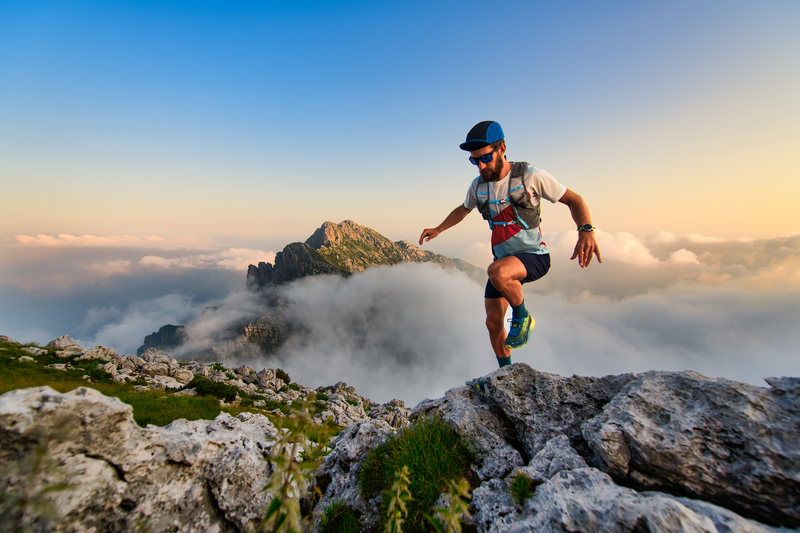
94% of researchers rate our articles as excellent or good
Learn more about the work of our research integrity team to safeguard the quality of each article we publish.
Find out more
REVIEW article
Front. Bioeng. Biotechnol. , 15 March 2022
Sec. Biosensors and Biomolecular Electronics
Volume 10 - 2022 | https://doi.org/10.3389/fbioe.2022.856591
This article is part of the Research Topic Integrated Biosensors Towards Clinical and Point-of-Care Diagnostics View all 8 articles
Gastric cancer is usually diagnosed at late stage and has a high mortality rate, whereas early detection of gastric cancer could bring a better prognosis. Conventional gastric cancer diagnostic methods suffer from long diagnostic times, severe trauma, and a high rate of misdiagnosis and rely heavily on doctors’ subjective experience. Raman spectroscopy is a label-free molecular vibrational spectroscopy technique that identifies the molecular fingerprint of various samples based on the inelastic scattering of monochromatic light. Because of its advantages of non-destructive, rapid, and accurate detection, Raman spectroscopy has been widely studied for benign and malignant tumor differentiation, tumor subtype classification, and section pathology diagnosis. This paper reviews the applications of Raman spectroscopy for the in vivo and in vitro diagnosis of gastric cancer, methodology related to the spectroscopy data analysis, and presents the limitations of the technique.
Gastric cancer is the fifth most commonly diagnosed cancer worldwide and the third most common cause of cancer-related death because it is usually advanced at the time of diagnosis (Bray et al., 2018). The development of gastric cancer is a multistep process, including chronic non-atrophic gastritis, multifocal atrophic gastritis, intestinal metaplasia, dysplasia, and invasive carcinoma (Correa and Piazuelo, 2008). The prognosis of gastric cancer is closely related to the disease course, and after effective treatment, the 5-year survival rate of early gastric cancer reaches more than 90% (Nallala et al., 2012). Early detection is the most important factor to improve gastric cancer survival and prognosis. Thus, efficient and sensitive examination techniques are critical for increasing early detection rates.
Endoscopic diagnosis, pathological diagnosis, and medical images are commonly used in clinical gastric cancer diagnosis, and these diagnostic techniques have the disadvantages of long diagnosis times, serious trauma, and a high rate of misjudgment, and rely heavily on doctors’ subjective experience. As a result, a new gastric cancer diagnosis technique that is fast, non-invasive, highly accurate, and sensitive is desperately needed. Raman spectroscopy, a molecular vibrational spectroscopy technique, can identify the properties and structures of various substances by obtaining fingerprinting information from molecules. Raman spectroscopy is currently used to diagnose a variety of tumors.
Kim H. H. reviewed the application of fiber optic Raman in the in vivo study of gastric cancer, but it was limited to in vivo study (Kim, 2015). In addition to this, Auner G. W. et al. summarized the applications of Raman spectroscopy in cancer diagnosis (Auner et al., 2018), and Baker M. J. et al. reviewed Raman spectroscopy in clinical applications (Baker et al., 2018). This paper focuses on the field of gastric cancer based on a review of 35 articles found using the keyword “gastric cancer Raman” and reviews the research progress of Raman spectroscopy in the early diagnosis of gastric cancer. This paper not only reviews the research related to Raman spectroscopy for the in vivo and vitro diagnosis of gastric cancer and its future prospects in the clinical field, but also focuses on Raman spectroscopy data analysis methods used in these applications.
In 1928, Indian scholar C. V. Raman discovered that when monochromatic light of a fixed frequency is incident on a medium, two scattering processes occur simultaneously: one is Rayleigh scattering with constant frequency, which is caused by elastic collisions between incident photons and matter, and the other is frequency-changing Raman scattering, which is caused by an energy exchange that occurs when incident photons collide with matter, causing a change in the molecules’ vibrational or rotational energy levels (Raman and Krishnan, 1928). In Raman scattering, the scattering in which a portion of the energy of the photon is transferred to the molecule is called Stokes Raman scattering, while the scattering in which the energy of the vibration and rotation of the molecule is transferred to the photon is anti-Stokes Raman scattering (Figure 1). The Raman shift, which is the transverse coordinate of the Raman spectrum that we see, is the difference between the frequency of the scattered light and the frequency of the incident light (Rayleigh scattering) during Raman scattering (Bergholt et al., 2014). The Raman shift is determined by the molecule’s vibrational energy level, which varies depending on the chemical group, regardless of the excitation wavelength. The intensity of the Raman peak, which is related to the excitation light wavelength, power, concentration, and molecular polarization rate of the substance being tested, as well as the instrument system’s collection efficiency, is the vertical coordinate of the Raman spectrum. All molecules, including gas, solid, and liquid molecules, have their own Raman spectra. As a result, Raman spectroscopy can determine the properties and structure of various substances without the need for sample labeling.
FIGURE 1. Principle of Raman scattering. (A) Raman scattering and Rayleigh scattering. (B) Energy level diagram of Raman scattering, Rayleigh scattering and infrared absorption.
The excitation light source, focusing system, filter, dispersion system, detector, and recording system are all components of a Raman spectrometer. Spontaneous Raman spectroscopy is a name given to distinguish other Raman scattering. Raman signals have low intensity and poor interference resistance, necessitating a long integration time in practical applications to obtain a spectrum with an acceptable signal-to-noise ratio, which is inconvenient for spectral acquisition and fast imaging. Furthermore, the Raman signal can be affected by the fluorescence background in biological samples. Various Raman signal enhancement techniques have emerged to address the challenges of slow acquisition speed and low signal-to-noise ratio. Resonance Raman spectroscopy (RRS) (Liu et al., 2020; Zhao and Mu, 2020), surface-enhanced Raman spectroscopy (SERS) (Kneipp et al., 1997; Lee and Meisel, 2002; Qian et al., 2008), tip-enhanced Raman spectroscopy (TERS) (Chen C. et al., 2014) and stimulated Raman spectroscopy (SRS) (Mittal et al., 2013) are the most commonly used Raman signal enhancement techniques. All of these techniques have high biochemical sensitivity and selectivity, and none of these techniques require the sample to be labeled, making them promising in biological and medical fields. Due to the complexity of the environment and the characteristics of the Raman spectroscopy signal, some overlapping peaks are inevitable in the signal acquisition process. It has been demonstrated that overlapped spectral peaks can be identified by combined symmetric zero-area conversion and L-M fitting (Bi et al., 2015). The evolving spectral pre-processing and classification algorithms greatly compensate for the influence of the external environment and spectrometer on the quality of the spectra, with high specificity and accuracy in numerous applications.
