- 1Institute for Biomechanics, ETH Zurich, Zurich, Switzerland
- 2Department of Chemical Engineering, Indian Institute of Technology Bombay, Mumbai, India
- 3Department for Biomedical Engineering and Mechanics, Virginia Tech, Blacksburg, VI, United States
In silico simulations aim to provide fast, inexpensive, and ethical alternatives to years of costly experimentation on animals and humans for studying bone remodeling, its deregulation during osteoporosis and the effect of therapeutics. Within the varied spectrum of in silico modeling techniques, bone cell population dynamics and agent-based multiphysics simulations have recently emerged as useful tools to simulate the effect of specific signaling pathways. In these models, parameters for cell and cytokine behavior are set based on experimental values found in literature; however, their use is currently limited by the lack of clinical in vivo data on cell numbers and their behavior as well as cytokine concentrations, diffusion, decay and reaction rates. Further, the settings used for these parameters vary across research groups, prohibiting effective cross-comparisons. This review summarizes and evaluates the clinical trial literature that can serve as input or validation for in silico models of bone remodeling incorporating cells and cytokine dynamics in post-menopausal women in treatment, and control scenarios. The GRADE system was used to determine the level of confidence in the reported data, and areas lacking in reported measures such as binding site occupancy, reaction rates and cell proliferation, differentiation and apoptosis rates were highlighted as targets for further research. We propose a consensus for the range of values that can be used for the cell and cytokine settings related to the RANKL-RANK-OPG, TGF-β and sclerostin pathways and a Levels of Evidence-based method to estimate parameters missing from clinical trial literature.
1 Introduction
Global life expectancy increased from 66.8 years in 2000 to 73.4 years in 2019 (Cao and Ho, 2020). This has led to a rise in the prevalence of osteoporosis (OP), which is associated with an increased risk of bone fracture. An estimated 250,000 deaths occurred in the European Union, Switzerland and the United Kingdom in 2019 as a direct result of hip or spine fragility fractures (Kanis et al., 2021); however, only approximately 25% of individuals at high risk for fracture receive pharmacologic therapies other than supplemental calcium and vitamin D (McCloskey et al., 2021). Given the high incidence of both the disease, related fractures, and patient morbidity and mortality, current diagnostic and treatment approaches appear to be insufficient for the healthcare needs of the world’s aging population. This is despite the wide spectrum of approved drugs for OP treatment, whose use has been shown to reduce the incidence of hip fracture in clinical trials by 40% (Black et al., 1996; Ettinger, 1999; Watts et al., 2003; Black et al., 2006; Black et al., 2012; Miller et al., 2012; Inderjeeth et al., 2014; Takakuwa and Iwamoto, 2015; Lello et al., 2017). The treatment gap is caused in part by the high cost of some therapeutic options (Suhm et al., 2008), which is in turn linked to the long and large clinical trials required to observe statistically significant changes in bone quality and fracture risk and obtain drug approval (May 2019).
Traditionally, research efforts to address these issues have focused on creating in vitro and preclinical models to add to the screening and clinical trial design steps in the drug discovery pipeline. However, the majority of new drugs fail in the animal model stage or fail to translate to effective treatment for humans, leading to a significant loss in time and financial resources. In silico simulations have been proposed to provide fast, inexpensive and ethical alternatives to years of costly experimentation on animals and humans (Webster, 2020; Nikolova-Simons et al., 2021; Sarrami-Foroushani et al., 2021). Existing in silico models have been developed to study bone remodeling, its deregulation during metabolic bone diseases and the effect of therapeutics and exercise (Lemaire et al., 2004; Pivonka et al., 2008; Lerebours et al., 2015; Pastrama et al., 2018; Boaretti et al., 2019; Tourolle, 2019; Kameo et al., 2020; Martínez-Reina et al., 2021a; Tourolle et al., 2021).
Bone remodeling is the local repair and adaptation of the mineralized collagen matrix within bone tissue by osteoblasts, bone forming cells, and osteoclasts, bone resorbing cells. This process is coordinated by osteocytes, mechanically sensitive cells located in interconnected cavities within the bone matrix. The balance between resorption and formation is a delicate equilibrium regulated by various cell-cytokine pathways including but not limited to: estrogen-induced or -inhibited apoptosis (Khosla et al., 2012); osteoclastogenesis regulated by receptor activator of nuclear factor ? β (RANK)-its ligand (RANKL) and Osteoprotegerin (OPG) (Boyce and Xing, 2008); mechanosensitivity via the Wingless and int-1 (Wnt) proteins, sclerostin and parathyroid hormone (PTH) (Jilka et al., 1999; Hadjidakis and Androulakis, 2006; Lewiecki, 2014; Kovács et al., 2019); osteoblastogenesis governed by levels of bone morphogenetic protein (BMP) and transforming growth factor-β (TGF-β) (Hanada et al., 1997; Zimmermann et al., 2005; Giannoudis et al., 2008); and interleukin-governed interplay between bone remodeling and immune reactions (Shaarawy et al., 2003; Pino et al., 2010; Sun et al., 2011; Giganti et al., 2012; Marques et al., 2013). Within the varied spectrum of in silico modeling techniques, only bone cell population dynamics models (Lemaire et al., 2004; Pivonka et al., 2008; Lerebours et al., 2015; Pastrama et al., 2018; Martínez-Reina et al., 2021a) and micro-multiphysics agent-based (micro-MPA) models (Boaretti et al., 2019; Tourolle, 2019; Kameo et al., 2020; Tourolle et al., 2021) explicitly incorporate the complex cellular and molecular mechanisms causing metabolic bone diseases and the pathways involved in their treatments.
Bone cell population dynamics models (Lemaire et al., 2004; Pivonka et al., 2008; Lerebours et al., 2015; Pastrama et al., 2018; Martínez-Reina et al., 2021a) divide bone into representative volume elements (RVE). Within the RVE, the model stores one concentration per cytokine and the population of every cell type. The resorption and formation activities of the cells in the RVE as well as their proliferation, differentiation and apoptosis are then determined as a function of the cytokine concentrations using Hill coefficients for repression and activation. Within each RVE, the local bone thinning or thickening is proportional to a weighted balance between the osteoclast and osteoblast numbers.
Evolving from the work of early bone cell population dynamics models, micro-MPA models of bone mechanobiology represent every cell as an independent agent that senses its local environment (i.e. cytokine concentrations and mechanical signals) and modifies it, leading to the emergence of complex patterns at the local and global level as observed in clinical patient data. To date, only micro-MPA models have the resolution to predict the effect of the complex cell-cytokine pathways in bone on the bone microarchitecture. Tourolle (Tourolle et al., 2021) and Kameo (Kameo et al., 2020) developed micro-MPA models of osteoporosis and its treatments with bisphosphonates and RANK ligand inhibitors, respectively, using microcomputed tomography (microCT) scans of iliac crest biopsies with resolutions on the order of 10 µm as input.
Despite the promise of such in silico models, extensive verification and validation are still needed, particularly using human data, for micro-MPA models or bone cell population dynamics models to be applied clinically. In these types of models, parameters for cell and cytokine behavior are set based on experimental values found in literature; however, the settings used vary from one research group to the next (Kameo et al., 2020; Tourolle et al., 2021) as well as from one model to another (Martínez-Reina et al., 2021a; Tourolle et al., 2021). The cell-cytokine signaling pathways included in micro-MPA models of bone remodeling were based on earlier bone cell population dynamics models. All bone cell population dynamics models and micro-MPA models of bone developed to date include the RANK-RANKL-OPG pathway and the three major bone cell types, osteoblasts, osteoclasts and osteocytes, but the choice of other cells and cytokines to include has varied across models as shown in Table 1. To ensure the longevity of micro-MPA models and bone cell population dynamics models and to facilitate their clinical translation, a common set of ground rules is required (Erdemir et al., 2020).
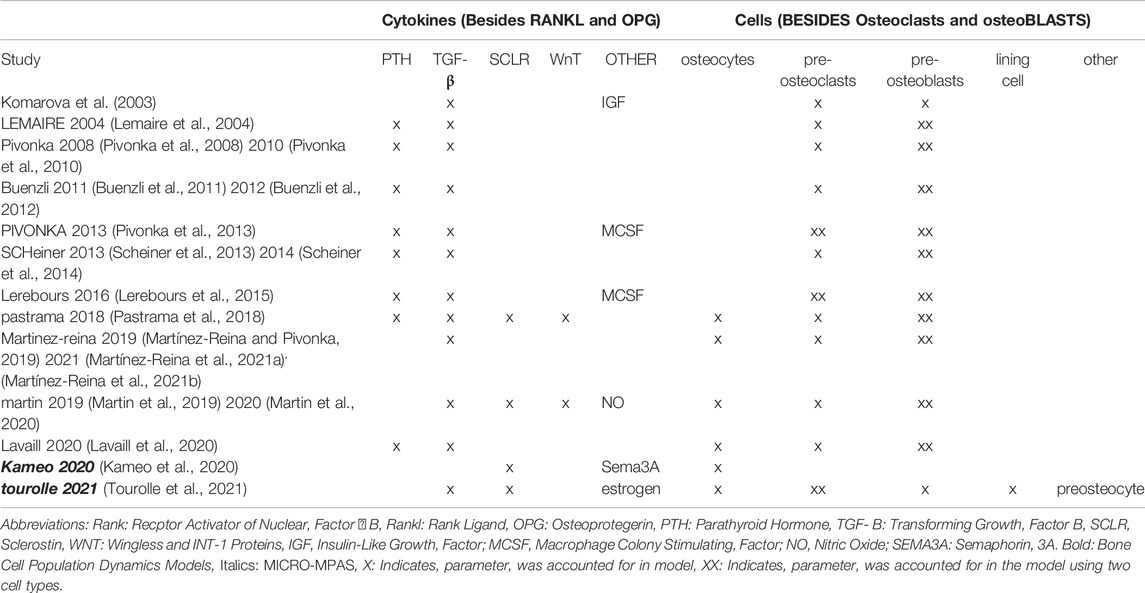
TABLE 1. Bone cell population dynamics models and micro-MPA models and the cell-cytokine pathways included in each model.
This review aims to evaluate and summarize clinical trial literature that can serve as input to micro-MPA models or bone cell population dynamics models of postmenopausal osteoporosis (PMO) and its treatments; thus, providing a consensus for the range of values that can be used for each cell or cytokine behavior setting. The literature will be assessed using the Grading of Recommendations, Assessment, Development and Evaluations (GRADE) system to determine the level of confidence of the reported data (Balshem et al., 2011). Areas lacking in reported cell or cytokine measures with high confidence will be highlighted as targets for further research and guidelines will be formulated to fill gaps in the clinical evidence based on an adaptation of the Oxford Center for Evidence Based Medicine (CEBM) Levels of Evidence.
2 Methods
2.1 Search Strategy
In this review, we collect and analyze data exclusively on the cell-cytokine pathways that have consistently been included in previous micro-MPA models and bone cell population dynamics models of bone, specifically osteoblasts, osteoclasts, osteocytes, RANK-RANKL-OPG, sclerostin, and TGF-β. As there is no direct correspondence between each precursor cell genotype used in micro-MPA models and bone cell population dynamics models and a specific in vivo cell genotype, precursors were excluded from this review. The aim of the search strategy outlined below was to identify articles containing data that could be used for the parametrization or verification of micro-MPA models of bone or bone cell population dynamics models, i.e. any peer-reviewed articles reporting measurements of parameters relating to cell and cytokine behavior in bone from postmenopausal women.
To identify scientific articles reporting measures relevant for model parametrization and verification, the researchers conducted searches of the three most widely used journal databases in the biomedical and life sciences; MEDLINE (PubMed), Scopus and Science Citation Index Expanded (Web of Science, WoS).
The search terms utilized to target relevant studies are shown in Table 2. All searches were accompanied with the keywords “postmenopausal” and “osteoporosis”, and articles with titles containing: “mice”, “rats”, “murine”, “pigs”, “in vitro”, “in silico”, “osteogenesis imperfecta”, “corticosteroid”, “glucocorticoid” were excluded. In PubMed, the search was restricted to “Clinical trials” and “Randomized Controlled Trial”. In Scopus the filters “Article”, “Journal” and “English” were also applied. In Web of Science, the search was restricted to articles; thus, reviews, editorial material, meeting abstracts, and proceedings papers were excluded.
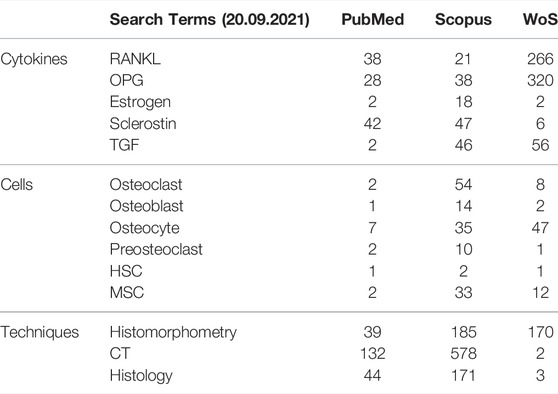
TABLE 2. Search terms and number of resultant quantitative reports on experimental measurements relating to parameters that have to date been used in agent-based models (micro-MPA models) of bone or bone cell population dynamics models.
The results of these searches were analyzed to identify articles providing data on cell and cytokine parameters in postmenopausal women receiving any approved treatments for PMO or acting as untreated controls. Each article identified by this search process was reviewed and included in the current work if it met all three of the following criteria:
1) The article was a case-control or a cohort study published as an original article
2) The findings of the study included quantitative reports on cell numbers or cell proliferation, differentiation, cytokine production or apoptosis rates or cytokine concentrations, diffusion, decay or reaction rates
3) The article reported the above data for post-menopausal women.
