- 1Julius Wolff Institute, Berlin Institute of Health at Charité—Universitätsmedizin Berlin, Berlin, Germany
- 2Centre des Matériaux, MINES Paris–PSL, Paris, France
- 3Chimie Moléculaire, Macromoléculaire et Matériaux, ESPCI Paris–PSL, Paris, France
- 4Laboratoire Mathématiques and Interactions J. A. Dieudonné, UMR 7351 CNRS, Université Côte d’Azur, Nice, France
Since 5–10% of all bone fractures result in non-healing situations, a thorough understanding of the various bone fracture healing phases is necessary to propose adequate therapeutic strategies. In silico models have greatly contributed to the understanding of the influence of mechanics on tissue formation and resorption during the soft and hard callus phases. However, the late-stage remodeling phase has not been investigated from a mechanobiological viewpoint so far. Here, we propose an in silico multi-tissue evolution model based on mechanical strain accumulation to investigate the mechanobiological regulation of bone remodeling during the late phase of healing. Computer model predictions are compared to histological data of two different pre-clinical studies of bone healing. The model predicted the bone marrow cavity re-opening and the resorption of the external callus. Our results suggest that the local strain accumulation can explain the fracture remodeling process and that this mechanobiological response is conserved among different mammal species. Our study paves the way for further understanding of non-healing situations that could help adapting therapeutic strategies to foster bone healing.
1 Introduction
Although bone usually has the capacity to heal spontaneously upon a given trauma, it is estimated that 5–10% of all fractures result in non-unions (Einhorn, 1995). A thorough understanding of the mechanisms driving the healing process can help to decipher non-healing situations and propose adequate therapeutic strategies. Bone fracture healing is a complex process that involves the coordination of multiple events. Traditionally, the bone healing process is described as following five overlapping stages: initial pro- and anti-inflammatory stages; soft callus formation; gradual mineralization towards a hard callus; and callus remodeling to restore the cortical bone geometry (Schell et al., 2017). The entire sequence of events has also been viewed from a different perspective, and the healing cascade has been proposed to consist of two distinct phases (Schindeler et al., 2008). First, during the anabolic stage, new bone and cartilage is formed, and during the catabolic phase, the cartilage is replaced by bone which is then further remodeled to restore the normal structure.
All these processes are known to be influenced by mechanical signals (Carter et al., 1988; Knecht et al., 2021), where it has been shown that specific strain and stress levels correlate with specific tissue type (bone, cartilage or fibrous tissue) formation or resorption. In silico fracture healing modelling has helped gaining knowledge on the mechanoregulation of the process, i.e., on the specific mechanical environment under which fracture healing takes place and how it drives tissue formation during the soft and hard callus phases (Prendergast et al., 1997; Claes et al., 1998; Lacroix and Prendergast, 2002; Isaksson et al., 2006). However, these bone healing computer models have generally failed at describing or ignored the remodeling stage of the healing, with bone being predicted in the whole fracture gap at the end of the healing period (Lacroix and Prendergast, 2002; Isaksson et al., 2008; Checa et al., 2011; Vetter et al., 2012; Borgiani et al., 2015; Wang and Yang, 2018). To our knowledge, only one model was used to simulate the bone fracture remodeling stage (Byrne et al., 2011), where the restoration of the bone cortices was predicted assuming the removal of the fracture fixation plate. However, remodeling has been observed to happen already in the presence of a fixation plate in vivo (Epari et al., 2006; Kruck et al., 2018; Wehrle et al., 2021).
Using a different approach, some in silico models have investigated bone remodeling as a response to controlled loading or un-loading of the bone (Beaupré et al., 1990; García et al., 2002; Hambli et al., 2011; Schulte et al., 2013). For instance, Doblaré et al., 2002; García et al., 2002 modelled the bone density evolution around hip implants or in a fracture, depending on various fracture fixation devices. They used a bone response model based on damage theory and an instantaneous mechanical stimulus to predict if bone would be formed, resorbed or keep the same density. Isaksson and others explained double cortex formation observed in mouse fracture healing by relating bone formation and resorption to thresholds in the local instantaneous strain energy density (Isaksson et al., 2009). In all studies, no other tissue taking part in the bone healing process (e.g., cartilage, fibrous tissue) was taken into account.
Here, we extended to 3D a previously developed 2D multi-tissue evolution model (Schmitt et al., 2016; Frame et al., 2017, 2018) and applied it to two different experimental set-ups (mouse and sheep long bone fracture healing) to investigate the mechanisms behind experimentally observed fracture remodeling patterns.
2 Materials and Methods
2.1 Computer Model of Tissue Remodeling
The computer model used to investigate tissue remodeling during bone fracture healing has been already described in 2D in the context of intact bone remodeling (Frame et al., 2017). Here, it was extended to 3D and implemented in COMSOL Multiphysics v.5.6 © (COMSOL AB, Sweden). The model consists of a mechanical finite element analysis, to determine the mechanical signals within the healing region, coupled with partial differential equations that simulate tissue evolution as a response to those signals. This model contains a continuous description of the tissue that corresponds to the averaging of all individual cell responses to their local mechanical environment.
The studied bone fracture geometries are discretized into tetrahedral finite elements (FE) for mechanical analysis and tissue evolution simulations: each FE of the callus (healing region) is hypothesized to contain tissue fractions of various types [fibrous tissue (F), cartilage (C), and bone (B)] and maturity [immature (I) and mature (M)], whose evolutions are subject to the rules described in the following sub-sections. The total tissue fraction
2.1.1 Biological Tissue Mechanosensing
The different biological tissue types are hypothesized to respond to the principal strains
Where
With
a Gaussian distribution with p, q, r given in Table 1, ensuring a controlled rate of change for
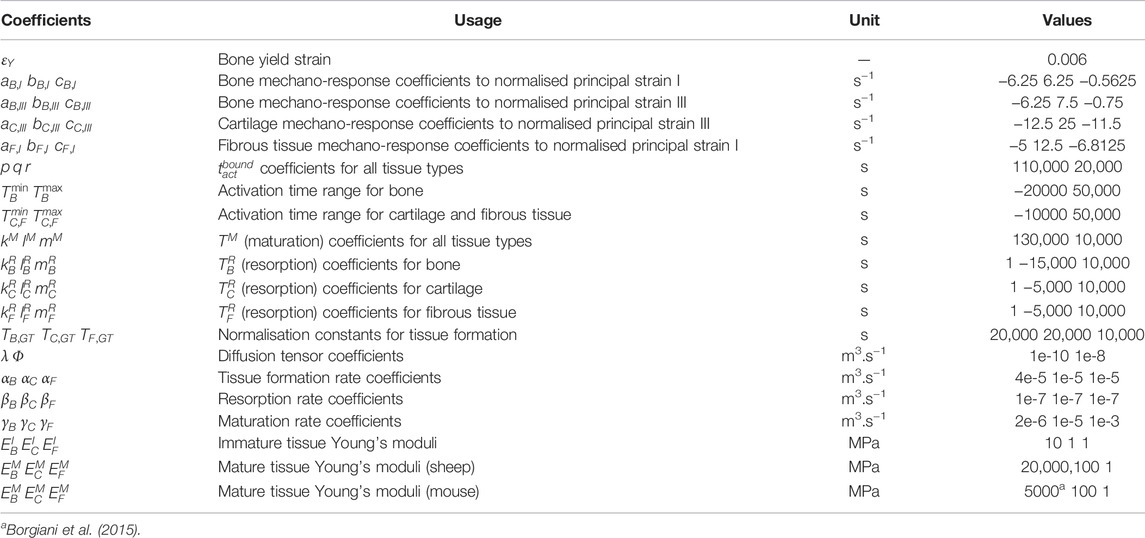
TABLE 1. Computer model parameters [adapted from (Frame et al., 2017)].
