- 1Division of Experimental Medicine, McGill University, Montreal, QC, Canada
- 2Department of Chemical Engineering, McGill University, Montreal, QC, Canada
- 3Department of Biomedical Engineering, McGill University, Montreal, QC, Canada
Organs-on-a-chip have emerged as next-generation tissue engineered models to accurately capture realistic human tissue behaviour, thereby addressing many of the challenges associated with using animal models in research. Mechanical features of the culture environment have emerged as being critically important in designing organs-on-a-chip, as they play important roles in both stimulating realistic tissue formation and function, as well as capturing integrative elements of homeostasis, tissue function, and tissue degeneration in response to external insult and injury. Despite the demonstrated impact of incorporating mechanical cues in these models, strategies to measure these mechanical tissue features in microfluidically-compatible formats directly on-chip are relatively limited. In this review, we first describe general microfluidically-compatible Organs-on-a-chip sensing strategies, and categorize these advances based on the specific advantages of incorporating them on-chip. We then consider foundational and recent advances in mechanical analysis techniques spanning cellular to tissue length scales; and discuss their integration into Organs-on-a-chips for more effective drug screening, disease modeling, and characterization of biological dynamics.
1 Introduction
Organs-on-a-chip (OoC) are microengineered cell and tissue culture platforms that drive cells to emulate highly realistic phenotypes, by recreating various features of the organ microenvironment (Leung et al., 2022). These microengineered tissues can then be used for drug testing, disease modeling, toxicology, and personalized medicine (Ingber, 2022). Studies over the last decade have suggested strong potential for these models in to capture relevant physiological or disease processes in a format that can be easily imaged and manipulated. Various OoCs have been developed to recreate the lung, blood vessel, heart, liver, gut, muscle, blood-brain barrier, skin barrier, amongst many others (Zhang et al., 2018); and have also been interconnected to evaluate organ-organ interactions in multi-organs on-a-chip and body-on-a-chip systems (Skardal et al., 2016; Ronaldson-Bouchard and Vunjak-Novakovic, 2018; Vogt, 2022).
The creation of a physiological or pathophysiological biomechanical environment is essential to successful recapitulation in vivo behavior in OoCs models (Clarke et al., 2021; Fuchs et al., 2021). In vivo, cells are exposed to biochemical and biomechanical stimuli critical for tissue development and cell response (Moraes et al., 2011), and recreating biomechanical cues such as substrate stiffness, geometric confinement, topography, stretch, compression, fluid shear stress, interstitial fluid flow, and hydrostatic pressure will likely continue to be essential components of OoC development (Zhang et al., 2018). As illustrative examples, lung-on-a-chip models incorporate cyclic mechanical strain mimicking the breathing motions which is essential in obtaining a physiological inflammatory response (Huh et al., 2012; Stucki et al., 2015; Huang et al., 2019; Zhu et al., 2022); Intestine-on-a-chip models incorporate peristalsis-like mechanical stretch to create a robust barrier to bacterial infection (Kasendra et al., 2018; Jalili-Firoozinezhad et al., 2019); and including shear stress in heart-on-a-chip and kidney-on-a-chip models alters inflammatory responses and drug uptake respectively (Lee et al., 2018; Ferrell et al., 2019).
Conversely, cells actively respond to these mechanical cues to further modulate the local mechanical microenvironment, in a tissue maturation feedback loop driven by the initial mechanical culture environment (Ergir et al., 2018). Monitoring these tissue features can provide important information about the state of development of the model, as well as the ways in which the model responds to toxicity and biochemical or biomechanical injury (Thompson et al., 2020). Recently, considerable focus has been placed on integrating biosensors directly into OoCs, to allow data collection in a non-invasive and continuous manner, with the potential to multiplex measurements over extended periods of time (Clarke et al., 2021; Fuchs et al., 2021). Biosensors have been developed to measure biochemical signals as metabolite concentration and barrier integrity, while other platforms quantify mechanics as stiffness and forces (Adam Kratz et al., 2019; Ferrari et al., 2020). Despite this focus, and the broad variety of electrochemical, optical, mechanical, acoustic, bead-based, and magnetic sensors that have been developed, demonstrations of their integration into OoCs have been relatively limited (Kilic et al., 2018; Rothbauer and Ertl, 2020). In this review, we briefly select sensors that have been integrated into OoCs and categorize these advances based on their utility for on-a-chip studies. We then consider foundational and recent advances in mechanical analysis techniques spanning cellular to tissue length scales and discuss their integration into OoCs for more effective drug screening, quantitative disease modeling, and characterization of biological dynamics in tissue fate and function.
2 Specific benefits of integrated sensors in the OoC context
Sensors and measurement tools play an essential role in interrogating all biological systems, and are often integrated into microfluidics to enhance their sensitivity, limits of detection, and utility in precious sample processing (Young and Moraes, 2015). However, some unique advantages arise from integrating sensing technologies directly into OoCs. In this section we identify and highlight the impact of these core measurement advantages using selected examples of OoC-sensor integrations.
2.1 Real-time measurements
In contrast with standard animal models, OoCs offer the promise of visualizing realistic cell behaviour and complex biological processes. The OoC structure allows both slow processes such as leukocyte infiltration (Gjorevski et al., 2020), or rapid processes such as fibrotic tissue stiffening (Asmani et al., 2018), to be recreated and observed directly on-chip; and measuring such phenomena via embedded sensors could offer crucial real-time insight into how biological systems evolve during homeostasis, pathology, and in response to candidate therapeutics (Soucy et al., 2019). Ideally, these real-time measurements would also be non-destructive to support repeated analysis of the same precious sample material; and would not interfere with the biological process itself (Wu et al., 2020; Danku et al., 2022). Direct measurement of transepithelial/transendothelial electrical resistance (TEER) across cell-generated barriers has emerged as an example of this, and is used to assess barrier integrity, drug toxicity, and transport phenomena (Park et al., 2019; Bossink et al., 2021) under physiologically-relevant insult and injury (van der Helm et al., 2019). This approach has been applied to probe on-chip models of gut (Henry et al., 2017), lung (Walter et al., 2016), skin (Alexander et al., 2018), retina (Chen et al., 2020), heart (Maoz et al., 2017), and the blood-brain barrier in response to shear stress (Tu et al., 2021).
Electrochemistry-based sensors have also emerged as key strategies in the context of real-time measurements, as they offer high measurement sensitivity, low limits of detection, and high selectivity, particularly when coupled with analyte-detection technologies (Adam Kratz et al., 2019). These approaches have been used to measure secreted analytes via antigen- (Lee et al., 2021), aptamer- (Shin et al., 2016; Tran et al., 2021) (Tran et al., 2021), enzyme- (Bavli et al., 2016), and bead- (Riahi et al., 2016) based binding assays. Ortega et al. (2019) recently used these approaches to measure real-time release of myokines from a muscle-on-a-chip, and demonstrated that tissue response to electrical stimulation specifically is highly transient and disappears within 30 min, further emphasizing the impact of incorporating real-time sensing on-chip.
2.2 Multiplexed measurements
Operating an organ-on-a-chip requires technical skill and ability, and failed experiments are common at this relatively early stage of the technology lifecycle. Hence, extracting multiple measurements from the same sample is crucially important in understanding the complex biological system emerging from disparate components (Picollet-D’hahan et al., 2021), but also providing sufficient return-on-investment for the system. Furthermore, the integrative nature of biology suggests that multimodal measurements are essential to capture disease processes and functional therapeutic activity (Ort et al., 2021). An excellent example of this is work by Zhang et al., who demonstrated an integrated platform of multiple organoid microbioreactors with integrated physical sensors to monitor pH, O2, and temperature; electrochemical sensors to measure protein biomarkers; and optical sensors to monitor organoid morphology, allowing them to combine qualitative and quantitative observations and capture holistic function-molecule relationships (Zhang et al., 2017a).
2.3 Spatial measurements
Despite the small volume of a typical OoC culture chamber, understanding the spatial distribution of features within that space is essential to comprehend the impact and evolution of biomarkers in physiological and pathological activity in diseases (Shin et al., 2021). For example, patterns of electrical activity in neural tissues are characteristic of disease state and can be measured via micropatterned multi-electrode arrays (MEA). These sensor arrays were used to be stimulate and measure spatial electrical activity in pre- and post-synaptic neuronal structures (Moutaux et al., 2018). A similar strategy applied to heart-on-a-chip platforms allowed Maoz et al. to integrate both MEA and TEER electrodes on a heart-on-a-chip platform, permitting real-time measurement of electrical activity across the cardiomyocyte cultures (Maoz et al., 2017).