A detailed list of the applied exclusion criteria may be found in Supplementary Appendix B.
2.2 Assessment of Quality of Evidence Using Grading of Recommendations, Assessment, Development and Evaluations Guidelines
Every article that fit the inclusion criteria was evaluated using the GRADE guidelines for the evaluation of the quality of evidence (Balshem et al., 2011) to create a trustworthiness score that could be used to explain quantitative differences in the values reported for each parameter.
Two reviewers (CL, AS) independently evaluated the evidence provided in each source for its quality using the systematic GRADE approach, which methodologically assesses studies for five characteristics: risk of bias (Guyatt et al., 2011a), inconsistency or heterogeneity of results across studies (Guyatt et al., 2011b), indirectness (Guyatt et al., 2011c), imprecision (Guyatt et al., 2011d) and publication bias (Guyatt et al., 2011e). The quality of a body of evidence for a particular outcome can be downgraded depending on the seriousness of the risk of bias; unexplained and important inconsistency; indirect study results with uncertain relation to relevant cytokine concentrations, cell numbers, etc. for postmenopausal women; small sample size that influences the width of the confidence intervals which express the uncertainty about the magnitude of the effect; and detection of publication bias. The two reviewers evaluated two articles together to coordinate their assessment strategy then proceeded to independently evaluate the same six articles to validate that the GRADE score assigned to each article was identical. The remaining articles were split among the two reviewers.
Note the GRADE guidelines were designed and are used as a tool to assess the quality of evidence for a drug in the context of therapeutic decision-making. In the context of assessing the quality of evidence for reported parameter values, the five main evaluation criteria (i.e. risk of bias, inconsistency, indirectness, imprecision and publication bias) remained the same in the current work; however, individual subcriteria were interpreted in a manner that differs slightly from the traditional approach. The specific subcriteria that were interpreted differently are outlined below:
Within the risk of bias category, the subcriterion incomplete outcome data was evaluated for randomized trials as the difference between the number of patients enrolled and the number of patients for which the parameter of interest was reported. In cases where there were unexplained differences, studies were downgraded based on this subcriterion.
Within the inconsistency category, the subcriterion probability that subgroup differences may be attributed to chance was refined. Here for the parametrization of cell numbers and cytokine concentrations, evidence was downgraded if different patient demographics displayed overlapping error bars and upgraded if the difference between different patient subgroups was highly significant (p-value < 0.01 set to further stratify the search results). When measures across subgroups were expected to be similar (e.g. gene polymorphisms), the statistical insignificance of differences between the means was used as an upgrade criterion since it indicated repeatability of the measurement.
Within the imprecision category, the quality of evidence was upgraded for sample size if there were more than 100 serum measurements of cytokines or more than 10 measurements of cytokines in bone marrow plasma or cell numbers in biopsies. As before, these thresholds were chosen to further stratify the search results.
All other criteria were assessed as outlined in detail elsewhere (Guyatt et al., 2011a; Guyatt et al., 2011b; Guyatt et al., 2011c; Guyatt et al., 2011d; Guyatt et al., 2011e) and a GRADE score for the quality of the evidence was determined out of 6 for each measurement.
2.3 Data Features
The searches in the databases PubMed, Scopus and WoS yielded a total of 1852 non-duplicate results suitable for analysis. Based on the title and abstracts only, 408 unique peer-reviewed articles in the databases PubMed, Scopus and WoS fit the inclusion criteria and were evaluated for relevance considering the full text. Then, quantitative measures relating to cytokine or cell numbers in postmenopausal women were identified and the quality of the evidence was evaluated using the GRADE guidelines. This reduced the total to 60 articles containing quantitative reports on cell and cytokine behavior in postmenopausal women. Finally, a bibliographic search was conducted on these 60 articles and an additional 17 articles were included based on full article review and GRADE analysis, as illustrated in Figure 1.
2.4 Methodology for Analysis of Physiologic Ranges for Cell and Cytokine Parameters
This review collects, where available from patient data, parameters such as cell numbers, cytokine concentrations, cytokine reaction rates, cell proliferation and apoptosis rates, cell motility, the effects of cytokines on cell behavior and the production rates of cytokines by cells. For cases where patient data is unavailable, cell and cytokine settings are supplemented with values from pre-clinical animal studies, in vitro work or computational studies.
For all cytokines that have to date been included in micro-MPA models of bone or bone cell population dynamics models, differences in the average concentrations reported in various papers were compared with differences in study design. Factors including measurement technique, the origin of the sample or location of the measurement in the body, characteristics of the patient population and the quality of the evidence as assessed using the GRADE guidelines were considered to explain differences in reported values. To facilitate comparison between cytokine concentrations reported in different units, mass concentrations were converted to molar concentrations using molar masses of 20.00 kDa for free RANKL, 19.90 kDa for OPG, 0.27 kDa for estrogen, 22.5 kDa for sclerostin and 4.76 kDa for TGF-β, following the recommendations of Biomedica, Vienna, Austria, the manufacturer of the most widely used human RANKL and OPG assays (as formulated in the instructions for use of ELISA kits BI-20403, BI-20462 and BI-20492).
Similar to the cytokine concentration analysis, differences in study design were accounted for prior to comparison of the reported average cell number for each of the cell number parameters included in micro-MPA models of bone or bone cell population dynamics models (Table 1). There is no consensus regarding conversion between cell surface and cell number therefore articles that assess osteoblast surface (Ob. S/BS, %) and osteoclast surface (Oc. S/BS, %) rather than number were not included in this review (Mullender et al., 2005). As an added challenge, cell numbers across studies are often reported in different units, and there is no standardized way to infer cell numbers in mm−1, mm−2 and mm−3 from each other (Parfitt et al., 1987). In this review, we used two inference methods to qualitatively compare cell numbers reported in different units.
Osteoclast and osteoblast numbers were all measured in histology sections from iliac crest biopsies and reported in units of either cells/mm or cells/mm2. Histology sections are typically analyzed with light microscopes coupled with specific analysis software (e.g., OsteoMeasure™, OsteoMetrics, Decatur, GA, United States). Cell numbers reported by this commercial software in cells/mm of surface are obtained by counting the number of osteoclasts or osteoblasts in a histological section and dividing it by the length of the interface between bone and marrow. We propose to divide these cell numbers in cells/mm by the product of the section thickness and a factor of 2.23 to account for curvature using the parallel surface method (Nishikawa et al., 1998; Jinnai et al., 2002). The resulting number must then be divided by the ratio of the average cell diameter to the section thickness to account for the fact that cells larger than the section thickness would be counted repeatedly if the section were displaced to its nearest neighboring location in the direction normal to the cutting plane. So in effect, the proposed inference method involves dividing the cell number in cells/mm by 2.23 times the cell diameter to estimate the cell number in cells/mm2. Whenever the cell diameter was not provided, a reference of 150 µm was used for osteoclasts and 40 µm for osteoblasts (Manolagas, 2000).
Osteocyte numbers are measured either using histology sections observed with a microscope and reported in cells/mm2 or using µCT imaging of bone biopsies and reported in cells/mm3. Our proposed inference method in this review involves dividing the osteocyte number by the section thickness to obtain the number of osteocytes per unit of volume of the histology section then adjusting for the fact that osteocytes are larger than the section thickness by multiplying by the ratio of section thickness to average osteocyte diameter, thus in effect dividing the osteocyte number in cells/mm2 by the long axis length of osteocyte lacunae (by default, 8 µm (Hannah et al., 2010)) to infer osteocyte numbers in cells/mm3. The surface density of osteoclasts and osteoblasts in cells/mm2 and the volumetric dispersion of osteocytes in cells/mm3 may directly be compared to the corresponding parameters in the 3-dimensional finite element matrix in agent-based models of bone.
Contradictory evidence in literature was reconciled by examining all factors of the study design that could contribute to the observed differences, including the number of postmenopausal women who participated, their average age, their BMD bracket at baseline and the GRADE score evaluating the level of confidence in the study design and execution.
Care must be taken when interpreting reported values as methodological differences in measuring the relevant cell numbers and cytokine concentrations exist between imaging methods, even when manufactured by the same supplier.
3 Results & Discussion
An overview of the parameters related to cell and cytokine signaling pathways that have to date been included in micro-MPA models of bone remodeling or bone cell population dynamics models is presented in Table 3. The table highlights the challenging reconciliation of the differences between values reported in various studies that often span several orders of magnitude.
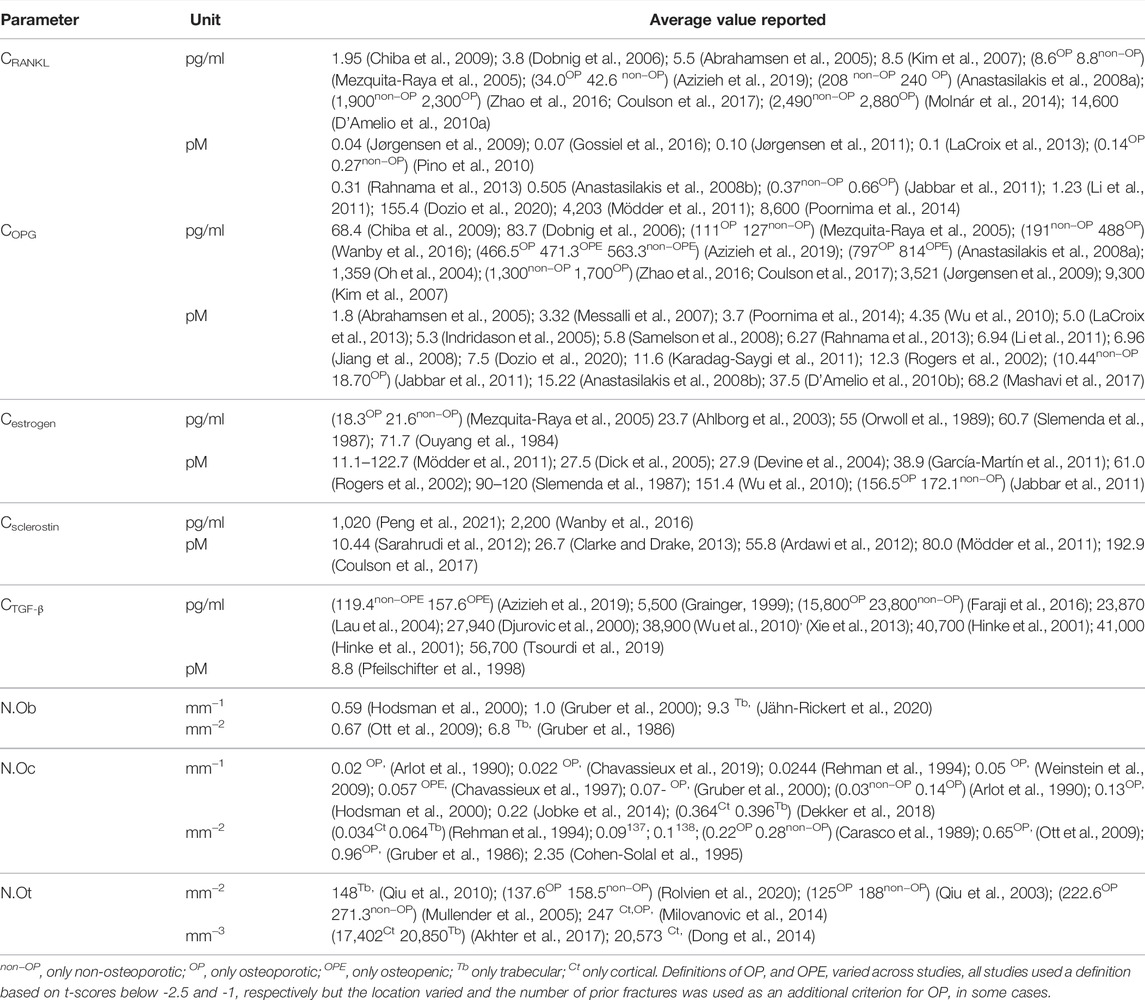
TABLE 3. Average values reported in the literature for bone cell numbers and cytokine concentrations in postmenopausal women, including all anatomical sites, imaging techniques and study designs.
3.1 Cytokine Concentrations
In total, 56 peer-reviewed articles reporting measurements of RANKL (CRANKL, n = 21), OPG (COPG, n = 28), estrogen (Cestrogen, n = 12), sclerostin (Csclerostin, n = 7) and TGF-β (CTGF-β, n = 10) concentrations in post-menopausal women achieved a sufficient GRADE score for further analyses; the corresponding average concentrations are listed in Tables 4–8, respectively. To assist in initialization and define physiologic ranges for each concentration, T-score inclusion criteria, average patient age, size of the patient population (N), origin of the sample and GRADE score were reported. Additionally, assay characteristics and inter-assay coefficient of variation (CV) were reported for CRANKL (Table 4), COPG (Table 5), Cestrogren (Table 6), Csclerostin (Table 7), and CTGF-β (Table 8) to account for differences in measurement technique. Note that RANKL assays must be differentiated in terms of whether they assess free soluble RANKL (FREE), the monomer with molecular weight 20 kDa, or total soluble RANKL (TOTAL), including both RANKL monomer and the RANKL-OPG trimer with molecular weight 60 kDa. As such, the nature of CRANKL was reported when possible (Table 4).
