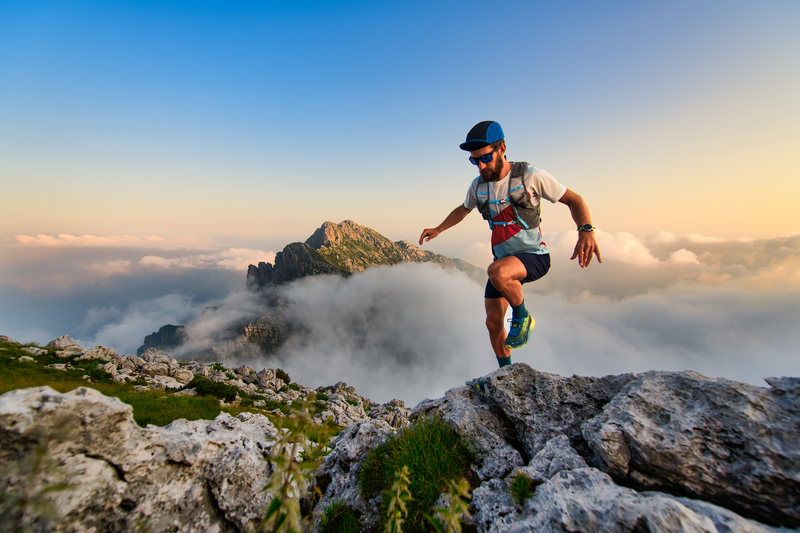
94% of researchers rate our articles as excellent or good
Learn more about the work of our research integrity team to safeguard the quality of each article we publish.
Find out more
ORIGINAL RESEARCH article
Front. Bioeng. Biotechnol. , 22 August 2022
Sec. Synthetic Biology
Volume 10 - 2022 | https://doi.org/10.3389/fbioe.2022.859600
This article is part of the Research Topic Synthetic Live Therapy View all 7 articles
Gut metabolites are pivotal mediators of host-microbiome interactions and provide an important window on human physiology and disease. However, current methods to monitor gut metabolites rely on heavy and expensive technologies such as liquid chromatography-mass spectrometry (LC-MS). In that context, robust, fast, field-deployable, and cost-effective strategies for monitoring fecal metabolites would support large-scale functional studies and routine monitoring of metabolites biomarkers associated with pathological conditions. Living cells are an attractive option to engineer biosensors due to their ability to detect and process many environmental signals and their self-replicating nature. Here we optimized a workflow for feces processing that supports metabolite detection using bacterial biosensors. We show that simple centrifugation and filtration steps remove host microbes and support reproducible preparation of a physiological-derived media retaining important characteristics of human feces, such as matrix effects and endogenous metabolites. We measure the performance of bacterial biosensors for benzoate, lactate, anhydrotetracycline, and bile acids, and find that they are highly sensitive to fecal matrices. However, encapsulating the bacteria in hydrogel helps reduce this inhibitory effect. Sensitivity to matrix effects is biosensor-dependent but also varies between individuals, highlighting the need for case-by-case optimization for biosensors’ operation in feces. Finally, by detecting endogenous bile acids, we demonstrate that bacterial biosensors could be used for future metabolite monitoring in feces. This work lays the foundation for the optimization and use of bacterial biosensors for fecal metabolites monitoring. In the future, our method could also allow rapid pre-prototyping of engineered bacteria designed to operate in the gut, with applications to in situ diagnostics and therapeutics.
The human gut microbiota contains a large number of interacting species of bacteria, archaea, bacteriophages, eukaryotic viruses, and fungi which together create a complex ecosystem able to influence human physiology, pathologies, and behavior (Oliphant et al., 2019; Fan and Pedersen, 2020). The microbiome plays important roles in human homeostasis mostly related to metabolism (Smith et al., 2013). Multiple studies have linked abnormal-gut microbiota with altered metabolite profiles of patients with different diseases such as metabolic liver disease (Jiang et al., 2015; Schwenger et al., 2019), inflammatory bowel disease (Duboc et al., 2013; Nikolaus et al., 2017; Franzosa et al., 2019) as well as metabolic disorders like obesity and malnutrition (Ridaura et al., 2013; Smith et al., 2013; Sharon et al., 2014).
The analysis of feces metabolites has opened a new window on the complex interactions occurring within the gut (Wang et al., 2011; Patterson et al., 2016; Woting and Blaut, 2016; Jia et al., 2018). In the clinics, simple and quantitative tests enable measurements of fatty acid content or malabsorption of carbohydrates by analyzing the pH of feces (Caballero et al., 1983; Eherer and Fordtran, 1992). The detection of fecal proteins, particularly calprotectin, helps diagnose and monitor inflammatory bowel diseases (Manceau et al., 2017). In addition, bacterial infections of the gut can be detected using culture-based or molecular genotyping strategies (Karu et al., 2018). Finally, mass spectrometry coupled with liquid chromatography (LC-MS) has been successfully applied to measure the levels of metabolites in human feces allowing the prediction of key associations between diet and microbiome (Bjerrum et al., 2015; Bar et al., 2020). While LC-MS is increasingly used in clinical diagnosis and allows for general and precise metabolic profiling, it is still impractical and expensive for daily monitoring of metabolites (Seger and Salzmann, 2020). Additionally, heavy methods such as LC-MS are not deployable in the field or at home, restricting large-scale prospective routine monitoring of patients. The development of innovative point-of-care (POC) testing could enable real time clinical decision-making, eliminating requirements for specialists to perform and analyze the test. Therefore, new technologies are needed to support fast, field-deployable, and cost-effective detection of the metabolites produced by the microbiome in human samples such as feces.
Programmable bacteria present an attractive technology for engineering portable biosensor devices that could help address these challenges. A biosensor is composed of a biological sensing component, which recognizes a chemical or physical change, coupled to a transducing element that produces a measurable signal in response to the environmental change (Daunert et al., 2000). A wide number of bacterial biosensors have been engineered to detect different types of analytes by connecting natural transcriptional responses to different reporter genes (Chang et al., 2017; Hicks et al., 2020). Bacterial biosensors have significant potential in applications for medical diagnosis as they perform analyte detection with a robust response, high sensitivity, and can be optimized to detect molecules in complex media such as clinical human samples (Courbet et al., 2015; Watstein and Styczynski, 2017; Chang et al., 2021). Moreover, they are inexpensive and easy to manipulate and store. Synthetic biology has enabled bacterial biosensor improvement by providing a large number of standardized genetic parts, together with systematic strategies for organism engineering, resulting in biosensors with a higher specificity, able to detect molecules in a relevant range of concentration (Hicks et al., 2020).
In addition, synthetic biology has demonstrated the promising in vivo application of engineered bacteria for the treatment of diseases, including metabolic disorders (Nelson et al., 2021), infections (Daeffler et al., 2017; Riglar and Silver, 2018), and modulation of the tumor microenvironment (Guan et al., 2021). Characterizing and optimizing the sensing performance of these bacteria under physiological conditions could improve their in vivo applications and therapeutics abilities.
