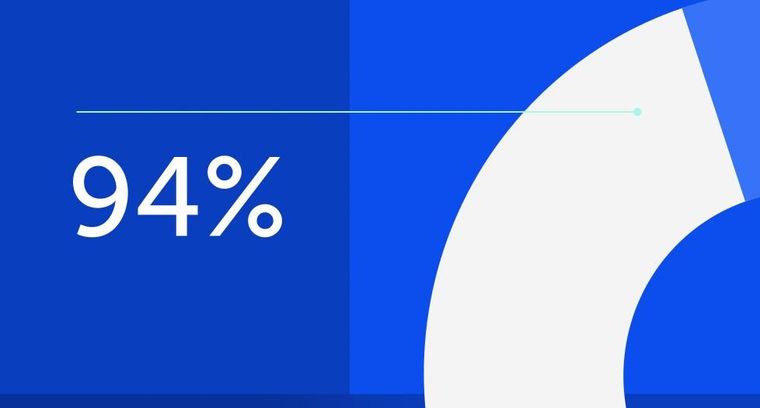
94% of researchers rate our articles as excellent or good
Learn more about the work of our research integrity team to safeguard the quality of each article we publish.
Find out more
REVIEW article
Front. Bioeng. Biotechnol., 15 June 2022
Sec. Cell and Gene Therapy
Volume 10 - 2022 | https://doi.org/10.3389/fbioe.2022.886360
This article is part of the Research TopicAdvanced Cell Culture Technologies to Boost Cell-Based Therapies, Volume IIView all 7 articles
Osteoarthritis (OA), a chronic debilitating joint disease affecting hundreds of million people globally, is associated with significant pain and socioeconomic costs. Current treatment modalities are palliative and unable to stop the progressive degeneration of articular cartilage in OA. Scientific attention has shifted from the historical view of OA as a wear-and-tear cartilage disorder to its recognition as a whole-joint disease, highlighting the contribution of other knee joint tissues in OA pathogenesis. Despite much progress in the field of microfluidic systems/organs-on-a-chip in other research fields, current in vitro models in use do not yet accurately reflect the complexity of the OA pathophenotype. In this review, we provide: 1) a detailed overview of the most significant recent developments in the field of microsystems approaches for OA modeling, and 2) an OA-pathophysiology-based bioengineering roadmap for the requirements of the next generation of more predictive and authentic microscale systems fit for the purpose of not only disease modeling but also of drug screening to potentially allow OA animal model reduction and replacement in the near future.
Osteoarthritis (OA), a chronic degenerative joint disease associated with substantial morbidity, disability, and reduced quality of life is the most common musculoskeletal disease affecting approx. 240 million people worldwide (March et al., 2016). Although OA is characterized by cartilage degeneration, inflammation, (premature) cartilage ageing, chondrocyte senescence, and phenotypic transitions (dedifferentiation and hypertrophic differentiation of chondrocytes), it is a disease of the entire joint (see Figure 1 for an overview), affecting all articular tissues because of their physical and functional association (Loeser et al., 2012). Current treatment strategies are only palliative and have little impact on the progressive degeneration of articular cartilage (Barry and Murphy, 2013). Driven by the unmet therapeutic need to reduce or reverse disease progression by either drugs or regenerative tissue engineering approaches, translational disease models for OA are key for the study of disease mechanisms, refinement of diagnostic methods, development of intervention strategies as well as identification of potent and effective disease-modifying therapeutic agents. The current review aims to outline the recent progress in OA modeling in vitro using advanced three-dimensional on-a-chip approaches and to provide a summary of essential aspects of the articular microenvironment and OA pathophysiology as the basis for a technological roadmap for the development of disease-relevant articular and OA joint tissue models in the second part of this review.
FIGURE 1. The fine line of tissue homeostasis in OA. (A) A plethora of structural and biochemical tissue factors such as extra- and pericellular matrix (ECM/PCM) biosynthesis, cytokine expression, protease expression and metabolic activity of cells guide the dysfunctional progression of OA joint tissues. (B) Changes in the distribution, occurrence, and activity of a variety of tissue-specific cell types mediate the structural decline of OA joint tissues with characteristic pathophysiological cell activation and proliferation, synovial lining thickening, and cell clone formation/clustering as well as pathological hypervascularization and bone deposition (calcification). Created with permissions from Biorender.com.
Throughout the human body, the three distinct types of skeletal joints are classified as either fibrous joints (synathroses), cartilaginous joints (amphiarthroses), or synovial joints (diarthroses) with their basic function of connecting skeletal parts such as the bone (Lawry and Bewyer, 2010; Zhang et al., 2015). Within the joint, the movements of the bones may be sliding, angular, and external or internal rotation. Among these types, synovial joints are the most complex structures with seven subclasses and a variety of mechanical functions (Lawry and Bewyer, 2010). The joint capsule is filled with synovial fluid (SF) and the synovial membrane continuously extends from the periosteum to the perichondrium secreting molecules such as lubricants into the joint cavity. Articular cartilage provides a smooth yet resilient surface for sliding between bone structures. Ensconced within the extracellular matrix of cartilage is a sparse population of chondrocytes (approx. 2% of the total volume of adult articular cartilage) as the sole resident cell type adapted to the low oxygen levels in its environment. Chondrocytes are phenotypically stable, maturationally arrested differentiated cells that maintain tissue homeostasis by synthesizing a very low level of matrix components to replace damaged matrix molecules, thereby preserving the structural integrity of the cartilage matrix (Goldring et al., 2011; Gilbert et al., 2021). Within their ECM, chondrocytes are surrounded by a narrow (2–4 μm thick) pericellular matrix (PCM) that is both biochemically and biomechanically (Young’s modulus 23–59 kPa) distinct from the ECM (Young’s modulus ≈500 kPa) and together with the ensconced cells is referred to as a chondron (Mow and Guo, 2002; Guilak et al., 2005; Gao et al., 2014; Chery et al., 2021). The extra- and pericellular matrix zones transmit the depth-dependent dynamic mechanical stimuli, comprising a combination of compression, hydrostatic pressure, shear stress, osmotic stress, and tensile strain, to the mechanosensitive chondrocytes, which in turn adjust cartilage metabolism depending on the magnitude, frequency, strain rate and nature of the applied load (Natenstedt et al., 2015; Gilbert et al., 2021; Statham et al., 2021). The bidirectional reciprocity in cartilage mechano-signaling enables chondrocytes to sense load application, including altered loading patterns, and in turn adjust matrix composition in response to mechanical cues. In this context, the PCM is pivotal in modulating the mechanical environment of the chondrocyte and regulating mechanotransduction in chondrocytes by transmitting biomechanical, biophysical, and biological signals between the ECM and chondrocytes (Mow and Guo, 2002; Gao et al., 2014; Chery et al., 2021). Based on the collagen fiber arrangement, distinct zonal chondrocyte phenotype, the density of proteoglycans, and expression of zone-specific markers, mature articular cartilage can be structurally and functionally divided into three distinct zones being the superficial (tangential) zone, the middle (transitional) zone, and the deep (radial) zone. A perpendicular tidemark region integrates the hyaline cartilage layer with the underlying calcified cartilage and subchondral bone (Bonnet and Walsh, 2005; Chauffier et al., 2012; Candela et al., 2016; Broeren et al., 2019). The subchondral bone is important for cartilage homeostasis as the stiffness of the underlying subchondral bone severely affects chondrocyte mechanosignaling (Carter et al., 2004; Zhen et al., 2021).
Reflecting on what has been mentioned earlier, successful recapitulation of an authentic joint environment in vitro needs to take many factors into account including general anatomical and structural as well as cellular, molecular, and biophysical properties.
Having established on the overall joint anatomy, mechanics, and function, the role of intra-articular pressure (IAP) and the role of synovial fluid will be briefly outlined in the following. As hyaline cartilage is avascular, aneural, and alymphatic, synovial fluid and adjacent tissues provide its nutrient and oxygen supply and waste removal via loading-dependent transport through the ECM (pore size 2–10 nm) (Chahine et al., 2005), resulting in an oxygen gradient of 9%–2% and an osmolarity of 350–450 mOsm (Brighton et al., 1971; Urban et al., 1993; Rajpurohit et al., 1996; Sieber et al., 2020). Synovial fluid is a viscoelastic ultrafiltrate of plasma through the semipermeable synovial membrane supplemented with macromolecules secreted by synoviocytes (Levick and McDonald, 1995; Freemont, 1996; Sabaratnam et al., 2005; Blewis et al., 2007). Due to the plasma ultrafiltration, which allows proteins to cross only to a limited extent, physiological SF total protein (TP) concentration is approximately 25%–35% of the plasma protein concentration, while the glucose and electrolyte concentrations are similar to plasma (Weinberger and Simkin, 1989; Gobezie et al., 2007; Ritter et al., 2013). In healthy knee joints, the synovial fluid pressure is similar to the negative subatmospheric IAP of around −3 mmHg (Jayson and St Dixon, 1970; Blake et al., 1989) and stabilizes the whole joint keeping tissue portions in place. During exercise, the biomechanical forces on anatomical knee structures can almost triple and consequently lead to a rise in IAP above the capillary perfusion pressure (Kutzner et al., 2010) as well as shear force generation of around 20–30 dyn/cm2 (Tirtaatmadja et al., 1984; Hlaváček, 1995; Levick and McDonald, 1995; Schett et al., 2001). In turn, this impedes synovial perfusion via blood vessels and results in a more hypoxic environment during activity (Geborek et al., 1989). A rise in IAP as well as hypoxia can be also observed in OA patients. In addition, fluid pressure turbulences caused by fluid shear at the endothelial cell surface can promote inflammatory escalation (Albarrán-Juárez et al., 2018).
The synovial membrane, which produces and maintains the specialized physical, cellular, and biochemical synovial environment, consists of two distinct layers: the synovium intima or lining, which is comprised of secretory fibroblast-like synoviocytes (FLS, 70–90% of the total cell population) and macrophage-like synoviocytes (MLS), and the underlying synovium subintima or sublining with an extensive system of lymphatics for clearance of transported molecules (Xu et al., 2003; Blom et al., 2004; Blewis et al., 2007; Hirschmann et al., 2007; Kiener et al., 2010; Zhang et al., 2019; Onuora, 2020). Both synoviocyte types are mechanosensitive and mechanoresponsive and exposed to a dynamic environment of mechanical stimuli including fluid- and contact-induced shear stress (Estell et al., 2017; Han et al., 2020; Thomson and Hilkens, 2021). Indeed, biomechanical stimuli, such as fluid-induced shear stress have been shown to influence FLS biosynthesis and modulate the effect of cytokines on FLS production of cartilage degrading enzymes (Estell et al., 2017; Han et al., 2020; Thomson and Hilkens, 2021). Similarly, mechanical cues are reported to guide macrophage activation and polarization as well as macrophage-chondrocyte cross-talk and to act as an immunomodulatory stimulus for macrophages (Estell et al., 2017; Han et al., 2020). Consequently, fluid pressure, fluid shear, as well as turbulences within the joint microenvironment can significantly influence the physiology and pathological progression of many musculoskeletal cell types including synoviocytes, endothelial cells, and chondrocytes.
This means that reengineering an authentic biophysical niche of a joint must consider many biophysical principles to create an in vivo-like environment for in vitro cell cultures.
Overall, the plethora of tissue types with distinct physical, mechanical, cellular, biochemical, and structural properties are conflating to create the complex microenvironment of a joint. This simple fact already teases that a single tissue-centered view cannot be a successful strategy to investigate a multifactorial musculoskeletal disease such as OA. The pathogenesis of OA is multifaceted, involving mechanical, cellular, and molecular processes, inflammation, metabolic dysfunction, and epigenetic modifications, and is orchestrated by cellular crosstalk of chondrocytes, synovial macrophages and fibroblasts, osteocytes, and infiltrating leukocytes, as well as alterations in the extracellular matrix (ECM) of articular tissues and synovial fluid composition (Loeser et al., 2012; Sellam and Berenbaum, 2013; Raman et al., 2018). OA can occur as a result of a variety of predisposing factors such as age, mechanical injury, genetics, gender, metabolic dysfunction, and obesity that incite a cascade of pathophysiological events within articular tissues (Loeser et al., 2012; Barboza et al., 2017). Irrespective of the initiating factors, the pathological progression of OA follows a consistent pattern (Goldring et al., 2011), indicating that a common molecular pathway [i.e., canonical NF-kB pathway (Marcu et al., 2010; Pichler et al., 2021, 2022)] links the biochemical and biomechanical processes that underlie the onset and progression of OA. Cartilage ECM debris caused by age-related wear or trauma is released into the synovial microenvironment activating synovial macrophages, synovial fibroblasts, and chondrocytes to produce inflammatory and catabolic mediators, which in turn disrupt cellular homeostasis and the balance between matrix synthesis and degradation in both tissues. Eventually, this creates a vicious cycle of tissue inflammation and breakdown (Barry and Murphy, 2013; Barboza et al., 2017). The normally quiescent chondrocytes, become activated and undergo a phenotypic shift characterized by cell proliferation, cluster formation, increased production of both extracellular matrix proteins and matrix-degrading enzymes, and hypertrophic differentiation (Barry and Murphy, 2013; Barboza et al., 2017). Exposure to inflammatory and oxidative mediators also enhances premature stress-induced senescence and ageing of chondrocytes resulting in an accumulation of senescent cells in the superficial layer of the articular cartilage. These cells in turn secrete a variety of inflammatory cytokines and matrix-degrading proteases linked to a senescence-associated secretory phenotype (SASP) (Bonnet and Walsh, 2005), which influences cell plasticity and propagates senescence and inflammation in surrounding cells and tissues. As an additional contributor, the subchondral bone is a source of inflammatory mediators implicated in clinical OA pain, hypertrophic differentiation of chondrocytes, and the degradation of the deep layer of cartilage, and it is involved in the abnormal distribution of stress on the bone-cartilage interface secondary to sclerosis and remodeling of the subchondral bone (Sellam and Berenbaum, 2013; Boris Chan et al., 2015; Aho et al., 2017; Hügle and Geurts, 2017). Recently, also adipose tissue as well as the synovial membrane are gaining more and more attention as significant contributors to the overall degradative and inflammatory biochemical microenvironment.
