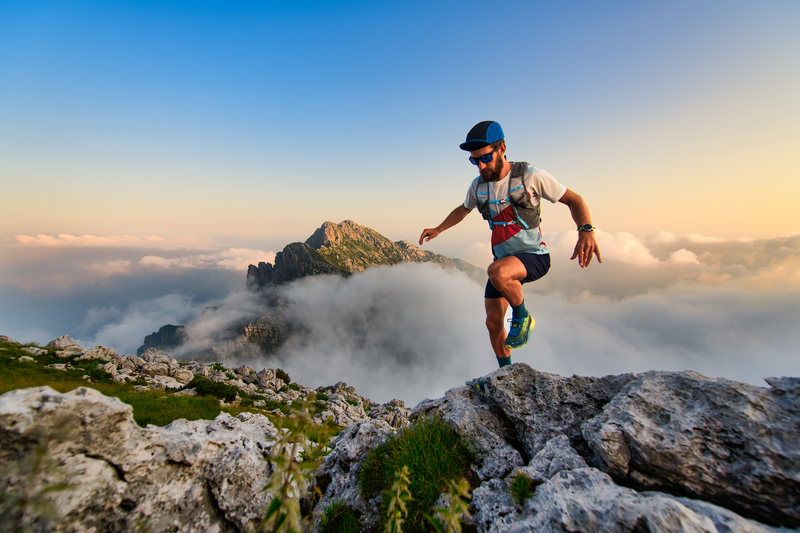
95% of researchers rate our articles as excellent or good
Learn more about the work of our research integrity team to safeguard the quality of each article we publish.
Find out more
PERSPECTIVE article
Front. Bioeng. Biotechnol. , 20 May 2022
Sec. Tissue Engineering and Regenerative Medicine
Volume 10 - 2022 | https://doi.org/10.3389/fbioe.2022.855042
This article is part of the Research Topic Biofabrication and Organs-on-Chips: Becoming More Automated and Realistic View all 7 articles
Biofabrication, specifically 3D-Bioprinting, has the potential to disruptively impact a wide range of future technological developments to improve human well-being. Organs-on-Chips could enable animal-free and individualized drug development, printed organs may help to overcome non-treatable diseases as well as deficiencies in donor organs and cultured meat may solve a worldwide environmental threat in factory farming. A high degree of manual labor in the laboratory in combination with little trained personnel leads to high costs and is along with strict regulations currently often a hindrance to the commercialization of technologies that have already been well researched. This paper therefore illustrates current developments in process automation in 3D-Bioprinting and provides a perspective on how the use of proven and new automation solutions can help to overcome regulatory and technological hurdles to achieve an economically scalable production.
Tissue engineering has played an important role in the field of regenerative medicine and biomedical engineering for many years. Through the targeted interaction of cells, suitable carrier materials, and growth stimuli, it is possible to produce a variety of tissue substitutes (Nerem and Sambanis, 2007) as well as in vitro models for preclinical studies (Godbey and Atala, 2002; Hirt et al., 2014). Anthony Atala, for example, succeeded in producing a human bladder and implanting it in a patient as early as 1999 (Atala et al., 2006). However, traditional tissue engineering methods often depend on the use of molds and cores and are thus restricted in their geometric freedom. Moreover, these methods are labor intensive and difficult to reproduce, which is not practical in the context of an economically scalable production, neither it is regarding standardization in terms of governmental regulation guidelines.
A promising technology to enable reproducibility and scalability while maintaining high quality and standardization is 3D-Bioprinting. In conventional additive manufacturing processes, 3D-Printing is used for rapid prototyping on the one hand and to move from mass production to mass customization on the other (Bak, 2003; Berman, 2012). In contrast, the use of 3D-Bioprinting in combination with further automation steps is intended to enable scalable and standardized production of printed tissue or microfluidic models to replace labor intensive handcraft. It also provides the capability to use digital models that can be easily adapted to individual needs for functional human tissue substitutes or replicas such as liver, skin or bones. 3D-Bioprinting covers a wide range of different processes and technologies that differ fundamentally in terms of the method of transferring bioink from the cartridge to a substrate or a previously printed layer. Besides procedural differences 3D-Bioprinting methods offer some common significant advantages. They allow the use of sensor-based control and regulation of the printing process and thus enable online quality monitoring, which offers great added value in terms of achieving reproducible printing results with a high shape-fidelity, printing resolution and cell viability. Sensor integration and online quality assessment (QA) has the potential to pave the way for standardized manufacturing platforms. In conjunction with robotic process automation, this could enable the industrially scalable production of clean meat or Organs-on-Chips (OoCs) through 3D-Bioprinting. In addition, real-time recording of sensor data and corresponding online quality control can provide a foundation for the certification of Advanced Therapy Medicinal Products (ATMPs), which is critical for the approval of clinical applications.
Bioprinting technology can be looked at from two different perspectives, a rather technical one or an economical one. The former is subject of current research and technical advantages are widely observed. The economic consideration, however, is often disregarded. Therefore, online QA and further automation, suitability for scalable production of printed tissue and standardization are often ignored. This paper places current automation solutions in the context of scalable biofabrication, considers transferable processes from other industries, and provides a perspective on the fully automated use of 3D-Bioprinting processes to move from handcraft to standardized production.
