- 1Research Center for Intelligent Sensing Systems, Zhejiang Lab, Hangzhou, China
- 2Anhui Institute of Optics and Fine Mechanics, Chinese Academy of Sciences (CAS), Hefei, China
- 3Science Island Branch, Graduate School of USTC, Hefei, China
- 4Research Center for Sensing Materials and Devices, Zhejiang Lab, Hangzhou, China
The study of brain science is vital to human health. The application of hyperspectral imaging in biomedical fields has grown dramatically in recent years due to their unique optical imaging method and multidimensional information acquisition. Hyperspectral imaging technology can acquire two-dimensional spatial information and one-dimensional spectral information of biological samples simultaneously, covering the ultraviolet, visible and infrared spectral ranges with high spectral resolution, which can provide diagnostic information about the physiological, morphological and biochemical components of tissues and organs. This technology also presents finer spectral features for brain imaging studies, and further provides more auxiliary information for cerebral disease research. This paper reviews the recent advance of hyperspectral imaging in cerebral diagnosis. Firstly, the experimental setup, image acquisition and pre-processing, and analysis methods of hyperspectral technology were introduced. Secondly, the latest research progress and applications of hyperspectral imaging in brain tissue metabolism, hemodynamics, and brain cancer diagnosis in recent years were summarized briefly. Finally, the limitations of the application of hyperspectral imaging in cerebral disease diagnosis field were analyzed, and the future development direction was proposed.
1 Introduction
Brain, the most important and complex organ in human body, is the vehicle for our cognition, emotion, mobility, language, memory, consciousness and self-awareness (Salles et al., 2019). Thanks to the invention of non-invasive imaging of the human brain, our understanding of the relationship between the brain and behaviors has undergone a major shift. Nowadays, a variety of imaging modalities are employed as guidance tools during the neurosurgeries e.g., computed tomography (CT) (Paty et al., 1988; Hövels et al., 2008), magnetic resonance imaging (MRI) (Dewenter et al., 2021; Xu et al., 2021) and fluorescent tumor markers (FTM) (Ferraro et al., 2016). Nevertheless, these methods have some limitations. For instance, CT is harmful to the patient’s brain tissue because of the higher radiation (Pelizzari et al., 1989; Gong et al., 2007). MRI has poor spatial resolution, significantly prolongs the operation duration, and only a certain quantity of images are available (Ganser et al., 1997). FTM is able to identify tumor boundaries, but it can only be used for high-grade tumors due to the patient-related chain reaction. These technologies have higher probabilities to cause terrible clinical results due to incomplete excision of diseased tissue or resection of adjacent normal tissue (Torbey et al., 2015). Therefore, a label-free and contactless imaging approach is urgently demanded to assist physicians during neurosurgery (Li et al., 2013; Lu, 2014; Halicek et al., 2019a).
Hyperspectral imaging (HSI), as an optical detection technique, is aimed to record the spectrum of each pixel in an image. In this sense, HSI is a natural extension of color (RGB) imaging. HSI has the advantage of acquiring two-dimensional images over a wide range of the electromagnetic spectrum and has numerous practical applications, including Oceanic exploration (Freitas et al., 2021; Wang, 2021), food quality and safety organizations (Lohumi, 2018; Kim et al., 2022), disaster monitoring (Farhadi et al., 2022), remote sensing (Abdulridha et al., 2019), and agriculture (Lu, 2020).
Recently, as a promising optical technology, HSI is extensively utilized in the field of biomedical engineering, for life science research, non-invasive diagnostics and image-guided surgery (Lu and Fei, 2014; Ortega et al., 2019; Fei and Amigo, 2020). In the past decades, there were two primary factors that aroused the interests of medical researchers for HSI technology. First, the interaction between electromagnetic radiation and tissues contains quantitative diagnostic information on histopathology. Second, for its non-invasive nature, HSI can provide real-time information of several biological processes in healthy and diseased tissues. Specifically, HSI measures the intensity changes at multiple wavelengths, demonstrating the reflection, emission or fluorescence interactions with the target tissues, which indicate the changes in the biological structure of its components and changes in the concentration of intrinsic light-absorbing or luminescent chromophores. Researchers have demonstrated the ability of HSI to detect a wide range of diseases, such as oximetry of the retinal (Gao et al., 2012; Hadoux et al., 2019; Lim et al., 2021), intestinal ischemia identification (Barberio et al., 2020; Mehdorn et al., 2020), histopathological tissue analysis (Khouj et al., 2018), detecting cancer metastases in lung and lymph node tissue (Zhang et al., 2021), blood vessel visualization enhancement (Bjorgan et al., 2015; Fouad Aref et al., 2021), identifying skin tumors (Leon et al., 2020; Courtenay et al., 2021), evaluating the cholesterol levels (Milanic et al., 2015), diabetic foot, etc. In the field of oncology, HSI technology has been successfully applied to detect head and neck cancer (Halicek et al., 2017; Eggert et al., 2022), thyroid and salivary glands (Halicek et al., 2020), gastric cancer (Li et al., 2019; Liu et al., 2020a), oral cancer (Jeyaraj et al., 2020), colon cancer (Baltussen et al., 2019; Manni, 2020; Maktabi, 2021) as well as breast cancer (Kho et al., 2019; Aboughaleb et al., 2020). Previously, other authors have published comprehensive overviews concerning the application of HSI in gastroenterology (Ortega et al., 2019), wound care (Saiko et al., 2020) or breast cancer therapy and diagnosis (Aref et al., 2020). However, to our knowledge, the application of HSI in cerebral disease has not been systematically reviewed.
The purpose of this review is to provide an overview of the main advanced studies concerning the use of hyperspectral imaging technology in cerebral disease diagnosis. The fundamental principles of HSI techniques are presented in detail, and their latest research applications in brain tissue metabolism, hemodynamics and brain cancer diagnosis are summarized and highlighted. In addition, issues encountered in HSI techniques are included and future trends in cerebral disease diagnosis applications are also discussed in the current review. It is noteworthy that this review focus on the application of HSI Technology of the exposed cortex in cerebral surgery. The diffuse HSI Technology in cerebral disease, such as neonatal, aging, neurodegenerative, and cardiac arrest/surgery brain monitoring applications are not included.
2 Basic Knowledge of Hyperspectral Image System
HSI uses hundreds of spectral bands, providing more information about the imaging target. The fundamental theory of HSI technology is that all target materials reflect, scatter or absorb energy in different ways due to differences in chemical composition and physical structure when subjected to electromagnetic radiation sources in different wavelength ranges. Light scattering is related to the particle diameter, cell structure, tissue composition and other physical properties of the target materials, HSI while light absorption is concerned with the chemical composition of the target materials. For biomedical applications, HSI can provide an easier way to identify any abnormality in any tissue or organ in the body, allowing for better identification and treatment of disease (Carrasco et al., 2003).
2.1 Experimental Devices
The commonly used experimental devices of HSI include light sources, wavelength dispersion modules, and photoelectric detectors. Typically, HSI systems use different detectors to cover different wavelength ranges (Lu, 2014). Charge-coupled devices (CCDs) or complementary metal oxide semiconductors (CMOS) typically encompass the spectral range from 400 to 1000 nm (visible and near-infrared, VNIR), while indium gallium arsenide (InGaAs) and mercury cadmium telluride (HgCdTe) sensors are employed to cover the range of 900–1700 nm (Near Infrared, NIR), 900–2500 nm (Near Short Wave Infrared, SWIR) and 2500–25,000 nm (Middle Infrared, MIR), respectively. Furthermore, HSI systems have a variety of different methods that are differentiated by how the hypercube is generated. They are differentiated according to the type of dispersive element and how the dispersive element projects the acquired information into the detector array. The three typical categories of HSI modes are: Spectral Scan, Spatial Scan and Snapshot HSI systems (Lucas et al., 2004; Gao and Smith, 2015).
Spectral scanning HSI systems (also be called as staring HSI systems), just as its name implies, generate hypercubes in one single spectral wavelength (Figure 1A). Basically, each of the obtained images occupies a single spatial slice (x, y) of the hypercube. The hypercube can be reconstructed at each image acquisition by stacking all slices along the spectral dimension (λ). Spatial scanning HSI systems can obtain all spectral information from one spatial scanning of the imaging area in each acquisition process and typically use a spectrometer as a spectrally dispersive element that splits light into its constituent spectral bands prior to the detection by the sensor array. Two primary scanning patterns of spatial scan HSI systems are: point scan (whiskbroom) (Figure 1B) and line scan (pushbroom) (Figure 1C) HSI systems. Compared with the formers, the snapshot HSI systems can simultaneously obtain spectral and spatial information in one image, eliminating the processes of successive scans and relative displacement between the object and the system (Figure 1D) (Halicek et al., 2019a).
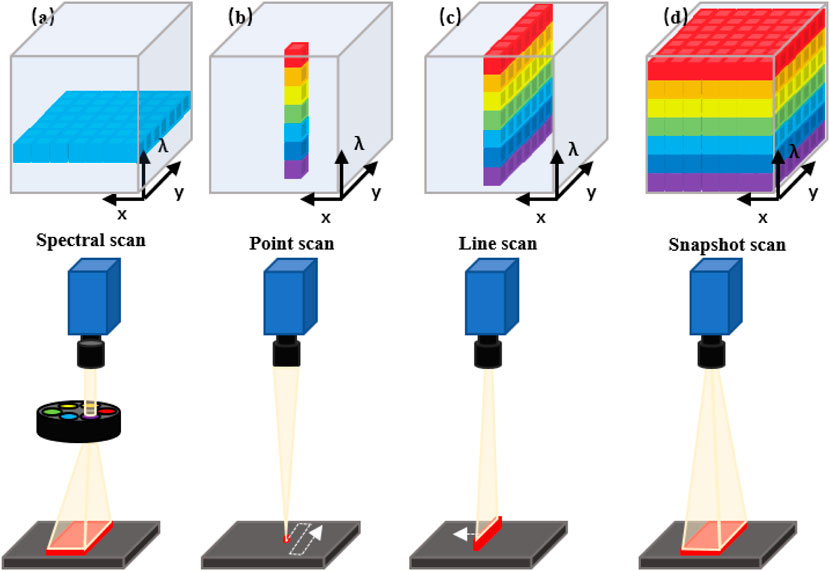
FIGURE 1. Schematic diagram of the acquisition approach of the hyperspectral data cube. (A) Spectral scanning: one wavelength band image at a time. (B) Point scanning (wiskbroom imaging): one spectrum of only one point in a single measurement. (C) Line scanning (pushbroom imaging): spectra of points on the same line in a single measurement. (D) Snapshot: covering the full spectrum in a single measurement.
