- 1Key Laboratory of Systems Bioengineering (Ministry of Education), SynBio Research Platform, Collaborative Innovation Center of Chemical Science and Engineering (Tianjin), Frontier Science Center for Synthetic Biology (Ministry of Education), Department of Biochemical Engineering, School of Chemical Engineering and Technology, Tianjin University, Tianjin, China
- 2Biodesign Center, Key Laboratory of Systems Microbial Biotechnology, Tianjin Institute of Industrial Biotechnology, Chinese Academy of Sciences, Tianjin, China
Bioelectrochemical systems (BESs) based on Shewanella oneidensis MR-1 offer great promise for sustainable energy/chemical production, but the low rate of electron generation remains a crucial bottleneck preventing their industrial application. Here, we reconstructed a genome-scale metabolic model of MR-1 to provide a strong theoretical basis for novel BES applications. The model iLJ1162, comprising 1,162 genes, 1,818 metabolites and 2,084 reactions, accurately predicted cellular growth using a variety of substrates with 86.9% agreement with experimental results, which is significantly higher than the previously published models iMR1_799 and iSO783. The simulation of microbial fuel cells indicated that expanding the substrate spectrum of MR-1 to highly reduced feedstocks, such as glucose and glycerol, would be beneficial for electron generation. In addition, 31 metabolic engineering targets were predicted to improve electricity production, three of which have been experimentally demonstrated, while the remainder are potential targets for modification. Two potential electron transfer pathways were identified, which could be new engineering targets for increasing the electricity production capacity of MR-1. Finally, the iLJ1162 model was used to simulate the optimal biosynthetic pathways for six platform chemicals based on the MR-1 chassis in microbial electrosynthesis systems. These results offer guidance for rational design of novel BESs.
Introduction
Growing concerns over the depletion of fossil resources and associated environmental problems have motivated the development of sustainable and renewable energy/chemical production using bioelectrochemical systems (BESs) (Apollon et al., 2022). BESs are engineered systems that utilize electrochemical interactions between microbes and electrodes, such as microbial fuel cells (MFCs) (Miyahara et al., 2013) and microbial electrosynthesis systems (MESs) (Nevin et al., 2010). In BESs, electrochemically active bacteria (EAB) play central roles in electrochemical interactions with electrodes (Logan et al., 2019). EAB can transfer electrons to or receive them from extracellularly located redox-active materials (e.g., electrodes) through so-called bi-directional extracellular electron transfer (EET) (Park et al., 2008). In MFCs, electricity is produced by EAB catalyzing the oxidation of feedstocks (e.g., organic compounds) at the electrode. MFCs offer great promise to remediate waste while producing electricity at the same time (Shi et al., 2016; Jourdin et al., 2019). In MESs, EABs accept electrons supplied by electrodes as a driving force for the synthesis of valuable chemicals (Rabaey and Rozendal, 2010; Song et al., 2019). In these processes, the rate of electron flow between electrodes and EABs is directly related to the metabolic activities of the bacteria. Consequently, it is important to study the metabolic network of an EAB and how it can be engineered to improve electricity generation in MFCs and chemical production in MESs (Hirose et al., 2019).
Shewanella oneidensis MR-1 has been used as a model EAB for EET research due to its remarkable respiratory versatility, which allows the bacterium to gain energy through the reduction of a variety of terminal electron acceptors, including oxygen, fumarate, nitrate, trimethylamine N-oxide (TMAO), metal oxides and electrodes (Heidelberg et al., 2002). Due to this capability, S. oneidensis MR-1 has been regarded as model microorganism in MFCs with many successful engineering strategies for the optimization of the EET rate from the perspectives of microbial synthetic biology (Choi et al., 2014; Sekar et al., 2016; Li et al., 2017), electrode materials (Zou et al., 2016), and bio-electrochemical reactor design (Giddings et al., 2015). In addition to transferring electrons to the anode, S. oneidensis MR-1 can also accept electrons from the cathode for the synthesis of chemicals such as 2,3-butanediol (Tefft and Teravest, 2019) and H2 (Rowe et al., 2017) in MESs. Despite these efforts, most studies to date obtained less than 40% Coulombic efficiency in MFCs (Lin et al., 2018), and the product yield of MESs was too low to make them economically competitive (Tefft and Teravest, 2019). The inefficient electron generation and transmembrane transfer are the main factors limiting the EET rate of MFCs. This might be owing to that the metabolic capacity and activity of the EABs have not been well controlled and optimized to a sufficient degree (Gong et al., 2020).
Genome-scale metabolic models (GEMs) aim to capture a systems-level representation of the entirety of metabolic functions using a stoichiometric matrix, which enables sophisticated mathematical analysis of metabolism at the level of whole-cell metabolism (Maarleveld et al., 2013). GEMs have been used to predict targets of chassis cells to enhance the production of many different chemicals (Jian et al., 2017; Gu et al., 2019; Kittikunapong et al., 2021). At present, two GEMs have been reconstructed for S. oneidensis MR-1. One is the iSO783 model, containing 783 genes, 870 reactions and 713 metabolites, which was published in 2010 (Pinchuk et al., 2010). The other is the updated version iMR1_799 containing 799 genes, 933 reactions and 744 metabolites reconstructed in 2014 (Ong et al., 2014). However, the number of genes contained in these two models accounted for less than 20% of annotated ORFs (18.7 and 19.0%, respectively). This resulted in low accuracy the for simulation of biological processes. For example, the iSO783 model cannot predict cell growth using threonine as carbon source, which is inconsistent with the literature (Pinchuk et al., 2010). Moreover, the periplasm compartment is not included in either of these models, yet it contains multiple respiratory chains in S. oneidensis MR-1. Since the publications of iSO783 and iMR1_799, numerous studies have produced new data related to gene and protein function (Hirose et al., 2019), which can be used to upgrade the gene-protein-reaction association of GEMs.
In order to facilitate internal electron generation and transfer in S. oneidensis MR-1, the multi-data and multi-quality-control genome-scale metabolic network model (GEM) iLJ1162 was reconstructed with significantly increased coverage of ORFs as well as accurate simulation of the accepted spectrum of substrates and electron acceptors. We employed iLJ1162 to simulate electricity generation in MFCs using different carbon sources, and identify the optimal pathways as well as overexpression targets for efficient electricity production. Finally, the production of six high-value-added chemicals in MESs using MR-1 as the chassis cell was simulated. These results offer new possibilities for the rational design of BESs.
Materials and Methods
Reconstruction of the Genome-Scale Metabolic Network
The genome of S. oneidensis MR-1 was obtained from GeneBank (GCA_000146165.2) and annotated using RAST, version 2.0 (Overbeek et al., 2005). The draft model was constructed using ModelSEED, version 2.0 (Overbeek et al., 2014). Annotation information from KEGG (Kanehisa et al., 2016), UniProt (Consortium, 2007), DeepEC (Ryu et al., 2019) and iMR1_799 was used to extended the model. For genes that were not included in the draft model but were annotated with EC numbers in KEGG, UniProt, DeepEC, or iMR1_799, reaction information used to supplement GPRs was extracted from the ModelSEED reaction database according to the corresponding EC numbers.
Biomass Synthesis Curation
The biomass composition was adapted from the experimental data of S. oneidensis MR-1, which is consistent with that used in iSO783 (Pinchuk et al., 2010). To simulate biomass synthesis, the cell growth and non-growth associated ATP maintenance values (GAM and NGAM, respectively) were assumed to be identical to those used in iSO783. Gapfilling was carried out under the conditions of an l-lactate uptake rate of 4.06 mmol/gDW/h, while exchange reactions of other basic nutrient components (including H2O, O2, NH3/NH4+, sulfite, phosphate and metal ions) were not restricted. The gapfilling algorithm developed based on weight-added pFBA was as follows:
where
The gap filling process consists of three steps. Firstly, identifying biomass compositions that cannot be synthesized. The demand reaction of each biomass composition was added and set as the optimization target for FBA calculation. If the result is 0, it means that the biomass composition cannot be synthesized, and there are gaps in its synthesis pathway. Then, the gaps for each composition were filled using the weight-added pFBA gapfilling algorithm (Eqs 1–5). The algorithm is implemented by adding 1,000 weights to all reactions in the Model_02 or Model_03 to minimize the number of gapfilling reactions introduced from ModelSEED. Finally, all the reactions used to fill the gaps are added into the Model_02 or Model_03 to enable the synthesis of all the biomass compositions.
Phenotype Microarray Analysis and Curation of Different Nutrient Sources
The wild-type strain S. oneidensis MR-1 used in this study was obtained from our laboratory. The strains were stored at −80°C and revived by growing at 30 °Con LB agar plates containing (per liter): 10 g tryptone, 5 g yeast extract, and 10 g NaCl. The Biolog phenotype microarray was used to evaluate the utilization capabilities of different carbon, nitrogen, sulfur and phosphorus sources in MR-1. The PM1, PM2A, PM3B, PM4A (A-E), PM4A (F-H) plates consist of 190 carbon sources, 95 nitrogen sources, 59 phosphorus sources and 35 sulfur sources, respectively. All these plates were inoculated with cell suspensions at 100 μL/well. The plates were placed at 30°C and monitored for 72 h with readings taken at 15 min intervals. The kinetic information was recorded and quantified using OmniLog OL_FM_12 kinetic software (Biolog, United States) followed by data analysis. In the event of substrate utilization, photographic readings of color intensity resulted from dye reduction were represented in OmniLog units (OU). The substrates that were 1.5-fold higher or 0.8-fold lower than the control were selected as positive or negative results, respectively.
Gapfilling was conducted for those substrates that could be used in the Biolog experiment but not in the model. Two categories were found: 1) Substrates that lack the exchange reactions (From extracellular to intracellular). These gaps were filled manually; 2) Substrates that lack utilization pathway. These gaps were filled using the gap-filling method described above.