In the application of tumor diagnosis, researchers collect Raman spectroscopy data from samples of tumor tissues, cancer cells, body fluids or tumor markers and use data processing methods such as statistics, machine learning, and deep learning to achieve the diagnosis of different tumors (Ralbovsky and Lednev, 2020). Raman spectroscopy is currently being used to diagnose malignant tumors in various parts of the human body, including the bladder (Bovenkamp et al., 2018), breast (Haka et al., 2009), brain (Mizuno et al., 1994), cervix (Kamemoto et al., 2010), colorectum (Lin et al., 2011), esophagus (Feng et al., 2013), gastrointestinal tract (Teh et al., 2008a), liver (Xiong et al., 2012), lung (Ye et al., 2014), and oral cavity (Cals et al., 2015), as well as in the diagnosis of nasopharyngeal carcinoma (Lin K. et al., 2017), laryngeal carcinoma (Lin et al., 2016b), and ovarian cancer (Baker et al., 2018).
Endoscopic diagnosis, histopathology, clinical imaging, tumor markers, and other clinical methods are currently used to detect gastric cancer. The “gold standard” in clinical tumor diagnosis is endoscopic and histopathological diagnosis, which is performed on a tissue and cellular scale. After staining the tissues, by observing the size, density and nucleoplasmic ratio of the cells under the microscope, the structure, number and arrangement of the glands, it is possible to determine the tissue type of normal gastric mucosa, precancerous lesions and gastric cancer. Its detection time is about 3 days. But this necessitates biopsy sampling for tissue diagnosis, which can result in gastrointestinal bleeding and perforation. Furthermore, pathological diagnosis accuracy is influenced to some extent by pathologists’ subjective factors, and test procedures are time consuming and results can be delayed. X-ray contrast radiography examination, computed tomography examination, magnetic resonance imaging (MRI) and other imaging diagnostic methods are commonly used as clinical diagnostic tools, but they can only make preliminary judgments about the shape and nature of pathological tissues. Because they can only make a preliminary assessment of the shape and nature of pathological tissues, and because their spatial resolution is low, these techniques have a low detection rate for microscopic tumors and early gastric cancer. The commonly used clinical markers are CEA, CA19-9, CA125, and CA72-4, etc. The use of tumor markers for gastric cancer diagnosis is susceptible to individual differences and some benign diseases, and their low specificity and sensitivity limit their application in gastric cancer diagnosis. Its detection time takes a few days. Its detection time takes a few days. More importantly, tumor markers are very difficult to detect. This emphasizes the value of Raman spectroscopy in the early detection of gastric cancer.
The composition and content of chemical substances in stomach tissues or cells will change to some extent during the cancerous process and can be directly reflected by the corresponding characteristic peaks and intensity of the Raman spectra. The comprehensive sensitivity and specificity of Raman spectroscopy for the diagnosis of gastric cancer are 0.89 and 0.92, respectively, when histopathology is used as the reference standard. As a result, Raman spectroscopy is an objective and sensitive optical diagnostic technique for detecting gastric cancer that is non-invasive to the body, allows for real-time diagnosis, and is simple to use (Ouyang et al., 2015).
The current samples in Raman-related studies of gastric cancer are primarily derived from tissues excised during surgery, isolated cell samples, body fluids, or endoscopic biopsies, as shown in Figure 2. To analyze tissue or cell samples in vitro, spectral data are collected primarily using spontaneous Raman spectroscopy with laser wavelengths of 532 nm, 785 nm, or 1,064 nm. In addition, some studies have used confocal Raman spectroscopy techniques (Shen et al., 2005; Hu et al., 2008), which has the advantage of having a high spatial resolution that effectively eliminates signal interference from layers beyond the focal plane. SERS was primarily used to study samples such as blood, breath, and saliva, with a maximum enhancement of 1015 times compared to normal Raman spectra of molecules (Hung et al., 2010). Furthermore, fiber optic Raman spectroscopy plays an important role in the real-time detection and in situ analysis of gastric cancer patients using fiber optic probes in vivo.
FIGURE 2. Sample types for Raman spectroscopy in gastric cancer diagnostic studies. ① Blood samples. Diagnostic analysis of serum, serum protein, serum RNA, or plasma by SERS sensor. ② Breath and saliva. SERS coupled with mass spectrometry to detect biomarkers in the sample. ③ Tissue or cell. Detection of isolated tissue samples by spontaneous Raman or confocal Raman spectroscopy. ④ In vivo detection. Raman in vivo measurements using fiber optic Raman in combination with endoscopy.
Raman spectroscopy of ex vivo samples was collected and analyzed to identify and classify chemical differences among different samples.
Raman spectroscopy data acquisition and classification of gastric tissues or cells is the most direct and widespread application of Raman spectroscopy in diagnosing the nature of gastric tumors. Traditional intraoperative histological diagnosis requires workflows, such as tissue transport, sample processing, section preparation and tissue diagnosis, while the Raman spectroscopy-based gastric cancer diagnosis method is able to collect data directly from fresh tissues.
Formalin-fixed paraffin-embedded (FFPE) samples (Ikeda et al., 2018), frozen samples (Hu et al., 2008; Kawabata et al., 2008; Luo et al., 2013; Zhou et al., 2016), and fresh tissue samples (Kalyan Kumar et al., 2007; Teh et al., 2008a; Teh et al., 2008b; Teh et al., 2010a; Bergholt et al., 2011a; Luo et al., 2013; Chen Y. et al., 2014) are the most common samples used in Raman studies of isolated gastric tissues and cells. When the diagnostic results are known, FFPE samples can be studied, but the fixation of the tissue may affect its biochemical composition, resulting in changes in Raman peaks (Ramos et al., 2015). Frozen tissues are closer to the fresh state, and their Raman spectra have a good signal-to-noise ratio and reproducibility (Haka et al., 2009; Kamemoto et al., 2010). However, the freezing and thawing process appears to change the intensity of some Raman peaks, making detection less accurate than fresh tissues (Wills et al., 2009).
A desktop Raman spectroscopy system is typically used for Raman spectroscopy of isolated tissues. NIR light sources, particularly 785 nm lasers located in the high transmittance “optical window” of biological tissues, are frequently used in medical Raman measurement systems due to the relatively low absorption coefficient of NIR light by molecules in biological tissues that primarily absorb light (e.g., melanin, water, and lipids) (Pence and Mahadevan-Jansen, 2016). Visible light with a wavelength of 532 nm has a higher scattering intensity than NIR light (Ikeda et al., 2018), but NIR light with wavelengths of 785 nm (Kalyan Kumar et al., 2007; Teh et al., 2008a; Teh et al., 2008b; Teh et al., 2010a; Teh et al., 2010b; Bergholt et al., 2011a; Luo et al., 2013; Chen Y. et al., 2014; Zhou et al., 2016) and 1,064 nm (Kawabata et al., 2008) is effective in reducing tissue autofluorescence and has been used more often in diagnostic studies of gastric cancer.