Building from the fact that this joint milieu is orchestrated by intricate cross-talk of a variety of different joint tissues, the inclusion of more sources of a pro and antiinflammatory mediator can and will shed more light on the contribution and mode of actions of individual tissues during onset as well as the progression of OA.
Another global factor of OA is the alteration of matrix biosynthesis and pathological matrix remodeling of a variety of tissues including cartilage, synovial membrane as well as subchondral bone. The ECM is key in mechanosignaling and mechanosensory regulation of matrix biosynthesis as well as pro and antiinflammatory processes. It has to be noted that the PCM as the closest cell interface is not a unique feature of chondrocytes even though most reported. PCM changes also correlate with loss of mechanotransduction activity in aging bone (Hagan et al., 2020), as well as fibrotic malformation of adipose (Divoux et al., 2010) and synovial tissue (Watson et al., 2011). Downregulation of matrix constituents such as perlecan and collagen VI further reduces PCM stiffness, which correlates with clone formation in the vicinity of cartilage defects (Foldager et al., 2014; Zelenski et al., 2015). OA alters the stiffness of the PCM (Zelenski et al., 2015; Danalache et al., 2019). These compositional changes can alter cell volume and morphology (Hall, 2019), TGF-β activation (Zhen et al., 2021) and proinflammatory cytokine and protease expression (Candela et al., 2016). An amplification of pathological mechanosignaling-related processes is further enhanced by overexpression of integrins due to mechanical overloading (Lucchinetti et al., 2004). Potentially, alterations in cell metabolism caused by dysfunctional matrix mechanosensing as described earlier further contribute to a more hypoxic synovial fluid environment that via hypoxia-induced oxidative stress lead to alterations in cell metabolism and activity (e.g., anaerobic glycolysis of chondrocytes, overproliferation, an increase of matrix biosynthesis, higher susceptibility to proinflammatory molecules, etc.) (Fermor et al., 2007; Mobasheri et al., 2017; Munjal et al., 2019). To give justice to the ultrastructural inadequacies of joint tissues in OA, in vitro models that claim to recapitulate matrix-related aspects should not be one-sided and focus on just a single aspect of matrix biology. The synergistic interplay between matrix biosynthesis, mechanosensation and cells as the three main pillars of dysfunctional matrix mechanobiology in OA must be accounted for in OA for the entire set of joint tissues to overcome the limitations of the current research approaches.
Overall, all the above physiological and pathobiological considerations at the tissue, cellular and molecular levels must be considered vital to develop an authentic microenvironmental niche. The implementation of these bioengineering parameters will in turn improve the predictiveness and authenticity of OA in vitro models. This is also evident as decades of cause-effect in vitro research analysis, i.e., proinflammatory cytokine secretion after a molecular OA stimulus has not resulted in any significant break-through innovation in disease therapy and tissue regeneration.
It is well-known nowadays that traditional two- dimensional (2D) culture models fail to replicate proper cell-cell and cell-matrix interactions necessary to mimic disease pathophysiology and are further limited by aberrant cell morphology, polarity, gene expression, and overall cell phenotype. Due to the lack of translational power, two-dimensional (2D) models are being progressively replaced by three-dimensional (3D) culture systems. Pellet, tissue explant, and micromass cultures more accurately mimic the native microenvironment found in musculoskeletal as well as any other tissue type. Explant models provide native tissue architecture and in vivo-like cell composition and thus feature the most authentic and “natural” microenvironment. Explant mono- and cocultures of equine (Haltmayer et al., 2019; Anderson et al., 2020) and porcine (Ding et al., 2014; Vernon et al., 2014) as well as human tissues (Topoluk et al., 2018; Dolzani et al., 2019) have been used to elaborate on the structure-function relationship between tissue architecture, the extracellular matrix composition and cell phenotypes in OA pathophysiology, but they are difficult to standardize due to the large interindividual variation. To improve standardization and thus comparability of data, pellet and micromass cultures, with and without natural (e.g., alginate, hyaluronan, collagen) or synthetic [e.g., polylactic acid, poly(ethyelene glycol)‐terephthalate] hydrogel-based scaffolds, have been developed (Smeriglio et al., 2015; Mouser et al., 2020). Thus, pellet cultures allowed, for example, to investigate the impact of disease-promoting factors, such as galectins, on ECM degradation via MMPs in the 3D context (Pichler et al., 2021, Pichler et al., 2022). Indeed, in 3D hydrogel culture, chondrocytes achieved near-native gene expression, chondral metabolism, and ECM turnover (Smeriglio et al., 2015) although the mechanical modulus of currently available hydrogels in the kPa magnitude range does not match the compression modulus of 9–13 MPa of healthy human cartilage tissue disks (Roberts et al., 1986; Cloyd et al., 2007; Guo et al., 2017). Moreover, 3D OA cartilage constructs in a poly(ethylene glycol)‐chondroitin sulfate hydrogel scaffold also exhibited pathological alterations in matrix biosynthesis such as decrease of COL2A1 expression, reduced glycosaminoglycan (GAG) content, and loss of compressive construct modulus (kPa) consistent with native OA samples when challenged with an inflammatory stimulus. Similarly, synovial micromass technologies comprising synovial fibroblasts, CD14+ monocytes and CD68+ macrophages (macrophage-like synoviocytes) demonstrated near-native cell composition and inflammatory response of the synovial intimal layer with increased proinflammatory cytokine expression, loss of antiinflammatory M2 macrophage phenotype, and synovial membrane hyperplasia when challenged with a proinflammatory stimulus (Broeren et al., 2019). Furthermore, 3D cocultures of synovial fibroblasts and endothelial cells have been established to model the contribution of synovial neoangiogenesis (Maracle et al., 2017), and even more complex 3D tricultures (e.g., including chondrocytes, synovial fibroblasts, and macrophages) modeled pathological chondrocyte activation and cartilage destruction in arthritis (Peck et al., 2018). Overall, primitive homo and heterotypic 3D culture techniques have given important insights into the complex structure-function relationship that influences matrix biosynthesis as well as the activity of any cell type within the joint tissue microenvironment.
As outlined earlier, the physiology of a joint requires not only structural and cellular cues but also a variety of biomechanical forces for cells and tissues to maintain homeostasis. On the one hand, 3D printed zonal cell–scaffold structures aim to recapitulate physiological zonal cell distributions by either seeding cells directly on printed scaffolds or printing materials with encapsulated cells have been developed (Guo et al., 2017; Mouser et al., 2020). This creates better architectural control over the biomechanical properties such as matrix stiffness and hardness to recapitulate the anisotropy of material properties within even a single joint tissue type. On the other hand, the integration within mechanical bioreactors allows explants or tissue-engineered constructs to investigate the impact of fluid flow, compressive loading, and other important biomechanical forces (see Figure 2) and to look into matrix catabolism, metabolic cell activation as well as inflammatory and nociceptive signaling (Fermor et al., 2002; Piscoya et al., 2005; Chauffier et al., 2012). Mechanical bioreactors have been used routinely to improve the chondrogenic microenvironment for stem cell-based systems as well as primary chondrocyte models (Fu et al., 2021). More authentic multidimensional actuation principles combining shear and compression can further approximate in vivo chondrocyte matrix biosynthesis as well as lubricin overexpression (Meinert et al., 2017). Simultaneous control over oxygen tension with feedback loops and nitrogen supply can further tune the topography of physiological matrix constituent deposition increasing GAG secretion to the superficial zones of a construct with upregulation of bulk COL2A1 and ACAN expression (Tekari et al., 2020). Overall, research on conventional 3D cultures demonstrated the complex relationship between structural, biochemical as well as biomechanical cues in dysfunctional tissue homeostasis found in OA.
FIGURE 2. The complexity of biomechanical activation. The mechanosensitivity of knee-joint tissues and resident cells is mediated by integrins as well as stretch- and loading-sensitive cell-bound ion channels (mechanosensors) and include a variety of transient receptor potential (TRP) channels, and other mechanosensitive ion channels such as Piezo1 and Piezo 2. These mechanosensors in turn react to a variety of biomechanical forces such as fluid and mechanical shear strain, stretching, and tensile loading as well as compression to either promote joint tissue physiology or escalate the dysfunctional mechanobiology in OA via pathological mechanosignaling. Created with permissions from Biorender.com.
As the most recent advancement in the palette of 3D culture techniques, organ-on-a-chip (OOAC) and microphysiological systems (MPS) aim for an even better recapitulation of a native tissue-like environment, tissue architecture and cell-specific responses relevant to OA modeling by combining stem cell or patient-derived primary cell-based models for cartilage and synovium (Fomby et al., 2010; Smeriglio et al., 2015; Tian et al., 2016; Li et al., 2017; Lin et al., 2019) as well as adipose (Loskill et al., 2017) and bone-like tissues (Mansoorifar et al., 2021; Nasello et al., 2021). In this context, microfluidic technologies can create an even more dynamic yet more controllable musculoskeletal disease microenvironment. Because OA has long been considered a cartilage disease, most microphysiological models of OA still focus on chondrocyte pathobiology. Analogous to traditional 3D cultures, microfluidic cartilage-on-a-chip approaches have demonstrated their ability to recapitulate near-native tissue-like conditions on a structural, architectural, and molecular level (e.g., morphology and matrix biosynthesis, and inflammatory signaling). Biomechanical actuation by compressive loading has also been demonstrated to be a critical parameter for biochip chondrocyte 3D cultures as shown in Figure 3A (Lee et al., 2018). Cell stimulation can be easily performed within biochips using integrated pneumatic deflectable actuator structures. Chondrocytes embedded in hydrogel can in turn be deformed in the presented approach up to 30% cell deformation for either static or cyclic compression routines with no alterations in chondrocyte health. In the same line, dynamic compressive loading in a physiological range (6–10%) improved in vivo like cartilage gene expression, while hyperphysiological compression around 30% loading triggered OA-like chondrocyte responses (Occhetta et al., 2019; Figure 3B). Overloading could significantly reduce aggrecan ACAN gene expression and Collagen II-to-I ratio while increasing gene expression levels for markers involved in chondrocyte hypertrophy and inflammation (i.e., collagen type X, MMP-13, IL-6, and IL-8). To integrate more natural molecule diffusion distances above 500 μm, musculoskeletal tissue models that feature single constructs with macroscopic dimensions have been established. A synovium-on-a-chip system combined with integrated sensors as shown in Figure 4 demonstrated the feasibility of time-resolved multiplex analysis schemes (Rothbauer et al., 2020). The study utilized light scattering to noninvasively probe dynamic synovial tissue-level responses when challenged with proinflammatory cytokines including synovial network architecture remodeling and organoid condensation altered by cadherin-11-mediated cell-cell adhesion. In addition, cartilage-on-a-chip systems as demonstrated by Rosser et al. (2019) can already well resemble cartilage hallmarks of the middle and superficial zone with proper chondrocyte morphology and gene expression while precisely controlling the molecule gradients within cartilage constructs due to the microfluidic flow (see Figure 5). Moreover, the authors used their miniaturized drug screening tool to recover cartilage-specific OA-like inflammation responses with a treatment. Notably, to further investigate and approximate in vivo-like tissue conditions, increase of microfluidic construct diameter and volumes by twofold increased collagen and glycosaminoglycan biosynthesis as well as the corresponding dynamic construct modulus (Tian et al., 2016). Both studies demonstrate well that the geometry and macroscopic dimensions of a construct influence the models’ diffusivity as well as topographical molecule gradients that in turn are vital to control parameters for organoid maturation and native tissue-like physiology.
