- 1Oregon Rural Practice-Based Research Network, Oregon Health and Science University, Portland, OR, United States
- 2Systems Science Program, Portland State University, Portland, OR, United States
- 3Independent Researcher, Portland, OR, United States
- 4Department of Medical Informatics and Clinical Epidemiology, Oregon Health and Science University, Portland, OR, United States
Traumatic brain injury (TBI) is a highly complex phenomenon involving a cascade of disruptions across biomechanical, neurochemical, neurological, cognitive, emotional, and social systems. Researchers and clinicians urgently need a rigorous conceptualization of brain injury that encompasses nonlinear and mutually causal relations among the factors involved, as well as sources of individual variation in recovery trajectories. System dynamics, an approach from systems science, has been used for decades in fields such as management and ecology to model nonlinear feedback dynamics in complex systems. In this mini-review, we summarize some recent uses of this approach to better understand acute injury mechanisms, recovery dynamics, and care delivery for TBI. We conclude that diagram-based approaches like causal-loop diagramming have the potential to support the development of a shared paradigm of TBI that incorporates social support aspects of recovery. When developed using adequate data from large-scale studies, simulation modeling presents opportunities for improving individualized treatment and care delivery.
Introduction
Worldwide, sixty-nine million people sustain traumatic brain injury (TBI) annually (Dewan et al., 2018). Experiencing TBI can have long-term health consequences that affect not only the patient, but also caregivers, extended families, and communities (Eunice Kennedy Shriver National Institute of Child Health and Human Development, 1993; Hyder et al., 2007; James et al., 2019; Malec et al., 2017; Qadeer et al., 2017; Iaccarino et al., 2018; Carlozzi et al., 2020; Sodders et al., 2020). Patients with TBI often experience physical, behavioral, emotional, and cognitive difficulties that can persist many years post-injury and affect crucial aspects of everyday life, including independence, mobility, employment, and community integration (Finnanger et al., 2013; Arango-Lasprilla et al., 2018; Seagly et al., 2018). Social and interpersonal impacts have been shown to significantly interfere with community living, occupational status, and sustainment of interpersonal relationships (Marsh and Knight 1991; Franulic et al., 2000). For caregivers of patients with TBI, compromised physical and mental health, social participation, family functioning, social and spousal relationships, employment, finances, and self-care have been well documented (Saban et al., 2016; Griffin et al., 2017; Brickell et al., 2019; Dreer et al., 2019).
TBI has been identified as the “most complex disease in the most complex organ” of the body, (Wheble and Menon 2016), spanning biomechanical, neurochemical, neurological, cognitive, emotional, and social dimensions (Kenzie et al., 2017; 2018). Despite the establishment of evidence-based treatment guidelines (Carney et al., 2017) and considerable investment in large, multi-centered research consortia (Yue et al., 2013; Cifu et al., 2015; Ivory and Bellgowan 2015; Maas et al., 2015), little progress has been made in acute care therapeutics for TBI, and clinical trials addressing acute care consistently fail (Narayan 2002; Samadani 2016). Similarly, rehabilitation for TBI has shown minimal progress. A 1999 systematic review of cognitive rehabilitation for TBI reported insufficient evidence for the effectiveness of this standard intervention (Carney et al., 1999). Twelve years later, the Institute of Medicine reported the same findings (IOM 2011).
Researchers and clinicians urgently need a rigorous conceptualization of brain injury that encompasses nonlinear and mutually causal relations among the factors involved, as well as sources of individual variation in recovery trajectories. The fundamental necessity of this type of systems approach has been advocated by medical and scientific research experts for nearly 30 years. The National Center for Medical Rehabilitation Research (NCMRR) published a research plan that rejected the traditional linear view of rehabilitation and encouraged the adoption of a systems approach as “an essential feature of medical rehabilitation research and, ultimately, all health care delivery” (1993). Unfortunately, this mandate has not been accomplished, and recent TBI research continues the call for a systems perspective (Bigler 2016; Kenzie et al., 2017; Kenzie et al., 2018).
System dynamics, an approach from complex systems science (Sterman 2000), involves modeling nonlinear feedback relationships that produce nonlinear behavior, either with diagrams or simulations based on ordinary differential equations (ODEs). This approach has been used in several ways to address the complexity of TBI and provide tools to facilitate understanding and aid diagnostic and prognostic capabilities. The purpose of this mini-review is to summarize and compare current applications of system dynamics modeling for TBI and highlight opportunities for further development.
Methods
To locate studies using system dynamics modeling for TBI research, we searched Google Scholar and PubMed using relevant terms. We focused on articles published in the past 15 years that used system dynamics modeling (e.g., causal-loop diagramming or system dynamics simulation) in some aspect of TBI pathophysiology, diagnosis, prognosis, treatment, recovery, or care delivery. Because much of this research is emerging, we included conference publications. Articles were not vetted for quality due to the small number of studies identified and the descriptive nature of this brief review.