Validation of the Metabolic Model
The bounds of exchange reactions were manually set to minimum medium conditions, with unrestricted H2O, O2, NH3/NH4+, sulfite, phosphate and metal ions. The uptake rate of the chosen carbon source was set to 4.06 mmol/gDW/h, while rates for other carbon sources were set to zero. For the simulation of terminal electron acceptors, ATPM (“rxn00062_c0”) was set as the objective function with an l-lactate uptake rate of 4.06 mmol/gDW/h. All the exchange reactions for terminal electron acceptors were turned off, but those for the chosen one ware not restricted. The metabolic model testing suite (MEMOTE) was used to evaluate the consistency and annotation of the model (Lieven et al., 2020). The validation of essential genes consists of two steps. Firstly, for each gene, the associated reactions are removed from the model; Then, biomass was set as objective function with an l-lactate uptake rate of 4.06 mmol/gDW/h. If the result is 0, it means that the gene is essential for growth.
Constraints-Based Flux Analysis Algorithm
Flux balance analysis (FBA) (Orth et al., 2010) is used for optimizing a pre-defined objective function in the specified metabolic constraints. Unless stated otherwise, maximization of the biomass production rate was considered as the objective function. Parsimonious flux balance analysis (pFBA) (Lewis et al., 2010) was chosen for analyzing the product biosynthesis pathway. These methods were conducted using the COBRApy (0.18.1) (Ebrahim et al., 2013). The optimization solvers GLPK and Gurobi were used for linear and quadratic programming. Flux scanning based on the enforced objective flux (FSEOF) algorithm was used to identify the metabolic modification targets (Choi et al., 2010).
Calculation of Coulombic Efficiency and Theoretical Maximum Yield
Coulombic efficiency (CE) is commonly used to quantify the performance of MFCs and is defined as the ratio of electrons actually transferred to the anode to that theoretically present in the starting carbon source (Mao and Verwoerd, 2014). For electrons exported to the anode, four reactions were added into the model to represent the electron production process. The “Electrode_Demand” reaction was set as objective to represent the generation of all electrons. Thus, CE could be represented by:
where
The theoretical maximum yield was calculated according to the ratio of reduction degrees of substrate and product.
where
Results
Reconstruction of a Genome-Scale Metabolic Network of S. oneidensis MR-1
To reconstruct the genome-scale metabolic network of S. oneidensis MR-1, the genome of MR-1 was annotated by RAST, then uploaded into ModelSEED to generate a draft metabolic model. Charges and formulas of metabolites provided by ModelSEED were added into the draft model. The temporary gene IDs (e.g., 211586.77. peg.1131) in the draft model were transformed into specific gene IDs of S. oneidensis MR-1 (e.g., SO_1198) by local Blastp which was utilized for homology matching under the criteria: e-value < 1E-30; identity = 100%. The results of Blastp are listed in Supplementary Datasheet S1 (Supplementary Table S1). Furthermore, all the exchange reaction in the draft model was not constraint. Thus, the bounds of exchange reactions were manually set to minimum medium conditions, while l-lactate (4.06 mmol/gDW/h) was used as sole carbon and energy source. The draft model contained 866 genes, 1,436 metabolites and 1,524 reactions (Figure 1), which was more than the published models iSO783 and iMR1_799. For instance, dihydrofolate reductase was annotated with SO_3646 and SO_0772 in the draft model, but it was only annotated with SO_3646 in iMR1_799.
However, the draft model could only cover 20.6% (866/4196) of the annotated ORFs. Some genes, which have been reported/annotated with clear metabolic functions, were not annotated by RAST. For example, SO_4706 was annotated as malate synthase (EC: 2.3.3.9) in KEGG, but it was missed in the draft model. In order to compensate for these genes and their corresponding reactions, manual extension of the draft model was performed based on the public biochemical databases KEGG and UniProt, in combination with the published model iMR1_799. Moreover, further protein annotation from DeepEC (Ryu et al., 2019), which is a deep learning-based computational tool for prediction of EC numbers, was also used to extend the draft model. A total of 265 genes, 217 reactions and 148 metabolites were extracted to extend the draft model and generate the Model_01. All these genes and reactions can be tracked in Supplementary Datasheet S2 (Supplementary Table S2).
Revision and Gapfilling of Genome-Scale Metabolic Network Model
As shown in Figure 1, the ATP production rate (up to the upper bound of the boundary 1,000) simulated by Model_01 were incorrect. Therefore, Model_01 had to be revised. Analysis of the ATP synthesis pathway revealed that there was infinite energy-generation caused by incorrect reaction directions in the Model_01. The wrong direction of some reactions led to the infinite generation of ATP or proton driving force, resulting in energy-generating cycles (Figure 2). Therefore, the MetaCyc database (Caspi et al., 2019) and iML1515 model (Monk et al., 2017) were used as references to correct the reaction directions in Model_01. The directions of 92 reactions were modified, and energy-generating cycles were avoided (Supplementary Datasheet S1, Supplementary Table S3).
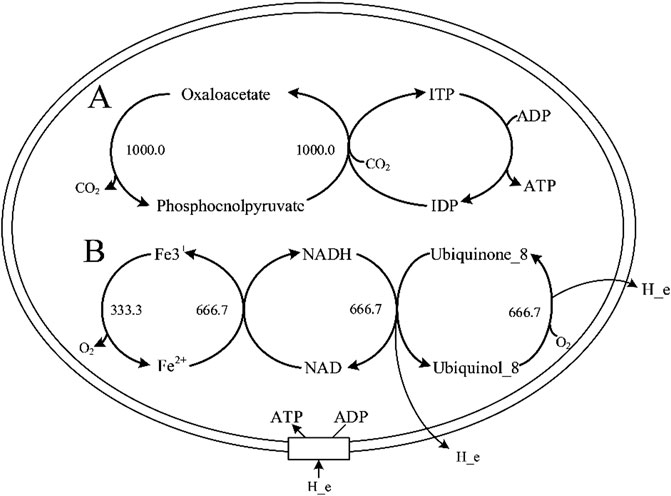
FIGURE 2. Two types of energy-generation cycles in Model_01. (A) represents the infinite generation of ATP in the energy-generation cycle. (B) represents the infinite generation of proton driving force in the energy-generation cycle. Abbreviation: ITP, inosine 5′-triphosphate; IDP, inosine 5′-diphosphate.
After these modifications, the optimal generation rate of ATP was changed from 1,000 to 31.3 mmol/gDW/h. The P/O of the aerobic respiratory chain in Model_01 was 1.5, which is lower than the 2.5 from iSO783 based on literature (Toledo-Cuevas et al., 1998). Thus, all aerobic respiration chains were curated based on iSO783. Consequently, a 54.5 mmol/gDW/h ATP generation rate was obtained. Furthermore, six anaerobic respiratory chains were modified and 11 anaerobic respiratory chains were added (Supplementary Datasheet S1, Supplementary Table S3). After these corrections, Model_02 was obtained (Figure 1). A total of 16 terminal electron acceptors of S. oneidensis MR-1, with known electron transport pathways confirmed by experimental data, were chosen for in silico simulations (Table 1). If the flux of energy generation is greater than 0, the terminal electron acceptor was regarded as a positive result in silico. The agreement of growth between the simulated results of iLJ1162 and in vivo results achieved 100% (16/16) of all tested electron acceptors. Nevertheless, 62.5% (10/16) and 56.25% (9/16) concordance was obtained by iMR1_799 and iSO783, respectively. This can be explained by the key reactions missing in these models. For instance, the electron transfer reaction for the conversion of AsO4− was missing in iMR1_799 and iSO783, but was successfully annotated to be associated with genes SO_4591, SO_1777, SO_1778 and SO_1779 in iLJ1162. Notably, when using metal ions such as Fe3+ and Co3+ as terminal electron acceptors, S. oneidensis MR-1 obtained the highest energy synthesis efficiency in silico. This may be due to the reduction of these electron acceptors to generate the proton driving force and resulting energy production.
However, the simulated growth rate of Model_02 was still zero, while that the reported experimentally measured growth rate of S. oneidensis MR-1 cultured in minimal medium was 0.085 h−1, and the corresponding l-lactate consumption rate was 4.06 mmol/gDW/h under aerobic conditions (Pinchuk et al., 2010). This can be explained by the inability of Model_02 to synthesize a number of biomass precursors, such as lipopolysaccharide and glycogen. Therefore, the weight-added pFBA algorithm was used to fill the gaps in Model_02 (Materials and Methods). A total of 14 reactions (Supplementary Datasheet S1, Supplementary Table S4) were added into Model_02 to generate Model_03. Under the same l-lactate uptake rate, the predicted cell growth rate of iSO783 was 0.108 h−1 while that of iMR1_799 was 0.192 h−1. However, Model_03 predicted a rate of 0.105 h−1, which was the closest to the actual in vivo value of 0.085 h−1 (Supplementary Datasheet S1, Supplementary Figure S1).
In order to improve the substrates utilization capacity of Model_03, 190 carbon sources, 95 nitrogen sources, 59 phosphorus sources and 35 sulfur sources were systematically investigated in Biolog phenotype microarray experiments (Supplementary Datasheet S1, Supplementary Table S5). The Biolog results showed that 27 carbon sources, 32 nitrogen sources, 47 phosphorus sources and 14 sulfur sources can be used by S. oneidensis MR-1. However, 54 of these could not be utilized by Model_03 due to missing transport or utilization reactions (Supplementary Datasheet S1, Supplementary Table S6). After supplementing 187 reactions obtained by the weight-added pFBA algorithm, iLJ1162 was generated (Supplementary Datasheet S2). A total of 289 substrates were used to evaluate the substrate utilization abilities in different models. 94 true positive (substrates that can be used in both the in vivo experiments and simulations) and 157 true negative (substrates that cannot be used in both the in vivo experiments and simulations) results were obtained by iLJ1162 (Figure 3). This means iLJ1162 has an agreement of 86.9% with the experimental data, which is significantly higher than the 62.3 and 61.9% obtained by iMR1_799 and iSO783, respectively (Figures 3B,C). This can be attributed to the extensively extended GPRs associated information and gapfilling curation for these substrates (Supplementary Datasheet S1, Supplementary Table S6). However, due to current limited understanding of metabolism of some substrates, there are still some inconsistencies of in vivo results with those obtained by iLJ1162. For instance, the utilization pathways of Tween 20, 40, and 80 as well as gelatin, are still unknown, and these cannot be simulated in silico.