FIGURE 3. Examples for biomechanically actuated joint-on-a-chip systems. (A) A microfluidic pneumatic-actuation device transmitting multiple mechanical stress conditions on chondrocyte-laden alginate hydrogel to study deriving mechanisms of bone growth. Via a pneumatic channel network, pressurized air deflates a silicone (PDMS) balloon to compress chondrocytes. For instance, hydrogel compression of 34% in the z direction (red fluorescence) leads to a compression of individual chondrocytes within the hydrogel by 16% (green fluorescent cells). Increase of the diameter of the silicone balloon gradually increases cell loading by up to 30% under constant and cyclic loading conditions (see graphs on the right). Reproduced with permissions from (Lee et al., 2018). (B) Development of a cartilage-on-a-chip (left panel) where a 3D cartilage construct (blue compartment) enclosed by two medium supplementation channels (red highlights) is actuated by pneumatic compression of a deflectable membrane (white membrane). Physiological compression of 10% results in a native tissue-like matrix biosynthesis (center panel) comprising high levels of aggrecan (green fluorescence), collagen type I (white fluorescence), and collagen type II (red fluorescence). Analysis of the Collagen type II-to-I ratio which is used as an indicator for cartilaginous matrix approximates native cartilage after 14 days of biomechanical on-chip cultivation. Reproduced with permissions from (Occhetta et al., 2019).
FIGURE 4. Noninvasive biosensor integration enables structural analysis of synovial on-chip organoids. (A) Light scattering measurements were combined with a human synovium-on-a-chip (top panels) to investigate structural alterations in rheumatoid arthritis (RA). (B) Light scatter analysis of on-chip generated untreated synovial organoids shows higher reproducibility than conventional synovial micromasses generated in microtiter plates while (C) retaining their ability to respond to TNF-α stimulation with upregulation of interleukins (IL-6/-8) and proteases (MMP-1/-3) (D) Synovial on-chip organoids showed synovial lining hyperplasia upon TNF-α stimulation characteristic for RA synovial intima. Reproduced with permissions from (Rothbauer et al., 2020).
FIGURE 5. Biochip technologies for native tissue-like molecular gradients. (A) Macroscopic cartilage-on-a-chip system presenting native-like tissue-like molecule diffusion dynamics analyzed by fluid dynamic simulations. (B) Steep nutrient and metabolite gradients do not alter the high chondrocyte viability of chondrocytes (green fluorescent CMFDA assay) and showed (C) cartilage-specific molecular expression levels of matrix and chondrocyte-related genes analyzed by RT-qPCR over 2 weeks of cultivation. Reproduced with permissions from (Rosser et al., 2019).
As outlined before, OA is considered a serious multifactorial disease affecting multiple tissue structures (Dieppe, 1999; Loeser et al., 2012; Andriacchi et al., 2020). Consequently, a deeper understanding of tissue communication is paramount to understand general as well as disease phenotype-specific mechanisms (Mobasheri and Batt, 2016; Mobasheri et al., 2019; Salgado et al., 2021). Combining cartilage-on-a-chip systems with other musculoskeletal tissues such as the synovium or subchondral bone has proven essential in providing a more holistic view of tissue-tissue interactions that may govern OA onset including inflammation, fibrosis, and degradation of joint tissues (Piluso et al., 2019). The impact of synovial secretoma on overall bone cell homeostasis was investigated using a coculture microsystem of human synoviocytes (i.e., SW982 sarcoma cells) with murine preosteoclasts (i.e., RAW264.7) and primary stem cell-derived osteoblasts (Ma et al., 2018). As shown in Figure 6, the migratory behavior analysis of activated synoviocytes towards osteoclasts in the bone compartment can potentially shed light on initial mechanisms of erosion. Integration of synovial with chondral compartments as shown in Figures 7A, B demonstrated that the soluble cell-cell communication by healthy synovial fibroblasts contributes to a more physiological chondrogenic microenvironment (i.e., round chondrocyte morphology with reduced cell activation and dedifferentiation) (Rothbauer et al., 2021). The addition of a chondrogenic differentiation medium induced a fibrosis-like catabolic synovial response with the disintegration of the synovial organoids. The inclusion of monocytes/macrophages, endothelial cells, and physiologically relevant fluid shear conditions within another chondro-synovial biochip shown in Figure 8A mimicked the synovial postcapillary venule. Monocyte chemotaxis and migration from the bloodstream into synovial tissue (Figure 8B) as a model for synovial immune cell infiltration was enhanced when TNF-α treatment was combined with the fluid flow (Mondadori et al., 2021). To demonstrate that nutrient and molecule gradients can be generated in macroscopic multiphasic constructs, 3D-printed multichamber bioreactors were used to bioengineer sophisticated anisotropic osteochondral architectures (Lin et al., 2014). A two-phasic construct was seeded with heterogeneously differentiated hBMSCs to develop prechondral and osseous phenotypes. Tissue-specific hallmarks included a tidemark-like region, anabolic gene expression, and matrix production. When stimulated with IL-1β, the model developed an OA-like tissue response including an expression decrease in chondral markers (e.g., SOX9, COL2A1 and ACAN) in the context of osteochondral tissue-level communication.
FIGURE 6. Compartmentalized organs-on-a-chip feature migration dynamics of an arthritic tissue microenvironment. (A) Using a triple culture biochip, the biochip model was used to analyze FLS migration towards a bone model (bottom panel) to simulate fibroblast invasion mechanisms in bone tissue. (B) The rate of synovial migration was highest for the triple coculture stimulated with Receptor Activator of NF-κB Ligand (RANKL; left graph). An increase in numbers of TRAP-positive catabolic osteoclasts and decrease of anabolic ALP-positive osteoblasts recapitulates in vivo-like processes happening during the synovial bone invasion and bone erosion. Reproduced with permissions from (Ma et al., 2018).
FIGURE 7. Tissue level crosstalk fosters an anabolic and antiinflammatory joint environment. (A) A millimeter-sized 3D coculture model recapitulating only the soluble synovial and chondral tissue-crosstalk was used as an RA model based on healthy cadaveric chondrocytes and RA patient-derived FLS embedded in 3D hydrogels. (B) Analysis of the potentially fibrotic effect of commercial differentiation medium (DM) on condensation analysis of chondral and synovial cocultures. Adapted with permissions from (Rothbauer et al., 2021).
FIGURE 8. Flow regimes alter immune cell migration in more holistic in vitro joint tissue studies. (A) A multicompartment biochip with heterotypic triple cultures comprising articular chondrocytes (blue cells) and an endothelialized synovial membrane model (turquoise endothelium with red FLS) for monitoring of monocyte extravasation and tissue infiltration processes in OA synovial tissue. (B) The combination of flow with synovial fluid and chemokines increases the number of extravasated monocytes within the synovial tissue compartment. Reproduced with permissions from (Mondadori et al., 2021).
For the next technological leap, we advocate a reverse engineering approach following the origins and strengths of organs-on-a-chip technology to recreate authentic organ or tissue-level function and architecture while deliberating on homo- and hetero-typic cell and tissue interactions and also the cellular identity and activation states that altogether regulate important anabolic and catabolic molecular but also structural aspects of tissue homeostasis and function during disease onset and progression.
In general, biomechanical cues including compression, shear, interstitial flow and hydrostatic and osmotic pressure can regulate pro or antiinflammatory responses in a variety of tissues and cell types (see Figure 9) highlighting the necessity for more complex and dynamic culture environments also in vitro (Li et al., 2018; Fahy et al., 2019). Mechanical stimuli provide a proper microenvironmental niche for in vitro disease models. Mechanosensory activation (e.g., Piezos, TRPs or integrins, etc.) during the osteoarthritic onset and progression is a critical, to date largely ignored, mechanosignaling aspect of microsystems, which must be closely investigated (Statham et al., 2021). Consequently, to improve existing models, we propose to apply anatomical and (bio)mechanical considerations for the next-generation microsystems. The structure-function relationship of an articular joint is very complex and multi-faceted and not only includes a variety of cellular and biochemical but also many important biophysical parameters. In addition to nutritional functions, the synovial fluid, as a common biofluid mediating tissue homeostasis and communication, has also an important biophysical and fluid-mechanical function. Because pathological conditions (i.e., the rise of IAP) can be biomechanically and microenvironmentally very similar to healthy exercising conditions, the selection of cells and tissues from disease origin will be critical for an authentic disease pathophenotype. Future joint-on-a-chip models must increase the controllability and precision of fluid-mechanical cues at the microscale. In combination with biomechanical loading, future microphysiological systems can offer better precision and control over spatio-temporal and regional multiparametric mechanical stimulations based on principles well established over two decades of microsystems engineering and microfabrication. In this line of thought, biomechanical cues within microfluidic joint-on-a-chip systems can be potentially further approximated to the biophysical complexity and anisotropy including more in vivo-like force orientation (Paggi et al., 2020) using a combination of multiple actuators working at various dimensional axes. Technical advancement of chip-based mechanostimulation from pneumatic to other actuation approaches will provide a better technological basis to create systems with higher throughput (Qian et al., 2020) including force-time analysis curves of human patients (Phinyomark et al., 2016; Kapri et al., 2021) as control input curve will provide cells with more biomimetic force patterns in vitro.
FIGURE 9. The biomechanical knee-joint environment matters. Synovial joints comprise a very diverse set of heterotypic tissue architectures including the synovium, meniscus, cartilage, and subchondral bone. Similar to their architecture, the respective biomechanical microenvironment during joint movement is tissue-specific and comprises distinct biomechanical principles. Created with permissions from Biorender.com.
The inclusion of a near-native and tissue-specific composition of ECM components including collagens and proteoglycan fillers is key to generating the proper cellular microenvironment found in OA. Collagen fiber arrangement and morphology also affect how the ECM responds mechanically to compressive, shear, hydrostatic, osmotic, and tensile loading. Changes in the composition and stiffness of the extracellular interterritorial, territorial and pericellular matrix (see Figure 10) provide essential cues for ECM-sensitive mechanosensors such as integrins and also connexins to further escalate pathological matrix remodeling towards an OA phenotype. Consequently, future microsystems must consider topographic and regional control over matrix compositions and orientational properties to create a more native pathophysiological template for disease-relevant cell-matrix interactions. The PCM has a pivotal role in the bidirectional reciprocity of cartilage mechanosignaling and homeostasis. Moreover, PCM degeneration is one of the earliest events during OA onset, altering the stress-strain microenvironment of chondrocytes leading to aberrant chondrocyte mechanotransduction (Gao et al., 2014; Gilbert et al., 2021). Microphysiological systems must start to recreate PCM and ECM compositions and architectures that are seen in actual OA tissues. Novel microencapsulation techniques could be modified to generate chondron-like structures that simulate the microenvironment of chondrocytes (Li et al., 2019). Furthermore, to recapitulate the native niche, attention needs to be paid to the hydraulic permeability coefficient, which governs fluid movement in cartilage loaded in compression and is in turn related to the matrix pore structure, size, and connectivity (Mow and Guo, 2002; Jackson and Gu, 2009; Sophia Fox et al., 2009). Overall, matrix hydrogel systems that have the same origin as the target tissue will show the highest potential to trigger native-like cell and tissue responses for both stem cells as well as primary cell types alike. This is an important aspect that has been investigated for decades for repopulated decellularized organ matrices (Guyette et al., 2016; Ohata and Ott, 2020) but still needs to be implemented properly for the next generation of organs-on-a-chip and microphysiological systems. This will obviously exclude the use of highly artificial hydrogel and scaffold systems (e.g., Matrigel, GelTrex, Fibrin, Gelatin, PEG, etc.), that cannot provide the right matrix architecture and composition found in healthy as well as diseased human tissues. Concerning matrix zonation and fiber orientation control, optical and extrusion bioprinting already show great promise to create biomimetic templates that are fit for musculoskeletal engineering as the alignment of individual structures can be controlled in a layer-by-layer fashion (Rothbauer et al., 2022).
FIGURE 10. The reciprocity of cellular mechanosensation escalates the pathological response during inflammatory onset and progression of OA. Microenvironmental factors of the extracellular matrix (extracellular matrix, ECM; pericellular matrix, PCM; Interterritorial matrix, and ITM) are monitored by cell-bound mechanosensitive structures such as integrins and connexins that again mediate dysfunctional cell activity and pathological matrix biosynthesis. Pathological mechanosignaling in turn influences the cellular sensation towards shear strain, stretching, and compressive loading. Created with permissions from Biorender.com.
