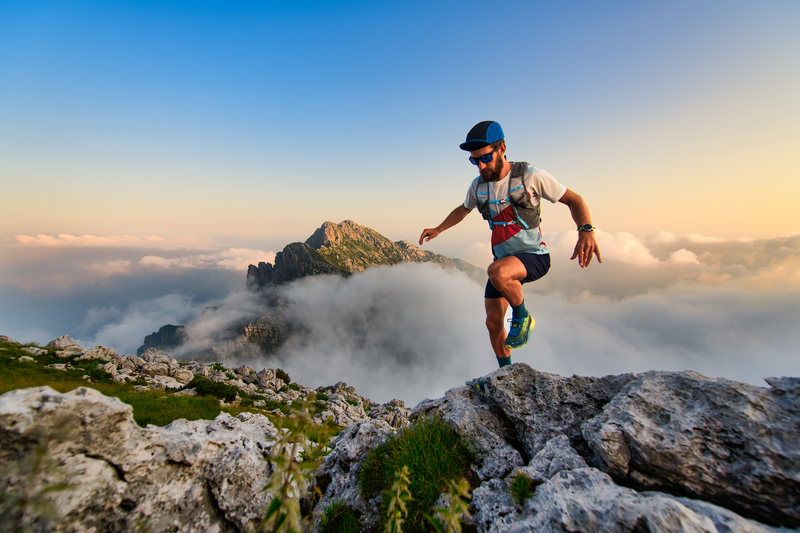
94% of researchers rate our articles as excellent or good
Learn more about the work of our research integrity team to safeguard the quality of each article we publish.
Find out more
ORIGINAL RESEARCH article
Front. Bioeng. Biotechnol. , 22 February 2022
Sec. Cell and Gene Therapy
Volume 10 - 2022 | https://doi.org/10.3389/fbioe.2022.829509
This article is part of the Research Topic Single Cell Intelligence and Tissue Engineering View all 10 articles
Intratumor heterogeneity is a major obstacle to effective cancer treatment. Current methods to study intratumor heterogeneity using single-cell RNA sequencing (scRNA-seq) lack information on the spatial organization of cells. While state-of-the art spatial transcriptomics methods capture the spatial distribution, they either lack single cell resolution or have relatively low transcript counts. Here, we introduce spatially annotated single cell sequencing, based on the previously developed functional single cell sequencing (FUNseq) technique, to spatially profile tumor cells with deep scRNA-seq and single cell resolution. Using our approach, we profiled cells located at different distances from the center of a 2D epithelial cell mass. By profiling the cell patch in concentric bands of varying width, we showed that cells at the outermost edge of the patch responded strongest to their local microenvironment, behaved most invasively, and activated the process of epithelial-to-mesenchymal transition (EMT) to migrate to low-confluence areas. We inferred cell-cell communication networks and demonstrated that cells in the outermost ∼10 cell wide band, which we termed the invasive edge, induced similar phenotypic plasticity in neighboring regions. Applying FUNseq to spatially annotate and profile tumor cells enables deep characterization of tumor subpopulations, thereby unraveling the mechanistic basis for intratumor heterogeneity.
Intratumor heterogeneity, both at the genetic and transcriptomic level, is commonly observed in various cancer types and complicates diagnosis and treatment (Gerlinger et al., 2012; Patel et al., 2014; Morrissy et al., 2017; Puram et al., 2017; Berglund et al., 2018). Rare populations of cells can contribute to increased tumor progression (Burrell et al., 2013; Patel et al., 2014), metastatic potential (Yachida et al., 2010; Navin et al., 2011) and therapy resistance (Sottoriva et al., 2013; Patel et al., 2014; Tirosh et al., 2016). Single-cell sequencing is key to characterizing the complexity of intratumor heterogeneity, but lacks information about functional properties and spatial organization of cells (Lawson et al., 2018). We have recently developed a functionally annotated transcriptomic profiling technique, called functional single cell sequencing (FUNseq), to study heterogeneous populations of tumor cells based on functional features (You et al., 2021). This technology uses live-cell imaging to identify cells with a phenotype of interest (e.g., cell migration or morphology), which can then be phototagged (via a photopatterned device) with a photoactivatable dye, isolated and subjected to single-cell RNA sequencing (scRNA-seq). Hence, FUNseq links phenotypic traits to gene expression profiles of rare subpopulations of tumor cells, thereby identifying the underlying mechanisms of intratumor heterogeneity. However, cells are currently labeled using a single dye, making it impossible to discern cells based on their spatial organization.
Here, we applied FUNseq to characterize intratumor heterogeneity in tumor subpopulations that are spatially located differently in an untransformed, mammary epithelial tumor model. We specifically focused on the epithelial-to-mesenchymal transition (EMT), as this is an important source for intratumor heterogeneity (Nieto et al., 2016). During EMT, epithelial cells gradually acquire a mesenchymal phenotype, thereby losing their cell-cell adhesion and cell polarity while gaining the ability to migrate and invade (Nieto et al., 2016; Pastushenko et al., 2018; Revenco et al., 2019). EMT can be induced by multiple stimuli, including various growth factors and a cell’s local microenvironment (Cook and Vanderhyden 2020). To illustrate, cells at the migrating front of tumors express higher levels of EMT marker genes than cells in the center (Puram et al., 2017). Recently, McFaline-Figueroa et al. (2019) made a similar observation using an in vitro tumor model, showing that untransformed MCF10A cells in the outer layer of a high-confluence patch of cells undergo EMT. However, the exact transcriptomic changes that cause this EMT are currently unknown. To identify the genes that drive the outward migration, one needs to profile the cells in the outermost layer of the cell patch (i.e., the invasive edge). This could be done by spatially annotating bands of cells before subjecting them to scRNA-seq, which enables specific characterization of the invasive edge.
