing infrastructure Sigma2—the National Infrastructure for High Performance Computing and Data Storage in Norway.
We extract contrast agent concentration estimates from the MR images post injection for comparison with computational predictions. The contrast agent shortens the T1 times as:
where c denotes the concentration of the contrast agent, r1 is known as the T1 relaxivity of the agent, and T1(c) and T1 (0) denote the T1 time with and without concentration, respectively. The T1 times can be computed using a T1 mapping (Taylor et al., 2016), such as the LookLocker sequence. Through a preliminary phantom study, the relaxivity constant for this LookLocker protocol was found to be 6.5 L mmol−1 s−1. The median T1 time over the parenchyma was used in Eq. 7 to estimate the concentration in the parenchyma. The CSF concentration was estimated by manually creating a region of interest (ROI) in the CSF, and using the average T1 time over the ROI with Eq. 7. Finally, to transform the concentration in the parenchyma to be that of the extracellular space, the concentration was multiplied by five.
The total amount of solute in a given region Ωi (i = F, P) at time t was computed as
where Vi refers to the volume of the respective region. To compare parenchymal influx between models, we compute the peak average concentration in the parenchyma and the time to reach this peak. We also compute the relative clearance of tracers after T1 = 3 days as 1—
All models induce non-trivial CSF flow through the ventricular system and subarachnoid space.
Models I–IV all reach maximal SAS velocities of 8.9 mm/s in the thinnest part of the aqueduct (Figures 3A–C). Despite their differences in efflux pathways, all of these models predict higher CSF flow velocities in the anterior regions of the SAS compared to the posterior regions. Model II displays the highest velocities in the SAS, reaching 50 μm/s. Models I (and III) reach peak CSF velocities of 40 μm/s. In model IV, CSF flow occurs mainly in the lower regions of the SAS, as CSF can exit the SAS along the entire boundary. Peak velocities in the SAS for model IV reach 20 μm/s. In models where CSF was allowed to exit through outflow routes other than the parasagittal dura (models II and IV), CSF velocity magnitudes were relatively small (<4 μm/s) in the SAS near the upper convexities of the brain.
FIGURE 3. Sagittal views (cut through the center of the aqueduct) of CSF velocity magnitudes induced by steady CSF production in the choroid plexus combined with different CSF efflux pathway models, or a reversed flow scenario. Subfigures show velocity fields resulting from CSF efflux through (A) the parasagittal dura (B) the cribriform plate (C) the meningeal lymphatics (D) production in the foramen magnum and absorption in the choroid plexus, (E) the cribriform plate with double production. The color map is capped at 0.05 mm/s for visualization purposes.
Model V predicts that, under its assumptions, CSF will predominantly flow from the foramen magnum directly to the CP, limiting CSF flow in other parts of the SAS (Figure 3D). Therefore the flow direction is reversed compared to models I–IV. In the foramen magnum, CSF velocity magnitudes reach 20 μm/s, while the velocity in the aqueduct remains at 8.9 mm/s. In the upper regions of the SAS, not directly associated with the 3rd ventricle, CSF velocities were typically lower than 0.1 μm/s.
Doubling the CSF production (model VI versus model II) results in a doubling of the CSF velocity field by linearity. Therefore, we observe velocities of approx. 100 μm/s in the CSF space (Figure 3E) and a velocity in the aqueduct of 17.8 mm/s for model VI.
When driven purely by diffusion (without convection or dispersion enhancements), the tracer spreads radially from the foramen magnum and distributes evenly throughout the brain. The distribution is slightly faster in the CSF than in the parenchyma, as the free diffusion coefficient in the CSF is larger. However, this effect is not very noticeable. For Models I–III the relative 1 year clearance is only 32.8%, 17.6 %, and 29.9%. Model IV displays faster clearance, clearing 92.5% over 1 week but with a late peak parenchyma concentration occurring after 79 h.
Including the CSF circulation-induced flow as a convective velocity substantially speeds up the clearance rates, both from the SAS and parenchyma.