Results
Ten publications were identified that fit the search criteria. Included studies span a variety of fields, approaches, and research questions. Table 1 summarizes the purpose of modeling and the approach used for each study.
Acute injury mechanisms
Some studies used system dynamics to model specific pathophysiological mechanisms, such as intracranial pressure (ICP) and inflammation. Because system dynamics modeling involves accumulations and flows, it is especially well suited for modeling ICP. Although the properties of brain regions can differ significantly, treating volumes and pressures in an aggregate fashion is appropriate due to the rigid volume constraint of the intracranial cavity.
Early researchers used electrical circuit analogs and their associated ODEs to study elevated ICP resulting from hematoma and brain swelling during the acute post-injury phase of TBI (Marmarou 1973 and Ursino 1988). The mathematics of system dynamics is identical to these electrical analogs and has the advantage of a diagrammatic visualization that is more comprehensible for most clinicians than electrical circuit diagrams. Wakeland and Goldstein (2008) for a review of computational ICP models. Early pioneers in the computational modeling of ICP dynamics were able to match empirical ICP trajectory data remarkably well, aided by their detailed logic for cerebral autoregulation (Marmarou et al., 1978; Ursino and Alberto Lodi 1997). Models developed by Ursino and his team included multiple aspects of cerebral autoregulation, calibrated with data from a prospective study in which patients were given mild ventilation challenges (Ursino et al., 2000), and showed excellent prediction potential. Wakeland et al. (2009) also calibrated a systems dynamics ICP model on patient data collected prospectively for pediatric TBI patients. In this model, patients were given mild physiologic challenges (raising or lowering the head of the bed or moderately adjusting ventilator settings) at multiple time points, but results revealed inadequate clinical prediction capability due to patient responses to a dramatically varying stimulus. Clinical usage of computer models to improve the treatment of pediatric patients will be limited until these differential responses seen clinically are better understood. Moreover, using an aggregate modeling method to understand the etiology of local regions of ischemia would likely be very difficult and would require instead a more spatially explicit methodology. In some cases, a hybrid methodology with some aspects being treated as aggregate quantities and others being highly localized might be effective. Disaggregated aspects could be modeled using finite elements or agent-oriented logic.
Scheff et al. (2013) present an intriguing differential equation-based model involving inflammatory responses to injury at multiple scales. Researchers first developed a data-driven physio-chemical network model of endotoxemia focused on transcriptional processes and cellular signaling cascades. Next, an indirect response model of the pharmacokinetics and dynamics of immunomodulatory was described. These molecular-level cascades influence cortisol and epinephrine and therefore heart rate variability, which previous research indicates is correlated with disease state. However, the model was not calibrated with or verified against patient data.
Vaughan et al. (2018) developed a differential equation model of the interactions between pro-and anti-inflammatory cytokines (IL-1B, IL-4, IL-10, IL-12), M1-and M2-like microglia, and central nervous system tissue damage. The model replicated the complex cascades associated with neuroinflammation and was calibrated using data collected during the first 5 days following severe TBI using the Matlab toolset. Patients were classified into three subgroups, and the optimal model parameter values by subgroup revealed distinct mechanistic differences in neuroinflammatory patterns. The resulting increase in understanding of microglia pathophysiology will help to improve acute TBI treatment.
Kochis et al. (2021) developed a simulation model describing the concentrations of glial fibrillary acidic protein (GFAP) and immunoglobulin G (IgG) in the bloodstream following TBI with and without repeated injury. Understanding the rates of change of these levels throughout recovery has the potential of informing the use of these biomarkers for clinical assessment of injury severity and recovery.
Complex recovery dynamics
To describe the complex dynamics of recovery across multiple levels, members of the current author team previously developed a conceptual systems framework for studying concussion (Kenzie et al., 2017), followed by a causal-loop diagram of mTBI recovery (Kenzie et al., 2018) and preliminary computational system dynamics model of mTBI recovery patterns (Wakeland and Kenzie 2019). The causal-loop diagram and subdiagrams, one of which is shown in Figure 1, visually illustrate interactions between variables at cellular, network, experiential, and social levels, particularly feedback relationships that form the basis of nonlinear system behavior. It was created through an iterative process involving literature review and consultation with subject matter experts. It serves as a synthesis of the current scientific understanding of recovery dynamics in mTBI.