Through the transmission system, light is irradiated onto the sample, and the Raman signal generated by the tissue sample is collected and processed by the detector and spectrometer. Raman spectra are typically acquired in the range of 400–3,500 cm−1, and this wavenumber region can be divided into three parts: the Raman fingerprint region (FP, 400–1800 cm−1), the high wavenumber region (HW, 2,800–3,200 cm−1), and the intermediate region, which typically lacks Raman characteristic peaks. Because the acquisition range of the spectra affects the acquisition time and redundancy of subsequent data processing, most studies only acquire spectral data in the FP region or both the FP and HW regions. According to related research, analyzing the FP region alone is superior to analyzing the HW region alone (Zhou et al., 2016), and analyzing the FP and HW regions simultaneously is superior to analyzing the FP and HW regions separately (Lin et al., 2016a). Furthermore, studies have shown that increasing the laser power has little effect on overall prediction accuracy, but increasing the integration time can significantly improve the prediction accuracy (Chen et al., 2019). The choice of different spectral ranges and spectral acquisition conditions can affect the diagnostic results, so the desired experimental conditions should be chosen based on the sample characteristics in the experiment.
As a fast and non-invasive method of detecting gastric tissues, Raman spectroscopy has made significant progress. Raman detection of isolated gastric tissues has been shown in other studies to diagnose gastric dysplasia (Teh et al., 2008b), differentiate gastric adenocarcinoma staging (Teh et al., 2010a), identify malignant gastric mucosal tissues (Kalyan Kumar et al., 2007), differentiate precancerous and cancerous tissues (Luo et al., 2013), and diagnose gastric cancer (Ikeda et al., 2018). In addition, Chen Y. et al. (2014). looked at the differences in the characteristic peak spectra of genomic DNA, nuclei, and tissues of normal and malignant gastric mucosa to see how they changed the spatial structure and biochemical composition of mucosal tissue during carcinogenesis. Teh et al. (2010b) also showed that NIR Raman spectroscopy can be used to diagnose Helicobacter-pylori infection and inflammatory damage in the stomach at the molecular level.
Cells are more refined than tissues on the detection scale. Based on Raman spectroscopy of cultured single cells, Shen et al. (2005) successfully screened suspected gastric mucosal malignant cells in 3 min. The main compositional differences between apoptosis-inducing cells and gastric cancer cells were discovered by Yao et al. (2009), allowing non-destructive Raman spectroscopy to be used to monitor apoptosis in gastric cancer cells and to test new drugs against the therapeutic effects of various cancer cells. The microscopic pictures of gastric cancer cells with Raman spectra are shown in Figure 3.
FIGURE 3. Micrographs of gastric cancer cells with Raman spectra. (A) Images of gastric carcinoma cells observed by differential interference contrast (DIC) microscope with the ×100 objective lens. (B) Raman spectra of untreated gastric carcinoma cells (curve a) and apoptotic cells (curve b). Curve c was the difference spectrum between a and b. The position of Raman bands at 782, 934, 1,001, 1,092, 1,156, 1,298, 1,340, 1,446, 1,523, 1,576, 1,615, and 1,655 cm−1 were marked. Reproduced from permission (Yao et al., 2009). Copyright (2009), with permission from Elsevier.
Blood components, such as plasma, red blood cells, white blood cells, and platelets, are important body fluids used to monitor numerous disease states. Raman spectroscopy has been used to detect blood components and whole blood for more than 40 years (Atkins et al., 2017). Since the individual components of cancerous blood undergo small changes that are difficult to detect by normal methods, the SERS technique, which is more sensitive, is mostly used in research to detect them.
Because molecules show unusually large Raman scattering cross-sections when adsorbed on metal surfaces at a certain nanometer size, the key to SERS is the preparation of enhanced substrates or nanoenhanced materials. Gold and silver nanomaterials are commonly used in SERS studies, and related studies have shown that 3D nanostructured gold nanoparticle/silicon nanowire array (Au/SiNWA) SERS substrates are also a good way to distinguish between gastric and normal serum SERS-active substrates (Wei et al., 2016). The Raman spectra of Au/SiNWA substrate compared with the SERS spectrum of normal gastric cancer are shown in Figure 4. Furthermore, plasma SERS spectra are affected by different laser polarizations (Feng et al., 2011a). According to a number of studies, using SRES to detect plasma and serum samples can not only identify gastric cancer (Feng et al., 2011b; Chen et al., 2012; Guo et al., 2019; Avram et al., 2020), but also differentiate between different stages of gastrointestinal tumors and different digestive system cancers (Lin J. et al., 2017).
FIGURE 4. The contrast of SERS spectrum of gastric cancer and normal with Au/SiNWA substrate. Reproduced from permission (Wei et al., 2016). Copyright (2016), with permission from Elsevier.
Specific odors in the breath were linked to certain diseases by ancient Greek physicians (Kim et al., 2012). Since 1971, studies have shown breath to contain a complex mix of nearly 3,000 volatile organic compounds (VOCs) (Peng et al., 2010; Amal et al., 2012; Hakim et al., 2012). Cancer cells or tissues that are subjected to metabolic or oxidative stress produce specific VOCs. As a result, they can be used as VOC biomarkers for cancer without affecting clinical symptoms (Konvalina and Haick, 2014). Furthermore, saliva can directly reflect a person’s health status and has obvious benefits for use in the diagnosis of gastric cancer. Studies have shown that using gas chromatography–mass spectrometry coupled with solid phase microextraction (SPME) and SERS to identify organic compound biomarkers in breath or saliva can identify early and advanced gastric cancer (Chen et al., 2016; Chen et al., 2018). Based on the SERS sensor, this non-invasive, rapid, and reliable analysis method offers a new strategy for identifying gastric cancer patients in the general population.
Many researchers want to bring Raman spectroscopy technology from the lab to in situ testing in the field, and fiber optic Raman technology allows for in situ testing and biomedical in vivo testing in the field. The fiber optic Raman probe, which is made up of an optical fiber and a small lens, can detect any part of a sample to obtain the Raman spectral information for the sample detection site. The use of fiber optic Raman combined with endoscopy in the diagnosis of gastric cancer allows not only the acquisition of real-time images of the tumor, but also simultaneous Raman spectral data acquisition for non-destructive and flexible detection of the digestive tract (Duraipandian et al., 2012; Wang et al., 2015). Raman spectra and gastric cancer images obtained using an image-guided Raman endoscopy system are shown in Figure 5. Before using fiber-optic Raman to acquire Raman spectra in vivo, it is necessary to first observe the gastric mucosa and the gastric lumen for any adhesions or fluids through the gastroscope. After rinsing and aspirating away the adherent, the location of the target Raman acquisition site can be determined with the aid of endoscopy to exclude interference from other tissues and blood vessels.
FIGURE 5. In vivo mean Raman spectra ±1 standard deviations (SD) of normal (n = 934), and cancer (n = 129) gastric tissue, as well as the corresponding white-light reflectance (WLR) image and narrow-band image (NBI) acquired during clinical gastroscopic examination. Reproduced from permission (Huang et al., 2010b). Copyright (2010), with permission from Elsevier.