Here we developed a rapid workflow for functional assessment of bacterial biosensors in fecal samples. We characterized the sensing performance of five bacterial biosensors on fecal solutions of IBD patients. Three of the biosensors evaluated are based on cytosolic transcription factor systems: a biosensor responding to benzoate, based on BenR activator and the pBEN promoter (Libis et al., 2016; Zúñiga et al., 2020); a biosensor responding to anhydrotetracycline (aTc), based on TetR repressor and the pTET promoter (Lutz and Bujard, 1997; Courbet et al., 2015), and a biosensor responding to l-lactate, based on LldR regulator and the pALPAGA promoter (Zúñiga et al., 2021). The other two biosensors, TcpP/TcpH and VtrA/VrtC, correspond to transmembrane chimeric receptors for bile salts, activated via ligand-induced dimerization (Chang et al., 2021).
We have assessed the matrix effect of feces on their response, which changed depending on the type of biosensor and the target molecule. Using this method, we were able to detect exogenously added metabolites in presence of fecal solution. Even more, we detected endogenous bile salts in some samples, demonstrating that the performance of bacterial biosensors could be optimized for fecal metabolite detection and used as a monitoring strategy. In the future, this method could be used for rapid pre-prototyping of engineered bacteria designed to operate in the gut, with applications to in vivo diagnosis and therapeutics.
Details about all bacterial biosensor used in this study are provided in Supporting Information (Supplementary Table S1). All experiments were performed using the E. coli strains DH5αZ1 and NEB10β (New England Biolabs). The different biosensors were grown in LB media with corresponding antibiotics (kanamycin 25 μg/ml or chloramphenicol 25 μg/ml). The inducers were: anhydrotetracycline used at a final concentration of 200 nM, benzoic acid used at a final concentration of 100 μM, l-lactate used at a final concentration of 10 mM and taurocholic acid (TCA) and glycodeoxycholic acid (GDCA) used at different final concentrations. All chemicals used in this research were purchased from Sigma-Aldrich.
Feces samples from routine monitoring of IBD patients were obtained from the Hepatogastroenterology and Bacteriology service at Centre Hospitalier Universitaire (CHU) Montpellier (France), in accordance with ethics committee approval (# 202101009). About 100–120 mg of samples were collected by using a Copan Liquid Amies Elution Swab (ESwab®) Collection and Transport System (ESWABR1, Copan ITALIA S. p.A). This consisted in a screw-cap tube filled with 1 ml of ESwab™ buffer, a modified liquid Amies medium (Amies, 1967), and is widely used for clinical samples preservation during transportation, both in the hospital and in field experiments, making our protocol compatible with existing workflows (Perry, 1997; Gumede et al., 2017; Saliba et al., 2020). ESwab™ buffer contains sodium chloride, potassium chloride, magnesium chloride, calcium chloride, monopotassium phosphate, disodium phosphate, and sodium thioglycollate (used to maintain the reducing condition of the media, and avoid oxidations of metabolites), but it does not contain charcoal as the original. After resuspension in the buffer, samples were immediately stored at −80°C until use. All experiments involving feces were performed in a containment level 2 laboratory.
Collected samples on ESwab™ buffer were defrosted and homogenized for 2 min by vortexing, then centrifuged at 4,000 rpm for 10 min in Eppendorf tubes. The supernatant was recovered in a new Eppendorf tube and stored at −20°C until use. Further processing by filtering was done by using a 13 mm diameter sterile syringe filter with a 0.45 µm or 0.2 µm pore size hydrophilic PVDF membrane (Millex-HV Syringe Filter, Millipore). The dilution of feces solution was done following the general mixing of volumes; 75 µl 2X LB medium, plus 1.5 µl of biosensor culture, plus 3 µl of inducer adjusted at the needed concentration and 70.5 µl of the feces solution diluted in ESwab™ buffer to have a final volume of 150 μl, as follow; for 10% final feces concentration: 15 µl of feces samples plus 55.5 µl of ESwab™ buffer, for 25% final feces concentration: 37.5 µl of feces samples plus 33 µl of ESwab™ buffer and for 50% dilution 75 µl of feces samples. See Supplementary Protocols for a step-by-step procedure.
Bacterial biosensors from glycerol stock were plated on LB agar plates supplemented with antibiotics and incubated at 37°C overnight. For functional characterization, three fresh colonies of each bacterial biosensor were picked and inoculated into 0.5 ml of LB with corresponding antibiotics and grown at 37°C for 16 h in 96 DeepWell polystyrene plates (Thermo Fisher Scientific, 278,606) sealed with AeraSeal film (Sigma-Aldrich, A9224-50EA) with shaking (200 rpm) and 80% of humidity in a Kuhner LT-X (Lab-Therm) incubator shaker. The next day, the cultures were diluted 1:100 into a final volume of 150 μl of LB supplemented or not with different dilutions of feces samples, and corresponding inducers, in 96-well plates, incubated at 37°C without shaking for 16 h, and analyzed by flow cytometry. Pooled or individual samples of fecal solution were used depending on the experiment. Pooled samples were used to avoid patient-specific matrix effect and describe the global effect of fecal solution in the biosensor performance. Individual patient samples, in contrast, were used to determine patient-specific variation and matrix effect, and to measure individual metabolites levels for bile salts. Pooled or individual fecal solutions were diluted with various volumes of ESwab homogenization buffer depending on the final target feces concentration. The cultures were incubated at 37°C for 16 h without shaking, with the goal of having the simplest protocol possible for future field application to metabolite detection. Next, cells were well mixed and 100-times diluted in 1X Attune Focusing Fluid (Thermo Fisher Scientific) before cytometry analysis (for more details on the method see Supplementary Figure S2). See Supplementary Protocols for a step-by-step procedure.
Total bile acids in feces were measured using Bile Acid Assay Kit (Sigma-Aldrich MAK309, Merck, France). The l-lactate concentration was measured using a l-lactate Assay Kit (Sigma-Aldrich MAK329, Merck, France). 20 µl of pre-treated and 0.4 µm filtered feces samples were used for each reaction. All measurements were performed in duplicate on two different days.
Flow cytometry was performed on an Attune NxT flow cytometer (Thermo Fisher) equipped with an autosampler and Attune NxTTM Version 2.7 Software. Experiments on Attune NxT were performed in 96-well plates with settings; FSC: 200 V, SSC: 380 V, green intensity BL1 488 nm laser, and a 510/10 nm filter. All events were collected with a cutoff of 20.000 events. A control cell-line of E. coli containing a reference construct was grown in parallel for each experiment. This in vivo reference construct has a constitutive promoter J23101 and RBS_B0032 controlling the expression of a superfolder GFP as a reporter gene in the plasmid pSB4K5. The cells were gated based on forward and side scatter graphs. The events on single-cell gates were selected and analyzed to remove debris from the analysis by using Flow-Jo (Treestar, Inc.) software. The gating strategy is depicted in Supplementary Figure S5.