Using a similar tumor model as McFaline-Figueroa et al., we applied FUNseq to profile MCF10A epithelial cells that were spatially located in the outer layer (∼1,000–1,500 μm bandwidth, ∼50 cell wide band) or the outermost layer (250 μm bandwidth, ∼10 cell wide band) of the cell mass. We demonstrated that cells in the outermost layer were progressing through EMT and induced similar phenotypic plasticity in neighboring regions. Using cell-cell communication network analysis, we also showed that the Ephrin, EGF and VEGF signaling pathways were involved in driving this invasive behavior. Our data indicates that FUNseq can spatially profile intratumor heterogeneity, thereby unraveling the underlying mechanisms for the observed phenotypic variations.
We applied FUNseq to profile spatial heterogeneity in an in vitro tumor model: untransformed, mammary epithelial MCF10A cells (Figure 1A). MCF10A cells expressing a GFP marker were seeded in a high-confluence, circular patch. After growing the cells for 6 days, cells at the leading edge of the patch acquired a spindle-like morphology and migrated to unoccupied areas of the dish (Supplementary Figure S1), indicating that they might have undergone EMT (Vuoriluoto et al., 2011).
FIGURE 1. Spatially profiling an in vitro tumor model using the FUNseq technology. (A) Schematic depiction of the assay, cell labeling and scRNA-seq analysis. For the cell labeling (middle panel), we either phototagged concentric rings of equal width (top; 1,000–1,500 μm bandwidth) or 250 μm wide bands at the invasive edge (bottom). In both approaches, the outer population was labeled with JF646 phototagging dye (red) and the middle population was labeled with both JF549 and JF646 (yellow). (B) Patch of MCF10A cells expressing a GFP marker that was phototagged with the larger bandwidth. Green: GFP, yellow: JF549, red: JF646. (C) Phototagging the invasive edge of a MCF10A cell patch yields well-demarcated bands of cells.
Next, we imaged the cells using our custom-built Ultrawide Field-of-view Optical (UFO) microscope (You et al., 2021) and identified the outer, middle and inner regions (with a bandwidth of 1,000–1,500 μm) of the patch. Cells were first incubated with photoactivatable Janelia Fluor 646 (JF646) dye, after which we phototagged the outer one-third of the patch (Figure 1B; cells emit red fluorescence (λex: ∼650 nm, λem: ∼665 nm) after photoactivation). Subsequently, we incubated cells with photoactivatable Janelia Fluor 549 dye (JF549) and phototagged the middle one-third of the patch (cells emit green fluorescence (λex: ∼550 nm, λem: ∼570 nm) after photoactivation). Hence, cells in the middle ring were labeled with both dyes, as the cytoplasmic JF646 dye is retained within cells. Labeled populations were isolated by flow cytometry and sequenced using SORTseq, a plate-based, modified CEL-seq2 scRNA-seq technology (Hashimshony et al., 2016; Muraro et al., 2016).
A similar labeling strategy can be used to profile the invasive edge at a higher resolution. For this, we phototagged cells in the outermost layer (250 μm bandwidth, ∼10 cell wide band) of the patch with JF646 and we phototagged cells in the next 250 μm with both JF549 and JF646. Live-cell imaging of the labeled patches showed well-demarcated bands of cells (Figure 1C), validating that FUNseq can be used to annotate and isolate confined tumor regions with desired spatial bandwidth.
To couple the observed phenotypic plasticity in the outer layer to underlying transcriptomic changes, tumor subpopulations were subjected to scRNA-seq. We sequenced two biological replicates of patches phototagged with the larger bandwidth, yielding a total of 743 analyzed single cell transcriptomes (Supplementary Figure S2). Dimensionality reduction using Uniform Manifold Approximation and Projection (UMAP) (McInnes et al., 2018) indicated a modest separation of the populations but did not form coherent clusters (Figure 2A), suggesting that there is substantial similarity of the gene expression profiles between the tumor regions.
FIGURE 2. scRNA-seq indicated that cells at the invasive edge were progressing through EMT. (A) UMAP embedding of cells labeled with the larger bandwidth showed a modest separation of tumor regions, but no coherent clusters were formed. (B) EMT scores between inner and outer populations vary significantly (p = .0017; Kruskal-Wallis test). (C) Inner and outermost tumor regions labeled with the smaller bandwidth separate clearly in UMAP space. (D) EMT scores gradually increase across the UMAP embedding. (E) Expression of classic epithelial markers decreases radially outwards while expression of classic mesenchymal markers increases. (F) EMT scores are significantly varying between adjacent populations (p < .0001; Kruskal-Wallis test). (G) Volcano plot indicating genes overexpressed in the outermost population (log2(FC) > .5) and in the inner population (log2(FC) < −.5). (H) Overrepresentation analysis using the MSigDB Hallmarks (red) and Wikipathways (black) databases.
To quantity the level of EMT in each subpopulation, we calculated EMT scores using Gene Set Variation Analysis (GSVA) (Hänzelmann et al., 2013). For each cell, an epithelial (E) and mesenchymal (M) score was calculated using two gene sets containing 65 epithelial and 115 mesenchymal genes (Cesano 2015). Following the approach of Sacchetti et al. (2021), we subtracted the E score from the M score to define a single EMT score for each cell (EMT = M – E). Cells in the outer layer had significantly higher EMT scores than cells in the center (Kruskal-Wallis test, p = .0017; Figure 2B). However, no significant changes between adjacent populations were observed, presumably because the relatively large number of cells per region led to substantial heterogeneity within each population (Supplementary Figure S3). This solidified our notion that one needs to specifically profile the invasive edge to reliably identify the transcriptomic drivers for migration and invasion. Hence, we next sought to profile the migrating front at a higher resolution.