The tissue-specific responses
Normalization constant for tissue formation
2.1.2 Tissue Evolution Equations
The different immature and mature tissue fractions evolve depending on the following mechanisms: diffusion, positive contribution of tissue formation and negative contribution of resorption and maturation for the immature tissue fractions (Eq. 8); positive contribution of maturation and negative contribution of resorption for the mature tissue fractions (Eq. 9) (Figure 1). In particular, time was taken into account in the FE simulations to determine changes in the mechanical strains within the healing region due to the formation, resorption and maturation of the different tissues over time. In each time step, the material properties of the FE model were updated based on the predicted tissue fractions and a mechanical analysis was performed to determine the mechanical principal strains. The evolution is implemented by means of diffusion-reaction equations for tissue type i (i = B, C or F):
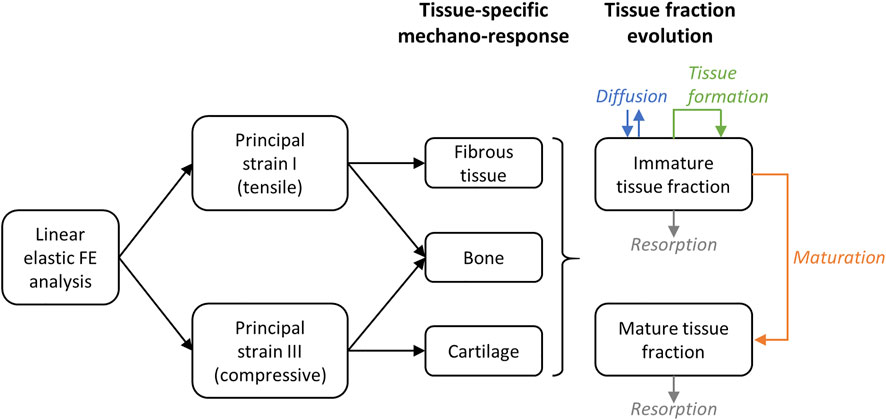
FIGURE 1. Principle of the mechano-sensing and tissue fraction evolution simulation. On the right (tissue fraction evolution), the direction of the arrow indicates if the contribution is positive (arrow pointing towards the box) or negative (arrow pointing away from the box).
The immature tissue diffusion term
The immature tissue formation term
2.1.3 Mechanical Analysis
The diffusion-reaction equations for the different tissue type evolutions are coupled to a mechanical analysis providing the principal strain values and stress directions. All materials are assumed to be linear isotropic elastic materials following the Hooke’s law. Each tissue type in immature and mature states is attributed specific Young’s moduli (Table 1) and a Poisson’s ratio of 0.3. A rule of mixtures is used to compute the FE Young’s modulus by averaging the material properties of its various tissue fractions:
2.2 Application to Fracture Healing Experiments
2.2.1 Experimental Set-Ups
The multi-tissue evolution model was applied to a mouse (Kruck et al., 2018) and a sheep fracture healing experiments (Epari et al., 2006).
Briefly, in the mouse experiment, a 0.5-mm osteotomy was performed at the femoral mid-shaft in adult mice. The defect was fixed using a polyether ether kethone (PEEK) external fixator and four titanium screws (Figure 2A). Follow-up histology (mid-sagittal cut with Movat-Pentachrome staining) was performed 7, 14 and 21 days post-surgery to reveal bone in yellow, cartilage in green and fibrous tissue in red (Kruck et al., 2018).
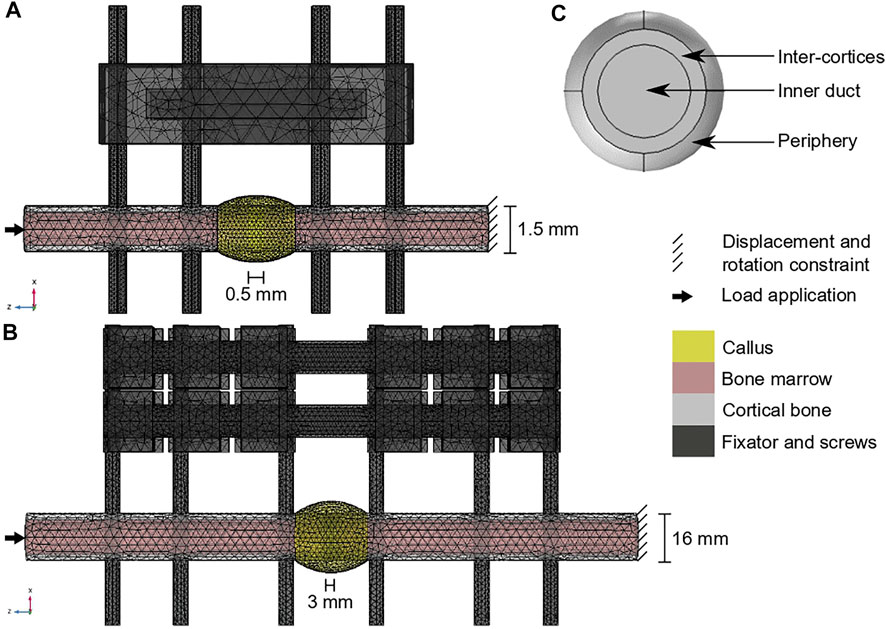
FIGURE 2. Computer model set-ups: (A) Mouse fracture healing geometry; (B) sheep fracture healing geometry; (C) region of interest definition in the callus radial view (shown in yellow on a and b). The color code for (A,B) is given on the right.
In the sheep experiment, a 3-mm osteotomy was performed at the tibial mid-shaft in adult sheep. The defect was fixed using a stainless-steel external fixator and six steel screws (Figure 2B). Follow-up histology (mid-sagittal cut with Safranin Orange/van Kossa staining) was performed 2, 3, 6 and 9 weeks post-surgery to reveal bone in black, cartilage in dark red and fibrous tissue in bright red (Epari et al., 2006).
2.2.2 Computer Model Geometries
Both experimental set-ups were reproduced in COMSOL v.5.6 to simulate tissue evolution in the callus (regenerating region). Intact bone, marrow cavity, fixator and screws were included in the models. Fixator shapes and dimensions were taken from the experiments. Simulation times were chosen to reproduce the experimentally observed tissue remodeling and were then related to physiological times based on the analysis results.
For the mouse model (Figure 2A), the intact bone extremities were modelled as cylinders of radius 0.75 mm containing the marrow cavity of radius 0.55 mm (Borgiani et al., 2015). The screws had a radius of 0.3 mm and a length of 10 mm. The callus was obtained by rotating a circle arc of height 0.3 mm around the 0.5-mm defect and covering 1 mm of the intact bone extremities. All parts were meshed with tetrahedral elements of following average sizes: 0.3 mm for the intact cortical bone extremities and the bone marrow cavities, 0.2 mm for the screws, 0.6 mm for the fixator, 0.16 mm for the callus inner duct, inter-cortices and periphery regions. The simulation was run for 3000 h (simulation time).
For the sheep model (Figure 2B), the intact bone extremities were modelled as cylinders of radius 8 mm containing the marrow cavity of radius 6 mm (Checa et al., 2011). The screws had a radius of 2.5 mm and a length of 100 mm. The callus was obtained by rotating a circle arc of height 4 mm around the 3-mm defect and covering 1 cm of the intact bone extremities. All parts were meshed with tetrahedral elements of following average sizes: 3.3 mm for the intact cortical bone extremities, 3.5 mm for the bone marrow cavities, 2.5 mm for the screws, 3.8 mm for the fixator, 1.1 mm for the callus inner duct and inter-cortices regions and 2.1 mm for the callus periphery. The simulation was run for 6000 h (simulated time).