Increased automation of bioprinting processes, for example, enables online quality control and live adjustments, as well as scalability of the printing processes, leading to an improvement of the current state from both an economic and medical perspective. Thus, the following chapter presents an overview of the most common hardware components regarding already established bioprinting automation, future automation potentials and procedural interfaces for further automation as well as transferrable technologies from other industries.
To identify automation potentials and find currently applied automation solutions, hardware components and process steps need to be observed and analyzed. Due to the wide range of different systems and printing methods those differ. Yet there are fundamental similarities that can be schematically illustrated cumulatively (Figure 1).
FIGURE 1. General 3D-Bioprinting process chain and illustration of hardware components with examples for the use of QA-Sensors towards live anomaly detection for process control (reprinted (adapted) with permission from (Jin et al., 2021). Copyright 2021 American Chemical Society) as well as the use of ROI imaging for bio-chemical quality control (Tröndle et al., 2019).
In general, the 3D-Bioprinting process chain can be distinguished in print preparation, the printing procedure, and post-printing tissue maturation (Figure 1). Print preparation covers the entire path from 3D design, to the generation of a data set for path calculation of the subsequent print path, to material synthesis and cell cultivation as well as the final bioink formulation. In the post-printing phase, the printed 3D structure is typically cultivated in an incubator, conditioned and is then available for further use, e.g., for OoCs, implantation or further examination of the tissue for research issues. Within the printing process which comprises fluid handling, robotics and control engineering to transfer bioink from the cartridge to a substrate or previously printed layer, a preparatory phase, the actual printing phase and the post-processing of the printed object can be subdivided in terms of automation. All these three phases offer automation potentials, and due to smooth transitions between the phases, not all components can necessarily be assigned to one phase. After hardware initialization and typically a system-depend cleaning protocol, the preparatory phase starts. There, the previously designed 3D data and the bioink are loaded into the printing system and the print cartridge, respectively. One or more bioinks are then deposited layer by layer according to the predefined print path. The way in which the material is applied and the shaping of the 3D structure are highly dependent on the specified bioprinting method and strategy (Duarte Campos and Blaeser, 2021). The most frequently used technologies and strategies are either dependent on traditional deposition techniques (extrusion- or inkjet-based) or optics-/light-based (stereolithography or laser-assisted) (Choudhury et al., 2018). Independent of the system, online process monitoring and control as well as QA-methods are taking on an increasingly important role during the printing process. The use of Artificial Intelligence (AI) for camera-based detection and evaluation of anomalies (Jin et al., 2021), bio-chemical quality control (Tröndle et al., 2019) and a monitoring system with direct print path feedback and correction (Armstrong et al., 2019) are just a few examples. AI could help to combine and accel those technologies even further. The post-processing phase includes all steps that take place after printing and before cultivation in the incubator, e.g. robotic transportation of the printed object and intermediate storage in a magazine for OoC-applications. Further examples of the current state and future potentials regarding the print-process automation are presented and discussed in the following chapters.
In addition to the general view and the former illustration (Figure 1), it is useful to get an overview of the different printing methods to connect them with hardware, sensors and control components. Table 1 shows in a structured way which process steps, components as well as sensors and actuators are applicable for which 3D-bioprinting method with regard to process automation.
TABLE 1. Assignment of process steps, involved printing elements and hardware components to their applicable printing methods.
The assignment shown here is not complete due to clarity reasons, but reflects the most important elements and a large part of the sensors and actuators for 3D-Bioprinting. Components that appear repeatedly in different print elements are listed only once, including their associated sensors and actuators. This provides a reasonable reference in a clear framework to the topic of automation and automation potentials. In addition to the above, from a technological cell biological point of view, further consideration of biosensors would be interesting, for example, in order to be able to draw conclusions about cell expression and contraction as well as proliferation and metabolic activities. Equally, from a manufacturing perspective, control and regulation, further sensors, e.g. for recording micro-vibrations, or safety-relevant elements such as end stops can be considered.
The term 3D-Bioprinting first emerged in scientific articles in the early 2000s, while the first bioprinter according to today’s understanding, a modified standard inkjet printer, originates from 2003 (Wilson and Boland, 2003). The first patents on bioprinting appeared in a similar time frame between 2001 and 2003 (Bicudo et al., 2021). Since its beginnings, the technology has already evolved greatly, advancing from a niche technology further and further into a wide variety of sectors to fulfill prerequisites for commercial applications (Combellack et al., 2018). During this development, automation is also becoming increasingly important in the context of process-driven research of 3D-Bioprinting in order to meet standardized, scalable and economic constraints (Santoni et al., 2021). Modern 3D-Bioprinting itself requires a certain level of automation to be functional, which leads to the current state of the art. The use of basic sensors, actuators and robotics as well as simple process monitoring measures is evident from the description of the different processes (Vanaei et al., 2021). Recent publications even already show single advanced technologies relying on QA methods for process prediction and adaptation (Rimann et al., 2016; Narayanan et al., 2018; Riba et al., 2020; Armstrong et al., 2021; Elbadawi et al., 2021). The goal of the latest research and development by universities, bioprinting companies, and companies in the life science and food industries are all-in-one platforms. These are designed to enable the use of multiple materials, tools for further processing and process monitoring, and the use of AI (Santoni et al., 2021). The described advances are mostly in the early stages and not ready yet for commercialization on a large and economic scale.