RANKL is the cytokine for which the range of average concentrations reported in peer-reviewed literature in post-menopausal women is the widest (from 0.0419 pM to 8,600 pM). From Table 4, reported RANKL measures may be divided into free RANKL ranging from 0.0419 to 2.1 pM and total RANKL ranging from 155.4 pM. to 8,600 pM; thus, tracking the differences in measurement technique reduces the parameter range from 5 orders of magnitude to 2. The type of RANKL (free vs. total) detected by a given assay was not always directly reported (Anastasilakis et al., 2008a; Molnár et al., 2014; Zhao et al., 2016), necessitating further investigation and assessment of manufacturer guidelines for individual assays. Lack of consistent reporting methods contributes to our inability to make conclusions regarding the correlation between RANKL and BMD in postmenopausal women. The study reporting the highest RANKL average concentration reported highly skewed measurements, with a median of 1,377 pM and an average of 8,600 pM94. Conflicting results were found regarding RANKL concentration in postmenopausal women with and without an OP diagnosis. One peer-reviewed article reported lower free RANKL concentration in postmenopausal women with OP than without (p = 0.021) (Pino et al., 2010), two found no significant difference (Mezquita-Raya et al., 2005; Azizieh et al., 2019) and one found higher free RANKL concentration in postmenopausal women with OP than without (p < 0.0001). Among the studies for which the type of RANKL detected (i.e. free vs. total) was unclear, two reported non-significant differences in average RANKL concentration between women with and without OP (Anastasilakis et al., 2008a; Zhao et al., 2016) and one reported higher RANKL concentrations in post-menopausal women with OP than in post-menopausal women with OPE (p < 0.027) (Molnár et al., 2014). Both the study reporting lower free RANKL in post-menopausal women with OP than without (Pino et al., 2010) and the study reporting higher free RANKL in post-menopausal women with OP than without (Jabbar et al., 2011) achieved a GRADE score of 4. so we have with this review found no evidence that free RANKL is different in the osteoporotic subgroup with respect to the non-osteoporotic subgroup. The wide range of average RANKL concentrations reported in literature may be attributed primarily to differences in measurement techniques across studies. Although a wide range of assays were used to assess RANKL concentration, only two studies reported measurements of RANKL concentration directly in bone marrow supernatant fluid. These studies both received high GRADE scores of 4. Eliminating studies with a GRADE score of 1, 2 or 3 reduces the parameter range to 0.04–0.66 pM for free RANKL and 155 pM to 4,203 pM for total RANKL.
OPG concentrations reported in literature span two orders of magnitude from 1.8 to 467 pM The type of OPG detected by a given assay was not always directly reported, necessitating further investigation and assessment of manufacturer guidelines for individual assays. There was significant overlap between the range of values reported for assays detecting the OPG monomer only and the range of values reported for assays detecting OPG in all its circulating forms, i.e. monomer, dimer and RANKL-OPG complex. Three articles comparing OPG concentrations in postmenopausal women with and without an OP diagnosis reported higher OPG concentrations in postmenopausal women with OP than without (p = 0.035 (Pino et al., 2010), p < 0.001 (Wanby et al., 2016), p < 0.0001 (Jabbar et al., 2011)) while three articles reported the opposite trend (p = 0.034 (Mezquita-Raya et al., 2005), p = 0.025 normal BMD vs. osteoporotic (Azizieh et al., 2019), p = 0.001 significance of positive association between OPG and WBMD (Coulson et al., 2017)). 12 of the 28 articles in Table 5 used OPG assays manufactured by Biomedica, Vienna, Austria, including the articles reporting the lowest OPG average concentration and the highest OPG average concentration, suggesting differences in assay characteristics cannot explain the wide range in reported values. The maximum interassay variability reported for the commercial assays used to perform these OPG concentration measurements in post-menopausal women was only 15%; thus, the interpatient variability in OPG cannot explain the wide range in the values reported for OPG either. Eliminating studies with GRADE scores of 1, 2 and/or 3 could not reduce the parameter range. Nonetheless, the GRADE score approach identified only one article with a GRADE score of 5 and this article reported an average OPG concentration of 12.3 pM which is within the allowable ranges of all high GRADE-score studies reporting in vivo OPG concentrations. Modelers are of course encouraged to directly measure OPG concentration levels in their own patient populations, especially to set OPG concentration levels for patient-specific work. The OPG concentration level in micro-MPA simulations or bone cell population dynamics simulations should be a target for model sensitivity testing since there are no conclusive values.
Estrogen concentrations were more consistent across studies with lower and upper bounds of 27.5 and 265.6 pM, respectively. The collected data supports a negative correlation between age and estrogen level. All reported estrogen concentrations for post-menopausal women in their fifties and sixties were higher than for post-menopausal women in their seventies. The GRADE score approach identified the articles of the highest quality. These articles reported on average values of 61.0 pM in postmenopausal women below the age of 70 and 27.5 pM in postmenopausal women above the age of 70 and these values are therefore recommended as a reference for both postmenopausal women with and without osteoporosis. Note that, as has been extensively reviewed elsewhere, for modeling purposes a question even more critical than the estrogen concentrations themselves may be the parameters relating to the effect of estrogen on bone cells as various occasionally conflicting mechanisms have been reported (Finkelman et al., 1992; Tomkinson et al., 1997; Kousteni et al., 2001; Eghbali-Fatourechi et al., 2003; Nakamura et al., 2007; Hawse et al., 2008; Pacifici, 2008; Imai et al., 2009; Martin-Millan et al., 2010).
Levels of sclerostin reported in the literature vary from 10.44 to 192.9 pM The receptor for sclerostin, LRP6, has been identified on osteoblasts, osteoclast precursors and osteocytes. Specifically, sclerostin impairs osteoblastogenesis and osteoblast survival, increases differentiation of osteoclast precursors to osteoclasts and increases production rates of sclerostin and RANKL by osteocytes (Lewiecki, 2014). The full range of concentrations reported in literature may be reduced to 10.44–80.0 pM using either the GRADE score or a differentiation based on demographics or measurement methods. The main role of sclerostin is to inhibit or stop bone formation. The data presented in Table 7 supports a positive correlation between sclerostin level and age. All reported average sclerostin concentrations in studies with average age of post-menopausal patients above 70 were higher than those reported in studies with average age of post-menopausal patients below 70. This corroborates the increase of sclerostin level with age previously reported over the entire adult lifespan (Clarke and Drake, 2013). Similar to RANKL, only one study reported sclerostin in bone marrow plasma rather than serum (Mödder et al., 2011). The average sclerostin concentration reported in this study was within the range of concentrations reported by studies measuring serum sclerostin (Sarahrudi et al., 2012; Coulson et al., 2017).
Levels of TGF-β reported in the literature vary from 4.77 to 2,268 pM, respectively. The full range of concentrations reported in literature may be reduced to 77–220 pM using either the GRADE score or a differentiation based on demographics or measurement methods. TGF-β binds to and regulates differentiation of both osteoblast precursors and osteoclast precursors. Studies using ELISA assays designed for the detection of TGF-β1 only reported significantly different values relative to studies using methods designed for the detection of both TGF-β1 and TGF-β2. The data presented in Table 8 does not support a correlation between TGF-β concentration and age though this may be due to the fact that the demographics of participants in the studies summarized in Table 8 were similar.
3.2 Cell Numbers
In total, 22 peer-reviewed articles reporting measurements of osteoblast (N.Ob, n = 4), osteoclast (N.OC, n = 12), osteocyte (N.Ot, n = 6) numbers in post-menopausal women achieved a sufficient GRADE score for further analyses, the corresponding average cell numbers are listed in Tables 9–11, respectively. As with the cytokine concentrations, BMD, average patient age, N, origin of the sample and GRADE score were reported to better characterize the datasets prior to comparison. Additionally, measurement technique was reported to account for differences in the reported values. To facilitate comparison between reported cell numbers, any osteoclast or osteoblast numbers reported in the original article in units of cells/mm are reported here in both cells/mm and cells/mm2 and any osteocyte numbers reported in the original article in cells/mm2 are reported here in both cells/mm2 and cells/mm3. Cell numbers in different units were inferred from each other as outlined in Section 2.4.
Osteoblasts are the cells responsible for bone formation. Osteoblast numbers reported in literature vary between 6.6/mm2 and 100/mm2. The patient demographics for the four studies that fulfilled the inclusion criteria were very similar so there is no data to suggest a relationship between osteoblast numbers and age. The study with the highest GRADE score (5) reported the highest average osteoblast number and was also the most recent study and the only study to use toluidine blue staining (Jähn-Rickert et al., 2020). We propose that the bulk of the variation in reported average osteoblast numbers for similar patient demographics may be attributed to the different criteria used for the identification of osteoblasts. Gruber et al., 2000 used Goldner’s staining and then identified osteoblasts based on morphology as plump flattened or cuboidal cells that lined the osteoid surface while Jähn-Rickert et al., 2020, used toluidine blue staining and the quantitative histology software OsteoMeasure (OsteoMetrics, Decatur, GA, United States).
Average osteoblast numbers inferred in cells/mm2 from measurements in cells/mm were slightly higher than average osteoblast numbers reported in cells/mm2 in the original article, suggesting it may be necessary to develop a calibrated inference method. For all three studies in which inferences were made the osteoblast characteristic length was assumed to be 40 µm. Osteoblast characteristic lengths reported in literature vary between 20 and 50 µm (Qiu et al., 2019) so varying this parameter within the range reported in literature cannot explain the differences between osteoblast numbers reported in different units. One major limitation of the inference method is that it assumes a simplified trabecular geometry and may not be directly applicable to cortical bone. All articles found to report osteoblast numbers in postmenopausal women measured these numbers in iliac crest biopsies, which include a combination of cortical and trabecular bone. The biopsy technique will impact the proportion of cortical to trabecular bone.
Osteoclast numbers reported in literature vary between 0.06/mm2 and 2.35/mm2. The data presented in Table 10 includes studies with average participant ages between 50 and 72.8 and does not support a correlation between osteoclast number and age. Differentiating the studies according to the units in which osteoclast numbers were reported leads to two groups with similar average osteoclast numbers. Additionally, the data reported supports an association between the staining method and the measured average osteoclast numbers. Osteoclast numbers measured on histology sections stained with TRAP vary between 1.5 × 10−1/mm2 and 9.6 × 10−1/mm2 while osteoclast numbers measured on histology sections that were not stained or stained with any other staining method than TRAP (e.g. Goldner’s trichrome, toluidine blue, May-Grünwald-Giemsa, solochrome cyanin R) vary between 6 × 10−2/mm2 and 2.5 × 10−1/mm2. Furthermore, the GRADE score approach may be used to reduce the range of osteoclast numbers measured using TRAP staining to between 1.5 × 10−1/mm2 and 6.5 × 10−1/mm2 as the two largest osteoclast numbers reported in literature originated from low GRADE score studies.
Osteocyte numbers reported in literature vary between 1.56 × 104/mm3 and 3.09 × 104/mm3. The measurement location and in particular the differentiation between trabecular and cortical bone is a key element that may be used to refine the estimate of the osteocyte number. The data collected in Table 11 corroborates previously reported conclusions that the osteocyte density is higher in cortical than in trabecular bone (Akhter et al., 2017). All three studies measuring osteocyte numbers in both osteoporotic and non-osteoporotic patients report lower osteocyte numbers in osteoporotic patients.
3.3 In Vitro and In Silico Reports to Fill Gaps in the Clinical Literature
Although the parameters summarized up to now form the basis for the initialization of micro-MPA models of bone or bone cell population dynamics models, other parameters are needed as well to accurately represent the complexity of the pathways responsible for bone remodeling. For cytokine dynamics, properties such as diffusion rate, rate of decay and the rate of production by bone cells are required. Regarding cell numbers, the rates of cell proliferation, apoptosis, differentiation, motility and reaction rates as well as binding site occupancy are needed. The proposed search strategy did not lead to the identification of any articles measuring these rates directly in postmenopausal women. As such, the authors suggest an alternative strategy to reduce the parameter range using a system inspired by the levels of evidence of the Oxford Center for Evidence-based Medicine, developed for therapeutic decision making (Howick et al., 2011).
Table 12 provides an overview of the proposed method to assess the quality of the evidence for the measure reported for each parameter necessary for the setup of a model of bone remodeling incorporating cell and cytokine dynamics. Ideally, cell and cytokine behavior parameters should be directly measured in vivo in the patient population of interest, i.e. postmenopausal women for the purpose of this review. The search strategy adopted in this review did not identify any such measurements and to the best of our knowledge there are to date no published reports of intravital cell imaging in humans. To reduce the parameter space, alternatives include measuring cell and cytokine behavior parameters in conditions resembling in vivo conditions by extracting a biopsy or bone marrow supernatant fluid, isolating the cells and culturing them in an environment resembling bone (evidence level 1 in Table 12) or to perform intravital cell imaging in animals (evidence level 2 in Table 12). However, cells are most often cultured in Petri dishes or other 2D environments that are not representative of bone (evidence level 3 in Table 12). Cell types studied in such environments may not be cells isolated from humans but rather analog cell strains designed specifically for cell culture (evidence level 4 in Table 12). Lastly, computational studies may shed light on the range of values that can theoretically be expected to generate simulations whose trabecular morphometrics resemble in vivo measurements (evidence level 5 in Table 12).
