- 1College of Environmental Sciences and Engineering, Peking University, Beijing, China
- 2Key Laboratory of Water and Sediment Sciences, Ministry of Education, Peking University, Beijing, China
Algal–bacterial water treatment is more effective for better harvesting and promotes energy savings than other traditional treatments, while the relationships between them are multifarious. Among all the interactions, quorum sensing plays an essential ecological role. However, the relative contributions of signaling in the interaction between algae and bacteria are not clear. To elucidate the role of quorum sensing by indole-3-acetic acid (IAA) in terms of the algal–bacterial interaction during the nitrogen removal process, the bioreactors, respectively, inoculated with Chlorella, Phormidium, and both of them were started. We manifest the existence of multiple signaling-related proteins by alignment with the constructed database, and the signaling was analyzed using metagenomic sequence data obtained during bioreactor operation. We found that IAA was mainly synthetized depending on indole-3-acetamide (IAM) and indole-3-pyruvic acid (IPA) pathways by calculating the gene abundance of IAA synthetase. Both Chlorella and the co-culture reactor possessed higher nitrogen removal rate (NRR) than the Phormidium reactor, and the abundance profile of the signaling-related gene is similar with the NRR. The signaling-related gene abundance increased in Chlorella and co-culture reactors but decreased in the Phormidium reactor. Pseudomonas, Hydrogenophaga, and Zoogloea are the dominant signaled bacteria. Chlorella is the dominant signaled algae. The relative abundance of total signaled bacteria in the whole bacterial community increased during the start-up in Chlorella and co-culture reactors. According to the network analysis, phytoplankton prefers to positively correlate with signaled bacteria than non-signaled bacteria, which indicated that the signaling influences the algal–bacterial interaction. These findings hint at the significance of algal–bacterial signaling in this interkingdom interaction during nitrogen removal.
Introduction
Algae-based wastewater treatment has already been extensively used for nitrogen removal (Abeysiriwardana-Arachchige et al., 2020). It has been found that the algal–bacterial interaction influences wastewater treatment. Nevertheless, the interaction between algae and bacteria is still elusive during the water treatment using algae (Ramanan et al., 2016). Treatment systems of algal–bacterial interaction have been widely used for wastewaters with rich nutrients since the 1950s. It was already been proved that bacteria help in the flocculation of algae and would be effective for better harvesting of the algal and bacterial biomass, also reducing the costs (Kim et al., 2014; Lee et al., 2013). Additionally, to effectively treat the dissolved methane in anaerobically treated wastewaters, the synergism between algae and methane-oxidizing bacteria was used, which are otherwise released into the atmosphere in other processes based on aeration (van der Ha et al., 2012). Considering the relatively minuscule utilization of energy, treatment efficiency, and biomass production which could be valorized, the algal–bacterial water treatment process could be the main alternative technology to aeration-based technologies such as activated sludge treatment (Kim et al., 2014; Ramanan et al., 2016). The conservative estimate shows that treatment with the algal–bacterial process can trigger 100-fold energy savings than traditional activated sludge treatment (Kang et al., 2015). Nevertheless, the attached systems with biofilms are more sophisticated compared to the systems of suspended culture, as systematic investigations on the fundamental processes are less understood, and studies on the role of algal–bacterial interactions, especially in the engineered membrane systems for treating sewage, are sparse (Kesaano and Sims, 2014).
Increasing attention has been paid to the interaction between algae and bacteria. It is the fundamental ecological relationship in water environments by regulating biomass production and nutrient cycling at the base of the aquatic food web (Seymour et al., 2017). Algal–bacterial interactions are diverse and often highly complex, from cooperative to competitive (Amin et al., 2012; Durham et al., 2015). The relationship between algae and bacteria is based on resource provision, and it can be either exploitative or reciprocal in an aquatic environment at the simplest level (Cole, 1982). Heterotrophic bacteria in a water environment obtain a large fraction of their carbon demand directly from phytoplankton. More than 50% of the carbon fixed by algae is ultimately consumed by heterotrophic bacteria (Fouilland et al., 2014; Fuhrman and Azam, 1982). Algal cells release large quantities of dissolved and labile organic carbon into the surrounding water, and bacteria derive organic material from algae primarily depending on assimilation (Biddanda and Pomeroy, 1988; Larsson and Hagstrom, 1979; Piontek et al., 2011; Teeling et al., 2012). From the perspective of an algal cell, bacteria can be competitors for inorganic nutrients, but they also provide the limiting macronutrients to algae by remineralization (Joint et al., 2002; Legendre and Rassoulzadegan, 1995). When the nutrient supply is limited, algal growth is particularly benefited from the bacterial delivery of the generated phosphorus and nitrogen (Amin et al., 2009; Croft et al., 2005; Falkowski, 1994). According to previous investigations, algae-associated bacterial communities mainly contain members of Alteromonadaceae, Flavobacteraceae, and Roseobacter clade (Amin et al., 2012; Buchan et al., 2014; Goecke et al., 2013; Green et al., 2015; Guannel et al., 2011; Rooney-Varga et al., 2005; Teeling et al., 2012; van Tol et al., 2017; Zhou et al., 2020). Additionally, algal–bacterial interactions involve the exchange of multifarious substances such as growth resources, nutrients, and infochemicals. The metabolism and sensing of these chemical currencies underpin the interaction between the algae and bacteria, spanning competition, antagonism, commensalism, and obligate mutualism (Mayali and Azam, 2004; Windler et al., 2014; Seymour et al., 2017).
Among all the interactions between algae and bacteria, interkingdom signaling is the language via chemical signals and plays an ecological role (Zhou et al., 2016; Cirri and Pohnert, 2019). The bacterial consortium associated with a globally distributed diatom has already been unraveled (Amin et al., 2015). The result showed that cell division of eukaryotic phytoplankton was promoted via the exchange of the auxin indole-3-acetic acid (IAA) from Sulfitobacter. The algal cyclin was regulated by bacterial IAA during the quorum sensing. Thereby, the results verified that the algal–bacterial interaction is regulated by the production and secretion of infochemicals. Generally, the bacterial synthesis of IAA contains three different pathways; they are, respectively, the indole-3-acetamide (IAM) pathway, indole-3-pyruvic acid (IPA) pathway, and indole-acetaldoxime/indole-3-acetonitrile (IAOx/IAN) pathway (Spaepen et al., 2007; Duca et al., 2014; Amin et al., 2015). In the IAM pathway, indoleacetamide hydrolase is the key enzyme which catalyzed the last step from indoleacetamide to IAA. In the IPA pathway, indoleacetaldehyde dehydrogenase is the key enzyme which catalyzed the last step from indoleacetaldehyde to IAA. In the IAOx/IAN pathway, indoleacetonitrile nitrilase is the key enzyme which catalyzed the last step from indoleacetonitrile to IAA. However, the role of interkingdom signaling in the algal–bacterial interaction needs to be more investigated, and the relative importance of quorum sensing is not clearly elucidated.