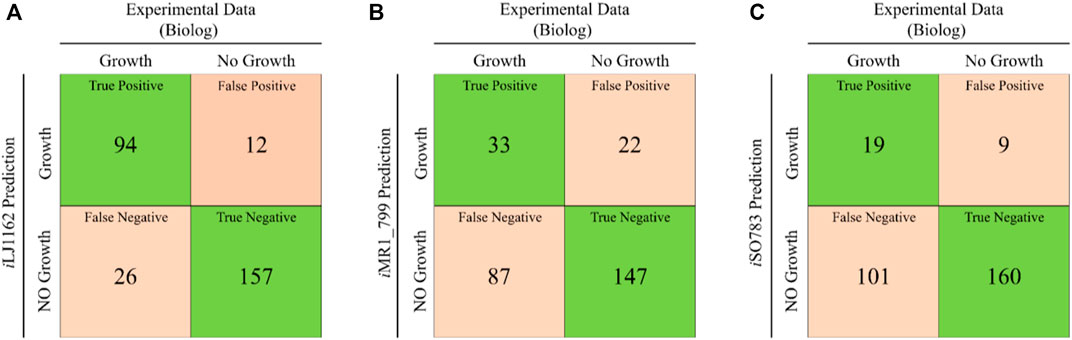
FIGURE 3. Comparison of in silico simulations and in vivo results for the utilization of various substrates. (A) The comparison results of iLJ1162. (B) The comparison results of iMR1_799. (C) The comparison results of iSO783. Green square represents correct predictions, and orange square represents incorrect predictions.
The final version of the model, iLJ1162, includes 1,162 genes, 1818 metabolites and 2084 reactions, which were divided into the three extracellular, periplasmic and cytosolic compartments. MEMOTE was used to assess the quality of iLJ1162 and the other two published GEMs of MR-1 (Supplementary Datasheet S3). In addition to the significant improvements in consistency as well as annotation of reactions and metabolites, iLJ1162 has clear annotations for genes and SBO-terms (Systems Biology Ontology) (Figure 4). These result in a clear advantage of iLJ1162 in the total score. All validation results confirmed the high reliability of iLJ1162, indicating that it can be used for further analysis.
Analysis of Essential Genes of S. voneidensis MR-1
Essential genes are an important characterization of model quality (Lee et al., 2014; Nouri et al., 2020). Therefore, iLJ1162 was used to analyze the essentiality of individual genes of MR-1 under aerobic conditions (Materials and Methods). From the report by Yang et al. (2014), we collected 781 unambiguous gene inactivation results whose gene IDs could be matched to the three MR-1 models. After comparison of in vivo results with in silico simulations, iLJ1162 achieved an accuracy of (681/781) 87.2%, significantly higher than (471/781) 60.3% of iSO783 and (477/781) 61.1% of iMR1_799 (Supplementary Datasheet S4, Supplementary Table S7). This can be majorly attributed to the significantly improved ORFs coverage in iLJ1162. However, there were still some inconsistent results, including 59 false negative results (essentiality for experiment, but non-essentiality for iLJ1162) and 35 false positive results (essentiality for iLJ1162, but non-essentiality for experiment). For these false negative results, it is probably caused by the cellular transcriptional regulation in MR-1. For example, SO_3415 was annotated as aspartate kinase and assessed as essential gene from the in vivo experiments. However, in the iLJ1162, there exist other three isoenzymes (SO_4055, SO_3427 and SO_3986), which might be strictly regulated and unexpressed in MR-1. As for false-positive results, it is likely caused by the incomplete annotation (coverage of ORFs, 27.8%) in iLJ1162. For example, SO_4234 was annotated as 3-isopropylmalate dehydratase and participates an essential role in l-leucine synthesis in all the three models. If the results of the experiment are accurate, MR-1 may have potential isoenzymes for 3-isopropylmalate dehydratase or alternative pathways for leucine synthesis.
Simulation of Electrons Generation Using Different Substrates
In MFCs, electrons obtained through the oxidation of the substrates are transferred to electrodes via the electron transport chain (Figure 5). The electrode is actually one of terminal electron acceptors, and can be set as a pseudo-metabolite which is similar to metal ions such as Fe3+ (Mao and Verwoerd, 2014). Thus, four artificial reactions (Electrode_Sink, → Oxidated_Electrode; Electrode_Reduction_1, 2.0 Oxidated_Electrode + mql7 → 2.0 Reduced_Electrode + Menaquinone_7 + 2.0 H_plus__e0; Electrode_Reduction_2, 2.0 Oxidated_Electrode + methymenaquinol_7 → 2.0 H_plus__e0 + 2.0 Reduced_Electrode + methylmenaquinone_7; Electrode_Demand, Reduced Electrode →) were added into the model for simulation of electron production (Supplementary Datasheet S4, Supplementary Table S8). The “Electrode_Demand” reaction was set as the objective function to represent the generation of electrons.
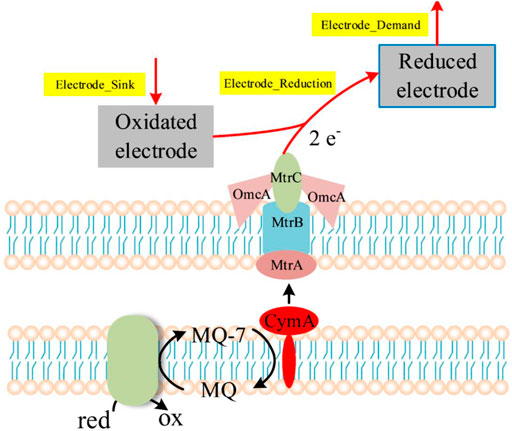
FIGURE 5. Respiratory electron transport in MFCs based on S. oneidensis MR-1. The arrows in the dotted box represent the reactions used for the simulation of electron generation.
To simulate the electron generation ability of MR-1 with different substrates, 15 available carbon sources experimentally validated using Biolog PM1-2 and five heterogenous carbon sources widely used as laboratory substrates were chosen for computational simulation. Taking both the biomass synthesis and electron generation into consideration, the biomass growth rate was set to 10% of the maximum theoretical value, then the maximization of electron generation was set as objective function. This is consistent with that MR-1 grows slowly in MFCs under anaerobic conditions (Logan et al., 2019).
In general, the simulated electron generation rate per C-mole substrate (Figure 6A) was positively correlated with the inherent electron number per C-mole substrate (Figure 6B). Glycerol was predicted to be the optimal substrate for electron generation with the highest rate (4.57 mmol per C-mol glycerol), which was ∼15% higher than that of the common laboratory common substrates l-lactate or N-Acetyl-d-glucosamine (NAG). Many substrates obtained high CE values over 95%, which means that the electrons of these substrates can practically to be fully transferred to the electrode by the metabolic network of MR-1 (Figure 6C). However, some substrates such as adenosine and inosine generated low C-mole electron generation rates despite their high inherent electron numbers (Figures 6A,B), which led to low CEs values from 40 to 70%. This is attributed to the production of intermediate metabolites during the metabolism of these substrates, such as hypoxanthine production for adenosine and inosine utilization, or succinate production for the utilization of the dipeptide glycyl-l-proline. However, these intermediate metabolites cannot be further metabolized by MR-1 under anaerobic conditions, and have to be transported outside of the cell, causing electron waste.
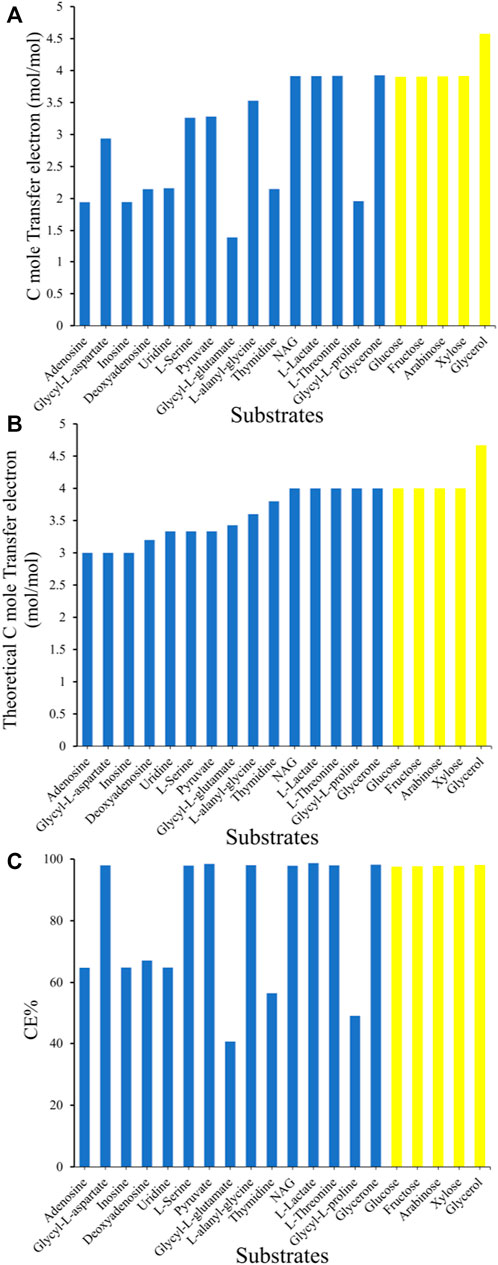
FIGURE 6. Comparison of the electron generation capability of S. oneidensis MR-1 with 20 different carbon sources in silico. (A) The simulated electron generation rate per C-mole substrate. (B) The inherent electron numbers per C-mole substrate. (C) The Coulombic efficiency (CE). The blue columns indicate substrates that are naturally utilized by MR-1, and the yellow columns indicate substrates that require the introduction of heterologous utilization pathways.