As shown in Figure 11A, the general architecture of joint tissues often comprises a vascular region that transitions to an avascular region over multiple tissue layers or phases. Osteochondral tissue, synovium, or menisci are good examples of vascular-avascular transitions (i.e., blood—synovial tissue—synovial fluid). As indicated in Figure 11B, multiphasic systems can generate a range of molecular and biomechanical gradients as not only blood perfusion as a function of vascularization degree but also matrix composition and stiffness vary significantly even within individual tissue types (i.e., cartilage zones), which are all part of a physiological cellular and tissue microenvironment. Similar to the ambitions of the body-on-a-chip community to create microsystems that logically connect vascularized organ models (Ryu et al., 2015; Kratz et al., 2019), also joint-on-a-chip systems need to improve their vascular (as well as lymphatic) content using either prelumenized vascular blueprints/templates or vascular networks (Shi et al., 2014; Whisler et al., 2014; Knezevic et al., 2017; Bachmann et al., 2018) that form within a predefined tissue compartment or individual tissue zones guided by self-assembly and endothelial sprouting. For applications that are too complex for simple bifurcated lumen structures or even self-assembly, on-chip optical bioprinting can create even more biomimetic structures such as vascular beds at very high resolution (Grigoryan et al., 2019). Adding biomimetic tissue complexity can elaborate on the manifold relationship of vascular signaling and pathological architecture on overall joint tissue homeostasis for obviously fibrous tissues such as synovium, fat pads, and meniscus but also for osteochondral bone. These tissues are severely affected by vascular invasion and other pathological events involving bone resorption and osteophyte formation (Bonnet and Walsh, 2005; Suri et al., 2007; Hamilton et al., 2016). Potentially, the next generation of models should also consider better fluid components that recapitulate the viscoelastic, rheological, and biochemical properties of blood and synovial fluid which will also impact osmotic and interstitial pressure as well as fluid shear on mechanosensitive joint tissues. Also, the gaseous microenvironment provided by flow gradients will influence cell and tissue identity. However, hypoxia incubators and chambers can only adjust the entire microenvironment rather than generating a gradient by regional flow and gas control. In addition to variable tissue-specific flow conditions provided by microfluidic technologies, the application and integration of scavenging materials, vacuum degassing regions, or simply a natural adjustment of oxygen content by cell numbers via cell metabolism (Zirath et al., 2018; Sticker et al., 2019), as well as spatially-resolved oxygen feedback loops using sensor arrays can further improve microsystem control from a tissue perspective (Kratz et al., 2019).
FIGURE 11. (A) A schematic overview of basic vascular transitions within musculoskeletal tissues which are affected by OA-related hypervascularization. (B) A proposed future strategy to model joint tissue-specific transitions with biomimetic vascularized joint-on-a-chip systems includes the creation of vascular blueprints/templates with bioprinting as well as the provision of more relevant biofluid properties (e.g., viscoelasticity of blood and synovial fluid surrogates) and fluid-dependent biomechanical principles including fluid interstitial, as well as intra-arcticular pressure. Created with permissions from Biorender.com.
The maturity and origin of cells for 3D in vitro models constitute an enormous challenge that needs significant technological improvements. Culturing cells in vitro also includes their cultivation in an artificial, stimulative, and supplemented fluid environment, mainly with the aim to promote proliferation. Especially stem cells are often differentiated into joint tissue-specific cells and progenitor cells (Lin et al., 2014; O’Grady et al., 2019; Pirosa et al., 2021) for bone, cartilage, adipose, or connective tissue cells using differential cultivation protocols (Lin et al., 2019). Given the cell population heterogeneity of resident mature and progenitor cell types in native OA tissues (Stephenson et al., 2018; Cheng et al., 2021; Wang et al., 2021; Liu et al., 2022), stem cell approaches for future in vitro models must provide tissue-specific mature cell types with high phenotypic and genotypic authenticity in addition to a variety of heterogeneous progenitor cells. The direct and indirect crosstalk between various progenitor and adult cell subpopulations will in turn further advance the authenticity as well as the inflammatory responsiveness of the next-generation microsystems. Considering the physical alignment of tissue-resident cells, mature and progenitor cells can simply be adjusted by the cell mixing ratio within microfluidic compartments (Rothbauer et al., 2018); however, advances in bioprinting also for microfluidic organs-on-a-chip demonstrate new degrees of freedom and capabilities of cell deposition within 3D volumes (Rothbauer et al., 2022). Since OA is a disease of an adult population, the application of cells in early developmental stages (i.e., stem cells) to recapitulate the cellular composition of pathological adult tissues must be questioned critically. Even adult cell types show tissue-specific functions as demonstrated, e.g., for the differences between abdominal and infrapatellar tissue adipocytes in inflammatory M1-macrophage response (Barboza et al., 2017). Moreover, the in vivo hormonal tissue environment (Nevitt et al., 2001; Linn et al., 2012; Jin et al., 2017; Maghbooli et al., 2019) prior to cell isolation (Xue et al., 2018) alters the in vitro performance of patient-derived primary cells. This means that the authenticity of soluble biochemical cues such as hormones and potentially also a variety of other bioactive molecules including fatty acids, adipokines, and glycans found in mature tissue is paramount to recreating an authentic pathophysiological cell and tissue phenotype of OA (Toegel et al., 2009, 2010; Pabst et al., 2010). Considering that the prevalence of OA increases with age and is higher in women, especially after menopause (Phinyomark et al., 2016), cell donor choice is essential to achieve a disease-relevant and authentic model.
Another aspect to consider is the need for multiphasic and heterogeneous differentiation protocols including divergent differentiation and cultivation durations for the proper maturation of cells within 3D models. A first step to address this challenge is to generate growth factor gradients to tune the differentiation of bioprinted multiphasic tissue constructs (Lin et al., 2019; Pirosa et al., 2021). Nonetheless, with the increasing complexity of the microsystem, the spatial and temporal control of growth factor administration decreases. We thus propose to better use and employ liquid handling approaches such as concentration gradient generators and μ-valves for temporal and spatial separation of individual tissue and cell compartments for future models.
The requirements to successfully transfer this future biomimetic OA microsystem from an academic technology to its envisioned application in the pharmaceutical industry as a drug screening tool should already be considered in the ongoing design and development phase. From a technological standpoint, the integration and application of the currently available palette of integrated on-chip functions that organ-on-a-chip and microphysiological systems can offer still lags behind the evident cellular and molecular biological advancements improving the tissue-like architecture, individual cell phenotypes, gene expression profiles, as well as pro and antiinflammatory secretion. Over the last decade, a variety of technological improvements has been made to integrate on-chip functions within cell-based microfluidic systems to gain better control over the overall homo and heterotypic cell-cell interaction as well as the biophysical and chemical microenvironment (e.g., shear flow, loading, oxygen concentration, ECM compositions and stiffness gradients, nutrient supply and waste removal, etc. (Ribas et al., 2018; Rothbauer et al., 2018; Piluso et al., 2019; Sticker et al., 2019). Consequently, many principles that have been successfully reported for biomechanical (micro)bioreactors, cell-based microfluidics, and lab-on-a-chip systems including functional materials, degassers, microactuators as well as multicompartmental networks (Sticker et al., 2017; Piluso et al., 2019; Shabestani Monfared et al., 2020) have already shown great potential to also increase the capabilities of current organs-on-a-chip and microphysiological systems.
Even though many promising studies have combined industrial microfabrication technologies such as polymer hot embossing and electroplating (Novak et al., 2013), current academic approaches mostly lack the technology transfer from small academic production to large scale series production of complex organs-on-a-chip and microphysiological systems due to the ongoing strong academic dependence on poly(dimethyl)siloxane (PDMS) material. PDMS microfabrication is very affordable and straightforward and it offers great material advantages such as optical transparency, acceptable biocompatibility as well as gas transparency. Another plausible explanation for this limiting material selection is the fact that in most cases a standard 3–4 years research project does not exceed production batches of around a few 1,000 pieces including several design optimizations and iterations within a normal project lifetime. Consequently, fabrication methods for biomedical-grade hard polymers are still limited to specialized research groups due to infrastructural bias or budgetary limitations for both material pellets as well as injection tools that would provide industrial prototyping qualities for mass production. Notably, computer numerical control (CNC) micromachining or hot embossing of hard polymer slides would bridge the gap between soft lithography and injection molding. Nonetheless, scalable and high potential state-of-the-art technologies to improve the functionality of organs-on-a-chip and microphysiological systems may include well-established approaches found mostly for lab-on-a-chip and micro-total-analysis (µTAS) systems including integrated microvalves, micropumps, and gradient generators to control the automated administration of fluids at the picolitre scale (Unger et al., 2000; Frey et al., 2014; Tian et al., 2016; Rothbauer et al., 2019). To further improve sample throughput, a combination of gravity-driven pumpless bidirectional fluid handling technology (Sung et al., 2010; Esch et al., 2016) with robotics (Novak et al., 2020) may also be a high potential candidate. For models that require unidirectional pumping, this approach can also be modified to provide unidirectional fluid flow and shear when required (Wang and Shuler, 2018). Even more biomimetic flow conditions combining microsystems with bioprinting technologies as demonstrated by cardiac microsystems need to be considered also for a musculoskeletal vascular environment (Zhang and Larsen, 2017; Grigoryan et al., 2019).
To improve the high-content capabilities of currently available microsystems, a broad palette of micro and biosensors as well as inline and off-chip analysis schemes have been reported mostly as proof-of-concept studies (Kratz et al., 2019). Overall, the applicability of noninvasive monitoring approaches is high; however, any optical, electrical or chemical approach requires defined technological prerequisites including highly specialized measuring set-ups (e.g., electrical contacting, optical read-out positioning, specialized tables, etc.). This in turn reduces the manufacturability as well as integration potential with conventional incubator systems found in academia as well as industry labs. Moreover, the inclusion of a variety of cell- and tissue-specific analysis parameters that potentially drive the content of such a microsystem will impede the throughput capabilities of microsystems due to the necessity of printed circuit boards (PCBs), external multiplexing systems as well as a fair extent of electrical and/or optical fiber wiring to provide good measurement signal-to-noise ratio (SNR) signals. The synergy of noninvasive and dynamic analysis schemes with conventional destructive endpoint analysis technologies will allow more insights into the time-resolved response of bioengineered systems prior to the relative read-out at defined endpoints to better question aspects of cellular and donor variability and heterogeneity (Masaeli et al., 2016; Moura et al., 2019) as well as disease pathogenesis and tissue-specific pathomechanisms. As a final remark for this bioengineering road ahead, the necessity of artificial intelligence and deep learning (Hashemzadeh et al., 2021) to support faster data analysis and interpretation arise, since throughput and content as both throughput and content of our bioengineered systems will also potentiate the number of individual analysis parameters (e.g., secretion profile, mRNA expression, structural imaging, multidimensional cell morphologies, and interactions, etc.). Multi-parametric datasets can further benefit from potent high-content analysis schemes such as single-cell sequencing (Liu et al., 2022) and advanced mass spectrometry imaging (Rossiter et al., 2021; Vandenbosch et al., 2021). Finally, validation of microphysiological disease models must ideally be benchmarked against human data; however, no human patient data are available for the early stages of arthritic disorders. To tackle this challenge, species studies from which legacy data are available across any temporal range of pathogenesis stages will provide a good validation strategy for disease onset and progression validation (Jang et al., 2019; Marx et al., 2020).
Biomimicry of the pathological articular microenvironment should consider authentic tissue architectures, matrix compositions, cell heterogeneity, gene expression, and protein secretion (i.e., cell-cell interactions via soluble factors) in addition to general biomechanical as well as biochemical gradients found in human articular tissues to improve the capabilities and potential of the current disease models. Recent methodological advances in microfluidic technologies already provide better spatial and temporal control and heterogenic distribution over heterotypic cell populations, as well as biochemical and biomechanical traits with regard to conventional tissue-like models. Many key aspects that guide dysfunctional tissue homeostasis in the osteoarthritic environment in vivo still need to be implemented. Given the complexity of the articular environment, OA pathophysiology, and bioengineering approaches, an interdisciplinary approach will be needed to develop a disease-relevant in vitro model. To facilitate the necessary integration of recent technological advances and current knowledge on joint physiology and OA etiopathogenesis, we here draw a bioengineering roadmap to define key requirements that will significantly improve the in vivo relevance, predictability, and applicability of future chip-based disease models as drug screening tools. The current progress especially for joint-on-a-chip technology as disease models and drug screening tools in arthritis may not seem surprising at first glance because the technological progression follows advancements of previous in vitro models and approaches for arthritic diseases: RA-related studies focus mostly on synovial and immune contribution to catabolic processes such as secretion of catabolic mediators and mechanisms of bone resorption whereas OA-related studies survey predominantly the effect of degradative molecules but also proinflammatory mechanisms to affect cartilage as the key target tissue. The combination of cartilage and synovial cell populations with bone, vascular, or even autologous immune cell subpopulations of individual primary patient origin will show significant impact on how far we can advance joint multi-tissue coculture approaches in the future. Concluding all that has been mentioned earlier, a transdisciplinary effort is necessary to achieve the main aim of recreating native tissue-like models for musculoskeletal diseases. We need to appreciate and integrate all the lessons learned so far on joint tissue homeostasis and pathogenesis including joint biomechanics and tissue-level anatomical architecture, tissue-specific variations in the composition of the ECM, regional differences and neuralization and vascularity as well as the cellular origin, identity and activation states to achieve a model with authentic tissue-level responses relevant to OA. This will necessitate a cross-disciplinary effort combining regenerative medicine, tissue engineering, bioengineering, chemical engineering, mechanical engineering, and biomechanics in addition to cell and molecular biology to create a biotechnological leap forward. For the creation of fit-for-purpose technology applicable for industrial drug screening applications, the scalability of the model regarding analysis throughput and the content will further require close collaboration with mechanical engineering experts and industrial chip manufacturers.
MR: writing—draft, writing—illustrations, conceptualization, and planning, writing—editing. ER: writing—draft, writing—illustrations/visualizations, and writing—editing. AF: writing—draft and writing—editing. FJ: writing—draft, writing—illustrations/visualizations, and writing—editing. RW: writing—draft and writing—editing; ST: writing—draft and writing—editing.