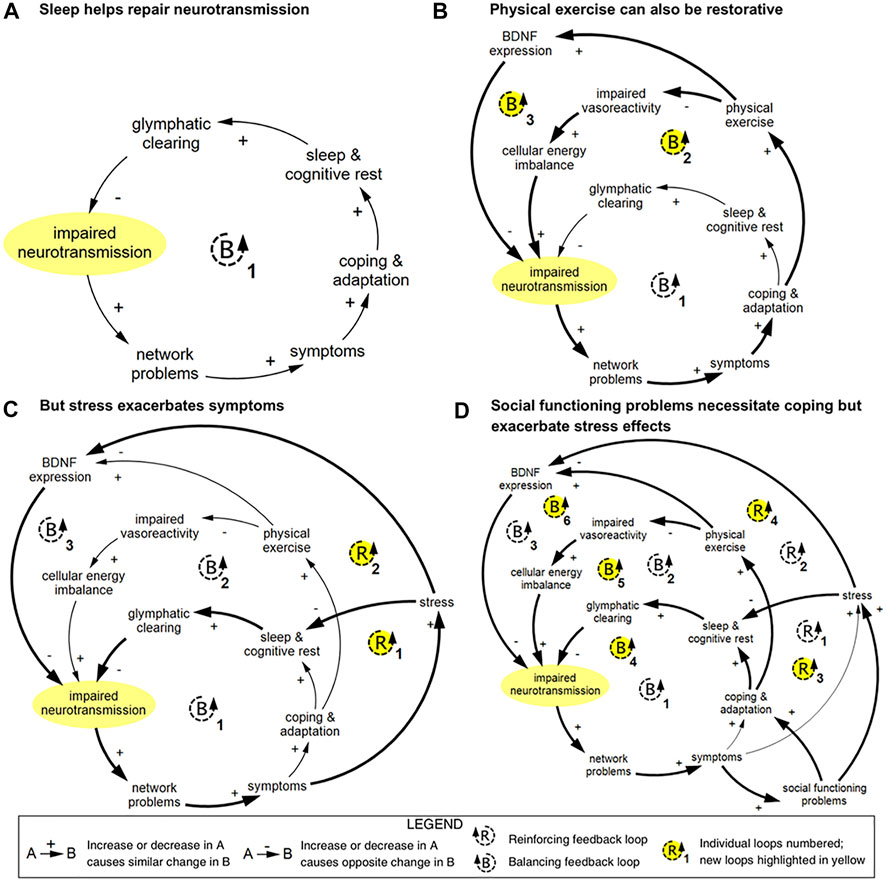
FIGURE 1. Example of causal-loop diagram showing feedback loops pertaining to impaired neurotransmission in TBI, reproduced from Kenzie et al. (2018). This series of diagrams illustrates how connected loops can have compounding and counteractive effects. (A) In loop B1, impaired neurotransmission affects the function of networks; these networks and network functions include limbic, intrinsic connectivity networks, attentional filtering, and processing speed. Disruption in these networks results in a range of symptoms that prompt coping and adaptation strategies. Restorative sleep processes lead to glymphatic clearing of brain waste and energy byproducts, which in turn results in improved neurotransmission via an improved cellular milieu and support of neuroplasticity. (B) In loop B2, physical exercise is used as a coping and adaptation strategy, which improves vasoreactivity and cellular energy imbalance, which supports neurotransmission. In loop B3, brain-derived neutrophic factor (BDNF) expression is strengthened, which reduces impaired neurotransmission via improved neuroplasticity. (C) Stress can disrupt sleep and inhibit BDNF expression, which creates two reinforcing loops. (D) Social functioning problems can prompt coping and adaptation, which introduces three additional balancing loops, and increase stress, which compounds the reinforcing effects of stress. Diagrams rendered in MapSys.
A computational model based upon the basic causal structure of the causal-loop diagram discussed above was able to generate estimated recovery trajectories for all severities of TBI given different inputs (Wakeland and Kenzie 2019). Due to limitations in obtaining high-quality time course data to inform the simulation model, many of the equations included in the model were approximations. The model therefore serves as an illustration of the potential capabilities of computational system dynamics to model TBI recovery trajectories.
Care delivery
Even when evidence-based guidelines for TBI exist, implementation of guidelines in clinical practice remains limited and challenging (Pilar et al., 2020). Yet systems dynamics approaches can also be used to create specific guideline-based care strategies for medical teams and hospitals in complex fast-paced, transdisciplinary working environments. Pilar et al. (2020) used systems dynamics group model building to elicit stakeholder knowledge about three regional pediatric intensive care units (ICUs) treating children with severe TBI. Three groups of stakeholders (nurses, trainees, and attending physicians) were engaged in nine group model building sessions focused on factors related to timely decision-making and guideline-based workflows. Gathering information in this way from multiple stakeholders revealed crucial dynamics consistent across each ICU hospital site regarding communication, standardized protocols, TBI-related knowledge and education of the clinical provider(s), resources, and leadership. These dynamics were captured in causal-loop diagrams of ICU workflow that were then integrated with a novel clinical implementation strategy—a computer technology-based “bedside guideline engine” to facilitate timely and consistent delivery of evidence-based TBI pediatric care (Pilar et al., 2020). Similar mixed methods systems have been utilized to improve emergency department care and analyze how systems dynamics in a single department affect broader hospital performance, patient care, and outcomes over time (Jones et al., 2012).