Quartz-based optical fibers, which have incomparable advantages in terms of light transmission, in vivo compatibility, and bending flexibility, are currently used for Raman spectroscopy detection of biological tissues. The structural design of the fiber optic probe is determined by the application; for example, for in vivo detection of the stomach, the probe must be smaller and more flexible. Furthermore, a previous study found that changing the tissue pressure and angle of the probe had no significant effect on the Raman spectrum (Shim et al., 2000).
A previous study using a small endoscopic system equipped with a miniature Raman probe demonstrated the safety of Raman detection in rats before it was used in human experiments (Hattori et al., 2007). Numerous studies have shown that the use of endoscopic Raman probes can diagnose gastric dysplasia (Huang et al., 2009; Huang et al., 2010a), benign and malignant ulcerative lesions of the stomach (Bergholt et al., 2010), and gastric cancer (Huang et al., 2010b). Otherwise, the probes can be used in vivo to collect the Raman spectra from different anatomical locations in the upper gastrointestinal tract (Bergholt et al., 2011a). Additionally, the combination of Raman spectroscopy and near-infrared autofluorescence can improve the accuracy of gastric cancer diagnosis (Bergholt et al., 2011b).
Data preprocessing, feature selection, and multivariate modeling are all part of a typical spectral analysis workflow in Raman spectroscopy (Allegrini and Olivieri, 2013). Table 1 lists the analytical methods used in the Raman studies of gastric cancer discussed in the text.
The spectral signal is preprocessed to remove unwanted variations and artifacts. Noise removal, baseline correction, scattering correction, and scaling are all common preprocessing techniques. Furthermore, spectral normalization (e.g., spectral area, maximum value, average intensity normalization, etc.) can help compare spectral shapes and relative peak intensities between different tissues by reducing the effects of fluctuations in the excitation intensity (Chen et al., 2013). Savitzky–Golay (SG) filtering is the most commonly used filtering method in studies applying Raman spectroscopy to gastric cancer (Table 1). The SG filter is a filtering method based on local polynomial least square fitting in the time domain, and its most significant characteristic is that it can ensure that the shape and width of the signal remain unchanged while filtering out noise.
The reason for feature selection is that spectral data contain a significant amount of invalid information, and analyzing the entire spectrum directly may affect the diagnostic effect while also increasing the computational cost. Algorithms are frequently used to perform feature selection and spectral extraction of the raw spectra, in addition to manually truncating the spectrum or selecting special peaks based on a priori knowledge. Principal component analysis (PCA) is the most commonly used feature extraction method in gastric cancer Raman studies (Table 1). The PCA algorithm is a popular unsupervised algorithm for reducing the difficulty of spectral data analysis before it is fed into multivariate models. However, reducing variables may result in the loss of useful information and a change in the spectral data’s original pattern.
In order to obtain the class information of the sample to be measured from the Raman spectral data, a mathematical relationship between the spectrum and the label needs to be established. Thus multivariate modeling is used to realize the identification and diagnosis of different samples. The most straightforward classification method is Raman peak intensity comparison (Shen et al., 2005; Hu et al., 2008; Chen Y. et al., 2014; Wei et al., 2016; Ikeda et al., 2018), but the accuracy is not high. The ant colony optimization algorithm (ACO) method of finding the best Raman features and the analysis method of discriminating peak feature intensities based on the Mahalanobis distance can improve diagnostic accuracy. However, when dealing with large amounts of data, it is common to use a variety of multivariate analysis tools to examine different spectral data. Linear discriminant analysis (LDA) and partial least squares (PLS) are more widely used in the application of Raman spectroscopy in gastric cancer. The idea of the LDA algorithm is to make the data as compact as possible for the same class and as dispersed as possible for different classes after projecting them into a low-dimensional space. PLS algorithm is used to extract the features of the sample data by linearly transforming the combined information of the dependent and independent variables. Other algorithms that have been used include the classification and regression tree (CART), characteristic ratio method (CRM), quadratic discriminant analysis (QDA), multinomial logistic regression (MNLR), and artificial neural network (ANN) (Table 1).
Internal and external validation, as well as sufficient supporting data, are required to improve the diagnostic model’s stability. Internal validation is the repeated training of a model using a portion of the dataset as a test set, primarily using cross-validation (e.g., leave-one-out cross validation). The use of a newly measured dataset to test the constructed model is known as external validation. The results obtained may be overly optimistic in the absence of external validation.
Machine learning and deep learning classification methods have been used to diagnose tumors from Raman spectra. Machine learning analysis methods, such as K-nearest neighbor (KNN) and support vector machine (SVM), as well as deep learning algorithms, such as ANN, convolutional neural network (CNN) (Yan et al., 2020), and self-encoder network, have wide applications in the diagnosis of other cancers (Ralbovsky and Lednev, 2020), in addition to the methods used in existing Raman studies of gastric cancer. SVM is a method to obtain data fitting models by training weight parameters, and ANN is built by simulating the human nervous system. ANN is divided into input layer, hidden layer and output layer, where the input layer imports sample data, the hidden layer describes the sample layer by layer, and the output layer is the result generated by regression analysis. Deep learning is developed based on ANN and has the advantage of handling big data and end-to-end processing. CNN, in particular, is less reliant on preprocessing (Acquarelli et al., 2017). The interpretability and reproducibility of deep learning methods, on the other hand, are important limiting factors in the increased application of spectral analysis (Yang et al., 2019).
Given that current gastric cancer detection methods have many limitations, there is an urgent need to develop new techniques that can rapidly diagnose gastric cancer. Raman spectroscopy is a new method for label-free gastric cancer diagnosis that can identify the differences in chemical composition between different types of gastric cancer samples. By analyzing the molecular composition and structural differences of substances in isolated tissue or body fluid samples, Raman spectroscopy distinguishes cancerous from normal samples at the molecular level, thus enabling the early diagnosis of gastric cancer. The integration of a Raman fiber optic probe and endoscope enables real-time in vivo detection to help diagnose the extent and site of cancer development. Improvements in Raman spectrometer equipment and the new methods emerging from Raman data analysis are also incrementally improving the efficiency and accuracy of Raman spectroscopy in diagnosing gastric cancer.
Although Raman spectroscopy has made great progress in the diagnosis of gastric cancer, there are still many problems. How to establish a database and evaluation system for Raman spectra related to gastric cancer, how to eliminate the spectral differences caused by different spectrometers, and how to obtain higher quality Raman spectra are all challenges that need to be overcome. First, how to obtain higher-quality Raman spectra more quickly during the collection of Raman spectral data is still a problem that needs to be investigated. Due to the signal background and the stability of the laser, there are differences in the spectra measured by different Raman spectrometers. In addition, the acquisition conditions, such as the laser wavelength, laser power, and integration time, as well as different sample processing methods, will affect the signal during Raman signal collection. Therefore, it is an important research direction to explore the data acquisition methods, establish a standard sampling process, and achieve detection repeatability and stability.
In terms of the analysis methods for Raman data, the existing data analysis methods are somewhat inadequate in finding the variability of Raman peaks, and more accurate and stable data processing methods should be found to cope with large or massive amounts of spectral data. Computer science and technology, such as deep learning, has great advantages in processing Raman spectral data and Raman imaging data. These technologies may even replace pathologists in the diagnosis of gastric cancer in the future.