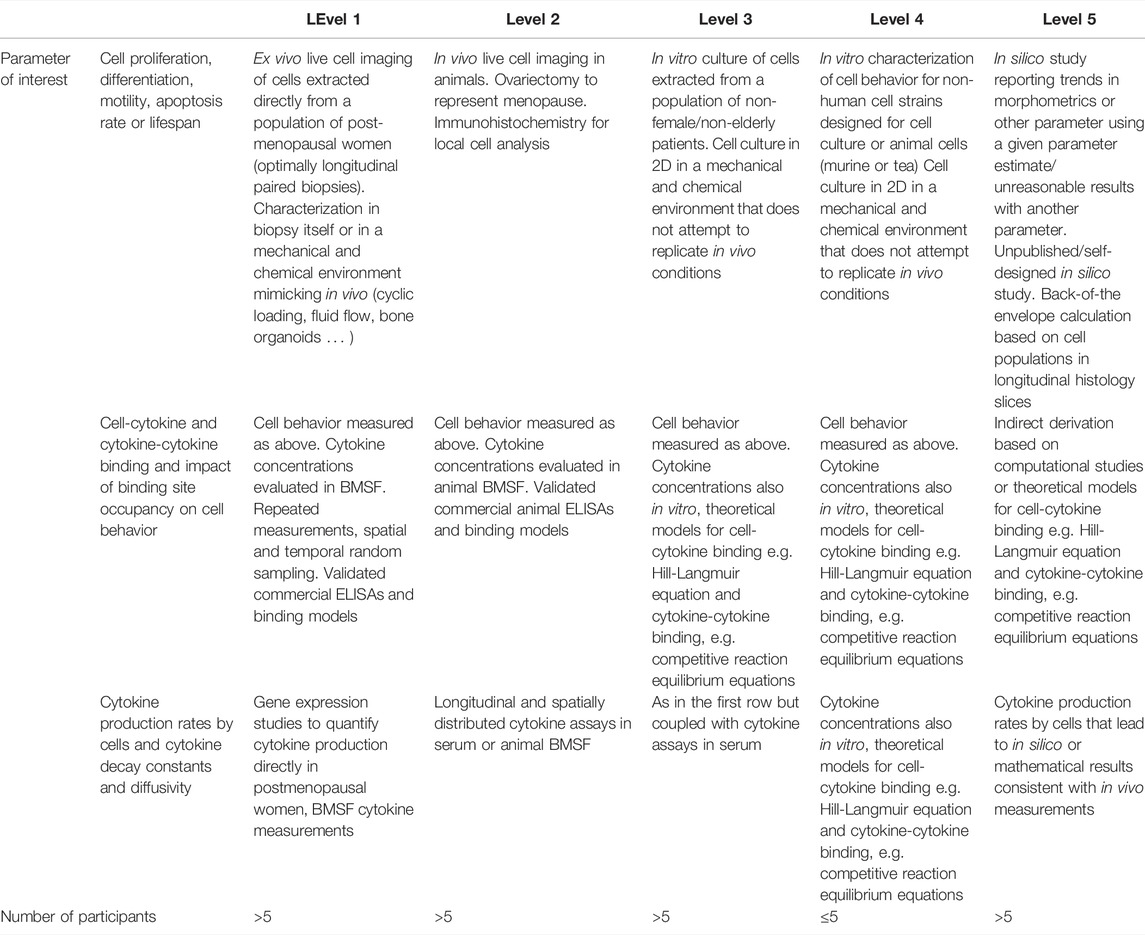
TABLE 12. Levels of Evidence approach to reduce the parameter range for missing parameters in micro-MPA models or bone cell population dynamics models of osteoporosis and its treatments in postmenopausal women. Inspired by the Levels of Evidence approach of the Oxford Centre for Evidence-Based Medicine (Levels of Evidence Working Group, 2011).
The proposed levels of confidence approach involves searching in the literature first for cell behavior parameters at evidence level 1, i.e. for human cells measured in an environment resembling that in vivo. Then, in the absence of any such data, investigating data with a lower confidence level and so on until evidence level 5 is reached. An appropriate illustration of this process for the determination of the apoptosis rate or lifespan of osteoclasts is as follows:
Conflicting evidence suggests osteoclasts have a lifespan from 2 weeks (Manolagas, 2000) up to 6 months (Jacome-Galarza et al., 2019; Søe, 2020), with the differences in those values reconciled at least in part by the discovery of osteomorphs (McDonald et al., 2021); this cell type is generated by the fission of osteoclasts into daughter cells as an alternative to apoptosis thereby increasing the cell lifespan. Both the osteoclast lifespan extremes, i.e. 2 weeks and 6 months, were measured in vitro in murine osteoclasts (Hughes et al., 1996; Jacome-Galarza et al., 2019) (evidence level 4). Fission and fusion into osteomorphs as an alternate cell fate to apoptosis (McDonald et al., 2021) was measured using intravital imaging in mice (evidence level 2) and in vitro culture of human osteoclasts (evidence level 3). Past in silico studies may have justified the use of any osteoclast lifespan within the denoted range to setup their model. Our recommendation moving forward is for conflicting evidence to be assessed according to the levels presented in Table 12 and only the top level of evidence used to parametrize future models.
Note that the level of evidence for parameters governing ligand-binding site kinetics is assessed slightly differently from that for cell behavior parameters (Table 12). Direct in vivo measurements of ligand-binding site kinetics were not found with the search strategy used in this review but are essential for the regulation of cellular behavior in micro-MPA models of bone mechanobiology. The absence of evidence of level 1 through 3 implies that the only way to estimate the ligand-binding site kinetics of interest is relying on level 4 and level 5 evidence. Level 4 evidence in this context includes reaction rates and binding site occupancy estimated from in vitro studies, e.g. 6,382 to 8,491 LRP6 receptors per cell (Lee et al., 2018). Level 5 evidence includes peer-reviewed computational studies, self-designed unpublished computational studies, and back of the envelope calculations with the level of confidence in the point estimates decreasing along that list. Examples of level 5 evidence include reports of 1000 TGF-β receptors/cell (Schafer et al., 2013), a maximum number of 2.703 × 106 RANK receptors per osteoblast (Scheiner et al., 2013) and a maximum number of 3 × 106 RANK receptors per osteoblast (Pivonka et al., 2008).
Finally, the proposed strategy to assess the level of evidence for cytokine production levels by bone cells and cytokine decay and diffusivity is presented in row 3 of Table 12. Evidence of level 1 for these parameters includes both gene expression studies in bone cell cultures derived from bone biopsies in postmenopausal women and longitudinal measurements of cytokine concentrations. ELISA measurements reporting 0.62 ± 0.23 ng of TGF-β1 stored per mg of mineralized bone in iliac crest biopsies from postmenopausal women are an example of level 1 evidence in this context (Bismar et al., 1999). This measurement may be used in an micro-MPA model to define the amount of TGF-β1 released when the bone matrix is resorbed by osteoclasts. Evidence of level 2 includes live cell imaging in animals coupled with genomics studies; however, to the best of our knowledge, there are no examples of this type of evidence for cytokine production rates within the literature. Evidence of level 3 involves live cell imaging in 2D cell cultures of human cells and genomic analyses and longitudinal cytokine assays of these in vitro cultures. Most of the evidence relating to cytokine production levels by bone cells may be categorized as level 3. As such, parameters may be derived indirectly from calculations based on measurements of cytokine concentrations and cell numbers (level 5) (Table 12). As an illustrative example, TGF-β production can be derived in such fashion as it is released from the bone matrix as a result of the activity of only 1 cell type, osteoclasts. One level 5 approach to reduce the parameter range for the amount of TGF-β released per active osteoclast is to divide the total change in TGF-β concentration in conditions of high stimulation such as fracture healing by the time and the osteoclast number.
Level 5 evidence may involve indirect derivations of the measures of interest based on other measures, as illustrated by the above estimate of TGF-β production levels. Features of individual cells and/or cellular units can be used for indirect verification. The functional unit of cells in bone, referred to as the basic multicellular unit (BMU), composed of osteoclasts followed by osteoblasts, has been reported to measure approximately 2 mm end to end (Parfitt, 1994). Further verification elements may come from lifespans reported for BMUs (e.g., 6–9 months (Manolagas, 2000)), stem cells in general (e.g., 5 months to 3 years (Sieburg et al., 2011)) and information concerning the make-up of the activation-resorption-formation cycle (e.g., osteoclasts start resorbing bone as a team of 10–20 cells (Manolagas, 2000)). Moreover, MSCs cannot proliferate beyond the Hayflick limit of approximately 50 population doublings and their long axis has a size of approximately 200 microns (Charbord, 2010).
The examples provided above are by no means exhaustive. The choice of parameters to include will vary from one micro-MPA model to the next; therefore, a comprehensive overview of the ex vivo, in vitro and in silico literature that may be used as tools to reduce the parameter range is outside the scope of this review. In addition, for a number of these parameters there is no evidence at any level, be it 1, 2, 3, 4 or 5, available in the literature. In these cases, we propose that the possible range for parameters of interest for micro-MPA models may be refined by running micro-MPA model simulations with a broad range of values for the missing parameters and comparing the densitometric, static morphometric and dynamic morphometric characteristics of the simulation output with in vivo measurements reported in clinical trials of treatments for osteoporosis to determine the range of settings giving stable physiologic output.
4 Limitations
Limitations of this review include the fact that the authors only considered parameters that have to date been included in published in silico micro-MPA models of OP and its treatments. Although other molecules and cell types have been hypothesized to play significant roles in bone remodeling, the authors chose to focus the review on existing model parameters as these are most likely to be implemented in future models. The authors acknowledge the off-label use of the GRADE approach, which is traditionally used as a framework for developing and presenting summaries of evidence for clinical practice recommendations. However, adaptations to the traditional approach were described in a detailed manner to ensure transparency and applied consistently across individual studies to assess the data presented in this study. Note that the adapted GRADE system described in this review may be implemented to develop other parameter sets for other diseases, but this may require that the data reporting become more standardized. A shortcoming to the confidence in the point estimates of the data included in this review would be the error introduced due to the assumptions made during the conversion of units of data as given in the published sources from the standard units presented in this review. To remove ambiguity we have mentioned the conversion factors whenever the data was transformed.
5 Conclusion
This systematic review has identified a collection of in vivo data to assist in the parametrization of cell and cytokine behavior in micro-MPA models and bone cell population dynamics models. For some of these parameters, we have highlighted criteria and methods that may be used to reconcile differences between measurements reported in various articles. Default levels of RANKL can be set for patients with and without OP. Sclerostin and estrogen should be adjusted by patient age. Measured osteoblast and osteoclast numbers may be affected by measurement methodology and in particular the staining procedure or morphological criteria used to identify the cell type of interest. Osteocyte numbers should be scaled to the disease state. Additionally, the selection of literature parameters to set up in silico models of bone may be facilitated by comparing the quality of conflicting evidence using an adaptation of the GRADE system. Outliers in the reported cell numbers, cytokine concentrations and even densitometric and morphometric trends were exclusively low GRADE score studies and in this respect the proposed adapted GRADE system proved to be a viable assessment method for modelers to evaluate the reliability of parameters reported in clinical studies. We have outlined how, by eliminating low GRADE score studies, the parameter range for cell numbers and cytokine concentrations may be reduced, while also defining the level of confidence in this parameter range reduction. The conclusions made here are based on published work and established methods, however with time and advances in measurement technique, the parameter range for each cell and cytokine behavior setting or predictive target will vary and be refined for more specific patient demographics, diseases and treatments. Thus, a living inventory is available as an open access resource here.
This review clarified the scarcity of clinical data on reaction rates, binding site occupancy, cytokine diffusion and decay and cytokine production levels by bone cells. Gaps in the clinical evidence may be filled with human ex vivo, animal in vivo, human in vitro, non-human in vitro or in silico evidence. To assess the reliability of point estimates used to fill gaps in the literature, we propose an adaptation of the Oxford CEBM Levels of Evidence approach. This approach can be used to determine the order of magnitude of micro-MPA model parameters for which there is no clinical evidence. Future studies should use this method and report the level of evidence for cell and cytokine behaviour parameters used in the parametrization of their model. The methods outlined in the review can be applied to other clinical trial literature and metabolic bone diseases, opening the door to better standardization across the field and a scientific consensus on quantitative characteristics of bone cell and cytokine behaviour and the identification of targets for further in silico research.
Data Availability Statement
The datasets presented in this study can be found in online repositories. The names of the repository/repositories and accession number(s) can be found below: 10.3929/ethz-b-000548897.
Author Contributions
CL, DB, RM, and CC contributed to the design of the study. CL and AS contributed to the collection, assembly and assessment of data, CL, DB, RM, and CC contributed to the analysis and interpretation of results, CL, DB. AS, RM, and CC wrote the main manuscript text. All authors contributed to revising the manuscript and approved the final version to be submitted.
Funding
The authors thankfully acknowledge the European Union’s MechAGE ERC-2016-ADG-741883 and Horizon 2020 research and innovation program under the Marie Skłodowska-Curie (grant agreement 841316) for financial support. Open access funding provided by ETH Zürich.
Conflict of Interest
The authors declare that the research was conducted in the absence of any commercial or financial relationships that could be construed as a potential conflict of interest.
Publisher’s Note
All claims expressed in this article are solely those of the authors and do not necessarily represent those of their affiliated organizations, or those of the publisher, the editors and the reviewers. Any product that may be evaluated in this article, or claim that may be made by its manufacturer, is not guaranteed or endorsed by the publisher.