Dynamic systems models of patient and clinical workflows over time thus provide strategies that may be singularly suited for implementing care guidelines consistently in multiple complex medical environments. Systems modeling techniques have also demonstrated utility for predicting what resources for clinical care may be needed in the future. For example, Lee et al. (2015) identified from government data the number of individuals in New South Wales whose characteristics met the criteria for ‘intellectual developmental disorders’ (including TBI) and then developed a computational systems dynamics model to predict the prevalence of these disorders in 2043, with the goal of more effectively preparing for future healthcare needs.
Discussion
To address the need for complexity-informed approaches for understanding TBI injury and recovery, system dynamics has been used in recent years to model isolated mechanisms, complex relationships between factors in recovery, and aspects of care delivery. Approaches range from highly speculative mathematical models to diagram-based and group modeling methods. The scope of these models also varies widely, from specific mechanisms to a comprehensive understanding of the broad spectrum of processes involved in recovery. These differences reflect the range of capabilities of system dynamics modeling more broadly (Sterman 2000).
A defining characteristic of system dynamics modeling is that it is mechanistic, meaning that it involves a priori determination of hypothesized causal relationships between variables in a system. This determination can be closely tied to prior scientific literature or other sources (Sterman 2000; Kenzie 2021) co-created through a group process (Andersen et al., 1997; Vennix 1999; Andersen et al., 2007; Luna-Reyes et al., 2018), or made by an individual modeler (Sterman 2000), depending on the aims of the modeling effort. Contrasted with data-driven modeling approaches that treat causal processes generating system behavior as a “black box,” system dynamics modeling requires direct consideration of causal processes and mechanisms, which can support the synthesis of current scientific knowledge about a phenomenon, and can generate new hypotheses, and shared understanding within a field (Ghaffarzadegan et al., 2011; Aerts et al., 2014; Naumann et al., 2019). System dynamics simulation models can be used for in silico experimentation to preliminarily test hypotheses when direct experimentation is impractical or unethical (Lombardo et al., 2021). These approaches, particularly diagram-based methods like causal-loop diagramming, allow for the integration of heterogeneous types of variables, such as the interactions between social support and biophysical mechanisms of recovery. Moreover, information can come from different sources with varying degrees of evidence support, which mirrors the way in which we integrate different kinds of knowledge into our own mental models or understanding.
System dynamics, as with any operational modeling, is time consuming and requires specialized training (Valcourt et al., 2020). Simulation modeling also requires either time series data or some other basis for determining parameter values, which can limit its utility. Moreover, because variables are modeled as aggregate quantities, the system dynamics approach excludes network effects or interactions between individual agents (Aerts et al., 2014). This limitation is significant for TBI, which is highly sensitive to localized network dynamics related to brain topography. Therefore, system dynamics models that attempt to capture acute recovery mechanisms should be used alongside approaches that account for highly-variable network within-subject variabilities and between-subject heterogeneities. For this reason, the systems dynamics models that succeed are those able to narrow spatiotemporal questions related to the time course of specific mechanisms and avoid generalizing individual mechanistic explanations from the aggregate patterns observed.
Of the range of applications detailed in this mini-review, the use of causal-loop diagramming to integrate findings from different knowledge and information sources is perhaps the most unique contribution. Causal-loop diagrams can be particularly relevant to understanding the counterintuitive outcomes often observed among survivors of TBI, after controlling for objective variables such as severity of injury (Hawkins et al., 1996; Rath et al., 2003; O’Donnell et al., 2005; Murphy et al., 2006). For example, McLean et al. (2014) found that while higher levels of social activity are associated with higher levels of happiness, increased social activities for TBI patients was insufficient to improve reported quality of life. Authors suggested that patients’ subjective experience of their social activities could be a mediating factor influencing the observed outcome. Systems modeling could serve to reveal the underlying processes generating this and other counterintuitive outcomes.
Future TBI research could benefit from group model building to engage survivors, family and caregivers, and other stakeholders in the process of visualizing the recovery process from the “as-lived” perspective (Hovmand 2014; Siokou et al., 2014; Condon 2019). From there, a simulation model could be co-constructed. Concurrent collection of large-scale observational data could facilitate population of the model with time course data, to generate TBI recovery trajectories. When adequately supported with empirical data, simulation modeling may be able to help improve individualized treatment and care delivery. Further use of causal-loop diagramming and simulation modeling to aid in implementation of evidence-based guidelines is also a promising application.
Author contributions
EK led article search and drafting. EP, NC, and WW contributed substantially to article review and drafting. EP provided additional support to article search. All authors reviewed and endorsed the final draft.
Conflict of interest
The authors declare that the research was conducted in the absence of any commercial or financial relationships that could be construed as a potential conflict of interest.