To explore the quorum sensing between phytoplankton and bacteria during the nitrogen removal process, bioreactors, respectively, inoculated with Chlorella, Phormidium, and both of them were started. The abundance of IAA-related proteins and the community structure of signaled bacteria and algae are analyzed by metagenomic sequencing. Additionally, the algal–bacterial interactions are also evaluated by a co-occurrence network. The results offer deeper insights into the signaling between phytoplankton and bacteria during the photo-bioreactor start-up process and hint at the relative importance of algal–bacterial signaling in the interactions.
Materials and Methods
Bioreactor Operation
Three sequence membrane photo-bioreactors (SMPBRs) were started up for the experiment; the first two reactors were inoculated with single algae Chlorella and Phormidium, and the other reactor was employed as a co-culture for the two algae with inoculation ratios of 1:1 according to dry weight. The initial biomass concentration in the three reactors was adjusted to 200 mg/L prior to the start of the experiments. The microalgae strains Chlorella sp. (FACHB-31) and Phordium sp. (FACHB-1129) were purchased from the Freshwater Algae Culture Collection at the Institute of Hydrobiology, Wuhan, China. The experimental conditions for the continuous operation of different phases are shown in Table 1. To evaluate the nitrogen removal rate (NRR) during the start-up, ammonium (NH4+-N), nitrite (NO2−-N), and nitrate (NO3−-N) were determined according to the APHA Standard Methods (APHA, 2005).
The sequence membrane photo-bioreactors (SMPBR) had a working volume of 3 L with dimensions of 0.16 m, 0.16 m, and 0.2 m (length, width, and height, respectively). A hollow fiber membrane module made of polyvinylidene fluoride (PVDF) with a nominal pore size of 0.45 μm, and a total effective filtration area of 0.1 m2, was directly submerged in the bioreactor. The magnetic stir was employed at 300 rpm to homogenize the liquid and avoid biofilm formation. An LED light was twined around the surface of the reactor, and a fixed 12 h dark/12 h light cycle was applied on a daily basis with a light intensity of 6,000 lux at the surface of the algal mixed liquor during light exposure. The pH in the reactor was controlled in the range of 7.5 ± 0.3 by NaOH and HCl solution. All the reactors were operated under a batch and semi-continuous mode, and the SMPBR cycle consists of the following phases: feeding (30 min), mixing (23 h), and withdrawal and filtration (30 min). The algal biomass was retained by the membrane and was weekly harvested. One-third of the mixed liquor (1 L) was directly discharged every 7 days, in a biomass retention time (BRT) of 21 days.
The reactors were fed with synthetic low C/N ratio wastewater and were operated in four phases. In phase I (acclimatization phase, days 1–14), the ammonium nitrogen was gradually increased from 30 mg/L to 60 mg/L to acclimate the algae, and the COD was 180 ± 5 mg/L. In phase II (days 15–42), the concentration of NH4+-N and COD was maintained at 60 ± 5 and 180 ± 5 mg/L, respectively. In phase III (days 43–70), the C/N ratio was decreased from 3:1 to 1:1, and the concentrations of NH4+-N and COD were both kept at 70 ± 5 mg/L. Other contents of the synergetic wastewater were constant in all phases, with 6.5 ± 0.5 mg/L total phosphorus (TP), in the form of PO4−-P, 60 mg/L NaHCO3, 20 mg/L MgSO4, 10 mg/L CaCl2, and 1 ml trace solution.
Metagenomic Sequencing
The biological samples were collected after 42 and 70 days, and 200 mg of sample in each reactor was centrifuged at 8,000 rpm for 5 min. The DNA extraction and metagenome sequencing were conducted as described in the supplemental material. The clean reads were classified using Kaiju to explore the microbiota compositions. The Kaiju was run against the nr_euk database (built in May 2020) using default parameters (Menzel et al., 2016).
Dataset Construction
The procedure for the construction of the sequence datasets related to IAA is performed as previously described in the construction for the bacterial communication gene (Tang et al., 2018). To explore the signaled bacterial community in a bioreactor culture, three identified proteins related to IAA synthetase of bacteria were selected (Amin et al., 2015; Duca et al., 2014; Spaepen et al., 2007). They are the last-step enzymes in three different pathways: indoleacetamide hydrolase, indoleacetaldehyde dehydrogenase, and indoleacetonitrile nitrilase. Furthermore, the algal cyclin regulated by IAA was also selected (Amin et al., 2015). We retrieved all available sequences from a non-redundant (November 2021) database using the NCBI search tool and Swiss-Prot of the UniProt database (November 2021). Each subtype of IAA and cyclin (Supplementary Tables S1, S2) was examined to obtain a clean version of the datasets by checking annotation, discarding redundant and partial sequences (Fang et al., 2014). Non-redundant sequences were picked by cd-hit at a 97% similarity (Li and Godzik, 2006; Yang et al., 2016).
Screening for Functional Genes in Reactors
The detection of bacterial IAA synthetase and algal cyclin from the metagenomic sequencing data of all samples was conducted by sequence homology alignments using the BLASTX algorithm with an E-value ≤10–5. If the best hit of a BCG read had >50% sequence identity and the alignment length was >25 amino acids, the read was annotated as an IAA synthetase-like read and algal cyclin-like read. To avoid bias, the abundance of bacterial IAA synthetase and algal cyclin was calculated by normalizing the reference sequence length. Therefore, the abundance of bacterial IAA synthetase and algal cyclin was calculated using the method of Reads Per Kilobase per Million mapped reads (RPKM) as previously described (Tang et al., 2018).
Taxonomic Annotation
To identify the bacterial hosts of the IAA synthetase-like reads and algal hosts of cyclin reads, all of the aligned reads were searched against the NCBI NR database using BLASTX algorithm. The top ten hits with E-values≤1 × 10–5 were imported to MEGAN (MEtaGenome ANalyzer, Version 6). Then, MEGAN was used to explore the taxonomical origin of the dataset, employing the NCBI taxonomy (January 2021) to summarize the results. The lowest common ancestor (LCA) parameters were set at a min score of 50, top percent of 10.0, and min support of 0.01 (Huson et al., 2016; Huson and Weber, 2013). Potentially missed assignments were manually corrected. The reads were only classified at the genus level to ensure accuracy.