Tracer distribution is shown for all models after 6 and 24 h and α = 10 in Figure 4, revealing substantial inter-model variations. For Model I, the tracer is mainly confined to the SAS and moves upwards towards the parasagittal dura, showing a clear preference for traveling along the SAS in the right hemisphere (data not shown). As there is no molecular resistance to outflow on the parasagittal dura in Model I, the tracer is instantly transported out when moving into this efflux route. In regions where the tracer concentration in the SAS is high, the tracer also enters the brain due to the large concentration gradient between the SAS and the brain (Figures 4A,B Model I). After 1 week, some traces are still found within the brain, slowly diffusing back towards the pial surface for clearance via convection in the SAS (data not shown). Models I and III (with outflow via the parasagittal dura) are the only models where a tracer reaches the upper convexities of the brain, resulting in a brain-wide distribution of tracers. In Model III, where a diffusive molecular resistance is added at the parasagittal dura, tracer accumulates near the outflux region (Figures 4B, Model III).
FIGURE 4. The figure shows a sagittal view of all the models at 6 h (A) and 24 h (B) after intrathecal injection of gadobutrol for α =10. For the simulation data, the colorscale shown is 0.1–5 mmol/L in (A) and 0.1–1 mmol/L in (B). For comparison, the T1 contrast enhanced image for the patient at the same time is included. The MR images are scaled separately for picture legibility.
Model V is the only model where tracer reaches the ventricular system, while Model IV has a localized accumulation of tracers around the brain stem. Model VI, with increased CSF production, shows a generally lower concentration of tracers and some accumulation near the outflux route at the cribriform plate.
The average concentration over time for all models, and α = 10, is compared in Figure 5, both for the ISF and CSF. The figure also contains in vivo concentration estimates in both spaces. We observe that a combination of the different outflow routes, i.e., Models I and V, gives a comparable result to that of the MR images. Models I and III both display higher concentrations than the data in both the CSF and parenchyma/ISF (Figure 5). Model II, IV, and V, on the other hand, yield comparable or lower concentrations.
FIGURE 5. The figure shows concentration in the CSF (A) and the ISF (B) for all models over 1 week. The tracer concentration data from T1 MR images for this patient is also shown.
Models I and II both display high 3-day clearance rates for all dispersion factors (Figures 6A,B). Specifically, the 3 day clearance rates are between 94.1% and 97.7% for Model I and between 88.9% and 94.9% for Model II (Table 3). The tracer concentration is initially higher in the SAS, allowing for diffusive influx to the brain. At later time points, the SAS has been cleared, mainly via convective flow, and the tracer partly remains inside the parenchyma, delaying the total clearance of tracers from the intracranial compartment. Model I has slightly higher peak average parenchyma concentration values than Model II, reaching 0.30 and 0.24 mmol/L, respectively. The time to peak in the parenchyma occurred after 7.8–19.0 h for Model I and 10.1–16.8 h for Model II.
FIGURE 6. Average concentration in the parenchyma (par) and CSF over a period of 72 h. Models I-VI is used with dispersion values α = 1, 10, 100, 1,000. Also plotted is the concentration data taken from T1-weighted images of this specific patient as a ground truth. The tracer injection (present from 0 to 2.24 h) is seen as a sharp increase in CSF concentration at early time points. When the injection is no longer present, the total amount of tracers within the intracranial compartment starts decreasing. The tracer concentration data from T1 contrast enhanced images of the patient is also included. (A) Model I (B) Model II (C) Model III (D) Model IV (E) Model V and (F) Model VI.
TABLE 3. The table shows time to peak concentration in the parenchyma (left), total mass clearance in the intracranial compartment after 72 h (middle), and peak average concentration values in the parenchyma (right) for the case of gadobutrol transport. Values are shown for all models and α values. M, Model; α: Dispersion factor, par, parenchyma; conc, concentration; avg, average.
For the models including a molecular resistance to outflow at the outflow site (i.e., Figures 6C,D, Model III and IV), the 3 day clearance rate is comparable to Models I and II, except for the case when α = 1,000 in Model III (Table 3). The highest 3 day clearance is obtained with α = 1 for both Model III and IV (95.7 and 99.0% clearance, respectively). The lowest 3 day clearance is obtained with α = 1,000 for model III (36.3% clearance) and α = 10 for model IV (89.5% clearance, Table 3). Model III reaches a peak parenchyma concentration of 0.50 mmol/L, while Model IV has a lower peak of 0.23 mmol/L. The time to peak exceeds 19.0 h for all dispersion factors in Model III, which is much later than the other models. Model IV, on the other hand, peaks between 11.2 and 17.9 h.