Publisher’s note
All claims expressed in this article are solely those of the authors and do not necessarily represent those of their affiliated organizations, or those of the publisher, the editors and the reviewers. Any product that may be evaluated in this article, or claim that may be made by its manufacturer, is not guaranteed or endorsed by the publisher.
References
Aerts, Jean-Marie, Haddad, Wassim M., An, Gary, and Vodovotz, Yoram (2014). From data patterns to mechanistic models in acute critical illness. J. Crit. Care 29 (4), 604–610. doi:10.1016/j.jcrc.2014.03.018
Andersen, David F., Richardson, George P., and Vennix, Jac A. M. (1997). Group model building: Adding more science to the craft. Syst. Dyn. Rev. 13 (2), 187–201. doi:10.1002/(SICI)1099-1727(199722)13:2<187::AID-SDR124>3.0.CO;2-O
Andersen, D. F., Vennix, J. A. M., Richardson, G. P., and Rouwette, E. A. J. A. (2007). Group model building: Problem structuring, policy simulation and decision support. J. Oper. Res. Soc. 58 (5), 691–694. doi:10.1057/palgrave.jors.2602339
Arango-Lasprilla, J. C., Olabarrieta-Landa, L., Benito-Sánchez, I., Ramos-Usuga, D., Valdivia Tagarife, E., and Villaseñor, T. (2018). The relationship between mental health and quality of life in children with traumatic brain injury three months after the injury Annals of Physical and rehabilitation medicine. 12th World Congr. Int. Soc. Phys. Rehabilitation Med. 8, e550. doi:10.1016/j.rehab.2018.05.1280
Bigler, Erin D. (2016). Systems biology, neuroimaging, neuropsychology, neuroconnectivity and traumatic brain injury. Front. Syst. Neurosci. 10, 55. doi:10.3389/fnsys.2016.00055
Brickell, T., Louis, A., French, M., Rachel, L., Angela, E., Wright, M. M., et al. (2019). Factors related to perceived burden among caregivers of service members/veterans following TBI. Rehabil. Psychol. 64 (3), 307–319. doi:10.1037/rep0000272
Carlozzi, Noelle E., Rael, T., Lange, Nicholas, Michael, A., Angelle, M., Robin., A., et al. (2020). TBI-CareQOL family disruption: Family disruption in caregivers of persons with TBI. Rehabil. Psychol. 65 (4), 390–400. doi:10.1037/rep0000297
Carney, Nancy, Totten, Annette M., O’Reilly, Cindy, Ullman, Jamie S., Hawryluk, Gregory W. J., Bell, Michael J., et al. (2017). Guidelines for the management of severe traumatic brain injury, fourth edition. Neurosurgery 80 (1), 6–15. doi:10.1227/NEU.0000000000001432
Carney, N., Chesnut, R. M., Maynard, H., Mann, N. C., Patterson, P., Helfand, M., et al. (1999). Effect of cognitive rehabilitation on outcomes for persons with traumatic brain injury: A systematic review. J. Head. Trauma Rehabil. 14 (3), 277–307. doi:10.1097/00001199-199906000-00008
Cifu, David X., Diaz-Arrastia, Ramon, Rick, L., Williams, William CarneMary McDougal, and Dixon, Kirsty (2015). The VA/DoD chronic effects of neurotrauma consortium: An overview at year 1. Fed. Pract. 32 (8), 44–48.
Condon, L. (2019). How patient stories can improve health services around the world. Health Expect. 22 (5), 837. doi:10.1111/hex.12987
Dewan, Michael C., Rattani, Abbas, Gupta, Saksham, Ronnie, E., Baticulon, Ya-Ching Hung, Punchak, Maria, et al. (2018). Estimating the global incidence of traumatic brain injury. J. Neurosurg. 130 (4), 1080–1097. doi:10.3171/2017.10.JNS17352
Dreer, Laura E., Molly, K., Herman, C., Malone, L. A., Herman, Cassandra, and Malone, Laurie A. (2019). Resilience among caregivers of injured service members: Finding the strengths in caregiving. Archives Phys. Med. Rehabilitation 100 (4S), S76–S84. doi:10.1016/j.apmr.2018.12.027
Eunice Kennedy Shriver National Institute of Child Health and Human Development (1993). Research plan for the national center for medical rehabilitation research (NCMRR) (reference only). Washington, DC: NCMRR. Available at: https://www.nichd.nih.gov/publications/product/109.