Simulation of Intracellular Regeneration of Reducing Equivalents
The enhancement of the regeneration of reducing equivalents, mostly in the form of NADH, has been reported to be one of the most successful metabolic engineering strategies for the improvement of MFCs (Li et al., 2018). In view of the important role of NADH regeneration in MFCs, the iLJ1162 model was used to predict potential modification targets for increasing the NADH regeneration capacity of S. oneidensis MR-1 using the FSEOF algorithm (Choi et al., 2010). An artificial reaction (NADH_G, NADH_c → NAD+_c + H+_c) was introduced into the model and used as the optimization objective to calculate the optimal pathways for NADH regeneration from NAD+. In addition, since there is low or no measurable activity of the pyruvate dehydrogenase (PDH) encoded by SO_0424 and SO_0425 in S. oneidensis MR-1 under anaerobic conditions (Yang et al., 2006), the reactions corresponding to PDH were temporarily turned off. In the simulation, the reactions with significant flux changes from optimal biomass synthesis to optimal NADH regeneration were chosen as potential targets. Two common laboratory substrates for Shewanella species, l-lactate and NAG were introduced as simulated substrates (Supplementary Datasheet S4, Supplementary Table S9). In the modification strategies obtained from the FSEOF simulation with l-lactate as substrate, 17 overexpression targets were mainly distributed in the serine cycle (Figures 7A,B). Among them, NAD+ dependent malate dehydrogenase (Mdh), pyruvate formate-lyase (Pfl) and NAD+ dependent formate dehydrogenase (Fdh) were consistent with previously reported experimental results (Li et al., 2018), which means that the modification strategies predicted by iLJ1162 are meaningful. Thus, other targets may be potentially effective and worth implementing to improve electron production in vivo. In the case of NAG (Figures 7A,C), the simulation results revealed that 31 potential targets, mainly distributed in the PP pathway (e.g., Rpe and RpiA) and serine bypass (e.g., SerA and Mdh).
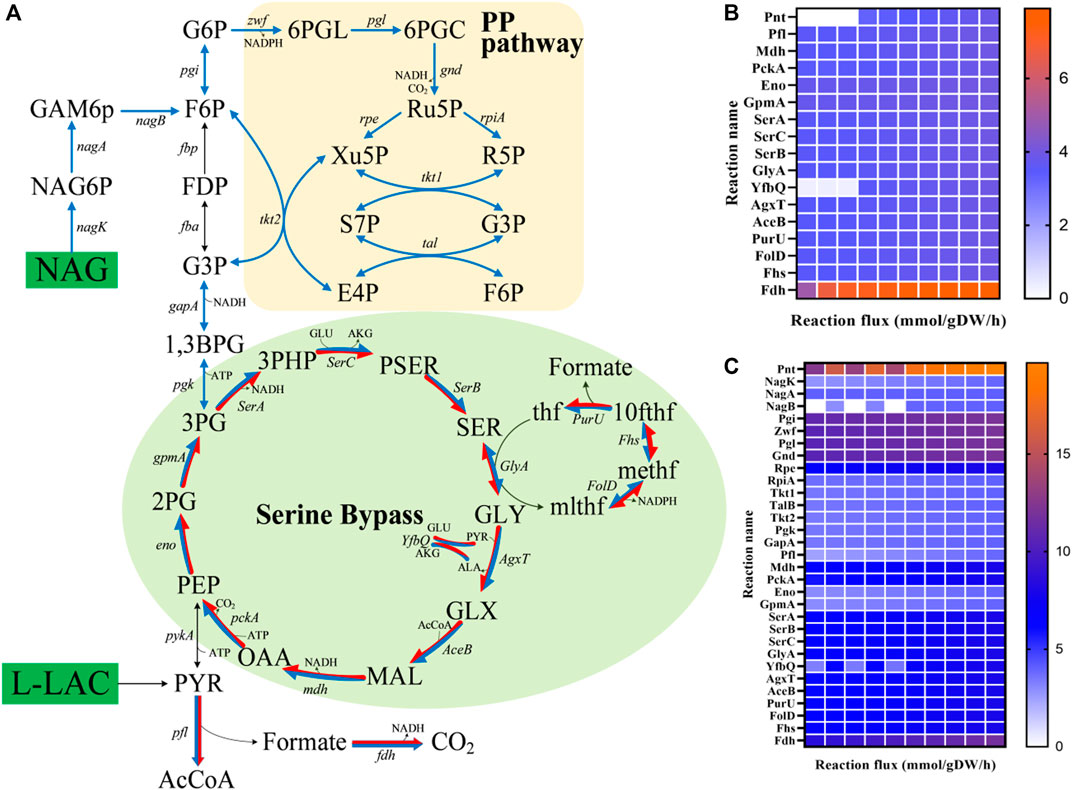
FIGURE 7. FSEOF analysis for NADH regeneration. (A) Distribution of targets predicted by the FSEOF algorithm. The green module represents the serine bypass, and the orange module represents the pentose phosphate pathway (PP pathway). Blue arrows represent overexpression targets when NAG was used as the substrate, and red arrows represent overexpression targets when l-lactate was used as the substrate. (B) Reaction flux changes of targets with an l-lactate uptake rate 4.06 mmol/gDW/h. (C) Reaction flux changes of targets with an NAG uptake rate of 4.06 mmol/gDW/h. Abbreviation: f6p, d-fructose 6-phosphate; fdp, d-fructose 1,6-bisphosphate; g3p, glyceraldehyde 3-phosphate; 1,3bpg, 1,3-Bisphospho-d-glycerate; 3pg, 3-phospho-d-glycerate; 2pg, 2-phospho-d-glycerate; pep, phosphoenolpyruvate; pyr, pyruvate; g6p, d-glucose 6-phosphate; 6pgl, 6-phospho-D-glucono-1,5-lactone; 6pgc, 6-phospho-d-gluconate; accoa, acetyl-CoA; akg, 2-oxoglutarate; oaa, oxaloacetate; mal, malate; glx, glyoxylate; 3php, 3-phosphonooxypyruvate; pser, phosphoserine; 10fthf, 10-formyltetrahydrofolate; methf, 5,10-methenyltetrahydrofolate; mlthf, 5,10-methylenetetrahydrofolate; Ser, l-serine; thf, 5,6,7,8-tetrahydrofolate; ru5p_D, d-ribulose 5-phosphate; xu5p_D, d-xylulose 5-phosphate; r5p, d-ribose 5-phosphate; s7p, sedoheptulose 7-phosphate; e4p, d-erythrose 4-phosphate.
Prediction of Potential NADH Dehydrogenases for Improving EET Rate
An important approach for increasing EET rates based on high intracellular regeneration of NADH is the transfer of electrons from NADH to the respiratory chain. The first step of the respiratory chain is the dehydrogenation of NADH by NADH dehydrogenases (NDHs) to release electrons, which are important for energy supply and cell growth (Figure 8A). Three types of prokaryotic NADH dehydrogenases are generally recognized in the literature (Melo et al., 2004), including those with proton-pumping activity (type I), proton/sodium-pumping activity (type II) and sodium-pumping activity (type III). Notably, the genome of S. oneidensis MR-1 encodes all three types of NDHs (type I: SO_1009-SO_1021, type II: SO_1103-SO_1108 and SO_0902-SO_0907, type III: SO_3517). It has been reported that electrical current production or growth rate with NAG as the substrate under anaerobic conditions would be extremely inhibited by knocking out NDHs (Kasai et al., 2019; Madsen and Teravest, 2019). However, the simulation results of iLJ1162 indicated that there was no obvious decrease of electron production or growth rate. Further analysis revealed that there are two alternative pathways with NDHs function, including 1) LdhA (pyruvate reductase encoded by SO_0968, EC: 1.1.1.28) and Dld (lactate dehydrogenase by SO_1521, EC: 1.1.2.4) (Figure 8B); 2) ProC (pyrroline-5-carboxylate reductase encoded by SO_3354, EC: 1.5.1.2) and PutA (proline dehydrogenase encoded by SO_3774, EC: 1.5.5.2) (Figure 8C). The former has been experimentally verified (Kasai et al., 2019), while the latter was uncovered by iLJ1162 for the first time. However, the two pathways failed to play a role in electricity production or cell growth, probably due to the strict transcriptional regulation and low pools of intermediate metabolites (d-lactate or l-proline) (Kasai et al., 2019; Madsen and Teravest, 2019). Thus, overexpression of NDHs and/or activation of LdhA-Dld/ProC-PutA might be an effective strategy for channeling more electrons toward MQ to improve EET rate.
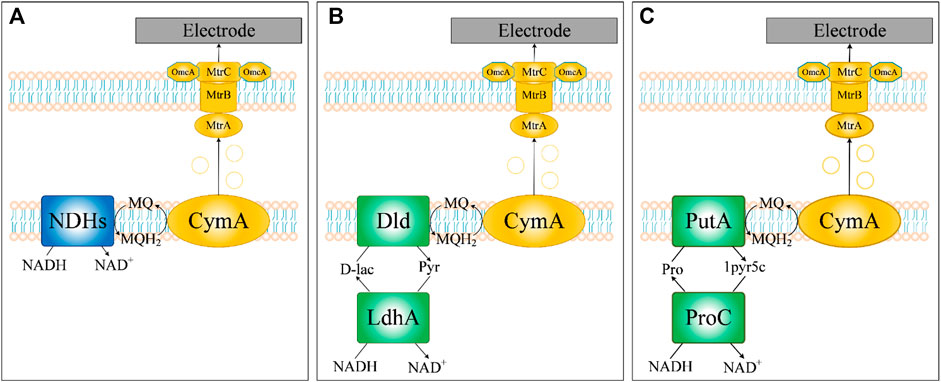
FIGURE 8. Three NADH dehydrogenases predicted by iLJ1162 for electron transfer in S. oneidensis MR-1. (A) Electron transfer based on NADH dehydrogenases. (B) Electron transfer based on LdhA-Dld. (C) Electron transfer based on ProC-PutA.