The authors declare that the research was conducted in the absence of any commercial or financial relationships that could be construed as a potential conflict of interest.
All claims expressed in this article are solely those of the authors and do not necessarily represent those of their affiliated organizations, or those of the publisher, the editors, and the reviewers. Any product that may be evaluated in this article, or claim that may be made by its manufacturer, is not guaranteed or endorsed by the publisher.
The authors acknowledge the TU Wien University Library for financial support through its Open Access Funding Program. ST acknowledges financial support from the Association For Orthopedic Research (AFOR) foundation grant and the Johnson&Johnson Medical Products GmbH scholarship GMAFS20512.
Aho, O.-M., Finnilä, M., Thevenot, J., Saarakkala, S., and Lehenkari, P. (2017). Subchondral Bone Histology and Grading in Osteoarthritis. PLoS One 12, e0173726. doi:10.1371/journal.pone.0173726
Albarrán-Juárez, J., Iring, A., Wang, S., Joseph, S., Grimm, M., Strilic, B., et al. (2018). Piezo1 and Gq/G11 Promote Endothelial Inflammation Depending on Flow Pattern and Integrin Activation. J. Exp. Med. 215, 2655–2672. doi:10.1084/JEM.20180483
Anderson, J. R., Phelan, M. M., Foddy, L., Clegg, P. D., and Peffers, M. J. (2020). Ex Vivo Equine Cartilage Explant Osteoarthritis Model: A Metabolomics and Proteomics Study. J. Proteome Res. 19, 3652–3667. doi:10.1021/acs.jproteome.0c00143
Andriacchi, T. P., Griffin, T. M., Loeser, R. F., Chu, C. R., Roos, E. M., Hawker, G. A., et al. (2020). Bridging Disciplines as a Pathway to Finding New Solutions for Osteoarthritis a Collaborative Program Presented at the 2019 Orthopaedic Research Society and the Osteoarthritis Research Society International. Osteoarthr. Cartil. Open 2, 100026. doi:10.1016/j.ocarto.2020.100026
Bachmann, B., Spitz, S., Rothbauer, M., Jordan, C., Purtscher, M., Zirath, H., et al. (2018). Engineering of Three-Dimensional Pre-vascular Networks within Fibrin Hydrogel Constructs by Microfluidic Control over Reciprocal Cell Signaling. Biomicrofluidics 12, 042216. doi:10.1063/1.5027054
Barboza, E., Hudson, J., Chang, W. P., Kovats, S., Towner, R. A., Silasi‐Mansat, R., et al. (2017). Profibrotic Infrapatellar Fat Pad Remodeling without M1 Macrophage Polarization Precedes Knee Osteoarthritis in Mice with Diet‐Induced Obesity. Arthritis & Rheumatology 69, 1221–1232. doi:10.1002/art.40056
Barry, F., and Murphy, M. (2013). Mesenchymal Stem Cells in Joint Disease and Repair. Nat. Rev. Rheumatol. 9, 584–594. doi:10.1038/nrrheum.2013.109
Blake, D. R., Unsworth, J., Outhwaite, J. M., Morris, C. J., Merry, P., Kidd, B. L., et al. (1989). Hypoxic-Reperfusion Injury in the Inflamed Human Joint. Lancet 333, 289–293. doi:10.1016/S0140-6736(89)91305-6
Blewis, M., Nugent-Derfus, G. E., Nugent-Derfus, G., Schmidt, T., Schumacher, B., and Sah, R. (2007). A Model of Synovial Fluid Lubricant Composition in Normal and Injured Joints. eCM 13, 26–39. doi:10.22203/ecm.v013a03
Blom, A. B., van Lent, P. L. E. M., Holthuysen, A. E. M., van der Kraan, P. M., Roth, J., van Rooijen, N., et al. (2004). Synovial Lining Macrophages Mediate Osteophyte Formation during Experimental Osteoarthritis. Osteoarthr. Cartil. 12, 627–635. doi:10.1016/j.joca.2004.03.003
Bonnet, C. S., and Walsh, D. A. (2005). Osteoarthritis, Angiogenesis and Inflammation. Rheumatology 44, 7–16. doi:10.1093/rheumatology/keh344
Boris Chan, P. M., Zhu, L., Wen, C. Y., and Chiu, K. Y. (2015). Subchondral Bone Proteomics in Osteoarthritis: Current Status and Perspectives. J. Orthop. Transl. 3, 71–77. doi:10.1016/j.jot.2015.02.002
Brighton, C. T., Heppenstall, R. B., and Labosky, D. A. (1971). An Oxygen Microelectrode Suitable for Cartilage and Cancellous Bone. Clin. Orthop. Relat. Res. 80, 161–166. doi:10.1097/00003086-197110000-00023
Broeren, M., Waterborg, C. E. J., Wiegertjes, R., Thurlings, R. M., Koenders, M. I., van Lent, P. L. E. M., et al. (2019). A Three-Dimensional Model to Study Human Synovial Pathology. ALTEX 36, 18–28. doi:10.14573/altex.1804161
Candela, M. E., Wang, C., Gunawardena, A. T., Zhang, K., Cantley, L., Yasuhara, R., et al. (2016). Alpha 5 Integrin Mediates Osteoarthritic Changes in Mouse Knee Joints. PLoS One 11, e0156783. doi:10.1371/journal.pone.0156783
Carter, D. R., Beaupré, G. S., Wong, M., Smith, R. L., Andriacchi, T. P., and Schurman, D. J. (2004). The Mechanobiology of Articular Cartilage Development and Degeneration. Clin. Orthop. Relat. Res. 427, S69–S77. doi:10.1097/01.blo.0000144970.05107.7e
Chahine, N. O., Chen, F. H., Hung, C. T., and Ateshian, G. A. (2005). Direct Measurement of Osmotic Pressure of Glycosaminoglycan Solutions by Membrane Osmometry at Room Temperature. Biophysical J. 89, 1543–1550. doi:10.1529/biophysj.104.057315
Chauffier, K., Laiguillon, M.-C., Bougault, C., Gosset, M., Priam, S., Salvat, C., et al. (2012). Induction of the Chemokine IL-8/Kc by the Articular Cartilage: Possible Influence on Osteoarthritis. Jt. Bone Spine 79, 604–609. doi:10.1016/j.jbspin.2011.12.013
Cheng, L., Wang, Y., Wu, R., Ding, T., Xue, H., Gao, C., et al. (2021). New Insights from Single-Cell Sequencing Data: Synovial Fibroblasts and Synovial Macrophages in Rheumatoid Arthritis. Front. Immunol. 12, 1–11. doi:10.3389/fimmu.2021.709178
Chery, D. R., Han, B., Zhou, Y., Wang, C., Adams, S. M., Chandrasekaran, P., et al. (2021). Decorin Regulates Cartilage Pericellular Matrix Micromechanobiology. Matrix Biol. 96, 1–17. doi:10.1016/j.matbio.2020.11.002
Cloyd, J. M., Malhotra, N. R., Weng, L., Chen, W., Mauck, R. L., and Elliott, D. M. (2007). Material Properties in Unconfined Compression of Human Nucleus Pulposus, Injectable Hyaluronic Acid-Based Hydrogels and Tissue Engineering Scaffolds. Eur. Spine J. 16, 1892–1898. doi:10.1007/s00586-007-0443-6
Danalache, M., Kleinert, R., Schneider, J., Erler, A. L., Schwitalle, M., Riester, R., et al. (2019). Changes in Stiffness and Biochemical Composition of the Pericellular Matrix as a Function of Spatial Chondrocyte Organisation in Osteoarthritic Cartilage. Osteoarthr. Cartil. 27, 823–832. doi:10.1016/j.joca.2019.01.008
Dieppe, P. (1999). Osteoarthritis: Time to Shift the Paradigm. Bmj 318, 1299–1300. doi:10.1136/bmj.318.7194.1299
Ding, L., Guo, D., Homandberg, G. A., Buckwalter, J. A., and Martin, J. A. (2014). A Single Blunt Impact on Cartilage Promotes Fibronectin Fragmentation and Upregulates Cartilage Degrading Stromelysin-1/matrix Metalloproteinase-3 in a Bovineex Vivomodel. J. Orthop. Res. 32, 811–818. doi:10.1002/jor.22610
Divoux, A., Tordjman, J., Lacasa, D., Veyrie, N., Hugol, D., Aissat, A., et al. (2010). Fibrosis in Human Adipose Tissue: Composition, Distribution, and Link with Lipid Metabolism and Fat Mass Loss. Diabetes 59, 2817–2825. doi:10.2337/db10-0585
Dolzani, P., Assirelli, E., Pulsatelli, L., Meliconi, R., Mariani, E., and Neri, S. (2019). Ex Vivo physiological Compression of Human Osteoarthritis Cartilage Modulates Cellular and Matrix Components. PLoS One 14, e0222947–16. doi:10.1371/journal.pone.0222947
Esch, M. B., Ueno, H., Applegate, D. R., and Shuler, M. L. (2016). Modular, Pumpless Body-On-A-Chip Platform for the Co-culture of GI Tract Epithelium and 3D Primary Liver Tissue. Lab. Chip 16, 2719–2729. doi:10.1039/c6lc00461j
Estell, E. G., Murphy, L. A., Silverstein, A. M., Tan, A. R., Shah, R. P., Ateshian, G. A., et al. (2017). Fibroblast-like Synoviocyte Mechanosensitivity to Fluid Shear Is Modulated by Interleukin-1α. J. Biomechanics 60, 91–99. doi:10.1016/j.jbiomech.2017.06.011
Fahy, N., Menzel, U., Alini, M., and Stoddart, M. J. (2019). Shear and Dynamic Compression Modulates the Inflammatory Phenotype of Human Monocytes In Vitro. Front. Immunol. 10. doi:10.3389/fimmu.2019.00383
Fermor, B., Christensen, S. E., Christensen, S., Youn, I., Cernanec, J., Davies, C., et al. (2007). Oxygen, Nitric Oxide and Articular Cartilage. eCM 13, 56–65. doi:10.22203/eCM.v013a06
Fermor, B., Weinberg, J. B., Pisetsky, D. S., Misukonis, M. A., Fink, C., and Guilak, F. (2002). Induction of Cyclooxygenase-2 by Mechanical Stress through a Nitric Oxide-Regulated Pathway. Osteoarthr. Cartil. 10, 792–798. doi:10.1053/joca.2002.0832
Foldager, C. B., Toh, W. S., Gomoll, A. H., Olsen, B. R., and Spector, M. (2014). Distribution of Basement Membrane Molecules, Laminin and Collagen Type IV, in Normal and Degenerated Cartilage Tissues. Normal Degenerated Cartil. TissuesCartilage 5, 123–132. doi:10.1177/1947603513518217
Fomby, P., Cherlin, A. J., Hadjizadeh, A., Doillon, C. J., Sueblinvong, V., Weiss, D. J., et al. (2010). Stem Cells and Cell Therapies in Lung Biology and Diseases: Conference Report. Ann. Am. Thorac. Soc. 12, 181–204. doi:10.1002/term
Freemont, A. J. (1996). Microscopic Analysis of Synovial Fluid-Tthe Perfect Diagnostic Test? Ann. Rheumatic Dis. 55, 695–697. doi:10.1136/ard.55.10.695
Frey, O., Misun, P. M., Fluri, D. A., Hengstler, J. G., and Hierlemann, A. (2014). Reconfigurable Microfluidic Hanging Drop Network for Multi-Tissue Interaction and Analysis. Nat. Commun. 5, 4250. doi:10.1038/ncomms5250
Fu, L., Li, P., Li, H., Gao, C., Yang, Z., Zhao, T., et al. (2021). The Application of Bioreactors for Cartilage Tissue Engineering: Advances, Limitations, and Future Perspectives. Stem Cells Int. 2021, 1–13. doi:10.1155/2021/6621806
Gao, Y., Liu, S., Huang, J., Guo, W., Chen, J., Zhang, L., et al. (2014). The ECM-Cell Interaction of Cartilage Extracellular Matrix on Chondrocytes. BioMed Res. Int. 2014, 1–8. doi:10.1155/2014/648459
Geborek, P., Forslind, K., and Wollheim, F. A. (1989). Direct Assessment of Synovial Blood Flow and its Relation to Induced Hydrostatic Pressure Changes. Ann. Rheumatic Dis. 48, 281–286. doi:10.1136/ard.48.4.281
Gilbert, S. J., Bonnet, C. S., and Blain, E. J. (2021). Mechanical Cues: Bidirectional Reciprocity in the Extracellular Matrix Drives Mechano-Signalling in Articular Cartilage. Ijms 22, 13595. doi:10.3390/ijms222413595