Supplementary Material
The Supplementary Material for this article can be found online at: https://www.frontiersin.org/articles/10.3389/fbioe.2022.901720/full#supplementary-material
References
Abrahamsen, B., Hjelmborg, J. v., Kostenuik, P., Stilgren, L. S., Kyvik, K., Adamu, S., et al. (2005). Circulating Amounts of Osteoprotegerin and RANK Ligand: Genetic Influence and Relationship with BMD Assessed in Female Twins. Bone 36, 727–735. doi:10.1016/j.bone.2004.12.015
Ahlborg, H. G., Johnell, O., Turner, C. H., Rannevik, G., and Karlsson, M. K. (2003). Bone Loss and Bone Size after Menopause. N. Engl. J. Med. 349, 327–334. doi:10.1056/NEJMOA022464
Akhter, M. P., Kimmel, D. B., Lappe, J. M., and Recker, R. R. (2017). Effect of Macroanatomic Bone Type and Estrogen Loss on Osteocyte Lacunar Properties in Healthy Adult Women. Calcif. Tissue Int. 100, 619–630. doi:10.1007/S00223-017-0247-6
Anastasilakis, A. D., Goulis, D. G., Polyzos, S. A., Gerou, S., Pavlidou, V., Koukoulis, G., et al. (2008). Acute Changes in Serum Osteoprotegerin and Receptor Activator for Nuclear Factor-Κb Ligand Levels in Women with Established Osteoporosis Treated with Teriparatide. Eur. J. Endocrinol. 158, 411–415. doi:10.1530/EJE-07-0528
Anastasilakis, A., Goulis, D., Polyzos, S., Gerou, S., Koukoulis, G., Kita, M., et al. (2008). Serum Osteoprotegerin and RANKL Are Not Specifically Altered in Women with Postmenopausal Osteoporosis Treated with Teriparatide or Risedronate: a Randomized, Controlled Trial. Horm. Metab. Res. 40, 281–285. doi:10.1055/S-2008-1046787
Ardawi, M.-S. M., Rouzi, A. A., and Qari, M. H. (2012). Physical Activity in Relation to Serum Sclerostin, Insulin-like Growth Factor-1, and Bone Turnover Markers in Healthy Premenopausal Women: A Cross-Sectional and a Longitudinal Study. J. Clin. Endocrinol. Metabolism 97, 3691–3699. doi:10.1210/jc.2011-3361
Arlot, M. E., Delmas, P. D., Chappard, D., and Meunier, P. J. (1990). Trabecular and Endocortical Bone Remodeling in Postmenopausal Osteoporosis: Comparison with Normal Postmenopausal Women. Osteoporos. Int. 1, 41–49. doi:10.1007/BF01880415
Azizieh, F. Y., Shehab, D., Jarallah, K. A., Gupta, R., and Raghupathy, R. (2019). Circulatory Levels of RANKL, OPG, and Oxidative Stress Markers in Postmenopausal Women with Normal or Low Bone Mineral Density. Biomark. Insights 14, 117727191984382. doi:10.1177/1177271919843825
Balshem, H., Helfand, M., Schünemann, H. J., Oxman, A. D., Kunz, R., Brozek, J., et al. (2011). GRADE Guidelines: 3. Rating the Quality of Evidence. J. Clin. Epidemiol. 64, 401–406. doi:10.1016/j.jclinepi.2010.07.015
Bismar, H., Klöppinger, T., Schuster, E. M., Balbach, S., Diel, I., Ziegler, R., et al. (1999). Transforming Growth Factor β (TGF-β) Levels in the Conditioned Media of Human Bone Cells: Relationship to Donor Age, Bone Volume, and Concentration of TGF-β in Human Bone Matrix In Vivo. Bone 24, 565–569. doi:10.1016/S8756-3282(99)00082-4
Black, D. M., Cummings, S. R., Karpf, D. B., Cauley, J. A., Thompson, D. E., Nevitt, M. C., et al. (1996). Randomised Trial of Effect of Alendronate on Risk of Fracture in Women with Existing Vertebral Fractures. Lancet 348, 1535–1541. doi:10.1016/S0140-6736(96)07088-2
Black, D. M., Reid, I. R., Boonen, S., Bucci‐Rechtweg, C., Cauley, J. A., Cosman, F., et al. (2012). The Effect of 3 versus 6 Years of Zoledronic Acid Treatment of Osteoporosis: A Randomized Extension to the HORIZON‐Pivotal Fracture Trial (PFT). J. Bone Min. Res. 27, 243–254. doi:10.1002/JBMR.1494
Black, D. M., Schwartz, A. V., Ensrud, K. E., Cauley, J. A., Levis, S., Quandt, S. A., et al. (2006). Effects of Continuing or Stopping Alendronate after 5 Years of Treatment. JAMA 296, 2927–2938. doi:10.1001/JAMA.296.24.2927
Boaretti, D., Tourolle, D., and Müller, R. (2019). Studying How the Link between Mechanical Stimulation and Cellular Activation Effects Bone Microarchitecture. European Society of Biomechanics. Vienna, Austria. Abstracts 25th Congress.
Boyce, B. F., and Xing, L. (2008). Functions of RANKL/RANK/OPG in Bone Modeling and Remodeling. Archives Biochem. Biophysics 473, 139–146. doi:10.1016/j.abb.2008.03.018
Buenzli, P. R., Pivonka, P., Gardiner, B. S., and Smith, D. W. (2012). Modelling the Anabolic Response of Bone Using a Cell Population Model. J. Theor. Biol. 307, 42–52. doi:10.1016/j.jtbi.2012.04.019
Buenzli, P. R., Pivonka, P., and Smith, D. W. (2011). Spatio-temporal Structure of Cell Distribution in Cortical Bone Multicellular Units: A Mathematical Model. Bone 48, 918–926. doi:10.1016/j.bone.2010.12.009
Cao, B., and Ho, J. (2020). WHO Methods and Data Sources for Life Tables 1990-2019. World Health Organ. WHO/DDI/DNA/GHE/2020.1.
Carasco, M. G., de Vernejoul, M. C., Sterkers, Y., Morieux, C., Kuntz, D., and Miravet, L. (1989). Decreased Bone Formation in Osteoporotic Patients Compared with Age-Matched Controls. Calcif. Tissue Int. 44, 173–175. doi:10.1007/BF02556560
Charbord, P. (2010). Bone Marrow Mesenchymal Stem Cells: Historical Overview and Concepts. Hum. Gene Ther. 21, 1045–1056. doi:10.1089/hum.2010.115
Chavassieux, P., Meunier, P. J., Roux, J. P., Portero-Muzy, N., Pierre, M., and Chapurlat, R. (2013). Bone Histomorphometry of Transiliac Paired Bone Biopsies after 6 or 12 Months of Treatment with Oral Strontium Ranelate in 387 Osteoporotic Women: Randomized Comparison to Alendronate. J. Bone Min. Res. 29, 618–628. doi:10.1002/jbmr.2074
Chavassieux, P., Chapurlat, R., Portero‐Muzy, N., Roux, J. P., Garcia, P., Brown, J. P., et al. (2019). Bone‐Forming and Antiresorptive Effects of Romosozumab in Postmenopausal Women with Osteoporosis: Bone Histomorphometry and Microcomputed Tomography Analysis after 2 and 12 Months of Treatment. J. Bone Min. Res. 34, 1597–1608. doi:10.1002/jbmr.3735
Chavassieux, P. M., Arlot, M. E., Reda, C., Wei, L., Yates, A. J., and Meunier, P. J. (1997). Histomorphometric Assessment of the Long-Term Effects of Alendronate on Bone Quality and Remodeling in Patients with Osteoporosis. J. Clin. Invest. 100, 1475–1480. doi:10.1172/JCI119668
Chiba, Y., Onouchi, T., Ikeda, T., Adachi, J., Tamura, Y., and Horiuchi, T. (2009). Implications of Measuring Soluble Receptor Activators of Nuclear Factor-κB Ligand and Osteoprotegerin in Bone Metabolism of Elderly Women. Gerontology 55, 275–280. doi:10.1159/000196280
Clarke, B. L., and Drake, M. T. (2013). Clinical Utility of Serum Sclerostin Measurements. Bonekey Rep. 2, 361. doi:10.1038/bonekey.2013.95
Cohen-Solal, M. E., Graulet, A. M., Gueris, J., Denne, M. A., Bergot, C., Morieux, C., et al. (1995). Bone Resorption at the Femoral Neck Is Dependent on Local Factors in Nonosteoporotic Late Postmenopausal Women: an In Vitro-In Vivo Study. J. Bone Min. Res. 10, 307–314. doi:10.1002/JBMR.5650100219
Coulson, J., Bagley, L., Barnouin, Y., Bradburn, S., Butler-Browne, G., Gapeyeva, H., et al. (2017). Circulating Levels of Dickkopf-1, Osteoprotegerin and Sclerostin Are Higher in Old Compared with Young Men and Women and Positively Associated with Whole-Body Bone Mineral Density in Older Adults. Osteoporos. Int. 28, 2683–2689. doi:10.1007/S00198-017-4104-2
D’Amelio, P., Cristofaro, M. A., Grimaldi, A., Ravazzoli, M., Pluviano, F., Grosso, E., et al. (2010). The Role of Circulating Bone Cell Precursors in Fracture Healing. Calcif. Tissue Int. 86, 463–469. doi:10.1007/s00223-010-9362-3
D’Amelio, P., Grimaldi, A., Cristofaro, M. A., Ravazzoli, M., Molinatti, P. A., Pescarmona, G. P., et al. (2010). Alendronate Reduces Osteoclast Precursors in Osteoporosis. Osteoporos. Int. 21, 1741–1750. doi:10.1007/S00198-009-1129-1
Dekker, H., Schulten, E. A. J. M., ten Bruggenkate, C. M., Bloemena, E., van Ruijven, L., and Bravenboer, N. (2018). Resorption of the Mandibular Residual Ridge: A Micro-CT and Histomorphometrical Analysis. Gerodontology 35, 221–228. doi:10.1111/GER.12343
Dempster, D. W., Brown, J. P., Fahrleitner-Pammer, A., Kendler, D., Rizzo, S., Valter, I., et al. (2018). Effects of Long-Term Denosumab on Bone Histomorphometry and Mineralization in Women with Postmenopausal Osteoporosis. J. Clin. Endocrinol. Metab. 103, 2498–2509. doi:10.1210/jc.2017-02669
Devine, A., Dick, I. M., Dhaliwal, S. S., Naheed, R., Beilby, J., and Prince, R. L. (2004). Prediction of Incident Osteoporotic Fractures in Elderly Women Using the Free Estradiol Index. Osteoporos. Int. 16, 216–221. doi:10.1007/S00198-004-1674-6
Dick, I. M., Devine, A., Beilby, J., and Prince, R. L. (2005). Effects of Endogenous Estrogen on Renal Calcium and Phosphate Handling in Elderly Women. Am. J. Physiology-Endocrinology Metabolism 288, E430–E435. doi:10.1152/AJPENDO.00140.2004
Djurovic, S., Os, I., Hofstad, A. E., Abdelnoor, M., Westheim, A., and Berg, K. (2000). Increased Plasma Concentrations of TGF-Beta1 after Hormone Replacement Therapy. J. Intern. Med. 247, 279–285. doi:10.1046/j.1365-2796.2000.00648.x
Dobnig, H., Hofbauer, L. C., Viereck, V., Obermayer-Pietsch, B., and Fahrleitner-Pammer, A. (2006). Changes in the RANK Ligand/osteoprotegerin System Are Correlated to Changes in Bone Mineral Density in Bisphosphonate-Treated Osteoporotic Patients. Osteoporos. Int. 17, 693–703. doi:10.1007/S00198-005-0035-4
Dong, P., Haupert, S., Hesse, B., Langer, M., Gouttenoire, P.-J., Bousson, V., et al. (2014). 3D Osteocyte Lacunar Morphometric Properties and Distributions in Human Femoral Cortical Bone Using Synchrotron Radiation Micro-CT Images. Bone 60, 172–185. doi:10.1016/j.bone.2013.12.008
Dozio, E., Passeri, E., Vianello, E., Palmieri, S., Eller-Vainicher, C., Corsi Romanelli, M., et al. (2020). Circulating IL-17A Levels in Postmenopausal Women with Primary Hyperparathyroidism. Mediat. Inflamm. 2020, 1–6. doi:10.1155/2020/3417329
Eghbali-Fatourechi, G., Khosla, S., Sanyal, A., Boyle, W. J., Lacey, D. L., and Riggs, B. L. (2003). Role of RANK Ligand in Mediating Increased Bone Resorption in Early Postmenopausal Women. J. Clin. Invest. 111, 1221–1230. doi:10.1172/jci200317215
Erdemir, A., Mulugeta, L., Ku, J. P., Drach, A., Horner, M., Morrison, T. M., et al. (2020). Credible Practice of Modeling and Simulation in Healthcare: Ten Rules from a Multidisciplinary Perspective. J. Transl. Med. 18, 1–18. doi:10.1186/S12967-020-02540-4
Ettinger, B. (1999). Reduction of Vertebral Fracture Risk in Postmenopausal Women with Osteoporosis Treated with RaloxifeneResults from a 3-Year Randomized Clinical Trial. JAMA 282, 637–645. doi:10.1001/JAMA.282.7.637
Faraji, A., Abtahi, S., Ghaderi, A., and Samsami Dehaghani, A. (2016). Transforming Growth Factor β1 (TGF-Β1) in the Sera of Postmenopausal Osteoporotic Females. Int. J. Endocrinol. Metab. 14, 14. doi:10.5812/IJEM.36511
Finkelman, R. D., Bell, N. H., Strong, D. D., Demers, L. M., and Baylink, D. J. (1992). Ovariectomy Selectively Reduces the Concentration of Transforming Growth Factor Beta in Rat Bone: Implications for Estrogen Deficiency-Associated Bone Loss. Proc. Natl. Acad. Sci. U.S.A. 89, 12190–12193. doi:10.1073/pnas.89.24.12190
García-Martín, A., Reyes-García, R., García-Castro, J. M., Rozas-Moreno, P., Escobar-Jiménez, F., and Muñoz-Torres, M. (2011). Role of Serum FSH Measurement on Bone Resorption in Postmenopausal Women. Endocrine 41, 302–308. doi:10.1007/S12020-011-9541-7
Giannoudis, P. V., Pountos, I., Morley, J., Perry, S., Tarkin, H. I., and Pape, H.-C. (2008). Growth Factor Release Following Femoral Nailing. Bone 42, 751–757. doi:10.1016/j.bone.2007.12.219
Giganti, M. G., Liuni, F., Celi, M., Gasbarra, E., Zenobi, R., Tresoldi, I., et al. (2012). Changes in Serum Levels of TNF-Alpha, IL-6, OPG, RANKL and Their Correlation with Radiographic and Clinical Assessment in Fragility Fractures and High Energy Fractures. J. Biol. Regul. Homeost. Agents 26, 671–680.