Simulation of the Synthesis of High-Value-Added Products by iLJ1162
In MES systems, electrons from the cathode are transferred to the microbial cells and used for the synthesis of value-added chemicals (Logan et al., 2019). The MES process of S. oneidensis MR-1 can be summarized as NADH generation by receiving extracellular electrons and protons using NAD+ (Yang et al., 2015; Rowe et al., 2017). Thus, the reaction of “NADH_R” (2.0 e− + H_p + NAD_c → NADH_c) and ‘Sink_e−’ (→ e−) were added to represent the electron receiving process. Six high value products were chosen for simulation in MESs, including 3-hydroxypropanoate (3HP), isopropanol (2ppoh), 3-hydroxybutanoate (3HB), acetoin (Actn), succinate (Suc), and 2,3-butanediol (23btdl). All these products require no more than two heterologous reactions in MR-1. The cell growth was omitted to simplify the complexity of biosynthesis pathways for different products, which is also consistent with that EAB almost did not grow in MESs (Chen et al., 2018; Tefft and Teravest, 2019) The demand reactions of these products are set as objective function respectively.
The simulation results of these six products in MESs using l-lactate as sole carbon source under anaerobic conditions are shown in Table 2. Without inward electron (no extra electron supply), the simulated yields were lower than their theoretical maximum yields calculated from reducing degrees. However, with inward electrons, all products achieved or exceeded the theoretical maximum yields calculated from the reduction degrees. In the simulation of MESs, CO2 is infinitely supplied, which is consistent with the actual MESs aerated with CO2 to ensure a strict anaerobic condition (Chen et al., 2018). Thus, with extra electron supply (inward electrons), it is theoretically feasible to fix environmental CO2, and consequently break mass balance merely based on substrate. As one of the C4 platform chemicals, 3-hydroxybutanoate (3HB) was chosen as an example for MESs simulation using iLJ1162. As shown in Figure 9, a simulated yield of 0.43 mol/mol was obtained using l-lactate as the sole electron source, which is only 64% of the theoretical maximum yield calculated from reducing degrees. This can be explained by the fact that the key intermediate metabolite formate cannot be further converted to 3HB by the metabolic network of MR-1, resulting in the loss of one third of total carbon in l-lactate. Moreover, to obtain NADPH for 3HB synthesis, a part of carbon fluxes was directed into the serine bypass and PP pathway, and oxidized to release electrons for NADPH generation.
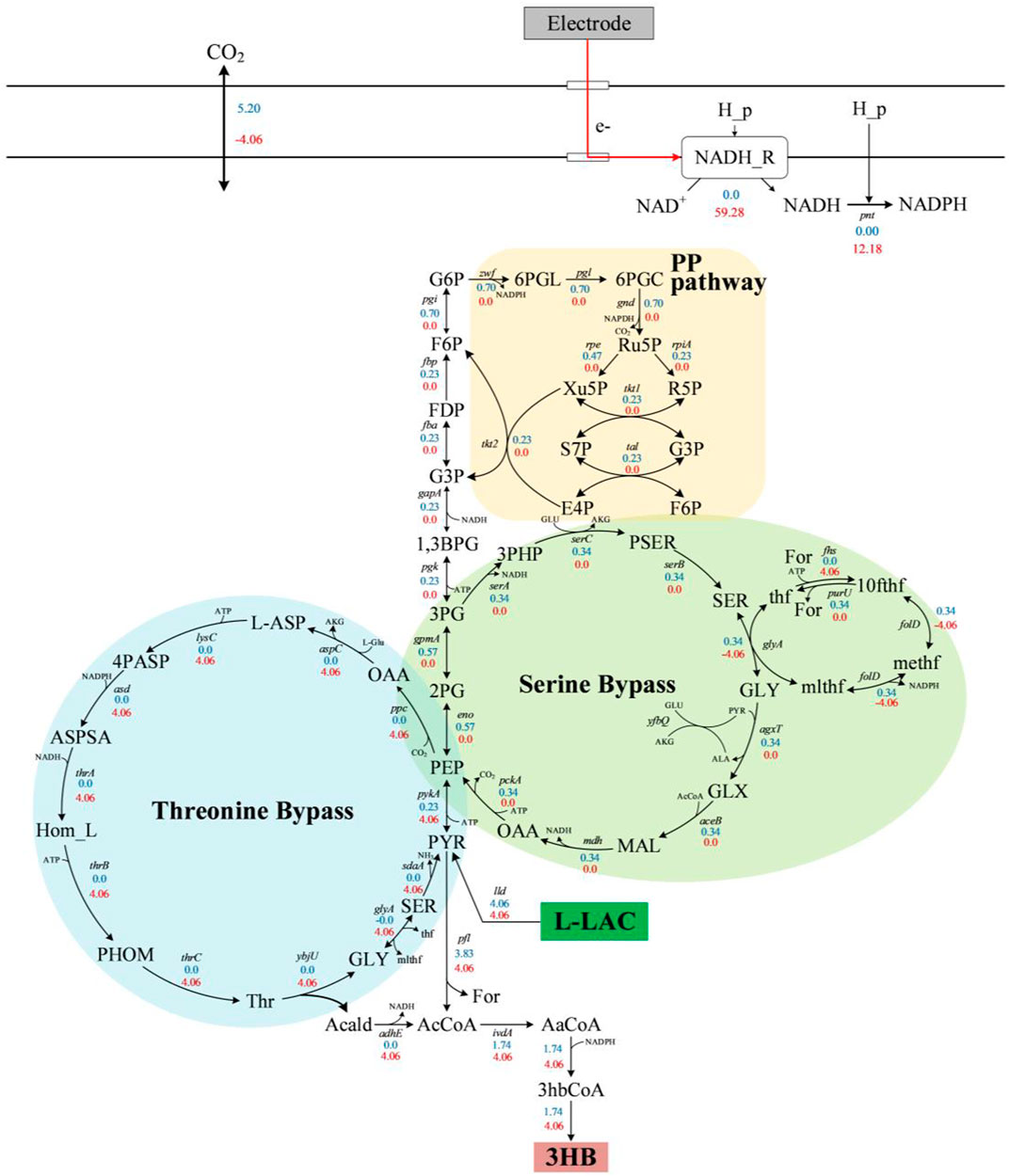
FIGURE 9. Simulation results of the flux distribution for 3HB synthesis. Blue or red fluxes represent iLJ1162 simulation results without or with inward electrons. The blue module represents the threonine bypass, the green module represents the serine bypass and orange represents the pentose phosphate pathway. Abbreviation: For, formate; L-asp, l-aspartate; 4pasp, 4-phospho-l-aspartate; aspsa, l-aspartate 4-semialdehyde; hom_L, l-homoserine; phom, O-phospho-l-homoserine; Thr, l-threonine; acald, acetaldehyde; AaCoA, acetoacetyl-CoA; 3hbCoA, 3-hydroxybutanoyl-CoA.
With inward electrons from an electrode, the simulated yield was significantly increased to 1 mol/mol l-lactate, which was 57% higher than the its theoretical maximum yield. This can be explained by the significantly increased fluxes of the threonine bypass (Figure 9) (Lin et al., 2015). The threonine bypass is able to not only utilize additional reducing equivalents to fix extracellular CO2 (4.06 mmol/gDW/h, Figure 9), but also re-assimilate formate released by PFL (4.06 mmol/gDW/h, Figure 9), and generate the key precursor acetyl-CoA with a higher yield than that obtained merely via the carbon-losing PFL pathway. As a result, 3HB can be generated at a rate of 4.06 mmol/gDW/h in silico, which represents the yield of 1 mol/mol l-lactate. It is worth mentioning that the 3HB yield would not be infinitely improved in MESs of MR-1, which might be owing to the lacking of key intermediate metabolite formate. Finally, these results indicated that metabolic regulation of the threonine bypass will be an important aspect of MR-1 for enhancing the yield of chemicals in future MES.
Since glycerol turns out to be the best substrate for election generation (Figure 6), we also simulated the product synthesis using glycerol in MESs (Supplementary Datasheet S4, Supplementary Table S10). Three major findings were obtained: 1) Without inward electrons, the product yields using glycerol are higher that using l-lactate, which is consistent with that in electron generation. 2) With inward electrons, the product yields using glycerol or l-lactate are equivalent, which means that extra electron supplying might eliminate the difference caused by different reducing degree in substrates. 3) The requirement of inward electrons for the same product is significantly lowered when using glycerol rather than l-lactate. This indicates that glycerol might still be a better substrate in MESs, which lowers the demand for the electron uptake rate of S. oneidensis strains.
Discussion
Genome-scale metabolic modeling is a systematic biology approach for the construction of a framework for the integrative analysis of metabolic functions of a microorganism. In this study, we reconstructed a well-curated genome-scale metabolic model for the facultative dissimilatory metal-reducing bacterium S. oneidensis MR-1, a model EAB with applications in energy-generation and bioelectrochemical product synthesis. The model iLJ1162 comprised 1,162 genes, 1837 metabolites and 2078 reactions located throughout the extracellular, periplasm and cytosol compartments. GPRs extracted from various databases were used to extend the model, so that iLJ1162 can cover 27.8% (1,162/4196) of the annotated ORFs, which is significantly higher than the 19% of iMR1_799 (799/4196) and 18.9% of iSO783 (783/4196) (Table 3). In addition, the curation of the respiratory chain not only enables the iLJ1162 model to simulate normal cellular energy production, but can also simulate the use of a variety of terminal electron acceptors. This indicated that iLJ1162 has the potential to analyze the evolution process of respiratory versatility in Shewanella. The replacement of the biomass composition and gapfilling enabled iLJ1162 to specifically simulate the growth and metabolic state of S. oneidensis MR-1. At the same time, the calculation results of the growth rate (0.105 h−1), which are the closest to the experimental data (0.085 h−1), indicated that iLJ1162 could accurately simulate the growth and metabolism of S. oneidensis MR-1. Furthermore, 289 substrates that were screened based on phenotypic assays were used to elevate and validate the model. The results of iLJ1162 reached 86.9% concordance with the wet-lab results, while iMR1_799 and iSO783 reached 62.3 and 61.9% respectively. This demonstrated that iLJ1162 could more accurately simulate substrate utilization than earlier models. Throughout the model construction process, the quality and accuracy of the model was gradually enhanced, and it achieved a high score of 90 in the MEMOTE evaluation. This not only proves the high quality of iLJ1162, but also indicates the rationality and comprehensiveness of the model construction process.