In other industries process automation is well known and broadly applied. It is also a well-researched subject area itself that is still constantly evolving. The following subsections present learncases from other industries that offer great potential to be applied to 3D-Bioprinting automation in the future. Of course, the elements and technologies use to overlap in the individual sectors, which is why hereafter the most advanced and most frequently used are mentioned in each case.
In the food industry, process automation solutions have been used in a variety of ways for many years. Gripping and transport systems as well as monitoring and ensuring a sterile working environment have a major role in the standardized and automated implementation of these measures (Ilyukhin et al., 2001; Holmes et al., 2013). For example, in large-scale processing of fruits and vegetables on plantations, non-invasive vacuum gripping systems are often used for fast and reliable pick and place applications to manipulate products and enable an end-to-end process chain (Blanes et al., 2011; Morales et al., 2014). To ensure high product quality and undamaged products, camera-based systems are used in combination with actuators that, for example, perform an automated sorting process (Bee and Honeywood, 2003; Bader and Rahimifard, 2020). In fully automated operations this is followed by direct packaging of the products according to specified standards. The processing of many foods requires a clean and uncontaminated atmosphere, for example to protect against the presence of salmonella. To meet the specified regulations, various methods are used here depending on the application (de Alwis and Fryer, 1990; Guzel-Seydim et al., 2004; Oscar, 2005; Huang et al., 2008; Velugoti et al., 2011).
Conventional printing processes are multiparametric and highly dynamic processes. For this reason, the use of sensors, actuators, mechanics and a control unit, which represents the structure of a mechatronic system (Tehrani et al., 2016), is highly advanced here in order to be able to achieve high and consistent print quality. Technologies for measuring and adjusting pressure, position, temperature and air bubbles play a crucial role in industrial printing applications and thus are broadly applied. To achieve better results, increase productivity and reduce downtimes, artificial intelligence methods are emerging more and more in modern industrial printing machines (Neeb et al., 2019).
In terms of its characteristics and general structure, conventional 3D-Printing is very similar to 3D-Bioprinting. This allows a good transfer of knowledge between the two technologies, so that 3D-Bioprinting can benefit from the more advanced knowledge of 3D-Printing. The focus here is specifically on material deposition (MacDonald and Wicker, 2016; Lee et al., 2017), robot kinematics (Qian et al., 2018; Jinghua et al., 2020), printing space and platform (Sitthi-Amorn et al., 2015), optical QA-methods and a fully digitized process chain or 3D-Printing factory (Rengier et al., 2010).
Artificial intelligence is entering everyday life more and more and offers a variety of innovative and useful methods to solve problems in an automated and intelligent way. Using machine learning, fast and precise predictions can be made about process parameters and results, and multi-dimensional sensor signals can be merged and interpreted. In modern industrial production facilities, sensor data fusion and predictive maintenance are already being used in the field to compare target and actual data and have proven to be accelerators here (Aliustaoglu et al., 2009; Hashemian, 2011; Indri et al., 2019; Nazir and Shao, 2021; Pech et al., 2021). Nowadays, medicine and medical technology use image processing methods to segment and classify structures in order to draw conclusions about diseases and the resulting therapies (Liu et al., 2019; Shi et al., 2021).
Between 2016 and 2021 the number of publications on the topic automation in bioprinting and tissue engineering indicated a strong increase in interest1. Individual publications show groundbreaking results on how the use of automation solutions can help to monitor and adjust crucial process parameters and to draw conclusions about cell biological characteristics or allow the printing of complex 3D structures with hydrogels (Hinton et al., 2015; Shi et al., 2018; Armstrong et al., 2019; Jin et al., 2021; Poologasundarampillai et al., 2021; Yang et al., 2021). At the same time, the number of patents and companies embracing 3D-Bioprinting continue to grow (Santoni et al., 2021). However, no technology has yet achieved the major breakthrough to commercial marketing and industrial production for a broad mass (Ng et al., 2019). One reason for this is the lack of interfaces between different process steps, respectively the frequent interruption of the process chain by human intervention for transport or inspection tasks. Application-specific placement and transport systems, as often used in the food industry (chapter 2.3.1), can act as an interface and close the chain in a relatively simple way, thus enabling, for example, large-scale production of OoCs. Even in 2011 a publication showed the deficiencies of the simple use of 3D-Bioprinters and argued with the requirement of an additional sophisticated production line to enable commercial biofabrication (Mironov et al., 2011). The smart use and aggregation of sensor data is another way to facilitate the commercial market entry of 3D-Bioprinting. Combined with AI applications, this allows real-time data to be analyzed, predictions to be made, and even parameter adjustments to be made automatically. This not only leads to higher process accuracy and productivity, but in the future can become the foundation for standardization and therefore also the driving force on the path to certification to meet regulatory guidelines for medical therapies or food products (Li and Faulkner, 2017; Haeusner et al., 2021; Schmidt et al., 2021). Current studies predict, for instance, that clean meat could become part of the everyday diet in a few years (Chriki and Hocquette, 2020; Lee et al., 2020). Automation technology will play a major role in making the transfer from laboratory applications to scalable production and economically viable commercialization, thus exploiting the technological potential of 3D-Bioprinting as far as possible.