We phototagged the migrating front (∼10 cell wide bands) and separated the outermost cells from the inner tumor mass (Figure 1C). Using this high-resolution phototagging approach, we analyzed 696 single cell transcriptomes from two biological replicates. Dimensionality reduction now revealed coherent clusters of cells that segregate based on the spatial populations (Figure 2C). The middle and outermost layer clustered together in the UMAP embedding, presumably since cells in both layers are progressing through EMT. Classically, EMT has been viewed as a discrete process in which cells pass through distinct transition stages before acquiring a fully mesenchymal morphology (Pastushenko and Blanpain 2019). Our UMAP embedding (Figure 2D) indicated that EMT scores vary continuously across the embedded cells, further solidifying recent findings that EMT is a continuous process (McFaline-Figueroa et al., 2019; Cook and Vanderhyden 2020). Expression of the classic epithelial markers E-cadherin (CDH1) and EPCAM gradually decreased from the center to the edge of the patch, while the mesenchymal markers VIM and FN1 showed a reciprocal pattern, suggesting that cells are exhibiting epithelial-mesenchymal plasticity (Zhao et al., 2015; Yang et al., 2020) (Figure 2E; Supplementary Figure S4). These changes in CDH1 expression were not detected by McFaline-Figueroa et al. (2019), underscoring the value of deep sequencing using FUNseq to resolve subtle transcriptomic changes. Moreover, we found that adjacent populations have significantly varying EMT scores (p < .0001; Figure 2F), further increasing our confidence that profiling the invasive edge of the tumor model could identify drivers of migration and invasion.
Next, we identified differentially expressed genes (DEGs) between the subpopulations and found that classic mesenchymal markers such as VIM and the EMT transcription factor SNAI2 were upregulated in the outermost population, while the epithelial markers CDH1 and EPCAM were upregulated in the center of the patch (Figure 2G; Supplementary Figure S4). Genes upregulated in the outermost (n = 165 DEGs) or center (n = 142 DEGs) population were used for overrepresentation analysis using the MSigDB Hallmarks gene set collection (Liberzon et al., 2015) and the Wikipathways database (Martens et al., 2021) (Figure 2H). As expected, genes involved in EMT and extracellular matrix interactions were overrepresented in the outermost population. Additionally, cells at the invasive edge were enriched for the vascular endothelial growth factor (VEGF) signaling pathway, which can induce cell migration and EMT (Anthony D. Yang et al., 2006; Gonzalez-Moreno et al., 2010; Bhattacharya et al., 2017). VEGF can activate the neuropilin-1 receptor (NRP1), which is upregulated in the outermost population (Figure 2G; Supplementary Figure S5) and promotes proliferation, migration and invasion of tumor cells (Goel and Mercurio 2013; Luo et al., 2016).
Since a wide range of transcription factors and extracellular stimuli are involved in stimulating EMT (Nieto et al., 2016), we next set out to map the cell-cell communication networks that regulate the EMT in our in vitro tumor model. We re-analyzed scRNA-seq data from the high-resolution labeling experiment to identify interactions between the different populations using CellPhoneDB, a repository of ligand-receptor complexes that can predict enriched cellular interactions based on the expression of ligands and receptors in cell populations (Efremova et al., 2020). The outermost population was highly enriched for fibronectin (FN1), laminin (LAMA3 and LAMC1) and collagen (COL8A1 and COL4A1) expression, extracellular matrix (ECM) proteins that can interact with the integrins expressed in the middle and inner populations (Figure 3). Specifically, interactions of fibronectin and laminin with the α3β1 integrin modulate cell adhesion to the ECM and cell motility (Meng et al., 2009; Hamill et al., 2010; Jia et al., 2010; Zhang et al., 2017). Interestingly, this analysis predicted multiple interactions in the Ephrin-signaling pathway, in which ligands and receptors activate bidirectional signals that can lead to somewhat paradoxical downstream effects (Pasquale 2008). To illustrate, cells in the outermost and middle populations expressed the EphB4 receptor and its ligand EphrinB2 (EFNB2) (Supplementary Figure S6). Activation of EphB4 induces cell migration and invasion in cancer cells (Steinle et al., 2002; Kumar et al., 2006; Nai-Ying Yang et al., 2006), although the exact opposite effect has also been reported (Noren et al., 2006). Additionally, reverse signaling through EphrinB2 can stimulate cell migration through the PI3K pathway (Steinle et al., 2002; Kumar et al., 2006).
FIGURE 3. Cell-cell interactions between cells in various patch regions labeled with the smaller bandwidth. Interactions were inferred based on the expression of ligands and receptors in the different cell populations. The first molecule in each interaction pair (rows) corresponds to the first region in each population pair (columns). Circles scaled by the significance of the interaction and colored by the average expression level of ligand and receptor.