BenR-pBEN biosensor was grown in LB medium with 25 μg/ml chloramphenicol at 37°C with shaking at 200 rpm for 16 h. Cells were then centrifuged at 4,000 rpm for 5 min and resuspended in fresh LB medium with 25 μg/ml chloramphenicol to an absorbance of 4 at 600 nm. A 5% w/v of alginate solution was prepared by dissolving medium viscosity alginate (Sigma-Aldrich A2033) in MilliQ water and autoclave sterilized. The liquid solution of the biosensor was mixed with the alginate solution at 1:1 ratio to reach 2.5% alginate and roughly 2 × 109 cells/ml. This mixture of alginate and bacteria was then dropped into a sterile 5% w/v CaCl2 solution (Sigma-Aldrich C1016) to form beads of 2 mm diameter. Beads were cross-linked for 5 min in CaCl2 solution. See Supplementary Protocols for a step-by-step procedure.
BenR-pBEN biosensor alginate beads and non-encapsulated biosensor with the same bacterial concentration were incubated with LB medium, chloramphenicol 25 μg/ml and pooled fecal solutions from four different patients at different percentages, containing or not exogenously added benzoate at 100 µM. Induction was performed in a black 384-well plate with a clear flat bottom (Corning, United States) at 37°C for 16 h without shaking. The ESwab™ buffer was used to adjust the different percentages of feces as described in the feces processing section. The fluorescence intensity of the reporter gene and the bacterial optical density were then measured using the Cytation3 plate reader (Biotek instruments). The experiment was done in triplicates in two different days. See Supplementary Protocols for a step-by-step procedure.
The calculation of relative promoter units (RPUs (Kelly et al., 2009)) was done by normalizing the fluorescence intensity measurements of each biosensor according to the fluorescence intensity of the control cell-line E. coli harboring a reference construct. We quantified the geometric mean of fluorescence intensity (MFI) of the flow cytometry data and calculated RPUs according to the following equation:
The goodness of fit and the EC50 for each data from bile acids biosensors set were calculated by applying non-linear regression using Agonist vs response-variable slope function using GraphPad Prism.
The fluorescence raw data from encapsulated BenR-pBEN biosensor was processed by subtracting autofluorescence and normalizing by the absorbance at 600 nm. Then the relative percentage of activity (RPA) of the biosensors was calculated using the following expression:
Where Fis corresponds to the normalized fluorescence in presence of different % of fecal solution plus the inducer while Fic corresponds to the normalized fluorescence without fecal solution in presence of the inducer.
Statistical analysis was performed using GraphPad Prism unpaired t-student test and two-way ANOVA with Fisher’s LSD multiple comparisons test.
Fecal samples preparation for matrix effect assessment on bacterial biosensor performance.
We first aimed to evaluate the matrix effect of feces on bacterial biosensors functionality. The first step in this process was to determine a simple and robust protocol to prepare a feces-derived solution (fecal solution thereafter) to which biosensors can be exposed. Our aim was to obtain from feces a sample that contains feces-derived metabolites yet is liquid so can be easily and reproducibly handled for testing with bacterial biosensors. The solution samples from 4 patients were recovered (see materials and methods for details) and pooled, to reduce the sample variability in the matrix effect estimation. Four treatment methods were applied to feces solution: 1) no filtration, meaning no change in the original microbial composition of the feces, 2) no filtration + antibiotics, to inhibit the growth of the endogenous bacterial microbiome during the experiment, 3) filtration at 0.45 µm and 4) filtration at 0.2 µm, to eliminate host-derived microorganisms in the samples (Figure 1A). Analysis of colony forming units (CFU) and absorbance at OD600 in LB medium confirmed growth of microorganisms only in fecal solutions without filtration (Supplementary Figure S1A), keeping a portion of the microbial composition able to grow under the conditions in which the bacterial biosensor performs. We evaluated the global matrix effect of pooled fecal samples by using a BenR-pBEN benzoate biosensor, based on a soluble regulator, due to the low concentration of this metabolite in human feces (Jenner et al., 2005; Muñoz-González et al., 2013; Gutiérrez-Díaz et al., 2018). We assessed the activity of the BenR-pBEN biosensor, with a limit of detection of 80 µM (Libis et al., 2016; Zúñiga et al., 2020), to sense exogenously added benzoate in presence of fecal solutions diluted at final concentration of 75%, 50%, and 25% vol/vol (Figure 1A) (see methods). We then evaluated the GFP fluorescence signal of the biosensor in the presence or in the absence of 100 µM benzoate (Figure 1B, Supplementary Figure S1). After 16 h of induction at 37°C, considerable inhibitory effects were observed in the benzoate biosensor output signal in 75% and 50% of feces (Supplementary Figure S1B). On the other hand, 25% of fecal solution allowed for a better sensing performance (Figure 1B). However, the fluorescence measurement was significantly lower in non-filtered samples compared to samples filtered with 0.45 and 0.2 µm (Figure 1B). In addition, a fraction of cells with no detectable fluorescence was observable in non-filtered feces induced with benzoate, probably corresponding to live or dead endogenous host microorganisms present in the matrix, reaching the 30% of the total population in the condition non-filtered but with antibiotics and more than 46% in condition with non-filtered fecal sample (Figure 1B and Supplementary Figure S1). Since the filtering of fecal samples at 0.2 µm did not show a significant improvement from the filtered at 0.4 µm, we chose the filtering at 0.4 µm to reduce the matrix effect of feces as a final protocol step. These results demonstrate that feces samples have significant inhibitory matrix effects on bacterial biosensors’ performance but by filtering and diluting fecal solutions, this effect can be reduced allowing bacterial biosensors to detect exogenously added metabolites.
FIGURE 1. Human feces preparation to reduce matrix effects on bacterial biosensor performance. (A) Schematic workflow of the fecal matter preparation to assess matrix effect on benzoate bacterial biosensor performance. Samples were defrosted, homogenized, and centrifuged before evaluating the pBEN biosensor performance on different final fecal solutions. (B) Matrix effects of different fecal solutions on BenR-pBEN bacterial biosensor. Cells were induced with 100 µM benzoate (bottom) or non-induced (top) in presence of a 4-time diluted feces sample and incubated at 37°C without shaking for 16 h. Dark gray and gray histograms correspond to non-fluorescent populations. Green histograms correspond to the GFP-fluorescence population. Dotted lines represent the mean of the fluorescence produced by the biosensor growing in LB only, yellow: without benzoate; orange with 100 µM of benzoate. Each histogram is representative of two independent experiments measured by flow cytometry.