Despite recent developments and an increased interest to use the power and knowledge of process automation to advance 3D-Bioprinting, the field has still a lot of room for further improvement. Within the nature of a multiparametric process with high standards towards every parameter and their combination, 3D-Bioprinting is a rather complex application. In addition to the high technological demands on the printing system and the materials for implementing the actual procedure, this places equally high standards on process automation and technological development for process optimization. This results in strict regulatory requirements for the approval of products, high research expenditure and high costs. To overcome these challenges multidisciplinary research should be conducted by experts in cell biology, pharmacy and medicine, but equally by engineers with knowledge in additive manufacturing, material science, artificial intelligence and mechatronics.
3D-Bioprinting, whether in the field of new regenerative medical products as biomimetic and bionic cell-loaded implants, the development of clean meat or for drug discovery via the use of OoCs, has emerged as a novel innovative technology with great potential for the future. This is demonstrated both by the recent strong increase of interest from scientific institutions via rising publication numbers as well as exponential increasing patent registrations and industrial interest. Further research in the field of 3D-Bioprinting process automation, especially in the area of live quality monitoring, is essential to overcome technological and regulatory challenges. Progress from this and further developments in AI will be supported in the future by the smart combination and use of already known sensor and control technologies that can be burrowed from other sectors (e.g. food industry, conventional printing and additive manufacturing industry) to fully exploit the great potential of 3D-Bioprinting. Thus, the production of new regenerative medicinal products, clean meat and OoCs for animal-free drug development can be further advanced to make commercial biofabrication more realistic from an economic and scalability perspective.
The original contributions presented in the study are included in the article/Supplementary Material, further inquiries can be directed to the corresponding authors.
NL wrote the drafts of the manuscript, reviewed the related literature, and prepared the table and figure. AB was responsible in a supervisory role for conceptualization and idea generation of the manuscript, implementation of technical details, and writing as well as revision of the authored document. The drafting of the manuscript took place in an exchange of ideas between both authors and both authors approved a submission of the final version.
We acknowledge support by the Deutsche Forschungsgemeinschaft (DFG–German Research Foundation) and the Open Access Publishing Fund of Technical University of Darmstadt.
The authors declare that the research was conducted in the absence of any commercial or financial relationships that could be construed as a potential conflict of interest.
All claims expressed in this article are solely those of the authors and do not necessarily represent those of their affiliated organizations, or those of the publisher, the editors and the reviewers. Any product that may be evaluated in this article, or claim that may be made by its manufacturer, is not guaranteed or endorsed by the publisher.
1According to PubMed, publications on the terms “bioprinting automation” and “tissue engineering automation” have increased from 20 in 2016 to 47 in 2021 per year, accessed January 2022.
Aliustaoglu, C., Ertunc, H. M., and Ocak, H. (2009). Tool Wear Condition Monitoring Using a Sensor Fusion Model Based on Fuzzy Inference System. Mech. Syst. Signal Process. 23, 539–546. doi:10.1016/J.YMSSP.2008.02.010
Armstrong, A. A., Norato, J., Alleyne, A. G., and Wagoner Johnson, A. J. (2019). Direct Process Feedback in Extrusion-Based 3D Bioprinting. Biofabrication 12, 015017. doi:10.1088/1758-5090/AB4D97
Armstrong, A. A., Pfeil, A., Alleyne, A. G., and Wagoner Johnson, A. J. (2021). Process Monitoring and Control Strategies in Extrusion-Based Bioprinting to Fabricate Spatially Graded Structures. Bioprinting 21, e00126. doi:10.1016/J.BPRINT.2020.E00126
Atala, A., Bauer, S. B., Soker, S., Yoo, J. J., and Retik, A. B. (2006). Tissue-engineered Autologous Bladders for Patients Needing Cystoplasty. The Lancet 367, 1241–1246. doi:10.1016/S0140-6736(06)68438-9