HSI systems integrate the advantages of imaging systems and spectroscopic instruments to provide spectral data with spatial resolution. HSI instruments can acquire hundreds or thousands of spectra in an x × y × λ data cube (Figure 2A), where x and y represent the spatial dimensions and λ represents the spectral dimension (Sawyer et al., 2017). Figure 2B shows the HSI acquisition system used in cerebral diagnosis. HSI measures the optical properties of brain tissue in broadband electromagnetic wavelengths range. The light interactions (scattering of photons) of brain tissues are captured to generate spectral images of narrow spectral bands (usually 100 or more images). Each image records the relative light absorption or reflectance of one wavelength band, and reveals the biological properties in brain, e.g., chromophores or tissue oxygenation. All these images are assembled into a discrete 3D volume element with two spatial dimensions and one spectral dimension to form a hyperspectral cube (also known as hyperspectral image or hypercube). Figure 2C presents the images at different wavelengths obtained from the brain HSI data cubes. The three-dimensional data cube (hypercubes) are acquired from hyperspectral images that consist of hundreds of images of the same object in different spectral bands. The spectrum of each pixel and image of each slice can present the composition of a specific position, and the spatial and surface feature information (Fabelo et al., 2019a; Manni et al., 2020). Spectral feature information of several brain tumor tissues in the VNIR range at the pixel of regions of interest (ROI) are shown in Figure 2D. The red, green, and blue line represent the spectral characteristics of tumor tissue, normal tissues, and blood vessels.
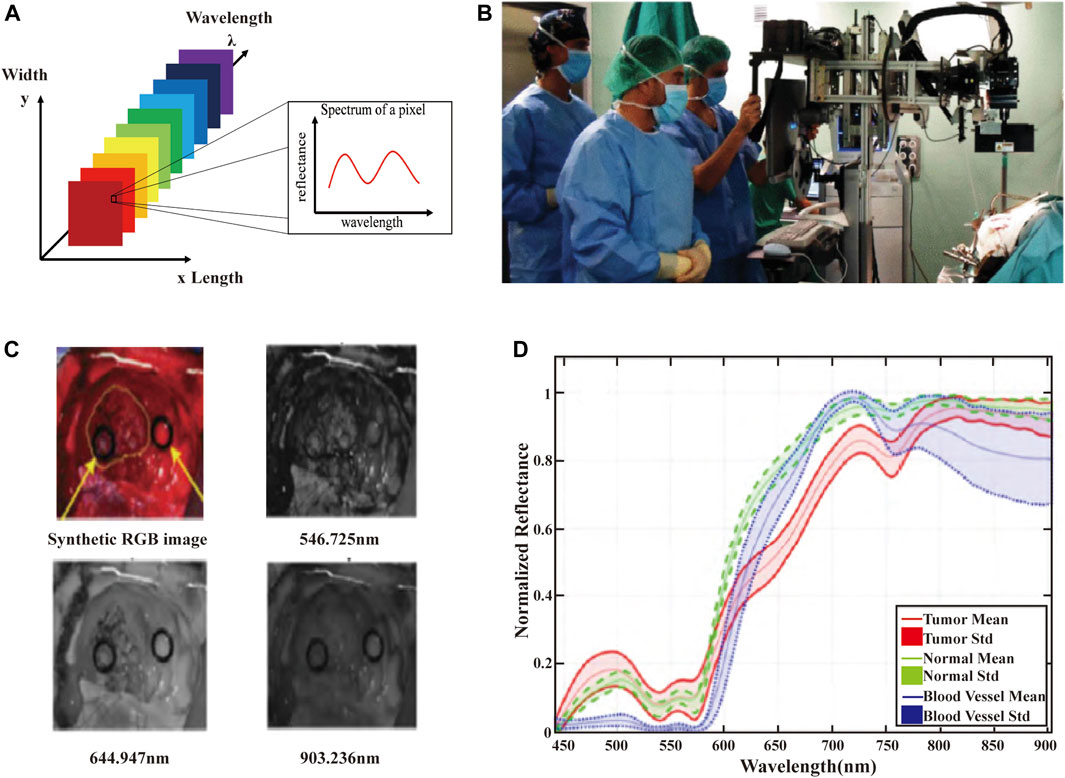
FIGURE 2. (A) The schematic of the HSI data cube. The data measured in the HSI is presented by means of data cubes. Each slice of the data cube includes an image of the scene at a specific wavelength. Each pixel is associated with a spectral response vector, also known as a spectral feature. (B) HSI acquisition system used in cerebral diagnosis applications. (C) Images at different wavelengths obtained from the brain HSI data cubes. (D) Spectral feature information of several brain tumor tissues in the VNIR range at the pixel of ROI (Fabelo et al., 2019a).
2.2 Hyperspectral Image Processing Methods
The obtained hyperspectral images commonly include many unexpected noise (e.g., brightness non-uniformity, pixel abnormality, redundant regions, data redundancy, etc.) due to the operating conditions of the equipment, research environment, specimen preparation, and other reasons. All these unwanted noises may introduce incorrect and irrelevant signals, affecting the subsequent processing of data. To reduce these variations and extract useful information from hyperspectral images, preprocessing methods are usually used. The workflow of hyperspectral images includes image acquisition, calibration, spectral or spatial pre-processing, downscaling and target-specific detection. Data pre-processing is located at the top of the hyperspectral image processing process and has a direct and important impact on the quality of further analysis. The main purpose of hyperspectral image preprocessing is to compensate for non-uniform illumination and suppress the effects of noisy image elements, extraneous regions and redundant information as much as possible, which can obtain pure images, non-mixed spectral signals and improve the efficiency of subsequent data processing. In the image preprocessing stage, techniques such as image segmentation (ROI selection by masking image regions), noise reduction, image smoothing, flattening, baseline correction, normalization, and image data compression are used. Since hyperspectral images involve both spectral and spatial information, other approaches in the field of spectroscopy analysis and image processing are also suitable for processing hyperspectral data.
More detailed information of the captured scene is obtained in the large amount of data. However, a large increase of the computing power is required to decouple the data with redundant information (Ghamisi et al., 2017). Therefore, it is necessary to employ processing algorithms that can reduce the dimensionality of HS data without losing relevant information. This dimensionality reduction process involves transforming data with high-dimensional features into a significant representation of the data in dimensionality reduction (Audebert et al., 2019). There are two primary dimensionality reduction methods: feature extraction and feature selection (Lu, 2014). Feature extraction algorithms can scale, rotate and reduce the original feature space of HS data utilizing transformation matrices. Common feature extraction methods include principal component analysis (PCA), partial least squares regression analysis, Kernel PCA, linear discriminant analysis, independent component analysis and local linear embedding, all of which retain the information required in practical application (Lv and Wang, 2020). Moreover, it is necessary to choose the most discriminative bands in order to reduce the dimensionality of the data. Optimization algorithms is the most common feature selection algorithms, including genetic algorithm (Mirjalili, 2019), particle swarm optimization (Liu et al., 2021) and ant colony optimization (Sharma and Buddhiraju, 2018).
2.3 Hyperspectral Image Analysis Methods
The hyperspectral data cube comprises of a wealth of diagnostic information obtained at the tissue, cellular and molecular levels. All spectral and spatial information in the hyperspectral data cube have important implications for disease screening, clinical diagnosis, and subsequent therapies. The hyperspectral datasets employ advanced image classification technologies to extract, decompose and classify entire spectral information from the acquired data. The purpose of the approach is to associate these molecular features with established disease states by resolving a mixture of spectral and spatial information into intrinsic molecular components expressions. The main classification methods used for hyperspectral imaging are supervised learning and unsupervised learning (Nathan et al., 2018). The classification methods (supervised learning) can be divided into traditional and deep learning methods. Typical methods include Support vector machines (SVMs) (Yi et al., 2014; Manni et al., 2019), Random Forest (Laffers et al., 2016), and K-nearest-neighbor (KNN) (Lu et al., 2016). SVMs is a powerful deep learning technique, the most prominently applied in hyperspectral image data classification and relying on statistical learning theory that separates the linearly separable feature space with maximum margin into classes. In cerebral HSI, SVMs have been already used to identify and classify different types of brain cancers (Fabelo et al., 2018a). SVMs provide good performance for classification of such data when the available number of training samples is limited.
More recently, most tumor recognition models based on HSI technology employ traditional machine learning algorithms. The performance of these tumor detection approaches depends on manually extracting features that are time-consuming, strenuous, and easily influenced by subjective factors. With the rise of deep learning technologies driven by factors such as data mining algorithm and high-performance computing, research on HS images analysis and classification based on deep learning is underway. Using deep learning models can realize autonomous learning of deep, abstract and semantic information from various data. Deep learning utilizes computational models to learn multi-level representations of data through simple combinations rather than non-linear modules, each of which translates the same level of information into a better, more abstract transformation. More deep learning concepts that are relevant and applicable to medical HS images analysis are reviewed in Ref. (Khan et al., 2021). The emergence of deep learning has given rise to more advanced feature extraction techniques by combining spatial and spectral information. Especially in recent years, HSI has begun to achieve promising results in brain disease diagnosis by utilizing cutting-edge deep learning algorithms, such as artificial neural networks (ANNs) (Jolivot et al., 2011), Spectral information dispersion (Guan et al., 2012), and Spectral angle mapping (SAM) (Martin et al., 2012).