Network Analysis Between Bacteria and Phytoplankton
To analyze the interaction between bacteria and phytoplankton, Spearman’s correlation coefficients were calculated. Correlations with ρ > 0.6 and p value < 0.01 were considered significant. In addition, the network was conducted and analyzed by Cytoscape (Shannon et al., 2003) to visualize the significant correlations between bacteria and phytoplankton.
Results
Nitrogen Removal Rates of Different Reactors
Both Chlorella and co-culture reactors possessed higher nitrogen removal rate (NRR) value than the Phormidium reactor (Figure 1) During the whole period of the start-up, the NRR value of the Chlorella reactor was 10.85 ± 2.80 mg-N·L−1·d−1, the NRR value of the Phormidium reactor was 7.82 ± 3.80 mg-N·L−1·d−1, and the NRR value of the co-culture reactor was 12.05 ± 4.25 mg-N·L−1·d−1. In three different phases, the NRR values of Chlorella and co-culture reactors are invariably significantly higher than those of the other Phormidium reactor, respectively (t test, p value <0.05).
Gene Abundance of Different IAA Synthetase Subtypes
The RPKM values of indoleacetamide hydrolase and indoleacetaldehyde dehydrogenase are significantly higher than those of indoleacetonitrile nitrilase in all samples (p value <0.05). In detail, the gene abundance of indoleacetamide hydrolase is 1,540.824 ± 651.879 RPKM in all the samples. The gene abundance of indoleacetaldehyde dehydrogenase is 3,350.513 ± 1976.065 RPKM in all the samples. Especially, the gene abundance of indoleacetonitrile nitrilase is merely 10.760 ± 13.169 RPKM in all the samples (Figure 2).
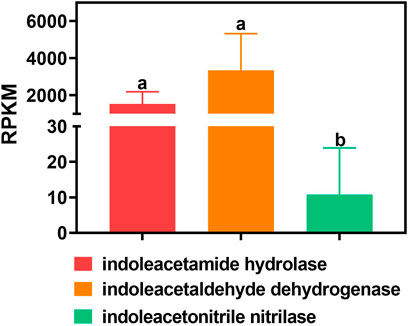
FIGURE 2. Gene abundance of different bacterial IAA synthetases in all samples. One-way ANOVA was used to analyze variations among all the treatments. Different small letters indicate significant differences at the p < 0.05 level.
Change Trends and Profiles of IAA-Related Protein in the Photo-Bioreactors
The change tendency of IAA synthetase is different between that of the photo-bioreactor inoculated with eukaryotic Chlorella and prokaryotic Phormidium. In the Chlorella and co-culture reactors, the gene abundance of indoleacetamide hydrolase, indoleacetaldehyde dehydrogenase, and indoleacetonitrile nitrilase all increased from day 42 to day 70 during the start-up (Figures 3, 4). In the Phormidium reactor, the gene abundance of these IAA synthetases all decreased from day 42 to day 70 during the start-up (Figure 5).
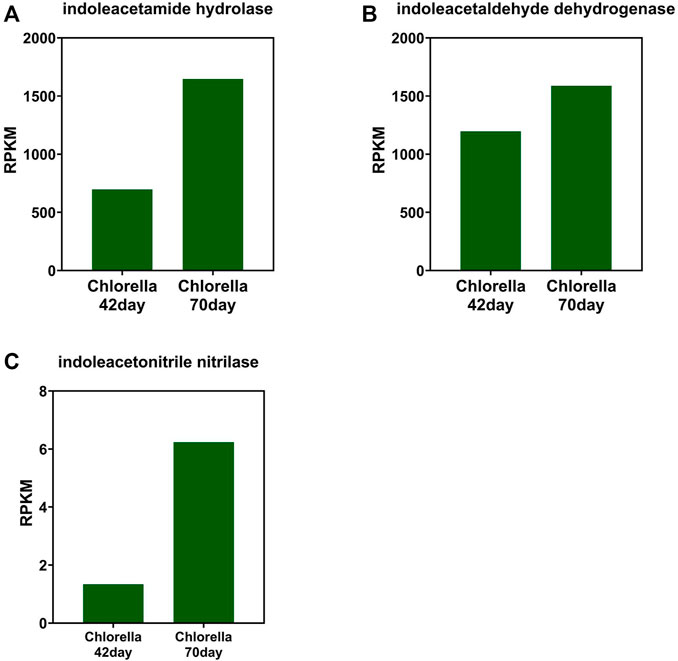
FIGURE 3. Gene abundance of bacterial IAA synthetase in the Chlorella reactor. (A) Indoleacetamide hydrolase, (B) indoleacetaldehyde dehydrogenase, and (C) indoleacetonitrile nitrilase.
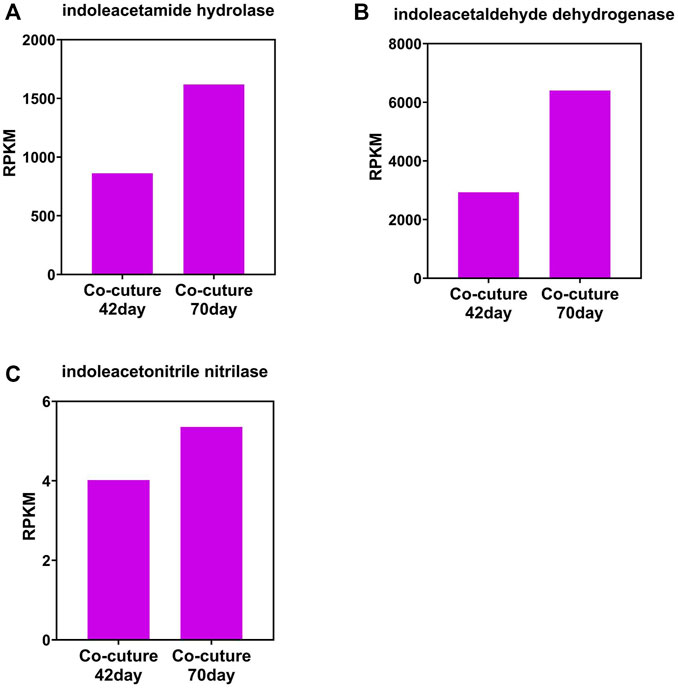
FIGURE 4. Gene abundance of bacterial IAA synthetase in the co-culture reactor. (A) Indoleacetamide hydrolase, (B) indoleacetaldehyde dehydrogenase, and (C) indoleacetonitrile nitrilase.