We then evaluated the sensing performance of different bacterial biosensors in presence of feces samples. We evaluated five different biosensors for: aTc; l-lactate; primary bile acids, taurocholic acid (TCA); secondary bile acids, glycodeoxycholic acid (GDCA); and benzoate. We evaluated the matrix effects on the sensing performance of these biosensors by adding feces samples at 2, 4, and 10-fold dilutions (50%, 20%, and 10% feces respectively, Figure 2A, see methods and Supplementary Figure S2). To observe the variability of the matrix effect we used solutions from six different patients (samples S1-S6). For TcpP/TcpH and VtrA/VtrC, in order to avoid interference from endogenous cognate ligands on the biosensor, the samples were chosen for their low content in total bile acids, as measured by an enzymatic assay, corresponding to samples S4-S6 (Figure 2A, Supplementary Table S2). All biosensors were able to detect their specific inducers in 10% feces samples. However, most of them failed to produce fluorescence in 50% of feces, except for lactate biosensors that showed a higher fluorescence when a higher concentration of feces was added. This was due to the endogenous presence of lactate in the samples (Supplementary Table S2), confirmed by enzymatic assay. Indeed, a feces sample with low measured lactate concentration (Figure 2A, patient S2) did not exhibit such increase in fluorescence. The different bacterial biosensors showed diverse degrees of sensitivity to fecal matrices (Figure 2B, Supplementary Figure S3, Table 1, and Supplementary Table S3). TetR was strongly inhibited (more than 50%), even in 10% feces. Interestingly, the VtrA/VtrC system exhibited a non-homogeneous response and was strongly inhibited too, although relying on a similar architecture to TcpP/TcpH, which was not significantly affected. This difference might be due to the fact that the behavior of TcpP was previously optimized by circuit tuning and directed evolution (Chang et al., 2021).
FIGURE 2. Performance of different bacterial biosensors in human feces. (A) Matrix effects of human feces samples on the performance of five different biosensors. Samples from different patients were used, S1-S6 represent the labels for the different patient samples. Biosensors at the top are based on cytosolic transcription factor systems: the BenR activator and the pBEN promoter; the TetR repressor and the pTET promoter and the LldR regulator and the pALPAGA promoter, induced with 100 µM benzoate, 200 nM aTc and 10 mM l-lactate, respectively. Sensors at the bottom are based on transmembrane receptors activated via ligand-induced dimerization, responding to bile acids: TcpP/TcpH to 100 µM TCA and VtrA/VtrC heterodimeric complex to 100 µM GDCA. Samples were used at final percentages; 10%, 25%, and 50% feces. Fluorescence intensities are expressed in relative promoter units (RPU) (see methods). The mean of three independent experiments performed in duplicate is plotted. The averages and standard deviations for these data are available in Table 1 and in Supplementary Data. For facilitating readability, note that the color scale was adjusted individually for each biosensor with the maximum value corresponding to values measured without the addition of fecal samples. (B) Representative histogram showing the fluorescence of the reporter gene for each biosensor expressed as a result of different induction conditions in 10% feces and measured by flow cytometry. Inducers; 100 µM benzoate, 200 nM aTc, 10 mM l-lactate, 100 µM TCA and 100 µM GDCA.
Importantly, a clear patient-to-patient variability was observed for every biosensor, suggesting that feces matrix effects responsible for biosensor inhibition could be due to specific factors having varying abundance in different patients. These results show that several bacterial biosensors can operate in diluted fecal solution, yet have diverse sensitivity to fecal matrices, requiring case-by-case optimization.
Our next goal was to evaluate if by using our workflow for fecal processing bacterial biosensors could be used to detect endogenous metabolites in patients’ fecal samples. As a demo, we chose to assess bile salts, that are present in high concentrations in feces of patients with inflammatory bowel disease (IBD) (Jansson et al., 2009; Jacobs et al., 2016; Franzosa et al., 2019; Lavelle and Sokol, 2020). We assessed the detection of endogenous bile salts by using the TcpP/TcpH and the VtrA/VtrC biosensors.
We first evaluated the dose-response curve of TcpP/TcpH for TCA, and VtrA/VtrC for GDCA, in the presence or absence of 10% fecal solution (Figure 3A). We selected feces samples with low bile salts concentrations (S5-S7) to avoid interference from endogenous ligands. The TcpP/TcpH biosensor responded remarkably to spiked TCA in 10% feces solution, with high fold change and comparable limit of detection to the biosensor operating in the absence of feces. On the other hand, and as observed previously, the VtrAC biosensor was strongly inhibited by the presence of feces solution, confirming the need for additional optimization before performing detection of bile salt under these conditions. We then tested TcpP/TcpH for endogenous bile salts detection in feces solutions from 12 patients with IBD. We measured the total bile salts concentration with a commercial enzymatic assay (Sigma-Aldrich MAK309, Merck, France) (Supplementary Table S2) and used the same samples to evaluate the performance of TcpP/TcpH to measure endogenous bile salts (Figure 3B).
FIGURE 3. Bile acids detection in fecal solution from IBD patients. (A) The response function of TcpP/TcpH biosensor (left) and VtrA/VtrC biosensor (right) to spiked taurocholic acid (TCA) and glycodeoxycholic acid (GDCA) in the presence of 10-fold diluted feces (three different samples were pooled; samples S5, S6 and S7, see Supplementary Table S2). Data points correspond to the mean value of four replicates on four different days. Error bars: ±SD. (B) Comparison of total bile acid detection between TcpP/TcpH (blue square) biosensor, right axis, and enzymatic assay (yellow line) left axis. Samples were ordered according to their total bile salts concentration measured by the enzymatic assay. Data points correspond to the mean value of three replicates performed in duplicate on three different days. Error bars: ±SD.
Samples exhibited different bile salts concentrations, all of them higher than 29 µM and two having very high bile salts concentrations (Samples S17 and S18, >500 μM, see Supplementary Table S2). The TcpP/TcpH system was able to detect bile salts in 5 different samples, with a strong response for samples S17 and S18. For the other samples, we observed a variable response from the biosensor (Figure 3B). These discrepancies might be due to several factors: 1) the biosensor LOD is too high for detecting low bile salts concentration. 2) Patient-specific matrix effects affect biosensor operation in some samples (e.g.S16) and 3) differential distribution of bile salts species depending on the patient: the enzymatic assay quantifies total bile salts, while the TcpP/TcpH system responds more specifically to primary bile salts, such as glycocholic acid (GCA), taurocholic acid (TCA), glycochenodeoxycholic acid (GCDCA), and taurochenodeoxycholic acid (TCDCA) (Chang et al., 2021). Importantly, in patients with IBD such as the ones in our study, the distribution between primary and secondary bile salts can greatly vary (Jacobs et al., 2016; Franzosa et al., 2019).
These results show that with an appropriate optimization of bacterial biosensors our workflow for fecal solution preparation could support the rapid detection of endogenous metabolites in human feces samples.