Finally, CellPhoneDB inferred enrichment of multiple EMT inducers and their receptors in the outermost population, such as tumor necrosis factor (TNFA) and genes involved in the EGF pathway (CD44, EGFR, EPGN, HBEGF) (Cheng et al., 2012; Revenco et al., 2019; Cook and Vanderhyden 2020). Conversely, cells in the center of the patch were enriched for DSC2 and DSG2, genes that encode components of desmosome cell-cell junctions (Garrod and Chidgey 2008; Nekrasova and Green 2013), hallmarks of an epithelial phenotype. Taken together, the identified cell-cell interactions indicated that cells at the migrating front responded to their local microenvironment and stimulated similar invasive behavior in neighboring regions.
Intratumor heterogeneity is a major challenge for effective cancer treatment. Single-cell genomics and transcriptomics proved themselves valuable methods to study this heterogeneity, but lack information about the spatial organization of cells. Recently, various spatial transcriptomics methods have been developed to add positional information from tissue sections to the single-cell transcriptomes (Ståhl et al., 2016; Vickovic et al., 2019; Stickels et al., 2021), providing numerous insights in cancer biology and other fields (Longo et al., 2021). However, these methods either lack single cell resolution or have substantially lower transcript counts per cell than conventional scRNA-seq. Here, we applied our recently developed FUNseq technology to spatially profile confined tumor regions. The strength of this method lies in the combination of labeling tumor regions guided by live-cell imaging and deep sequencing of single cells. This allowed us to profile gene expression in isolated tumor regions using 34,000 transcripts per cell (Supplementary Figure S2), compared to the 494 and 11.5 transcripts per 10 μm bead for Slide-seq V2 and HDST, respectively (Stickels et al., 2021). The increased sensitivity of FUNseq allows us to study low abundance transcripts, enabling deep characterization of tumor cells.
We profiled tumor heterogeneity in an in vitro tumor model (McFaline-Figueroa et al., 2019) by annotating cells located at different distances from the center of a 2D epithelial cell mass. Cells in the outermost layer or invasive edge (∼10 cell wide band) of this patch were progressing through EMT, suggesting that these cells sense their local microenvironment and acquire a mesenchymal phenotype to migrate to unoccupied areas of the dish. Taking advantage of the FUNseq’s deep sequencing, we characterized cell-cell interaction networks between the different tumor regions. We identified various interactions between outermost cells and ECM components that can stimulate cell migration and we showed that outermost cells are enriched for ligands and receptors that can stimulate EMT, such as components of the Ephrin, EGF and VEGF signaling pathways.
By combining phototagging of confined tumor regions and deep sequencing of single cells, we characterized the transcriptomic heterogeneity in a population of untransformed epithelial cells. To fully explore the potential of FUNseq, the next step would be to profile tumor sections, which have much higher complexity than relatively homogeneous cell lines. We envision that FUNseq might address important questions about intratumor heterogeneity, such as how tumor cells interact with the tumor microenvironment and how tumor composition affects treatment outcome.
In summary, we demonstrated that FUNseq can spatially annotate and profile subpopulations of an in vitro tumor model. We showed that cells at the invasive edge (∼10 cells wide band) of a high-confluence patch of cells underwent EMT, migrated to low-confluence areas and induced similar phenotypic plasticity in neighboring cells. Spatially profiling tumor cells using FUNseq enables deep characterization of intratumor heterogeneity, thereby laying the foundation for a more complete understanding of tumor biology.
MCF10A_H2B_GFP human breast epithelial cells were a kind gift of Reuven Agami (Netherlands Cancer Institute). Cells were cultured at 37°C and 5% CO2 in DMEM/F12 medium without phenol red (Gibco), supplemented with 5% Donor Equine Serum, 1% penicillin/streptomycin, 20 ng/ml EGF, 500 ng/ml hydrocortisone, 100 ng/ml cholera toxin and 10 μg/ml insulin.
Before conducting experiments, cells were seeded on 20 mm glass bottom dishes (Cellvis), coated with 0.1 mg/ml fibronectin (EMD Millipore). 10,000 cells were seeded in a droplet in the center of the dish, such that a circular patch of cells was formed in the center of the dish. After 4.5 h, dishes were washed with Dulbecco’s PBS (Sigma) to remove non-adherent cells. The patch of cells was then cultured in MCF10A medium at 37°C and 5% CO2 for 6 days.
Cell labeling was performed on the Ultrawide Field-of-view Optical (UFO) microscope developed previously (You et al., 2021). Cells were incubated with 40 µM photoactivatable Janelia Fluor 646 (JF646) dye (Tocris) for 20 min and washed with MCF10A culture medium. Bright-field images were used to localize the patch of cells, after which we identified the cells to be labeled using a low-resolution or high-resolution approach. In the low-resolution tagging approach, we fit three concentric rings with equal bandwidth (1,000–1,500 µm bandwidth) in the area of the patch. In the high-resolution approach, we divide the patch of cells in three layers: the outermost 250 µm of cells (∼10 cell wide band), the next 250 μm, and the inside of the patch.
In both approaches, the outer population of cells was then selectively illuminated for 2 min with 405 nm light using a digital micromirror device (DMD), thereby phototagging these cells with JF646. Next, cells were incubated with 40 µM photoactivatable Janelia Fluor 549 (JF549) dye (Grimm et al., 2016) (Tocris) for 20 min and washed with MCF10A culture medium. The imaging and labeling process was repeated, but now illuminating the middle population of cells. These cells are thus phototagged with JF549 and JF646, as both dyes are present in the cytoplasm and become activated upon illumination. For visualization purposes, image background was subtracted and image contrast was adjusted using ImageJ.