Ayan, B., Heo, D. N., Zhang, Z., Dey, M., Povilianskas, A., Drapaca, C., et al. (2020). Aspiration-assisted Bioprinting for Precise Positioning of Biologics. Sci. Adv. 6, 5111. doi:10.1126/SCIADV.AAW5111
Bader, F., and Rahimifard, S. (2020). A Methodology for the Selection of Industrial Robots in Food Handling. Innovative Food Sci. Emerging Tech. 64, 102379. doi:10.1016/J.IFSET.2020.102379
Bak, D. (2003). Rapid Prototyping or Rapid Production? 3D Printing Processes Move Industry towards the Latter. Assembly Automation 23, 340–345. doi:10.1108/01445150310501190
Bee, S. C., and Honeywood, M. J. (2003). Colour Sorting in the Food Industry. Mach. Vis. Insp. Nat. Prod. 163, 189. doi:10.1007/1-85233-853-9_5
Berman, B. (2012). 3-D Printing: The New Industrial Revolution. Business Horizons 55, 155–162. doi:10.1016/J.BUSHOR.2011.11.003
Bicudo, E., Faulkner, A., and Li, P. (2021). Patents and the Experimental Space: Social, Legal and Geographical Dimensions of 3D Bioprinting. Int. Rev. L. Comput. Techn. 35, 2–23. doi:10.1080/13600869.2020.1785066
Blanes, C., Mellado, M., Ortiz, C., and Valera, A. (2011). Review. Technologies for Robot Grippers in Pick and Place Operations for Fresh Fruits and Vegetables. Span J. Agric. Res. 9, 1130. doi:10.5424/sjar/20110904-501-10
Choudhury, D., Anand, S., and Naing, M. W. (2018). The Arrival of Commercial Bioprinters - towards 3D Bioprinting Revolution!. Int. J. Bioprint 4, 139. doi:10.18063/IJB.V4I2.139
Chriki, S., and Hocquette, J.-F. (2020). The Myth of Cultured Meat: A Review. Front. Nutr. 7, 7. doi:10.3389/FNUT.2020.00007
Chung, J. H. Y., Naficy, S., Yue, Z., Kapsa, R., Quigley, A., Moulton, S. E., et al. (2013). Bio-ink Properties and Printability for Extrusion Printing Living Cells. Biomater. Sci. 1, 763–773. doi:10.1039/C3BM00012E
Combellack, E., Jessop, Z. M., and Whitaker, I. S. (2018). The Commercial 3D Bioprinting Industry. 3d Bioprinting Reconstr. Surg. Tech. Appl., 413–421. doi:10.1016/B978-0-08-101103-4.00029-6
de Alwis, A. A. P., and Fryer, P. J. (1990). The Use of Direct Resistance Heating in the Food Industry. J. Food Eng. 11, 3–27. doi:10.1016/0260-8774(90)90036-8
Duarte Campos, D. F., and Blaeser, A. (2021). 3D-Bioprinting. Cham: Springer, 201–232. doi:10.1007/978-3-030-66749-8_9
Elbadawi, M., Mccoubrey, L. E., Gavins, F. K. H., Ong, J. J., Goyanes, A., Gaisford, S., et al. (2021). Disrupting 3D Printing of Medicines with Machine Learning. Trends Pharmacol. Sci. 42, 745–757. doi:10.1016/j.tips.2021.06.002
Godbey, W. T., and Atala, A. (2002). In VitroSystems for Tissue Engineering. Ann. N. Y. Acad. Sci. 961, 10–26. doi:10.1111/J.1749-6632.2002.TB03041.X
Gómez-Blanco, J. C., Galván-Chacón, V., Patrocinio, D., Matamoros, M., Sánchez-Ortega, Á. J., Marcos, A. C., et al. (2021). Improving Cell Viability and Velocity in μ-Extrusion Bioprinting with a Novel Pre-incubator Bioprinter and a Standard FDM 3D Printing Nozzle. Materials 14, 3100. doi:10.3390/MA14113100
Grigoryan, B., Sazer, D. W., Avila, A., Albritton, J. L., Padhye, A., Ta, A. H., et al. (2021). Development, Characterization, and Applications of Multi-Material Stereolithography Bioprinting. Sci. Rep. 11, 1–13. doi:10.1038/s41598-021-82102-w
Gu, B. K., Choi, D. J., Park, S. J., Kim, Y.-J., and Kim, C.-H. (2018). 3D Bioprinting Technologies for Tissue Engineering Applications. Adv. Exp. Med. Biol. 1078, 15–28. doi:10.1007/978-981-13-0950-2_2
Guzel-Seydim, Z. B., Greene, A. K., and Seydim, A. C. (2004). Use of Ozone in the Food Industry. LWT - Food Sci. Techn. 37, 453–460. doi:10.1016/J.LWT.2003.10.014
Haeusner, S., Herbst, L., Bittorf, P., Schwarz, T., Henze, C., Mauermann, M., et al. (2021). From Single Batch to Mass Production-Automated Platform Design Concept for a Phase II Clinical Trial Tissue Engineered Cartilage Product. Front. Med. 8, 712917. doi:10.3389/FMED.2021.712917
Hashemian, H. M. (2011). State-of-the-art Predictive Maintenance Techniques. IEEE Trans. Instrum. Meas. 60, 226–236. doi:10.1109/TIM.2010.2047662
Hinton, T. J., Jallerat, Q., Palchesko, R. N., Park, J. H., Grodzicki, M. S., Shue, H.-J., et al. (2015). Three-dimensional Printing of Complex Biological Structures by Freeform Reversible Embedding of Suspended Hydrogels. Sci. Adv. 1, 1500758. doi:10.1126/SCIADV.1500758
Hirt, M. N., Hansen, A., and Eschenhagen, T. (2014). Cardiac Tissue Engineering. Circ. Res. 114, 354–367. doi:10.1161/CIRCRESAHA.114.300522