Hydrogels beads have been studied as a desirable materials for encapsulating bacteria because they provide survival under stress conditions, allowing cell growth and biosensing functionality (Choi et al., 2013; Courbet et al., 2015; Li et al., 2017; Tang et al., 2021). In addition, hydrogel beads offer an attractive option for bacterial biocontainment (Li et al., 2017; Tang et al., 2021). To determine if encapsulation helps to reduce the inhibitory effect of fecal solutions we evaluated the relative percentage of activity (RPA) of BenR-pBEN biosensor encapsulated in alginate beads under different fecal solutions percentages (Figure 4). We optimized the protocol of bacteria encapsulation in alginate beads to get at least 1.3 × 109 CFU/mL of live bacteria (Supplementary Figure S4) and ensure the biosensing process in fecal solution. Hydrogel beads containing the bacterial biosensor incubated in LB media for 16 h produced high fluorescence in presence of exogenously added benzoate under different concentrations of pooled fecal solution (Figure 4A). The high intensity of the fluorescence was observable under blue light (Figure 4B). We calculated the relative percentage of activity (RPA) and compared the sensing performance of biosensors in liquid culture versus encapsulated ones. We observed a significant performance improvement in beads in the presence of 50% of fecal solution compared to the liquid non-beads condition (Figure 4C), confirming a positive effect of beads encapsulation on reducing the inhibitory matrix effects of fecal solution.
FIGURE 4. Matrix effect of fecal solution on the performance of encapsulated BenR-pBEN biosensor. (A) Normalized fluorescence of encapsulated BenR-pBEN biosensor on different percentages of fecal solutions. BenR-pBEN biosensor in alginate beads was induced or not with 100 µM benzoate in presence of pooled fecal solutions from four different patients at final percentages; 10%, 25%, and 50% and incubated at 37°C without shaking for 16 h. Fluorescence was measured by plate reader and normalized by absorbance at 600 nm. The mean value and standard deviation of beads analyzed in triplicate on two different days are plotted. *: p-value < 0.05 two-way ANOVA with Fisher’s LSD multiple comparisons test. The asterisk represents significant differences between non-induced versus induced condition (red) and non-fecal solution versus different percentages of fecal solution (balck). (B) Photograph of biosensor beads at the end of the sensing experiment performed (top) on different percentages of fecal solutions under blue light. The biosensor beads contained 2.5% alginate and measured 2 mm in diameter. Black bar: 2 mm. Error bars: ±SD. RPA was calculated as described in methods. An unpaired t-student test was performed. The asterisk represents significant differences between conditions. *: p-value < 0.05. (C) Relative percentage of activity (RPA) of encapsulated BenR-pBEN biosensor at final percentages of fecal solution; 10%, 25%, and 50%. Bars correspond to the mean of non-encapsulated (in liquid condition) and encapsulated (in beads condition).
In this work, we provide an optimized method to prepare fecal solutions for prototyping bacterial biosensors in human feces. We showed that a simple filtration step is enough to remove host microbes and reproducibly obtain a physiological-derived media retaining essential characteristics of human feces, such as matrix effects and endogenous metabolites (e.g. bile acids and l-lactate). We found significant inhibitory matrix effects of feces on the bacterial biosensors tested, although the robustness of the different biosensors varied. In addition, matrix effects varied significantly from patient to patient. This patient-to-patient variability could be due to host or microbiome-derived molecules that interfere with biosensor physiology. It is also possible that some medications inhibit the biosensors. Detailed knowledge of patients’ full clinical picture and current treatments will be essential to interpret the biosensor’s response. In all, the biosensors tested here are highly sensitive to fecal samples, and the optimal working conditions in our studies were general at a 10% feces sample dilution in liquid culture. However, we show that encapsulating bacteria in alginate leads improves the robustness of the biosensor against inhibitory matrix effects. We observed a significant improvement in the sensing performance of the benzoate biosensor in alginate beads compared to non-encapsulated cells in presence of 50% of fecal solution, without the need for any other optimization. This improvement may be due to two complementary factors; first, hydrogel beads can locally concentrate bacteria at a higher density, while providing water and nutrients to the cells and allows the diffusion of the inducer molecules to sense. Second, the alginate hydrogel itself offers a physical protection under stress conditions while conserving bacterial biosensing ability, as already shown in previous work by our group and others (Choi et al., 2013; Courbet et al., 2015; Li et al., 2017; Tang et al., 2021).
From our study, we can highlight several important points for future bacterial biosensor prototyping in feces: 1) because of biosensor-specific sensitivity to fecal matrices, case-by-case optimization of every new bacterial biosensor for operation in feces is required, 2) testing the biosensor over various individual samples coming from different patients is critical to obtain a bacterial biosensor working over a wide range of real-world conditions, and 3) encapsulation of biosensors can improve their performance in fecal solution by reducing their susceptibility to matrix effects. Using our workflow, these assays can be performed rapidly.
Interestingly, the TcpP/TcpH bile salts biosensor, that detects primary bile salts, was capable of detecting high concentrations of endogenous bile salts in samples from five different patients, in accordance with enzymatic measurements. Patients with IBD have altered fecal bile salts profiles (Duboc et al., 2013; Torres et al., 2018; Lavelle and Sokol, 2020), with lower levels of secondary bile salts but higher levels of primary (Jansson et al., 2009; Jacobs et al., 2016; Franzosa et al., 2019). For other samples, data was not in accordance with the enzymatic measurements, possibly because of different, patient-specific bile salts profiles, some bile salts not being detected by our bacterial biosensor. A more definitive answer could be provided in the future by using LC/MS to determine the exact bile salts species distribution in each sample. Nevertheless, as a proof of concept, these data demonstrate, for the first time to our knowledge, the possibility of using bacterial biosensors to detect endogenous metabolites in human feces.
How could bacterial biosensors operating in fecal samples be optimized in the future? First, other reporters having a higher signal-to-noise ratio, such as luciferase, might be evaluated. Yet, unless using the luxCDABE operon, which has lower performance, optimized luciferase systems such as nanoluc, while providing a lower limit-of-detection, work better after cell lysis, which would complicate the assay protocol (Lopreside et al., 2019). Second, amplifying genetic devices such as recombinase switches or hrp transcription factors might help combat matrix effects and enable operation at higher concentrations, thereby supporting lower limits of detection (Courbet et al., 2015; Wan et al., 2019). Recombinase-mediated inversion or excision could also allow post facto analysis of biomarker presence through DNA sequencing or PCR (Courbet et al., 2015; Wan et al., 2019). Furthermore, an appropriate coating on hydrogel beads complemented with genetic devices biocontainment (e.g. auxotrophs) (Steidler et al., 2003; Chan et al., 2016; Moya-Ramírez et al., 2022) could not only allow for a better performance of the biosensor on feces but also prevent bacteria leakage in the environment allowing the deployment of these biosensors as a point-of-care metabolite monitoring device.
The method shown here could be performed on a lab-on-chip device enabling successive feces samples filtration, dilution, and sensing assay in an automated manner (Wu et al., 2017, 2018; Arshavsky-Graham and Segal, 2020). Such devices would open the door to field-deployable, point-of-care gut metabolite detection either for diagnostics or epidemiological purposes (Figure 5).
FIGURE 5. Summary of future applications for rapid prototyping of biosensors and metabolites detection in human fecal samples. By applying this method, human feces could be collected and homogenized at the hospital, and directly used to measure the levels of targeted metabolites by using bacterial biosensors. These measurements will help to understand metabolite fluctuations under a particular diet intake, for example. Similarly, this strategy would allow the engineering of point-of-care biosensors for performing medical diagnostics by measuring a set of targeted metabolites in feces. Finally, this prototyping approach could also support the engineering of therapeutic bacteria by allowing a fast characterization and optimization of their sensing performance closer to physiological conditions in fecal matrices.