Numerous traditional ANN-based algorithms have been used to solve classification and regression problems in the field of biomedical applications. Nevertheless, they perform poorly on independent test data because of overfitting for the plenty of parameters available in HS images. Convolutional Neural Networks (CNN), a very prevalent deep learning algorithm for classifying input images to distinguish objects by identifying patterns and features, has emerged as an effective technique of deep learning for image analysis assignments. They are currently the preferred method for image classification because they exploit spatial features efficiently by executing local patterns in HSI images. Additionally, CNNs are able to capture the correlations between the spectra of a given pixel, exploiting its robustness to training sample variance and to extract features from a large amount of training data, showing great performance in image classification (Halicek et al., 2019b; Halicek et al., 2019c).
In hyperspectral imaging of cerebral experiments, Leave-One-Patient-Out Cross-Validation (LOPOCV) was employed to avoid the double usage of the same patient and three metrics are analyzed, such as the overall accuracy, sensitivity and specificity. Mathematically, sensitivity and specificity are defined as follows:
Where TP is the number of true positives, FN is the number of false negatives, TN is the number of true negatives and FP is the number of false positives. In addition, the receiver operating characteristic (ROC) curve was calculated and the area under the curve (AUC) metric was provided for each class in the results.
These algorithms inevitably face two main challenges when applied to brain HSI data: limited number of samples and high dimensionality. It is not necessary in other areas of application, but is more prevalent in cerebral HSI due to the variations of spectral features between patients.
3 Hyperspectral Imaging in Cerebral Diagnosis
HSI is an emerging technology, of which the application in the biomedical field is still in its early stages. Therefore, there are limited publications on the application of HSI technique in cerebrology. This section summarizes several advanced researches works in this field, which are categorized according to the taxonomy shown in Figure 3. This taxonomy classifies cerebral HSI applications according to the target of application.
3.1 Brain Tissue Metabolic and Hemodynamic
Recent biomedical applications of HSI have demonstrated the enormous potential to retrieve in vivo hemodynamic and metabolic signals from animal or human brains. HSI provides real-time quantitative information on the biological processes in healthy or diseased brain tissues. This is achieved by measuring the intensity change at multiple wavelengths after reflection, emission, or fluorescence interacts with tissue (Jacques, 2013), which reflecting the changes in optical properties (mainly scattering and absorption) of the target tissue in the brain, and indicating the changes in the biological structure of its constituents and changes in the concentration of chromophores that inherently absorb or emit light (Wang, 2012). In this section, we mainly focus on the research advances of HSI in brain tissue metabolic and hemodynamic.
3.1.1 Monitoring Brain Oxygenation and Hemodynamic
Functional metabolic activity of the human brain is inextricably linked to all biochemical processes associated with normal brain function (Cunnane et al., 2011). Studies have shown a strong relationship between the oxygenation of human brain tissue and its metabolic activation (Malonek and Grinvald, 1996; Devor et al., 2003). Near-infrared HSI is one of the major methods that have been used to achieve brain oxygenation and hemodynamics monitoring (Nguyen et al., 2019). Nosrati, et al. employed near-infrared HSI to measure the concentration changes of oxyhemoglobin (HBO2) and deoxyhaemoglobin (HHb), along with the oxidation state of mitochondrial cytochrome c-oxidase (CCO) in the prefrontal cortex on the left and right sides of the human brain simultaneously, which aimed to study the brain metabolic activity of 16 healthy right-handed participants during the simulated driving (aged between 22 and 32) (Nosrati et al., 2016). The results present the absorption spectra of four different tissue chromophores. This data presents the spectral characteristics of HBO2, HHb, CCO, and H2O in the spectral range 715–900 nm. It is observed that the absorption maxima of HHb and HBO2 are located around 760 and 870 nm, respectively. Calculation of concentration changes based on measured spectral features can be applied to the assessment of cerebral oxygen metabolism related events. Giannoni, et al. using Monte Carlo (MC) simulations to demonstrate the applicability of HSI for mapping and quantifying changes in HBO2, HHb, and oxCCO concentrations in exposed cerebral cortex under hypoxia (Giannoni et al., 2020). Soon after, the same research group firstly present a novel HSI system for imaging and monitoring of HbO2, HHb and CCO in exposed mouse cerebral cortex under different oxygenation state environments (hyperoxia, hypoxia and anoxia) (Giannoni et al., 2021). In this work, the same 8 bands of NIR spectral and 3 bands of visible wavelengths are combined to enhance the image contrast of hemoglobin and oxCCO. Modification and optimization of the HSI system to increase the temporal resolution to the sub-second level enables a more in-depth study of brain function, including brain function activation and neurovascular coupling, etc.
Detecting and measuring brain metabolic signals by imaging allows the identification and localization of changes in brain activity or function under several circumstances, for example, the resting state, through functional activation or stimuli, abnormal physiological environments (e.g., tissue deficiencies oxygen, hyperoxia, and even acute ischemia). These conditions interrupt regular metabolism in a noxious way (e.g., hypoxia and ischemia), and cause damages to brain tissues. Targeting the exposed small animal cortex using HSI is also a preferred option in several studies that evaluating hemodynamic responses and brain tissue oxygenation during induction of hypoxia and hyperoxia (Shonat et al., 1997; Yin et al., 2013; Nishidate et al., 2017; Fu et al., 2020). Shonat, et al. were the first to use HSI to study hemodynamic changes in exposed mouse cortex (Shonat et al., 1997). Mori, et al. reported a study on assessing cortical hemodynamics in rats and humans using HSI system (Mori et al., 2014). In this study, the authors capture continuous spectral data (HS data) of the brain cortical surface in the visible wavelength band and convert these data into optical intrinsic signals to show the measure results of brain surface oxygen saturation. Then, based on previous research, Iwaki et al. successfully demonstrated a new method for predicting cerebral hyperperfusion syndrome (CHS) after bypass surgery for moyamoya (MMD) disease using a hyperspectral imaging system (Iwaki et al., 2021). The authors performed hyperspectral imaging of the cerebral cortex before and after anastomosis in 29 patients with MMD who underwent superficial temporal artery (STA)-middle cerebral artery (MCA) after surgery, and analyzed the changes in oxygen saturation after anastomosis to evaluate its correlation with CHS. Compared with non-CHS patients, CHS patients had significantly higher cerebral cortical oxygen saturation (SO2) after anastomosis (33 ± 28 vs. 8 ± 14%, p < 0.0001). Therefore, HSI may help pre-identify patients at high risk for postoperative CHS before the onset. This early prediction facilitates early intervention to prevent or reduce irreversible brain disease caused by CHS.
3.1.2 Surgical Assistance
The brain tissue hemodynamics imaging via HSI during neural activation is not limited to small animals. Sorts of attempts applied hyperspectral methods to the imaging of exposed human cortex (Klaessens et al., 2011). Currently, many research groups have been devoted to the development of HSI based tools to visualize the hemodynamics during surgeries. Pichette et al. proposed an optical imaging system using snapshot hyperspectral to visualize the hemodynamic behaviours of the brain (Pichette et al., 2016). Firstly, the method has been validated in simulated tissue models to quantify the relative concentrations of up to three absorbing dyes in a mixture with guaranteed accuracy of <10%. Subsequently, the authors applied the tissue model to practical disease treatment with a volunteer of 35-year-old female patient who underwent epileptogenic tissue resection. The HS images were obtained in vivo using a hyperspectral HSI Snapshot imaging system covering the spectral range from 481 to 632 nm. This work shows the intra-operative concentration changes of oxyhemoglobin (HbO), deoxyhemoglobin (HbR), and total hemoglobin (HbT)for a single time frame. A reconstructed RGB image for the surface of the brain, realizing visualization of the blood vessels. It also presents the intraoperative concentration changes of HbO, HbR and HbT in a single time period. The relative concentrations in the red region increase while the relative concentrations in the blue region decrease. The results demonstrated that the relative changes in HbO, HbR, and HbT may be aroused through the combined effect of vasomotion, Meyer waves, and response to epileptic spikes.
Several studies monitored brain activities by quantifying changes in oxygen-hemoglobin (ΔCHbO2), deoxygenated hemoglobin (ΔCHb) and oxidative state of cytochrome-c-oxidase (ΔCoxCCO) concentrations in the cerebral cortex (Bale et al., 2016; Caredda et al., 2020a; Caredda et al., 2020b). Caredda, et al. proposed a method to identify the optimal spectral bands using commercial cameras and assessed brain functional areas and cellular metabolic energy by quantitatively modelling and measuring changes in ΔCHbO2, ΔCHb, and ΔCoxCCO through video acquisition during neurosurgery (Caredda et al., 2020a; Caredda et al., 2020b).
This section aims to provide a review of the literatures on HSI based cerebral tissue hemodynamics and metabolism research, which has an important implication for a deeper and broader understanding of the physiologies of brain tissues, to precisely record and map brain activities following neuronal activations.
3.2 Brain Cancer Diagnosis
HSI has been proved to be useful in brain disease detection and diagnosis, especially brain cancer. Brain tumors can be classified in terms of their histological and molecular specificity parameters (Louis et al., 2016). Malignant gliomas have become the predominant form of brain tumors in adults, responsible for 2–3% of cancer deaths all over the world (Villa et al., 2018). In addition to methods such as radiotherapy and chemotherapy, surgery has become the best option for treating brain tumors (Kim et al., 2019). However, as brain tumors permeate and spread to the surrounding normal brain tissue, it is difficult for the surgeon’s naked eye to accurately identify tumor tissue from normal brain tissue. Meanwhile, many studies have illustrated the residual tumor tissue to be the main reason for morbidity and mortality during surgeries. Therefore, as a label-free and contactless imaging modality, HSI is a potential tool for tissue boundary identification and classification in brain cancer surgery.