Holmes, J. F., Russell, G., and Allen, J. K. (2013). Supervisory Control and Data Acquisition (SCADA) and Related Systems for Automated Process Control in the Food Industry: an Introduction. Robot. Autom. Food Ind. Curr. Futur. Technol., 130–142. doi:10.1533/9780857095763.1.130
Huang, Y.-R., Hung, Y.-C., Hsu, S.-Y., Huang, Y.-W., and Hwang, D.-F. (2008). Application of Electrolyzed Water in the Food Industry. Food Control 19, 329–345. doi:10.1016/J.FOODCONT.2007.08.012
Ilyukhin, S. V., Haley, T. A., and Singh, R. K. (2001). A Survey of Automation Practices in the Food Industry. Food Control 12, 285–296. doi:10.1016/S0956-7135(01)00015-9
Indri, M., Lachello, L., Lazzero, I., Sibona, F., and Trapani, S. (2019). Smart Sensors Applications for a New Paradigm of a Production Line. Sensors 19, 650. doi:10.3390/s19030650
Jin, Z., Zhang, Z., Shao, X., and Gu, G. X. (2021). Monitoring Anomalies in 3D Bioprinting with Deep Neural Networks. ACS Biomater. Sci. Eng. doi:10.1021/ACSBIOMATERIALS.0C01761
Jinghua, X., Xueqing, F., Jun, C., and Shuyou, Z. (2020). Precision Forward Design for 3D Printing Using Kinematic Sensitivity via Jacobian Matrix Considering Uncertainty. Int. J. Adv. Manuf. Technol. 110, 3257–3271. doi:10.1007/S00170-020-05940-4
Kačarević, Ž., Rider, P., Alkildani, S., Retnasingh, S., Smeets, R., Jung, O., et al. (2018). An Introduction to 3D Bioprinting: Possibilities, Challenges and Future Aspects. Materials 11, 2199. doi:10.3390/MA11112199
Kingsley, D. M., Roberge, C. L., Rudkouskaya, A., Faulkner, D. E., Barroso, M., Intes, X., et al. (2019). Laser-based 3D Bioprinting for Spatial and Size Control of Tumor Spheroids and Embryoid Bodies. Acta Biomater. 95, 357–370. doi:10.1016/J.ACTBIO.2019.02.014
Kumar, H., and Kim, K. (2020). Stereolithography 3D Bioprinting. Methods Mol. Biol. 2140, 93–108. doi:10.1007/978-1-0716-0520-2_6
Lee, H. J., Yong, H. I., Kim, M., Choi, Y.-S., and Jo, C. (2020). Status of Meat Alternatives and Their Potential Role in the Future Meat Market - A Review. Asian-australas J. Anim. Sci. 33, 1533–1543. doi:10.5713/AJAS.20.0419
Lee, J.-Y., An, J., and Chua, C. K. (2017). Fundamentals and Applications of 3D Printing for Novel Materials. Appl. Mater. Today 7, 120–133. doi:10.1016/J.APMT.2017.02.004
Li, P., and Faulkner, A. (2017). 3D Bioprinting Regulations: a UK/EU Perspective. Eur. J. Risk Regul. 8, 441–447. doi:10.1017/ERR.2017.19
Li, P., and Liu, X. (2019). Common Sensors in Industrial Robots: A Review. J. Phys. Conf. Ser. 1267, 012036. doi:10.1088/1742-6596/1267/1/012036
Liu, C.-H., and Chiu, C.-H. (2017). “Optimal Design of a Soft Robotic Gripper with High Mechanical Advantage for Grasping Irregular Objects,” in 2017 IEEE International Conference on Robotics and Automation (ICRA), May 29–June 3, 2846–2851. doi:10.1109/ICRA.2017.7989332
Liu, X., Deng, Z., and Yang, Y. (2019). Recent Progress in Semantic Image Segmentation. Artif. Intell. Rev. 52, 1089–1106. doi:10.1007/S10462-018-9641-3
MacDonald, E., and Wicker, R. (2016). Multiprocess 3D Printing for Increasing Component Functionality. Science 35, 353. doi:10.1126/SCIENCE.AAF2093
Mironov, V., Kasyanov, V., and Markwald, R. R. (2011). Organ Printing: from Bioprinter to Organ Biofabrication Line. Curr. Opin. Biotechnol. 22, 667–673. doi:10.1016/J.COPBIO.2011.02.006
Moldovan, N. I., Hibino, N., and Nakayama, K. (2017). Principles of the Kenzan Method for Robotic Cell Spheroid-Based Three-Dimensional Bioprinting. Tissue Eng. B: Rev. 23, 237–244. doi:10.1089/ten.teb.2016.0322
Morales, R., Badesa, F. J., Garcia-Aracil, N., Sabater, J. M., and Zollo, L. (2014). Soft Robotic Manipulation of Onions and Artichokes in the Food Industry. Adv. Mech. Eng. 6, 345291. doi:10.1155/2014/345291
Narayanan, L. K., Thompson, T. L., Shirwaiker, R. A., and Starly, B. (2018). Label Free Process Monitoring of 3D Bioprinted Engineered Constructs via Dielectric Impedance Spectroscopy. Biofabrication 10, 035012. doi:10.1088/1758-5090/AACCBF
Nazir, Q., and Shao, C. (2021). Online Tool Condition Monitoring for Ultrasonic Metal Welding via Sensor Fusion and Machine Learning. J. Manufacturing Process. 62, 806–816. doi:10.1016/J.JMAPRO.2020.12.050
Neeb, S., Norrick, N. R., Henn, A., Fehlner, A., and Wolf, T. (2019). Method for Detecting and Compensating for Defective Printing Nozzles in an Inkjet Printing Machine Using a Threshold Calculation Based on Weighting FactorsU.S. Patent No.: US 2019/0291461 A1. Washington, DC: U.S. Patent and Trademark Office.