Model V (with reversal of CSF flow in the aqueduct) results in low parenchymal enrichment compared to Models I–IV (Table 3, Figure 6E). The 3 day clearance rate is between 75.1% and 90.0% depending on α and the peak average concentration is 0.18 mmol/L in the parenchyma (Table 3). The time to peak concentration in the parenchyma is long for α = 1, occurring later than after 1 week, but for larger dispersion factors, the peak occurs between 10.8 and 22.4 h.
Model VI, with double the sCSF production of the other models, displayed rapid clearance from the CSF (Figure 6F). The rapid turnover of CSF limited the influx and facilitated clearance within the parenchyma. The 3 day clearance rate for all dispersion factors ranged between 95.4%–97.9% (Table 3). The peak average parenchyma concentration occurs early, between 7.8 and 11.2 h, and reaches at most 0.15 mmol/L, when α = 1,000.
In this paper we have simulated molecular transport by diffusion and convection for six different models investigating the distribution of gadobutrol molecules entering the intracranial compartment via the foramen magnum. The different models represent different outflow routes, and CSF flow patterns vary considerably between models. The effects of outflow route and dispersion factor modify the distribution and clearance patterns in a non-linear and unpredictable manner. Outflow through either the parasagittal dura, the cribriform plate, or through meningeal lymphatics, typically clears 80%–99% of injected tracers over a time period of 3 days. These three models, however, display very different spatial distributions of tracers. In Models I and III tracer are distributed more or less throughout the frontal cortex, while when outflow occurs through meningeal lymphatics, tracers are mainly located at the brain stem at the base of the brain.
With a daily production of 0.5 and 1.0 L/day in our models, the velocity reaches 10 and 17.8 mm/s in the aqueduct. Pulsatile aqueductal flow velocities of several cm/s have been measured experimentally (Lee et al., 2004; Tawfik et al., 2017; Spijkerman et al., 2019) in the range of 1–10 cm/s. Average velocities and max velocities were reported at around 5 cm/s, corresponding to a total volume flux of 0.3 ml per cycle, of which 0.01 ml was net (Lindstrøm et al., 2018; Eide et al., 2021b). As the net flow is around 1/30 of the total flux, the corresponding net max velocity can then be estimated as 5/30 cm/s, somewhat below the velocities estimated here. Further, in iNPH patients, it has been reported that phase-contrast MR has reported retrograde net flow in the aqueduct (Lindstrøm et al., 2018). Model V is motivated by retrograde aqueductal flow and we see that this model is distinct from the other models in that there is significant ventricular enrichment, as often seen in iNPH (Ringstad et al., 2018).
On the pial surface of the brain, we observe velocities of up to 20–50 μm/s in models I-IV, and up to 100 μm/s in model VI. These velocities align relatively well with experimentally observed bulk flow velocities of around 20 μm/s observed in mice (Mestre et al., 2018a; Bedussi et al., 2018). Thus, CSF flow observed in these studies may very well be a result of CSF production and absorption driven by small static pressure differences. It should be noted that mice have approximately 3x faster CSF turnover compared to humans (Pardridge, 2016). Given otherwise similar CSF dynamics between the species, one would thus expect CSF production to cause higher velocities at the surface of the mouse brain compared to the human. In comparing model II and model VI, the increased CSF efflux to the cribriform plate limits tracer influx to the brain, in line with the hypothesis of Ma et al. (2019a). We observe that models with a short distance between injection and absorption site (models II, IV, and V) limit the influx of tracers to the parenchyma. In general, tracers will enter the brain if they are present on the surface over a long period of time. For a given tracer, the amount of tracers entering the brain will thus be affected by both the CSF velocity and the distance from the injection site to the absorption site.