Finally, although Raman spectroscopy is highly accurate in identifying the differences between gastric cancer and normal tissues, this accuracy is not sufficient to detect the benignity and malignancy of tumors for the accurate diagnosis of patients’ tumors, so it is necessary to explore more deeply how to use Raman spectroscopy for the diagnosis of subtypes and stages of gastric cancer. The combination of fiber optics and endoscopy is an important direction for in situ real-time tumor diagnosis and is a novel and rapid method for tumor diagnosis. Although many existing studies have started to use Raman endoscopy for in vivo detection, more in vitro testing is needed for clinical application.
In conclusion, the Raman spectroscopy-based method has broad application prospects in gastric cancer diagnosis, and its application as a mature detection method in clinical diagnosis is imminent.
KL, XZ, and BL mainly conceived and listed the outline of the review. KL mainly wrote the review. XZ and QZ contributed to review modification and editing. All authors contributed to the article and approved the submitted version.
This study was supported, in part, by National Natural Science Foundation of China (31801037, 82172861); Guangdong Basic and Applied Basic Research Foundation (2021A1515011743), China Postdoctoral Sciense Foundation (2019M663306), The Science Foundation of Army Medical University (410310543403, 2019JCZX05).
The authors declare that the research was conducted in the absence of any commercial or financial relationships that could be construed as a potential conflict of interest.
All claims expressed in this article are solely those of the authors and do not necessarily represent those of their affiliated organizations, or those of the publisher, the editors, and the reviewers. Any product that may be evaluated in this article, or claim that may be made by its manufacturer, is not guaranteed or endorsed by the publisher.
Acquarelli, J., van Laarhoven, T., Gerretzen, J., Tran, T. N., Buydens, L. M. C., and Marchiori, E. (2017). Convolutional Neural Networks for Vibrational Spectroscopic Data Analysis. Analytica Chim. Acta 954, 22–31. doi:10.1016/j.aca.2016.12.010
Allegrini, F., and Olivieri, A. C. (2013). An Integrated Approach to the Simultaneous Selection of Variables, Mathematical Pre-processing and Calibration Samples in Partial Least-Squares Multivariate Calibration. Talanta 115 (17), 755–760. doi:10.1016/j.talanta.2013.06.051
Amal, L., Ding, L., Liu, B. B., Tisch, U., Xu, Z. Q., Shi, D. Y., et al. (2012). The Scent Fingerprint of Hepatocarcinoma: In-Vitro Metastasis Prediction with Volatile Organic Compounds (VOCs). Int. J. Nanomedicine 7, 4135–4146. doi:10.2147/IJN.S32680
Atkins, C. G., Buckley, K., Blades, M. W., and Turner, R. F. B. (2017). Raman Spectroscopy of Blood and Blood Components. Appl. Spectrosc. 71 (5), 767–793. doi:10.1177/0003702816686593
Auner, G. W., Koya, S. K., Huang, C., Broadbent, B., Trexler, M., Auner, Z., et al. (2018). Applications of Raman Spectroscopy in Cancer Diagnosis. Cancer Metastasis Rev. 37 (4), 691–717. doi:10.1007/s10555-018-9770-9
Avram, L., Iancu, S. D., Stefancu, A., Moisoiu, V., Colnita, A., Marconi, D., et al. (2020). SERS-Based Liquid Biopsy of Gastrointestinal Tumors Using a Portable Raman Device Operating in a Clinical Environment. J. Clin. Med. 9 (1), 212. doi:10.3390/jcm9010212
Baker, M. J., Byrne, H. J., Chalmers, J., Gardner, P., Goodacre, R., Henderson, A., et al. (2018). Clinical Applications of Infrared and Raman Spectroscopy: State of Play and Future Challenges. Analyst 143 (8), 1735–1757. doi:10.1039/c7an01871a
Bergholt, M. S., Zheng, W., Ho, K. Y., Teh, M., Yeoh, K. G., Yan So, J. B., et al. (2014). Fiberoptic Confocal Raman Spectroscopy for Real-Time In Vivo Diagnosis of Dysplasia in Barrett's Esophagus. Gastroenterology 146 (1), 27–32. doi:10.1053/j.gastro.2013.11.002
Bergholt, M. S., Zheng, W., Lin, K., Ho, K. Y., Teh, M., Yeoh, K. G., et al. (2011a). Characterizing Variability in In Vivo Raman Spectra of Different Anatomical Locations in the Upper Gastrointestinal Tract toward Cancer Detection. J. Biomed. Opt. 16 (3), 037003. doi:10.1117/1.3556723
Bergholt, M. S., Zheng, W., Lin, K., Ho, K. Y., Teh, M., Yeoh, K. G., et al. (2011b). Combining Near-Infrared-Excited Autofluorescence and Raman Spectroscopy Improves In Vivo Diagnosis of Gastric Cancer. Biosens. Bioelectron. 26 (10), 4104–4110. doi:10.1016/j.bios.2011.04.005
Bergholt, M. S., Zheng, W., Lin, K., Ho, K. Y., Teh, M., Yeoh, K. G., et al. (2011c). In Vivo diagnosis of Gastric Cancer Using Raman Endoscopy and Ant colony Optimization Techniques. Int. J. Cancer 128 (11), 2673–2680. doi:10.1002/ijc.25618
Bergholt, M. S., Zheng, W., Lin, K., Ho, K. Y., Teh, M., Yeoh, K. G., et al. (2010). Raman Endoscopy for In Vivo Differentiation between Benign and Malignant Ulcers in the Stomach. Analyst 135 (12), 3162–3168. doi:10.1039/c0an00336k
Bi, Y. F., Li, Y., Du, Z. F., Chen, L., and Zheng, R. E. (2015). Automatic Recognition of Overlapped Spectral Peaks by Combined Symmetric Zero-Area Conversion and L-M Fitting. Guang Pu Xue Yu Guang Pu Fen Xi 35 (8), 2339–2342. doi:10.3964/j.issn.1000-0593(2015)08-2339-04
Bovenkamp, D., Sentosa, R., Rank, E., Erkkilä, M., Placzek, F., Püls, J., et al. (2018). Combination of High-Resolution Optical Coherence Tomography and Raman Spectroscopy for Improved Staging and Grading in Bladder Cancer. Appl. Sci. 8 (12), 2371. doi:10.3390/app8122371
Bray, F., Ferlay, J., Soerjomataram, I., Siegel, R. L., Torre, L. A., and Jemal, A. (2018). Global Cancer Statistics 2018: GLOBOCAN Estimates of Incidence and Mortality Worldwide for 36 Cancers in 185 Countries. CA: A Cancer J. Clinicians 68 (6), 394–424. doi:10.3322/caac.21492