Finnanger, , , Torun, G., Skandsen, T., Andersson, S., Lydersen, S., Vik, A., et al. (2013). Differentiated patterns of cognitive impairment 12 Months after severe and moderate traumatic brain injury. Brain Inj. 27 (13–14), 1606–1616. doi:10.3109/02699052.2013.831127
Franulic, A., Horta, E., Maturana, R., Scherpenisse, J., and Carbonell, C. (2000). Organic personality disorder after traumatic brain injury: Cognitive, anatomic and psychosocial factors. A 6 Month follow-up. Brain Inj. 14 (5), 431–439. doi:10.1080/026990500120538
Ghaffarzadegan, Navid, Lyneis, John, and Richardson, George P. (2011). How small system dynamics models can help the public policy process. Syst. Dyn. Rev. 27 (1), 22–44. doi:10.1002/sdr.442
Griffin, Joan M., Minji, K., Lee, Lauren R. Bangerter, Friedemann-Sánchez, Greta, Phelan, Sean M., Carlson, Kathleen F., et al. (2017). Burden and mental health among caregivers of veterans with traumatic brain injury/polytrauma. Am. J. Orthopsychiatry 87 (2), 139–148. doi:10.1037/ort0000207
Hawkins, M. L., Lewis, F. D., and Medeiros, R. S. (1996). Serious traumatic brain injury: An evaluation of functional outcomes. J. Trauma Inj. Infect. Crit. Care 41 (2), 257–264. doi:10.1097/00005373-199608000-00010
Hovmand, Peter S. (2014). Group model building and community-based system dynamics process. New York, NY: Springer New York, 17. doi:10.1007/978-1-4614-8763-0_2
Hyder, Adnan A., Colleen, A., Wunderlich, P. P., Gururaj, G., and Kobusingye, Olive C. (2007). The impact of traumatic brain injuries: A global perspective. NeuroRehabilitation 22 (5), 341–353. doi:10.3233/nre-2007-22502
Iaccarino, Corrado, Carretta, Alessandro, Nicolosi, Federico, and Morselli, Carlotta (2018). Epidemiology of severe traumatic brain injury. J. Neurosurg. Sci. 62 (5), 535–541. doi:10.23736/S0390-5616.18.04532-0
Ivory, M., and Bellgowan, P. (2015). Federal interagency traumatic brain injury research (FITBIR) bioinformatics platform for the advancement of collaborative traumatic brain injury research and analysis, 2015.
James, S. L., Theadom, A., Ellenbogen, R. G., Bannick, M. S., Montjoy-Venning, W., et al. (2019). Global, regional, and national burden of traumatic brain injury and spinal cord injury, 1990-2016: A systematic analysis for the global burden of disease study 2016. Lancet Neurol. 18 (1), 56–87. doi:10.1016/S1474-4422(18)30415-0
Jones, Peter, Chalmers, Linda, Wells, Susan, Ameratunga, Shanthi, Carswell, Peter, Ashton, Toni, et al. (2012). Implementing performance improvement in New Zealand emergency departments: The six hour time target policy national research project protocol. BMC Health Serv. Res. 12 (1), 45. doi:10.1186/1472-6963-12-45
Kenzie, Erin S., Elle, L., Parks, E., James, C., Chesnutt, G., Hawryluk, W. J., et al. (2018). The dynamics of concussion: Mapping pathophysiology, persistence, and recovery with causal-loop diagramming. Front. Neurol. 9, 203. doi:10.3389/fneur.2018.00203
Kenzie, Erin S. (2021). Get your model out there: Advancing methods for developing and using causal-loop diagrams. Portland, OR: Dissertation, Portland State University. Available at: https://pdxscholar.library.pdx.edu/open_access_etds/5664/.
Kenzie, Erin S., Parks, L., Bigler, D., Wakeland, Wayne, Chesnutt, J. C., Wakeland, W., et al. (2017). Concussion as a multi-scale complex system: An interdisciplinary synthesis of current knowledge. Front. Neurol. 8, 513. doi:10.3389/fneur.2017.00513
Kochis, Ryan M., Ahota, Aditya, Bueno Garcia, Hassler, Ryan, Z., Edward Banuelos Ruelas, Gottlieb, and Cauwenberghs, Gert (2021). “Modeling the dynamics of a secondary neurodegenerative injury following a mild traumatic brain injury,”, Mexico, 9 December 2021 (Virtual: EMBC), 4469. doi:10.1109/EMBC46164.2021.96299602021 43rd annual international conference of the IEEE engineering in medicine biology society
IOM (2011). in Cognitive rehabilitation therapy for traumatic brain injury: Evaluating the evidence. Editors Rebecca Koehler, Erin E. Wilhelm, and Ira Shoulson (Washington, DC: The National Academies Press). doi:10.17226/13220
Lee, Lynette, et al. Lee, Lynette, Heffernan, Mark, McDonnell, Geoffrey, Short, Stephanie D., and and Naganathan, Vasi (2015). A system dynamics modelling approach to studying the increasing prevalence of people with intellectual developmental disorders in New South Wales. Aust. Health Rev. 40 (3), 235. doi:10.1071/AH14150
Lombardo, Franco, Bentzien, Jörg, Berellini, Giuliano, and Muegge, Ingo (2021). In silico models of human PK parameters. Prediction of volume of distribution using an extensive data set and a reduced number of parameters. J. Pharm. Sci. 110 (1), 500–509. doi:10.1016/j.xphs.2020.08.023