Finally, another potential and compelling application of our method is its use for rapid and simple prototyping of engineered “smart” gut probiotics. Engineered bacteria have recently been developed to detect and/or treat many pathological conditions such as inflammation, diabetes, phenylketonuria, hyperammonemia, and cancer (Isabella et al., 2018; Riglar and Silver, 2018). As of now, these strains have been evaluated in animal or in cellular co-culture models (Mimee et al., 2016; Daeffler et al., 2017; Taketani et al., 2020; Nelson et al., 2021). While providing valuable information, these models present limitations in terms of time, physiological relevance, and amenability to screening. The use of human fecal samples could complement these approaches by providing a fast and efficient method to assess the matrix effects of fecal matter on bacterial sensors and therapeutics and optimize their behavior.
The original contributions presented in the study are included in the article/Supplementary Material, further inquiries can be directed to the corresponding author/s.
The studies involving human participants were reviewed and approved by the Internal Review Board of the Montpellier University Hospital IRB ID: 202101009. The patients/participants provided their written informed consent to participate in this study.
AZ, IC, and JB designed the research. AZ designed and performed all the experiments. GM-G performed all the experiments concerning alginate beads. PM contributed to perform the experiments at the L2 laboratory. LB, G-PP, and RA collected clinical samples. AZ, GM-G, and JB analyzed the data. AZ and JB wrote the article. All authors reviewed and approved the manuscript.
This work was supported by the ANR SynBioDiag grant (ANR-18CE33-0015) and ERC starting “COMPUCELL” (Grant No. 657579).
We thank members of the synthetic biology group and of the CBS for fruitful discussions and feedback. We are grateful to the patients for participating in this study and providing their samples, and to the personnel of the Montpellier CHU hospital for collecting the samples. JB also acknowledges the INSERM Atip-Avenir program and the Bettencourt-Schueller Foundation for continuous support. The CBS acknowledges support from the French Infrastructure for Integrated Structural Biology (FRISBI) ANR-10-INSB-05-01.
The authors declare that the research was conducted in the absence of any commercial or financial relationships that could be construed as a potential conflict of interest.
All claims expressed in this article are solely those of the authors and do not necessarily represent those of their affiliated organizations, or those of the publisher, the editors and the reviewers. Any product that may be evaluated in this article, or claim that may be made by its manufacturer, is not guaranteed or endorsed by the publisher.
The Supplementary Material for this article can be found online at: https://www.frontiersin.org/articles/10.3389/fbioe.2022.859600/full#supplementary-material
Amies, C. R. (1967). A modified formula for the preparation of Stuart’s Transport Medium. Can. J. Public Health 58 (7), 296–300.
Arshavsky-Graham, S., and Segal, E. (2020). Lab-on-a-Chip devices for point-of-care medical diagnostics. Adv. Biochem. Eng. Biotechnol. doi:10.1007/10_2020_127
Bar, N., Korem, T., Weissbrod, O., Zeevi, D., Rothschild, D., Leviatan, S., et al. (2020). A reference map of potential determinants for the human serum metabolome. Nature 588, 135–140. doi:10.1038/s41586-020-2896-2
Bjerrum, J. T., Wang, Y., Hao, F., Coskun, M., Ludwig, C., Günther, U., et al. (2015). Metabonomics of human fecal extracts characterize ulcerative colitis, Crohn’s disease and healthy individuals. Metabolomics 11, 122–133. doi:10.1007/s11306-014-0677-3
Caballero, B., Solomons, N. W., and Torún, B. (1983). Fecal reducing substances and breath hydrogen excretion as indicators of carbohydrate malabsorption. J. Pediatr. Gastroenterol. Nutr. 2, 487–490. doi:10.1097/00005176-198302030-00016
Chan, C. T. Y., Lee, J. W., Cameron, D. E., Bashor, C. J., and Collins, J. J. (2016). Deadman” and “Passcode” microbial kill switches for bacterial containment. Nat. Chem. Biol. 12, 82–86. doi:10.1038/nchembio.1979
Chang, H.-J., Voyvodic, P. L., Zúñiga, A., and Bonnet, J. (2017). Microbially derived biosensors for diagnosis, monitoring and epidemiology. Microb. Biotechnol. 10, 1031–1035. doi:10.1111/1751-7915.12791
Chang, H.-J., Zúñiga, A., Conejero, I., Voyvodic, P. L., Gracy, J., Fajardo-Ruiz, E., et al. (2021). Programmable receptors enable bacterial biosensors to detect pathological biomarkers in clinical samples. Nat. Commun. 12, 5216. doi:10.1038/s41467-021-25538-y
Choi, M., Choi, J. W., Kim, S., Nizamoglu, S., Hahn, S. K., and Yun, S. H. (2013). Light-guiding hydrogels for cell-based sensing and optogenetic synthesis in vivo. Nat. Photonics 7, 987–994. doi:10.1038/nphoton.2013.278
Courbet, A., Endy, D., Renard, E., Molina, F., and Bonnet, J. (2015). Detection of pathological biomarkers in human clinical samples via amplifying genetic switches and logic gates. Sci. Transl. Med. 7, 289ra83. doi:10.1126/scitranslmed.aaa3601
Daeffler, K. N.-M., Galley, J. D., Sheth, R. U., Ortiz-Velez, L. C., Bibb, C. O., Shroyer, N. F., et al. (2017). Engineering bacterial thiosulfate and tetrathionate sensors for detecting gut inflammation. Mol. Syst. Biol. 13, 923. doi:10.15252/msb.20167416
Daunert, S., Barrett, G., Feliciano, J. S., Shetty, R. S., Shrestha, S., and Smith-Spencer, W. (2000). Genetically engineered whole-cell sensing systems: Coupling biological recognition with reporter genes. Chem. Rev. 100, 2705–2738. doi:10.1021/cr990115p
Duboc, H., Rajca, S., Rainteau, D., Benarous, D., Maubert, M.-A., Quervain, E., et al. (2013). Connecting dysbiosis, bile-acid dysmetabolism and gut inflammation in inflammatory bowel diseases. Gut 62, 531–539. doi:10.1136/gutjnl-2012-302578