Cals, F. L., Bakker Schut, T. C., Hardillo, J. A., Baatenburg de Jong, R. J., Koljenović, S., and Puppels, G. J. (2015). Investigation of the Potential of Raman Spectroscopy for Oral Cancer Detection in Surgical Margins. Lab. Invest. 95 (10), 1186–1196. doi:10.1038/labinvest.2015.85
Chen, B., Li, S., Li, J., Guo, Z., Chen, Q., and Mai, H. (2013). Optimal Multivariate Method for Raman Spectroscopy Based Diagnosis of Nasopharyngeal Carcinoma. J. Appl. Phys. 114 (24), 244702. doi:10.1063/1.4847915
Chen, C., Hayazawa, N., and Kawata, S. (2014). A 1.7 Nm Resolution Chemical Analysis of Carbon Nanotubes by Tip-Enhanced Raman Imaging in the Ambient. Nat. Commun. 5, 3312. doi:10.1038/ncomms4312
Chen, H., Li, X., Broderick, N. G. R., and Xu, W. (2019). Low‐resolution Fiber‐optic Raman Spectroscopy for Bladder Cancer Diagnosis: A Comparison Study of Varying Laser Power, Integration Time, and Classification Methods. J. Raman Spectrosc. 51 (2), 323–334. doi:10.1002/jrs.5783
Chen, Y., Chen, G., Zheng, X., He, C., Feng, S., Chen, Y., et al. (2012). Discrimination of Gastric Cancer from normal by Serum RNA Based on Surface-Enhanced Raman Spectroscopy (SERS) and Multivariate Analysis. Med. Phys. 39 (9), 5664–5668. doi:10.1118/1.4747269
Chen, Y., Cheng, S., Zhang, A., Song, J., Chang, J., Wang, K., et al. (2018). Salivary Analysis Based on Surface Enhanced Raman Scattering Sensors Distinguishes Early and Advanced Gastric Cancer Patients from Healthy Persons. J. Biomed. Nanotechnol 14 (10), 1773–1784. doi:10.1166/jbn.2018.2621
Chen, Y., Dai, J., Zhou, X., Liu, Y., Zhang, W., and Peng, G. (2014). Raman Spectroscopy Analysis of the Biochemical Characteristics of Molecules Associated with the Malignant Transformation of Gastric Mucosa. PLoS One 9 (4), e93906. doi:10.1371/journal.pone.0093906
Chen, Y., Zhang, Y., Pan, F., Liu, J., Wang, K., Zhang, C., et al. (2016). Breath Analysis Based on Surface-Enhanced Raman Scattering Sensors Distinguishes Early and Advanced Gastric Cancer Patients from Healthy Persons. ACS Nano 10 (9), 8169–8179. doi:10.1021/acsnano.6b01441
Correa, P., and Piazuelo, M. B. (2008). Natural History of Helicobacter pylori Infection. Dig. Liver Dis. 40 (7), 490–496. doi:10.1016/j.dld.2008.02.035
Duraipandian, S., Sylvest Bergholt, M., Zheng, W., Yu Ho, K., Teh, M., Guan Yeoh, K., et al. (2012). Real-time Raman Spectroscopy for In Vivo, Online Gastric Cancer Diagnosis during Clinical Endoscopic Examination. J. Biomed. Opt. 17 (8), 081418. doi:10.1117/1.JBO.17.8.081418
Feng, S., Chen, R., Lin, J., Pan, J., Wu, Y., Li, Y., et al. (2011a). Gastric Cancer Detection Based on Blood Plasma Surface-Enhanced Raman Spectroscopy Excited by Polarized Laser Light. Biosens. Bioelectron. 26 (7), 3167–3174. doi:10.1016/j.bios.2010.12.020
Feng, S., Lin, J., Huang, Z., Chen, G., Chen, W., Wang, Y., et al. (2013). Esophageal Cancer Detection Based on Tissue Surface-Enhanced Raman Spectroscopy and Multivariate Analysis. Appl. Phys. Lett. 102 (4), 043702. doi:10.1063/1.4789996
Feng, S., Pan, J., Wu, Y., Lin, D., Chen, Y., Xi, G., et al. (2011b). Study on Gastric Cancer Blood Plasma Based on Surface-Enhanced Raman Spectroscopy Combined with Multivariate Analysis. Sci. China Life Sci. 54 (9), 828–834. doi:10.1007/s11427-011-4212-8
Guo, L., Li, Y., Huang, F., Dong, J., Li, F., Yang, X., et al. (2019). Identification and Analysis of Serum Samples by Surface-Enhanced Raman Spectroscopy Combined with Characteristic Ratio Method and PCA for Gastric Cancer Detection. J. Innov. Opt. Health Sci. 12 (02), 1950003. doi:10.1142/s1793545819500032
Haka, A. S., Volynskaya, Z., Gardecki, J. A., Nazemi, J., Shenk, R., Wang, N., et al. (2009). Diagnosing Breast Cancer Using Raman Spectroscopy: Prospective Analysis. J. Biomed. Opt. 14 (5), 054023. doi:10.1117/1.3247154
Hakim, M., Broza, Y. Y., Barash, O., Peled, N., Phillips, M., Amann, A., et al. (2012). Volatile Organic Compounds of Lung Cancer and Possible Biochemical Pathways. Chem. Rev. 112 (11), 5949–5966. doi:10.1021/cr300174a
Hattori, Y., Komachi, Y., Asakura, T., Shimosegawa, T., Kanai, G.-I., Tashiro, H., et al. (2007). In Vivo Raman Study of the Living Rat Esophagus and Stomach Using a Micro-raman Probe under an Endoscope. Appl. Spectrosc. 61 (6), 579–584. doi:10.1366/000370207781269747
Hu, Y., Shen, A., Jiang, T., Ai, Y., and Hu, J. (2008). Classification of normal and Malignant Human Gastric Mucosa Tissue with Confocal Raman Microspectroscopy and Wavelet Analysis. Spectrochimica Acta A: Mol. Biomol. Spectrosc. 69 (2), 378–382. doi:10.1016/j.saa.2007.04.009
Huang, Z., Bergholt, M. S., Zheng, W., Lin, K., Ho, K. Y., Teh, M., et al. (2010a). In Vivo early Diagnosis of Gastric Dysplasia Using Narrow-Band Image-Guided Raman Endoscopy. J. Biomed. Opt. 15 (3), 037017. doi:10.1117/1.3420115
Huang, Z., Teh, S. K., Zheng, W., Lin, K., Ho, K. Y., Teh, M., et al. (2010b). In Vivo detection of Epithelial Neoplasia in the Stomach Using Image-Guided Raman Endoscopy. Biosens. Bioelectron. 26 (2), 383–389. doi:10.1016/j.bios.2010.07.125
Huang, Z., Teh, S. K., Zheng, W., Mo, J., Lin, K., Shao, X., et al. (2009). Integrated Raman Spectroscopy and Trimodal Wide-Field Imaging Techniques for Real-Time In Vivo Tissue Raman Measurements at Endoscopy. Opt. Lett. 34 (6), 758–760. doi:10.1364/ol.34.000758
Hung, J., Liang, W., Luo, J., Shi, Z., Jen, A. K.-Y., and Li, X. (2010). Rational Design Using Dewar's Rules for Enhancing the First Hyperpolarizability of Nonlinear Optical Chromophores. J. Phys. Chem. C. 114 (50), 22284–22288. doi:10.1021/jp107803q