3 Measuring mechanics-on-a-chip
Cell mechanics play a fundamental role in several biological processes, defining fate and function of cells and tissues. Several methods have been developed to quantify and characterize the mechanical microenvironment that cells live in, and have been designed to measure both cell-generated forces and mechanical properties of cells and tissues. Overviews of force measurement and mechanical characterization methods are extensively reviewed elsewhere (Polacheck and Chen, 2016; Roca-Cusachs et al., 2017; Nguyen and Kilian, 2020; Obenaus et al., 2020), and here we focus specifically on the opportunities and challenges that come with integrating these sensors into OoC and microtissue engineered models, and how real-time, multiplexed, and spatially-defined information about tissue function can be obtained.
To meaningfully review the techniques used for measuring mechanics in OoC models, it is helpful to simplify the core principles underlying measurement techniques. To do this, we present a highly simplified view of mechanical characterization techniques, in which systems can be considered as a single spring with spring constant k which undergoes displacement x from an applied force F according to Hooke’s Law F = −kx. Measuring forces (F) requires knowing the stiffness of the deforming spring element, while measuring stiffness (k) requires applying a known force, and measuring the resulting displacement (Figure 1). While this linear, elastic simplification does not capture more complex mechanical behaviours such as viscoelastic or plastic deformations, this simple model can ultimately be extended towards these more challenging measurements by capturing the force, displacement, and stiffness (k) features as a function of time or stress to match the appropriate constitutive model. In the following sections, we highlight how this simple model has been used in OoC and microtissue analysis to improve our understanding of biological systems.
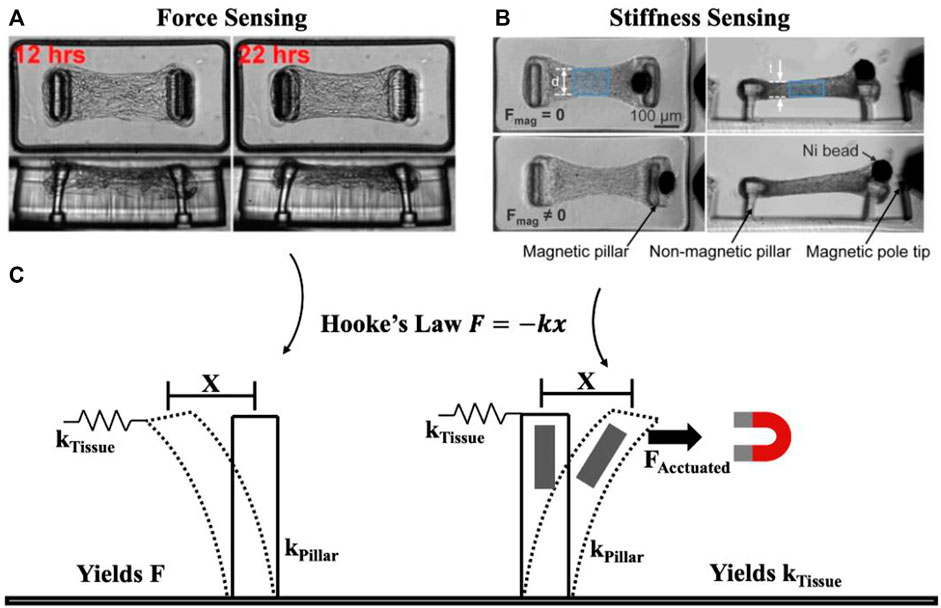
FIGURE 1. Simplified view of underlying principle behind force and mechanical property (e.g., stiffness) measurement. Tissue force sensing (A) and stiffness sensing (B) are both possible using micropillars. Considering pillars to behave as springs, force is measured based on pillar deformation x and pillar spring constant kPillar to yield force F, while stiffness is measured by pillar deformation x as a result of applied force FActuated to yield the spring constant kTissue (C). Essentially, mechanical property measurement requires a form of actuation unlike force sensing. (A) Representative time course imaging of a contracting microtissue. Reprinted with permission from Legant et al. (2009), Proc. Natl. Acad. Sci. 106(25). 10097-10102 (2009). (B) Microtissue before (Fmag = 0) and after (Fmag ≠ 0) magnetic force is applied to stretch the tissue. Reprinted with permission from Zhao et al. (2013), Adv. Mat. 25(12): 1699-1705 (2013). Copyright 2013 John Wiley and Sons.
3.1 Force measurement
Cell-generated forces are known to play critical roles in biological development, stabilizing tissue structure, as well as determining tissue fate and function. Processes like cell migration, muscle contraction, wound healing, morphogenesis, and cancer progression have been found to be fundamentally governed by cell-generated forces, prompting the development of methods to quantify such forces. Measuring cell forces generally relies on relating cell-induced displacements of mechanically characterized substrates to the applied force. Therefore, techniques have been established to quantify forces spanning subcellular to tissue scales, in both 2D and 3D contexts, by measuring the force-controlled deformation of well-defined structures (Figure 1).
The most common technique to quantify contractile forces exerted by adherent cells pulling on a flat 2D surface of known stiffness, referred to as traction force microscopy (TFM) (Pelham and Wang, 1999; Munevar et al., 2001; Wang et al., 2001). Linearly elastic substrates are embedded with fiduciary markers, either as dispersed fluorescent microbeads (Oliver et al., 1998; Dembo and Wang, 1999; Maruthamuthu et al., 2011) or ordered micropatterned arrays (Balaban et al., 2001; Polio et al., 2012) to facilitate stress field computation. Tracking the displacement of these markers in response to cell-generated forces can then be used to obtain a displacement field. If the mechanical properties of the substrate are well known, this field can then be used to calculate the stresses generated by cells. Commonly used materials to make substrates with tunable stiffness include silicones (Lee et al., 1994), PDMS (Yoshie et al., 2018; Yoshie et al., 2019), and hydrogels (Pelham and Wang, 1999), both of which are compatible with current organ-on-a-chip fabrication technologies (Campbell et al., 2021).
Continuous surfaces present a uniform adhesion profile to cells, and this may not replicate the adhesive profile present in many biological systems. To address this, micropillar arrays can be fabricated in silicone rubber with precise control over pillar dimensions and material stiffness. Tracking the deflection of these micropillars can then be used to calculate local stresses at that point (Tan et al., 2003), while independently manipulating the stiffness and adhesive profile presented (Fu et al., 2010). Cells can also be cultured as well-connected colonies on both flat (Trepat et al., 2009; Tambe et al., 2011; Serra-Picamal et al., 2012) and micropillar substrates (Rouredu et al., 2005; Ganz et al., 2006), to understand both cell-cell and cell-substrate forces. Given that these silicone rubber-based fabrication methods are very similar to those commonly used in OoC design, these sensors can be readily integrated into microfluidic systems.
Cell-generated forces can also be measured in more physiologically relevant 3D contexts by embedding cells or tissues in engineered, natural, or composite matrices. As in two-dimensional systems, fiducial markers can be tracked in a 3D deformable matrix as they move due to forces generated by embedded single cells (Legant et al., 2010; Steinwachs et al., 2016; Malandrino et al., 2019) or microtissue constructs of varying geometries (Gjorevski et al., 2015; Leggett et al., 2020; Mark et al., 2020). As these methods are computationally intensive, an alternative strategy has emerged whereby well-defined structures are embedded within a tissue, and deformation of those structures can be used to calculate local stresses. Cantilevers surrounding an engineered microtissue (Figure 1A) (Legant et al., 2009; Thavandiran et al., 2013; Sakar et al., 2016; Chen et al., 2019) (Aoun et al., 2019) can be used to report tensional and compressive stresses at these peripheral sites. This broadly useful strategy can also be used to tune the mechanical features presented to tissues by manipulating tissue geometry (Asmani et al., 2018; Bose et al., 2019). In more recent and complex OoCs, this strategy has been used to measure stresses generated by activation of a neuromuscular junction on-chip (Vila et al., 2021), demonstrating the ability to make real-time, and multiplexed measurements in highly realistic engineered model systems.