Cells were harvested using trypsin-EDTA without phenol red (Sigma), centrifuged and resuspended in HBSS buffer (Gibco). Live single cells (validated by Draq7 viability staining) were sorted into 384-well plates using the BD FACSMelody Cell Sorter (BD Biosciences), spun-down and stored at −80°C. Library preparation and single-cell RNA sequencing was performed by Single Cell Discoveries (Utrecht, Netherlands) using their custom SORT-seq protocol (Muraro et al., 2016). cDNA libraries were sequenced at 150 k reads/cell on the Illumina NextSeq 500 platform.
scRNA-seq data was aligned and preprocessed by Single Cell Discoveries as described by Muraro et al. (2016). Gene expression matrices were processed using Seurat v4 (Hao et al., 2021). Cells containing 2,000–9,000 features and less than 40% mitochondrial genes were selected. Gene expression was either normalized using the SCTransform (Hafemeister and Satija 2019) function for dimensionality reduction, or log-normalized for all other downstream analysis. Cell cycle scoring and regression was performed using a set of G2/M and S phase markers (Tirosh et al., 2016). We performed a Principal Component Analysis (PCA) on the normalized gene expression data and used the first 40 principal components for dimensionality reduction using UMAP.
Differentially expressed genes between the inner and outer populations were identified with Seurat’s findMarkers function using a Wilcoxon rank-sum test and filtering for genes with a Bonferroni corrected p-value < 1 × 10−5. Genes with log2 fold change >0.5 were marked as upregulated and genes with log2 fold change <−0.5 were marked as downregulated. Next, overrepresentation analysis (ORA) was performed with the ClusterProfiler v4 package (Wu et al., 2021). The enricher function was used with default settings (one-sided Fisher’s exact test with Benjamini-Hochberg adjusted p-values) and the most significantly enriched processes were visualized.
To calculate the level of EMT in each cell, we followed the approach of Sacchetti et al. (2021) Gene Set Variation analysis was performed using the GSVA package (Hänzelmann et al., 2013), where we used a set of EMT markers that is publicly available from the Nanostring nCounter PanCancer Progression Panel (Cesano 2015). This gene set contained 65 epithelial (E) and 115 mesenchymal (M) genes (Supplementary Table S1). For each cell we calculated its GSVA enrichment scores for the epithelial and mesenchymal genes, after which we subtracted the E score from the M score to define the cell’s EMT score.
Enriched ligand-receptor interactions between the different populations of cells were inferred using the CellphoneDB package (Efremova et al., 2020). This analysis uses empirical shuffling to identify enriched ligand-receptor interactions based on the expression levels in the different populations, while requiring that all subunits from heteromeric ligand-receptor complexes are expressed. Log-normalized gene expression matrices were used as input files and the statistical analysis (without subsampling) was performed using a p-value threshold of .01 and requiring that at least 20% of the cells in a population expresses a specific ligand-receptor interaction. To identify highly specific interactions between populations, we filtered for interactions with rank ≤.444. In this way, we filtered for ligand-receptor interactions that were significantly enriched in ≤4 population pairs (out of 9 population pairs in our setup). After this initial prioritization of the predicted interactions, we manually selected biologically relevant interactions for visualization.
TThe raw data used to generate for this study can be found at NCBI’s GEO DataSets site with an ID number of GSE196245.
MS conducted the experiments and performed the single cell RNA sequencing and CellPhoneDB analysis. KF conducted part of the experiments and scripted the single cell RNA sequencing analysis code. LY scripted the photopatterned code and performed some of the image analysis. JS upgraded the microscope and program for the spatial phototagging experiment. YB helped with the whole FUNseq pipeline and cell sorting. CB contributed to part of the cell culture preparation for the experiments. MS, KF, and M-PC designed most of the experiments. MS and M-PC wrote the paper with input from all authors. M-PC contributed to and supervised all aspects of the project.
The authors declare that the research was conducted in the absence of any commercial or financial relationships that could be construed as a potential conflict of interest.
All claims expressed in this article are solely those of the authors and do not necessarily represent those of their affiliated organizations, or those of the publisher, the editors and the reviewers. Any product that may be evaluated in this article, or claim that may be made by its manufacturer, is not guaranteed or endorsed by the publisher.
M-PC acknowledges support from the Oncode Institute, Cancer GenomiCs.nl (CGC), NWO (Netherlands Organization for Scientific Research) Veni Grant, Stichting Ammodo and Erasmus MC grant. M-PC appreciates Josephine Nefkens Stichting’s support on the UFO microscope. We thank Reuven Agami for the kind gift of the MCF10A-H2B-GFP cell line.