2.2.3 Material Properties
In addition to the regenerating tissue material properties (Table 1), intact cortical bone, bone marrow, steel (sheep screw and fixator), PEEK (mouse fixator) and titanium (mouse screws) were assumed to be isotropic linear elastic materials, with properties defined in Table 2.
2.2.4 Loading and Boundary Conditions
In both set-ups, the distal end of the bone was clamped (all translation and rotation degrees of freedom were set equal to 0). For the mouse (average weight 0.025 kg), 1.5 N compression, 0.3 N lateral-medial bending and 0.3 N antero-posterior bending were applied on the proximal end of the bone (Borgiani et al., 2015). For the sheep (average weight 60 kg), 1200 N compression and 75 N antero-posterior bending were applied on the proximal end of the bone (Duda et al., 1997; Checa et al., 2011).
2.2.5 Initial Tissue Distributions
The multi-tissue evolution model aimed at investigating the experimentally observed late remodeling phase in the fracture healing experiments. Therefore, the 14-day histology data (mouse, 7 samples) and 6-week histology data (sheep, 1 sample) were segmented and quantified for mature tissue content. The average mature tissue fractions were computed to define the initial tissue distribution in the callus at the start of the remodeling simulation, in the three regions of interest (ROIs) defined in Figure 2C: inner duct, inter-cortices, periphery (Table 3). The immature tissue fractions were assumed to be equal to 5% initially as they could not be visualized in the experimental data.

TABLE 3. Initial tissue fractions in the regions of interest, computed from the quantification of the 14-day and 6-week histology data for mouse and sheep, respectively; the immature tissue fractions were all assumed to be equal to 5% initially.
2.3 Computer Model Output Analysis
Histology-like images were derived from the computer model predictions at start and end of the simulation corresponding to 14 and 21 days (mouse) and 6 and 9 weeks (sheep) post-surgery: cartilage density was plotted in green (mouse) or red (sheep) shades, fibrous tissue density in red shades, bone density in yellow (mouse) or black (sheep) shades. In addition, to investigate the observed tissue evolution, the distribution of the minimal and maximal principal strains and the bone activation functions TB were plotted over time in the mid-sagittal plane. Besides, the average mature bone, cartilage and fibrous tissue fractions were quantified over time in the three ROIs (Figure 2C).
3 Results
3.1 Mouse Fracture Healing
Experimentally, mice experienced tissue remodeling between 14 and 21 days post-surgery (Figure 3A), with a re-opening of the bone marrow cavity. The simulation started from a homogeneous high-density bone in the inner duct and inter-cortices (similar to the histology 14 days post-surgery) and led to denser bone formation in the inter-cortices and resorption in the inner duct and the periphery (Figure 3B). The addition of one intermediate time-point (Figure 3B) and the quantification over time (Figure 4) gave more insight into the healing process: the bone volume fraction decreased over time in the inner duct and in the periphery. In the inter-cortices, the bone volume fraction stagnated, slightly increased, in particular in the side opposite to the fixation plate, and slightly decreased again (Figures 3B, 4). Cartilage and fibrous tissue volume fractions decreased only slightly over time (Figures 3C,D, 4).
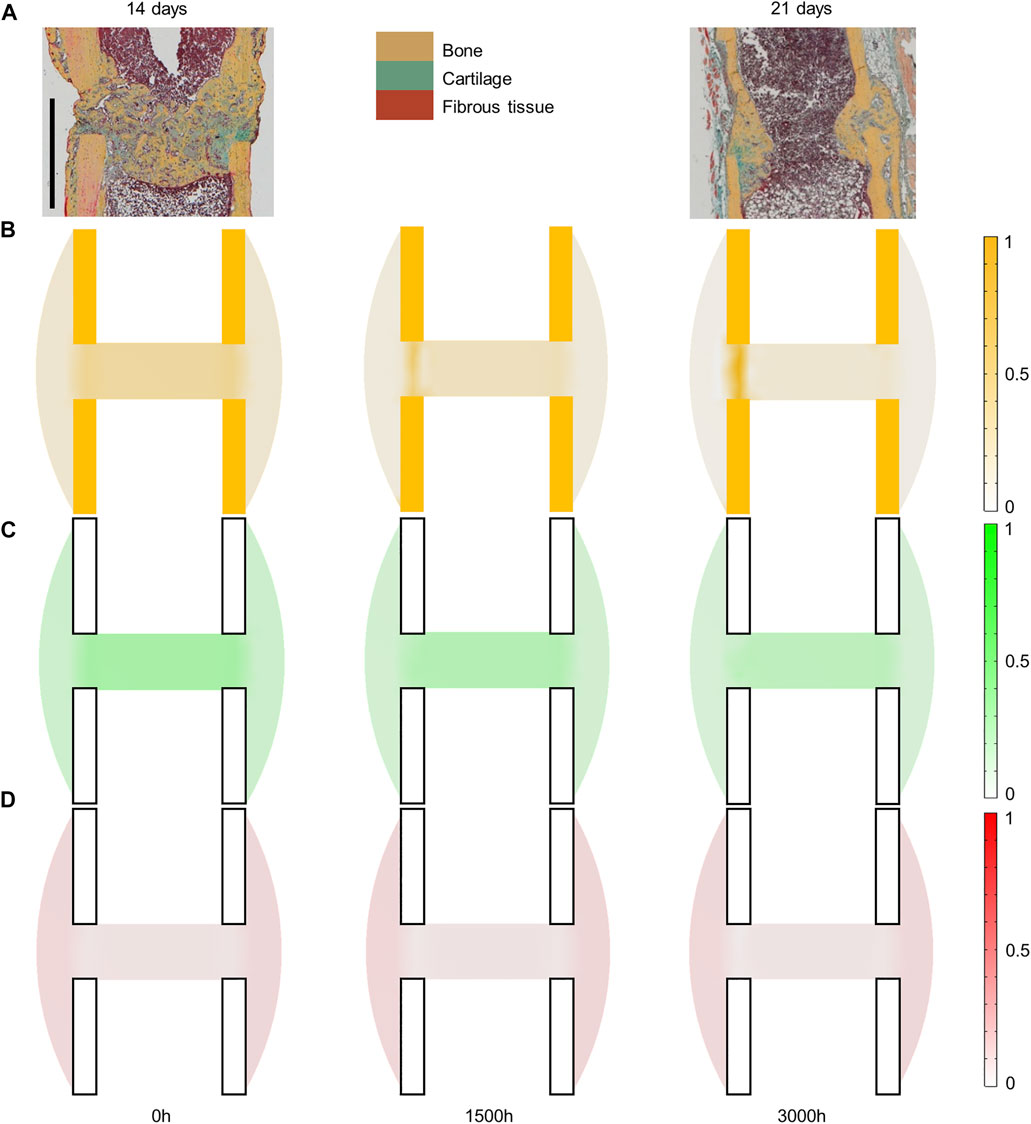
FIGURE 3. Mouse fracture tissue distribution in the mid-sagittal plane: (A) experimental 14- and 21-day histology (image courtesy: Bettina Kruck); (B) predicted mature bone fraction at 0, 1,500 and 3000 h; (C) predicted mature cartilage fraction at 0, 1,500 and 3000 h; (D) predicted mature fibrous tissue fraction at 0, 1,500 and 3000 h. Scale bar: 1 mm; the fixator was placed on the right of all images (medial side).