Alternative structures to cantilevered pillars have also been used to measure forces on-chip. Silicone films of well-defined thickness will curl as cells cultured on their surface contract, and this approach has been used to study beating myocardial microtissues (Grosberg et al., 2011; Agarwal et al., 2013). Soft silicone pillars can be embedded within tissues to measure stresses during tissue contraction (Moraes et al., 2015). Wires with well-defined material properties can also be embedded at each end of contractile tissues, and the extent of their deflection is correlated with the applied force (Wang et al., 2019; Zhao et al., 2019). Co-culture wire systems have recently been used to study spatially patterned microtissues and effects on myocardial function (Zhao et al., 2019). Current wire-based OoC designs provide perfusable models by integrating a hollow fiber at the core of seeded tissues (Xie et al., 2020), while other designs guide tissue formation in self-assembling constructs (Portillo-Esquivel et al., 2020). In the perfusable hollow fiber model, the fiber walls are observed to contract and deform under the applied load of the tissue surrounding the fiber, highlighting the possibility of measuring tissue-generated forces on the fiber (Xie et al., 2020). For self-assembling tissue constructs, the wire provides an initial supporting structure, and deforms with the tissue during self-assembly, providing a readout of evolving contractility (Portillo-Esquivel et al., 2020). In both designs, mechanical forces can be suggested to play a role in defining overall tissue shape, which is known to regulate biological fate and function. Typical OoC platforms provide the necessary mechanical support to study tissues with varying geometries in 3D, highlighting a unique advantage of such systems. Thus, wire-based designs can further be utilized to study the effects of forces in varying tissue geometries and perfusable systems on-a-chip.
A key limitation in these techniques is that the measurement structure defines the tissue shape and dimensions, and thereby intrinsically changes the system being studied. To avoid this problem, several groups have recently developed dispersible cell-sized mechanosensors with sufficient compliance to resolve cellular scale forces and their spatial patterns within tissues. Oil microdroplets can be used to measure anisotropic forces (Lucio et al., 2017; Serwane et al., 2017), while hydrogel microdroplets can be used to measure isotropic forces like tension and compression (Dolega et al., 2017; Mohagheghian et al., 2018; Mongera et al., 2018; Lee et al., 2019). These sensors are easily embedded and mechanical properties are tuned for appropriate applications, from measuring applied compressive stresses on the order of kilopascals (Dolega et al., 2017) to cell-generated tensile forces (Lee et al., 2019). Fibrous matrices can also be used to resolve cell-scale measurements at the single cell state (Sheets et al., 2016) and in multicellular constructs (Ma et al., 2018; Wang et al., 2020) by deflecting under locally applied forces in the tissue. Since these types of sensors are incorporated within tissues, they can easily be integrated into OoC models to provide higher spatial resolution and multiplexed force readouts in real time.
3.2 Mechanical properties
Mechanical properties of tissues have been consistently proven to be a fundamental factor in cell behavior, tissue morphogenesis, and function (Guimarães et al., 2020), but defining the characteristics of an ideal measurement can be challenging. The length scale at which mechanical properties are measured in biological systems plays an important role as sub-cellular, cellular, and tissue-level measurements can produce distinctive results. Given the heterogenous nature of biological tissues, the precise location of the measurement can also have a considerable impact on the results. Finally, the mechanical model being used to describe a tissue may not adequately capture the tissues’ mechanical behaviour: tissues are often assumed to be linearly elastic and are evaluated by their elastic modulus (E), but more often exhibit time-dependent viscous properties which are much more challenging to measure (Huang et al., 2019). Despite these broad challenges, all methods require the application of a stress or strain on the material being tested, and this can be present some challenges in tightly regulated OoC systems.
Traditional materials characterization techniques of whole-tissue tensile and compression testing can be challenging to integrate with OoC systems, as the scale, enclosed nature, and constraining geometries in most OoCs are not compatible with this equipment. However, they can be applied to certain open systems in which the tissue can be more readily accessed. For example, micro tweezers allow compression testing to measure the bulk stiffness of a tissue (Jaiswal et al., 2017). Exposed surfaces can allow the use of conventional systems that resolve spatial tissue profiles. Conventional indentation tests can be applied at a range of length and depth scales, with indentation tips ranging from ∼10 µm to centimeters (Diez-Perez et al., 2010; Beekmans et al., 2017; Maccabi et al., 2018). Nanoindentation techniques such as atomic force microscopy (AFM) further miniaturize this concept and can measure mechanical properties in regions as small as a single cell (Wu et al., 1998; Oyen, 2013; Chen, 2014). Embedded sensors such as magnetic microparticles can measure the shear modulus of cells within a tissue using magnetic twisting cytometry (Kasza et al., 2011; Coughlin et al., 2013; Zhang et al., 2017b). Emerging technologies may also play a role in these studies, including sound-based acoustic elastography, which can be a minimal-contact strategy to measure mechanical properties by monitoring the surface wave speed, and this technique can be coupled with embedded stress sensors to produce quantitative results (Zhang and Greenleaf, 2007; Li et al., 2012; Kennedy et al., 2015). Brillouin microscopy, a form of optical elastography, uses the scattering of light from vibrational waves to determine the longitudinal modulus which can be related to other mechanical properties (Prevedel et al., 2019; Antonacci et al., 2020).
Each of these strategies require considerable peripheral equipment, and limit the dimensions and characteristics of the tissues being studied. Fortunately, several recent examples have embedded bulk mechanical characterization of microengineered tissues directly into the design of the OoC device. For example, MacQueen et al. (2012) integrated on-chip strain sensors to measure tissue compression in response to deformations applied by pneumatically-actuated compressing micro-platens. This system allowed mechanical characterization of microtissues embedded in a fluidically-controlled device. These systems allow for simultaneous and high-throughput measurement of tissue mechanical properties on-chip, but does require integration of complex electromechanical components for both micro-scale actuation and measurement. Alternatively, passive sensing structures can be used in combination with external actuation systems such as pneumatic- or magnetically-actuated components. For examples, cells adhering to micropillar force-sensing surfaces can be stretched at individual adhesions (Sniadecki et al., 2008) or as a whole (Mann et al., 2012) (Lam et al., 2012) by integrating magnetic nanowires, or stretching the whole array on a pneumatic device. Similarly, cantilevers used for measuring active 3D tissue contractility can be mounted with magnetic beads or bars (Zhao et al., 2013; Xu et al., 2015; Liu et al., 2016), on stretchable frames (Asmani et al., 2018) or on vacuum-actuated chambers to apply deformations to the tissues (Walker et al., 2020).
While actuated cantilevers consider the mechanical behaviour of whole tissues in real time, their spatial resolution is limited and cannot resolve variations at the single cell scale. To do so, sufficiently compliant, actuatable, and measurable sensors must be embedded within tissues. Magnetic ferrofluids were recently incorporated in deformable oil microdroplets, yielding actuatable microdroplets that have the dual purposes of measuring forces when not actuated and measuring stiffness when actuated by a magnetic field (Serwane et al., 2017). Incorporating such sensors inside tissues seeded in OoC platforms can further refine the spatial resolution of multiplexed measurements taken on a chip, providing both force and mechanical property readouts in real time. As a simpler alternative to avoid the experimental complexity of creating and manipulating a uniform magnetic actuating field, thermally actuated size-changing hydrogel beads have been dispersed within microtissues and used to measure stiffness at the length scale of individual cells (Proestaki et al., 2019; Mok et al., 2020). High-stiffness environments limit the expansion of these microgels when the temperature is dropped below 37°C, allowing users to measure the apparent stiffness at the site of sensor inclusion. While these systems have thus far only been used to measure spatially-defined mechanical properties in organisms, organs, and engineered microtissues, their optical addressability and minimal additional infrastructure requirements make them ideally suited to study OoC systems.
4 Perspective and conclusion
Although OoC platforms present a unique opportunity to understand transitions in realistic biological systems and have been popularized for over a decade, the study of mechanically-driven tissue evolution remains relatively limited, especially compared to advances in molecular, genomic, proteomic, and secretory analysis techniques. We believe that while molecular-based analysis techniques are comparatively straightforward to apply to OoCs, the need to both apply and measure forces and displacements do not lend themselves easily to the small volume, enclosed nature, and relatively low robustness of these technologies. Hence, developing new strategies to integrate mechano-sensors that directly address these issues will be of critical importance in establishing the time-resolved, multiplexed, and spatially-defined sensing capabilities that have been particularly impactful in conventional sensor-OoC integrations.