Gossiel, F., Hoyle, C., McCloskey, E. V., Naylor, K. E., Walsh, J., Peel, N., et al. (2016). The Effect of Bisphosphonate Treatment on Osteoclast Precursor Cells in Postmenopausal Osteoporosis: The TRIO Study. Bone 92, 94–99. doi:10.1016/j.bone.2016.08.010
Grainger, D. (1999). Genetic Control of the Circulating Concentration of Transforming Growth Factor Type Beta1. Hum. Mol. Genet. 8, 93–97. doi:10.1093/HMG/8.1.93
Gruber, H. E., Ivey, J. L., Thompson, E. R., Chesnut, C. H., and Baylink, D. J. (1986). Osteoblast and Osteoclast Cell Number and Cell Activity in Postmenopausal Osteoporosis. Min. Electrolyte Metab. 12, 246–254.
Gruber, H. E., Grigsby, J., and Chesnut, III, C. H. (2000). Osteoblast Numbers after Calcitonin Therapy: A Retrospective Study of Paired Biopsies Obtained during Long-Term Calcitonin Therapy in Postmenopausal Osteoporosis. Calcif. Tissue Int. 66, 29–34. doi:10.1007/s002230010007
Guyatt, G. H., Oxman, A. D., Kunz, R., Brozek, J., Alonso-Coello, P., Rind, D., et al. (2011). GRADE Guidelines 6. Rating the Quality of Evidence-Imprecision. J. Clin. Epidemiol. 64, 1283–1293. doi:10.1016/j.jclinepi.2011.01.012
Guyatt, G. H., Oxman, A. D., Kunz, R., Woodcock, J., Brozek, J., Helfand, M., et al. (2011). GRADE Guidelines: 8. Rating the Quality of Evidence-Indirectness. J. Clin. Epidemiol. 64, 1303–1310. doi:10.1016/j.jclinepi.2011.04.014
Guyatt, G. H., Oxman, A. D., Kunz, R., Woodcock, J., Brozek, J., Helfand, M., et al. (2011). GRADE Guidelines: 7. Rating the Quality of Evidence-Inconsistency. J. Clin. Epidemiol. 64, 1294–1302. doi:10.1016/j.jclinepi.2011.03.017
Guyatt, G. H., Oxman, A. D., Montori, V., Vist, G., Kunz, R., Brozek, J., et al. (2011). GRADE Guidelines: 5. Rating the Quality of Evidence-Publication Bias. J. Clin. Epidemiol. 64, 1277–1282. doi:10.1016/j.jclinepi.2011.01.011
Guyatt, G. H., Oxman, A. D., Vist, G., Kunz, R., Brozek, J., Alonso-Coello, P., et al. (2011). GRADE Guidelines: 4. Rating the Quality of Evidence-Study Limitations (Risk of Bias). J. Clin. Epidemiol. 64, 407–415. doi:10.1016/j.jclinepi.2010.07.017
Hadjidakis, D. J., and Androulakis, I. I. (2006). Bone Remodeling. Ann. N. Y. Acad. Sci. 1092, 385–396. doi:10.1196/annals.1365.035
Hampson, G., Edwards, S., Conroy, S., Blake, G. M., Fogelman, I., and Frost, M. L. (2013). The Relationship between Inhibitors of the Wnt Signalling Pathway (Dickkopf-1(DKK1) and Sclerostin), Bone Mineral Density, Vascular Calcification and Arterial Stiffness in Post-menopausal Women. Bone 56, 42–47. doi:10.1016/j.bone.2013.05.010
Hanada, K., Dennis, J. E., and Caplan, A. I. (1997). Stimulatory Effects of Basic Fibroblast Growth Factor and Bone Morphogenetic Protein-2 on Osteogenic Differentiation of Rat Bone Marrow-Derived Mesenchymal Stem Cells. J. Bone Min. Res. 12, 1606–1614. doi:10.1359/jbmr.1997.12.10.1606
Hannah, K. M., Thomas, C. D. L., Clement, J. G., De Carlo, F., and Peele, A. G. (2010). Bimodal Distribution of Osteocyte Lacunar Size in the Human Femoral Cortex as Revealed by Micro-CT. Bone 47, 866–871. doi:10.1016/j.bone.2010.07.025
Hawse, J. R., Subramaniam, M., Ingle, J. N., Oursler, M. J., Rajamannan, N. M., and Spelsberg, T. C. (2008). Estrogen-Tgfβ Cross-Talk in Bone and Other Cell Types: Role of TIEG, Runx2, and Other Transcription Factors. J. Cell. Biochem. 103, 383–392. doi:10.1002/jcb.21425
Hinke, V., Seck, T., Clanget, C., Scheidt-Nave, C., Ziegler, R., and Pfeilschifter, J. (2001). Association of Transforming Growth Factor-B1 (TGFb1) T29?C Gene Polymorphism with Bone Mineral Density (BMD), Changes in BMD, and Serum Concentrations of TGF-B1 in a Population-Based Sample of Postmenopausal German Women. Calcif. Tissue Int. 69, 315–320. doi:10.1007/S002230020024
Hodsman, A. B., Kisiel, M., Adachi, J. D., Fraher, L. J., and Watson, P. H. (2000). Histomorphometric Evidence for Increased Bone Turnover without Change in Cortical Thickness or Porosity after 2 Years of Cyclical hPTH(1-34) Therapy in Women with Severe Osteoporosis. Bone 27, 311–318. doi:10.1016/S8756-3282(00)00316-1
Howick, J., Glasziou, P., Greenhalgh, T., Carl Heneghan, A., Moschetti, I., Phillips, B., et al. (2011). Explanation of the Oxford Centre for Evidence-Based Medicine (OCEBM) Levels of Evidence (Background Document). Oxford Centre for Evidence-Based Medicine.
Hughes, D. E., Dai, A., Tiffee, J. C., Li, H. H., Mundy, G. R., and Boyce, B. F. (1996). Estrogen Promotes Apoptosis of Murine Osteoclasts Mediated by TGF-β. Nat. Med. 2, 1132–1136. doi:10.1038/NM1096-1132
Imai, Y., Youn, M.-Y., Kondoh, S., Nakamura, T., Kouzmenko, A., Matsumoto, T., et al. (2009). Estrogens Maintain Bone Mass by Regulating Expression of Genes Controlling Function and Life Span in Mature Osteoclasts. Ann. N. Y. Acad. Sci. 1173, E31–E39. doi:10.1111/j.1749-6632.2009.04954.x
Inderjeeth, C., Glendenning, P., Ratnagobal, S., Inderjeeth, D., and Ondhia, C. (2014). Long-term Efficacy, Safety, and Patient Acceptability of Ibandronate in the Treatment of Postmenopausal Osteoporosis. Ijwh 7, 7–17. doi:10.2147/IJWH.S73944
Indridason, O. S. L., Franzson, L., and Sigurdsson, G. (2005). Serum Osteoprotegerin and its Relationship with Bone Mineral Density and Markers of Bone Turnover. Osteoporos. Int. 16, 417–423. doi:10.1007/S00198-004-1699-X
Jabbar, S., Drury, J., Fordham, J. N., Datta, H. K., Francis, R. M., and Tuck, S. P. (2011). Osteoprotegerin, RANKL and Bone Turnover in Postmenopausal Osteoporosis. J. Clin. Pathol. 64, 354–357. doi:10.1136/jcp.2010.086595
Jacome-Galarza, C. E., Percin, G. I., Muller, J. T., Mass, E., Lazarov, T., Eitler, J., et al. (2019). Developmental Origin, Functional Maintenance and Genetic Rescue of Osteoclasts. Nature 568, 541–545. doi:10.1038/s41586-019-1105-7
Jähn-Rickert, K., Wölfel, E. M., Jobke, B., Riedel, C., Hellmich, M., Werner, M., et al. (2020). Elevated Bone Hardness under Denosumab Treatment, with Persisting Lower Osteocyte Viability during Discontinuation. Front. Endocrinol. 11, 250. doi:10.3389/fendo.2020.00250
Jiang, L.-S., Zhang, Z.-M., Jiang, S.-D., Chen, W.-H., and Dai, L.-Y. (2008). Differential Bone Metabolism between Postmenopausal Women with Osteoarthritis and Osteoporosis. J. Bone Min. Res. 23, 475–483. doi:10.1359/JBMR.071114
Jilka, R. L., Weinstein, R. S., Bellido, T., Roberson, P., Parfitt, A. M., and Manolagas, S. C. (1999). Increased Bone Formation by Prevention of Osteoblast Apoptosis with Parathyroid Hormone. J. Clin. Invest. 104, 439–446. doi:10.1172/JCI6610
Jinnai, H., Watashiba, H., Kajihara, T., Nishikawa, Y., Takahashi, M., and Ito, M. (2002). Surface Curvatures of Trabecular Bone Microarchitecture. Bone 30, 191–194. doi:10.1016/S8756-3282(01)00672-X
Jobke, B., Milovanovic, P., Amling, M., and Busse, B. (2014). Bisphosphonate-osteoclasts: Changes in Osteoclast Morphology and Function Induced by Antiresorptive Nitrogen-Containing Bisphosphonate Treatment in Osteoporosis Patients. Bone 59, 37–43. doi:10.1016/j.bone.2013.10.024
Jørgensen, L., Hansen, J.-B., Brox, J., Mathiesen, E., Vik, A., and Jacobsen, B. K. (2011). Serum Osteoprotegerin Levels Are Related to Height Loss: The Tromsø Study. Eur. J. Epidemiol. 26, 305–312. doi:10.1007/s10654-011-9555-8
Jørgensen, L., Vik, A., Emaus, N., Brox, J., Hansen, J.-B., Mathiesen, E., et al. (2009). Bone Loss in Relation to Serum Levels of Osteoprotegerin and Nuclear Factor-Κb Ligand: the Tromsø Study. Osteoporos. Int. 21, 931–938. doi:10.1007/S00198-009-1035-6
Kameo, Y., Miya, Y., Hayashi, M., Nakashima, T., and Adachi, T. (2020). In Silico experiments of Bone Remodeling Explore Metabolic Diseases and Their Drug Treatment. Sci. Adv. 6, eaax0938. doi:10.1126/sciadv.aax0938
Kanis, J. A., Norton, N., Harvey, N. C., Jacobson, T., Johansson, H., Lorentzon, M., et al. (2021). SCOPE 2021: a New Scorecard for Osteoporosis in Europe. Arch. Osteoporos. 16, 1–82. doi:10.1007/S11657-020-00871-9
Karadag-Saygi, E., Akyuz, G., Bizargity, P., and Ay, P. (2011). The Effect of Risedronate Treatment on Serum Osteoprotegerin and Bone Marker Levels in Postmenopausal Women with Osteoporosis. Gynecol. Endocrinol. 27, 1033–1036. doi:10.3109/09513590.2011.579657
Khosla, S., Oursler, M. J., and Monroe, D. G. (2012). Estrogen and the Skeleton. Trends Endocrinol. Metabolism 23, 576–581. doi:10.1016/j.tem.2012.03.008
Kim, J. G., Kim, J. H., Kim, J. Y., Ku, S. Y., Jee, B. C., Suh, C. S., et al. (2007). Association between Osteoprotegerin (OPG), Receptor Activator of Nuclear Factor-Κb (RANK), and RANK Ligand (RANKL) Gene Polymorphisms and Circulating OPG, Soluble RANKL Levels, and Bone Mineral Density in Korean Postmenopausal Women. Menopause 14, 913–918. doi:10.1097/GME.0B013E31802D976F
Komarova, S. V., Smith, R. J., Dixon, S. J., Sims, S. M., and Wahl, L. M. (2003). Mathematical Model Predicts a Critical Role for Osteoclast Autocrine Regulation in the Control of Bone Remodeling. Bone 33, 206–215. doi:10.1016/S8756-3282(03)00157-1
Kousteni, S., Bellido, T., Plotkin, L. I., and Bodenner, D. L. (2001). Nongenotropic, Sex-Nonspecific Signaling through the Estrogen or Androgen Receptors Dissociation from Transcriptional Activity. Cell 104, 719–730.