3.2.1 Identification of Tumour Tissue
It is extremely important to identify tumor borders and tumor infiltration into normal brain tissue in order to prevent the removal of excessive normal brain tissue and incomplete resection of residual tumor tissue during neurosurgery. The European project HELICoiD was launched to use HSI technology to identify normal and abnormal brain tissue during neurosurgery (Szolna et al., 2016; Salvador et al., 2017). Fabelo, et al. presented the first image database of in vivo HS human brain generated in the HELICoiD (Hyperspectral Imaging Cancer Detection) project (; Fabelo et al., 2019a; Fabelo et al., 2019b; Fabelo et al., 2019c). Ortega, et al. used HSI data to automatically detect and identify pathological sections of human brain tumor tissue taken from 10 different patients with confirmed high-grade glioma (Ortega et al., 2018). The authors employed a custom-built microscopic HS acquisition system that enables clear acquisition of HS images of pathological sections in the VNIR range (400–1000 nm). Thirty-six HS cubes were obtained from these collected pathological section samples, and over 665,000 spectral characteristics of tumor tissue and normal brain tissue from human were labeled. Nevertheless, the large amount of HSI data often contains redundant or irrelevant information. In order to improve the prediction accuracy and reduce the execution time of the classification algorithm, it is central to confirm the most relevant wavelengths for a specific application. Martinez, et al. proposed an optimization algorithm based method to identify the relevant wavelengths through obtaining the correlation spectra of the visible and near-infrared regions to establish the SVM model (Martinez et al., 2019). In this study, the in vivo human brain cancer database was derived from 26 HS images of 6 adult patients. To determine the minimum number of sampling wavelengths in HS images, the authors evaluated different band selection algorithms using a supervised classifier. Using the 48 selected bands gives better quantitative and qualitative results than using the full band in some cases.
Processing hyperspectral images in vivo is difficult for the high-dimensional nature of the HS data, of which the real-time processing is a challenge. Ravì, et al. introduced a new dimensionality reduction scheme (named Fixed Reference T-distributed Stochastic Neighbours, FR-t-SNE) and a novel processing pipeline to acquire detailed tumor classification maps for margin determination in brain surgery (Ravi et al., 2017). In this work, an extension of the FR-t-SNE method was used to decrease the data dimensionality of the HS database and the embedded results were semantically segmented using the Semantic Text Forest (STF) method to achieve brain tumor tissue classification.
Deep learning-based HSI data analysis showed a broad prospect in the field of intelligent assisted diagnosis. Fabelo, et al. proposed a deep learning-based framework to identify human brain tumors, and achieved an overall accuracy of 80% using multi-class classification on a dataset of 26 in vivo HSI samples (Fabelo et al., 2019a). Manni, et al. employed a 3D-2D hybrid convolutional neural network for the extraction of spectral-spatial features to classify brain tissues. Based on this network, tumor, normal tissue and blood vessels in the human brain were effectively distinguished. Compared to traditional feature extraction methods, the deep learning-based methods transform informative features from raw images utilizing hierarchical structures. The 3D–2D hybrid CNN achieves the best results with a mean accuracy of 80%, sensitivity of 76, 68, 74, 96%, specificity of 87, 98, 92, 87%, and AUC of 78, 70, 84, 91%, for normal, tumor, blood vessels and background, respectively (Manni et al., 2020). Furthermore, Hao, et al. reported a multiple deep model fusion (include three neural networks) based extraction method to achieve an overall accuracy of 96.34% for the identification of GBM tumors (Hao et al., 2021). This method employed 1-D deep neural network (1D-DNN) and 2-D convolution neural network (2D-CNN) to extract spectral characteristics and spectral spatial characteristic for the HSI classification of human brain. The authors also utilized edge-preserving filters to fuse and optimize spectral and spectral-spatial classification results and used fully convolutional network (FCN) to segment the background from images. The experimental data showed that the method has good classification performance.
3.2.2 Classification of Critical Tissue
It is also important to classify the critical parts of the brain tissue in real time during neurosurgery or experiments. Fu, et al. provided a method using HSI to demonstrate the possibility of differentiate between infarcted and normal brain tissue (Fu et al., 2020). The authors adopted the specific value of spectral reflectance at 545 and 560 nm (R545/R560) to recognize the spectral features of normal rat tissues and tissues with different levels of ischemia, which evaluated the utility value of the rat ischemic stroke model. The results illustrated that hyperspectral images processed with the ratio of R545 and R560 could not only recognize early cerebral ischemia within 1 h, but also accurately display ischemic regions. Fabelo, et al. used a semi-automatic approach based on a SAM algorithm to define 4 distinct classes, which included normal tissue, tumor tissue, blood vessels, and background (Fabelo et al., 2019b). Then, Urbanos, et al. used three different algorithms to classify brain tissue in vivo from 13 patients with high-grade gliomas, including support vector machines (SVM), random forests (RF), and convolutional neural networks (Urbanos et al., 2021). In this work, the authors evaluated three classification algorithms with an HSI snapshot camera with limited wavelength bands and distinguished five different brain tissue types (tumor, arterial blood vessel, venous blood vessel, dura mater and healthy tissue). According to the different training conditions, the overall accuracy results obtained from the experiments ranged from 60 to 95%.
In order to facilitate the operation of the surgeon during tumor resection, it is essential to develop a real-time classification system that can delineate the boundaries of the cancer with high accuracy. Fabelo, et al. proposed a novel algorithm of brain cancer detection to help neurosurgeons to classify brain tumor tissues, which consisted of a hybrid framework that combined both supervised and unsupervised machine learning methods (Fabelo et al., 2018b). The authors interfaced the calculator with a hardware gas pedal, allowing the system to provide neurosurgeons with a categorized map of scenes obtained in approximately 1 min during surgery. These preliminary results extracted from the supervised classification of pre-labeled tissues by experts showed that normal tissue, tumor tissue, blood vessels and background could be accurately distinguished with an overall accuracy higher than 99%. Due to the high-dimensional nature of the data acquired with the HS camera and the need to perform intraoperative surgical guidance tools in real time, a highly parallel high-performance processing platform must be used to process the HS data. Among the various available parallel technologies, the Graphics Processing Unit (GPU) is the most attractive solution, which can execute complex, intrinsically parallel algorithms on large amounts of data. Florimbi, et al. employed GPU technology to classify the largest (worst-case) image in the database in less than 3 s, which satisfied the surgery limitation of real-time setting within 1 min, and became a potential method for hyperspectral video processing in the immediate future (Florimbi et al., 2020).
Although machine learning methods and deep learning method are used extensively in brain cancer diagnosis, the integrity of data information and the timeliness of data processing are still the shortcomings of these methods (Barberio et al., 2021). More recently, several research groups are focusing on hybrid deep learning method for clinical diagnosis of brain cancer (Rinesh et al., 2022).
3.3 Hyperspectral Imaging Application Summary
To sum up, we summarized the most relevant works in the field of cerebral diagnosis using HSI in Table 1. This table is organized as follows: 1) the type of application; 2) the type of subjects involved in each study; 3) the type of sample involved in each study; 4) the spectral range of HSI technology; 5) the data processing and analysis methods or algorithms employed.
4 Discussion and Outlook
Based upon the above studies, it has been demonstrated that hyperspectral imaging has great potential for applications in cerebral diagnosis, for the excellent sensitive of the spectral signal to the biophysical and biochemical characteristics of cell and tissue samples.
Compared with traditional imaging methods, the outstanding advantage of HSI lies in the simultaneous generation of tissue structure information and hemodynamic parameters, which cannot be achieved by conventional technologies (e.g., CT or MRI). Furthermore, HSI is a real-time online, non-invasive, non-ionizing imaging technique that solves the sampling and time-consuming issues associated with hematoxylin and eosin (H&E) and 2,3,4-triphenyltetrazolium chloride (TTC) staining. Moreover, compared to common RGB images, HS images have more spectral channels and higher spectral resolution, which may contain more useful information about the surgeon’s tissue physiology and pathophysiolog. In brain tissue oxygenation and hemodynamics, HSI techniques have superior spectral resolution in imaging light-absorbing chromophores, for example, HbO2, HHb and oxCCO. Although small animals remain a prime target for metabolic activity monitoring, the study of humans in neurosurgery is becoming more common and can provide important insights into the effects of different stimuli on the human brain.
In summary, these related literatures present promising results for many kinds of cerebral diagnosis applications according to biomedical HSI and for surgical guidance. However, there are still some limitations in technology insufficiency and small datasets. Pilot studies encouraged further research of all organ systems to determine the role of HSI in clinical. For the advantages of contactless, non-invasive, label-free, and non-ionizing, HSI is a promising imaging method with great potential. However, the reliability, reproducibility, and generalizability should be further validated before the extensive usage in biomedical applications.
Although many studies have demonstrated its potential in cerebral diseases, these areas still suffer from variability in hardware and software, short of comparison or unified standard evaluation system, and lack of sufficiently large sample sizes. We can improve from the following aspects: 1) The limitation of imaging depth. Combining other techniques such as photoacoustic imaging systems to improve imaging depth remains to be studied (Liu et al., 2020b). 2) Performance improvement of HSI systems. In fact, the available hyperspectral imaging systems are still relatively bulky, and are not suitable for minimally invasive surgery. The continuous improvement of HSI systems in imaging, spatial resolution, spectral range, and resolution will enable portable and smaller HSI devices, and facilitate widespread medical applications in cerebral diagnosis. In addition, scholars have also begun to use multiple HS cameras to acquire more images in the broad spectral band, with the aim of analyzing different characteristics of different types of normal brain and tumor tissue in vivo (Leon et al., 2021a, Leon et al., 2021b). 3) Real-time processing of HSI video. HSI techniques are combined with deep learning processes to establish models for clinical diagnosis and surgical treatment of brain disorders. However, processing the huge amount of data captured by HSI sensors and improving the efficiency of data processing are the main issues for realizing real-time HSI video processing in the future (Sancho et al., 2021). 4) Formation of a multimodal imaging system. Fluorescence spectral imaging and Raman spectral imaging methods also have been introduced by researchers for different kinds of biological imaging (Sun et al., 2021; Wang et al., 2021). Furthermore, hyperspectral imaging combined with other biomedical imaging modalities to form a multimodal imaging system which can completely explain and predict the behavior of brain tissue or brain cells. 5) Establishment unified diagnostic standard. By establishing a database of characteristic spectra of various parts of the brain tissue and different brain diseases, online sharing is realized, and a unified diagnostic standard is continuously formed. Finally, this review systematically revealed the application of HSI method on the exposed cortex in cerebral surgery. However, the HSI Technology covers also the diffuse imaging of the brain with wide applications, including neonatal, aging, neurodegenerative, and cardiac arrest/surgery brain monitoring, which would not be discussed here. In conclusion, biomedical HSI remains an explosively expanding field, and new-type technological advances may lead to new discoveries and enhanced understanding of the mechanisms underlying brain function.