There are several use-cases in which mechanical sensors used in these contexts would be of critical importance. In diseases such as fibrosis and asthma, mechanical changes directly influence disease progression. Fibrosis is the progressive stiffening of tissue by the chronic accumulation of extracellular matrix (ECM), and has been associated with morbidity and mortality in disease affecting the lung, liver, kidney, and heart valve. Recreating the initial architecture and mechanical state of such tissues, the acute and chronic wounding and inflammation-driving stimuli, and the positive feedback cycle of tissue stiffening and cellular remodeling all require advanced OoC-tissue engineering strategies. Coupled with the broad impact of fibrosis-oriented diseases, and the very clear relationship between environmental mechanics and disease progression, tracking these changes via OoC sensors has already been shown to have great potential in drug screening (Asmani et al., 2018). However, the focal nature of this disease strongly suggests that spatially-resolved measurements coupled with physiologically realistic recreation of tissue insult can both help us understand the mechanical nature of disease progression, but also allow us to develop strategies to reverse these conditions, rather than halting disease progression. The slow progression of the disease will also require time-resolved measurements, in which small cumulative changes can be detected as they occur, which is particularly challenging to do in conventional cultures. Finally, the need to understand complex multimodal relationships such as tissue stiffness and oxygenation, strongly suggest that a time-resolved, multimodal, and spatially-defined knowledge of the tissue are essential to address this complex disease.
In contrast to the slow progression of fibrosis, diseases such as asthma involve enhanced force generation and stiffening of the airway smooth muscle cells, contributing to excessive narrowing of the airways and hyperresponsiveness in asthma. These changes occur within minutes, and understanding the capacity of the involved cells to contract, generate forces, and remodel the tissue may provide novel perspectives of the underlying mechanisms of asthma pathophysiology. Understanding these diseases will require high time-resolution measurements to both understand the effectiveness of potential therapies, and the factors that trigger attacks. These tools must therefore rapidly capture a large dynamic range of sensor readings, and will hence have distinctive characteristics over long-term sensors needed for fibrosis studies, highlighting the need to design and integrate application-specific sensors into OoC models.
In addition to understanding the application, it is also helpful in both the mechanical sensor design and development of the scientific application to precisely conceptualize the scale at which the measurement is being made (Figure 2). Measurements at the sub-cellular, cellular, and tissue length scales are all possible with various OoC formats, but address very different biological questions. For example, our group has consistently found that despite tissues having well-defined global patterns of force generation (Lee et al., 2019) and mechanical stiffness (Mok et al., 2020), designing cellular-length scale sensors reveals considerable heterogeneity in both force and stiffness, likely due to the highly-local action of specialized cells within a tissue construct, and the distinct structures being interrogated at various length scales. Different technological approaches will likely be required for each of these length scales (Figure 2), with different design approaches. For example, sub-cellular measurements of force using traction force microscopy-based approaches may best be accomplished by simply incorporating fiducial particles into a soft substrate, here used to quantify cellular contractility while exposing the cells to shear flow in a microfluidic channel (Jang et al., 2019; Zhang et al., 2019). These stresses are highly diverse, and cannot be easily integrated to capture stresses that drive disease-specific phenotypes. Measuring cellular level forces requires those fiducial particles to have defined mechanical properties and capabilities, but cannot capture highly focal applications of force that may be relevant for certain biological processes. Measuring tissue-level forces requires anchored pillars that limit tissue shape, but provide sufficient resistance to the movement that forces can be measured (Vila et al., 2021).
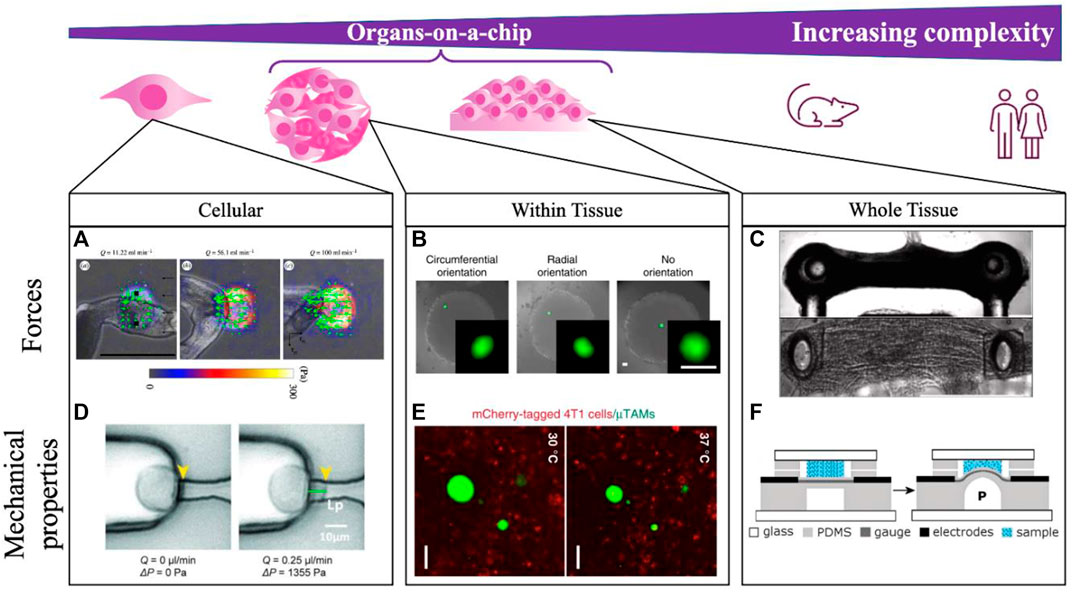
FIGURE 2. Integrating mechanical measurements on-a-chip can be done to study tissues spanning a range of complexities. Measurements are made at the single cell scale (A,D), locally within self-assembled tissues (B,E), and across whole dynamic tissues (C,F). (A) Schistosoma mansoni generated forces are quantified by integrating TFM-on-a-chip and modulating a shear force applied to cells with flowrate Q in the microfluidic channel. Scale bar 500 μm. Reprinted with permission from Zhang et al. (2019), J. R. Soc. Interface 16: 20180675 (2018). Copyright 2018 The Royal Society (UK) (B) Within tissures, compliant hydrogel microdroplets deform under applied loads in a fibroblast multicellular spheroid, with bead orientation indicating the direction of applied cell-generated forces. Scale bare 50 μm. Reprinted with permission from Lee et al. (2019), Nat. Commun. 10, 144 (2019). Copyright 2019 Author(s), licensed under a Creative Commons Attribution (CC BY) license. (C) Contractility of microtissues is measured according to the deflection of embedded pillars in neuromuscular-junction on-a-chip. Scale bar 500 μm. Reprinted from Vila et al. (2021), Biomat., 276:121033 (2021). Copyright 2021 Elsevier. (D) Mechanical properties can further be measured on-a-chip by locally aspirating and deforming the cell membrane with a known vacuum pressure applied through a microfluidic channel. Reprinted with permission from Lee and Liu (2014), Lab on a Chip 15 (1), 264–273 (2015). Copyright 2015 Royal Society of Chemistry (UK) (E) Within tissues, local stiffness measurements are made by incorporating thermoresponsive hydrogel beads, where the extent of bead expansion is correlated with surrounding tissue stiffness. Scale bar 50 μm. Reprinted with permission from Mok et al. (2020), Nat. Commun. 11, 4757 (2020). Copyright 2020 Author(s), licensed under a Creative Commons Attribution (CC BY) license. (F) Whole tissue stiffness is quantified by applying a known pressure to the tissue through a bulging device integrating on-a-chip. Reprinted with permission from MacQueen et al. (2012), Lab on a Chip 20 (12), 4178 (2012). Copyright 2012 Royal Society of Chemistry (UK).