Gobezie, R., Kho, A., Krastins, B., Sarracino, D. A., Thornhill, T. S., Chase, M., et al. (2007). High Abundance Synovial Fluid Proteome: Distinct Profiles in Health and Osteoarthritis. Arthritis Res. Ther. 9, R36. doi:10.1186/ar2172
Goldring, M., Otero, M., Otero, M., Plumb, D., Dragomir, C., Favero, M., et al. (2011). Roles of Inflammatory and Anabolic Cytokines in Cartilage Metabolism: Signals and Multiple Effectors Converge upon MMP-13 Regulation in Osteoarthritis. eCM 21, 202–220. doi:10.22203/eCM.v021a16
Grigoryan, B., Paulsen, S. J., Corbett, D. C., Sazer, D. W., Fortin, C. L., Zaita, A. J., et al. (2019). Multivascular Networks and Functional Intravascular Topologies within Biocompatible Hydrogels. Science 364, 458–464. doi:10.1126/science.aav9750
Guilak, F., Alexopoulos, L. G., Haider, M. A., Ting-Beall, H. P., and Setton, L. A. (2005). Zonal Uniformity in Mechanical Properties of the Chondrocyte Pericellular Matrix: Micropipette Aspiration of Canine Chondrons Isolated by Cartilage Homogenization. Ann. Biomed. Eng. 33, 1312–1318. doi:10.1007/s10439-005-4479-7
Guo, T., Lembong, J., Zhang, L. G., and Fisher, J. P. (2017). Three-Dimensional Printing Articular Cartilage: Recapitulating the Complexity of Native Tissue. Tissue Eng. Part B Rev. 23, 225–236. doi:10.1089/ten.teb.2016.0316
Guyette, J. P., Charest, J. M., Mills, R. W., Jank, B. J., Moser, P. T., Gilpin, S. E., et al. (2016). Bioengineering Human Myocardium on Native Extracellular Matrix. Circ. Res. 118, 56–72. doi:10.1161/CIRCRESAHA.115.306874
Hagan, M. L., Yu, K., Zhu, J., Vinson, B. N., Roberts, R. L., Montesinos Cartagena, M., et al. (2020). Decreased Pericellular Matrix Production and Selection for Enhanced Cell Membrane Repair May Impair Osteocyte Responses to Mechanical Loading in the Aging Skeleton. Aging Cell 19. doi:10.1111/acel.13056
Hall, A. C. (2019). The Role of Chondrocyte Morphology and Volume in Controlling Phenotype-Implications for Osteoarthritis, Cartilage Repair, and Cartilage Engineering. Curr. Rheumatol. Rep. 21. doi:10.1007/s11926-019-0837-6
Haltmayer, E., Ribitsch, I., Gabner, S., Rosser, J., Gueltekin, S., Peham, J., et al. (2019). Co-culture of Osteochondral Explants and Synovial Membrane as In Vitro Model for Osteoarthritis. PLoS One 14, e0214709. doi:10.1371/journal.pone.0214709
Hamilton, J. L., Nagao, M., Levine, B. R., Chen, D., Olsen, B. R., and Im, H.-J. (2016). Targeting VEGF and its Receptors for the Treatment of Osteoarthritis and Associated Pain. J. Bone Min. Res. 31, 911–924. doi:10.1002/jbmr.2828
Han, D., Fang, Y., Tan, X., Jiang, H., Gong, X., Wang, X., et al. (2020). The Emerging Role of Fibroblast‐like Synoviocytes‐mediated Synovitis in Osteoarthritis: An Update. J. Cell. Mol. Med. 24, 9518–9532. doi:10.1111/jcmm.15669
Hashemzadeh, H., Shojaeilangari, S., Allahverdi, A., Rothbauer, M., Ertl, P., and Naderi-Manesh, H. (2021). A Combined Microfluidic Deep Learning Approach for Lung Cancer Cell High Throughput Screening toward Automatic Cancer Screening Applications. Sci. Rep. 11, 9804. doi:10.1038/s41598-021-89352-8
Hirschmann, M. T., Zschábitz, A., and Stofft, E. (2007). Immunohistochemical Characterization of Human Synovial Bursa Cells by Light and Transmission Electron Microscopy: Where Do These Cells Come from? Int. J. Morphol. 25, 5–14. doi:10.4067/S0717-95022007000100001
Hlaváček, M. (1995). The Role of Synovial Fluid Filtration by Cartilage in Lubrication of Synovial Joints-IV. Squeeze-Film Lubrication: The Central Film Thickness for Normal and Inflammatory Synovial Fluids for Axial Symmetry under High Loading Conditions. J. Biomech. 28, 1199–1205. doi:10.1016/0021-9290(94)00178-7
Hügle, T., and Geurts, J. (2017). What Drives Osteoarthritis?-Synovialversussubchondral Bone Pathology. Rheumatology 56, kew389–1471. doi:10.1093/rheumatology/kew389
Jackson, A., and Gu, W. (2009). Transport Properties of Cartilaginous Tissues. Crr 5, 40–50. doi:10.2174/157339709787315320
Jang, K.-J., Otieno, M. A., Ronxhi, J., Lim, H.-K., Ewart, L., Kodella, K. R., et al. (2019). Reproducing Human and Cross-Species Drug Toxicities Using a Liver-Chip. Sci. Transl. Med. 11, eaax5516. doi:10.1126/scitranslmed.aax5516
Jayson, M. I., and St Dixon, A. J. (1970). Intra-articular Pressure in Rheumatoid Arthritis of the Knee. I. Pressure Changes during Passive Joint Distension. Ann. Rheumatic Dis. 29, 261–265. doi:10.1136/ard.29.3.261
Jin, X., Wang, B. H., Wang, X., Antony, B., Zhu, Z., Han, W., et al. (2017). Associations between Endogenous Sex Hormones and MRI Structural Changes in Patients with Symptomatic Knee Osteoarthritis. Osteoarthr. Cartil. 25, 1100–1106. doi:10.1016/j.joca.2017.01.015
Kapri, E., Mehta, M., and S, K. (2021). Biomechanics of Running: An Overview on Gait Cycle. Int. J. Phys. Educ. Fit. Sports 10, 1–9. doi:10.34256/ijpefs2131
Kiener, H. P., Watts, G. F. M., Cui, Y., Wright, J., Thornhill, T. S., Sköld, M., et al. (2010). Synovial Fibroblasts Self-Direct Multicellular Lining Architecture and Synthetic Function in Three-Dimensional Organ Culture. Arthritis & Rheumatism 62, 742–752. doi:10.1002/art.27285
Knezevic, L., Schaupper, M., Mühleder, S., Schimek, K., Hasenberg, T., Marx, U., et al. (2017). Engineering Blood and Lymphatic Microvascular Networks in Fibrin Matrices. Front. Bioeng. Biotechnol. 5. doi:10.3389/fbioe.2017.00025
Kratz, S., Höll, G., Schuller, P., Ertl, P., and Rothbauer, M. (2019). Latest Trends in Biosensing for Microphysiological Organs-On-A-Chip and Body-On-A-Chip Systems. Biosensors 9, 110. doi:10.3390/bios9030110
Kutzner, I., Heinlein, B., Graichen, F., Bender, A., Rohlmann, A., Halder, A., et al. (2010). Loading of the Knee Joint during Activities of Daily Living Measured In Vivo in Five Subjects. J. Biomechanics 43, 2164–2173. doi:10.1016/j.jbiomech.2010.03.046
Lawry, G. V., and Bewyer, D. (2008). “Anatomy of Joints, General Considerations, and Principles of Joint Examination,” in Fam’s Musculoskeletal Examination and Joint Injection Techniques. Editors G. V Lawry, H. J. Kreder, and G. A. Hawker. Second Edition (Philadelphia: Mosby), 1–5. doi:10.1016/b978-0-323-06504-7.10001-6
Lee, D., Erickson, A., You, T., Dudley, A. T., and Ryu, S. (2018). Pneumatic Microfluidic Cell Compression Device for High-Throughput Study of Chondrocyte Mechanobiology. Lab. Chip 18, 2077–2086. doi:10.1039/c8lc00320c
Levick, J. R., and McDonald, J. N. (1995). Fluid Movement across Synovium in Healthy Joints: Role of Synovial Fluid Macromolecules. Ann. Rheumatic Dis. 54, 417–423. doi:10.1136/ard.54.5.417
Li, R., Serrano, J. C., Xing, H., Lee, T. A., Azizgolshani, H., Zaman, M., et al. (2018). Interstitial Flow Promotes Macrophage Polarization toward an M2 Phenotype. MBoC 29, 1927–1940. doi:10.1091/mbc.E18-03-0164
Li, Y., Fan, Q., Jiang, Y., Gong, F., and Xia, H. (2017). Effects of Insulin-like Growth Factor 1 and Basic Fibroblast Growth Factor on the Morphology and Proliferation of Chondrocytes Embedded in Matrigel in a Microfluidic Platform. Exp. Ther. Med. 14, 2657–2663. doi:10.3892/etm.2017.4808
Li, Y. Y., Lam, K. L., Chen, A. D., Zhang, W., and Chan, B. P. (2019). Collagen Microencapsulation Recapitulates Mesenchymal Condensation and Potentiates Chondrogenesis of Human Mesenchymal Stem Cells - A Matrix-Driven In Vitro Model of Early Skeletogenesis. Biomaterials 213, 119210. doi:10.1016/j.biomaterials.2019.05.021
Lin, H., Lozito, T. P., Alexander, P. G., Gottardi, R., and Tuan, R. S. (2014). Stem Cell-Based Microphysiological Osteochondral System to Model Tissue Response to Interleukin-1β. Mol. Pharm. 11, 2203–2212. doi:10.1021/mp500136b
Lin, Z., Li, Z., Li, E. N., Li, X., Del Duke, C. J., Shen, H., et al. (2019). Osteochondral Tissue Chip Derived from iPSCs: Modeling OA Pathologies and Testing Drugs. Front. Bioeng. Biotechnol. 7, 411. doi:10.3389/fbioe.2019.00411
Linn, S., Murtaugh, B., and Casey, E. (2012). Role of Sex Hormones in the Development of Osteoarthritis. PM&R 4, S169–S173. doi:10.1016/j.pmrj.2012.01.013
Liu, W., Chen, Y., Zeng, G., Yang, S., Yang, T., Ma, M., et al. (2022). Single-Cell Profiles of Age-Related Osteoarthritis Uncover Underlying Heterogeneity Associated with Disease Progression. Front. Mol. Biosci. 8, 1–18. doi:10.3389/fmolb.2021.748360
Loeser, R. F., Goldring, S. R., Scanzello, C. R., and Goldring, M. B. (2012). Osteoarthritis: A Disease of the Joint as an Organ. Arthritis & Rheumatism 64, 1697–1707. doi:10.1002/art.34453
Loskill, P., Sezhian, T., Tharp, K. M., Lee-Montiel, F. T., Jeeawoody, S., Reese, W. M., et al. (2017). WAT-on-a-chip: A Physiologically Relevant Microfluidic System Incorporating White Adipose Tissue. Lab. Chip 17, 1645–1654. doi:10.1039/c6lc01590e
Lucchinetti, E., Bhargava, M. M., and Torzilli, P. A. (2004). The Effect of Mechanical Load on Integrin Subunits α5 and β1 in Chondrocytes from Mature and Immature Cartilage Explants. Cell Tissue Res. 315, 385–391. doi:10.1007/s00441-003-0836-8
Ma, H.-P., Deng, X., Chen, D.-Y., Zhu, D., Tong, J.-L., Zhao, T., et al. (2018). A Microfluidic Chip-Based Co-culture of Fibroblast-like Synoviocytes with Osteoblasts and Osteoclasts to Test Bone Erosion and Drug Evaluation. R. Soc. Open Sci. 5, 180528. doi:10.1098/rsos.180528
Maghbooli, Z., Hossein-nezhad, A., Khoshechin, G., Niketeghad, G., Moradi, S., Adabi, E., et al. (2019). Possible Association between Circulating CTRP3 and Knee Osteoarthritis in Postmenopausal Women. Aging Clin. Exp. Res. 31, 927–934. doi:10.1007/s40520-018-1035-5
Mansoorifar, A., Gordon, R., Bergan, R. C., and Bertassoni, L. E. (2021). Bone‐on‐a‐Chip: Microfluidic Technologies and Microphysiologic Models of Bone Tissue. Adv. Funct. Mat. 31, 2006796. doi:10.1002/adfm.202006796
Maracle, C. X., Kucharzewska, P., Helder, B., van der Horst, C., Correa de Sampaio, P., Noort, A.-R., et al. (2017). Targeting Non-canonical Nuclear Factor-Κb Signalling Attenuates Neovascularization in a Novel 3D Model of Rheumatoid Arthritis Synovial Angiogenesis. Rheumatology 56, 294–302. doi:10.1093/rheumatology/kew393
March, L., Cross, M., Arden, N., Gates, L., Leyland, K. M., Hawker, G., et al. (2016). Osteoarthritis: A Serious Disease, Submitted to the U. S. Silver Spring, MD: Food and Drug Administration.