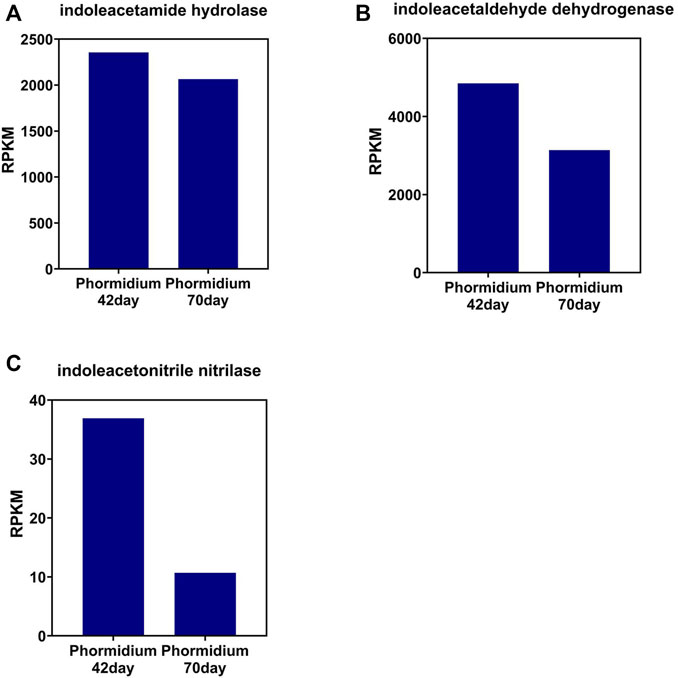
FIGURE 5. Gene abundance of bacterial IAA synthetase in the Phormidium reactor. (A) Indoleacetamide hydrolase, (B) indoleacetaldehyde dehydrogenase, and (C) indoleacetonitrile nitrilase.
The change tendency of cyclin is also different in that of the three kinds of reactors. In the Chlorella reactor, the gene abundance of cyclin increased from day 42 to day 70 during the start-up (Figure 6). In the Phormidium and co-culture reactors, the gene abundance of cyclin decreased from day 42 to day 70 during the start-up. Additionally, the abundance of algal cyclin in Chlorella and co-culture reactors is higher than that of the Phormidium reactor. In detail, the gene abundance of cyclin in the Chlorella reactor is 3,241.20 RPKM and 4,369.73 RPKM (day 42 and day 70, respectively). The gene abundance of cyclin in the co-culture reactor is 1752.07 RPKM and 138.84 RPKM (day 42 and day 70). Nevertheless, the gene abundance of cyclin in the Phormidium reactor is merely 88.73 and 9.77 (day 42 and day 70).
Community Structure of the Signaled Bacteria and Phytoplankton
The bacteria carrying IAA synthetase are the signaled bacteria in the different algal reactors (Figure 7). The relative abundance of the total signaled bacteria in the whole bacterial community increased during the start-up in Chlorella and co-culture reactors but decreased in the Phormidium reactor (Figure 7A). Hydrogenophaga, Zoogloea, Bradyrhizobium, and Thauera are the dominant signaled bacteria carrying indoleacetamide hydrolase. Pseudomonas is the only signaled bacterium carrying indoleacetaldehyde dehydrogenase in different reactors. Nevertheless, the bacteria carrying indoleacetonitrile nitrilase are unclassified in all reactors (Figure 7B).
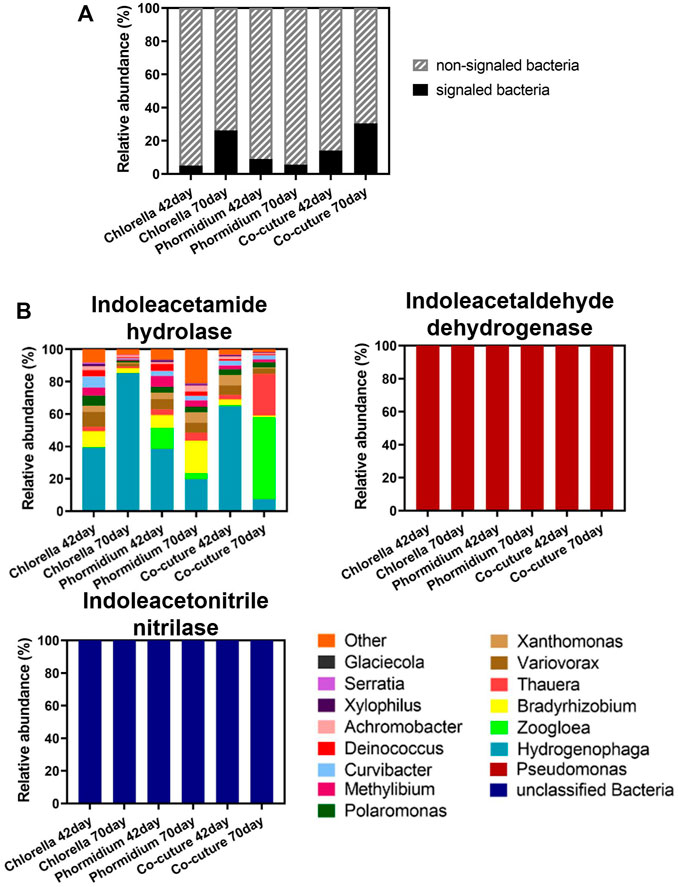
FIGURE 7. Community structure of signaled bacteria. (A) Subcommunity of signaled bacteria carrying IAA synthetase. (B) The relative abundance of signaled bacteria carrying IAA synthetase in the bacterial community.
The algae carrying cyclin protein are the signaled algae in the different algal reactors (Figure 8). The signaled phytoplankton possess high abundance in Chlorella reactors and co-culture reactors in the total community (algae and bacteria), and Chlorella is the dominant signaled phytoplankton carrying cyclin in these samples. Conversely, the signaled algae possess an extremely low abundance in the Phormidium reactor in the total community (algae and bacteria). Phaeodactylum, Auxenochlorella, Thalassiosira, Symbiodinium, and Fragilariopsisare are the indigenous algal genera from influent water.