Author Contributions
This paper was written by YW and HD provided guidance of the structural arrangement for this paper. All revisions were discussed by WY, ZN, and ZX. All authors read and approved the final manuscript.
Funding
The work is supported in part by Center-initiated Research Project of Zhejiang Lab (Grant No. 2021MH0AL01), Postdoctoral research project of Zhejiang Lab (Grant No. 113009-UA2007QJ), Startup Grant of Zhejiang Lab (Grant No.113010-PI2108), Zhejiang Provincial Natural Science Foundation of China (No. LQ21F010003), and China Postdoctoral Science Foundation (No. 2020M681952).
Conflict of Interest
The authors declare that the research was conducted in the absence of any commercial or financial relationships that could be construed as a potential conflict of interest.
Publisher’s Note
All claims expressed in this article are solely those of the authors and do not necessarily represent those of their affiliated organizations, or those of the publisher, the editors and the reviewers. Any product that may be evaluated in this article, or claim that may be made by its manufacturer, is not guaranteed or endorsed by the publisher.
Acknowledgments
This is a short text to acknowledge the contributions of specific colleagues, institutions, or agencies that aided the efforts of the authors.
References
Abdulridha, J., Batuman, O., and Ampatzidis, Y. (2019). UAV-based Remote Sensing Technique to Detect Citrus Canker Disease Utilizing Hyperspectral Imaging and Machine Learning. Remote Sens. 11 (11), 1373. doi:10.3390/rs11111373
Aboughaleb, I. H., Aref, M. H., and El-Sharkawy, Y. H. (2020). Hyperspectral Imaging for Diagnosis and Detection of Ex-Vivo Breast Cancer. Photodiagnosis and Photodyn. Ther. 31, 101922. doi:10.1016/j.pdpdt.2020.101922
Aref, M., Aboughaleb, I. H., Youssef, A.-B., and El-Sharkawy, Y. (2020). Novel Approach Exploiting the Hyperspectral Imaging System for Breast Cancer Therapy and Diagnosis. Arch. Breast Cancer 7 (4), 189–201. doi:10.32768/abc.202074189-201
Audebert, N., Le Saux, B., and Lefevre, S. (2019). Deep Learning for Classification of Hyperspectral Data: A Comparative Review. IEEE Geosci. Remote Sens. Mag. 7 (2), 159–173. doi:10.1109/mgrs.2019.2912563
Baig, N., Fabelo, H., Ortega, S., Callico, G. M., Alirezaie, J., and Umapathy, K. (2021). Empirical Mode Decomposition Based Hyperspectral Data Analysis for Brain Tumor Classification. 43rd Annual International Conference of the IEEE Engineering in Medicine & Biology Society.
Bale, G., Elwell, C. E., and Tachtsidis, I. (2016). From Jöbsis to the Present Day: a Review of Clinical Near-Infrared Spectroscopy Measurements of Cerebral Cytochrome-C-Oxidase. J. Biomed. Opt. 21 (9), 091307. doi:10.1117/1.jbo.21.9.091307
Baltussen, E. J. M., Kok, E. N. D., Brouwer de Koning, S. G., Sanders, J., Aalbers, A. G. J., Kok, N. F. M., et al. (2019). Hyperspectral Imaging for Tissue Classification, a Way toward Smart Laparoscopic Colorectal Surgery. J. Biomed. Opt. 24 (1), 1–9. doi:10.1117/1.JBO.24.1.016002
Barberio, M., Benedicenti, S., Pizzicannella, M., Felli, E., Collins, T., Jansen-Winkeln, B., et al. (2021). Intraoperative Guidance Using Hyperspectral Imaging: A Review for Surgeons. Diagnostics 11 (11), 2066. doi:10.3390/diagnostics11112066
Barberio, M., Felli, E., Seyller, E., Longo, F., Chand, M., Gockel, I., et al. (2020). Quantitative Fluorescence Angiography versus Hyperspectral Imaging to Assess Bowel Ischemia: A Comparative Study in Enhanced Reality. Surgery 168 (1), 178–184. doi:10.1016/j.surg.2020.02.008
Bjorgan, A., Denstedt, M., Milanic, M., Paluchowski, L. A., and Randeberg, L. L. (2015). Vessel Contrast Enhancement in Hyperspectral Images, 9318. United states: SPIE BiOSSPIE.
Bravo, J. J., Olson, J. D., Davis, S. C., Roberts, D. W., Paulsen, K. D., and Kanick, S. C. (2017). Hyperspectral Data Processing Improves PpIX Contrast during Fluorescence Guided Surgery of Human Brain Tumors. Sci. Rep. 7 (1), 1–13. doi:10.1038/s41598-017-09727-8
Caredda, C., Mahieu-Williame, L., Sablong, R., Sdika, M., Guyotat, J., and Montcel, B. (2020). Intraoperative Functional and Metabolic Brain Mapping Using Hyperspectral Imaging, 11225. US: SPIE BiOS.
Caredda, C., Mahieu-Williame, L., Sablong, R., Sdika, M., Guyotat, J., and Montcel, B. (2020). Optimal Spectral Combination of a Hyperspectral Camera for Intraoperative Hemodynamic and Metabolic Brain Mapping. Appl. Sci. 10 (15), 5158. doi:10.3390/app10155158
Carrasco, O., Gomez, R. B., Chainani, A., and Roper, W. E. (2003). “Hyperspectral Imaging Applied to Medical Diagnoses and Food Safety,” in Geo-Spatial and Temporal Image and Data Exploitation III (US: SPIE). doi:10.1117/12.502589
Courtenay, L. A., González-Aguilera, D., Lagüela, S., del Pozo, S., Ruiz-Mendez, C., Barbero-García, I., et al. (2021). Hyperspectral Imaging and Robust Statistics in Non-melanoma Skin Cancer Analysis. Biomed. Opt. Express 12 (8), 5107–5127. doi:10.1364/boe.428143
Cruz-Guerrero, I. A., Leon, R., Campos-Delgado, D. U., Ortega, S., Fabelo, H., and Callico, G. M. (2020). Classification of Hyperspectral In Vivo Brain Tissue Based on Linear Unmixing. Appl. Sci. 10 (16), 5686. doi:10.3390/app10165686
Cunnane, S., Nugent, S., Roy, M., Courchesne-Loyer, A., Croteau, E., Tremblay, S., et al. (2011). Brain Fuel Metabolism, Aging, and Alzheimer's Disease. Nutrition 27 (1), 3–20. doi:10.1016/j.nut.2010.07.021
Devor, A., Dunn, A. K., Andermann, M. L., Ulbert, I., Boas, D. A., and Dale, A. M. (2003). Coupling of Total Hemoglobin Concentration, Oxygenation, and Neural Activity in Rat Somatosensory Cortex. Neuron 39 (2), 353–359. doi:10.1016/s0896-6273(03)00403-3
Dewenter, A., Gesierich, B., Telgte, A. T., Wiegertjes, K., Cai, M., Jacob, M. A., et al. (2021). Systematic Validation of Structural Brain Networks in Cerebral Small Vessel Disease. J. Cereb. Blood Flow Metabolism 41 (11), 1–13. doi:10.1177/0271678x211069228
Eggert, D., Bengs, M., Westermann, S., Gessert, N., Gerstner, A. O. H., Mueller, N. A., et al. (2022). In Vivo detection of Head and Neck Tumors by Hyperspectral Imaging Combined with Deep Learning Methods. J. Biophot. 15, e202100167. doi:10.1002/jbio.202100167
Fabelo, H., Halicek, M., Oetega, S., Szolna, A., Morera, J., and Sarmiento, R., Surgical Aid Visualization System for Glioblastoma Tumor Identification Based on Deep Learning and In-Vivo Hyperspectral Images of Human Patients. Proc. SPIE Int. Soc. Opt. Eng., 2019. 10951: 1095110. SPIE.doi:10.1117/12.2512569
Fabelo, H., Halicek, M., Ortega, S., Szolna, A., Morera, J., Sarmiento, R., et al. (2019). Surgical Aid Visualization System for Glioblastoma Tumor Identification Based on Deep Learning and In-Vivo Hyperspectral Images of Human Patients. Proc. SPIE Int. Soc. Opt. Eng. 10951, 1095110. doi:10.1117/12.2512569
Fabelo, H., Halicek, M., Ortega, S., Shahedi, M., Szolna, A., Piñeiro, J., et al. (2019). Deep Learning-Based Framework for In Vivo Identification of Glioblastoma Tumor Using Hyperspectral Images of Human Brain. Sensors 19 (4), 920. doi:10.3390/s19040920
Fabelo, H., Ortega, S., Lazcano, R., Madroñal, D., M. Callicó, G., Juárez, E., et al. (2018). An Intraoperative Visualization System Using Hyperspectral Imaging to Aid in Brain Tumor Delineation. Sensors 18 (2), 430. doi:10.3390/s18020430
Fabelo, H., Ortega, S., Ravi, D., Kiran, B. R., Sosa, C., Bulters, D., et al. (2018). Spatio-spectral Classification of Hyperspectral Images for Brain Cancer Detection during Surgical Operations. PLOS ONE 13 (3), e0193721. doi:10.1371/journal.pone.0193721
Fabelo, H., Ortega, S., Szolna, A., Bulters, D., Pineiro, J. F., Kabwama, S., et al. (2019). In-vivo Hyperspectral Human Brain Image Database for Brain Cancer Detection. IEEE Access 7, 39098–39116. doi:10.1109/access.2019.2904788
Farhadi, H., Esmaeily, A., and Najafzadeh, M. (2022). Flood Monitoring by Integration of Remote Sensing Technique and Multi-Criteria Decision Making Method. Comput. Geosciences 160, 105045. doi:10.1016/j.cageo.2022.105045
Fei, B. (2020). “Chapter 3.6 - Hyperspectral Imaging in Medical Applications,” in Data Handling in Science and Technology. Editor J. M. Amigo (Amsterdam, Netherlands: Elsevier), 523–565.