Several core challenges exist in designing and applying these sensors to OoCs that remain to be addressed. First, whether a measurement system interferes or modifies the biological system being studied will remain an open question and must be carefully answered on a case-by-case comparative basis. For example, whether soft hydrogel particles included in a tissue to measure local force (Lee et al., 2019) change the function of that tissue remains an open question. While steps can be taken to coat the particles with cell- and tissue-like materials, the simple inclusion of a mechanically distinct region within the tissues may itself alter the biological system, and must be validated carefully against control conditions for the variable of interest. Second, the measurement system must be compatible with both the material properties and processing attributes of the OoC system. For example, despite being similar materials, integrating silicone micropillars into standard silicone devices presents significant challenges in releasing the pillars without collapsing them due to surface tension effects, and bonding surfaces via plasma oxidation without changing the surface mechanical properties of the sensor. Third, OoC platforms are often touted as scalable alternatives to animal models, and integrating mechanical sensors significantly increases their complexity, and hence susceptibility to failure. This is particularly important in applications that require high-throughput analyses and experimentation such as drug screening, and developing these sensing systems to be sufficiently robust for such applications constitutes an important design challenge for the future.
Author contributions
IM: Conceptualization; Writing—original draft (lead); Writing—review and editing (equal). C-MB: Conceptualization; Writing—original draft (lead); Writing—review and editing (equal). BEC: Conceptualization; Writing—original draft (lead); Writing—review and editing (equal). CM: Conceptualization; Funding acquisition (lead); Supervision (lead); Writing—original draft (supporting); Writing—review and editing (equal).
Funding
This work was supported by the McGill Interdisciplinary Initiative in Infection and Immunity (MI4), the Canadian Cancer Society (Grant 706002), the Natural Sciences and Engineering Research Council of Canada (RGPIN-2022-05165), and the Canada Research Chairs in Advanced Cellular Microenvironments to CM.
Conflict of interest
The authors declare that the research was conducted in the absence of any commercial or financial relationships that could be construed as a potential conflict of interest.
Publisher’s note
All claims expressed in this article are solely those of the authors and do not necessarily represent those of their affiliated organizations, or those of the publisher, the editors and the reviewers. Any product that may be evaluated in this article, or claim that may be made by its manufacturer, is not guaranteed or endorsed by the publisher.
References
Adam Kratz, S. R., Höll, G., Schuller, P., Ertl, P., and Rothbauer, M. (2019). Latest trends in biosensing for microphysiological organs-on-a-chip and body-on-a-chip systems. Biosensors 9, 110. doi:10.3390/bios9030110
Agarwal, A., Goss, J. A., Cho, A., McCain, M. L., and Parker, K. K. (2013). Microfluidic heart on a chip for higher throughput pharmacological studies. Lab. Chip 13, 3599–3608. doi:10.1039/c3lc50350j
Alexander, F. A., Eggert, S., and Wiest, J. (2018). Skin-on-a-chip: Transepithelial electrical resistance and extracellular acidification measurements through an automated air-liquid interface. Genes 9, 114. doi:10.3390/genes9020114
Antonacci, G., Beck, T., Bilenca, A., Czarske, J., Elsayad, K., Guck, J., et al. (2020). Recent progress and current opinions in Brillouin microscopy for life science applications. Biophys. Rev. 12, 615–624. doi:10.1007/s12551-020-00701-9
Aoun, L., Larnier, S., Weiss, P., Cazales, M., Herbulot, A., Ducommun, B., et al. (2019). Measure and characterization of the forces exerted by growing multicellular spheroids using microdevice arrays. PLOS ONE 14, e0217227. doi:10.1371/journal.pone.0217227
Asmani, M., Velumani, S., Li, Y., Wawrzyniak, N., Hsia, I., Chen, Z., et al. (2018). Fibrotic microtissue array to predict anti-fibrosis drug efficacy. Nat. Commun. 9, 2066. doi:10.1038/s41467-018-04336-z
Balaban, N. Q., Schwarz, U. S., Riveline, D., Goichberg, P., Tzur, G., Sabanay, I., et al. (2001). Force and focal adhesion assembly: a close relationship studied using elastic micropatterned substrates. Nat. Cell Biol. 3, 466–472. doi:10.1038/35074532
Bavli, D., Prill, S., Ezra, E., Levy, G., Cohen, M., Vinken, M., et al. (2016). Real-time monitoring of metabolic function in liver-onchip microdevices tracks the dynamics of Mitochondrial dysfunction. Proc. Natl. Acad. Sci. U. S. A. 113, E2231–E2240. doi:10.1073/pnas.1522556113
Beekmans, S. V., Emanuel, K. S., Smit, T. H., and Iannuzzi, D. (2017). Minimally invasive micro-indentation: mapping tissue mechanics at the tip of an 18G needle. Sci. Rep. 7, 11364. doi:10.1038/s41598-017-10526-4
Bose, P., Eyckmans, J., Nguyen, T. D., Chen, C. S., and Reich, D. H. (2019). Effects of geometry on the mechanics and alignment of three-dimensional engineered microtissues. ACS Biomater. Sci. Eng. 5, 3843–3855. doi:10.1021/acsbiomaterials.8b01183
Bossink, E. G. B. M., Zakharova, M., De Bruijn, D. S., Odijk, M., and Segerink, L. I. (2021). Measuring barrier function in organ-on-chips with cleanroom-free integration of multiplexable electrodes. Lab. Chip 21, 2040–2049. doi:10.1039/d0lc01289k
Campbell, S. B., Wu, Q., Yazbeck, J., Liu, C., Okhovatian, S., and Radisic, M. (2021). Beyond polydimethylsiloxane: Alternative materials for fabrication of organ-on-a-chip devices and microphysiological systems. ACS Biomater. Sci. Eng. 7, 2880–2899. doi:10.1021/acsbiomaterials.0c00640
Chen, Z., Lu, J., Zhang, C., Hsia, I., Yu, X., Marecki, L., et al. (2019). Microclot array elastometry for integrated measurement of thrombus formation and clot biomechanics under fluid shear. Nat. Commun. 10, 2051. doi:10.1038/s41467-019-10067-6
Chen, L. J., Raut, B., Nagai, N., Abe, T., and Kaji, H. (2020). Prototyping a versatile two-layer multi-channel microfluidic device for direct-contact cell-vessel co-culture. Micromachines 11, 79. doi:10.3390/mi11010079
Chen, J. (2014). Nanobiomechanics of living cells: a review. Interface Focus 4, 20130055. doi:10.1098/rsfs.2013.0055
Clarke, G. A., Hartse, B. X., Asli, A. E. N., Taghavimehr, M., Hashemi, N., Shirsavar, M. A., et al. (2021). Advancement of sensor integrated organ-on-chip devices. Sensors Switz. 21, 1367–1444. doi:10.3390/s21041367
Coughlin, M. F., Bielenberg, D. R., Lenormand, G., Marinkovic, M., Waghorne, C. G., Zetter, B. R., et al. (2013). Cytoskeletal stiffness, friction, and fluidity of cancer cell lines with different metastatic potential. Clin. Exp. Metastasis 30, 237–250. doi:10.1007/s10585-012-9531-z