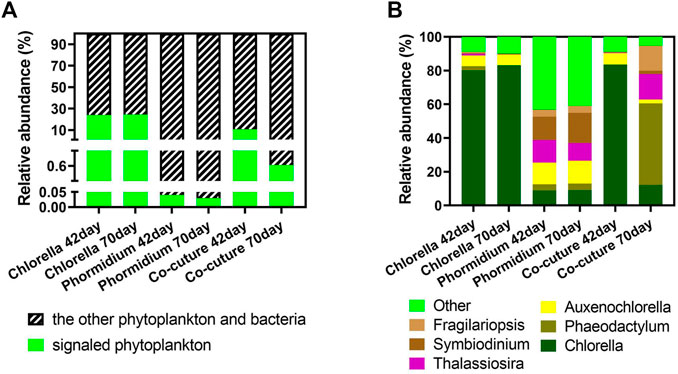
FIGURE 8. Community structure of signaled phytoplankton. (A) Subcommunity of signaled phytoplankton carrying cyclin. (B) The relative abundance of signaled phytoplankton carrying cyclin in the whole community.
Co-Occurrence Network Between Bacteria and Phytoplankton
Since the bacterial IAA only influences the reactors inoculated with eukaryotic Chlorella, the network analysis was performed to elucidate the correlation between bacteria and eukaryotic phytoplankton (Figure 9). The density, heterogeneity, and centralization value of the network between signaled bacteria and phytoplankton are higher than those of the network between non-signaled bacteria and phytoplankton (Table 2). The significant correlations between signaled bacteria and Chlorella are all positive, and the signaled bacteria contain Serratia, Hydrogenophaga, Polaromonas, Curvibactera, and Variovorax. The significant correlations between non-signaled bacteria and Chlorella are all negative, and the non-signaled bacteria contain Bosea, Rhizobium, and Hyphomicrobium.
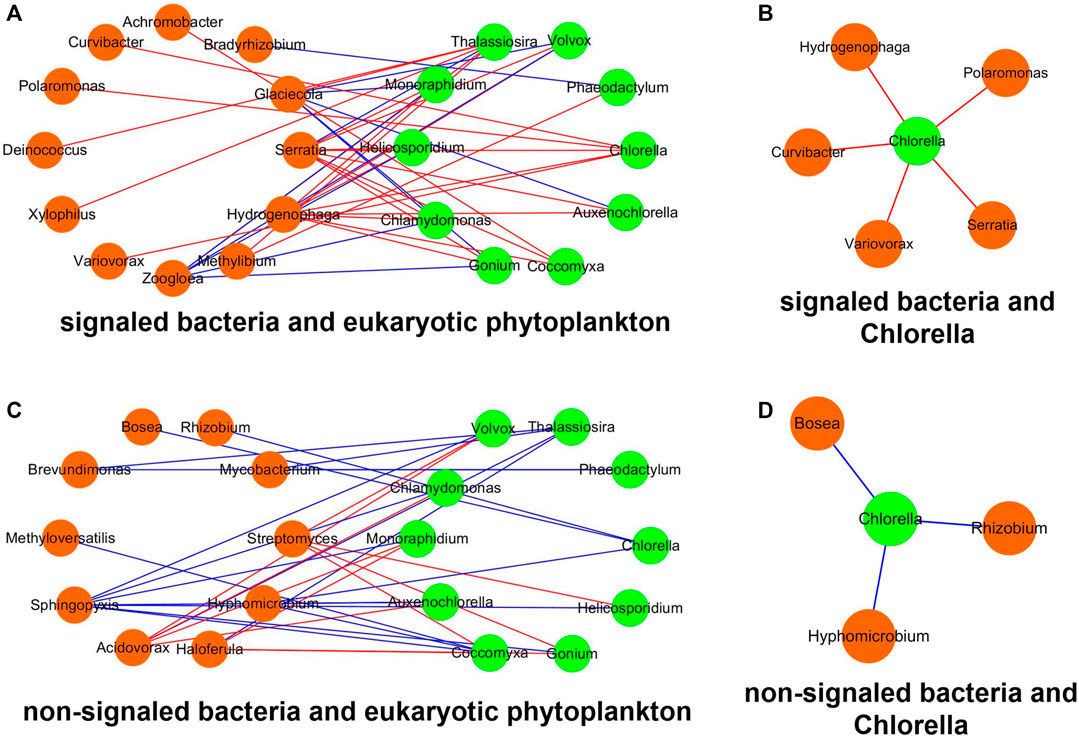
FIGURE 9. Network between bacteria and phytoplankton. (A) Signaled bacteria and eukaryotic phytoplankton, (B) non-signaled bacteria and eukaryotic phytoplankton, (C) signaled bacteria and Chlorella, and (D) non- signaled bacteria and Chlorella. Orange nodes represent bacteria, and green nodes represent phytoplankton. Red lines represent positive correlation, and blue lines represent negative correlation.
The Relative Importance of Algal–Bacterial Signaling in the Interaction
To figure out the relative importance of algal–bacterial signaling in the interactions, the network only using significantly positive correlations between bacteria and phytoplankton was analyzed (Figure 10). The network between signaled bacteria and phytoplankton possesses 26 pairs of significantly positive correlations, which is more than that of the network between non-signaled bacteria and phytoplankton (11 pairs). The signaling by IAA accounted for 70.27% positive correlations in the network between phytoplankton and bacteria. The positively signaled bacteria contain Serratia, Hydrogenophaga, Methylibium, Zoogloea, Variovorax, Xylophilus, Deinococcus, Polaromonas, Curvibacter, Achromobacter, and Glaciecola. The positively non-signaled bacteria contain Streptomyces, Acidovorax, and Haloferula (Figure 10).
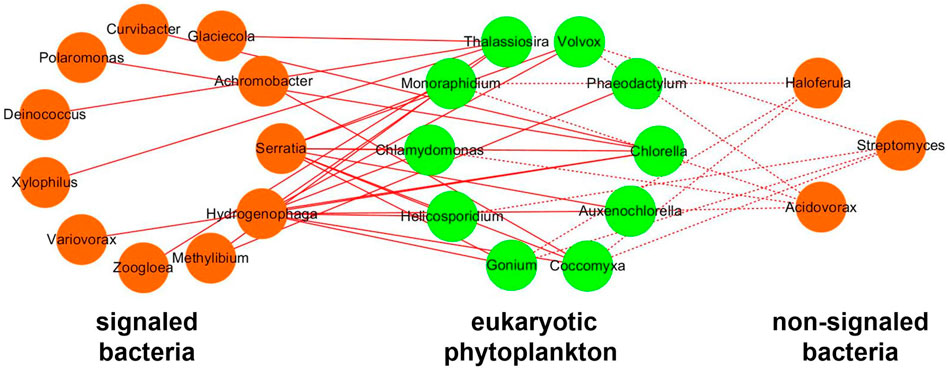
FIGURE 10. Network of positive correlation between bacteria and eukaryotic phytoplankton. Orange nodes represent bacteria, and green nodes represent phytoplankton. Solid lines represent positive correlation between signaled bacteria and phytoplankton, and dotted lines represent positive correlation between non-signaled bacteria and phytoplankton.