Gadobutrol injections have been studied in human subjects in several papers. Eide, Ringstad and colleagues have reported MR intensity increases for a large number of subjects (Ringstad et al., 2017; Ringstad et al., 2018; Eide et al., 2020; Eide et al., 2021a), while Watts et al. (2019) quantified gadobutrol concentrations over time in a single patient. These studies show an initial sharp increase in tracer concentration in the SAS, typically reaching a peak at around 2–6 h. In the parenchyma, peak values occur between 10 and 24 h, depending on the region of interest. Gray matter regions closer to the pial surface typically peak at around 10 h, while for specific white matter regions, peak values may occur closer to 24–40 h post-injection (Ringstad et al., 2018; Watts et al., 2019). In all our models, peak CSF concentration occurs at the time when the gadobutrol influx at the foramen magnum is turned off, i.e., after approximately 2 h. More interestingly, the ISF concentration peaks later, and the time to peak is between 10 and 20 h in 15 out of 24 models tested. ISF concentration is reported to decay relatively slow, with an approximate concentration at 48 h at half its peak value (Eide et al., 2021a). Furthermore, the peak concentration of gadobutrol has been measured as 0.5 mmol/L in the CSF and around 0.12 mmol/L in the ISF (Watts et al., 2019), in line with both the estimates of the concentration in the iNPH patient and the results from our models. Both Model II (outflow through the cribriform plate) and Model IV (outflow via meningeal lymphatics) match all these criteria well when the dispersion in the SAS was modeled by α = 10. Model I (outflow through the parasagittal dura) replicates experimentally observed ISF concentration without additional dispersion in the SAS, but clearance from the SAS is delayed in this model compared to experimental data. With a molecular resistance to outflow on the parasagittal dura (Model III), simulations reproduce accumulation of tracers in this region, but clearance kinetics are slower than expected. With a doubling of CSF production (Model VI), the kinetics of ISF and CSF clearance is faster than expected for all dispersion factors tested. In the model with reversed flow in the aqueduct (Model V), we qualitatively reproduce the tracer enhancement in the aqueduct as seen in iNPH patients (Eide et al., 2020). However, rapid flow through the aqueduct and into the choroid plexus prevents the expected brain-wide enhancement of tracers (Ringstad et al., 2018), and in Model V, tracers are confined to the foramen magnum or in the vicinity of the lateral ventricles. Combined, these results suggest that a combination of production and efflux sites may be needed to reproduce the observed tracer distribution (Ringstad et al., 2018; Eide et al., 2020; Eide et al., 2021a).
The role of different outflow routes from the SAS has been debated and challenged over years. In particular, the traditional view of outflow predominately through arachnoid granulations has been criticized recently (Proulx, 2021). Our Model I is conceptually similar to outflow through arachnoid granulations with CSF draining close to the dural sinus. The results from our simulations cannot exclude any of the proposed major outflow routes, as all of them resemble experimental data in at least some measure. A specific weighting between inflow and outflow routes may potentially be sufficient to explain differences between groups (e.g., iNPH vs. control) or differences between individuals. The results do show unequivocally that CSF flow and clearance are major players in CNS clearance. Convective flow in the SAS speeds up intracranial clearance from years to hours and days, an enormous effect compared to the effect of bulk flow of around 1 μm/s within the ECS (Croci et al., 2019). Furthermore, changes in the dispersion factor (increased diffusion due to mixing) only in the SAS changed both peak values and clearance rates within the brain ECS.
In terms of limitations, we only performed the simulations on a single patient. Furthermore, the patient of interest was diagnosed with iNPH, which may alter CSF dynamics (Eide et al., 2021b). Creating one patient-specific mesh with high mesh quality that includes all anatomical regions of interest was time-consuming, and increasing the amount of subjects was not the scope of this study. To resolve all regions of the SAS, the SAS was expanded by 1 mm. This modification increases the volume of which fluid flows, and thus slightly reduces the velocities we find in the SAS. The total CSF volume was increased by around 33%, we thus assume that our reported SAS flow velocities of 20–50 μm/s are lower estimates. In the SAS, we assumed that the dispersion factor was similar in all subregions. In reality, dispersion would be expected to be enhanced close to larger arteries (Ringstad et al., 2017) and in regions where pulsatile CSF flow is substantial (e.g., near the foramen magnum). Furthermore, we did not include ISF velocities in the foramen magnum. There is very little knowledge about how the velocity fields are directed (Croci et al., 2019), especially without a priori knowledge of the location of blood vessels. In addition, the purpose of this study was to assess the effect of SAS convection, independent of potential bulk flow within the brain. Finally, we should note that we assumed that all injected gadobutrol reached the foramen magnum, while around 33% of CSF has been proposed to be drained along the spinal canal (Edsbagge et al., 2004). The latter point may explain the fact that most of the reasonable models tested (Models I, II, and IV) all generally display a slight overestimation of the SAS peak concentration in our models compared to the data.
In conclusion, we have demonstrated that convection in the SAS yields rapid clearance both from the SAS and the ISF, even when pure diffusive transport is assumed in the ECS. Convective fluid flow in the SAS has the potential to speed up clearance from years (as would be the case for purely diffusive transport) to days. As none of the models tested were able to reproduce the observed data perfectly (both qualitatively and quantitatively), a combination of the different outflow routes seems most plausible, and their relative weight may differ between groups (Eide et al., 2020).