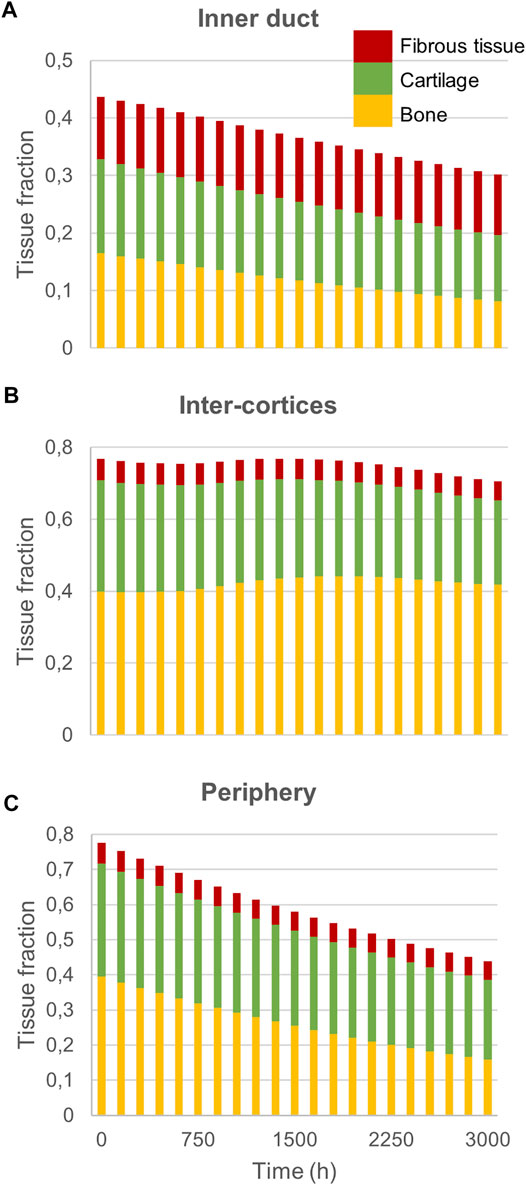
FIGURE 4. Mouse fracture quantification: Mature bone, cartilage and fibrous tissue fraction evolution over time in (A) the inner duct; (B) the inter-cortices and (C) the periphery.
The principal strain values remained lower than 0.12% in compression and 0.06% in tension (Figures 5A,B), leading to fibrous tissue and cartilage mechano-responses that were always minimal, thus yielding resorption of those tissue types (Figures 3C,D). The bone mechano-response was positive (bone formation) mainly in the inter-cortices region, and rather on the side opposite to the external fixator (left on the figures), at late time-points (Figure 5C). The time evolution of the bone mechano-response corresponded thus to the observed stagnation and slight increase in bone volume fraction in the inter-cortices (Figures 3B, 4). In the inner duct and the periphery, the response was always negative, leading to bone resorption (Figures 4, 5). Bone resorption was associated with absolute minimal and maximal principal strain levels of <0.08% and <0.03%, respectively, whereas bone formation was associated with absolute minimal and maximal principal strain levels of approximately 0.08 and 0.03%, respectively.
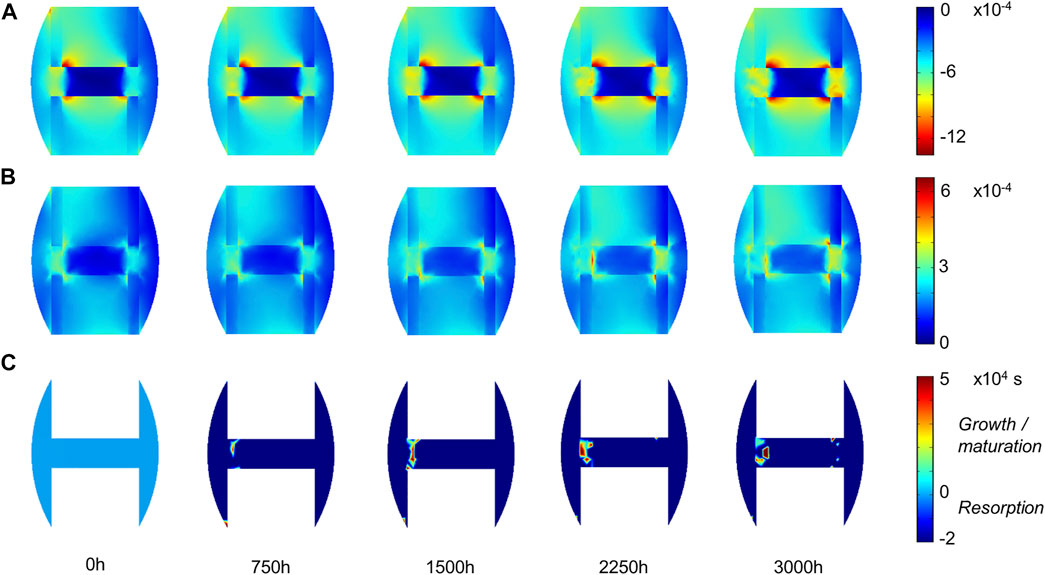
FIGURE 5. Mouse fracture tissue mechano-sensation in mid-sagittal plane: (A) minimal principal strain
3.2 Sheep Fracture Healing
Experimentally, sheep experienced remodeling of the hard callus between 6 and 9 weeks post-surgery (Figure 6A), with a re-opening of the bone marrow cavity and resorption of the peripheral callus. The simulation started from a homogeneous high-density bone in the inter-cortices and intermediate-density in the periphery (similar to the histology 6 weeks post-surgery) and led to slightly less dense bone formation in the inter-cortices and stronger resorption in the periphery and the inner duct regions (Figure 6B). The addition of one intermediate time-point (Figure 6B) and the quantification over time (Figure 7) gave more insight into the healing process: the bone volume fraction decreased over time in the inner duct, while it increased slightly and decreased again in the periphery. In the inter-cortices, the initially very high bone volume fraction first decreased and then reached a plateau at ca. 45%. Cartilage and fibrous tissue volume fractions decreased slowly over time (Figures 6C,D, 7).
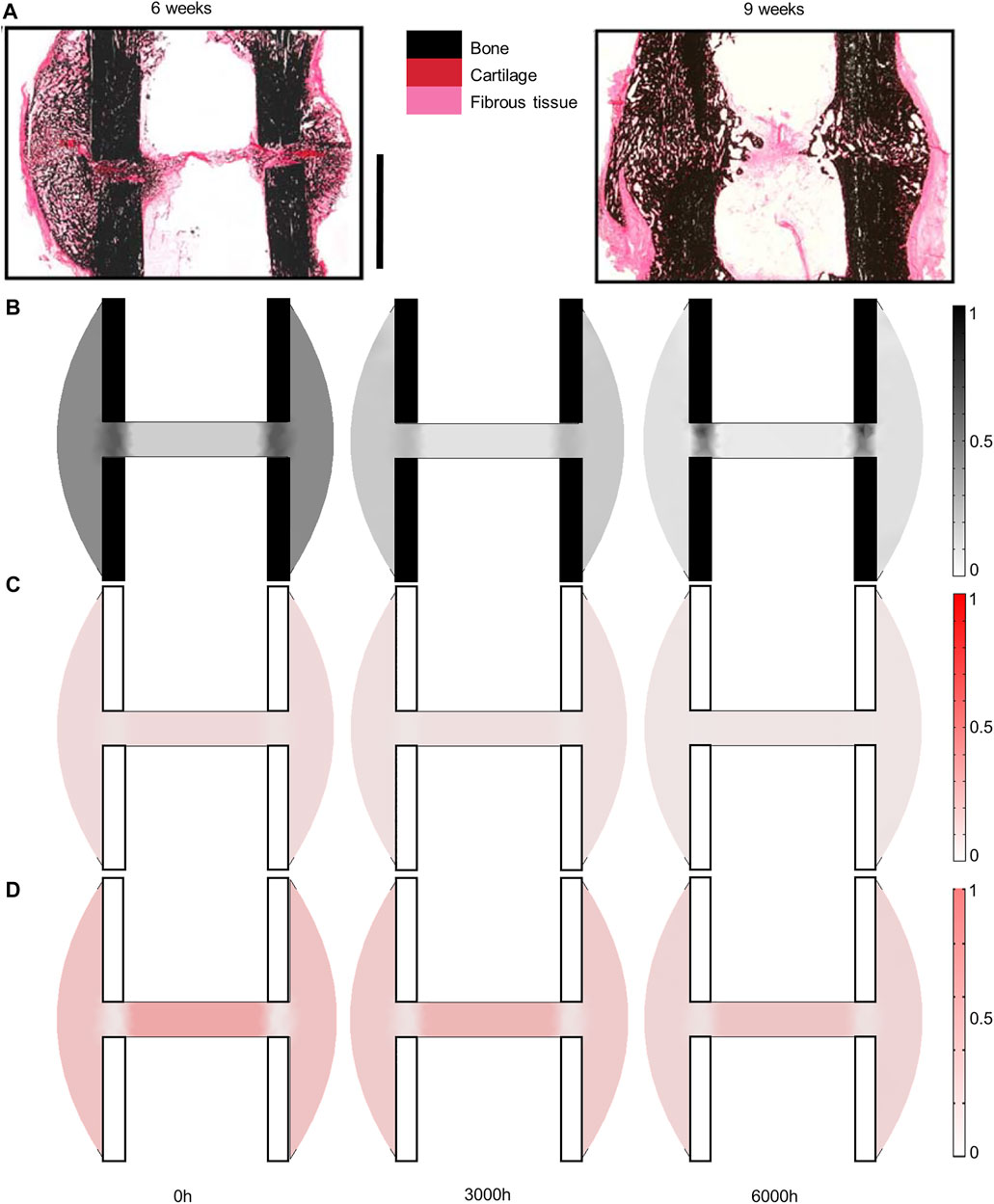
FIGURE 6. Sheep fracture tissue distribution in the mid-sagittal plane: (A) experimental 6- and 9-week histology (reproduced with permission from (Epari et al., 2006) Copyright © 2005 Elsevier Inc.); (B) predicted mature bone fraction at 0, 3,000 and 6000h; (C) predicted mature cartilage fraction at 0, 3,000 and 6000h; (D) predicted mature fibrous tissue fraction at 0, 3,000 and 6000 h. Scale bar: 10 mm; fixator was placed on the right of all images (medial side).