Luna-Reyes, , L. F., Black, L. J., Ran, W., Deborah, L. A, Jarman, H., Richardson, G. P., and Andersen, D. F. (2018). Modeling and simulation as boundary objects to facilitate interdisciplinary ResearchModeling and simulation as boundary objects to facilitate interdisciplinary research: Modelling and simulation for interdisciplinary research. Syst. Res. Behav. Sci. 36, 494–513. doi:10.1002/sres.2564
Maas, Andrew I. R., Menon, David K., Steyerberg, Ewout W., Citerio, Giuseppe, Lecky, Fiona, Manley, Geoffrey T., et al. (2015). Participants and InvestigatorsCollaborative European NeuroTrauma effectiveness research in traumatic brain injury (CENTER-TBI): A prospective longitudinal observational study. Neurosurgery 76 (1), 67–80. doi:10.1227/NEU.0000000000000575
Malec, James F., Courtney Harold Van Houtven, , , Tanielian, Terri, Adrian, Atizado, and Dorn, Michael C. (2017). Impact of TBI on caregivers of veterans with TBI: Burden and interventions. Brain Inj. 31 (9), 1235–1245. doi:10.1080/02699052.2016.1274778
Marmarou, A., Shulman, K., and Rosende, R. M. (1978). A nonlinear analysis of the cerebrospinal fluid system and intracranial pressure dynamics. J. Neurosurg. 48 (3), 332–344. doi:10.3171/jns.1978.48.3.0332
Marsh, N. V., and Knight, R. G. (1991). Behavioral assessment of social competence following severe head injury. J. Clin. Exp. Neuropsychology 13 (5), 729–740. doi:10.1080/01688639108401086
McLean, Alison M., Jarus, Tal, Hubley, Anita M., and Jongbloed, Lyn (2014). Associations between social participation and subjective quality of life for adults with moderate to severe traumatic brain injury. Disabil. Rehabilitation 36 (17), 1409–1418. doi:10.3109/09638288.2013.834986
Murphy, Lesley, Chamberlain, Elaine, Weir, Jim, Berry, Alister, Nathaniel-James, David, Agnew, Robert, et al. (2006). Effectiveness of vocational rehabilitation following acquired brain injury: Preliminary evaluation of a UK specialist rehabilitation programme. Brain Inj. 20 (11), 1119–1129. doi:10.1080/02699050600664335
Naumann, Rebecca B., Austin, Anna E., Sheble, Laura, and Hassmiller Lich, Kristen (2019). System dynamics applications to injury and violence prevention: A systematic review. Curr. Epidemiol. Rep. 6 (2), 248–262. doi:10.1007/s40471-019-00200-w
O’Donnell, Meaghan L., Creamer, Mark, Elliott, Peter, Atkin, Christopher, and Kossmann, Thomas (2005). Determinants of quality of life and role-related disability after injury: Impact of acute psychological responses. J. Trauma Inj. Infect. Crit. Care 59 (6), 1328–1335. doi:10.1097/01.ta.0000197621.94561.4e
Pilar, Meagan R., Proctor, Enola K., and Pineda, Jose A. (2020). Development, implementation, and evaluation of a novel guideline engine for pediatric patients with severe traumatic brain injury: A study protocol. Implement. Sci. Commun. 1 (1), 31. doi:10.1186/s43058-020-00012-w
Qadeer, Anam, Khalid, Usama, Amin, Mahwish, Murtaza, Sajeela, Khaliq, Muhammad F., Shoaib, Maria, et al. (2017). Caregiver’s burden of the patients with traumatic brain injury. Cureus 9 (8), e1590. doi:10.7759/cureus.1590
Rath, Joseph F., Simon, Dvorah, Langenbahn, Donna M., Sherr, Rose Lynn, and Leonard, Diller (2003). Group treatment of problem‐solving deficits in outpatients with traumatic brain injury: A randomised outcome study. Neuropsychol. Rehabil. 13 (4), 461–488. doi:10.1080/09602010343000039
Saban, Karen L., Griffin, Joan M., Urban, Amanda, Janusek, Marissa A., Louise-Bender Pape, Theresa, Collins, Eileen, et al. (2016). Perceived health, caregiver burden, and quality of life in women partners providing care to veterans with traumatic brain injury. J. Rehabil. Res. Dev. 53 (6), 681–692. doi:10.1682/JRRD.2015.07.0143
Scheff, Jeremy D., Mavroudis, Panteleimon D., Foteinou, Panagiota T., Gary, An, Calvano, Steve E., Doyle, John, et al. (2013). A multiscale modeling approach to inflammation: A case study in human endotoxemia. J. Comput. Phys. 244, 279–289. doi:10.1016/j.jcp.2012.09.024
Seagly, Katharine S., O’Neil, Rochelle L., and Hanks, Robin A. (2018). Pre-injury psychosocial and demographic predictors of long-term functional outcomes post-TBI. Brain Inj. 32 (1), 78–83. doi:10.1080/02699052.2017.1374467
Siokou, Christine, Morgan, Rebecca, and Shiell, Alan (2014). Group model building: A participatory approach to understanding and acting on systems. Public Health Res. Pract. 25 (1), e2511404. doi:10.17061/phrp2511404
Sodders, Mark D., Killien, Y., Lynn, G., Stansbury, M., Vavilala, S., Moore., Megan, et al. (2020). Race/ethnicity and informal caregiver burden after traumatic brain injury: A scoping study. Health Equity 4 (1), 304–315. doi:10.1089/heq.2020.0007
Sterman, John (2000). Business dynamics: Systems thinking and modeling for a complex world. New York: Irwin/McGraw-Hill.