Danku, A. E., Dulf, E-H., Braicu, C., Jurj, A., and Berindan-Neagoe, I. (2022). Organ-on-A-chip: A survey of technical results and problems. Front. Bioeng. Biotechnol. 10, 840674. doi:10.3389/fbioe.2022.840674
Dembo, M., and Wang, Y-L. (1999). Stresses at the cell-to-substrate interface during locomotion of fibroblasts. Biophysical J. 76, 2307–2316. doi:10.1016/s0006-3495(99)77386-8
Diez-Perez, A., Güerri, R., Nogues, X., Cáceres, E., Peña, M. J., Mellibovsky, L., et al. (2010). Microindentation for in vivo measurement of bone tissue mechanical properties in humans. J. Bone Min. Res. 25, 1877–1885. doi:10.1002/jbmr.73
Dolega, M. E., Delarue, M., Ingremeau, F., Prost, J., Delon, A., and Cappello, G. (2017). Cell-like pressure sensors reveal increase of mechanical stress towards the core of multicellular spheroids under compression. Nat. Commun. 8, 14056. doi:10.1038/ncomms14056
Ergir, E., Bachmann, B., Redl, H., Forte, G., and Ertl, P. (2018). Small force, big impact: Next generation organ-on-a-chip systems incorporating biomechanical cues. Front. Physiol. 9, 1417. doi:10.3389/fphys.2018.01417
Ferrari, E., Palma, C., Vesentini, S., Occhetta, P., and Rasponi, M. (2020). Integrating biosensors in organs-on-chip devices: A perspective on current strategies to monitor microphysiological systems. Biosensors 10(9). doi:10.3390/bios10090110
Ferrell, N., Sandoval, R. M., Molitoris, B. A., Brakeman, P., Roy, S., and Fissell, W. H. (2019). Application of physiological shear stress to renal tubular epithelial cells. Methods Cell Biol. 153, 43–67. doi:10.1016/bs.mcb.2019.04.010
Fu, J., Wang, Y-K., Yang, M. T., Desai, R. A., Yu, X., Liu, Z., et al. (2010). Mechanical regulation of cell function with geometrically modulated elastomeric substrates. Nat. Methods 7, 733–736. doi:10.1038/nmeth.1487
Fuchs, S., Tjell, A. Ø., Werr, G., Mayr, T., and Tenje, M. (2021). In-line analysis of organ-on-chip systems with sensors : Integration , fabrication , challenges , and potential. Biomater. Sci. Eng. 7, 2926. doi:10.1021/acsbiomaterials.0c01110
Ganz, A., Lambert, M., Saez, A., Silberzan, P., Buguin, A., Mège, R. M., et al. (2006). Traction forces exerted through N-cadherin contacts. Biol. Cell 98, 721–730. doi:10.1042/bc20060039
Gjorevski, N., Piotrowski, S. A., Varner, V. D., and Nelson, C. M. (2015). Dynamic tensile forces drive collective cell migration through three-dimensional extracellular matrices. Sci. Rep. 5, 11458. doi:10.1038/srep11458
Gjorevski, N., Avignon, B., Gérard, R., Cabon, L., Roth, A. B., Bscheider, M., et al. (2020). Neutrophilic infiltration in organ-on-a-chip model of tissue inflammation. Lab. Chip 20, 3365–3374. doi:10.1039/d0lc00417k
Grosberg, A., Alford, P. W., McCain, M. L., and Parker, K. K. (2011). Ensembles of engineered cardiac tissues for physiological and pharmacological study: Heart on a chip. Lab. Chip 11, 4165–4173. doi:10.1039/c1lc20557a
Guimarães, C. F., Gasperini, L., Marques, A. P., and Reis, R. L. (2020). The stiffness of living tissues and its implications for tissue engineering. Nat. Rev. Mat. 5, 351–370. doi:10.1038/s41578-019-0169-1
Henry, O. Y. F., Villenave, R., Cronce, M., Leineweber, W., Benz, M., and Ingber, D. E. (2017). Organs-on-Chips with integrated electrodes for Trans-Epithelial Electrical Resistance (TEER) measurements of human epithelial barrier function. Lab. Chip 17, 2264–2271. doi:10.1039/c7lc00155j
Huang, D., Huang, Y., Xiao, Y., Yang, X., Lin, H., Feng, G., et al. (2019). Viscoelasticity in natural tissues and engineered scaffolds for tissue reconstruction. Acta Biomater. 97, 74–92. doi:10.1016/j.actbio.2019.08.013
Huh, D., Leslie, D. C., Matthews, B. D., Fraser, J. P., Jurek, S., Hamilton, G. A., et al. (2012). A human disease model of drug toxicity-induced pulmonary edema in a lung-on-a-chip microdevice. Sci. Transl. Med. 4, 159ra147. doi:10.1126/scitranslmed.3004249
Ingber, D. E. (2022). Human organs-on-chips for disease modelling, drug development and personalized medicine. Nat. Rev. Genet. 23, 467–491. doi:10.1038/s41576-022-00466-9
Jaiswal, D., Cowley, N., Bian, Z., Zheng, G., Claffey, K. P., and Hoshino, K. (2017). Stiffness analysis of 3D spheroids using microtweezers. PLOS ONE 12, e0188346. doi:10.1371/journal.pone.0188346
Jalili-Firoozinezhad, S., Gazzaniga, F. S., Calamari, E. L., Camacho, D. M., Fadel, C. W., Bein, A., et al. (2019). A complex human gut microbiome cultured in an anaerobic intestine-on-a-chip. Nat. Biomed. Eng. 3, 520–531. doi:10.1038/s41551-019-0397-0
Jang, H., Kim, J., Shin, J. H., Fredberg, J. J., Park, C. Y., and Park, Y. (2019). Traction microscopy with integrated microfluidics: responses of the multi-cellular island to gradients of HGF. Lab. Chip 19, 1579–1588. doi:10.1039/c9lc00173e
Kasendra, M., Tovaglieri, A., Sontheimer-Phelps, A., Jalili-Firoozinezhad, S., Bein, A., Chalkiadaki, A., et al. (2018). Development of a primary human Small Intestine-on-a-Chip using biopsy-derived organoids. Sci. Rep. 8, 2871. doi:10.1038/s41598-018-21201-7
Kasza, K. E., Vader, D., Köster, S., Wang, N., and Weitz, D. A. (2011). Magnetic twisting cytometry. Cold Spring Harb. Protoc. 2011, pdb.prot5599. doi:10.1101/pdb.prot5599
Kennedy, K. M., Chin, L., McLaughlin, R. A., Latham, B., Saunders, C. M., Sampson, D. D., et al. (2015). Quantitative micro-elastography: imaging of tissue elasticity using compression optical coherence elastography. Sci. Rep. 5, 15538. doi:10.1038/srep15538
Kilic, T., Navaee, F., Stradolini, F., Renaud, P., and Carrara, S. (2018). Organs-on-chip monitoring: sensors and other strategies. Microphysiol. Syst. 1, 1. doi:10.21037/mps.2018.01.01
Lam, R. H. W., Weng, S., Lu, W., and Fu, J. (2012). Live-cell subcellular measurement of cell stiffness using a microengineered stretchable micropost array membrane. Integr. Biol. 4, 1289–1298. doi:10.1039/c2ib20134h
Lee, L. M., and Liu, A. P. (2014). A microfluidic pipette array for mechanophenotyping of cancer cells and mechanical gating of mechanosensitive channels. Lab. Chip 15, 264–273. doi:10.1039/c4lc01218f
Lee, J., Leonard, M., Oliver, T., Ishihara, A., and Jacobson, K. (1994). Traction forces generated by locomoting keratocytes. J. Cell Biol. 127, 1957–1964. doi:10.1083/jcb.127.6.1957
Lee, J., Estlack, Z., Somaweera, H., Wang, X., Lacerda, C. M. R., and Kim, J. (2018). A microfluidic cardiac flow profile generator for studying the effect of shear stress on valvular endothelial cells. Lab. Chip 18, 2946–2954. doi:10.1039/c8lc00545a
Lee, W., Kalashnikov, N., Mok, S., Halaoui, R., Kuzmin, E., Putnam, A. J., et al. (2019). Dispersible hydrogel force sensors reveal patterns of solid mechanical stress in multicellular spheroid cultures. Nat. Commun. 10, 144. doi:10.1038/s41467-018-07967-4
Lee, J., Mehrotra, S., Zare-Eelanjegh, E., Rodrigues, R. O., Akbarinejad, A., Ge, D., et al. (2021). A heart-breast cancer-on-a-chip platform for disease modeling and monitoring of cardiotoxicity induced by cancer chemotherapy. Small 17, 2004258. doi:10.1002/smll.202004258