The raw data supporting the conclusion of this article will be made available by the authors without undue reservation.
The studies involving human participants were reviewed and approved by The study was approved by the Regional Committee for Medical and Health Research Ethics (REK) of Health Region South-East, Norway (2015/96), the Institutional Review Board of Oslo University Hospital (2015/1868), and the National Medicines Agency (15/04932-7), and was registered in the Oslo University Hospital Research Registry (ePhorte 2015/1868). The conduct of the study was governed by ethical standards according to the Declaration of Helsinki of 1975 (and as revised in 1983). Study participants were included after written and oral informed consent. The patients/participants provided their written informed consent to participate in this study.
MH, VV, LV, PE, GR, MR, and K-AM conceived the simulations; LV and MH segmented and meshed MR images; MH conducted the simulations; VV, MH, and LV did the analysis of the results; and MH made the figures. All authors discussed the simulations and results. MH, VV, LV, MR, and K-AM wrote the first draft. All the authors revised and approved the final manuscript.
K-AM acknowledges support from the Research Council of Norway, Grant 300305 and 301013, and the national infrastructure for computational science in Norway, Sigma2, Grant NN9279K. MR has received funding from the European Research Council (ERC) under the European Union’s Horizon 2020 research and innovation programme under Grant agreement 714892.
The authors declare that the research was conducted in the absence of any commercial or financial relationships that could be construed as a potential conflict of interest.
All claims expressed in this article are solely those of the authors and do not necessarily represent those of their affiliated organizations, or those of the publisher, the editors, and the reviewers. Any product that may be evaluated in this article, or claim that may be made by its manufacturer, is not guaranteed or endorsed by the publisher.
The Supplementary Material for this article can be found online at: https://www.frontiersin.org/articles/10.3389/fbioe.2022.932469/full#supplementary-material
CP, choroid plexus; CSF, cerebrospinal fluid; ECS, extracellular space; iNPH, idiopathic normal pressure hydrocephalus; ISF, interstitial fluid; MRI, magnetic resonance imaging; SAS, subarachnoid space; PVS, perivascular space; ROI, region of interest.
Abbotts, N. J., Pizzo, M. E., Preston, J. E., Janigro, D., and Thorne, R. G. (2018). The role of brain barriers in fluid movement in the cns: Is there a ‘glymphatic’system? Acta Neuropathol. 135, 387–407. doi:10.1007/s00401-018-1812-4
Alnæs, M., (2015). The fenics project version 1.5. Archive Numer. Softw. 3. doi:10.11588/ans.2015.100.20553
Asgari, M., De Zélicourt, D., and Kurtcuoglu, V. (2016). Glymphatic solute transport does not require bulk flow. Sci. Rep. 6, 38635–38711. doi:10.1038/srep38635
Bedussi, B., Almasian, M., de Vos, J., VanBavel, E., and Bakker, E. N. (2018). Paravascular spaces at the brain surface: Low resistance pathways for cerebrospinal fluid flow. J. Cereb. Blood Flow. Metab. 38, 719–726. doi:10.1177/0271678x17737984
Bradley, W. G., Haughton, V., and Mardal, K.-A. (2016). Cerebrospinal fluid flow in adults. Handb. Clin. Neurology 135, 591–601. doi:10.1016/B978-0-444-53485-9.00028-3
Croci, M., Vinje, V., and Rognes, M. E. (2019). Uncertainty quantification of parenchymal tracer distribution using random diffusion and convective velocity fields. Fluids Barriers CNS 16, 32–21. doi:10.1186/s12987-019-0152-7