Marcu, K. B., Otero, M., Olivotto, E., Maria Borzi, R., and Goldring, M. (2010). NF-κB Signaling: Multiple Angles to Target OA. Curr. Drug Targets 11, 599–613. doi:10.2174/138945010791011938
Marx, U., Akabane, T., Andersson, T. B., Baker, E., Beilmann, M., Beken, S., et al. (2020). Biology-inspired Microphysiological Systems to Advance Medicines for Patient Benefit and Animal Welfare. ALTEX 37, 365–394. doi:10.14573/altex.2001241
Masaeli, M., Gupta, D., O’Byrne, S., Tse, H. T. K., Gossett, D. R., Tseng, P., et al. (2016). Multiparameter Mechanical and Morphometric Screening of Cells. Sci. Rep. 6, 37863. doi:10.1038/srep37863
Meinert, C., Schrobback, K., Hutmacher, D. W., and Klein, T. J. (2017). A Novel Bioreactor System for Biaxial Mechanical Loading Enhances the Properties of Tissue-Engineered Human Cartilage. Sci. Rep. 7, 1–14. doi:10.1038/s41598-017-16523-x
Mobasheri, A., and Batt, M. (2016). An Update on the Pathophysiology of Osteoarthritis. Ann. Phys. Rehabilitation Med. 59, 333–339. doi:10.1016/j.rehab.2016.07.004
Mobasheri, A., Rayman, M. P., Gualillo, O., Sellam, J., Van Der Kraan, P., and Fearon, U. (2017). The Role of Metabolism in the Pathogenesis of Osteoarthritis. Nat. Rev. Rheumatol. 13, 302–311. doi:10.1038/nrrheum.2017.50
Mobasheri, A., Saarakkala, S., Finnilä, M., Karsdal, M. A., Bay-Jensen, A.-C., and van Spil, W. E. (2019). Recent Advances in Understanding the Phenotypes of Osteoarthritis. F1000Res 8, 2091. doi:10.12688/f1000research.20575.1
Mondadori, C., Palombella, S., Salehi, S., Talò, G., Visone, R., Rasponi, M., et al. (2021). Recapitulating Monocyte Extravasation to the Synovium in an Organotypic Microfluidic Model of the Articular Joint. Biofabrication 13, 045001. doi:10.1088/1758-5090/ac0c5e
Moura, C. C., Bourdakos, K. N., Tare, R. S., Oreffo, R. O. C., and Mahajan, S. (2019). Live-imaging of Bioengineered Cartilage Tissue Using Multimodal Non-linear Molecular Imaging. Sci. Rep. 9. doi:10.1038/s41598-019-41466-w
Mouser, V. H. M., Levato, R., Mensinga, A., Dhert, W. J. A., Gawlitta, D., and Malda, J. (2020). Bio-ink Development for Three-Dimensional Bioprinting of Hetero-Cellular Cartilage Constructs. Connect. Tissue Res. 61, 137–151. doi:10.1080/03008207.2018.1553960
Mow, V. C., and Guo, X. E. (2002). Mechano-electrochemical Properties of Articular Cartilage: Their Inhomogeneities and Anisotropies. Annu. Rev. Biomed. Eng. 4, 175–209. doi:10.1146/annurev.bioeng.4.110701.120309
Munjal, A., Bapat, S., Hubbard, D., Hunter, M., Kolhe, R., and Fulzele, S. (2019). Advances in Molecular Biomarker for Early Diagnosis of Osteoarthritis. Biomol. Concepts 10, 111–119. doi:10.1515/bmc-2019-0014
Nasello, G., Cóndor, M., Vaughan, T., and Schiavi, J. (2021). Designing Hydrogel-Based Bone-On-Chips for Personalized Medicine. Appl. Sci. 11, 4495. doi:10.3390/app11104495
Natenstedt, J., Kok, A. C., Dankelman, J., and Tuijthof, G. J. (2015). What Quantitative Mechanical Loading Stimulates In Vitro Cultivation Best? J. Exp. Ortop. 2, 1–15. doi:10.1186/s40634-015-0029-x
Nevitt, M. C., Felson, D. T., Williams, E. N., and Grady, D. (2001). The Effect of Estrogen Plus Progestin on Knee Symptoms and Related Disability in Postmenopausal Women: The Heart and Estrogen/progestin Replacement Study, a Randomized, Double-Blind, Placebo-Controlled Trial. Arthritis Rheum. 44 (4), 811–818. doi:10.1002/1529-0131(200104)44:4<811::AID-ANR137>3.0.CO;2-F
Novak, R., Ingram, M., Marquez, S., Das, D., Delahanty, A., Herland, A., et al. (2020). Robotic Fluidic Coupling and Interrogation of Multiple Vascularized Organ Chips. Nat. Biomed. Eng. 4, 407–420. doi:10.1038/s41551-019-0497-x
Novak, R., Ranu, N., and Mathies, R. A. (2013). Rapid Fabrication of Nickel Molds for Prototyping Embossed Plastic Microfluidic Devices. Lab. Chip 13, 1468–1471. doi:10.1039/c3lc41362d
O'Grady, B., Balikov, D. A., Wang, J. X., Neal, E. K., Ou, Y.-C., Bardhan, R., et al. (2019). Spatiotemporal Control and Modeling of Morphogen Delivery to Induce Gradient Patterning of Stem Cell Differentiation Using Fluidic Channels. Biomater. Sci. 7, 1358–1371. doi:10.1039/c8bm01199k
Occhetta, P., Mainardi, A., Votta, E., Vallmajo-Martin, Q., Ehrbar, M., Martin, I., et al. (2019). Hyperphysiological Compression of Articular Cartilage Induces an Osteoarthritic Phenotype in a Cartilage-On-A-Chip Model. Nat. Biomed. Eng. 3, 545–557. doi:10.1038/s41551-019-0406-3
Ohata, K., and Ott, H. C. (2020). Human-scale Lung Regeneration Based on Decellularized Matrix Scaffolds as a Biologic Platform. Surg. Today 50, 633–643. doi:10.1007/s00595-020-02000-y
Onuora, S. (2020). Synovial Fibroblast Expansion in RA Is Driven by Notch Signalling. Nat. Rev. Rheumatol. 16, 349. doi:10.1038/s41584-020-0453-x
Pabst, M., Wu, S. Q., Grass, J., Kolb, A., Chiari, C., Viernstein, H., et al. (2010). IL-1β and TNF-α Alter the Glycophenotype of Primary Human Chondrocytes In Vitro. Carbohydr. Res. 345, 1389–1393. doi:10.1016/j.carres.2010.02.017
Paggi, C. A., Venzac, B., Karperien, M., Leijten, J. C. H., and Le Gac, S. (2020). Monolithic Microfluidic Platform for Exerting Gradients of Compression on Cell-Laden Hydrogels, and Application to a Model of the Articular Cartilage. Sensors Actuators B Chem. 315, 127917. doi:10.1016/j.snb.2020.127917
Peck, Y., Leom, L. T., Low, P. F. P., and Wang, D.-A. (2018). Establishment of an In Vitro Three-Dimensional Model for Cartilage Damage in Rheumatoid Arthritis. J. Tissue Eng. Regen. Med. 12, e237–e249. doi:10.1002/term.2399
Phinyomark, A., Osis, S. T., Hettinga, B. A., Kobsar, D., and Ferber, R. (2016). Gender Differences in Gait Kinematics for Patients with Knee Osteoarthritis. BMC Musculoskelet. Disord. 17, 1–12. doi:10.1186/s12891-016-1013-z
Pichler, K. M., Fischer, A., Alphonsus, J., Chiari, C., Schmidt, S., Kenn, M., et al. (2022). Galectin Network in Osteoarthritis: Galectin-4 Programs a Pathogenic Signature of Gene and Effector Expression in Human Chondrocytes In Vitro. Histochem. Cell Biol. 157, 139–151. doi:10.1007/s00418-021-02053-1
Pichler, K. M., Weinmann, D., Schmidt, S., Kubista, B., Lass, R., Martelanz, L., et al. (2021). The Dysregulated Galectin Network Activates NF-Κb to Induce Disease Markers and Matrix Degeneration in 3D Pellet Cultures of Osteoarthritic Chondrocytes. Calcif. Tissue Int. 108, 377–390. doi:10.1007/s00223-020-00774-4
Piluso, S., Li, Y., Abinzano, F., Levato, R., Moreira Teixeira, L., Karperien, M., et al. (2019). Mimicking the Articular Joint with In Vitro Models. Trends Biotechnol. 37, 1063–1077. doi:10.1016/j.tibtech.2019.03.003
Pirosa, A., Gottardi, R., Alexander, P. G., Puppi, D., Chiellini, F., and Tuan, R. S. (2021). An In Vitro Chondro-Osteo-Vascular Triphasic Model of the Osteochondral Complex. Biomaterials 272, 120773. doi:10.1016/j.biomaterials.2021.120773
Piscoya, J. L., Fermor, B., Kraus, V. B., Stabler, T. V., and Guilak, F. (2005). The Influence of Mechanical Compression on the Induction of Osteoarthritis-Related Biomarkers in Articular Cartilage Explants. Osteoarthr. Cartil. 13, 1092–1099. doi:10.1016/j.joca.2005.07.003
Qian, J.-Y., Hou, C.-W., Li, X.-J., and Jin, Z.-J. (2020). Actuation Mechanism of Microvalves: A Review. Micromachines 11, 172. doi:10.3390/mi11020172
Rajpurohit, R., Koch, C. J., Tao, Z., Teixeira, C. M., and Shapiro, I. M. (1996). Adaptation of Chondrocytes to Low Oxygen Tension: Relationship between Hypoxia and Cellular Metabolism. J. Cell. Physiol. 168, 424–432. doi:10.1002/(sici)1097-4652(199608)168:2<424::aid-jcp21>3.0.co;2-1
Raman, S., FitzGerald, U., and Murphy, J. M. (2018). Interplay of Inflammatory Mediators with Epigenetics and Cartilage Modifications in Osteoarthritis. Front. Bioeng. Biotechnol. 6, 1–9. doi:10.3389/fbioe.2018.00022
Ribas, J., Pawlikowska, J., and Rouwkema, J. (2018). Microphysiological Systems: Analysis of the Current Status, Challenges and Commercial Future. Microphysiol Syst. 1, 1. doi:10.21037/mps.2018.10.01
Ritter, S. Y., Subbaiah, R., Bebek, G., Crish, J., Scanzello, C. R., Krastins, B., et al. (2013). Proteomic Analysis of Synovial Fluid from the Osteoarthritic Knee: Comparison with Transcriptome Analyses of Joint Tissues. Arthritis & Rheumatism 65, 981–992. doi:10.1002/art.37823
Roberts, S., Weightman, B., Urban, J., and Chappell, D. (1986). Mechanical and Biochemical Properties of Human Articular Cartilage in Osteoarthritic Femoral Heads and in Autopsy Specimens. J. Bone Jt. Surg. Br. volume 68-B, 278–288. doi:10.1302/0301-620x.68b2.3958016
Rosser, J., Bachmann, B., Jordan, C., Ribitsch, I., Haltmayer, E., Gueltekin, S., et al. (2019). Microfluidic Nutrient Gradient-Based Three-Dimensional Chondrocyte Culture-On-A-Chip as an In Vitro Equine Arthritis Model. Mater. Today Bio 4, 100023. doi:10.1016/j.mtbio.2019.100023
Rossiter, H., Copic, D., Direder, M., Gruber, F., Zoratto, S., Marchetti-Deschmann, M., et al. (2021). Autophagy Protects Murine Preputial Glands against Premature Aging, and Controls Their Sebum Phospholipid and Pheromone Profile. Autophagy 00, 1–15. doi:10.1080/15548627.2021.1966716
Rothbauer, M., Byrne, R. A., Schobesberger, S., Olmos Calvo, I., Fischer, A., Reihs, E. I., et al. (2021). Establishment of a Human Three-Dimensional Chip-Based Chondro-Synovial Coculture Joint Model for Reciprocal Cross Talk Studies in Arthritis Research. Lab. Chip 21, 4128–4143. doi:10.1039/d1lc00130b
Rothbauer, M., Charwat, V., Bachmann, B., Sticker, D., Novak, R., Wanzenböck, H., et al. (2019). Monitoring Transient Cell-To-Cell Interactions in a Multi-Layered and Multi-Functional Allergy-On-A-Chip System. Lab. Chip 19, 1916–1921. doi:10.1039/c9lc00108e
Rothbauer, M., Eilenberger, C., Spitz, S., Bachmann, B. E. M., Kratz, S. R. A., Reihs, E. I., et al. (2022). Recent Advances in Additive Manufacturing and 3D Bioprinting for Organs-On-A-Chip and Microphysiological Systems. Front. Bioeng. Biotechnol. 10, 837087. doi:10.3389/fbioe.2022.837087
Rothbauer, M., Höll, G., Eilenberger, C., Kratz, S. R. A., Farooq, B., Schuller, P., et al. (2020). Monitoring Tissue-Level Remodelling during Inflammatory Arthritis Using a Three-Dimensional Synovium-On-A-Chip with Non-invasive Light Scattering Biosensing. Lab. Chip 20, 1461–1471. doi:10.1039/c9lc01097a
Rothbauer, M., Zirath, H., and Ertl, P. (2018). Recent Advances in Microfluidic Technologies for Cell-To-Cell Interaction Studies. Lab. Chip 18, 249–270. doi:10.1039/c7lc00815e
Ryu, H., Oh, S., Lee, H. J., Lee, J. Y., Lee, H. K., and Jeon, N. L. (2015). Engineering a Blood Vessel Network Module for Body-On-A-Chip Applications. SLAS Technol. 20, 296–301. doi:10.1177/2211068214562831