Legant, W. R., Pathak, A., Yang, M. T., Deshpande, V. S., McMeeking, R. M., and Chen, C. S. (2009). Microfabricated tissue gauges to measure and manipulate forces from 3D microtissues. Proc. Natl. Acad. Sci. U. S. A. 106, 10097–10102. doi:10.1073/pnas.0900174106
Legant, W. R., Miller, J. S., Blakely, B. L., Cohen, D. M., Genin, G. M., and Chen, C. S. (2010). Measurement of mechanical tractions exerted by cells in three-dimensional matrices. Nat. Methods 7, 969–971. doi:10.1038/nmeth.1531
Leggett, S. E., Patel, M., Valentin, T. M., Gamboa, L., Khoo, A. S., Williams, E. K., et al. (2020). Mechanophenotyping of 3D multicellular clusters using displacement arrays of rendered tractions. Proc. Natl. Acad. Sci. U. S. A. 117, 5655–5663. doi:10.1073/pnas.1918296117
Leung, C. M., de Haan, P., Ronaldson-Bouchard, K., Kim, G-A., Ko, J., Rho, H. S., et al. (2022). A guide to the organ-on-a-chip. Nat. Rev. Methods Prim. 2, 33–29. doi:10.1038/s43586-022-00118-6
Li, C., Guan, G., Li, S., Huang, Z., and Wang, R. K. (2012). Evaluating elastic properties of heterogeneous soft tissue by surface acoustic waves detected by phase-sensitive optical coherence tomography. J. Biomed. Opt. 17, 057002. doi:10.1117/1.jbo.17.5.057002
Liu, A. S., Wang, H., Copeland, C. R., Chen, C. S., Shenoy, V. B., and Reich, D. H. (2016). Matrix viscoplasticity and its shielding by active mechanics in microtissue models: experiments and mathematical modeling. Sci. Rep. 6, 33919. doi:10.1038/srep33919
Lucio, A. A., Mongera, A., Shelton, E., Chen, R., Doyle, A. M., and Campàs, O. (2017). Spatiotemporal variation of endogenous cell-generated stresses within 3D multicellular spheroids. Sci. Rep. 7, 12022. doi:10.1038/s41598-017-12363-x
Ma, Z., Huebsch, N., Koo, S., Mandegar, M. A., Siemons, B., Boggess, S., et al. (2018). Contractile deficits in engineered cardiac microtissues as a result of MYBPC3 deficiency and mechanical overload. Nat. Biomed. Eng. 2, 955–967. doi:10.1038/s41551-018-0280-4
Maccabi, A., Shin, A., Namiri, N. K., Bajwa, N., John M, St., Taylor, Z. D., et al. (2018). Quantitative characterization of viscoelastic behavior in tissue-mimicking phantoms and ex vivo animal tissues. PLoS One 13, e0191919. doi:10.1371/journal.pone.0191919
MacQueen, L., Chebotarev, O., Simmons, C. A., and Sun, Y. (2012). Miniaturized platform with on-chip strain sensors for compression testing of arrayed materials. Lab. Chip 12, 4178–4184. doi:10.1039/c2lc40670e
Malandrino, A., Trepat, X., Kamm, R. D., and Mak, M. (2019). Dynamic filopodial forces induce accumulation, damage, and plastic remodeling of 3D extracellular matrices. PLoS Comput. Biol. 15, e1006684. doi:10.1371/journal.pcbi.1006684
Mann, J. M., Lam, R. H. W., Weng, S., Sun, Y., and Fu, J. (2012). A silicone-based stretchable micropost array membrane for monitoring live-cell subcellular cytoskeletal response. Lab. Chip 12, 731–740. doi:10.1039/c2lc20896b
Maoz, B. M., Herland, A., Henry, O. Y. F., Leineweber, W. D., Yadid, M., Doyle, J., et al. (2017). Organs-on-Chips with combined multi-electrode array and transepithelial electrical resistance measurement capabilities. Lab. Chip 17, 2294–2302. doi:10.1039/c7lc00412e
Mark, C., Grundy, T. J., Strissel, P. L., Böhringer, D., Grummel, N., Gerum, R., et al. (2020). Collective forces of tumor spheroids in three-dimensional biopolymer networks. eLife 9, e51912. doi:10.7554/elife.51912
Maruthamuthu, V., Sabass, B., Schwarz, U. S., and Gardel, M. L. (2011). Cell-ECM traction force modulates endogenous tension at cell–cell contacts. Proc. Natl. Acad. Sci. U. S. A. 108, 4708–4713. doi:10.1073/pnas.1011123108
Mohagheghian, E., Luo, J., Chen, J., Chaudhary, G., Chen, J., Sun, J., et al. (2018). Quantifying compressive forces between living cell layers and within tissues using elastic round microgels. Nat. Commun. 9, 1878. doi:10.1038/s41467-018-04245-1
Mok, S., Al Habyan, S., Ledoux, C., Lee, W., MacDonald, K. N., McCaffrey, L., et al. (2020). Mapping cellular-scale internal mechanics in 3D tissues with thermally responsive hydrogel probes. Nat. Commun. 11, 4757. doi:10.1038/s41467-020-18469-7
Mongera, A., Rowghanian, P., Gustafson, H. J., Shelton, E., Kealhofer, D. A., Carn, E. K., et al. (2018). A fluid-to-solid jamming transition underlies vertebrate body axis elongation. Nature 561, 401–405. doi:10.1038/s41586-018-0479-2
Moraes, C., Sun, Y., and Simmons, C. A. (2011). (Micro)managing the mechanical microenvironment. Integr. Biol. 3, 959–971. doi:10.1039/c1ib00056j
Moraes, C., Labuz, J. M., Shao, Y., Fu, J., and Takayama, S. (2015). Supersoft lithography: candy-based fabrication of soft silicone microstructures. Lab. Chip 15, 3760–3765. doi:10.1039/c5lc00722d
Moutaux, E., Charlot, B., Genoux, A., Saudou, F., and Cazorla, M. (2018). An integrated microfluidic/microelectrode array for the study of activity-dependent intracellular dynamics in neuronal networks. Lab. Chip 18, 3425–3435. doi:10.1039/c8lc00694f
Munevar, S., Wang, Y., and Dembo, M. (2001). Traction force microscopy of migrating normal and H-ras transformed 3T3 fibroblasts. Biophysical J. 80, 1744–1757. doi:10.1016/s0006-3495(01)76145-0
Nguyen, A. K., and Kilian, K. A. (2020). Physicochemical tools for visualizing and quantifying cell-generated forces. ACS Chem. Biol. 15, 1731–1746. doi:10.1021/acschembio.0c00304
Obenaus, A. M., Mollica, M. Y., and Sniadecki, N. J. (2020). (De)form and function: Measuring cellular forces with deformable materials and deformable structures. Adv. Healthc. Mat. 9, 1901454. doi:10.1002/adhm.201901454
Oliver, T., Jacobson, K., and Dembo, M. (1998). Design and use of substrata to measure traction forces exerted by cultured cells. Methods Enzymol. 298, 497–521. doi:10.1016/s0076-6879(98)98042-9
Ort, C., Lee, W., Kalashnikov, N., and Moraes, C. (2021). Disentangling the fibrous microenvironment: designer culture models for improved drug discovery. Expert Opin. Drug Discov. 16, 159–171. doi:10.1080/17460441.2020.1822815
Ortega, M. A., Fernández-Garibay, X., Castaño, A. G., De Chiara, F., Hernández-Albors, A., Balaguer-Trias, J., et al. (2019). Muscle-on-a-chip with an on-site multiplexed biosensing system for in situ monitoring of secreted IL-6 and TNF-α. Lab. Chip 19, 2568–2580. doi:10.1039/c9lc00285e
Oyen, M. l. (2013). Nanoindentation of biological and biomimetic materials. Exp. Tech. 37, 73–87. doi:10.1111/j.1747-1567.2011.00716.x
Park, T. E., Mustafaoglu, N., Herland, A., Hasselkus, R., Mannix, R., FitzGerald, E. A., et al. (2019). Hypoxia-enhanced Blood-Brain Barrier Chip recapitulates human barrier function and shuttling of drugs and antibodies. Nat. Commun. 10, 2621–2712. doi:10.1038/s41467-019-10588-0
Pelham, R. J., and Wang, Y. (1999). High resolution detection of mechanical forces exerted by locomoting fibroblasts on the substrate. MBoC. 10, 935–945. doi:10.1091/mbc.10.4.935