The Supplementary Material for this article can be found online at: https://www.frontiersin.org/articles/10.3389/fbioe.2022.829509/full#supplementary-material
Berglund, E., Jonas, M., Schultz, N., Friedrich, S., Marklund, M., Joseph, B., et al. (2018). Spatial Maps of Prostate Cancer Transcriptomes Reveal an Unexplored Landscape of Heterogeneity. Nat. Commun. 9, 2419. doi:10.1038/s41467-018-04724-5
Bhattacharya, R., Fan, F., Wang, R., Ye, X., Xia, L., Boulbes, D., et al. (2017). Intracrine VEGF Signalling Mediates Colorectal Cancer Cell Migration and Invasion. Br. J. Cancer 117 (6), 848–855. doi:10.1038/bjc.2017.238
Burrell, R. A., McGranahan, N., Bartek, J., and Swanton, C. (2013). The Causes and Consequences of Genetic Heterogeneity in Cancer Evolution. Nature 501, 338–345. doi:10.1038/nature12625
Cesano, A. (2015). nCounter(®) PanCancer Immune Profiling Panel (NanoString Technologies, Inc., Seattle, WA). J. Immunother. Cancer 3, 42–43. doi:10.1186/s40425-015-0088-7
Cheng, J.-C., Auersperg, N., Leung, P. C. K., and Leung, K. (2012). EGF-induced EMT and Invasiveness in Serous Borderline Ovarian Tumor Cells: A Possible Step in the Transition to Low-Grade Serous Carcinoma Cells? PLOS ONE 7, e34071. doi:10.1371/journal.pone.0034071
Cook, D. P., and Vanderhyden, B. C. (2020). Context Specificity of the EMT Transcriptional Response. Nat. Commun. 11, 2142–2149. doi:10.1038/s41467-020-16066-2
Efremova, M., Vento-Tormo, M., Teichmann, S. A., and Vento-Tormo, R. (20202020). CellPhoneDB: Inferring Cell-Cell Communication from Combined Expression of Multi-Subunit Ligand-Receptor Complexes. Nat. Protoc. 15 (4), 1484–1506. doi:10.1038/s41596-020-0292-x
Garrod, D., and Chidgey, M. (2008). Desmosome Structure, Composition and Function. Biochim. Biophys. Acta (Bba) - Biomembranes 1778, 572–587. doi:10.1016/j.bbamem.2007.07.014
Gerlinger, M., Rowan, A. J., Horswell, S., Larkin, J., Endesfelder, D., Gronroos, E., et al. (2012). Intratumor Heterogeneity and Branched Evolution Revealed by Multiregion Sequencing. N. Engl. J. Med. 366, 883–892. doi:10.1056/nejmoa1113205
Goel, H. L., and Mercurio, A. M. (2013). VEGF Targets the Tumour Cell. Nat. Rev. Cancer 13 (12), 871–882. doi:10.1038/nrc3627
Gonzalez-Moreno, O., Lecanda, J., Green, J. E., Segura, V., Catena, R., Serrano, D., et al. (2010). VEGF Elicits Epithelial-Mesenchymal Transition (EMT) in Prostate Intraepithelial Neoplasia (PIN)-like Cells via an Autocrine Loop. Exp. Cell Res 316, 554–567. doi:10.1016/j.yexcr.2009.11.020
Grimm, J., English, B., and Choi, H. (2016). Bright Photoactivatable Fluorophores for Single-Molecule Imaging. Nat. Methods 13, 985–988. doi:10.1038/nmeth.4034
Hafemeister, C., and Satija, R. (2019). Normalization and Variance Stabilization of Single-Cell RNA-Seq Data Using Regularized Negative Binomial Regression. Genome Biol. 20 (1), 1–15. doi:10.1186/s13059-019-1874-1
Hamill, K. J., Paller, A. S., and Jones., J. C. (2010). Adhesion and Migration, the Diverse Functions of the Laminin Alpha3 Subunit. Dermatol. Clin. 28, 79–87. doi:10.1016/j.det.2009.10.009
Hänzelmann, S., Castelo, R., and Guinney, J. (20132013). GSVA: Gene Set Variation Analysis for Microarray and RNA-Seq Data. BMC Bioinformatics 14 (1), 7–15. doi:10.1186/1471-2105-14-7
Hao, Y., Hao, S., Andersen-Nissen, E., Mauck, W. M., Zheng, S., Butler, A., et al. (2021). Integrated Analysis of Multimodal Single-Cell Data. Cell 184, 3573–3587. doi:10.1016/j.cell.2021.04.048
Hashimshony, T., Senderovich, N., Avital, G., Klochendler, A., de Leeuw, Y., Anavy, L., et al. (2016). CEL-Seq2: Sensitive Highly-Multiplexed Single-Cell RNA-Seq. Genome Biol. 17, 77–7. doi:10.1186/s13059-016-0938-8
Jia, D., Yan, M., Wang, X., Hao, X., Liang, L., Liu, L., et al. (2010). Development of a Highly Metastatic Model that Reveals a Crucial Role of Fibronectin in Lung Cancer Cell Migration and Invasion. BMC Cancer 10, 1–12. doi:10.1186/1471-2407-10-364
Kumar, S. R., Singh, J., Xia, G., Krasnoperov, V., Hassanieh, L., Ley, E. J., et al. (2006). Receptor Tyrosine Kinase EphB4 Is a Survival Factor in Breast Cancer. Am. J. Pathol. 169, 279–293. doi:10.2353/ajpath.2006.050889
Lawson, D. A., Kessenbrock, K., Davis, R. T., Pervolarakis, N., and Werb, Z. (2018). Tumour Heterogeneity and Metastasis at Single-Cell Resolution. Nat. Cell Biol 20 (12), 1349–1360. doi:10.1038/s41556-018-0236-7
Liberzon, A., Birger, C., Thorvaldsdóttir, H., Ghandi, M., Mesirov, J. P., and Tamayo, P. (2015). The Molecular Signatures Database (MSigDB) Hallmark Gene Set Collection. Cell Syst 1, 417–425. doi:10.1016/j.cels.2015.12.004