Ursino, Mauro, and Alberto Lodi, Carlo (1997). A simple mathematical model of the interaction between intracranial pressure and cerebral hemodynamics. J. Appl. Physiology 82, 1256–1269. doi:10.1152/jappl.1997.82.4.1256
Valcourt, Nicholas, Walters, Jeffrey, Amy, Javernick‐Will, and Linden, Karl (2020). Assessing the efficacy of group model building workshops in an applied setting through purposive text analysis. Syst. Dyn. Rev. 36 (2), 135–157. doi:10.1002/sdr.1657
Vaughan, Leah E., Prerna, R., Ranganathan, Raj G. Kumar, Amy K. Wagner, Rubin, Jonathan E., and Rubin, J. E. (2018). A mathematical model of neuroinflammation in severe clinical traumatic brain injury. J. Neuroinflammation 15 (1), 345. doi:10.1186/s12974-018-1384-1
Vennix, Jac A. M. (1999). Group model-building: Tackling messy problems. Syst. Dyn. Rev. 15 (4), 379–401. doi:10.1002/(sici)1099-1727(199924)15:4<379::aid-sdr179>3.0.co;2-e
Vogel, M., Gillani, B., Hovmand, P., Proctor, E., and Soto, J. P. (2019). Implementing evidence-based guidelines for severe pediatric traumatic brain injury: A system dynamics approach. Washington: AcademyHealth.
Wakeland, Wayne, Agbeko, Rachel, Vinecore, Kevin, Peters, Mark, and Goldstein, Brahm (2009). Assessing the prediction potential of an in silico computer model of intracranial pressure dynamics. Crit. Care Med. 37 (3), 1079–1089. doi:10.1097/CCM.0b013e31819b629d
Wakeland, Wayne, and Goldstein., Brahm (2008). A review of physiological simulation models of intracranial pressure dynamics. Comput. Biol. Med. 38 (9), 1024–1041. doi:10.1016/j.compbiomed.2008.07.004
Wakeland, Wayne, and Kenzie, Erin (2019). A computational model for recovery from traumatic brain injury. Proceedings of the 63rd Annual Meeting of the ISSS - 2019 Corvallis, OR, USA, NovemberAvailable at: https://journals.isss.org/index.php/proceedings63rd/article/view/3639.
Wheble, Joanna L. C., and Menon, D. K. (2016). TBI—the most complex disease in the most complex organ: The CENTER-TBI trial—a commentary: Table 1. J. R. Army Med. Corps 162 (2), 87–89. doi:10.1136/jramc-2015-000472
Yue, John K., Vassar, Mary J., Cooper, R., Cooper, S. R., Okonkwo, D. O., Valadka, A. B., et al. (2013). Transforming research and clinical knowledge in traumatic brain injury pilot: Multicenter implementation of the common data elements for traumatic brain injury. J. Neurotrauma 30 (22), 1831–1844. doi:10.1089/neu.2013.2970
Keywords: traumatic brain injury, system dynamics, modeling, systems science, complexity, simulation
Citation: Kenzie ES, Parks EL, Carney N and Wakeland W (2022) System dynamics modeling for traumatic brain injury: Mini-review of applications. Front. Bioeng. Biotechnol. 10:854358. doi: 10.3389/fbioe.2022.854358
Received: 13 January 2022; Accepted: 05 July 2022;
Published: 12 August 2022.
Edited by:
Michelle LaPlaca, Georgia Institute of Technology, United StatesReviewed by:
Ray Daniel, Independent researcher, Austin, TX, United StatesDulce Oliveira, University of Porto, Portugal
Copyright © 2022 Kenzie, Parks, Carney and Wakeland. This is an open-access article distributed under the terms of the Creative Commons Attribution License (CC BY). The use, distribution or reproduction in other forums is permitted, provided the original author(s) and the copyright owner(s) are credited and that the original publication in this journal is cited, in accordance with accepted academic practice. No use, distribution or reproduction is permitted which does not comply with these terms.
*Correspondence: Erin S. Kenzie, kenzie@ohsu.edu