Nerem, R. M., and Sambanis, A. (2007). Tissue Engineering: From Biology to Biological Substitutes. Larchmont, NY: Mary Ann Liebert, Inc.. doi:10.1089/TEN.1995.1.3
Ng, W. L., Chua, C. K., and Shen, Y.-F. (2019). Print Me an Organ! Why We Are Not There yet. Prog. Polym. Sci. 97, 101145. doi:10.1016/J.PROGPOLYMSCI.2019.101145
Ng, W. L., Lee, J. M., Yeong, W. Y., and Win Naing, M. (2017). Microvalve-based Bioprinting - Process, Bio-Inks and Applications. Biomater. Sci. 5, 632–647. doi:10.1039/C6BM00861E
Oscar, T. P. (2005). Development and Validation of Primary, Secondary, and Tertiary Models for Growth of Salmonella Typhimurium on Sterile Chicken†. J. Food Prot. 68, 2606–2613. doi:10.4315/0362-028X-68.12.2606
Ozbolat, I. T., and Hospodiuk, M. (2016). Current Advances and Future Perspectives in Extrusion-Based Bioprinting. Biomaterials 76, 321–343. doi:10.1016/J.BIOMATERIALS.2015.10.076
Pech, M., Vrchota, J., and Bednář, J. (2021). Predictive Maintenance and Intelligent Sensors in Smart Factory: Review. Sensors 2021, 1470. doi:10.3390/S21041470
Poologasundarampillai, G., Haweet, A., Jayash, S. N., Morgan, G., Moore, J. E., and Candeo, A. (2021). Real-time Imaging and Analysis of Cell-Hydrogel Interplay within an Extrusion-Bioprinting Capillary. Bioprinting 23, e00144. doi:10.1016/J.BPRINT.2021.E00144
Qian, S., Bao, K., Zi, B., and Wang, N. (2018). Kinematic Calibration of a Cable-Driven Parallel Robot for 3D Printing. Sensors 18, 2898. doi:10.3390/S18092898
Ramiah, P., du Toit, L. C., Choonara, Y. E., Kondiah, P. P. D., and Pillay, V. (2020). Hydrogel-Based Bioinks for 3D Bioprinting in Tissue Regeneration. Front. Mater. 7, 76. doi:10.3389/FMATS.2020.00076
Rengier, F., Mehndiratta, A., Von Tengg-Kobligk, H., Zechmann, C. M., Unterhinninghofen, R., Kauczor, H.-U., et al. (2010). 3D Printing Based on Imaging Data: Review of Medical Applications. Int. J. CARS 5, 335–341. doi:10.1007/S11548-010-0476-X
Riba, J., Schoendube, J., Zimmermann, S., Koltay, P., and Zengerle, R. (2020). Single-cell Dispensing and 'real-Time' Cell Classification Using Convolutional Neural Networks for Higher Efficiency in Single-Cell Cloning. Sci. Rep. 10, 1–9. doi:10.1038/s41598-020-57900-3
Rimann, M., Bono, E., Annaheim, H., Bleisch, M., and Graf-Hausner, U. (2016). Standardized 3D Bioprinting of Soft Tissue Models with Human Primary Cells. J. Lab. Autom. 21, 496–509. doi:10.1177/2211068214567146
Santoni, S., Gugliandolo, S. G., Sponchioni, M., Moscatelli, D., and Colosimo, B. M. (2021). 3D Bioprinting: Current Status and Trends-A Guide to the Literature and Industrial Practice. Bio-des. Manuf. 5, 14–42. doi:10.1007/S42242-021-00165-0
Schmidt, A., Frey, J., Hillen, D., Horbelt, J., Schandar, M., Schneider, D., et al. (2021). “A Framework for Automated Quality Assurance and Documentation for Pharma 4.0,” in 40th International Conference on Computer Safety, Reliability and Security, September 8–10, 2021, Volume LNCS 12852, 226–239. doi:10.1007/978-3-030-83903-1_15
Shi, F., Wang, J., Shi, J., Wu, Z., Wang, Q., Tang, Z., et al. (2021). Review of Artificial Intelligence Techniques in Imaging Data Acquisition, Segmentation, and Diagnosis for COVID-19. IEEE Rev. Biomed. Eng. 14, 4–15. doi:10.1109/RBME.2020.2987975
Shi, J., Wu, B., Song, B., Song, J., Li, S., Trau, D., et al. (2018). Learning-Based Cell Injection Control for Precise Drop-On-Demand Cell Printing. Ann. Biomed. Eng. 46, 1267–1279. doi:10.1007/S10439-018-2054-2
Sitthi-Amorn, P., Ramos, J. E., Wangy, Y., Kwan, J., Lan, J., Wang, W., et al. (2015). MultiFab. ACM Trans. Graph. 34, 1–11. doi:10.1145/2766962
Tan, B., Kuang, S., Li, X., Cheng, X., Duan, W., Zhang, J., et al. (2021). Stereotactic Technology for 3D Bioprinting: from the Perspective of Robot Mechanism. Biofabrication 13, 043001. doi:10.1088/1758-5090/AC1846
Tehrani, A. H., Dörsam, E., and Neumann, J. (2016). Improving Automation and Process Control of an Indirect Gravure (Pad) Printing Machine.