Discussion
Key Role of Quorum Sensing in the Algal–Bacterial Interaction
Overall, phytoplankton prefer to positively correlate with signaled bacteria carrying IAA synthetase than non-signaled bacteria in algal reactors, which indicated that the signaling influences the algal–bacterial interaction during nitrogen removal. Particularly, the signaling between phytoplankton and bacteria accounts for 70.27% positive interaction (Figure 10), which indicates that quorum sensing might be the most crucial factor in algal–bacterial interactions in algal reactors. This finding is beneficial for understanding the mechanism of algal–bacterial interaction during water treatment using algae.
Previous research implied that algal–bacterial interaction in the phycosphere is similar with plaint–bacterial interaction in the rhizosphere (Ramanan et al., 2016; Seymour et al., 2017). The phycosphere of algal reactors represents the region surrounding a phytoplankton cell in water. Bacteria secreting IAA to the root can easily associate with plants in a soil environment (Duca et al., 2014; Philippot et al., 2013; Spaepen et al., 2007). Here, we found that signaled bacteria are more inclined to positively correlate with phytoplankton, which supports the perspective that the phycosphere is similar with the rhizosphere (Seymour et al., 2017).
IAA Enhanced Nitrogen Removal by Promoting Biological Activity
The change tendency of IAA-related protein abundance is consistent with the nitrogen removal rate (NRR). The abundance of IAA synthetase increased during the start-up in Chlorella and co-culture bioreactors but decreased during the start-up in Phormidium bioreactors. The abundance of algal cyclin in Chlorella and co-culture reactors is also higher than that of the Phormidium reactor. Similarly, the NRR in Chlorella and co-culture reactors is higher than the NRR in the Phormidium reactor. These results imply that IAA might aggravate nitrogen removal. The bacterial IAA regulates algal cyclin after being secreted to algae, and the algal cyclin regulates cell proliferation as previously described (Amin et al., 2015; Seymour et al., 2017). Then, the biomass and activity of phytoplankton are promoted by the exchange of IAA, which might intensify the nitrogen removal efficiency in the reactors.
The main pathways of IAA synthetase during the nitrogen removal process are the IAM and IPA pathways, according to the abundance of different types of synthetase. This finding is supported by the previous research that the IAOx/IAN pathway is not the main pathway (Duca et al., 2014; Spaepen et al., 2007). Interestingly, the abundance of IAA synthetase and cyclin in different reactors implies that IAA only promotes the algal cyclin in the eukaryotic Chlorella reactor rather than the prokaryotic Phormidium reactor, which is also consistent with previous research that IAA regulates the growth of eukaryotic plants as the auxin (Duca et al., 2014; Philippot et al., 2013; Spaepen et al., 2007).
Signaled Bacteria Carrying IAA Synthetase
The relative abundance of the total signaled bacteria in Chlorella and co-culture reactors is higher than that in the Phormidium reactor. The change trend is also similar with the NRR, which implied the signaled bacteria are also important for biological activity during nitrogen removal. Pseudomonas, Hydrogenophaga, and Zoogloea are the dominant signaled bacteria carrying IAA synthetase in all the reactors, and they secrete IAA into phytoplankton like rhizosphere bacteria in the soil. For instance, Pseudomonas provides IAA for the plant root in the soil environment (Duca et al., 2014; Spaepen et al., 2007). This is also the evidence to demonstrate that the phycosphere is similar with the rhizosphere in the soil environment which is consistent with previous research (Ramanan et al., 2016; Seymour et al., 2017). Therefore, the addition of IAA producers into algal reactors might also enhance the nitrogen removal in future studies, and it can be used in future studies, which is helpful for planning strategies of water treatment.
Conclusion
Both Chlorella and co-culture reactors possessed higher nitrogen removal efficiency than the Phormidium reactor. The signaling dominates the algal–bacterial interaction during nitrogen removal. Quorum sensing by indole-3-acetic acid (IAA) accounts for 70.27% positive interaction between phytoplankton and bacteria. IAA was mainly synthetized depending on the indole-3-acetamide (IAM) and indole-3-pyruvic acid (IPA) pathways, and it only regulates eukaryotic phytoplankton rather than prokaryotic cyanophyta. The relative abundance of the total signaled bacteria in the whole bacterial community increased during the start-up in Chlorella and co-culture reactors with higher nitrogen removal rates. The signaling between phytoplankton and bacteria carrying IAA synthetase such as Pseudomonas, Hydrogenophaga, and Zoogloea promotes the biological activity in sequence membrane photo-bioreactors, which can enhance the nitrogen removal efficiency.
Data Availability Statement
The metagenomic sequences were submitted to the GenBank Sequence Read Archive (SRA) database in the National Center for Biotechnology Information (NCBI) under the accession number SRP354739.
Author Contributions
XW, LK, and SL contributed to conception and designed the study. XW and LK performed experiments and generated the data. XW analyzed the data with assistance from LK, YF, and JP. XW wrote the first draft of the manuscript. XW and SL led the writing of the manuscript.
Funding
This work was supported by the National Natural Science Foundation of China (Nos. 51878008 and 51922016).
Conflict of Interest
The authors declare that the research was conducted in the absence of any commercial or financial relationships that could be construed as a potential conflict of interest.
Publisher’s Note
All claims expressed in this article are solely those of the authors and do not necessarily represent those of their affiliated organizations, or those of the publisher, the editors, and the reviewers. Any product that may be evaluated in this article, or claim that may be made by its manufacturer, is not guaranteed or endorsed by the publisher.
Supplementary Material
The Supplementary Material for this article can be found online at: https://www.frontiersin.org/articles/10.3389/fbioe.2022.851376/full#supplementary-material
References
Abeysiriwardana-Arachchige, I. S. A., Munasinghe-Arachchige, S. P., Delanka-Pedige, H. M. K., and Nirmalakhandan, N. (2020). Removal and Recovery of Nutrients from Municipal Sewage: Algal vs. Conventional Approaches. Water Res. 175, 115709. doi:10.1016/j.watres.2020.115709
Amin, S. A., Green, D. H., Hart, M. C., Kupper, F. C., Sunda, W. G., and Carrano, C. J. (2009). Photolysis of Iron-Siderophore Chelates Promotes Bacterial-Algal Mutualism. Proc. Natl. Acad. Sci. 106, 17071–17076. doi:10.1073/pnas.0905512106
Amin, S. A., Hmelo, L. R., van Tol, H. M., Durham, B. P., Carlson, L. T., and Heal, K. R. (2015). Interaction and Signalling between a Cosmopolitan Phytoplankton and Associated Bacteria. Nature 522, 98–101. doi:10.1038/nature14488
Amin, S. A., Parker, M. S., and Armbrust, E. V. (2012). Interactions between Diatoms and Bacteria. Microbiol. Mol. Biol. Rev. 76, 667, 684-+. doi:10.1128/Mmbr.00007-12
APHA (2005). Standard Methods for the Examination of Water and Wastewater. 21th ed. Washington, DC: American Public Health Association.