MFCs based on bio-electrochemical processes initially gained significant attention due to their unique potential to generate energy. In MFCs, the electron pool of EAB plays an important role in electricity production. In this work, we simulated the potential electron generation capacity of different substrates, screened genetic targets for increasing intracellular NADH regeneration, as well as the transfer pathway for electrons from NADH to enter the respiratory chain, providing guidance for controlling and optimizing the metabolic capacity and activity of MR-1 to improve the EET rate. Firstly, we analyzed the electrons produced from all the carbon sources that can be utilized by MR-1 (Figure 6). Glycerol, which is a heterologous substrate for S. oneidensis MR-1, was predicted to be the optimal substrate for electron generation. Moreover, the FSEOF algorithm was used to find targets for increasing the ratio of NADH/NAD+. When using l-lactate as electron donor, PflB, FDH and MDH were predicted as engineering targets for efficient electricity generation, which was consistent with previous experimental results (Li et al., 2018). This indicates that our simulation results are meaningful, and other targets also merit further wet-lab verification to improve the electricity production of S. oneidensis MR-1 in MFCs. In future, a systematic simulation based on iLJ1162 using more algorithms such as OptForce (Ranganathan et al., 2010), MOMA (Segrè et al., 2002) and FVSEOF (Park et al., 2012), is expected to provide a more comprehensive guidance for rational design of novel BESs. Notably, the serine bypass was predicted to be the main target distribution pathway regardless of whether lactate or NAG was used as the substrate. Under anaerobic conditions, succinate cannot be directly converted to fumarate, resulting in interruption of the TCA cycle and an increase of metabolic flow into the serine bypass to produce NADH. Thus, controlling the expression level of the targets in the serine bypass may enhance electron generation. Furthermore, increasing the flow of NADH to the respiratory chain could also increase the EET rate. Tao et al. (2016) found that the MFC current density produced by an engineered strain with upregulation of non-proton-pumping NADH dehydrogenase was 3.3-fold higher than that of wild-type MR-1. Our results indicated that l-proline and d-lactate could serve as electron-transfer intermediates between NADH and quinol, and the functions of NDHs could be replaced by corresponding enzymes (LdhA/Dld and ProC/PutA). The function of d-lactate was confirmed by Watanabe et al. (Nakagawa et al., 2015; Kasai et al., 2019) using glucose or NAG as carbon source. However, to the best of our knowledge, none of the studies has reported that l-proline can be used as a temporary sink for electron transfer in S. oneidensis MR-1. Thus, if genetic manipulation is feasible, controlling the expression levels of LdhA/Dld and/or ProC/PutA might increase the rate of EET.
MESs represents a sustainable platform that converts waste into resources, using microorganisms within an electrochemical cell (Gajda et al., 2021). As initially reported by Ross et al. (2011), Rowe et al., 2017 as well as Tefft et al. (Tefft and Teravest, 2019), the electrons from cathode are confirmed to be transferred to MQ7, from where they are further passed to NAD+ to form NADH with proton-motive force from a light-driven proton pump (proteorhodopsin). These works open up the possibility to develop efficient MESs based on MR-1 chassis. Here, we used the iLJ1162 to profile the optimal biosynthesis pathways for six different chemicals in MESs. Notably, these optimal pathways are quite different with those without inward electrons. Taking 3HB as an example (Figure 9), the NADH derived from inward electrons can not only save the fluxes once into the serine bypass and PP pathway, but also provide extra reducing force for CO2 and formate fixation through the threonine bypass. Thus, the simulated yield with inward electrons exceeded its maximum theoretical yield (Table 2). It is worth mentioning that the threonine bypass has been proven to be an efficient engineering target for improving for acetyl-CoA derived poly (3-hydroxybutyrate) production in E. coli in our previous work (Lin et al., 2015). Therefore, our simulation results can also promisingly guide further engineering of MR-1 chassis for efficient MESs in future.
Conclusion
In this study, we reconstructed a high-quality metabolic model of S. oneidensis MR-1. The model, named iLJ1162, is more comprehensive in scope than previous models and updated based on the latest gene annotations, databases and literature. The model validation was achieved by comparing model predictions to experimentally obtained results or the literature, and demonstrated a high degree of concordance. As a genome-scale metabolic network model, iLJ1162 was used to simulate and analyze the performance MR-1 in BESs from a systems perspective. Particularly in MFCs, iLJ1162 was used to analyze the electricity production capacity of 20 substrates. The model identified more than 31 genetic modification targets and two alternative NDHs pathways, which could enhance the electricity production capacity and EET rate of MR-1 in MFCs. In addition, iLJ11621 was also successfully used to simulate and analyze the synthesis of six high-value chemicals in MESs, offering preliminary theoretical exploration to guide the feasible direction of bioelectrochemical synthesis using MR-1. This advanced and comprehensive genome-scale metabolic model offers a new platform for a better understanding the metabolic features of S. oneidensis MR-1 at a systems-level, enabling the development of improved strains for BESs applications.
Data Availability Statement
The datasets presented in this study can be found in online repositories. The names of the repository/repositories and accession number(s) can be found in the article/Supplementary Material.
Author Contributions
Model reconstruction, JL, QY, WY, and XL; Experiments, JL, YM, SK, and YG; Simulation, JL, QY, FW, and JC; Data analysis, JL, QY, and YM; Original draft writing, JL, QY, and YM. Review and editing, JL, QY, YM, JZ, ZW, and HM. All authors contributed to the article and approved the submitted version.
Funding
This work was financially supported by the National Key Research and Development Program of China (2018YFA0901300), the National Natural Science Foundation of China (21908239, 32101186), the Tianjin Synthetic Biotechnology Innovation Capacity Improvement Project (TSBICIP-PTJS-001), and Youth Innovation Promotion Association CAS.
Conflict of Interest
The authors declare that the research was conducted in the absence of any commercial or financial relationships that could be construed as a potential conflict of interest.
Publisher’s Note
All claims expressed in this article are solely those of the authors and do not necessarily represent those of their affiliated organizations, or those of the publisher, the editors and the reviewers. Any product that may be evaluated in this article, or claim that may be made by its manufacturer, is not guaranteed or endorsed by the publisher.
Supplementary Material
The Supplementary Material for this article can be found online at: https://www.frontiersin.org/articles/10.3389/fbioe.2022.913077/full#supplementary-material
References
Apollon, W., Rusyn, I., González-Gamboa, N., Kuleshova, T., Luna-Maldonado, A. I., Vidales-Contreras, J. A., et al. (2022). Improvement of Zero Waste Sustainable Recovery Using Microbial Energy Generation Systems: A Comprehensive Review. Sci. Total Environ. 817, 153055. doi:10.1016/j.scitotenv.2022.153055
Bretschger, O., Obraztsova, A., Sturm, C. A., Chang, I. S., Gorby, Y. A., Reed, S. B., et al. (2007). Current Production and Metal Oxide Reduction by Shewanella Oneidensis Mr-1 Wild Type and Mutants. Appl. Environ. Microbiol. 73, 7003–7012. doi:10.1128/aem.01087-07
Caspi, R., Billington, R., Keseler, I. M., Kothari, A., Krummenacker, M., Midford, P. E., et al. (2019). The MetaCyc Database of Metabolic Pathways and Enzymes - a 2019 Update. Nucleic Acids Res. 48, D445–D453. doi:10.1093/nar/gkz862
Chen, X., Cao, Y., Li, F., Tian, Y., and Song, H. (2018). Enzyme-Assisted Microbial Electrosynthesis of Poly(3-Hydroxybutyrate) via CO2 Bioreduction by Engineered Ralstonia Eutropha. ACS Catal. 8, 4429–4437. doi:10.1021/acscatal.8b00226
Choi, D., Lee, S. B., Kim, S., Min, B., Choi, I.-G., and Chang, I. S. (2014). Metabolically Engineered Glucose-Utilizing Shewanella Strains under Anaerobic Conditions. Bioresour. Technol. 154, 59–66. doi:10.1016/j.biortech.2013.12.025