Eherer, A. J., and Fordtran, J. S. (1992). Fecal osmotic gap and pH in experimental diarrhea of various causes. Gastroenterology 103, 545–551. doi:10.1016/0016-5085(92)90845-p
Fan, Y., and Pedersen, O. (2020). Gut microbiota in human metabolic health and disease. Nat. Rev. Microbiol. 19, 55–71. doi:10.1038/s41579-020-0433-9
Franzosa, E. A., Sirota-Madi, A., Avila-Pacheco, J., Fornelos, N., Haiser, H. J., Reinker, S., et al. (2019). Gut microbiome structure and metabolic activity in inflammatory bowel disease. Nat. Microbiol. 4, 293–305. doi:10.1038/s41564-018-0306-4
Guan, H., Pu, Y., Liu, C., Lou, T., Tan, S., Kong, M., et al. (2021). Comparison of fecal collection methods on variation in gut metagenomics and untargeted metabolomics. mSphere 6 (5), e0063621. doi:10.1128/mSphere.00636-21
Gumede, L., Radebe, F., Nhlapo, D., Maseko, V., Kufa-Chakezha, T., and Kularatne, R. (2017). Evaluation of the Copan eSwab®, a liquid-based microbiology transport system, for the preservation ofNeisseria gonorrhoeaeat different temperatures. South. Afr. J. Infect. Dis. 32, 96–99. doi:10.1080/23120053.2017.1313935
Gutiérrez-Díaz, I., Fernández-Navarro, T., Salazar, N., Bartolomé, B., Moreno-Arribas, M. V., López, P., et al. (2018). Could fecal phenylacetic and phenylpropionic acids Be used as indicators of health status? J. Agric. Food Chem. 66, 10438–10446. doi:10.1021/acs.jafc.8b04102
Hicks, M., Bachmann, T. T., and Wang, B. (2020). Synthetic biology enables programmable cell-based biosensors. Chemphyschem 21, 132–144. doi:10.1002/cphc.201900739
Isabella, V. M., Ha, B. N., Castillo, M. J., Lubkowicz, D. J., Rowe, S. E., Millet, Y. A., et al. (2018). Development of a synthetic live bacterial therapeutic for the human metabolic disease phenylketonuria. Nat. Biotechnol. 36, 857–864. doi:10.1038/nbt.4222
Jacobs, J. P., Goudarzi, M., Singh, N., Tong, M., McHardy, I. H., Ruegger, P., et al. (2016). A disease-associated microbial and metabolomics state in relatives of pediatric inflammatory bowel disease patients. Cell. Mol. Gastroenterol. Hepatol. 2, 750–766. doi:10.1016/j.jcmgh.2016.06.004
Jansson, J., Willing, B., Lucio, M., Fekete, A., Dicksved, J., Halfvarson, J., et al. (2009). Metabolomics reveals metabolic biomarkers of Crohn’s disease. PLoS One 4, e6386. doi:10.1371/journal.pone.0006386
Jenner, A. M., Rafter, J., and Halliwell, B. (2005). Human fecal water content of phenolics: The extent of colonic exposure to aromatic compounds. Free Radic. Biol. Med. 38, 763–772. doi:10.1016/j.freeradbiomed.2004.11.020
Jia, W., Xie, G., and Jia, W. (2018). Bile acid-microbiota crosstalk in gastrointestinal inflammation and carcinogenesis. Nat. Rev. Gastroenterol. Hepatol. 15, 111–128. doi:10.1038/nrgastro.2017.119
Jiang, W., Wu, N., Wang, X., Chi, Y., Zhang, Y., Qiu, X., et al. (2015). Dysbiosis gut microbiota associated with inflammation and impaired mucosal immune function in intestine of humans with non-alcoholic fatty liver disease. Sci. Rep. 5, 8096. doi:10.1038/srep08096
Karu, N., Deng, L., Slae, M., Guo, A. C., Sajed, T., Huynh, H., et al. (2018). A review on human fecal metabolomics: Methods, applications and the human fecal metabolome database. Anal. Chim. Acta 1030, 1–24. doi:10.1016/j.aca.2018.05.031
Kelly, J. R., Rubin, A. J., Davis, J. H., Ajo-Franklin, C. M., Cumbers, J., Czar, M. J., et al. (2009). Measuring the activity of BioBrick promoters using an in vivo reference standard. J. Biol. Eng. 3, 4. doi:10.1186/1754-1611-3-4
Lavelle, A., and Sokol, H. (2020). Gut microbiota-derived metabolites as key actors in inflammatory bowel disease. Nat. Rev. Gastroenterol. Hepatol. 17, 223–237. doi:10.1038/s41575-019-0258-z
Li, P., Müller, M., Chang, M. W., Frettlöh, M., and Schönherr, H. (2017). Encapsulation of autoinducer sensing reporter bacteria in reinforced alginate-based microbeads. ACS Appl. Mat. Interfaces 9, 22321–22331. doi:10.1021/acsami.7b07166
Libis, V., Delépine, B., and Faulon, J.-L. (2016). Expanding biosensing abilities through computer-aided design of metabolic pathways. ACS Synth. Biol. 5, 1076–1085. doi:10.1021/acssynbio.5b00225
Lopreside, A., Wan, X., Michelini, E., Roda, A., and Wang, B. (2019). Comprehensive profiling of diverse genetic reporters with application to whole-cell and cell-free biosensors. Anal. Chem. 91, 15284–15292. doi:10.1021/acs.analchem.9b04444
Lutz, R., and Bujard, H. (1997). Independent and tight regulation of transcriptional units in Escherichia coli via the LacR/O, the TetR/O and AraC/I1-I2 regulatory elements. Nucleic Acids Res. 25, 1203–1210. doi:10.1093/nar/25.6.1203
Manceau, H., Chicha-Cattoir, V., Puy, H., and Peoc’h, K. (2017). Fecal calprotectin in inflammatory bowel diseases: Update and perspectives. Clin. Chem. Lab. Med. 55, 474–483. doi:10.1515/cclm-2016-0522
Mimee, M., Tucker, A. C., Voigt, C. A., and Lu, T. K. (2016). Programming a human commensal bacterium, Bacteroides thetaiotaomicron, to sense and respond to stimuli in the murine gut microbiota. Cell Syst. 2, 214. doi:10.1016/j.cels.2016.03.007
Moya-Ramírez, I., Kotidis, P., Marbiah, M., Kim, J., Kontoravdi, C., and Polizzi, K. (2022). Polymer encapsulation of bacterial biosensors enables coculture with mammalian cells. ACS Synth. Biol. 11, 1303–1312. doi:10.1021/acssynbio.1c00577
Muñoz-González, I., Jiménez-Girón, A., Martín-Álvarez, P. J., Bartolomé, B., and Moreno-Arribas, M. V. (2013). Profiling of microbial-derived phenolic metabolites in human feces after moderate red wine intake. J. Agric. Food Chem. 61, 9470–9479. doi:10.1021/jf4025135
Nelson, M. T., Charbonneau, M. R., Coia, H. G., Castillo, M. J., Holt, C., Greenwood, E. S., et al. (2021). Characterization of an engineered live bacterial therapeutic for the treatment of phenylketonuria in a human gut-on-a-chip. Nat. Commun. 12, 2805. doi:10.1038/s41467-021-23072-5
Nikolaus, S., Schulte, B., Al-Massad, N., Thieme, F., Schulte, D. M., Bethge, J., et al. (2017). Increased tryptophan metabolism is associated with activity of inflammatory bowel diseases. Gastroenterology 153, 1504–1516.e2. doi:10.1053/j.gastro.2017.08.028e2