Biddanda, B., and Pomeroy, L. (1988). Microbial Aggregation and Degradation of Phytoplankton-Derived Detritus in Seawater. I. Microbial Succession. Mar. Ecol. Prog. Ser. 42, 79–88. doi:10.3354/Meps042079
Buchan, A., LeCleir, G. R., Gulvik, C. A., and González, J. M. (2014). Master Recyclers: Features and Functions of Bacteria Associated with Phytoplankton Blooms. Nat. Rev. Microbiol. 12, 686–698. doi:10.1038/nrmicro3326
Cirri, E., and Pohnert, G. (2019). Algae−bacteria Interactions that Balance the Planktonic Microbiome. New Phytol. 223, 100–106. doi:10.1111/nph.15765
Cole, J. J. (1982). Interactions between Bacteria and Algae in Aquatic Ecosystems. Annu. Rev. Ecol. Syst. 13, 291–314. doi:10.1146/annurev.es.13.110182.001451
Croft, M. T., Lawrence, A. D., Raux-Deery, E., Warren, M. J., and Smith, A. G. (2005). Algae Acquire Vitamin B12 through a Symbiotic Relationship with Bacteria. Nature 438, 90–93. doi:10.1038/nature04056
Duca, D., Lorv, J., Patten, C. L., Rose, D., and Glick, B. R. (2014). Indole-3-acetic Acid in Plant-Microbe Interactions. Antonie van Leeuwenhoek 106, 85–125. doi:10.1007/s10482-013-0095-y
Durham, B. P., Sharma, S., Luo, H., Smith, C. B., Amin, S. A., and Bender, S. J. (2015). Cryptic Carbon and Sulfur Cycling between Surface Ocean Plankton. Proc. Natl. Acad. Sci. USA 112, 453–457. doi:10.1073/pnas.1413137112
Falkowski, P. G. (1994). The Role of Phytoplankton Photosynthesis in Global Biogeochemical Cycles. Photosynth Res. 39, 235–258. doi:10.1007/Bf00014586
Fang, H., Cai, L., Yang, Y., Ju, F., Li, X., and Yu, Y. (2014). Metagenomic Analysis Reveals Potential Biodegradation Pathways of Persistent Pesticides in Freshwater and marine Sediments. Sci. Total Environ. 470-471, 983–992. doi:10.1016/j.scitotenv.2013.10.076
Fouilland, E., Tolosa, I., Bonnet, D., Bouvier, C., Bouvier, T., and Bouvy, M. (2014). Bacterial Carbon Dependence on Freshly Produced Phytoplankton Exudates under Different Nutrient Availability and Grazing Pressure Conditions in Coastal marine Waters. FEMS Microbiol. Ecol. 87, 757–769. doi:10.1111/1574-6941.12262
Fuhrman, J. A., and Azam, F. (1982). Thymidine Incorporation as a Measure of Heterotrophic Bacterioplankton Production in marine Surface Waters: Evaluation and Field Results. Mar. Biol. 66, 109–120. doi:10.1007/Bf00397184
Goecke, F., Thiel, V., Wiese, J., Labes, A., and Imhoff, J. F. (2013). Algae as an Important Environment for Bacteria - Phylogenetic Relationships Among New Bacterial Species Isolated from Algae. Phycologia 52, 14–24. doi:10.2216/12-24.1
Green, D. H., Echavarri-Bravo, V., Brennan, D., and Hart, M. C. (20152015). Bacterial Diversity Associated with the Coccolithophorid AlgaeEmiliania huxleyiandCoccolithus pelagicusf.Braarudii. Biomed. Res. Int. 2015, 1–15. doi:10.1155/2015/194540
Guannel, M., Horner-Devine, M., and Rocap, G. (2011). Bacterial Community Composition Differs with Species and Toxigenicity of the Diatom Pseudo-nitzschia. Aquat. Microb. Ecol. 64, 117–133. doi:10.3354/ame01513
Huson, D. H., Beier, S., Flade, I., Górska, A., El-Hadidi, M., and Mitra, S. (2016). MEGAN Community Edition - Interactive Exploration and Analysis of Large-Scale Microbiome Sequencing Data. Plos Comput. Biol. 12, e1004957. doi:10.1371/journal.pcbi.1004957
Huson, D. H., and Weber, N. (2013). Microbial Community Analysis Using MEGAN. Microb. Metagenomics, Metatranscriptomics, Metaproteomics 531, 465–485. doi:10.1016/B978-0-12-407863-5.00021-6
Joint, I., Henriksen, P., Fonnes, G., Bourne, D., Thingstad, T., and Riemann, B. (2002). Competition for Inorganic Nutrients between Phytoplankton and Bacterioplankton in Nutrient Manipulated Mesocosms. Aquat. Microb. Ecol. 29, 145–159. doi:10.3354/Ame029145
Kang, Z., Kim, B.-H., Ramanan, R., Choi, J.-E., Yang, J.-W., and Oh, H.-M. (2015). A Cost Analysis of Microalgal Biomass and Biodiesel Production in Open Raceways Treating Municipal Wastewater and under Optimum Light Wavelength. J. Microbiol. Biotechnol. 25, 109–118. doi:10.4014/jmb.1409.09019
Kesaano, M., and Sims, R. C. (2014). Algal Biofilm Based Technology for Wastewater Treatment. Algal Res. 5, 231–240. doi:10.1016/j.algal.2014.02.003
Kim, B.-H., Kang, Z., Ramanan, R., Choi, J.-E., Cho, D.-H., and Oh, H.-M. (2014). Nutrient Removal and Biofuel Production in High Rate Algal Pond Using Real Municipal Wastewater. J. Microbiol. Biotechnol. 24, 1123–1132. doi:10.4014/jmb.1312.12057
Larsson, U., and Hagstrom, A. (1979). Phytoplankton Exudate Release as an Energy Source for the Growth of Pelagic Bacteria. Mar. Biol. 52, 199–206. doi:10.1007/Bf00398133
Lee, J., Cho, D.-H., Ramanan, R., Kim, B.-H., Oh, H.-M., and Kim, H.-S. (2013). Microalgae-associated Bacteria Play a Key Role in the Flocculation of Chlorella Vulgaris. Bioresour. Tech. 131, 195–201. doi:10.1016/j.biortech.2012.11.130
Legendre, L., and Rassoulzadegan, F. (1995). Plankton and Nutrient Dynamics in Marine Waters. Ophelia 41, 153–172. doi:10.1080/00785236.1995.10422042