Ferraro, N., Barbarite, E., Albert, T. R., Berchmans, E., Shah, A. H., Bregy, A., et al. (2016). The Role of 5-aminolevulinic Acid in Brain Tumor Surgery: a Systematic Review. Neurosurg. Rev. 39 (4), 545–555. doi:10.1007/s10143-015-0695-2
Florimbi, G., Fabelo, H., Torti, E., Lazcano, R., Madroñal, D., Ortega, S., et al. (2018). Accelerating the K-Nearest Neighbors Filtering Algorithm to Optimize the Real-Time Classification of Human Brain Tumor in Hyperspectral Images. Sensors 18 (7), 2314. doi:10.3390/s18072314
Florimbi, G., Fabelo, H., Torti, E., Ortega, S., Marrero-Martin, M., Callico, G. M., et al. (2020). Towards Real-Time Computing of Intraoperative Hyperspectral Imaging for Brain Cancer Detection Using Multi-GPU Platforms. IEEE Access 8, 8485–8501. doi:10.1109/access.2020.2963939
Fouad Aref, M. H., Sharawi, A. A. R., and El-Sharkawy, Y. H. (2021). Delineation of the Arm Blood Vessels Utilizing Hyperspectral Imaging to Assist with Phlebotomy for Exploiting the Cutaneous Tissue Oxygen Concentration. Photodiagnosis Photodyn. Ther. 33, 102190. doi:10.1016/j.pdpdt.2021.102190
Freitas, S., Silva, H., and Silva, E. (2021). Remote Hyperspectral Imaging Acquisition and Characterization for Marine Litter Detection. Remote Sens. 13 (13), 2536. doi:10.3390/rs13132536
Fu, C., Ma, K., Li, Z., Wang, H., Chen, T., Zhang, D., et al. (2020). Rapid, Label-free Detection of Cerebral Ischemia in Rats Using Hyperspectral Imaging. J. Neurosci. Methods 329, 108466. doi:10.1016/j.jneumeth.2019.108466
Ganser, K. A., Dickhaus, H., Staubert, A., Bonsanto, M. M., Wirtz, C. R., Tronnier, V. M., et al. (1997). Quantification of Brain Shift Effects in MRI Images. Biomed. Tech. Berl. 42, 247–248. doi:10.1515/bmte.1997.42.s2.247
Gao, L., and Smith, R. T. (2015). Optical Hyperspectral Imaging in Microscopy and Spectroscopy - a Review of Data Acquisition. J. Biophot. 8 (6), 441–456. doi:10.1002/jbio.201400051
Gao, L., Smith, R. T., and Tkaczyk, T. S. (2012). Snapshot Hyperspectral Retinal Camera with the Image Mapping Spectrometer (IMS). Biomed. Opt. Express 3 (1), 48–54. doi:10.1364/boe.3.000048
Ghamisi, P., Plaza, J., Chen, Y., Li, J., and Plaza, A. J. (2017). Advanced Spectral Classifiers for Hyperspectral Images: A Review. IEEE Geosci. Remote Sens. Mag. 5 (1), 8–32. doi:10.1109/mgrs.2016.2616418
Giannoni, L., Lange, F., and Tachtsidis, I. (2020). Investigation of the Quantification of Hemoglobin and Cytochrome-C-Oxidase in the Exposed Cortex with Near-Infrared Hyperspectral Imaging: a Simulation Study. J. Biomed. Opt. 25 (4), 1–25. doi:10.1117/1.JBO.25.4.046001
Giannoni, L., Lange, F., Davies, A. L., Dua, A., Gustavson, B., Smith, K. J., et al. (2018). “Hyperspectral Imaging of the Hemodynamic and Metabolic States of the Exposed Cortex: Investigating a Commercial Snapshot Solution,” in Oxygen Transport to Tissue XL. Editors O. Thews, J. C. LaManna, and D. K. Harrison (Cham:: Springer International Publishing), 13–20. doi:10.1007/978-3-319-91287-5_3
Giannoni, L., Lange, F., Sajic, M., Smith, K. J., and Tachtsidis, I. (2021). A Hyperspectral Imaging System for Mapping Haemoglobin and Cytochrome-C-Oxidase Concentration Changes in the Exposed Cerebral Cortex. IEEE J. Sel. Top. Quantum Electron. 27 (4), 1–11. doi:10.1109/jstqe.2021.3053634
Gong, T., Liu, R., Tan, C. L., Farzad, N., Lee, C. K., Pang, B. C., et al. (2007). Classification of CT Brain Images of Head Trauma. Berlin, Heidelberg: Springer Berlin Heidelberg.
Guan, Y., Li, Q., Liu, H., Zhu, Z., and Wang, Y. (2012). Pathological Leucocyte Segmentation Algorithm Based on Hyperspectral Imaging Technique. Opt. Eng. 51 (5), 053202. doi:10.1117/1.oe.51.5.053202
Hadoux, X., Hui, F., Lim, J. K. H., Masters, C. L., Pébay, A., Chevalier, S., et al. (2019). Non-invasive In Vivo Hyperspectral Imaging of the Retina for Potential Biomarker Use in Alzheimer's Disease. Nat. Commun. 10 (1), 4227. doi:10.1038/s41467-019-12242-1
Halicek, M., Little, J. V., Wang, X., Chen, A. Y., and Fei, B. (2019). Optical Biopsy of Head and Neck Cancer Using Hyperspectral Imaging and Convolutional Neural Networks. J. Biomed. Opt. 24 (3), 1–9. doi:10.1117/1.JBO.24.3.036007
Halicek, M., Dormer, J. D., Little, J. V., Chen, A. Y., and Fei, B. (2020). Tumor Detection of the Thyroid and Salivary Glands Using Hyperspectral Imaging and Deep Learning. Biomed. Opt. Express 11 (3), 1383–1400. doi:10.1364/boe.381257
Halicek, M., Dormer, J. D., Little, J. V., Chen, A. Y., Myers, L., Sumer, B. D., et al. (2019). Hyperspectral Imaging of Head and Neck Squamous Cell Carcinoma for Cancer Margin Detection in Surgical Specimens from 102 Patients Using Deep Learning. Cancers 11 (9), 1367. doi:10.3390/cancers11091367
Halicek, M., Fabelo, H., Ortega, S., Callico, G. M., and Fei, B. (2019). In-Vivo and Ex-Vivo Tissue Analysis through Hyperspectral Imaging Techniques: Revealing the Invisible Features of Cancer. Cancers 11 (6), 756. doi:10.3390/cancers11060756
Halicek, M., Lu, G., Little, J. V., Wang, X., Patel, M., Griffith, C. C., et al. (2017). Deep Convolutional Neural Networks for Classifying Head and Neck Cancer Using Hyperspectral Imaging. J. Biomed. Opt. 22 (6), 060503. doi:10.1117/1.jbo.22.6.060503
Hao, Q., Pei, Y., Zhou, R., Sun, B., Sun, J., Li, S., et al. (2021). Fusing Multiple Deep Models for In Vivo Human Brain Hyperspectral Image Classification to Identify Glioblastoma Tumor. IEEE Trans. Instrum. Meas. 70, 1–14. doi:10.1109/tim.2021.3117634
Hövels, A. M., Heesakkers, R. A. M., Adang, E. M., Jager, G. J., Strum, S., Hoogeveen, Y. L., et al. (2008). The Diagnostic Accuracy of CT and MRI in the Staging of Pelvic Lymph Nodes in Patients with Prostate Cancer: a Meta-Analysis. Clin. Radiol. 63 (4), 387–395. doi:10.1016/j.crad.2007.05.022
Iwaki, K., Takagishi, S., Arimura, K., Murata, M., Chiba, T., Nishimura, A., et al. (2021). A Novel Hyperspectral Imaging System for Intraoperative Prediction of Cerebral Hyperperfusion Syndrome after Superficial Temporal Artery-Middle Cerebral Artery Anastomosis in Patients with Moyamoya Disease. Cerebrovasc. Dis. 50 (2), 208–215. doi:10.1159/000513289
Jacques, S. L. (2013). Optical Properties of Biological Tissues: a Review. Phys. Med. Biol. 58 (11), R37–R61. doi:10.1088/0031-9155/58/11/r37
Jeyaraj, P. R., Panigrahi, B. K., and Samuel Nadar, E. R. (2020). Classifier Feature Fusion Using Deep Learning Model for Non-invasive Detection of Oral Cancer from Hyperspectral Image. IETE J. Res., 1–12. doi:10.1080/03772063.2020.1786471
Jolivot, R., Vabres, P., and Marzani, F. (2011). Reconstruction of Hyperspectral Cutaneous Data from an Artificial Neural Network-Based Multispectral Imaging System. Comput. Med. imaging Graph. 35 (2), 85–88. doi:10.1016/j.compmedimag.2010.07.001
Khan, U., Paheding, S., Elkin, C. P., and Devabhaktuni, V. K. (2021). Trends in Deep Learning for Medical Hyperspectral Image Analysis. IEEE Access 9, 79534–79548. doi:10.1109/access.2021.3068392
Kho, E., Dashtbozorg, B., de Boer, L. L., Van de Vijver, K. K., Sterenborg, H. J. C. M., and Ruers, T. J. M. (2019). Broadband Hyperspectral Imaging for Breast Tumor Detection Using Spectral and Spatial Information. Biomed. Opt. Express 10 (9), 4496–4515. doi:10.1364/boe.10.004496
Khouj, Y., Dawson, J., Coad, J., and Vona-Davis, L. (2018). Hyperspectral Imaging and K-Means Classification for Histologic Evaluation of Ductal Carcinoma In Situ. Front. Oncol. 8, 17. doi:10.3389/fonc.2018.00017
Kim, G., Lee, H., Baek, I., Cho, B.-K., and Kim, M. S. (2022). Quantitative Detection of Benzoyl Peroxide in Wheat Flour Using Line-Scan Short-Wave Infrared Hyperspectral Imaging. Sensors Actuators B Chem. 352, 130997. doi:10.1016/j.snb.2021.130997
Kim, W., Novotna, K., Amatya, B., and Khan, F. (2019). Clinical Practice Guidelines for the Management of Brain Tumours: A Rehabilitation Perspective. J. Rehabil. Med. 51 (2), 89–96. doi:10.2340/16501977-2509
Klaessens, J. H. G., de Roode, R., Verdaasdonk, R., and Noordmans, H. J. (2011). Hyperspectral Imaging System for Imaging O2Hb and HHb Concentration Changes in Tissue for Various Clinical Applications, 7890. US: SPIE BiOS.