Choi, H. S., Lee, S. Y., Kim, T. Y., and Woo, H. M. (2010). In Silico Identification of Gene Amplification Targets for Improvement of Lycopene Production. Appl. Environ. Microbiol. 76, 3097–3105. doi:10.1128/aem.00115-10
Consortium, T. U. (2007). The Universal Protein Resource (Uniprot). Nucleic Acids Res. 35, D193–D197. doi:10.1093/nar/gkl929
Coursolle, D., and Gralnick, J. A. (2010). Modularity of the Mtr Respiratory Pathway of Shewanella Oneidensis Strain Mr-1. Mol. Microbiol. 77, 995–1008. doi:10.1111/j.1365-2958.2010.07266.x
Dos Santos, J.-P., Iobbi-Nivol, C., Couillault, C., Giordano, G., and Méjean, V. (1998). Molecular Analysis of the Trimethylamine N -oxide (TMAO) Reductase Respiratory System from a Shewanella Species. J. Mol. Biol. 284, 421–433. doi:10.1006/jmbi.1998.2155
Dugar, D., and Stephanopoulos, G. (2011). Relative Potential of Biosynthetic Pathways for Biofuels and Bio-Based Products. Nat. Biotechnol. 29, 1074–1078. doi:10.1038/nbt.2055
Ebrahim, A., Lerman, J. A., Palsson, B. O., and Hyduke, D. R. (2013). Cobrapy: Constraints-Based Reconstruction and Analysis for Python. BMC Syst. Biol. 7, 74–76. doi:10.1186/1752-0509-7-74
Gajda, I., You, J., Mendis, B. A., Greenman, J., and Ieropoulos, I. A. (2021). Electrosynthesis, Modulation, and Self-Driven Electroseparation in Microbial Fuel Cells. iScience 24, 102805. doi:10.1016/j.isci.2021.102805
Gao, H., Barua, S., Liang, Y., Wu, L., Dong, Y., Reed, S., et al. (2010). Impacts of Shewanella Oneidensis C -type Cytochromes on Aerobic and Anaerobic Respiration. Microb. Biotechnol. 3, 455–466. doi:10.1111/j.1751-7915.2010.00181.x
Gao, H., Yang, Z. K., Barua, S., Reed, S. B., Romine, M. F., Nealson, K. H., et al. (2009). Reduction of Nitrate in Shewanella Oneidensis Depends on Atypical Nap and Nrf Systems with Napb as a Preferred Electron Transport Protein from Cyma to Napa. Isme J. 3, 966–976. doi:10.1038/ismej.2009.40
Giddings, C. G., Nevin, K. P., Woodward, T., Lovley, D. R., and Butler, C. S. (2015). Simplifying Microbial Electrosynthesis Reactor Design. Front. Microbiol. 6, 468. doi:10.3389/fmicb.2015.00468
Gong, Z., Yu, H., Zhang, J., Li, F., and Song, H. (2020). Microbial Electro-Fermentation for Synthesis of Chemicals and Biofuels Driven by Bi-directional Extracellular Electron Transfer. Synthetic Syst. Biotechnol. 5, 304–313. doi:10.1016/j.synbio.2020.08.004
Gralnick, J. A., Vali, H., Lies, D. P., and Newman, D. K. (2006). Extracellular Respiration of Dimethyl Sulfoxide by Shewanella Oneidensis Strain Mr-1. Proc. Natl. Acad. Sci. U.S.A. 103, 4669–4674. doi:10.1073/pnas.0505959103
Gu, C., Kim, G. B., Kim, W. J., Kim, H. U., and Lee, S. Y. (2019). Current Status and Applications of Genome-Scale Metabolic Models. Genome Biol. 20, 121. doi:10.1186/s13059-019-1730-3
Hau, H. H., Gilbert, A., Coursolle, D., and Gralnick, J. A. (2008). Mechanism and Consequences of Anaerobic Respiration of Cobalt by Shewanella Oneidensis Strain Mr-1. Appl. Environ. Microbiol. 74, 6880–6886. doi:10.1128/aem.00840-08
Heidelberg, J. F., Paulsen, I. T., Nelson, K. E., Gaidos, E. J., Nelson, W. C., Read, T. D., et al. (2002). Genome Sequence of the Dissimilatory Metal Ion-Reducing Bacterium Shewanella Oneidensis. Nat. Biotechnol. 20, 1118–1123. doi:10.1038/nbt749
Hirose, A., Kouzuma, A., and Watanabe, K. (2019). Towards Development of Electrogenetics Using Electrochemically Active Bacteria. Biotechnol. Adv. 37, 107351. doi:10.1016/j.biotechadv.2019.02.007
Jian, X., Li, N., Chen, Q., and Hua, Q. (2017). Model-Guided Identification of Novel Gene Amplification Targets for Improving Succinate Production in Escherichia Coli Nzn111. Integr. Biol. 9, 830–835. doi:10.1039/c7ib00077d
Jourdin, L., Winkelhorst, M., Rawls, B., Buisman, C. J. N., and Strik, D. P. B. T. B. (2019). Enhanced Selectivity to Butyrate and Caproate above Acetate in Continuous Bioelectrochemical Chain Elongation from CO2: Steering with CO2 Loading Rate and Hydraulic Retention Time. Bioresour. Technol. Rep. 7, 100284. doi:10.1016/j.biteb.2019.100284
Kanehisa, M., Furumichi, M., Tanabe, M., Sato, Y., and Morishima, K. (2016). Kegg: New Perspectives on Genomes, Pathways, Diseases and Drugs. Nucleic Acids Res. 45, D353–D361. doi:10.1093/nar/gkw1092
Kasai, T., Suzuki, Y., Kouzuma, A., and Watanabe, K. (2019). Roles of D-Lactate Dehydrogenases in the Anaerobic Growth of Shewanella Oneidensis Mr-1 on Sugars. Appl. Environ. Microbiol. 85, e02668–02618. doi:10.1128/AEM.02668-18
Kittikunapong, C., Ye, S., Magadán-Corpas, P., Pérez-Valero, Á., Villar, C. J., Lombó, F., et al. (2021). Reconstruction of a Genome-Scale Metabolic Model of Streptomyces Albus J1074: Improved Engineering Strategies in Natural Product Synthesis. Metabolites 11, 304. doi:10.3390/metabo11050304
Lee, N. R., Lakshmanan, M., Aggarwal, S., Song, J. W., Karimi, I. A., Lee, D. Y., et al. (2014). Genome-Scale Metabolic Network Reconstruction and In Silico Flux Analysis of the Thermophilic Bacterium Thermus Thermophilus Hb27. Microb. Cell Fact. 13, 61–14. doi:10.1186/1475-2859-13-61
Lewis, N. E., Hixson, K. K., Conrad, T. M., Lerman, J. A., Charusanti, P., Polpitiya, A. D., et al. (2010). Omic Data from Evolved E. coli Are Consistent with Computed Optimal Growth from Genome‐scale Models. Mol. Syst. Biol. 6, 390. doi:10.1038/msb.2010.47
Li, F., Li, Y., Sun, L., Li, X., Yin, C., An, X., et al. (2017). Engineering Shewanella Oneidensis Enables Xylose-Fed Microbial Fuel Cell. Biotechnol. Biofuels 10, 196. doi:10.1186/s13068-017-0881-2
Li, F., Li, Y., Sun, L., Chen, X., An, X., Yin, C., et al. (2018). Modular Engineering Intracellular Nadh Regeneration Boosts Extracellular Electron Transfer of Shewanella Oneidensis Mr-1. ACS Synth. Biol. 7, 885–895. doi:10.1021/acssynbio.7b00390
Lieven, C., Beber, M. E., Olivier, B. G., Bergmann, F. T., Ataman, M., Babaei, P., et al. (2020). Memote for Standardized Genome-Scale Metabolic Model Testing. Nat. Biotechnol. 38, 272–276. doi:10.1038/s41587-020-0446-y
Lin, T., Ding, W., Sun, L., Wang, L., Liu, C.-G., and Song, H. (2018). Engineered Shewanella Oneidensis-Reduced Graphene Oxide Biohybrid with Enhanced Biosynthesis and Transport of Flavins Enabled a Highest Bioelectricity Output in Microbial Fuel Cells. Nano Energy 50, 639–648. doi:10.1016/j.nanoen.2018.05.072
Lin, Z., Zhang, Y., Yuan, Q., Liu, Q., Li, Y., Wang, Z., et al. (2015). Metabolic Engineering of Escherichia Coli for Poly(3-Hydroxybutyrate) Production via Threonine Bypass. Microb. Cell Fact. 14, 185. doi:10.1186/s12934-015-0369-3
Logan, B. E., Rossi, R., Ragab, A. A., and Saikaly, P. E. (2019). Electroactive Microorganisms in Bioelectrochemical Systems. Nat. Rev. Microbiol. 17, 307–319. doi:10.1038/s41579-019-0173-x
Maarleveld, T. R., Khandelwal, R. A., Olivier, B. G., Teusink, B., and Bruggeman, F. J. (2013). Basic Concepts and Principles of Stoichiometric Modeling of Metabolic Networks. Biotechnol. J. 8, 997–1008. doi:10.1002/biot.201200291
Madsen, C. S., and Teravest, M. A. (2019). Nadh Dehydrogenases Nuo and Nqr1 Contribute to Extracellular Electron Transfer by Shewanella Oneidensis Mr-1 in Bioelectrochemical Systems. Sci. Rep. 9, 14959. doi:10.1038/s41598-019-51452-x
Maier, T. M., Myers, J. M., and Myers, C. R. (2003). Identification of the Gene Encoding the Sole Physiological Fumarate Reductase in Shewanella Oneidensis Mr-1. J. Basic Microbiol. 43, 312–327. doi:10.1002/jobm.200390034
Mao, L., and Verwoerd, W. S. (2014). Theoretical Exploration of Optimal Metabolic Flux Distributions for Extracellular Electron Transfer by Shewanella Oneidensis Mr-1. Biotechnol. Biofuels 7, 118–120. doi:10.1186/s13068-014-0118-6
Marshall, M. J., Beliaev, A. S., Dohnalkova, A. C., Kennedy, D. W., Shi, L., Wang, Z., et al. (2006). C-type Cytochrome-dependent Formation of U(Iv) Nanoparticles by Shewanella Oneidensis. PLoS Biol. 4, e268. doi:10.1371/journal.pbio.0040268
Melo, A. M. P., Bandeiras, T. M., and Teixeira, M. (2004). New Insights into Type II NAD(P)H:Quinone Oxidoreductases. Microbiol. Mol. Biol. Rev. 68, 603–616. doi:10.1128/mmbr.68.4.603-616.2004
Miyahara, M., Hashimoto, K., and Watanabe, K. (2013). Use of Cassette-Electrode Microbial Fuel Cell for Wastewater Treatment. J. Biosci. Bioeng. 115, 176–181. doi:10.1016/j.jbiosc.2012.09.003
Monk, J. M., Lloyd, C. J., Brunk, E., Mih, N., Sastry, A., King, Z., et al. (2017). Iml1515, a Knowledgebase that Computes Escherichia Coli Traits. Nat. Biotechnol. 35, 904–908. doi:10.1038/nbt.3956