Tröndle, K., Koch, F., Finkenzeller, G., Stark, G. B., Zengerle, R., Koltay, P., et al. (2019). Bioprinting of High Cell-Density Constructs Leads to Controlled Lumen Formation With Self-Assembly of Endothelial Cells. J. Tissue Eng. Regen. Med. 13, 1883–1895. doi:10.1002/TERM.2939
Vanaei, S., Parizi, M. S., Vanaei, S., Salemizadehparizi, F., and Vanaei, H. R. (2021). An Overview on Materials and Techniques in 3D Bioprinting toward Biomedical Application. Engineered Regen. 2, 1–18. doi:10.1016/J.ENGREG.2020.12.001
Velugoti, P. R., Bohra, L. K., Juneja, V. K., Huang, L., Wesseling, A. L., Subbiah, J., et al. (2011). Dynamic Model for Predicting Growth of Salmonella Spp. In Ground Sterile Pork. Food Microbiol. 28, 796–803. doi:10.1016/J.FM.2010.05.007
Wang, Z., and Hirai, S. (2018). “Geometry and Material Optimization of a Soft Pneumatic Gripper for Handling Deformable Object,” in Proceedings of the 2018 IEEE International Conference on Robotics and Biomimetics, Kuala Lumpur, Malaysia, December 12–15, 2018, 612–617. doi:10.1109/ROBIO.2018.8665234
Wilson, W. C., and Boland, T. (2003). Cell and Organ Printing 1: Protein and Cell Printers. Anat. Rec. 272A, 491–496. doi:10.1002/AR.A.10057
Xu, H., Zhang, Z., and Xu, C. (2019). Sedimentation Study of Bioink Containing Living Cells. J. Appl. Phys. 125, 114901. doi:10.1063/1.5089245
Yang, S., Wang, L., Chen, Q., and Xu, M. (2021). In Situ process Monitoring and Automated Multi-Parameter Evaluation Using Optical Coherence Tomography during Extrusion-Based Bioprinting. Additive Manufacturing 47, 102251. doi:10.1016/J.ADDMA.2021.102251
Keywords: automation, artificial intelligence, biofabrication, 3D-Bioprinting, manufacturing, Organs-on-Chips, process automation
Citation: Lindner N and Blaeser A (2022) Scalable Biofabrication: A Perspective on the Current State and Future Potentials of Process Automation in 3D-Bioprinting Applications. Front. Bioeng. Biotechnol. 10:855042. doi: 10.3389/fbioe.2022.855042
Received: 14 January 2022; Accepted: 01 April 2022;
Published: 20 May 2022.
Edited by:
Hyun-Wook Kang, Ulsan National Institute of Science and Technology, South KoreaReviewed by:
Henrique de Amorim Almeida, Polytechnic Institute of Leiria, PortugalCopyright © 2022 Lindner and Blaeser. This is an open-access article distributed under the terms of the Creative Commons Attribution License (CC BY). The use, distribution or reproduction in other forums is permitted, provided the original author(s) and the copyright owner(s) are credited and that the original publication in this journal is cited, in accordance with accepted academic practice. No use, distribution or reproduction is permitted which does not comply with these terms.
*Correspondence: Nils Lindner, bGluZG5lckBpZGQudHUtZGFybXN0YWR0LmRl; Andreas Blaeser, YmxhZXNlckBpZGQudHUtZGFybXN0YWR0LmRl
Disclaimer: All claims expressed in this article are solely those of the authors and do not necessarily represent those of their affiliated organizations, or those of the publisher, the editors and the reviewers. Any product that may be evaluated in this article or claim that may be made by its manufacturer is not guaranteed or endorsed by the publisher.
Research integrity at Frontiers
Learn more about the work of our research integrity team to safeguard the quality of each article we publish.