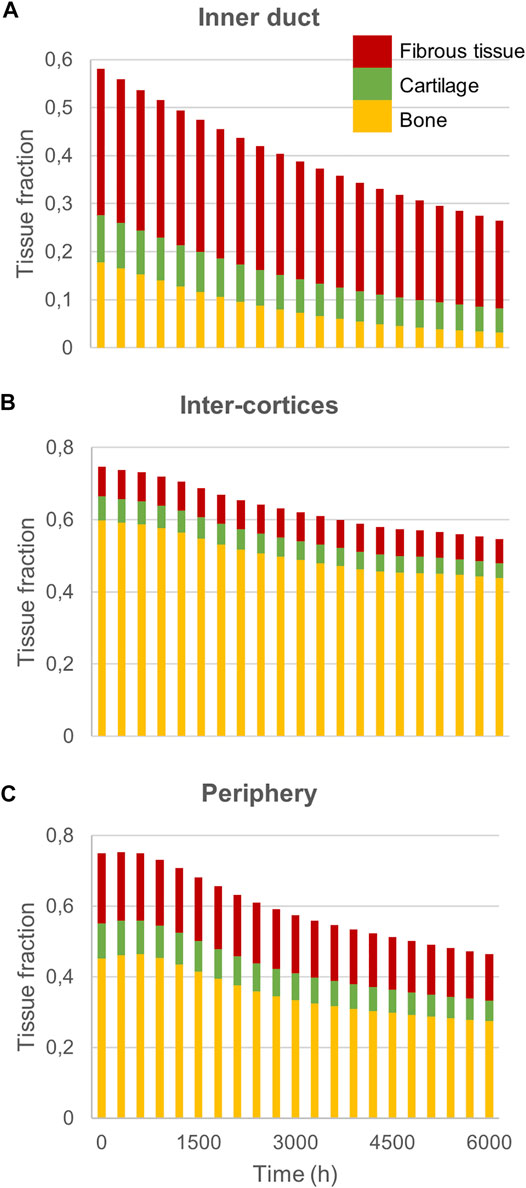
FIGURE 7. Sheep fracture quantification: Mature bone, cartilage and fibrous tissue fraction evolution over time in (A) the inner duct; (B) the inter-cortices and (C) the periphery.
The principal strain values remained lower than 0.1% in compression and tension (Figures 8A,B), leading to fibrous tissue and cartilage mechano-responses that were always minimal, thus yielding resorption of those tissue types (Figures 6C,D). The bone mechano-response was positive only in the inter-cortices region and at late time-points; it was negative during the rest of the simulation as the regenerating zone was very stiff, with low strain levels (Figures 8A,B). The time evolution of the bone mechano-response corresponded thus to the observed decrease and stagnation in bone volume fraction in the inter-cortices, and the decrease in the inner duct and periphery (Figures 6B, 7). Bone resorption was associated with absolute minimal and maximal principal strain levels of <0.05% and <0.01%, respectively, whereas bone formation was associated with absolute minimal and maximal principal strain levels of approximately 0.05–0.1 and 0.03%, respectively.
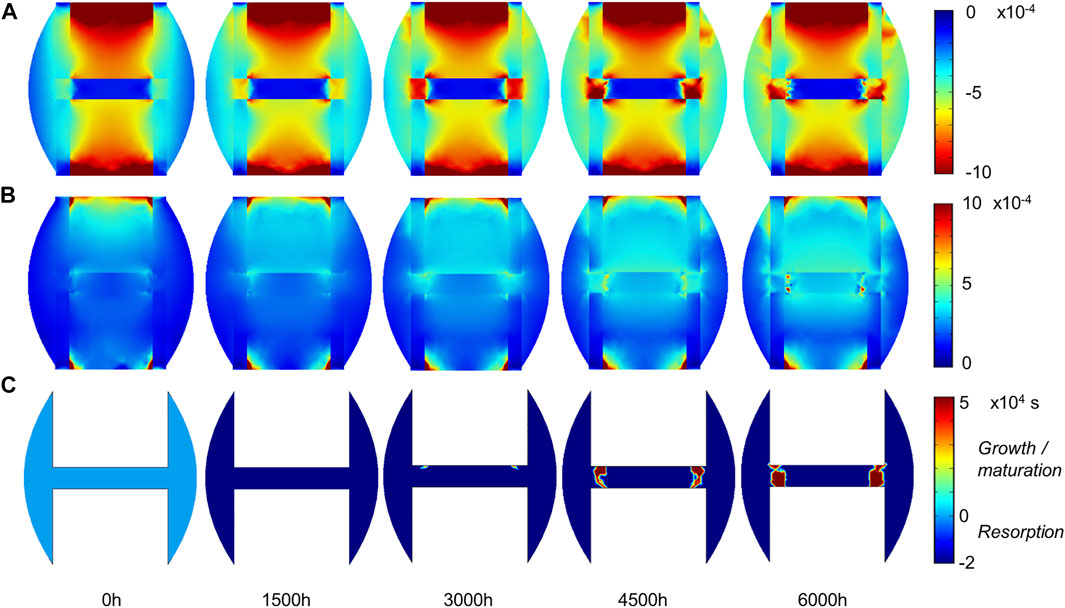
FIGURE 8. Sheep fracture tissue mechano-sensation in mid-sagittal plane: (A) minimal principal strain.
4 Discussion
We presented here a multi-tissue evolution computer model that proved capable of predicting experimentally observed fracture healing remodeling in mice (Kruck et al., 2018) and sheep (Epari et al., 2006) assuming a tissue response to strain accumulation over time. Aim of the simulation studies was to understand the principles behind the observed tissue remodeling in the 21-day (respectively 9-week) data for the mouse (resp. sheep), starting from the 14-day (resp. 6-week) data. In particular, the computer model predicted callus resorption and re-opening of the bone marrow cavity as observed experimentally.