Daversin-Catty, C., Vinje, V., Mardal, K.-A., and Rognes, M. E. (2020). The mechanisms behind perivascular fluid flow. Plos one 15, e0244442. doi:10.1371/journal.pone.0244442
Edsbagge, M., Tisell, M., Jacobsson, L., and Wikkelso, C. (2004). Spinal csf absorption in healthy individuals. Am. J. Physiology-Regulatory, Integr. Comp. Physiology 287, R1450–R1455. doi:10.1152/ajpregu.00215.2004
Eide, P. K., Valnes, L. M., Pripp, A. H., Mardal, K.-A., and Ringstad, G. (2020). Delayed clearance of cerebrospinal fluid tracer from choroid plexus in idiopathic normal pressure hydrocephalus. J. Cereb. Blood Flow. Metab. 40, 1849–1858. doi:10.1177/0271678x19874790
Eide, P. K., Vinje, V., Pripp, A. H., Mardal, K.-A., and Ringstad, G. (2021). Sleep deprivation impairs molecular clearance from the human brain. Brain 144, 863–874. doi:10.1093/brain/awaa443
Eide, P. K., Valnes, L. M., Lindstrøm, E. K., Mardal, K.-A., and Ringstad, G. (2021). Direction and magnitude of cerebrospinal fluid flow vary substantially across central nervous system diseases. Fluids Barriers CNS 18, 16–18. doi:10.1186/s12987-021-00251-6
Hladky, S. B., and Barrand, M. A. (2014). Mechanisms of fluid movement into, through and out of the brain: Evaluation of the evidence. Fluids Barriers CNS 11, 26–32. doi:10.1186/2045-8118-11-26
Iliff, J. J., Wang, M., Liao, Y., Plogg, B. A., Peng, W., Gundersen, G. A., et al. (2012). A paravascular pathway facilitates csf flow through the brain parenchyma and the clearance of interstitial solutes, including amyloid β. Sci. Transl. Med. 4, 147ra111. doi:10.1126/scitranslmed.3003748
Kohn, M. I., Tanna, N. K., Herman, G. T., Resnick, S. M., Mozley, P. D., Gur, R. E., et al. (1991). Analysis of brain and cerebrospinal fluid volumes with mr imaging. part i. methods, reliability, and validation. Radiology 178, 115–122. doi:10.1148/radiology.178.1.1984289
Lee, J. H., Lee, H. K., Kim, J. K., Kim, H. J., Park, J. K., and Choi, C. G. (2004). Csf flow quantification of the cerebral aqueduct in normal volunteers using phase contrast cine mr imaging. Korean J. Radiol. 5, 81–86. doi:10.3348/kjr.2004.5.2.81
Lindstrøm, E. K., Ringstad, G., Mardal, K.-A., and Eide, P. K. (2018). Cerebrospinal fluid volumetric net flow rate and direction in idiopathic normal pressure hydrocephalus. NeuroImage Clin. 20, 731–741. doi:10.1016/j.nicl.2018.09.006
Lindstrøm, E. K., Ringstad, G., Sorteberg, A., Sorteberg, W., Mardal, K. A., and Eide, P. K. (2019). Magnitude and direction of aqueductal cerebrospinal fluid flow: Large variations in patients with intracranial aneurysms with or without a previous subarachnoid hemorrhage. Acta Neurochir. (Wien). 161, 247–256. doi:10.1007/s00701-018-3730-6
Logg, A., Mardal, K.-A., and Wells, G. (2012). Automated solution of differential equations by the finite element method: The FEniCS book, 84. Berlin: Springer Science & Business Media.
Louveau, A., Plog, B. A., Antila, S., Alitalo, K., Nedergaard, M., and Kipnis, J. (2017). Understanding the functions and relationships of the glymphatic system and meningeal lymphatics. J. Clin. Invest. 127, 3210–3219. doi:10.1172/jci90603
Ma, Q., Ineichen, B. V., Detmar, M., and Proulx, S. T. (2017). Outflow of cerebrospinal fluid is predominantly through lymphatic vessels and is reduced in aged mice. Nat. Commun. 8, 1434–1513. doi:10.1038/s41467-017-01484-6
Ma, Q., Ries, M., Decker, Y., Muller, A., Riner, C., Bucker, A., et al. (2019). Rapid lymphatic efflux limits cerebrospinal fluid flow to the brain. Acta Neuropathol. 137, 151–165. doi:10.1007/s00401-018-1916-x
Ma, Q., Decker, Y., Müller, A., Ineichen, B. V., and Proulx, S. T. (2019). Clearance of cerebrospinal fluid from the sacral spine through lymphatic vessels. J. Exp. Med. 216, 2492–2502. doi:10.1084/jem.20190351