Konecky, S. D., Wilson, R. H., Hagen, N., Mazhar, A., Tkaczyk, T. S., Frostig, R. D., et al. (2015). Hyperspectral Optical Tomography of Intrinsic Signals in the Rat Cortex. Neurophoton 2 (4), 045003. doi:10.1117/1.nph.2.4.045003
Laffers, W., Westermann, S., Regeling, B., Martin, R., Thies, B., Gerstner, A. O. H., et al. (2016). Früherkennung kanzeröser Läsionen in Oropharynx und Mundhöhle. HNO 64 (1), 27–33. doi:10.1007/s00106-015-0109-3
Leon, R., Fabelo, H., Ortega, S., Piñeiro, J. F., Szolna, A., Hernandez, M., et al. (2021a). VNIR-NIR Hyperspectral Imaging Fusion Targeting Intraoperative Brain Cancer Detection. Sci. Rep. 11 (1), 19696. doi:10.1038/s41598-021-99220-0
Leon, R., Martinez-Vega, B., Fabelo, H., Ortega, S., Melian, V., Castaño, I., et al. (2020). Non-Invasive Skin Cancer Diagnosis Using Hyperspectral Imaging for In-Situ Clinical Support. Jcm 9 (6), 1662. doi:10.3390/jcm9061662
Leon, R., Fabelo, H, Ortega, S, and Callico, G. M (2021b). Hyperspectral VNIR and NIR Sensors for the Analysis of Human Normal Brain and Tumor Tissue. in XXXVI Conference on Design of Circuits and Integrated Systems. 24-26th 2021. Portugal (DCIS). doi:10.1109/dcis53048.2021.9666168
Li, Q., He, X., Wang, Y., Liu, H., Xu, D., and Guo, F. (2013). Review of Spectral Imaging Technology in Biomedical Engineering: Achievements and Challenges. J. Biomed. Opt. 18 (10), 100901–100929. doi:10.1117/1.JBO.18.10.100901
Li, Y., Xie, X., Yang, X., Guo, L., Liu, Z., Zhao, X., et al. (2019). Diagnosis of Early Gastric Cancer Based on Fluorescence Hyperspectral Imaging Technology Combined with Partial-Least-Square Discriminant Analysis and Support Vector Machine. J. Biophot. 12 (5), e201800324. doi:10.1002/jbio.201800324
Lim, J. K. H., Li, Q.-X., Ryan, T., Bedggood, P., Metha, A., Vingrys, A. J., et al. (2021). Retinal Hyperspectral Imaging in the 5xFAD Mouse Model of Alzheimer's Disease. Sci. Rep. 11 (1), 6387. doi:10.1038/s41598-021-85554-2
Liu, N., Chen, Z., and Xing, D. (2020). Integrated Photoacoustic and Hyperspectral Dual-Modality Microscopy for Co-imaging of Melanoma and Cutaneous Squamous Cell Carcinoma In Vivo. J. Biophot. 13 (8), e202000105. doi:10.1002/jbio.202000105
Liu, N., Guo, Y., Jiang, H., and Yi, W. (2020). Gastric Cancer Diagnosis Using Hyperspectral Imaging with Principal Component Analysis and Spectral Angle Mapper. J. Biomed. Opt. 25 (6), 1–9. doi:10.1117/1.JBO.25.6.066005
Liu, X., Zhang, C., Cai, Z., Yang, J., Zhou, Z., and Gong, X. (2021). Continuous Particle Swarm Optimization-Based Deep Learning Architecture Search for Hyperspectral Image Classification. Remote Sens. 13 (6), 1082. doi:10.3390/rs13061082
Lohumi, S. (2018). Calibration and Testing of a Raman Hyperspectral Imaging System to Reveal Powdered Food Adulteration. PLOSONE.
López-Moral, M., García-Álvarez, Y, Molines-Barroso, R. J, Tardáguila-García, A, García-Madrid, M, and Lázaro-Martínez, J. L (2022). A comparison of hyperspectral imaging with routine vascular noninvasive techniques to assess the healing prognosis in patients with diabetic foot ulcers. Journal of Vascular Surgery, 75(1), 255–261. doi:10.1016/j.jvs.2021.07.123
Louis, D. N., Perry, A., Reifenberger, G., von Deimling, A., Figarella-Branger, D., Cavenee, W. K., et al. (2016). The 2016 World Health Organization Classification of Tumors of the Central Nervous System: a Summary. Acta Neuropathol. 131 (6), 803–820. doi:10.1007/s00401-016-1545-1
Lu, B. (2020). Recent Advances of Hyperspectral Imaging Technology and Applications in Agriculture. remote Sens. 12. doi:10.3390/rs12162659
Lu, G., and Fei, B. (2014). Medical Hyperspectral Imaging: a Review. J. Biomed. Opt. 19 (1), 010901. doi:10.1117/1.jbo.19.1.010901
Lu, G. (2014). Medical Hyperspectral Imaging: a Review. J. Biomed. Opt. 19 (1), 10901. doi:10.1117/1.jbo.19.1.010901
Lu, G., Qin, X., Wang, D., Muller, S., Zhang, H., Chen, A., et al. (2016). Hyperspectral Imaging of Neoplastic Progression in a Mouse Model of Oral Carcinogenesis. SPIE Medical Imaging, 9788. US: SPIE. doi:10.1117/12.2216553
Lucas, R., Rowlands, A., Niemann, O., and Merton, R. (2004). “Hyperspectral Sensors and Applications,” in Advanced Image Processing Techniques for Remotely Sensed Hyperspectral Data (Berlin, Heidelberg: Springer Berlin Heidelberg), 11–49. doi:10.1007/978-3-662-05605-9_2
Lv, W., and Wang, X. (2020). Overview of Hyperspectral Image Classification. J. Sensors 2020, 4817234. doi:10.1155/2020/4817234
Maktabi, M. (2021).Using Physiological Parameters Measured by Hyperspectral Imaging to Detect Colorectal Cancer, 43rd Annual International Conference of the IEEE Engineering in Medicine & Biology Society. Jul 26, 2021. Guadalajara, Mexico
Malonek, D., and Grinvald, A. (1996). Interactions between Electrical Activity and Cortical Microcirculation Revealed by Imaging Spectroscopy: Implications for Functional Brain Mapping. Science 272 (5261), 551–554. doi:10.1126/science.272.5261.551
Manni, F. (2020). Hyperspectral Imaging for Colon Cancer Classification in Surgical Specimens: towards Optical Biopsy during Image-Guided Surgery. 42nd Annual International Conference of the IEEE Engineering in Medicine & Biology Society. Jul 20, 2020. Canada. EMBC. doi:10.1109/embc44109.2020.9176543
Manni, F., van der Sommen, F., Zinger, S., Kho, E., de Koning, S. B., Ruers, T., et al. (2019). Automated Tumor Assessment of Squamous Cell Carcinoma on Tongue Cancer Patients with Hyperspectral Imaging. SPIE Medical Imaging, 10951. US: SPIE. doi:10.1117/12.2512238
Manni, F., van der Sommen, F., Fabelo, H., Zinger, S., Shan, C., Edström, E., et al. (2020). Hyperspectral Imaging for Glioblastoma Surgery: Improving Tumor Identification Using a Deep Spectral-Spatial Approach. Sensors 20 (23), 6955. doi:10.3390/s20236955
Martin, R., Thies, B., and Gerstner, A. O. (2012). Hyperspectral Hybrid Method Classification for Detecting Altered Mucosa of the Human Larynx. Int. J. Health Geogr. 11 (1), 21–29. doi:10.1186/1476-072X-11-21
Martinez, B., Leon, R., Fabelo, H., Ortega, S., Piñeiro, J. F., Szolna, A., et al. (2019). Most Relevant Spectral Bands Identification for Brain Cancer Detection Using Hyperspectral Imaging. Sensors 19 (24), 5481. doi:10.3390/s19245481
Mehdorn, M., Köhler, H., Rabe, S. M., Niebisch, S., Lyros, O., Chalopin, C., et al. (2020). Hyperspectral Imaging (HSI) in Acute Mesenteric Ischemia to Detect Intestinal Perfusion Deficits. J. Surg. Res. 254, 7–15. doi:10.1016/j.jss.2020.04.001
Milanic, M., Bjorgan, A., Larsson, M., Strömberg, T., and Randeberg, L. L. (2015). “Detection of Hypercholesterolemia Using Hyperspectral Imaging of Human Skin,” in Clinical and Biomedical Spectroscopy and Imaging IV (Munich: Optica Publishing Group). doi:10.1364/ecbo.2015.95370c
Mirjalili, S. (2019). “Genetic Algorithm”, in Evolutionary Algorithms and Neural Networks: Theory and Applications (Cham: Springer International Publishing), 43–55. doi:10.1007/978-3-319-93025-1_4
Mori, M., Chiba, T., Nakamizo, A., Kumashiro, R., Murata, M., Akahoshi, T., et al. (2014). Intraoperative Visualization of Cerebral Oxygenation Using Hyperspectral Image Data: a Two-Dimensional Mapping Method. Int. J. CARS 9 (6), 1059–1072. doi:10.1007/s11548-014-0989-9
Nathan, M., Kabatznik, A. S., and Mahmood, A. (2018).Hyperspectral Imaging for Cancer Detection and Classification. 3rd Biennial South African Biomedical Engineering Conference. 4-6 April 2018. South Africa.