Ikeda, H., Ito, H., Hikita, M., Yamaguchi, N., Uragami, N., Yokoyama, N., et al. (2018). Raman Spectroscopy for the Diagnosis of Unlabeled and Unstained Histopathological Tissue Specimens. World J. Gastrointest. Oncol. 10 (11), 439–448. doi:10.4251/wjgo.v10.i11.439
Kalyan Kumar, K., Anand, A., Chowdary, M. V. P., KeerthiKurien, J., Kurien, J., Murali Krishna, C., et al. (2007). Discrimination of normal and Malignant Stomach Mucosal Tissues by Raman Spectroscopy: A Pilot Study. Vibrational Spectrosc. 44 (2), 382–387. doi:10.1016/j.vibspec.2007.03.007
Kamemoto, L. E., Misra, A. K., Sharma, S. K., Goodman, M. T., Luk, H., Dykes, A. C., et al. (2010). Near-infrared Micro-raman Spectroscopy for In Vitro Detection of Cervical Cancer. Appl. Spectrosc. 64 (3), 255–261. doi:10.1366/000370210790918364
Kawabata, T., Mizuno, T., Okazaki, S., Hiramatsu, M., Setoguchi, T., Kikuchi, H., et al. (2008). Optical Diagnosis of Gastric Cancer Using Near-Infrared Multichannel Raman Spectroscopy with a 1064-nm Excitation Wavelength. J. Gastroenterol. 43 (4), 283–290. doi:10.1007/s00535-008-2160-2
Kim, H. H. (2015). Endoscopic Raman Spectroscopy for Molecular Fingerprinting of Gastric Cancer: Principle to Implementation. Biomed. Res. Int. 2015, 670121. doi:10.1155/2015/670121
Kim, K.-H., Jahan, S. A., and Kabir, E. (2012). A Review of Breath Analysis for Diagnosis of Human Health. Trac Trends Anal. Chem. 33, 1–8. doi:10.1016/j.trac.2011.09.013
Kneipp, K., Wang, Y., Kneipp, H., Perelman, L. T., Itzkan, I., Dasari, R. R., et al. (1997). Single Molecule Detection Using Surface-Enhanced Raman Scattering (SERS). Phys. Rev. Lett. 78 (9), 1667–1670. doi:10.1103/PhysRevLett.78.1667
Konvalina, G., and Haick, H. (2014). Sensors for Breath Testing: from Nanomaterials to Comprehensive Disease Detection. Acc. Chem. Res. 47 (1), 66–76. doi:10.1021/ar400070m
Lee, P. C., and Meisel, D. (2002). Adsorption and Surface-Enhanced Raman of Dyes on Silver and Gold Sols. J. Phys. Chem. 86 (17), 3391–3395. doi:10.1021/j100214a025
Lin, D., Feng, S., Pan, J., Chen, Y., Lin, J., Chen, G., et al. (2011). Colorectal Cancer Detection by Gold Nanoparticle Based Surface-Enhanced Raman Spectroscopy of Blood Serum and Statistical Analysis. Opt. Express 19 (14), 13565–13577. doi:10.1364/OE.19.013565
Lin, J., Wang, J., Xu, C., Zeng, Y., Chen, Y., Li, L., et al. (2017). Differentiation of Digestive System Cancers by Using Serum Protein-Based Surface-Enhanced Raman Spectroscopy. J. Raman Spectrosc. 48 (1), 16–21. doi:10.1002/jrs.4982
Lin, K., Wang, J., Zheng, W., Ho, K. Y., Teh, M., Yeoh, K. G., et al. (2016a). Rapid Fiber-Optic Raman Spectroscopy for Real-Time In Vivo Detection of Gastric Intestinal Metaplasia during Clinical Gastroscopy. Cancer Prev. Res. 9 (6), 476–483. doi:10.1158/1940-6207.CAPR-15-0213
Lin, K., Zheng, W., Lim, C. M., and Huang, Z. (2016b). Real-time In Vivo Diagnosis of Laryngeal Carcinoma with Rapid Fiber-Optic Raman Spectroscopy. Biomed. Opt. Express 7 (9), 3705–3715. doi:10.1364/BOE.7.003705
Lin, K., Zheng, W., Lim, C. M., and Huang, Z. (2017). Real-time In Vivo Diagnosis of Nasopharyngeal Carcinoma Using Rapid Fiber-Optic Raman Spectroscopy. Theranostics 7 (14), 3517–3526. doi:10.7150/thno.16359
Liu, S., Ma, H., Zhu, J., Han, X. X., and Zhao, B. (2020). Ferrous Cytochrome C-Nitric Oxide Oxidation for Quantification of Protein S-Nitrosylation Probed by Resonance Raman Spectroscopy. Sensors Actuators B: Chem. 308, 127706. doi:10.1016/j.snb.2020.127706
Luo, S., Chen, C., Mao, H., and Jin, S. (2013). Discrimination of Premalignant Lesions and Cancer Tissues from normal Gastric Tissues Using Raman Spectroscopy. J. Biomed. Opt. 18 (6), 067004. doi:10.1117/1.JBO.18.6.067004
Mittal, R., Balu, M., Krasieva, T., Potma, E. O., Elkeeb, L., Zachary, C. B., et al. (2013). Evaluation of Stimulated Raman Scattering Microscopy for Identifying Squamous Cell Carcinoma in Human Skin. Lasers Surg. Med. 45 (8), 496–502. doi:10.1002/lsm.22168
Mizuno, A., Kitajima, H., Kawauchi, K., Muraishi, S., and Ozaki, Y. (1994). Near-infrared Fourier Transform Raman Spectroscopic Study of Human Brain Tissues and Tumours. J. Raman Spectrosc. 25 (1), 25–29. doi:10.1002/jrs.1250250105
Nallala, J., Gobinet, C., Diebold, M.-D., Untereiner, V., Bouché, O., Manfait, M., et al. (2012). Infrared Spectral Imaging as a Novel Approach for Histopathological Recognition in colon Cancer Diagnosis. J. Biomed. Opt. 17 (11), 116013. doi:10.1117/1.JBO.17.11.116013
Ouyang, H., Xu, J., Zhu, Z., Long, T., and Yu, C. (2015). Rapid Discrimination of Malignant Lesions from normal Gastric Tissues Utilizing Raman Spectroscopy System: a Meta-Analysis. J. Cancer Res. Clin. Oncol. 141 (10), 1835–1844. doi:10.1007/s00432-015-1971-9
Pence, I., and Mahadevan-Jansen, A. (2016). Clinical Instrumentation and Applications of Raman Spectroscopy. Chem. Soc. Rev. 45 (7), 1958–1979. doi:10.1039/c5cs00581g
Peng, G., Hakim, M., Broza, Y. Y., Billan, S., Abdah-Bortnyak, R., Kuten, A., et al. (2010). Detection of Lung, Breast, Colorectal, and Prostate Cancers from Exhaled Breath Using a Single Array of Nanosensors. Br. J. Cancer 103 (4), 542–551. doi:10.1038/sj.bjc.6605810
Qian, X., Peng, X.-H., Ansari, D. O., Yin-Goen, Q., Chen, G. Z., Shin, D. M., et al. (2008). In Vivo tumor Targeting and Spectroscopic Detection with Surface-Enhanced Raman Nanoparticle Tags. Nat. Biotechnol. 26 (1), 83–90. doi:10.1038/nbt1377
Ralbovsky, N. M., and Lednev, I. K. (2020). Towards Development of a Novel Universal Medical Diagnostic Method: Raman Spectroscopy and Machine Learning. Chem. Soc. Rev. 49 (20), 7428–7453. doi:10.1039/d0cs01019g
Raman, C. V., and Krishnan, K. S. (1928). A New Type of Secondary Radiation. Nature 121 (3048), 501–502. doi:10.1038/121501c0
Ramos, I. R. M., Malkin, A., and Lyng, F. M. (2015). Current Advances in the Application of Raman Spectroscopy for Molecular Diagnosis of Cervical Cancer. Biomed. Res. Int. 2015, 561242. doi:10.1155/2015/561242