Mestre, H., Tithof, J., Du, T., Song, W., Peng, W., Sweeney, A. M., et al. (2018). Flow of cerebrospinal fluid is driven by arterial pulsations and is reduced in hypertension. Nat. Commun. 9, 4878–4879. doi:10.1038/s41467-018-07318-3
Mestre, H., Hablitz, L. M., Xavier, A. L., Feng, W., Zou, W., Pu, T., et al. (2018). Aquaporin-4-dependent glymphatic solute transport in the rodent brain. Elife 7, e40070. doi:10.7554/elife.40070
Nicholson, C., and Phillips, J. (1981). Ion diffusion modified by tortuosity and volume fraction in the extracellular microenvironment of the rat cerebellum. J. physiology 321, 225–257. doi:10.1113/jphysiol.1981.sp013981
Nilsson, C., Stahlberg, F., Thomsen, C., Henriksen, O., Herning, M., and Owman, C. (1992). Circadian variation in human cerebrospinal fluid production measured by magnetic resonance imaging. Am. J. Physiology-Regulatory, Integr. Comp. Physiology 262, R20–R24. doi:10.1152/ajpregu.1992.262.1.r20
Pardridge, W. M. (2016). Csf, blood-brain barrier, and brain drug delivery. Expert Opin. drug Deliv. 13, 963–975. doi:10.1517/17425247.2016.1171315
Proulx, S. T. (2021). Cerebrospinal fluid outflow: A review of the historical and contemporary evidence for arachnoid villi, perineural routes, and dural lymphatics. Cell. Mol. Life Sci. 78, 2429–2457. doi:10.1007/s00018-020-03706-5
Ray, L., Iliff, J. J., and Heys, J. J. (2019). Analysis of convective and diffusive transport in the brain interstitium. Fluids Barriers CNS 16, 6–18. doi:10.1186/s12987-019-0126-9
Ray, L. A., Pike, M., Simon, M., Iliff, J. J., and Heys, J. J. (2021). Quantitative analysis of macroscopic solute transport in the murine brain. Fluids Barriers CNS 18, 55–19. doi:10.1186/s12987-021-00290-z
Ringstad, G., and Eide, P. K. (2020). Cerebrospinal fluid tracer efflux to parasagittal dura in humans. Nat. Commun. 11, 354–359. doi:10.1038/s41467-019-14195-x
Ringstad, G., Vatnehol, S. A. S., and Eide, P. K. (2017). Glymphatic mri in idiopathic normal pressure hydrocephalus. Brain 140, 2691–2705. doi:10.1093/brain/awx191
Ringstad, G., Valnes, L. M., Dale, A. M., Pripp, A. H., Vatnehol, S. A. S., Emblem, K. E., et al. (2018). Brain-wide glymphatic enhancement and clearance in humans assessed with mri. JCI insight 3, 121537. doi:10.1172/jci.insight.121537
Schubert, J. J., Veronese, M., Marchitelli, L., Bodini, B., Tonietto, M., Stankoff, B., et al. (2019). Dynamic 11c-pib pet shows cerebrospinal fluid flow alterations in alzheimer disease and multiple sclerosis. J. Nucl. Med. 60, 1452–1460. doi:10.2967/jnumed.118.223834
Sharp, M. K., Carare, R. O., and Martin, B. A. (2019). Dispersion in porous media in oscillatory flow between flat plates: Applications to intrathecal, periarterial and paraarterial solute transport in the central nervous system. Fluids Barriers CNS 16, 13–17. doi:10.1186/s12987-019-0132-y
Shokri-Kojori, E., Wang, G. J., Wiers, C. E., Demiral, S. B., Guo, M., Kim, S. W., et al. (2018). β-amyloid accumulation in the human brain after one night of sleep deprivation. Proc. Natl. Acad. Sci. U. S. A. 115, 4483–4488. doi:10.1073/pnas.1721694115
Smith, A. J., and Verkman, A. S. (2018). The “glymphatic” mechanism for solute clearance in alzheimer’s disease: Game changer or unproven speculation? FASEB J. 32, 543–551. doi:10.1096/fj.201700999
Spijkerman, J. M., Geurts, L. J., Siero, J. C., Hendrikse, J., Luijten, P. R., and Zwanenburg, J. J. (2019). Phase contrast mri measurements of net cerebrospinal fluid flow through the cerebral aqueduct are confounded by respiration. J. Magn. Reson. Imaging 49, 433–444. doi:10.1002/jmri.26181
SVMTK (2021). SurfaceVolumeMeshingToolKit. Available at: Original-date: 2017-09-22T15:36:28Z https://github.com/SVMTK/SVMTK.