Longo, S. K., Guo, M. G., Ji, A. L., and Khavari, P. A. (2021). Integrating Single-Cell and Spatial Transcriptomics to Elucidate Intercellular Tissue Dynamics. Nat. Rev. Genet. 22, 627–644. doi:10.1038/s41576-021-00370-8
Luo, M., Hou, L., Li, J., Shao, S., Huang, S., Meng, D., et al. (2016). VEGF/NRP-1 axis Promotes Progression of Breast Cancer via Enhancement of Epithelial-Mesenchymal Transition and Activation of NF-Κb and β-catenin. Cancer Lett. 373, 1–11. doi:10.1016/j.canlet.2016.01.010
Martens, M., Ammar, A., Riutta, A., Waagmeester, A., Slenter, D. N., Hanspers, K., et al. (2021). WikiPathways: Connecting Communities. Nucleic Acids Res. 49, D613–D621. doi:10.1093/nar/gkaa1024
McFaline-Figueroa, J. L., Hill, A. J., Qiu, X., Jackson, D., Shendure, J., and Trapnell, C. (2019). A Pooled Single-Cell Genetic Screen Identifies Regulatory Checkpoints in the Continuum of the Epithelial-To-Mesenchymal Transition. Nat. Genet. 51, 1389–1398. doi:10.1038/s41588-019-0489-5
McInnes, L., Healy, J., and James, M. (2018). UMAP: Uniform Manifold Approximation and Projection for Dimension Reduction. J. Open Source Softw. 3, 861. doi:10.21105/joss.00861
Meng, X. N., Jin, Y., Yu, Y., Bai, J., Liu, G. Y., Zhu, J., et al. (2009). Characterisation of Fibronectin-Mediated FAK Signalling Pathways in Lung Cancer Cell Migration and Invasion. Br. J. Cancer 101 (2), 327–334. doi:10.1038/sj.bjc.6605154
Morrissy, A. S., Cavalli, F. M. G., Remke, M., Ramaswamy, V., Shih, D. J. H., Holgado, B. L., et al. (2017). Spatial Heterogeneity in Medulloblastoma. Nat. Genet. 49, 780–788. doi:10.1038/ng.3838
Muraro, M. J., Dharmadhikari, G., Grün, D., Groen, N., Dielen, T., Jansen, E., et al. (2016). A Single-Cell Transcriptome Atlas of the Human Pancreas. Cell Syst. 3, 385–394. doi:10.1016/j.cels.2016.09.002
Navin, N., Kendall, J., Troge, J., Andrews, P., Rodgers, L., McIndoo, J., et al. (2011). Tumour Evolution Inferred by Single-Cell Sequencing. Nature 472 (7341), 90–94. doi:10.1038/nature09807
Nekrasova, O., and Green, K. J. (2013). Desmosome Assembly and Dynamics. Trends Cell Biol. 23, 537–546. doi:10.1016/j.tcb.2013.06.004
Nieto, M. A., Huang, R. Y.-J., Jackson, R. A., and Thiery, J. P. (2016). Emt: 2016. Cell 166, 21–45. doi:10.1016/j.cell.2016.06.028
Noren, N. K., Foos, G., Hauser, C. A., and Pasquale, E. B. (2006). The EphB4 Receptor Suppresses Breast Cancer Cell Tumorigenicity through an Abl-Crk Pathway. Nat. Cell Biol 8, 815–825. doi:10.1038/ncb1438
Pasquale, E. B. (2008). Eph-Ephrin Bidirectional Signaling in Physiology and Disease. Cell 133, 38–52. doi:10.1016/j.cell.2008.03.011
Pastushenko, I., Brisebarre, A., Sifrim, A., Fioramonti, M., Revenco, T., Boumahdi, S., et al. (2018). Identification of the Tumour Transition States Occurring during EMT. Nature 556, 463–468. doi:10.1038/s41586-018-0040-3
Pastushenko, I., and Blanpain, C. (2019). EMT Transition States during Tumor Progression and Metastasis. Trends Cell Biol. 29, 212–226. doi:10.1016/j.tcb.2018.12.001
Patel, A. P., Tirosh, I., Trombetta, J. J., Shalek, A. K., Gillespie, S. M., Wakimoto, H., et al. (2014). Single-cell RNA-Seq Highlights Intratumoral Heterogeneity in Primary Glioblastoma. Science 344, 1396–1401. doi:10.1126/science.1254257
Puram, S. V., Tirosh, I., Parikh, A. S., Patel, A. P., Yizhak, K., Gillespie, S., et al. (2017). Single-Cell Transcriptomic Analysis of Primary and Metastatic Tumor Ecosystems in Head and Neck Cancer. Cell 171, 1611–1624. doi:10.1016/j.cell.2017.10.044
Revenco, T., Nicodème, A., Pastushenko, I., Sznurkowska, M. K., Latil, M., Sotiropoulou, P. A., et al. (2019). Context Dependency of Epithelial-To-Mesenchymal Transition for Metastasis. Cell Rep. 29, 1458–1468. doi:10.1016/j.celrep.2019.09.081
Sacchetti, A., Teeuwssen, M., Verhagen, M., Joosten, R., Xu, T., Stabile, R., et al. (2021). Phenotypic Plasticity Underlies Local Invasion and Distant Metastasis in colon Cancer. eLife 10, e61461. doi:10.7554/elife.61461
Sottoriva, A., Spiteri, I., Piccirillo, S. G. M., Touloumis, A., Collins, V. P., Marioni, J. C., et al. (2013). Intratumor Heterogeneity in Human Glioblastoma Reflects Cancer Evolutionary Dynamics. Proc. Natl. Acad. Sci. 110, 4009–4014. doi:10.1073/pnas.1219747110
Ståhl, P. L., Salmén, F., Vickovic, S., Lundmark, A., Navarro, J. F., Magnusson, J., et al. (2016). Visualization and Analysis of Gene Expression in Tissue Sections by Spatial Transcriptomics. Science 353, 78–82. doi:10.1126/science.aaf2403