Kovács, B., Vajda, E., and Nagy, E. E. (2019). Regulatory Effects and Interactions of the Wnt and OPG-RANKL-RANK Signaling at the Bone-Cartilage Interface in Osteoarthritis. Ijms 20, 4653. doi:10.3390/ijms20184653
LaCroix, A. Z., Jackson, R. D., Aragaki, A., Kooperberg, C., Cauley, J. A., Chen, Z., et al. (2013). OPG and sRANKL Serum Levels and Incident Hip Fracture in Postmenopausal Caucasian Women in the Women's Health Initiative Observational Study. Bone 56, 474–481. doi:10.1016/j.bone.2013.05.018
Lau, H. H. L., Ho, A. Y. Y., Luk, K. D. K., and Kung, A. W. C. (2004). Transforming Growth Factor-1 Gene Polymorphisms and Bone Turnover, Bone Mineral Density and Fracture Risk in Southern Chinese Women. Calcif. Tissue Int. 74, 516–521. doi:10.1007/S00223-004-0163-4
Lavaill, M., Trichilo, S., Scheiner, S., Forwood, M. R., Cooper, D. M. L., and Pivonka, P. (2020). Study of the Combined Effects of PTH Treatment and Mechanical Loading in Postmenopausal Osteoporosis Using a New Mechanistic PK-PD Model. Biomech. Model Mechanobiol. 19, 1765–1780. doi:10.1007/S10237-020-01307-6
Lee, N.-K., Zhang, Y., Su, Y., Bidlingmaier, S., Sherbenou, D. W., Ha, K. D., et al. (2018). Cell-type Specific Potent Wnt Signaling Blockade by Bispecific Antibody. Sci. Rep. 8, 1–16. doi:10.1038/s41598-017-17539-z
Lello, S., Capozzi, A., and Scambia, G. (2017). The Tissue-Selective Estrogen Complex (Bazedoxifene/Conjugated Estrogens) for the Treatment of Menopause. Int. J. Endocrinol. 2017, 1–9. doi:10.1155/2017/5064725
Lemaire, V., Tobin, F. L., Greller, L. D., Cho, C. R., and Suva, L. J. (2004). Modeling the Interactions between Osteoblast and Osteoclast Activities in Bone Remodeling. J. Theor. Biol. 229, 293–309. doi:10.1016/j.jtbi.2004.03.023
Lerebours, C., Buenzli, P. R., Scheiner, S., and Pivonka, P. (2015). A Multiscale Mechanobiological Model of Bone Remodelling Predicts Site-specific Bone Loss in the Femur during Osteoporosis and Mechanical Disuse. Biomech. Model Mechanobiol. 15, 43–67. doi:10.1007/S10237-015-0705-X
Levels of Evidence Working Group (2011). The Oxford Levels of Evidence 2. Oxf. Centre Evidence-Based Med.
Lewiecki, E. M. (2014). Role of Sclerostin in Bone and Cartilage and its Potential as a Therapeutic Target in Bone Diseases. Ther. Adv. Musculoskelet. 6, 48–57. doi:10.1177/1759720X13510479
Li, H.-D., Yang, Y.-H., Li, B., Dai, L.-Y., and Jiang, L. S. (2011). Comparative Analysis of Serum Sex Hormone-Binding Globulin Levels between Postmenopausal Female Patients with Osteoarthritis and Osteoporosis. J. Shanghai Jiaot. Univ. Sci. 31, 1134. doi:10.3969/J.ISSN.1674-8115.2011.08.020
Manolagas, S. C. (2000). Birth and Death of Bone Cells: Basic Regulatory Mechanisms and Implications for the Pathogenesis and Treatment of Osteoporosis*. Endocr. Rev. 21, 115–137. doi:10.1210/edrv.21.2.0395
Marques, E. A., Mota, J., Viana, J. L., Tuna, D., Figueiredo, P., Guimarães, J. T., et al. (2013). Response of Bone Mineral Density, Inflammatory Cytokines, and Biochemical Bone Markers to a 32-week Combined Loading Exercise Programme in Older Men and Women. Archives Gerontology Geriatrics 57, 226–233. doi:10.1016/j.archger.2013.03.014
Martin, M., Sansalone, V., Cooper, D. M. L., Forwood, M. R., and Pivonka, P. (2020). Assessment of Romosozumab Efficacy in the Treatment of Postmenopausal Osteoporosis: Results from a Mechanistic PK-PD Mechanostat Model of Bone Remodeling. Bone 133, 115223. doi:10.1016/j.bone.2020.115223
Martin, M., Sansalone, V., and Pivonka, P. (2019). Computational Biomechanics for Medicine. Springer, Cham, 85–107.
Martin-Millan, M., Almeida, M., Ambrogini, E., Han, L., Zhao, H., Weinstein, R. S., et al. (2010). The Estrogen Receptor-α in Osteoclasts Mediates the Protective Effects of Estrogens on Cancellous but Not Cortical Bone. Mol. Endocrinol. 24, 323–334. doi:10.1210/me.2009-0354
Martínez-Reina, J., Calvo-Gallego, J. L., and Pivonka, P. (2021). Combined Effects of Exercise and Denosumab Treatment on Local Failure in Post-menopausal Osteoporosis–Insights from Bone Remodelling Simulations Accounting for Mineralisation and Damage. Frontiers 0, 402. doi:10.3389/FBIOE.2021.635056
Martínez-Reina, J., Calvo-Gallego, J. L., and Pivonka, P. (2021). Are Drug Holidays a Safe Option in Treatment of Osteoporosis? - Insights from an In Silico Mechanistic PK-PD Model of Denosumab Treatment of Postmenopausal Osteoporosis. J. Mech. Behav. Biomed. Mater. 113, 104140. doi:10.1016/j.jmbbm.2020.104140
Martínez-Reina, J., and Pivonka, P. (2019). Effects of Long-Term Treatment of Denosumab on Bone Mineral Density: Insights from an In-Silico Model of Bone Mineralization. Bone 125, 87–95. doi:10.1016/j.bone.2019.04.022
Mashavi, M. M., Menaged, M., and Shargorodsky, M. (2017). Circulating Osteoprotegerin in Postmenopausal Osteoporotic Women: Marker of Impaired Glucose Regulation or Impaired Bone Metabolism. Menopause 24, 1264–1268. doi:10.1097/GME.0000000000000914
May, M. (2019). Clinical Trial Costs Go under the Microscope. Nat. Med. doi:10.1038/d41591-019-00008-7
McCloskey, E., Rathi, J., Heijmans, S., Blagden, M., Cortet, B., Czerwinski, E., et al. (2021). The Osteoporosis Treatment Gap in Patients at Risk of Fracture in European Primary Care: a Multi-Country Cross-Sectional Observational Study. Osteoporos. Int. 32, 251–259. doi:10.1007/S00198-020-05557-Z
McDonald, M. M., Khoo, W. H., Ng, P. Y., Xiao, Y., Zamerli, J., Thatcher, P., et al. (2021). Osteoclasts Recycle via Osteomorphs during RANKL-Stimulated Bone Resorption. Cell 184, 1330–1347. doi:10.1016/j.cell.2021.02.002
Messalli, E. M. G., Mainini, G., Scaffa, C., Cafiero, A., Salzillo, P. L., Ragucci, A., et al. (2007). Raloxifene Therapy Interacts with Serum Osteoprotegerin in Postmenopausal Women. Maturitas 56, 38–44. doi:10.1016/j.maturitas.2006.05.007
Mezquita-Raya, P., de la Higuera, M., García, D. F., Alonso, G., Ruiz-Requena, M. E., de Dios Luna, J., et al. (2005). The Contribution of Serum Osteoprotegerin to Bone Mass and Vertebral Fractures in Postmenopausal Women. Osteoporos. Int. 16, 1368–1374. doi:10.1007/S00198-005-1844-1
Miller, P. D., Recker, R. R., Reginster, J.-Y., Riis, B. J., Czerwinski, E., Masanauskaite, D., et al. (2012). Efficacy of Monthly Oral Ibandronate Is Sustained over 5 years: the MOBILE Long-Term Extension Study. Osteoporos. Int. 23, 1747–1756. doi:10.1007/S00198-011-1773-0
Milovanovic, P., Rakocevic, Z., Djonic, D., Zivkovic, V., Hahn, M., Nikolic, S., et al. (2014). Nano-structural, Compositional and Micro-architectural Signs of Cortical Bone Fragility at the Superolateral Femoral Neck in Elderly Hip Fracture Patients vs. Healthy Aged Controls. Exp. Gerontol. 55, 19–28. doi:10.1016/j.exger.2014.03.001
Mödder, U. I., Roforth, M. M., Hoey, K., McCready, L. K., Peterson, J. M., Monroe, D. G., et al. (2011). Effects of Estrogen on Osteoprogenitor Cells and Cytokines/bone-Regulatory Factors in Postmenopausal Women. Bone 49, 202–207. doi:10.1016/j.bone.2011.04.015
Molnár, I., Bohaty, I., and Somogyiné-Vári, É. (2014). IL-17A-mediated sRANK Ligand Elevation Involved in Postmenopausal Osteoporosis. Osteoporos. Int. 25, 783–786. doi:10.1007/S00198-013-2548-6
Mullender, M. G., Tan, S. D., Vico, L., Alexandre, C., and Klein-Nulend, J. (2005). Differences in Osteocyte Density and Bone Histomorphometry between Men and Women and between Healthy and Osteoporotic Subjects. Calcif. Tissue Int. 77, 291–296. doi:10.1007/S00223-005-0043-6
Nakamura, T., Imai, Y., Matsumoto, T., Sato, S., Takeuchi, K., Igarashi, K., et al. (2007). Estrogen Prevents Bone Loss via Estrogen Receptor α and Induction of Fas Ligand in Osteoclasts. Cell 130, 811–823. doi:10.1016/j.cell.2007.07.025
National Center for Biotechnology Information (2022a). PubChem Compound Summary for CID 5870 Estrone. https://pubchem.ncbi.nlm.nih.gov/compound/Estrone Accessed June 30, 2022.
National Center for Biotechnology Information (2022b). PubChem Compound Summary for CID 5757, Estradiol. https://pubchem.ncbi.nlm.nih.gov/compound/Estradiol Accessed June 30, 2022.
National Center for Biotechnology Information (2022c). PubChem Compound Summary for CID 155011957, TGF-Beta. https://pubchem.ncbi.nlm.nih.gov/compound/TGF-Beta Accessed June 30, 2022.
Nikolova-Simons, M., Golas, S. B., den Buijs, J. o., Palacholla, R. S., Garberg, G., Orenstein, A., et al. (2021). A Randomized Trial Examining the Effect of Predictive Analytics and Tailored Interventions on the Cost of Care. npj Digit. Med. 4, 1–10. doi:10.1038/s41746-021-00449-w
Nishikawa, Y., Jinnai, H., Koga, T., Hashimoto, T., and Hyde, S. T. (1998). Measurements of Interfacial Curvatures of Bicontinuous Structure from Three-Dimensional Digital Images. 1. A Parallel Surface Method. Langmuir 14, 1242–1249. doi:10.1021/LA970868B
Oh, K. W., Rhee, E. J., Lee, W. Y., Kim, S. W., Oh, E. S., Baek, K. H., et al. (2004). The Relationship between Circulating Osteoprotegerin Levels and Bone Mineral Metabolism in Healthy Women. Clin. Endocrinol. 61, 244–249. doi:10.1111/J.1365-2265.2004.02090.X
Orwoll, E. S., McClung, M. R., Oviatt, S. K., Recker, R. R., and Weigel, R. M. (1989). Histomorphometric Effects of Calcium or Calcium Plus 25-hydroxyvitamin D3 Therapy in Senile Osteoporosis. J. Bone Min. Res. 4, 81–88. doi:10.1002/JBMR.5650040112
Ott, S. M., Oleksik, A., Lu, Y., Harper, K., and Lips, P. (2009). Bone Histomorphometric and Biochemical Marker Results of a 2-Year Placebo-Controlled Trial of Raloxifene in Postmenopausal Women. J. Bone Min. Res. 17, 341–348. doi:10.1359/jbmr.2002.17.2.341
Ouyang, P.-C., Hsieh, C.-Y., and Chow, S.-N. (1984). Serum Total Estrogen Levels and Urinary Excretion of Calcium and Hydroxyproline in Premenopausal and Postmenopausal Chinese Women. Asia Ocean. J. Obstet. Gynaecol. 10, 467–471. doi:10.1111/J.1447-0756.1984.TB00713.X
Pacifici, R. (2008). Estrogen Deficiency, T Cells and Bone Loss. Cell. Immunol. 252, 68–80. doi:10.1016/j.cellimm.2007.06.008
Parfitt, A. M., Glorieux, F. H., Kanis, J. A., Malluche, H., Meunier, P. J., Ott, S. M., et al. (1987). Bone Histomorphometry: Standardization of Nomenclature, Symbols, and Units. Report of the ASBMR Histomorphometry Nomenclature Committee. J. Bone Min. Res. 2, 595–610. doi:10.1002/jbmr.5650020617
Parfitt, A. M. (1994). Osteonal and Hemi-Osteonal Remodeling: The Spatial and Temporal Framework for Signal Traffic in Adult Human Bone. J. Cell. Biochem. 55, 273–286. doi:10.1002/jcb.240550303
Pastrama, M.-I., Scheiner, S., Pivonka, P., and Hellmich, C. (2018). A Mathematical Multiscale Model of Bone Remodeling, Accounting for Pore Space-specific Mechanosensation. Bone 107, 208–221. doi:10.1016/j.bone.2017.11.009
Peng, J., Dong, Z., Hui, Z., Aifei, W., Lianfu, D., and Youjia, X. (2021). Bone Sclerostin and Dickkopf-Related Protein-1 Are Positively Correlated with Bone Mineral Density, Bone Microarchitecture, and Bone Strength in Postmenopausal Osteoporosis. BMC Musculoskelet. Disord. 22, 1–8. doi:10.1186/S12891-021-04365-8
Pfeilschifter, J., Diel, I., Scheppach, B., Bretz, A., Krempien, R., Erdmann, J., et al. (1998). Concentration of Transforming Growth Factor Beta in Human Bone Tissue: Relationship to Age, Menopause, Bone Turnover, and Bone Volume. J. Bone Min. Res. 13, 716–730. doi:10.1359/jbmr.1998.13.4.716