Tarasoff-Conway, J. M., Carare, R. O., Osorio, R. S., Glodzik, L., Butler, T., Fieremans, E., et al. (2015). Clearance systems in the brain—Implications for alzheimer disease. Nat. Rev. Neurol. 11, 457–470. doi:10.1038/nrneurol.2015.119
Tawfik, A. M., Elsorogy, L., Abdelghaffar, R., Naby, A. A., and Elmenshawi, I. (2017). Phase-contrast mri csf flow measurements for the diagnosis of normal-pressure hydrocephalus: Observer agreement of velocity versus volume parameters. Am. J. Roentgenol. 208, 838–843. doi:10.2214/ajr.16.16995
Taylor, A. J., Salerno, M., Dharmakumar, R., and Jerosch-Herold, M. (2016). T1 mapping: Basic techniques and clinical applications. JACC Cardiovasc. Imaging 9, 67–81. doi:10.1016/j.jcmg.2015.11.005
Thal, D. R., Griffin, W. S. T., de Vos, R. A., and Ghebremedhin, E. (2008). Cerebral amyloid angiopathy and its relationship to alzheimer’s disease. Acta Neuropathol. 115, 599–609. doi:10.1007/s00401-008-0366-2
Valnes, L. M., Mitusch, S. K., Ringstad, G., Eide, P. K., Funke, S. W., and Mardal, K. A. (2020). Apparent diffusion coefficient estimates based on 24 hours tracer movement support glymphatic transport in human cerebral cortex. Sci. Rep. 10, 9176–9212. doi:10.1038/s41598-020-66042-5
Vinje, V., Ringstad, G., Lindstrom, E. K., Valnes, L. M., Rognes, M. E., Eide, P. K., et al. (2019). Respiratory influence on cerebrospinal fluid flow–a computational study based on long-term intracranial pressure measurements. Sci. Rep. 9, 9732–9813. doi:10.1038/s41598-019-46055-5
Vinje, V., Eklund, A., Mardal, K.-A., Rognes, M. E., and Støverud, K.-H. (2020). Intracranial pressure elevation alters csf clearance pathways. Fluids Barriers CNS 17, 29–19. doi:10.1186/s12987-020-00189-1
Watts, R., Steinklein, J., Waldman, L., Zhou, X., and Filippi, C. (2019). Measuring glymphatic flow in man using quantitative contrast-enhanced mri. AJNR. Am. J. Neuroradiol. 40, 648–651. doi:10.3174/ajnr.a5931
Weed, L. H. (1922). The cerebrospinal fluid. Physiological reviews 2 (2), 171–203. doi:10.1152/physrev.1922.2.2.171
Xie, L., Kang, H., Xu, Q., Chen, M. J., Liao, Y., Thiyagarajan, M., et al. (2013). Sleep drives metabolite clearance from the adult brain. science 342, 373–377. doi:10.1126/science.1241224
Yamada, S., Ishikawa, M., and Yamamoto, K. (2016). Comparison of csf distribution between idiopathic normal pressure hydrocephalus and alzheimer disease. Am. J. Neuroradiol. 37, 1249–1255. doi:10.3174/ajnr.a4695
Keywords: mathematical modelling, CSF dynamics, subarachnoid space, convection-diffusion, clearance, glymphatics
Citation: Hornkjøl M, Valnes LM, Ringstad G, Rognes ME, Eide P-K, Mardal K-A and Vinje V (2022) CSF circulation and dispersion yield rapid clearance from intracranial compartments. Front. Bioeng. Biotechnol. 10:932469. doi: 10.3389/fbioe.2022.932469
Received: 14 May 2022; Accepted: 17 August 2022;
Published: 12 September 2022.
Edited by:
Seungik Baek, Michigan State University, United StatesReviewed by:
Caterina Guiot, University of Turin, ItalyCopyright © 2022 Hornkjøl, Valnes, Ringstad, Rognes, Eide, Mardal and Vinje. This is an open-access article distributed under the terms of the Creative Commons Attribution License (CC BY). The use, distribution or reproduction in other forums is permitted, provided the original author(s) and the copyright owner(s) are credited and that the original publication in this journal is cited, in accordance with accepted academic practice. No use, distribution or reproduction is permitted which does not comply with these terms.
*Correspondence: Martin Hornkjøl, bWFydGluLmhvcm5ram9sQGhvdG1haWwuY29t
Disclaimer: All claims expressed in this article are solely those of the authors and do not necessarily represent those of their affiliated organizations, or those of the publisher, the editors and the reviewers. Any product that may be evaluated in this article or claim that may be made by its manufacturer is not guaranteed or endorsed by the publisher.
Research integrity at Frontiers
Learn more about the work of our research integrity team to safeguard the quality of each article we publish.