Oliphant, K., Parreira, V. R., Cochrane, K., and Allen-Vercoe, E. (2019). Drivers of human gut microbial community assembly: Coadaptation, determinism and stochasticity. ISME J. 13, 3080–3092. doi:10.1038/s41396-019-0498-5
Patterson, E., Ryan, P. M., Cryan, J. F., Dinan, T. G., Ross, R. P., Fitzgerald, G. F., et al. (2016). Gut microbiota, obesity and diabetes. Postgrad. Med. J. 92, 286–300. doi:10.1136/postgradmedj-2015-133285
Perry, J. L. (1997). Assessment of swab transport systems for aerobic and anaerobic organism recovery. J. Clin. Microbiol. 35, 1269–1271. doi:10.1128/jcm.35.5.1269-1271.1997
Ridaura, V. K., Faith, J. J., Rey, F. E., Cheng, J., Duncan, A. E., Kau, A. L., et al. (2013). Gut microbiota from twins discordant for obesity modulate metabolism in mice. Science 341, 1241214. doi:10.1126/science.1241214
Riglar, D. T., and Silver, P. A. (2018). Engineering bacteria for diagnostic and therapeutic applications. Nat. Rev. Microbiol. 16, 214–225. doi:10.1038/nrmicro.2017.172
Saliba, R., Zahar, J. R., El Allaoui, F., Carbonnelle, E., and Lescat, M. (2020). Impact of freeze/thaw cycles and single freezing at -80 °C on the viability of aerobic bacteria from rectal swabs performed with the ESwabTM system. Diagn. Microbiol. Infect. Dis. 96, 114895. doi:10.1016/j.diagmicrobio.2019.114895
Schwenger, K. J., Clermont-Dejean, N., and Allard, J. P. (2019). The role of the gut microbiome in chronic liver disease: The clinical evidence revised. JHEP Rep. 1, 214–226. doi:10.1016/j.jhepr.2019.04.004
Seger, C., and Salzmann, L. (2020). After another decade: LC–MS/MS became routine in clinical diagnostics. Clin. Biochem. 82, 2–11. doi:10.1016/j.clinbiochem.2020.03.004
Sharon, G., Garg, N., Debelius, J., Knight, R., Dorrestein, P. C., and Mazmanian, S. K. (2014). Specialized metabolites from the microbiome in health and disease. Cell Metab. 20, 719–730. doi:10.1016/j.cmet.2014.10.016
Smith, M. I., Yatsunenko, T., Manary, M. J., Trehan, I., Mkakosya, R., Cheng, J., et al. (2013). Gut microbiomes of Malawian twin pairs discordant for kwashiorkor. Science 339, 548–554. doi:10.1126/science.1229000
Steidler, L., Neirynck, S., Huyghebaert, N., Snoeck, V., Vermeire, A., Goddeeris, B., et al. (2003). Biological containment of genetically modified Lactococcus lactis for intestinal delivery of human interleukin 10. Nat. Biotechnol. 21, 785–789. doi:10.1038/nbt840
Taketani, M., Zhang, J., Zhang, S., Triassi, A. J., Huang, Y.-J., Griffith, L. G., et al. (2020). Genetic circuit design automation for the gut resident species Bacteroides thetaiotaomicron. Nat. Biotechnol. 38, 962–969. doi:10.1038/s41587-020-0468-5
Tang, T.-C., Tham, E., Liu, X., Yehl, K., Rovner, A. J., Yuk, H., et al. (2021). Hydrogel-based biocontainment of bacteria for continuous sensing and computation. Nat. Chem. Biol. 17, 724–731. doi:10.1038/s41589-021-00779-6
Wan, X., Volpetti, F., Petrova, E., French, C., Maerkl, S. J., and Wang, B. (2019). Cascaded amplifying circuits enable ultrasensitive cellular sensors for toxic metals. Nat. Chem. Biol. 15, 540–548. doi:10.1038/s41589-019-0244-3
Wang, Z., Klipfell, E., Bennett, B. J., Koeth, R., Levison, B. S., Dugar, B., et al. (2011). Gut flora metabolism of phosphatidylcholine promotes cardiovascular disease. Nature 472, 57–63. doi:10.1038/nature09922
Watstein, D., and Styczynski, M. (2017). Development of a pigment-based whole-cell zinc biosensor for human serum. ACS Synth. Biol. 7, 267–275. doi:10.1021/acssynbio.7b00292
Woting, A., and Blaut, M. (2016). The intestinal microbiota in metabolic disease. Nutrients 8, 202. doi:10.3390/nu8040202
Wu, J., Dong, M., Rigatto, C., Liu, Y., and Lin, F. (2018). Lab-on-chip technology for chronic disease diagnosis. npj Digit. Med. 1, 7. doi:10.1038/s41746-017-0014-0
Wu, J., Dong, M., Santos, S., Rigatto, C., Liu, Y., and Lin, F. (2017). Lab-on-a-Chip platforms for detection of cardiovascular disease and cancer biomarkers. Sensors 17, 2934. doi:10.3390/s17122934
Zúñiga, A., Chang, H.-J., Fristot, E., and Bonnet, J. (2021). An engineered lactate responding promoter system operating in glucose-rich and anaerobic environments. bioRxiv 2021, 425364. doi:10.1101/2021.01.06.425364
Keywords: synthetic biology, diagnostics, whole-cell biosensor, engineered bacteria, metabolite detection, gut microbiome
Citation: Zúñiga A, Muñoz-Guamuro G, Boivineau L, Mayonove P, Conejero I, Pageaux G-P, Altwegg R and Bonnet J (2022) A rapid and standardized workflow for functional assessment of bacterial biosensors in fecal samples. Front. Bioeng. Biotechnol. 10:859600. doi: 10.3389/fbioe.2022.859600
Received: 21 January 2022; Accepted: 05 July 2022;
Published: 22 August 2022.
Edited by:
Yang Tan, Shenzhen Institutes of Advanced Technology (CAS), ChinaReviewed by:
Elliot S. Friedman, University of Pennsylvania, United StatesCopyright © 2022 Zúñiga, Muñoz-Guamuro, Boivineau, Mayonove, Conejero, Pageaux, Altwegg and Bonnet. This is an open-access article distributed under the terms of the Creative Commons Attribution License (CC BY). The use, distribution or reproduction in other forums is permitted, provided the original author(s) and the copyright owner(s) are credited and that the original publication in this journal is cited, in accordance with accepted academic practice. No use, distribution or reproduction is permitted which does not comply with these terms.
*Correspondence: Ana Zúñiga, YW5hLnp1bmlnYUBpbnNlcm0uZnI=; Jerome Bonnet, amVyb21lLmJvbm5ldEBpbnNlcm0uZnI=
Disclaimer: All claims expressed in this article are solely those of the authors and do not necessarily represent those of their affiliated organizations, or those of the publisher, the editors and the reviewers. Any product that may be evaluated in this article or claim that may be made by its manufacturer is not guaranteed or endorsed by the publisher.
Research integrity at Frontiers
Learn more about the work of our research integrity team to safeguard the quality of each article we publish.