Previous in silico fracture healing studies were able to describe the soft callus and hard callus phases by coupling tissue formation to the local mechanics in the healing region (Lacroix and Prendergast, 2002; Isaksson et al., 2008; Checa et al., 2011; Vetter et al., 2012; Borgiani et al., 2015; Wilson et al., 2017; Wang and Yang, 2018). However, they did not predict the last remodeling stage and bone was predicted to remain in the complete bone marrow cavity at the end of the simulation. A further in silico study validated a bone remodeling model against mouse fracture healing experiments (Isaksson et al., 2009), which could successfully predict the double cortex formation observed in late-stage mouse healing. However, this study did not include other tissue types in the process and focused only on the mouse where this specific phenomenon is observed. An in silico fracture healing model was used to simulate the callus remodeling phase in a human tibia 3-mm defect (Byrne et al., 2011). Although the predictions were not compared to experimental data, this model predicted the restoration of the cortices and the removal of the cartilage phase by using a mechanoregulation theory based on octahedral shear strain and relative fluid velocity. Interestingly, the fracture remodeling was predicted only once the fixator was removed from the simulation (Byrne et al., 2011). In our study, the experimental data suggested that remodeling happened in the presence of the fixator, what could be simulated by our model assuming a response to mechanical strain accumulation over time.
Other bone healing computer models have investigated bone remodeling, but they did not include fibrous tissue or cartilage fractions (Doblaré et al., 2002; García et al., 2002; Hambli et al., 2011; Goda et al., 2016; Mercuri et al., 2016). Multi-tissue evolution constitutes therefore a unique feature of the model used in this study (Frame et al., 2017), making it particularly suitable for fracture healing description where multiple tissues are involved (Schindeler et al., 2008). In particular, the investigation of the mechanical stimuli over time revealed negative fibrous tissue and cartilage response functions, leading to their resorption; and specific patterns in the bone response function leading to the observed higher bone density in the region joining the intact cortices. In the sheep, strain levels leading to bone resorption were lower than 100 microstrains (µε) in tension and 500 µε in compression, whereas bone formation happened under 500–1000 µε compression but very low tension (<300 µε). In the mouse, thresholds between bone resorption and formation were around 300 µε in tension and 800 µε in compression. Similar value ranges have been used for the strain energy density threshold in bone remodeling literature: 1000 µε in (Dunlop et al., 2009), 500 µε in (Isaksson et al., 2009), 700–1000 µε in (Schulte et al., 2013); Razi and others also found a lower threshold for bone response in tension (300 µε) compared to compression (1200 µε) (Razi et al., 2015). Here, these values are no exact thresholds as the accumulation of strain over a few hours was taken into account as a mechano-response. Indeed, when strain levels increased after the initial bone resorption in the inter-cortices region in the sheep (3000 h) and the mouse (1500 h), the bone mechano-response remained negative and would turn positive only later (Figures 5C, 8C). The implementation of this hysteresis rule avoided the oscillations sometimes seen in bone healing predictions that rely only on one threshold between bone resorption and formation (Perier-Metz et al., 2020). More importantly, this is a way to take into account the accumulation of damage and micro-cracks taking part in the bone remodeling process (Carter et al., 1988; Mori and Burr, 1993; Prendergast and Taylor, 1994; Doblaré et al., 2002; Martin and Seeman, 2008). Previous computer models of bone remodeling based on strain accumulation have been able to predict the femur head bone density distribution (Frame et al., 2018) and healing patterns in a mandible (Schmitt et al., 2016); however, other mechanical signals have also proven to be valid, e.g., the strain energy density (Schulte et al., 2013). In general, a consensus about the mechanical stimulus driving the bone remodeling process does not exist (Webster and Müller, 2011; Weinkamer et al., 2019).
We applied the same computer model in two different species (mouse and sheep) and found no inter-species difference. This finding contrasts with previous results in fracture healing simulations where different mechanosensitivity levels were hypothesized to explain fracture healing patterns in the mouse, rat and sheep (Checa et al., 2011; Borgiani et al., 2015). Here, adapted material properties, geometries, loading conditions and initial tissue distribution (intermediate fracture healing stage) were sufficient to reproduce each animal’s specific tissue patterns. Experimental studies also suggested similar remodeling behaviors among various mammal species and humans (Garetto et al., 1995; Christen et al., 2014).
The computer model used in this study had several limitations and simplifications. First, the simulation time did not match the biological time, although tissue formation rates were derived from experimental literature. Remodeling was observed in 7 days in the mouse and 3 weeks in the sheep, whereas in silico approximately 88 “model days” were needed for the mouse and 225 for the sheep; the computer model prediction time should therefore be divided by approximately 10–12 to describe the real biological time. However, the ratio between both animals was similar in silico and in vivo, with a remodeling process happening ca. 3 times faster in the mouse than in the sheep. Therefore, we discarded the simulation time as having any biological meaning and used it only for analysis purposes of the tissue evolution in the healing process. Fibrous tissue and cartilage remnants were predicted, for instance in the inner gap region where the bone marrow cavity should actually form back. These wrong predictions are likely due to the absence of modelling the restoration of the bone marrow in the fracture gap and of other surrounding tissues (for the periphery region). A more complete model could include these tissues. A further limitation consisted in restricting the regenerating region to the callus; in fact, experimental observations suggest that the intact cortical bone is also remodeled during fracture healing (Vetter et al., 2010; Wehrle et al., 2021); it could thus be included in the diffusion-formation-resorption process in future studies. Here, mechano-response was hypothesized to be driven solely by principal strains, as hypothesized in previous bone remodeling theories (Frost, 1996); however, combinations of shear strains and relative fluid velocity (Prendergast et al., 1997) or minimal principal strain and hydrostatic stress (Claes and Heigele, 1999) have been suggested as mechanical stimuli in fracture healing. A more comprehensive mechanoregulation theory might better describe fibrocartilage resorption over the course of healing. Moreover, isotropic linear elastic descriptions were chosen for the different materials; poroelasticity and anisotropy of the bone (Giorgio et al., 2016), and hyperelasticity of the cartilage and fibrous tissue (Freutel et al., 2014) could be included in future studies for more realistic tissue descriptions. Lastly, the tissue mechano-response and the evolution equations require several parameters that are derived from literature or experimental values; a parameter sensitivity analysis was performed to identify the most critical parameters for the predictions (Supplementary Material S1). A few bone mechano-response parameters (bB,III and cB,III, bB,I and cB,I, mBM, mBR) were found to influence strongly the predicted bone density (Supplementary Figure S2). The values for bB,III, cB,III, bB,I and cB,I, relating the bone mechano-response to the third and first principal strain values, respectively, were chosen based on literature (Frost, 1996; Prendergast et al., 1997; Turner, 1998; Claes and Heigele, 1999; Martin and Seeman, 2008). The values for mBM and mBR, relating the bone maturation and resorption functions to the accumulated strain, were determined based on preliminary studies to achieve consistent results (Frame et al., 2017).
In summary, we presented here a multi-tissue evolution computer model in a fracture healing context and validated its predictive capability in mouse and sheep late-stage fracture healing and remodeling. This model improved the understanding of bone fracture remodeling and suggested that this healing stage can be explained by an accumulation of mechanical strains, namely that the loading history over a few hours can account for observed tissue patterning and remodeling. Previously, the model has been shown to explain bone remodeling in the femoral head (Frame et al., 2018) and in the mandible (Schmitt et al., 2016). Future work should take advantage of this model to investigate impaired healing situations or non-unions, e.g., due to osteoporosis or other co-morbidities, and evaluate the validity of the model in this context. Moreover, different fixation or implant-based systems could be tested to evaluate their healing potential in specific situations.