Li, W., and Godzik, A. (2006). Cd-hit: a Fast Program for Clustering and Comparing Large Sets of Protein or Nucleotide Sequences. Bioinformatics 22, 1658–1659. doi:10.1093/bioinformatics/btl158
Mayali, X., and Azam, F. (2004). Algicidal Bacteria in the Sea and Their Impact on Algal Blooms1. J. Eukaryot. Microbiol. 51, 139–144. doi:10.1111/j.1550-7408.2004.tb00538.x
Menzel, P., Ng, K. L., and Krogh, A. (2016). Fast and Sensitive Taxonomic Classification for Metagenomics with Kaiju. Nat. Commun. 7. doi:10.1038/Ncomms11257
Philippot, L., Raaijmakers, J. M., Lemanceau, P., and van der Putten, W. H. (2013). Going Back to the Roots: the Microbial Ecology of the Rhizosphere. Nat. Rev. Microbiol. 11, 789–799. doi:10.1038/nrmicro3109
Piontek, J., Handel, N., De Bodt, C., Harlay, J., Chou, L., and Engel, A. (2011). The Utilization of Polysaccharides by Heterotrophic Bacterioplankton in the Bay of Biscay (North Atlantic Ocean). J. Plankton Res. 33, 1719–1735. doi:10.1093/plankt/fbr069
Ramanan, R., Kim, B.-H., Cho, D.-H., Oh, H.-M., and Kim, H.-S. (2016). Algae-bacteria Interactions: Evolution, Ecology and Emerging Applications. Biotechnol. Adv. 34, 14–29. doi:10.1016/j.biotechadv.2015.12.003
Rooney-Varga, J. N., Giewat, M. W., Savin, M. C., Sood, S., LeGresley, M., and Martin, J. L. (2005). Links between Phytoplankton and Bacterial Community Dynamics in a Coastal marine Environment. Microb. Ecol. 49, 163–175. doi:10.1007/s00248-003-1057-0
Seymour, J. R., Amin, S. A., Raina, J.-B., and Stocker, R. (2017). Zooming in on the Phycosphere: the Ecological Interface for Phytoplankton-Bacteria Relationships. Nat. Microbiol. 2. doi:10.1038/Nmicrobiol.2017.65
Shannon, P., Markiel, A., Ozier, O., Baliga, N. S., Wang, J. T., and Ramage, D. (2003). Cytoscape: A Software Environment for Integrated Models of Biomolecular Interaction Networks. Genome Res. 13, 2498–2504. doi:10.1101/gr.1239303
Spaepen, S., Vanderleyden, J., and Remans, R. (2007). Indole-3-acetic Acid in Microbial and Microorganism-Plant Signaling. FEMS Microbiol. Rev. 31, 425–448. doi:10.1111/j.1574-6976.2007.00072.x
Tang, X., Guo, Y., Jiang, B., and Liu, S. (2018). Metagenomic Approaches to Understanding Bacterial Communication during the Anammox Reactor Start-Up. Water Res. 136, 95–103. doi:10.1016/j.watres.2018.02.054
Teeling, H., Fuchs, B. M., Becher, D., Klockow, C., Gardebrecht, A., and Bennke, C. M. (2012). Substrate-Controlled Succession of Marine Bacterioplankton Populations Induced by a Phytoplankton Bloom. Science 336, 608–611. doi:10.1126/science.1218344
van der Ha, D., Nachtergaele, L., Kerckhof, F.-M., Rameiyanti, D., Bossier, P., and Verstraete, W. (2012). Conversion of Biogas to Bioproducts by Algae and Methane Oxidizing Bacteria. Environ. Sci. Technol. 46, 13425–13431. doi:10.1021/es303929s
van Tol, H. M., Amin, S. A., and Armbrust, E. V. (2017). Ubiquitous marine Bacterium Inhibits Diatom Cell Division. Isme J. 11, 31–42. doi:10.1038/ismej.2016.112
Windler, M., Bova, D., Kryvenda, A., Straile, D., Gruber, A., and Kroth, P. G. (2014). Influence of Bacteria on Cell Size Development and Morphology of Cultivated Diatoms. Phycol Res. 62, 269–281. doi:10.1111/pre.12059
Yang, Y., Jiang, X., Chai, B., Ma, L., Li, B., and Zhang, A. (2016). ARGs-OAP: Online Analysis Pipeline for Antibiotic Resistance Genes Detection from Metagenomic Data Using an Integrated Structured ARG-Database. Bioinformatics 32, 2346–2351. doi:10.1093/bioinformatics/btw136
Zhou, J., Lyu, Y., Richlen, M. L., Anderson, D. M., and Cai, Z. (2016). Quorum Sensing Is a Language of Chemical Signals and Plays an Ecological Role in Algal-Bacterial Interactions. Crit. Rev. Plant Sci. 35, 81–105. doi:10.1080/07352689.2016.1172461
Keywords: metagenomic, nitrogen removal, signaling, algal–bacterial interaction, indole-3-acetic acid
Citation: Wu X, Kong L, Pan J, Feng Y and Liu S (2022) Metagenomic Approaches to Explore the Quorum Sensing-Mediated Interactions Between Algae and Bacteria in Sequence Membrane Photo-Bioreactors. Front. Bioeng. Biotechnol. 10:851376. doi: 10.3389/fbioe.2022.851376
Received: 09 January 2022; Accepted: 25 February 2022;
Published: 05 April 2022.
Edited by:
Eduardo Jacob-Lopes, Federal University of Santa Maria, BrazilReviewed by:
G. Venkata Subhash, Reliance Industries, IndiaJiangxin Wang, Shenzhen University, China
Copyright © 2022 Wu, Kong, Pan, Feng and Liu. This is an open-access article distributed under the terms of the Creative Commons Attribution License (CC BY). The use, distribution or reproduction in other forums is permitted, provided the original author(s) and the copyright owner(s) are credited and that the original publication in this journal is cited, in accordance with accepted academic practice. No use, distribution or reproduction is permitted which does not comply with these terms.
*Correspondence: Sitong Liu, bGl1c2l0b25nQHBrdS5lZHUuY24=