Steinle, J. J., Meininger, C. J., Forough, R., Wu, G., Wu, M. H., and Granger, H. J. (2002). Eph B4 Receptor Signaling Mediates Endothelial Cell Migration and Proliferation via the Phosphatidylinositol 3-Kinase Pathway. J. Biol. Chem. 277, 43830–43835. doi:10.1074/jbc.m207221200
Stickels, R. R., Murray, E., Kumar, P., Li, J., Marshall, J. L., Di Bella, D. J., et al. (2021). Highly Sensitive Spatial Transcriptomics at Near-Cellular Resolution with Slide-seqV2. Nat. Biotechnol. 39, 313–319. doi:10.1038/s41587-020-0739-1
Tirosh, I., Izar, B., Prakadan, S. M., Wadsworth, M. H., Treacy, D., Trombetta, J. J., et al. (2016). Dissecting the Multicellular Ecosystem of Metastatic Melanoma by Single-Cell RNA-Seq. Science 352, 189–196. doi:10.1126/science.aad0501
Vickovic, S., Eraslan, G., Salmén, F., Klughammer, J., Stenbeck, L., Schapiro, D., et al. (2019). High-definition Spatial Transcriptomics for In Situ Tissue Profiling. Nat. Methods 16, 987–990. doi:10.1038/s41592-019-0548-y
Vuoriluoto, K., Haugen, H., Kiviluoto, S., Mpindi, J.-P., Nevo, J., Gjerdrum, C., et al. (2011). Vimentin Regulates EMT Induction by Slug and Oncogenic H-Ras and Migration by Governing Axl Expression in Breast Cancer. Oncogene 30, 1436–1448. doi:10.1038/onc.2010.509
Wu, T., Hu, E., Xu, S., Chen, M., Guo, P., Dai, Z., et al. (2021). clusterProfiler 4.0: A Universal Enrichment Tool for Interpreting Omics Data. Innovation (N Y) 2, 100141. doi:10.1016/j.xinn.2021.100141
Yachida, S., Jones, S., Bozic, I., Antal, T., Leary, R., Fu, B., et al. (2010). Distant Metastasis Occurs Late during the Genetic Evolution of Pancreatic Cancer. Nature 467 (7319), 1114–1117. doi:10.1038/nature09515
Yang, A. D., Camp, E. R., Fan, F., Shen, L., Gray, M. J., Liu, W., et al. (2006). Vascular Endothelial Growth Factor Receptor-1 Activation Mediates Epithelial to Mesenchymal Transition in Human Pancreatic Carcinoma Cells. Cancer Res. 66, 46–51. doi:10.1158/0008-5472.can-05-3086
Yang, J., Antin, P., Berx, G., Blanpain, C., Brabletz, T., Bronner, M., et al. (2020). Guidelines and Definitions for Research on Epithelial-Mesenchymal Transition. Nat. Rev. Mol. Cell Biol 21, 341–352. doi:10.1038/s41580-020-0237-9
Yang, N.-Y., Pasquale, E. B., Owen, L. B., and Ethell, I. M. (2006). The EphB4 Receptor-Tyrosine Kinase Promotes the Migration of Melanoma Cells through Rho-Mediated Actin Cytoskeleton Reorganization. J. Biol. Chem. 281, 32574–32586. doi:10.1074/jbc.m604338200
You, L., Su, P.-R., Betjes, M., Rad, R. G., Beerens, C., van Oosten, E., et al. (2021). Functional Single Cell Selection and Annotated Profiling of Dynamically Changing Cancer Cells. Nat. Biomed. Eng. In Press. doi:10.1101/2021.10.12.464054
Zhang, Y., Xi, S., Chen, J., Zhou, D., Gao, H., Zhou, Z., et al. (2017). Overexpression of LAMC1 Predicts Poor Prognosis and Enhances Tumor Cell Invasion and Migration in Hepatocellular Carcinoma. J. Cancer 8, 2992–3000. doi:10.7150/jca.21038
Keywords: spatial transcriptomics, single cell sequencing, functional single cell sequencing, intratumoral heterogeneity, epithelial-to-mesenchym transition (EMT)
Citation: Smit MM, Feller KJ, You L, Storteboom J, Begce Y, Beerens C and Chien M-P (2022) Spatially Annotated Single Cell Sequencing for Unraveling Intratumor Heterogeneity. Front. Bioeng. Biotechnol. 10:829509. doi: 10.3389/fbioe.2022.829509
Received: 05 December 2021; Accepted: 11 January 2022;
Published: 22 February 2022.
Edited by:
Zhaoyuan Fang, Zhejiang University, ChinaReviewed by:
Jianhua Xing, University of Pittsburgh, United StatesCopyright © 2022 Smit, Feller, You, Storteboom, Begce, Beerens and Chien. This is an open-access article distributed under the terms of the Creative Commons Attribution License (CC BY). The use, distribution or reproduction in other forums is permitted, provided the original author(s) and the copyright owner(s) are credited and that the original publication in this journal is cited, in accordance with accepted academic practice. No use, distribution or reproduction is permitted which does not comply with these terms.
*Correspondence: Miao-Ping Chien, bS5wLmNoaWVuQGVyYXNtdXNtYy5ubA==
†These authors share senior authorship
Disclaimer: All claims expressed in this article are solely those of the authors and do not necessarily represent those of their affiliated organizations, or those of the publisher, the editors and the reviewers. Any product that may be evaluated in this article or claim that may be made by its manufacturer is not guaranteed or endorsed by the publisher.
Research integrity at Frontiers
Learn more about the work of our research integrity team to safeguard the quality of each article we publish.