Data Availability Statement
The raw data supporting the conclusion of this article will be made available by the authors, without undue reservation.
Author Contributions
All authors contributed to conception and design of the study. CP-M and RA developed the in silico models. CP-M collected and interpreted the data. CP-M wrote the first draft of the manuscript. RA and SC provided technical guidance on the project. All authors revised and edited the manuscript and approved its content.
Funding
This study was part of CP-M’ PhD project funded by MINES ParisTech—PSL Research University.
Conflict of Interest
The authors declare that the research was conducted in the absence of any commercial or financial relationships that could be construed as a potential conflict of interest.
Publisher’s Note
All claims expressed in this article are solely those of the authors and do not necessarily represent those of their affiliated organizations, or those of the publisher, the editors and the reviewers. Any product that may be evaluated in this article, or claim that may be made by its manufacturer, is not guaranteed or endorsed by the publisher.
Supplementary Material
The Supplementary Material for this article can be found online at: https://www.frontiersin.org/articles/10.3389/fbioe.2022.835094/full#supplementary-material
Abbreviations
⊗, Tensor product; B (subscript), Bone; C (subscript), Cartilage; Δ, Laplacian; D, Diffusion tensor; E, Young’s modulus;
References
Beaupré, G. S., Orr, T. E., and Carter, D. R. (1990). An Approach for Time-dependent Bone Modeling and Remodeling-Theoretical Development. J. Orthop. Res. 8, 651–661. doi:10.1002/jor.1100080506
Borgiani, E., Duda, G., Willie, B., and Checa, S. (2015). Bone Healing in Mice: Does it Follow Generic Mechano-Regulation Rules? Facta Universitatis, Ser. Mech. Eng. 13, 217–227.
Byrne, D. P., Lacroix, D., and Prendergast, P. J. (2011). Simulation of Fracture Healing in the Tibia: Mechanoregulation of Cell Activity Using a Lattice Modeling Approach. J. Orthop. Res. 29, 1496–1503. doi:10.1002/jor.21362
Carter, D. R., Blenman, P. R., and Beaupré, G. S. (1988). Correlations between Mechanical Stress History and Tissue Differentiation in Initial Fracture Healing. J. Orthop. Res. 6, 736–748. doi:10.1002/jor.1100060517
Checa, S., Prendergast, P. J., and Duda, G. N. (2011). Inter-species Investigation of the Mechano-Regulation of Bone Healing: Comparison of Secondary Bone Healing in Sheep and Rat. J. Biomech. 44, 1237–1245. doi:10.1016/j.jbiomech.2011.02.074
Christen, P., Ito, K., Ellouz, R., Boutroy, S., Sornay-Rendu, E., Chapurlat, R. D., et al. (2014). Bone Remodelling in Humans Is Load-Driven but Not Lazy. Nat. Commun. 5, 4855. doi:10.1038/ncomms5855
Claes, L. E., and Heigele, C. A. (1999). Magnitudes of Local Stress and Strain along Bony Surfaces Predict the Course and Type of Fracture Healing. J. Biomech. 32, 255–266. doi:10.1016/S0021-9290(98)00153-5
Claes, L. E., Heigele, C. A., Neidlinger-Wilke, C., Kaspar, D., Seidl, W., Margevicius, K. J., et al. (1998). Effects of Mechanical Factors on the Fracture Healing Process. Clin. Orthopaedics Relat. Res. 355S, S132–S147. doi:10.1097/00003086-199810001-00015
Doblaré, M., García, J. M., and Cegoñino, J. (2002). Development of an Internal Bone Remodeling Theory and Applications to Some Problems in Orthopaedic Biomechanics. Meccanica 37, 365–374. doi:10.1023/A:1020835720405
Duda, G. N., Eckert-Hübner, K., Sokiranski, R., Kreutner, A., Miller, R., and Claes, L. (1997). Analysis of Inter-fragmentary Movement as a Function of Musculoskeletal Loading Conditions in Sheep. J. Biomech. 31, 201–210. doi:10.1016/S0021-9290(97)00127-9
Dunlop, J. W. C., Hartmann, M. A., Bréchet, Y. J., Fratzl, P., and Weinkamer, R. (2009). New Suggestions for the Mechanical Control of Bone Remodeling. Calcif Tissue Int. 85, 45–54. doi:10.1007/s00223-009-9242-x
Einhorn, T. A. (1995). Enhancement of Fracture-Healing. J. Bone Jt. Surg. 77, 940–956. doi:10.2106/00004623-199506000-00016
Epari, D. R., Schell, H., Bail, H. J., and Duda, G. N. (2006). Instability Prolongs the Chondral Phase during Bone Healing in Sheep. Bone 38, 864–870. doi:10.1016/j.bone.2005.10.023
Frame, J. C., Rohan, P.-Y., Corté, L., and Allena, R. (2018). Optimal Bone Structure Is Dependent on the Interplay between Mechanics and Cellular Activities. Mech. Res. Commun. 92, 43–48. doi:10.1016/j.mechrescom.2018.07.005
Frame, J., Rohan, P.-Y., Corté, L., and Allena, R. (2017). A Mechano-Biological Model of Multi-Tissue Evolution in Bone. Continuum Mech. Thermodyn. 31, 1–31. doi:10.1007/s00161-017-0611-9
Freutel, M., Schmidt, H., Dürselen, L., Ignatius, A., and Galbusera, F. (2014). Finite Element Modeling of Soft Tissues: Material Models, Tissue Interaction and Challenges. Clin. Biomech. 29, 363–372. doi:10.1016/j.clinbiomech.2014.01.006
Frost, H. M. (1996). Perspectives: A Proposed General Model of the "mechanostat" (Suggestions from a New Skeletal-Biologic Paradigm). Anat. Rec. 244, 139–147. doi:10.1002/(sici)1097-0185(199602)244:2<139::aid-ar1>3.0.co;2-x
García, J. M., Doblaré, M., and Cegoñino, J. (2002). Bone Remodeling Simulation: a Tool for Implant Design. Comput. Mater. Sci. 25, 100–114. doi:10.1016/S0927-0256(02)00254-9
Garetto, L. P., Chen, J., Parr, J. A., and Roberts, W. E. (1995). Remodeling Dynamics of Bone Supporting Rigidly Fixed Titanium Implants: a Histomorphometric Comparison in Four Species Including Humans. Implant Dentistry 4, 235–243. doi:10.1097/00008505-199500440-00002
Giorgio, I., Andreaus, U., Scerrato, D., and dell’Isola, F. (2016). A Visco-Poroelastic Model of Functional Adaptation in Bones Reconstructed with Bio-Resorbable Materials. Biomech. Model. Mechanobiol 15, 1325–1343. doi:10.1007/s10237-016-0765-6
Goda, I., Ganghoffer, J.-F., and Maurice, G. (2016). Combined Bone Internal and External Remodeling Based on Eshelby Stress. Int. J. Sol. Structures 94-95 (95), 138–157. doi:10.1016/j.ijsolstr.2016.04.036
Hambli, R., Katerchi, H., and Benhamou, C.-L. (2011). Multiscale Methodology for Bone Remodelling Simulation Using Coupled Finite Element and Neural Network Computation. Biomech. Model. Mechanobiol 10, 133–145. doi:10.1007/s10237-010-0222-x
Isaksson, H., Gröngröft, I., Wilson, W., van Donkelaar, C. C., van Rietbergen, B., Tami, A., et al. (2009). Remodeling of Fracture Callus in Mice Is Consistent with Mechanical Loading and Bone Remodeling Theory. J. Orthop. Res. 27, 664–672. doi:10.1002/jor.20725