Seng, K. T., Zheng, W., Ho, K. Y., Ming, T., Yeoh, K. G., and Huang, Z. (2008). Diagnosis of Gastric Cancer Using Near-Infrared Raman Spectroscopy and Classification and Regression Tree Techniques. J. Biomed. Opt. 13 (3), 034013. doi:10.1117/1.2939406
Shen, A. G., Ye, Y., Zhang, J. W., Wang, X. H., Hu, J. M., Xie, W., et al. (2005). Screening of Gastric Carcinoma Cells in the Human Malignant Gastric Mucosa by Confocal Raman Microspectroscopy. Vibrational Spectrosc. 37 (2), 225–231. doi:10.1016/j.vibspec.2004.10.001
Shim, M. G., Wong Kee Song, L.-M., Marcon, N. E., and Wilson, B. C. (2000). In Vivo Near-infrared Raman Spectroscopy: Demonstration of Feasibility during Clinical Gastrointestinal Endoscopy¶. Photochem. Photobiol. 72 (1), 146–150. doi:10.1562/0031-8655(2000)072<0146:ivnirs>2.0.co;2
Teh, S. K., Zheng, W., Ho, K. Y., Teh, M., Yeoh, K. G., and Huang, Z. (2008a). Diagnosis of Gastric Cancer Using Near-Infrared Raman Spectroscopy and Classification and Regression Tree Techniques. J. Biomed. Opt. 13 (3), 034013. doi:10.1117/1.2939406
Teh, S. K., Zheng, W., Ho, K. Y., Teh, M., Yeoh, K. G., and Huang, Z. (2008b). Diagnostic Potential of Near-Infrared Raman Spectroscopy in the Stomach: Differentiating Dysplasia from normal Tissue. Br. J. Cancer 98 (2), 457–465. doi:10.1038/sj.bjc.6604176
Teh, S. K., Zheng, W., Ho, K. Y., Teh, M., Yeoh, K. G., and Huang, Z. (2010a). Near-infrared Raman Spectroscopy for Early Diagnosis and Typing of Adenocarcinoma in the Stomach. Br. J. Surg. 97 (4), 550–557. doi:10.1002/bjs.6913
Teh, S. K., Zheng, W., Ho, K. Y., Teh, M., Yeoh, K. G., and Huang, Z. (2010b). Near-infrared Raman Spectroscopy for Optical Diagnosis in the Stomach: Identification of Helicobacter-Pylori Infection and Intestinal Metaplasia. Int. J. Cancer 126 (8), 1920–1927. doi:10.1002/ijc.24935
Wang, J., Lin, K., Zheng, W., Ho, K. Y., Teh, M., Yeoh, K. G., et al. (2015). Comparative Study of the Endoscope-Based Bevelled and Volume Fiber-Optic Raman Probes for Optical Diagnosis of Gastric Dysplasia In Vivo at Endoscopy. Anal. Bioanal. Chem. 407 (27), 8303–8310. doi:10.1007/s00216-015-8727-x
Wei, Y., Zhu, Y.-y., and Wang, M.-l. (2016). Surface-enhanced Raman Spectroscopy of Gastric Cancer Serum with Gold Nanoparticles/silicon Nanowire Arrays. Optik 127 (19), 7902–7907. doi:10.1016/j.ijleo.2016.05.146
Wills, H., Kast, R., Stewart, C., Rabah, R., Pandya, A., Poulik, J., et al. (2009). Raman Spectroscopy Detects and Distinguishes Neuroblastoma and Related Tissues in Fresh and (Banked) Frozen Specimens. J. Pediatr. Surg. 44 (2), 386–391. doi:10.1016/j.jpedsurg.2008.10.095
Xiong, Y., Li, Y. T., Guo, Y., Rao, F. F., Zhang, J. M., Si, M. Z., et al. (2012). Analysis of Surface Enhanced Raman Scattering Spectra of Oxyhemoglobin for Liver Cancer with Combined Multivariate Statistics. Guang Pu Xue Yu Guang Pu Fen Xi 32 (9), 2427–2432. doi:10.3964/j.issn.1000-0593(2012)09-2427-06
Yan, H., Yu, M., Xia, J., Zhu, L., Zhang, T., Zhu, Z., et al. (2020). Diverse Region-Based CNN for Tongue Squamous Cell Carcinoma Classification with Raman Spectroscopy. IEEE Access 8, 127313–127328. doi:10.1109/access.2020.3006567
Yang, J., Xu, J., Zhang, X., Wu, C., Lin, T., and Ying, Y. (2019). Deep Learning for Vibrational Spectral Analysis: Recent Progress and a Practical Guide. Analytica Chim. Acta 1081, 6–17. doi:10.1016/j.aca.2019.06.012
Yao, H., Tao, Z., Ai, M., Peng, L., Wang, G., He, B., et al. (2009). Raman Spectroscopic Analysis of Apoptosis of Single Human Gastric Cancer Cells. Vibrational Spectrosc. 50 (2), 193–197. doi:10.1016/j.vibspec.2008.11.003
Ye, L.-P., Hu, J., Liang, L., and Zhang, C.-y. (2014). Surface-enhanced Raman Spectroscopy for Simultaneous Sensitive Detection of Multiple microRNAs in Lung Cancer Cells. Chem. Commun. 50 (80), 11883–11886. doi:10.1039/c4cc05598e
Zhao, L., and Mu, X. (2020). Visualization of Vibrational-Resolution Charge Transfer Enhanced Resonance Raman Scattering Spectroscopy. Spectrochimica Acta Part A: Mol. Biomol. Spectrosc. 229, 117945. doi:10.1016/j.saa.2019.117945
Keywords: Raman spectroscopy, gastric cancer, clinical diagnostics, on-site applications, machine learning
Citation: Liu K, Zhao Q, Li B and Zhao X (2022) Raman Spectroscopy: A Novel Technology for Gastric Cancer Diagnosis. Front. Bioeng. Biotechnol. 10:856591. doi: 10.3389/fbioe.2022.856591
Received: 17 January 2022; Accepted: 23 February 2022;
Published: 15 March 2022.
Edited by:
Can Dincer, University of Freiburg, GermanyReviewed by:
Manas Ranjan Gartia, Louisiana State University, United StatesCopyright © 2022 Liu, Zhao, Li and Zhao. This is an open-access article distributed under the terms of the Creative Commons Attribution License (CC BY). The use, distribution or reproduction in other forums is permitted, provided the original author(s) and the copyright owner(s) are credited and that the original publication in this journal is cited, in accordance with accepted academic practice. No use, distribution or reproduction is permitted which does not comply with these terms.
*Correspondence: Bei Li, YmVpbGlAY2lvbXAuYWMuY24=; Xia Zhao, emhhb3hpYTFAc3lzdWNjLm9yZy5jbg==
Disclaimer: All claims expressed in this article are solely those of the authors and do not necessarily represent those of their affiliated organizations, or those of the publisher, the editors and the reviewers. Any product that may be evaluated in this article or claim that may be made by its manufacturer is not guaranteed or endorsed by the publisher.
Research integrity at Frontiers
Learn more about the work of our research integrity team to safeguard the quality of each article we publish.