Myers, J. M., Antholine, W. E., and Myers, C. R. (2004). Vanadium(V) Reduction by Shewanella Oneidensis Mr-1 Requires Menaquinone and Cytochromes from the Cytoplasmic and Outer Membranes. Appl. Environ. Microbiol. 70, 1405–1412. doi:10.1128/aem.70.3.1405-1412.2004
Nakagawa, G., Kouzuma, A., Hirose, A., Kasai, T., Yoshida, G., and Watanabe, K. (2015). Metabolic Characteristics of a Glucose-Utilizing Shewanella Oneidensis Strain Grown under Electrode-Respiring Conditions. Plos One 10, e0138813. doi:10.1371/journal.pone.0138813
Nevin, K. P., Woodard, T. L., Franks, A. E., Summers, Z. M., Lovley, D. R., and Colwell, R. R. (2010). Microbial Electrosynthesis: Feeding Microbes Electricity to Convert Carbon Dioxide and Water to Multicarbon Extracellular Organic Compounds. MBio 1, e00103–00110. doi:10.1128/mBio.00103-10
Nouri, H., Fouladiha, H., Moghimi, H., and Marashi, S.-A. (2020). A Reconciliation of Genome-Scale Metabolic Network Model of Zymomonas Mobilis Zm4. Sci. Rep. 10, 7782. doi:10.1038/s41598-020-64721-x
Ong, W. K., Vu, T. T., Lovendahl, K. N., Llull, J. M., Serres, M. H., Romine, M. F., et al. (2014). Comparisons of Shewanella Strains Based on Genome Annotations, Modeling, and Experiments. BMC Syst. Biol. 8, 31–11. doi:10.1186/1752-0509-8-31
Orth, J. D., Thiele, I., and Palsson, B. Ø. (2010). What Is Flux Balance Analysis? Nat. Biotechnol. 28, 245–248. doi:10.1038/nbt.1614
Overbeek, R., Begley, T., Butler, R. M., Choudhuri, J. V., Chuang, H. Y., Cohoon, M., et al. (2005). The Subsystems Approach to Genome Annotation and its Use in the Project to Annotate 1000 Genomes. Nucleic Acids Res. 33, 5691–5702. doi:10.1093/nar/gki866
Overbeek, R., Olson, R., Pusch, G. D., Olsen, G. J., Davis, J. J., Disz, T., et al. (2014). The Seed and the Rapid Annotation of Microbial Genomes Using Subsystems Technology (Rast). Nucl. Acids Res. 42, D206–D214. doi:10.1093/nar/gkt1226
Park, H. I., Sanchez, D., Cho, S. K., and Yun, M. (2008). Bacterial Communities on Electron-Beam Pt-Deposited Electrodes in a Mediator-Less Microbial Fuel Cell. Environ. Sci. Technol. 42, 6243–6249. doi:10.1021/es8006468
Park, J. M., Park, H. M., Kim, W. J., Kim, H. U., Kim, T. Y., and Lee, S. Y. (2012). Flux Variability Scanning Based on Enforced Objective Flux for Identifying Gene Amplification Targets. BMC Syst. Biol. 6, 106. doi:10.1186/1752-0509-6-106
Pinchuk, G. E., Hill, E. A., Geydebrekht, O. V., De Ingeniis, J., Zhang, X., Osterman, A., et al. (2010). Constraint-Based Model of Shewanella Oneidensis Mr-1 Metabolism: A Tool for Data Analysis and Hypothesis Generation. PLoS Comput. Biol. 6, e1000822–8. doi:10.1371/journal.pcbi.1000822
Rabaey, K., and Rozendal, R. A. (2010). Microbial Electrosynthesis - Revisiting the Electrical Route for Microbial Production. Nat. Rev. Microbiol. 8, 706–716. doi:10.1038/nrmicro2422
Ranganathan, S., Suthers, P. F., and Maranas, C. D. (2010). Optforce: An Optimization Procedure for Identifying All Genetic Manipulations Leading to Targeted Overproductions. PLoS Comput. Biol. 6, e1000744. doi:10.1371/journal.pcbi.1000744
Ross, D. E., Flynn, J. M., Baron, D. B., Gralnick, J. A., and Bond, D. R. (2011). Towards Electrosynthesis in Shewanella: Energetics of Reversing the Mtr Pathway for Reductive Metabolism. Plos One 6, e16649. doi:10.1371/journal.pone.0016649
Rowe, S. F., Le Gall, G., Ainsworth, E. V., Davies, J. A., Lockwood, C. W. J., Shi, L., et al. (2017). Light-Driven H2 Evolution and C═C or C═O Bond Hydrogenation by Shewanella Oneidensis: A Versatile Strategy for Photocatalysis by Nonphotosynthetic Microorganisms. ACS Catal. 7, 7558–7566. doi:10.1021/acscatal.7b02736
Ryu, J. Y., Kim, H. U., and Lee, S. Y. (2019). Deep Learning Enables High-Quality and High-Throughput Prediction of Enzyme Commission Numbers. Proc. Natl. Acad. Sci. U.S.A. 116, 13996–14001. doi:10.1073/pnas.1821905116
Segrè, D., Vitkup, D., and Church, G. M. (2002). Analysis of Optimality in Natural and Perturbed Metabolic Networks. Proc. Natl. Acad. Sci. U.S.A. 99, 15112–15117. doi:10.1073/pnas.232349399
Sekar, R., Shin, H. D., and Dichristina, T. J. (2016). Activation of an Otherwise Silent Xylose Metabolic Pathway in Shewanella Oneidensis. Appl. Environ. Microbiol. 82, 3996–4005. doi:10.1128/aem.00881-16
Shen, J., Huang, L., Zhou, P., Quan, X., and Puma, G. L. (2017). Correlation between Circuital Current, Cu(Ii) Reduction and Cellular Electron Transfer in Eab Isolated from Cu(Ii)-Reduced Biocathodes of Microbial Fuel Cells. Bioelectrochemistry 114, 1–7. doi:10.1016/j.bioelechem.2016.11.002
Shi, L., Dong, H., Reguera, G., Beyenal, H., Lu, A., Liu, J., et al. (2016). Extracellular Electron Transfer Mechanisms between Microorganisms and Minerals. Nat. Rev. Microbiol. 14, 651–662. doi:10.1038/nrmicro.2016.93
Shirodkar, S., Reed, S., Romine, M., and Saffarini, D. (2011). The Octahaem SirA Catalyses Dissimilatory Sulfite Reduction inShewanella oneidensisMR-1. Environ. Microbiol. 13, 108–115. doi:10.1111/j.1462-2920.2010.02313.x
Song, T.-S., Wang, G., Wang, H., Huang, Q., and Xie, J. (2019). Experimental Evaluation of the Influential Factors of Acetate Production Driven by a Dc Power System via Co2 Reduction through Microbial Electrosynthesis. Bioresour. Bioprocess. 6, 29. doi:10.1186/s40643-019-0265-5
Tao, L., Xie, M., Chiew, G. G. Y., Wang, Z., Chen, W. N., and Wang, X. (2016). Improving Electron Trans-inner Membrane Movements in Microbial Electrocatalysts. Chem. Commun. 52, 6292–6295. doi:10.1039/c6cc00976j
Tefft, N. M., and Teravest, M. A. (2019). Reversing an Extracellular Electron Transfer Pathway for Electrode-Driven Acetoin Reduction. ACS Synth. Biol. 8, 1590–1600. doi:10.1021/acssynbio.8b00498
Toledo-Cuevas, M., Barquera, B., Gennis, R. B., Wikström, M., and Garcı́a-Horsman, J. A. (1998). The Cbb3-type Cytochrome C Oxidase from Rhodobacter Sphaeroides, a Proton-Pumping Heme-Copper Oxidase. Biochimica Biophysica Acta (BBA) - Bioenergetics 1365, 421–434. doi:10.1016/s0005-2728(98)00095-4
Wang, J., Wu, M., Lu, G., and Si, Y. (2016). Biotransformation and Biomethylation of Arsenic by Shewanella Oneidensis Mr-1. Chemosphere 145, 329–335. doi:10.1016/j.chemosphere.2015.11.107
Yang, C., Rodionov, D. A., Li, X., Laikova, O. N., Gelfand, M. S., Zagnitko, O. P., et al. (2006). Comparative Genomics and Experimental Characterization of N-Acetylglucosamine Utilization Pathway of Shewanella Oneidensis. J. Biol. Chem. 281, 29872–29885. doi:10.1074/jbc.m605052200
Yang, H., Krumholz, E. W., Brutinel, E. D., Palani, N. P., Sadowsky, M. J., Odlyzko, A. M., et al. (2014). Genome-Scale Metabolic Network Validation of Shewanella Oneidensis Using Transposon Insertion Frequency Analysis. PLoS Comput. Biol. 10, e1003848. doi:10.1371/journal.pcbi.1003848
Yang, Y., Ding, Y., Hu, Y., Cao, B., Rice, S. A., Kjelleberg, S., et al. (2015). Enhancing Bidirectional Electron Transfer of Shewanella Oneidensis by a Synthetic Flavin Pathway. ACS Synth. Biol. 4, 815–823. doi:10.1021/sb500331x
Yang, Z.-N., Hou, Y.-N., Zhang, B., Cheng, H.-Y., Yong, Y.-C., Liu, W.-Z., et al. (2020). Insights into Palladium Nanoparticles Produced by Shewanella Oneidensis Mr-1: Roles of Nadh Dehydrogenases and Hydrogenases. Environ. Res. 191, 110196. doi:10.1016/j.envres.2020.110196
Keywords: genome-scale metabolic model, Shewanella oneidensis MR-1, microbial fuel cells, microbial electrosynthesis, constraint-based flux analysis
Citation: Luo J, Yuan Q, Mao Y, Wei F, Zhao J, Yu W, Kong S, Guo Y, Cai J, Liao X, Wang Z and Ma H (2022) Reconstruction of a Genome-Scale Metabolic Network for Shewanella oneidensis MR-1 and Analysis of its Metabolic Potential for Bioelectrochemical Systems. Front. Bioeng. Biotechnol. 10:913077. doi: 10.3389/fbioe.2022.913077
Received: 05 April 2022; Accepted: 26 April 2022;
Published: 12 May 2022.
Edited by:
Shihui Yang, Hubei University, ChinaReviewed by:
Long Liu, Jiangnan University, ChinaChen-Guang Liu, Shanghai Jiao Tong University, China
Copyright © 2022 Luo, Yuan, Mao, Wei, Zhao, Yu, Kong, Guo, Cai, Liao, Wang and Ma. This is an open-access article distributed under the terms of the Creative Commons Attribution License (CC BY). The use, distribution or reproduction in other forums is permitted, provided the original author(s) and the copyright owner(s) are credited and that the original publication in this journal is cited, in accordance with accepted academic practice. No use, distribution or reproduction is permitted which does not comply with these terms.
*Correspondence: Zhiwen Wang, end3QHRqdS5lZHUuY24=; Hongwu Ma, bWFfaHdAdGliLmNhcy5jbg==
†These authors have contributed equally to this work