Isaksson, H., van Donkelaar, C. C., Huiskes, R., and Ito, K. (2008). A Mechano-Regulatory Bone-Healing Model Incorporating Cell-Phenotype Specific Activity. J. Theor. Biol. 252, 230–246. doi:10.1016/j.jtbi.2008.01.030
Isaksson, H., van Donkelaar, C. C., Huiskes, R., and Ito, K. (2006). Corroboration of Mechanoregulatory Algorithms for Tissue Differentiation during Fracture Healing: Comparison with In Vivo Results. J. Orthop. Res. 24, 898–907. doi:10.1002/jor.20118
Knecht, R. S., Bucher, C. H., Van Linthout, S., Tschöpe, C., Schmidt-Bleek, K., and Duda, G. N. (2021). Mechanobiological Principles Influence the Immune Response in Regeneration: Implications for Bone Healing. Front. Bioeng. Biotechnol. 9, 81. doi:10.3389/fbioe.2021.614508
Kruck, B., Zimmermann, E. A., Damerow, S., Figge, C., Julien, C., Wulsten, D., et al. (2018). Sclerostin Neutralizing Antibody Treatment Enhances Bone Formation but Does Not Rescue Mechanically Induced Delayed Healing. J. Bone Miner Res. 33, 1686–1697. doi:10.1002/jbmr.3454
Lacroix, D., and Prendergast, P. J. (2002). Three-dimensional Simulation of Fracture Repair in the Human Tibia. Comp. Methods Biomech. Biomed. Eng. 5, 369–376. doi:10.1080/1025584021000025014
Martin, T. J., and Seeman, E. (2008). Bone Remodelling: Its Local Regulation and The Emergence of Bone Fragility. Best Pract. Res. Clin. Endocrinol. Metab. 22, 701–722. doi:10.1016/j.beem.2008.07.006
Mori, S., and Burr, D. B. (1993). Increased Intracortical Remodeling Following Fatigue Damage. Bone 14, 103–109. doi:10.1016/8756-3282(93)90235-3
Mercuri, E. G. F., Daniel, A. L., Hecke, M. B., and Carvalho, L. (2016). Influence of Different Mechanical Stimuli in a Multi-Scale Mechanobiological Isotropic Model for Bone Remodelling. Med. Eng. Phys. 38, 904–910. doi:10.1016/j.medengphy.2016.04.018
Perier-Metz, C., Duda, G. N., and Checa, S. (2020). Mechano-Biological Computer Model of Scaffold-Supported Bone Regeneration: Effect of Bone Graft and Scaffold Structure on Large Bone Defect Tissue Patterning. Front. Bioeng. Biotechnol. 8. doi:10.3389/fbioe.2020.585799
Prendergast, P. J., Huiskes, R., and Søballe, K. (1997). Biophysical Stimuli on Cells during Tissue Differentiation at Implant Interfaces. J. Biomech. 30, 539–548. doi:10.1016/S0021-9290(96)00140-6
Prendergast, P. J., and Taylor, D. (1994). Prediction of Bone Adaptation Using Damage Accumulation. J. Biomech. 27, 1067–1076. doi:10.1016/0021-9290(94)90223-2
Razi, H., Birkhold, A. I., Weinkamer, R., Duda, G. N., Willie, B. M., and Checa, S. (2015). Aging Leads to a Dysregulation in Mechanically Driven Bone Formation and Resorption: Mechanoregulation of (Re)Modeling. J. Bone Miner. Res. 30, 1864–1873. doi:10.1002/jbmr.2528
Schell, H., Duda, G. N., Peters, A., Tsitsilonis, S., Johnson, K. A., and Schmidt-Bleek, K. (2017). The Haematoma and its Role in Bone Healing. J. Exp. Ortop 4, 5. doi:10.1186/s40634-017-0079-3
Schindeler, A., McDonald, M. M., Bokko, P., and Little, D. G. (2008). Bone Remodeling during Fracture Repair: The Cellular Picture. Semin. Cel Develop. Biol. 19, 459–466. doi:10.1016/j.semcdb.2008.07.004
Schmitt, M., Allena, R., Schouman, T., Frasca, S., Collombet, J. M., Holy, X., et al. (2016). Diffusion Model to Describe Osteogenesis within a Porous Titanium Scaffold. Comp. Methods Biomech. Biomed. Eng. 19, 171–179. doi:10.1080/10255842.2014.998207
Schulte, F. A., Ruffoni, D., Lambers, F. M., Christen, D., Webster, D. J., Kuhn, G., et al. (2013). Local Mechanical Stimuli Regulate Bone Formation and Resorption in Mice at the Tissue Level. PLOS ONE 8, e62172. doi:10.1371/journal.pone.0062172
Turner, C. H. (1998). Three Rules for Bone Adaptation to Mechanical Stimuli. Bone 23, 399–407. doi:10.1016/S8756-3282(98)00118-5
Vetter, A., Epari, D. R., Seidel, R., Schell, H., Fratzl, P., Duda, G. N., et al. (2010). Temporal Tissue Patterns in Bone Healing of Sheep. J. Orthop. Res. 28, 1440–1447. doi:10.1002/jor.21175
Vetter, A., Witt, F., Sander, O., Duda, G. N., and Weinkamer, R. (2012). The Spatio-Temporal Arrangement of Different Tissues during Bone Healing as a Result of Simple Mechanobiological Rules. Biomech. Model. Mechanobiol 11, 147–160. doi:10.1007/s10237-011-0299-x
Wang, M., and Yang, N. (2018). Three-dimensional Computational Model Simulating the Fracture Healing Process with Both Biphasic Poroelastic Finite Element Analysis and Fuzzy Logic Control. Sci. Rep. 8, 6744. doi:10.1038/s41598-018-25229-7
Webster, D., and Müller, R. (2011). In Silico Models of Bone Remodeling From Macro to Nano—From Organ to Cell. Wiley Interdiscip. Rev. Syst. Biol. Med. 3, 241–251. doi:10.1002/wsbm.115
Weinkamer, R., Eberl, C., and Fratzl, P. (2019). Mechanoregulation of Bone Remodeling and Healing as Inspiration for Self-Repair in Materials. Biomimetics 4, 46. doi:10.3390/biomimetics4030046
Wehrle, E., Tourolle né Betts, D. C., Kuhn, G. A., Floreani, E., Nambiar, M. H., Schroeder, B. J., et al. (2021). Spatio-temporal Characterization of Fracture Healing Patterns and Assessment of Biomaterials by Time-Lapsed In Vivo Micro-computed Tomography. Sci. Rep. 11, 8660. doi:10.1038/s41598-021-87788-6
Keywords: bone fracture remodeling, multi-tissue evolution, in silico modeling, in vivo validation, mechanobiology
Citation: Perier-Metz C, Corté L, Allena R and Checa S (2022) A 3D in Silico Multi-Tissue Evolution Model Highlights the Relevance of Local Strain Accumulation in Bone Fracture Remodeling. Front. Bioeng. Biotechnol. 10:835094. doi: 10.3389/fbioe.2022.835094
Received: 14 December 2021; Accepted: 08 March 2022;
Published: 31 March 2022.
Edited by:
Stefan Scheiner, Vienna University of Technology, AustriaReviewed by:
Jose Manuel Garcia-Aznar, University of Zaragoza, SpainRidha Hambli, Polytech Orléans, France
Copyright © 2022 Perier-Metz, Corté, Allena and Checa. This is an open-access article distributed under the terms of the Creative Commons Attribution License (CC BY). The use, distribution or reproduction in other forums is permitted, provided the original author(s) and the copyright owner(s) are credited and that the original publication in this journal is cited, in accordance with accepted academic practice. No use, distribution or reproduction is permitted which does not comply with these terms.
*Correspondence: Sara Checa, c2FyYS5jaGVjYUBjaGFyaXRlLmRl
†These authors share last authorship