Pino, A. M., Ríos, S., Astudillo, P., Fernández, M., Figueroa, P., Seitz, G., et al. (2010). Concentration of Adipogenic and Proinflammatory Cytokines in the Bone Marrow Supernatant Fluid of Osteoporotic Women. J. Bone Min. Res. 25, 492–498. doi:10.1359/jbmr.090802
Pivonka, P., Buenzli, P. R., Scheiner, S., Hellmich, C., and Dunstan, C. R. (2013). The Influence of Bone Surface Availability in Bone Remodelling-A Mathematical Model Including Coupled Geometrical and Biomechanical Regulations of Bone Cells. Eng. Struct. 47, 134–147. doi:10.1016/j.engstruct.2012.09.006
Pivonka, P., Zimak, J., Smith, D. W., Gardiner, B. S., Dunstan, C. R., Sims, N. A., et al. (2008). Model Structure and Control of Bone Remodeling: A Theoretical Study. Bone 43, 249–263. doi:10.1016/j.bone.2008.03.025
Pivonka, P., Zimak, J., Smith, D. W., Gardiner, B. S., Dunstan, C. R., Sims, N. A., et al. (2010). Theoretical Investigation of the Role of the RANK-RANKL-OPG System in Bone Remodeling. J. Theor. Biol. 262, 306–316. doi:10.1016/j.jtbi.2009.09.021
Poornima, I. G., Mackey, R. H., Buhari, A. M., Cauley, J. A., Matthews, K. A., and Kuller, L. H. (2014). Relationship between Circulating Serum Osteoprotegerin and Total Receptor Activator of Nuclear κ-B Ligand Levels, Triglycerides, and Coronary Calcification in Postmenopausal Women. Menopause 21, 702–710. doi:10.1097/GME.0000000000000127
Qiu, S., Phipps, R. J., Ebetino, F. H., Palnitkar, S., and Sudhaker Rao, D. (2010). Effect of Risedronate on Osteocyte Viability and Bone Turnover in Paired Iliac Bone Biopsies from Early Postmenopausal Women. Calcif. Tissue Int. 87, 392–397. doi:10.1007/S00223-010-9411-Y
Qiu, S., Rao, D. S., Palnitkar, S., and Parfitt, A. M. (2003). Reduced Iliac Cancellous Osteocyte Density in Patients with Osteoporotic Vertebral Fracture. J. Bone Min. Res. 18, 1657–1663. doi:10.1359/jbmr.2003.18.9.1657
Qiu, Z.-Y., Cui, Y., and Wang, X.-M. (2019). “Natural Bone Tissue and its Biomimetic,”. Editors Z-Y. Qiu, X-M. Wang, and H. Cui (Kidlington, United Kingdom: Duxford), 1, 1–22. doi:10.1016/b978-0-08-102717-2.00001-1
Rahnama, M., Jastrzębska-Jamrogiewicz, I., Jamrogiewicz, R., Nogalski, A., and Jagielak, M. (2013). Influence of Hormone Replacement Therapy on Osteoprotegerin and Receptor Activator of Nuclear Factor Kappa-B Ligand Concentrations in Menopausal Women. J. Interferon & Cytokine Res. 33, 485–492. doi:10.1089/jir.2012.0149
Recker, R., Dempster, D., Langdahl, B., Giezek, H., Clark, S., Ellis, G., et al. (2020). Effects of Odanacatib on Bone Structure and Quality in Postmenopausal Women with Osteoporosis: 5-Year Data from the Phase 3 Long-Term Odanacatib Fracture Trial (LOFT) and its Extension. J. Bone Min. Res. 35, 1289–1299. doi:10.1002/JBMR.3994
Recker, R. R., Weinstein, R. S., Chesnut, C. H., Schimmer, R. C., Mahoney, P., Hughes, C., et al. (2004). Histomorphometric Evaluation of Daily and Intermittent Oral Ibandronate in Women with Postmenopausal Osteoporosis: Results from the BONE Study. Osteoporos. Int. 15, 231–237. doi:10.1007/S00198-003-1530-0
Rehman, M. T., Hoyland, J. A., Denton, J., and Freemont, A. J. (1994). Age Related Histomorphometric Changes in Bone in Normal British Men and Women. J. Clin. Pathology 47, 529–534. doi:10.1136/JCP.47.6.529
Reid, I. R., Miller, P. D., Brown, J. P., Kendler, D. L., Fahrleitner-Pammer, A., Valter, I., et al. (2010). Effects of Denosumab on Bone Histomorphometry: The FREEDOM and STAND Studies. J. Bone Min. Res. 25, 2256–2265. doi:10.1002/jbmr.149
Rogers, A., Saleh, G., Hannon, R. A., Greenfield, D., and Eastell, R. (2002). Circulating Estradiol and Osteoprotegerin as Determinants of Bone Turnover and Bone Density in Postmenopausal Women. J. Clin. Endocrinol. Metabolism 87, 4470–4475. doi:10.1210/jc.2002-020396
Rolvien, T., Milovanovic, P., Schmidt, F. N., Kroge, S., Wölfel, E. M., Krause, M., et al. (2020). Long‐Term Immobilization in Elderly Females Causes a Specific Pattern of Cortical Bone and Osteocyte Deterioration Different from Postmenopausal Osteoporosis. J. Bone Min. Res. 35, 1343–1351. doi:10.1002/JBMR.3970
Samelson, E. J., Broe, K. E., Demissie, S., Beck, T. J., Karasik, D., Kathiresan, S., et al. (2008). Increased Plasma Osteoprotegerin Concentrations Are Associated with Indices of Bone Strength of the Hip. J. Clin. Endocrinol. Metab. 93, 1789–1795. doi:10.1210/jc.2007-2492
Sarahrudi, K., Thomas, A., Albrecht, C., and Aharinejad, S. (2012). Strongly Enhanced Levels of Sclerostin during Human Fracture Healing. J. Orthop. Res. 30, 1549–1555. doi:10.1002/jor.22129
Sarrami-Foroushani, A., Lassila, T., MacRaild, M., Asquith, J., Roes, K. C. B., Byrne, J. V., et al. (2021). In-silico Trial of Intracranial Flow Diverters Replicates and Expands Insights from Conventional Clinical Trials. Nat. Commun. 12, 1–12. doi:10.1038/s41467-021-23998-w
Schafer, A. L., Burghardt, A. J., Sellmeyer, D. E., Palermo, L., Shoback, D. M., Majumdar, S., et al. (2013). Postmenopausal Women Treated with Combination Parathyroid Hormone (1-84) and Ibandronate Demonstrate Different Microstructural Changes at the Radius vs. Tibia: The PTH and Ibandronate Combination Study (PICS). Osteoporos. Int. 24, 2591–2601. doi:10.1007/S00198-013-2349-Y
Scheiner, S., Pivonka, P., and Hellmich, C. (2013). Coupling Systems Biology with Multiscale Mechanics, for Computer Simulations of Bone Remodeling. Comput. Methods Appl. Mech. Eng. 254, 181–196. doi:10.1016/j.cma.2012.10.015
Scheiner, S., Pivonka, P., Smith, D. W., Dunstan, C. R., and Hellmich, C. (2014). Mathematical Modeling of Postmenopausal Osteoporosis and its Treatment by the Anti‐catabolic Drug Denosumab. Int. J. Numer. Meth. Biomed. Engng. 30, 1–27. doi:10.1002/CNM.2584
Shaarawy, M., Zaki, S., Sheiba, M., and El-Minawi, A. M. (2003). Circulating Levels of Osteoclast Activating Cytokines, Interleukin-11 and Transforming Growth Factor-Beta2, as Valuable Biomarkers for the Assessment of Bone Turnover in Postmenopausal Osteoporosis. Clin. Lab. 49, 625–636.
Sieburg, H. B., Rezner, B. D., and Muller-Sieburg, C. E. (2011). Predicting Clonal Self-Renewal and Extinction of Hematopoietic Stem Cells. Proc. Natl. Acad. Sci. U.S.A. 108, 4370–4375. doi:10.1073/PNAS.1011414108
Slemenda, C., Hui, S. L., Longcope, C., and Johnston, C. C. (1987). Sex Steroids and Bone Mass. A Study of Changes about the Time of Menopause. J. Clin. Invest. 80, 1261–1269. doi:10.1172/JCI113201
Søe, K. (2020). Osteoclast Fusion: Physiological Regulation of Multinucleation through Heterogeneity-Potential Implications for Drug Sensitivity. Ijms 21, 7717–17. doi:10.3390/IJMS21207717
Suhm, N., Lamy, O., and Lippuner, K. (2008). Management of Fragility Fractures in Switzerland: Results of a Nationwide Survey. Swiss Med. Wkly. 138, 674–683. doi:10.4414/SMW.2008.12294
Sun, T., Wang, X., Liu, Z., Chen, X., and Zhang, J. (2011). Plasma Concentrations of Pro- and Anti-inflammatory Cytokines and Outcome Prediction in Elderly Hip Fracture Patients. Injury 42, 707–713. doi:10.1016/j.injury.2011.01.010
Takakuwa, M., and Iwamoto, J. (2015). Five-Year Experience with Risedronate Therapy for Patients with Increased Fracture Risk: A Practice-Based Observational Study. Biol. Pharm. Bull. 38, 88–95. doi:10.1248/BPB.B14-00592
Tomkinson, A., Reeve, J., Shaw, R. W., and Noble, B. S. (1997). The Death of Osteocytes via Apoptosis Accompanies Estrogen Withdrawal in Human Bone*. J. Clin. Endocrinol. Metab. 82, 3128–3135. doi:10.1210/jcem.82.9.4200
Tourolle, D. (2019). A Micro-scale Multiphysics Framework for Fracture Healing and Bone Remodelling ETH Library. ETH Zurich Res. Collect. doi:10.3929/ethz-b-000364637
Tourolle, D. C., Dempster, D. W., Ledoux, C., Boaretti, D., Aguilera, M., Saleem, N., et al. (2021). Ten‐Year Simulation of the Effects of Denosumab on Bone Remodeling in Human Biopsies. JBMR plus 5, 5. doi:10.1002/JBM4.10494
Tsourdi, E., Makras, P., Rachner, T. D., Polyzos, S., Rauner, M., Mandanas, S., et al. (2019). Denosumab Effects on Bone Density and Turnover in Postmenopausal Women with Low Bone Mass with or without Previous Treatment. Bone 120, 44–49. doi:10.1016/j.bone.2018.10.001
Wanby, P., Nobin, R., Von, S.-P., Brudin, L., and Carlsson, M. (2016). Serum Levels of the Bone Turnover Markers Dickkopf-1, Sclerostin, Osteoprotegerin, Osteopontin, Osteocalcin and 25-hydroxyvitamin D in Swedish Geriatric Patients Aged 75 Years or Older with a Fresh Hip Fracture and in Healthy Controls. J. Endocrinol. Invest. 39, 855–863. doi:10.1007/S40618-015-0421-5
Watts, N. B., Josse, R. G., Hamdy, R. C., Hughes, R. A., Manhart, M. D., Barton, I., et al. (2003). Risedronate Prevents New Vertebral Fractures in Postmenopausal Women at High Risk. J. Clin. Endocrinol. Metab. 88, 542–549. doi:10.1210/jc.2002-020400
Webster, P. (2020). Virtual Health Care in the Era of COVID-19. Lancet 395, 1180–1181. doi:10.1016/S0140-6736(20)30818-7
Weinstein, R. S., Roberson, P. K., and Manolagas, S. C. (2009). Giant Osteoclast Formation and Long-Term Oral Bisphosphonate Therapy. N. Engl. J. Med. 360, 53–62. doi:10.1056/NEJMoa0802633
Wu, X.-Y., Wu, X.-P., Luo, X.-H., Xie, H., Zhang, H., Peng, Y.-Q., et al. (2010). The Relationship between the Levels of Gonadotropic Hormones and OPG, Leptin, TGF-Β1 and TGF-Β2 in Chinese Adult Women. Clin. Chim. Acta 411, 1296–1305. doi:10.1016/j.cca.2010.05.006
Xie, G.-Q., Lei, D.-D., He, H.-B., Gong, J.-J., Chen, C., Chen, P., et al. (2013). Relationship between Serum TGF-Β1, OPG Levels and Osteoporotic Risk in Native Chinese Women. Clin. Chim. Acta 423, 116–121. doi:10.1016/j.cca.2013.04.019
Yoshiji, Y., Miyauchi, A., and Goto, J. (1998). Association of a Polymorphism of the Transforming Growth Factor-Β1 Gene with Genetic Susceptibility to Osteoporosis in Postmenopausal Japanese Women. J. Bone Min. Res. 13, 1569–1576. doi:10.1359/JBMR.1998.13.10.1569
Zhao, R., Wang, X., and Feng, F. (2016). Upregulated Cellular Expression of IL-17 by CD4+ T-Cells in Osteoporotic Postmenopausal Women. Ann. Nutr. Metab. 68, 113–118. doi:10.1159/000443531
Keywords: bone, osteoporosis, aging, agent - based modeling, cell population dynamics, cytokine, parametrization approach, verification and validation
Citation: Ledoux C, Boaretti D, Sachan A, Müller R and Collins CJ (2022) Clinical Data for Parametrization of In Silico Bone Models Incorporating Cell-Cytokine Dynamics: A Systematic Review of Literature. Front. Bioeng. Biotechnol. 10:901720. doi: 10.3389/fbioe.2022.901720
Received: 22 March 2022; Accepted: 13 June 2022;
Published: 12 July 2022.
Edited by:
Fabian Spill, University of Birmingham, United KingdomReviewed by:
Francesco Grassi, Rizzoli Orthopedic Institute (IRCCS), ItalyAbdelwahed Barkaoui, International University of Rabat, Morocco
Copyright © 2022 Ledoux, Boaretti, Sachan, Müller and Collins. This is an open-access article distributed under the terms of the Creative Commons Attribution License (CC BY). The use, distribution or reproduction in other forums is permitted, provided the original author(s) and the copyright owner(s) are credited and that the original publication in this journal is cited, in accordance with accepted academic practice. No use, distribution or reproduction is permitted which does not comply with these terms.
*Correspondence: Caitlyn J. Collins, Y2FpdGx5bi5jb2xsaW5zQGhlc3QuZXRoei5jaA==