Nguyen, T. N., Wu, W., Woldermichael, E., Toronov, V., and Lin, S. (2019). Hyperspectral Near-Infrared Spectroscopy Assessment of the Brain during Hypoperfusion. J. Biomed. Opt. 24 (3), 1–6. doi:10.1117/1.JBO.24.3.035007
Nishidate, I., Ishizuka, T., Mustari, A., Yoshida, K., Kawauchi, S., Sato, S., et al. (2017). Evaluation of Cerebral Hemodynamics and Tissue Morphology of In Vivo Rat Brain Using Spectral Diffuse Reflectance Imaging. Appl. Spectrosc. 71 (5), 866–878. doi:10.1177/0003702816657569
Nosrati, R., Vesely, K., Schweizer, T. A., and Toronov, V. (2016). Event-related Changes of the Prefrontal Cortex Oxygen Delivery and Metabolism during Driving Measured by Hyperspectral fNIRS. Biomed. Opt. Express 7 (4), 1323–1335. doi:10.1364/boe.7.001323
Ortega, S., Fabelo, H., Camacho, R., de la Luz Plaza, M., Callicó, G. M., and Sarmiento, R. (2018). Detecting Brain Tumor in Pathological Slides Using Hyperspectral Imaging. Biomed. Opt. Express 9 (2), 818–831. doi:10.1364/boe.9.000818
Ortega, S., Fabelo, H., Iakovidis, D., Koulaouzidis, A., and Callico, G. (2019). Use of Hyperspectral/Multispectral Imaging in Gastroenterology. Shedding Some-Different-Light into the Dark. Jcm 8 (1), 36. doi:10.3390/jcm8010036
Ortega, S., Halicek, M., Fabelo, H., Camacho, R., Plaza, M. d. l. L., Godtliebsen, F., et al. (2020). Hyperspectral Imaging for the Detection of Glioblastoma Tumor Cells in H&E Slides Using Convolutional Neural Networks. Sensors 20 (7), 1911. doi:10.3390/s20071911
Paty, D. W., Oger, J. J. F., Kastrukoff, L. F., Hashimoto, S. A., Hooge, J. P., Eisen, A. A., et al. (1988). MRI in the Diagnosis of MS: A Prospective Study with Comparison of Clinical Evaluation, Evoked Potentials, Oligoclonal Banding, and CT. Neurologyevoked potentials, oligoclonal Band. CT 38 (2), 180. doi:10.1212/wnl.38.2.180
Pelizzari, C. A., Chen, G. T. Y., Spelbring, D. R., Weichselbaum, R. R., and Chen, C.-T. (1989). Accurate Three-Dimensional Registration of CT, PET, And/or MR Images of the Brain. J. Comput. assisted Tomogr. 13 (1), 20–26. doi:10.1097/00004728-198901000-00004
Pichette, J., Laurence, A., Angulo, L., Lesage, F., Bouthillier, A., Nguyen, D. K., et al. (2016). Intraoperative Video-Rate Hemodynamic Response Assessment in Human Cortex Using Snapshot Hyperspectral Optical Imaging. Neurophotonics 3 (4), 045003. doi:10.1117/1.NPh.3.4.045003
Ravi, D., Fabelo, H., Callic, G. M., and Yang, G.-Z. (2017). Manifold Embedding and Semantic Segmentation for Intraoperative Guidance with Hyperspectral Brain Imaging. IEEE Trans. Med. Imaging 36 (9), 1845–1857. doi:10.1109/tmi.2017.2695523
Rinesh, S., Maheswari, K., Arthi, B., Sherubha, P., Vijay, A., Sridhar, S., et al. (2022). Investigations on Brain Tumor Classification Using Hybrid Machine Learning Algorithms. J. Healthc. Eng. 2022, 2761847. doi:10.1155/2022/2761847
Saiko, G., Lombardi, P., Au, Y., Queen, D., Armstrong, D., and Harding, K. (2020). Hyperspectral Imaging in Wound Care: a Systematic Review. Int. Wound J. 17 (6), 1840–1856. doi:10.1111/iwj.13474
Salles, A., Bjaalie, J. G., Evers, K., Farisco, M., Fothergill, B. T., Guerrero, M., et al. (2019). The Human Brain Project: Responsible Brain Research for the Benefit of Society. Neuron 101 (3), 380–384. doi:10.1016/j.neuron.2019.01.005
Salvador, R., Ortega, S., Madronal, D., and Fabelo, H. (2027). HELICoiD: Interdisciplinary and Collaborative Project for Real-Time Brain Cancer Detection. in Proceedings of the Computing Frontiers Conference. May 15 - 17, 2017, New York, United States.
Sancho, J., Sutradhar, P., Rosa, G., Chavarrías, M., Perez-Nuñez, A., Salvador, R., et al. (2021). GoRG: Towards a GPU-Accelerated Multiview Hyperspectral Depth Estimation Tool for Medical Applications. Sensors 21 (12), 4091. doi:10.3390/s21124091
Sawyer, T. W., Luthman, A. S., and Bohndiek, S. E. (2017). Evaluation of Illumination System Uniformity for Wide-Field Biomedical Hyperspectral Imaging. J. Opt. 19 (4), 045301. doi:10.1088/2040-8986/aa6176
Sharma, S., and Buddhiraju, K. M. (2018). Spatial-spectral Ant Colony Optimization for Hyperspectral Image Classification. Int. J. Remote Sens. 39 (9), 2702–2717. doi:10.1080/01431161.2018.1430403
Shonat, R. D., Wachman, E. S., Niu, W., Koretsky, A. P., and Farkas, D. L. (1997). Near-simultaneous Hemoglobin Saturation and Oxygen Tension Maps in Mouse Brain Using an AOTF Microscope. Biophysical J. 73 (3), 1223–1231. doi:10.1016/s0006-3495(97)78155-4
Sun, L., Zhou, M., Li, Q., Hu, M., Wen, Y., and Zhang, J. (2021). Diagnosis of Cholangiocarcinoma from Microscopic Hyperspectral Pathological Dataset by Deep Convolution Neural Networks. Methods S1046-2023 (21), 00097–00099. doi:10.1016/j.ymeth.2021.04.005
Szolna, A., Jesus, M., Gustavo, M. C., Himar, F., and Samuel, O. (2016). O-ONC-03-Hyperspectral Imaging as a Novel Instrument for Intraoperative Brain Tumor Detection. Neurocirugia.
Torbey, M. T., Bösel, J., Rhoney, D. H., Rincon, F., Staykov, D., Amar, A. P., et al. (2015). Evidence-Based Guidelines for the Management of Large Hemispheric Infarction. Neurocrit Care 22 (1), 146–164. doi:10.1007/s12028-014-0085-6
Torti, E., Florimbi, G., Castelli, F., Ortega, S., Fabelo, H., Callicó, G., et al. (2018). Parallel K-Means Clustering for Brain Cancer Detection Using Hyperspectral Images. Electronics 7 (11), 283. doi:10.3390/electronics7110283
Urbanos, G., Martín, A., Vázquez, G., Villanueva, M., Villa, M., Jimenez-Roldan, L., et al. (2021). Supervised Machine Learning Methods and Hyperspectral Imaging Techniques Jointly Applied for Brain Cancer Classification. Sensors 21 (11), 3827. doi:10.3390/s21113827
Villa, C., Miquel, C., Mosses, D., Bernier, M., and Di Stefano, A. L. (2018). The 2016 World Health Organization Classification of Tumours of the Central Nervous System. Presse Med. 47 (11), e187–e200. doi:10.1016/j.lpm.2018.04.015
Wang, Q., Sun, L., Wang, Y., Zhou, M., Hu, M., Chen, J., et al. (2021). Identification of Melanoma from Hyperspectral Pathology Image Using 3D Convolutional Networks. IEEE Trans. Med. Imaging 40 (1), 218–227. doi:10.1109/tmi.2020.3024923
Wang, Z. (2021). Hyperspectral Imaging for Underwater Object Detection. Sensor Rev. 41. doi:10.1108/sr-07-2020-0165
Xu, M., Bo, B., Pei, M., Chen, Y., Shu, C. Y., Qin, Q., et al. (2021). High-resolution Relaxometry-Based Calibrated fMRI in Murine Brain: Metabolic Differences between Awake and Anesthetized States. J. Cereb. Blood Flow Metabolism 42 (5), 811–825. doi:10.1177/0271678x211062279
Yi, W., Zhang, J., Jiang, H., and Zhang, N. (2014). Gastric Cancer Target Detection Using Near-Infrared Hyperspectral Imaging with Chemometrics. International Society for Optics and Photonics.
Yin, C., Zhou, F., Wang, Y., Luo, W., Luo, Q., and Li, P. (2013). Simultaneous Detection of Hemodynamics, Mitochondrial Metabolism and Light Scattering Changes during Cortical Spreading Depression in Rats Based on Multi-Spectral Optical Imaging. NeuroImage 76, 70–80. doi:10.1016/j.neuroimage.2013.02.079
Keywords: hyperspectral imaging, biomedical, cerebral disease, brain tissue metabolic and hemodynamic, brain cancer diagnosis
Citation: Wu Y, Xu Z, Yang W, Ning Z and Dong H (2022) Review on the Application of Hyperspectral Imaging Technology of the Exposed Cortex in Cerebral Surgery. Front. Bioeng. Biotechnol. 10:906728. doi: 10.3389/fbioe.2022.906728
Received: 29 March 2022; Accepted: 09 May 2022;
Published: 27 May 2022.
Edited by:
Huan-Hsuan Hsu, Tufts University, United StatesReviewed by:
Qingli Li, East China Normal University, ChinaVladislav Toronov, Ryerson University, Canada
Copyright © 2022 Wu, Xu, Yang, Ning and Dong. This is an open-access article distributed under the terms of the Creative Commons Attribution License (CC BY). The use, distribution or reproduction in other forums is permitted, provided the original author(s) and the copyright owner(s) are credited and that the original publication in this journal is cited, in accordance with accepted academic practice. No use, distribution or reproduction is permitted which does not comply with these terms.
*Correspondence: Hao Dong, cnhaodong@zhejianglab.com