Sabaratnam, S., Arunan, V., Coleman, P. J., Mason, R. M., and Levick, J. R. (2005). Size Selectivity of Hyaluronan Molecular Sieving by Extracellular Matrix in Rabbit Synovial Joints. J. Physiol. 567, 569–581. doi:10.1113/jphysiol.2005.088906
Salgado, C., Jordan, O., and Allémann, E. (2021). Osteoarthritis In Vitro Models: Applications and Implications in Development of Intra-articular Drug Delivery Systems. Pharmaceutics 13, 60–23. doi:10.3390/pharmaceutics13010060
Schett, G., Tohidast-Akrad, M., Steiner, G., and Smolen, J. (2001). The Stressed Synovium. Arthritis Res. Ther. 3, 80–86. doi:10.1186/ar144
Sellam, J., and Berenbaum, F. (2013). Is Osteoarthritis a Metabolic Disease? Jt. Bone Spine 80, 568–573. doi:10.1016/j.jbspin.2013.09.007
Shabestani Monfared, G., Ertl, P., and Rothbauer, M. (2020). An On-Chip Wound Healing Assay Fabricated by Xurography for Evaluation of Dermal Fibroblast Cell Migration and Wound Closure. Sci. Rep. 10. doi:10.1038/s41598-020-73055-7
Shi, J., Liang, Q., Zuscik, M., Shen, J., Chen, D., Xu, H., et al. (2014). Distribution and Alteration of Lymphatic Vessels in Knee Joints of Normal and Osteoarthritic Mice. Arthritis & Rheumatology 66, 657–666. doi:10.1002/art.38278
Sieber, S., Michaelis, M., Gühring, H., Lindemann, S., and Gigout, A. (2020). Importance of Osmolarity and Oxygen Tension for Cartilage Tissue Engineering. BioResearch Open Access 9, 106–115. doi:10.1089/biores.2020.0009
Smeriglio, P., Lai, J. H., Yang, F., and Bhutani, N. (2015). 3D Hydrogel Scaffolds for Articular Chondrocyte Culture and Cartilage Generation. JoVE 2015, 1–6. doi:10.3791/53085
Sophia Fox, A. J., Bedi, A., and Rodeo, S. A. (2009). The Basic Science of Articular Cartilage: Structure, Composition, and Function. Sports Health 1, 461–468. doi:10.1177/1941738109350438
Statham, P., Jones, E., Jennings, L. M., and Fermor, H. L. (2021). Reproducing the Biomechanical Environment of the Chondrocyte for Cartilage Tissue Engineering. Tissue Eng. Part B Rev. 00, 1–16. doi:10.1089/ten.teb.2020.0373
Stephenson, W., Donlin, L. T., Butler, A., Rozo, C., Bracken, B., Rashidfarrokhi, A., et al. (2018). Single-cell RNA-Seq of Rheumatoid Arthritis Synovial Tissue Using Low-Cost Microfluidic Instrumentation. Nat. Commun. 9, 1–10. doi:10.1038/s41467-017-02659-x
Sticker, D., Lechner, S., Jungreuthmayer, C., Zanghellini, J., and Ertl, P. (2017). Microfluidic Migration and Wound Healing Assay Based on Mechanically Induced Injuries of Defined and Highly Reproducible Areas. Anal. Chem. 89, 2326–2333. doi:10.1021/acs.analchem.6b03886
Sticker, D., Rothbauer, M., Ehgartner, J., Steininger, C., Liske, O., Liska, R., et al. (2019). Oxygen Management at the Microscale: A Functional Biochip Material with Long-Lasting and Tunable Oxygen Scavenging Properties for Cell Culture Applications. ACS Appl. Mat. Interfaces 11, 9730–9739. doi:10.1021/acsami.8b19641
Sung, J. H., Kam, C., and Shuler, M. L. (2010). A Microfluidic Device for a Pharmacokinetic-Pharmacodynamic (PK-PD) Model on a Chip. Lab. Chip 10, 446–455. doi:10.1039/b917763a
Suri, S., Gill, S. E., Massena de Camin, S., McWilliams, D. F., Wilson, D., and Walsh, D. A. (2007). Neurovascular Invasion at the Osteochondral Junction and in Osteophytes in Osteoarthritis. Ann. Rheumatic Dis. 66, 1423–1428. doi:10.1136/ard.2006.063354
Tekari, A., Egli, R. J., Schmid, V., Justiz, J., and Luginbuehl, R. (2020). A Novel Bioreactor System Capable of Simulating the In Vivo Conditions of Synovial Joints. Tissue Eng. Part C. Methods 26, 617–627. doi:10.1089/ten.tec.2020.0161
Thomson, A., and Hilkens, C. M. U. (2021). Synovial Macrophages in Osteoarthritis: The Key to Understanding Pathogenesis? Front. Immunol. 12, 1–9. doi:10.3389/fimmu.2021.678757
Tian, K., Zhong, W., Zhang, Y., Yin, B., Zhang, W., and Liu, H. (2016). Microfluidics-based Optimization of Neuroleukin-Mediated Regulation of Articular Chondrocyte Proliferation. Mol. Med. Rep. 13, 67–74. doi:10.3892/mmr.2015.4540
Tirtaatmadja, V., Boger, D. V., and Fraser, J. R. E. (1984). The Dynamic and Steady Shear Properties of Synovial Fluid and of the Components Making up Synovial Fluid. Rheol. Acta 23, 311–321. doi:10.1007/BF01332196
Toegel, S., Pabst, M., Wu, S. Q., Grass, J., Goldring, M. B., Chiari, C., et al. (2010). Phenotype-related Differential α-2,6- or α-2,3-sialylation of Glycoprotein N-Glycans in Human Chondrocytes. Osteoarthr. Cartil. 18, 240–248. doi:10.1016/j.joca.2009.09.004
Toegel, S., Plattner, V. E., Wu, S. Q., Goldring, M. B., Chiari, C., Kolb, A., et al. (2009). Lectin Binding Patterns Reflect the Phenotypic Status of In Vitro Chondrocyte Models. Vitro Cell.Dev.Biol.-Animal 45, 351–360. doi:10.1007/s11626-009-9186-5
Topoluk, N., Steckbeck, K., Siatkowski, S., Burnikel, B., Tokish, J., and Mercuri, J. (2018). Amniotic Mesenchymal Stem Cells Mitigate Osteoarthritis Progression in a Synovial Macrophage‐mediated In Vitro Explant Coculture Model. J. Tissue Eng. Regen. Med. 12, 1097–1110. doi:10.1002/term.2610
Unger, M. A., Chou, H.-P., Thorsen, T., Scherer, A., and Quake, S. R. (2000). Monolithic Microfabricated Valves and Pumps by Multilayer Soft Lithography. Science 288, 113–116. doi:10.1126/science.288.5463.113
Urban, J. P. G., Hall, A. C., and Gehl, K. A. (1993). Regulation of Matrix Synthesis Rates by the Ionic and Osmotic Environment of Articular Chondrocytes. J. Cell. Physiol. 154, 262–270. doi:10.1002/jcp.1041540208
Vandenbosch, M., Nauta, S. P., Svirkova, A., Poeze, M., Heeren, R. M. A., Siegel, T. P., et al. (2021). Sample Preparation of Bone Tissue for MALDI-MSI for Forensic and (Pre)clinical Applications. Anal. Bioanal. Chem. 413, 2683–2694. doi:10.1007/s00216-020-02920-1
Vernon, L., Abadin, A., Wilensky, D., Huang, C.-Y. C., and Kaplan, L. (2014). Subphysiological Compressive Loading Reduces Apoptosis Following Acute Impact Injury in a Porcine Cartilage Model. Sports Health 6, 81–88. doi:10.1177/1941738113504379
Wang, X., Ning, Y., Zhang, P., Poulet, B., Huang, R., Gong, Y., et al. (2021). Comparison of the Major Cell Populations Among Osteoarthritis, Kashin-Beck Disease and Healthy Chondrocytes by Single-Cell RNA-Seq Analysis. Cell Death Dis. 12. doi:10.1038/s41419-021-03832-3
Wang, Y. I., and Shuler, M. L. (2018). UniChip Enables Long-Term Recirculating Unidirectional Perfusion with Gravity-Driven Flow for Microphysiological Systems. Lab. Chip 18, 2563–2574. doi:10.1039/c8lc00394g
Watson, R. S., Levings, P. P., Wright, T., and Ghivizzani, S. C. (2011). A Model of Arthrofibrosis Using Intraarticular Gene Delivery of Transforming Growth Factor-Beta1. J. Cell Commun. Signal. 5, 268–269.
Weinberger, A., and Simkin, P. A. (1989). Plasma Proteins in Synovial Fluids of Normal Human Joints. Seminars Arthritis Rheumatism 19, 66–76. doi:10.1016/0049-0172(89)90087-5
Whisler, J. A., Chen, M. B., and Kamm, R. D. (2014). Control of Perfusable Microvascular Network Morphology Using a Multiculture Microfluidic System. Tissue Eng. Part C. Methods 20, 543–552. doi:10.1089/ten.tec.2013.0370
Xu, H., Edwards, J., Banerji, S., Prevo, R., Jackson, D. G., and Athanasou, N. A. (2003). Distribution of Lymphatic Vessels in Normal and Arthritic Human Synovial Tissues. Ann. Rheumatic Dis. 62, 1227–1229. doi:10.1136/ard.2003.005876
Xue, X.-T., Zhang, T., Cui, S.-J., He, D.-Q., Wang, X.-D., Yang, R.-L., et al. (2018). Sexual Dimorphism of Estrogen-Sensitized Synoviocytes Contributes to Gender Difference in Temporomandibular Joint Osteoarthritis. Oral Dis. 24, 1503–1513. doi:10.1111/odi.12905
Zelenski, N. A., Leddy, H. A., Sanchez-Adams, J., Zhang, J., Bonaldo, P., Liedtke, W., et al. (2015). Type VI Collagen Regulates Pericellular Matrix Properties, Chondrocyte Swelling, and Mechanotransduction in Mouse Articular Cartilage. Arthritis & Rheumatology 67, 1286–1294. doi:10.1002/art.39034
Zhang, F., Wei, K., Wei, K., Slowikowski, K., Fonseka, C. Y., Rao, D. A., et al. (2019). Defining Inflammatory Cell States in Rheumatoid Arthritis Joint Synovial Tissues by Integrating Single-Cell Transcriptomics and Mass Cytometry. Nat. Immunol. 20, 928–942. doi:10.1038/s41590-019-0378-1
Zhang, R., and Larsen, N. B. (2017). Stereolithographic Hydrogel Printing of 3D Culture Chips with Biofunctionalized Complex 3D Perfusion Networks. Lab. Chip 17, 4273–4282. doi:10.1039/c7lc00926g
Zhang, X., Blalock, D., and Wang, J. (2015). “Classifications and Definitions of Normal Joints,” in Osteoarthritis - Progress in Basic Research and Treatment. Editor D. Blalock (Rijeka: IntechOpen). Ch. 1. doi:10.5772/59977
Zhen, G., Guo, Q., Li, Y., Wu, C., Zhu, S., Wang, R., et al. (2021). Mechanical Stress Determines the Configuration of TGFβ Activation in Articular Cartilage. Nat. Commun. 12, 1706. doi:10.1038/s41467-021-21948-0
Keywords: osteoarthritis, organs-on-a-chip, microphysiological system (MPS), roadmap, disease modeling, alternative methods to animal testing
Citation: Rothbauer M, Reihs EI, Fischer A, Windhager R, Jenner F and Toegel S (2022) A Progress Report and Roadmap for Microphysiological Systems and Organ-On-A-Chip Technologies to Be More Predictive Models in Human (Knee) Osteoarthritis. Front. Bioeng. Biotechnol. 10:886360. doi: 10.3389/fbioe.2022.886360
Received: 28 February 2022; Accepted: 21 April 2022;
Published: 15 June 2022.
Edited by:
Dimitrios Kouroupis, University of Miami, United StatesReviewed by:
Lucie Low, National Center for Advancing Translational Sciences (NIH), United StatesCopyright © 2022 Rothbauer, Reihs, Fischer, Windhager, Jenner and Toegel. This is an open-access article distributed under the terms of the Creative Commons Attribution License (CC BY). The use, distribution or reproduction in other forums is permitted, provided the original author(s) and the copyright owner(s) are credited and that the original publication in this journal is cited, in accordance with accepted academic practice. No use, distribution or reproduction is permitted which does not comply with these terms.
*Correspondence: Mario Rothbauer, bWFyaW8ucm90aGJhdWVyQG1lZHVuaXdpZW4uYWMuYXQ=
†These authors have contributed equally to this work
Disclaimer: All claims expressed in this article are solely those of the authors and do not necessarily represent those of their affiliated organizations, or those of the publisher, the editors and the reviewers. Any product that may be evaluated in this article or claim that may be made by its manufacturer is not guaranteed or endorsed by the publisher.
Research integrity at Frontiers
Learn more about the work of our research integrity team to safeguard the quality of each article we publish.