Picollet-D’hahan, N., Zuchowska, A., Lemeunier, I., and Le Gac, S. (2021). Multiorgan-on-a-Chip: A systemic approach to model and decipher inter-organ communication. Trends Biotechnol. 39, 788. Special Issue: Microphysiological Systems. doi:10.1016/j.tibtech.2020.11.014
Polacheck, W. J., and Chen, C. S. (2016). Measuring cell-generated forces: a guide to the available tools. Nat. Methods 13, 415–423. doi:10.1038/nmeth.3834
Polio, S. R., Rothenberg, K. E., Stamenović, D., and Smith, M. L. (2012). A micropatterning and image processing approach to simplify measurement of cellular traction forces. Acta Biomater. 8, 82–88. doi:10.1016/j.actbio.2011.08.013
Portillo-Esquivel, L. E., Nanduri, V., Zhang, F., Liang, W., and Zhang, B. (2020). Z-wire: A microscaffold that supports guided tissue assembly and intramyocardium delivery for cardiac repair. Adv. Healthc. Mat. 9, 2000358. doi:10.1002/adhm.202000358
Prevedel, R., Diz-Muñoz, A., Ruocco, G., and Antonacci, G. (2019). Brillouin microscopy: an emerging tool for mechanobiology. Nat. Methods 16, 969–977. doi:10.1038/s41592-019-0543-3
Proestaki, M., Ogren, A., Burkel, B., and Notbohm, J. (2019). Modulus of fibrous collagen at the length scale of a cell. Exp. Mech. 59, 1323–1334. doi:10.1007/s11340-018-00453-4
Riahi, R., Shaegh, S. A. M., Ghaderi, M., Zhang, Y. S., Shin, S. R., Aleman, J., et al. (2016). Automated microfluidic platform of bead-based electrochemical immunosensor integrated with bioreactor for continual monitoring of cell secreted biomarkers. Sci. Rep. 6, 24598–24614. doi:10.1038/srep24598
Roca-Cusachs, P., Conte, V., and Trepat, X. (2017). Quantifying forces in cell biology. Nat. Cell Biol. 19, 742–751. doi:10.1038/ncb3564
Ronaldson-Bouchard, K., and Vunjak-Novakovic, G. (2018). Organs-on-a-Chip: A fast track for engineered human tissues in drug development. Cell Stem Cell 22, 310–324. doi:10.1016/j.stem.2018.02.011
Rothbauer, M., and Ertl, P. (2020). Emerging biosensor trends in organ-on-a-chip. Adv. Biochem. Eng. Biotechnol. 179, 343. doi:10.1007/10_2020_129
Rouredu, O., Saez, A., Buguin, A., Austin, R. H., Chavrier, P., Siberzan, P., et al. (2005). Force mapping in epithelial cell migration. Proc. Natl. Acad. Sci. U. S. A. 102, 2390–2395. doi:10.1073/pnas.0408482102
Sakar, M. S., Eyckmans, J., Pieters, R., Eberli, D., Nelson, B. J., and Chen, C. S. (2016). Cellular forces and matrix assembly coordinate fibrous tissue repair. Nat. Commun. 7, 11036. doi:10.1038/ncomms11036
Serra-Picamal, X., Conte, V., Vincent, R., Anon, E., Tambe, D. T., Bazellieres, E., et al. (2012). Mechanical waves during tissue expansion. Nat. Phys. 8, 628–634. doi:10.1038/nphys2355
Serwane, F., Mongera, A., Rowghanian, P., Kealhofer, D. A., Lucio, A. A., Hockenbery, Z. M., et al. (2017). In vivo quantification of spatially-varying mechanical properties in developing tissues. Nat. Methods 14, 181–186. doi:10.1038/nmeth.4101
Sheets, K., Wang, J., Zhao, W., Kapania, R., and Nain, A. S. (2016). Nanonet force microscopy for measuring cell forces. Biophysical J. 111, 197–207. doi:10.1016/j.bpj.2016.05.031
Shin, S. R., Zhang, Y. S., Kim, D-J., Manbohi, A., Avci, H., Silvestri, A., et al. (2016). Aptamer-based microfluidic electrochemical biosensor for monitoring cell-secreted trace cardiac biomarkers. Anal. Chem. 88, 10019–10027. doi:10.1021/acs.analchem.6b02028
Shin, H., Jeong, S., Lee, J-H., Sun, W., Choi, N., and Cho, I-J. (2021). 3D high-density microelectrode array with optical stimulation and drug delivery for investigating neural circuit dynamics. Nat. Commun. 12, 492. doi:10.1038/s41467-020-20763-3
Skardal, A., Shupe, T., and Atala, A. (2016). Organoid-on-a-chip and body-on-a-chip systems for drug screening and disease modeling. Drug Discov. Today 21, 1399–1411. doi:10.1016/j.drudis.2016.07.003
Sniadecki, N. J., Lamb, C. M., Liu, Y., Chen, C. S., and Reich, D. H. (2008). Magnetic microposts for mechanical stimulation of biological cells: Fabrication, characterization, and analysis. Rev. Sci. Instrum. 79, 044302. doi:10.1063/1.2906228
Soucy, J. R., Bindas, A. J., Koppes, A. N., and Koppes, R. A. (2019). Instrumented microphysiological systems for real-time measurement and manipulation of cellular electrochemical processes. iScience 21, 521–548. doi:10.1016/j.isci.2019.10.052
Steinwachs, J., Metzner, C., Skodzek, K., Lang, N., Thievessen, I., Münster, S., et al. (2016). Three-dimensional force microscopy of cells in biopolymer networks. Nat. Methods 13, 171–176. doi:10.1038/nmeth.3685
Stucki, A. O., Stucki, J. D., Hall, S. R. R., Felder, M., Mermoud, Y., Schmid, R. A., et al. (2015). A lung-on-a-chip array with an integrated bio-inspired respiration mechanism. Lab. Chip 15, 1302–1310. doi:10.1039/c4lc01252f
Tambe, D. T., Corey Hardin, C., Angelini, T. E., Rajendran, K., Park, C. Y., Serra-Picamal, X., et al. (2011). Collective cell guidance by cooperative intercellular forces. Nat. Mat. 10, 469–475. doi:10.1038/nmat3025
Tan, J. L., Tien, J., Pirone, D. M., Gray, D. S., Bhadriraju, K., and Chen, C. S. (2003). Cells lying on a bed of microneedles: An approach to isolate mechanical force. Proc. Natl. Acad. Sci. U. S. A. 100, 1484–1489. doi:10.1073/pnas.0235407100
Thavandiran, N., Dubois, N., Mikryukov, A., Massé, S., Beca, B., Simmons, C. A., et al. (2013). Design and formulation of functional pluripotent stem cell-derived cardiac microtissues. Proc. Natl. Acad. Sci. U. S. A. 110, E4698–E4707. doi:10.1073/pnas.1311120110
Thompson, C. L., Fu, S., Heywood, H. K., Knight, M. M., and Thorpe, S. D. (2020). Mechanical stimulation: A crucial element of organ-on-chip models. Front. Bioeng. Biotechnol. 8, 602646. doi:10.3389/fbioe.2020.602646
Tran, T. T. T., Delgado, A., and Jeong, S. (2021). Organ-on-a-Chip: The future of therapeutic aptamer research? BioChip J. 15, 109–122. doi:10.1007/s13206-021-00016-1
Trepat, X., Wasserman, M. R., Angelini, T. E., Millet, E., Weitz, D. A., Butler, J. P., et al. (2009). Physical forces during collective cell migration. Nat. Phys. 5, 426–430. doi:10.1038/nphys1269
Tu, K. H., Yu, L. S., Sie, Z. H., Hsu, H. Y., Al-Jamal, K. T., Wang, J. T. W., et al. (2021). Development of real-time transendothelial electrical resistance monitoring for an in vitro blood-brain barrier system. Micromachines 12, 37–11. doi:10.3390/mi12010037
van der Helm, M. W., Henry, O. Y. F., Bein, A., Hamkins-Indik, T., Cronce, M. J., Leineweber, W. D., et al. (2019). Non-invasive sensing of transepithelial barrier function and tissue differentiation in organs-on-chips using impedance spectroscopy. Lab. Chip 19, 452–463. doi:10.1039/c8lc00129d
Vila, O. F., Chavez, M., Ma, S. P., Yeager, K., Zholudeva, L. V., Colón-Mercado, J. M., et al. (2021). Bioengineered optogenetic model of human neuromuscular junction. Biomaterials 276, 121033. doi:10.1016/j.biomaterials.2021.121033
Vogt, N. (2022). Modeling multi-organ systems on a chip. Nat. Methods 19, 641. doi:10.1038/s41592-022-01533-z
Walker, M., Rizzuto, P., Godin, M., and Pelling, A. E. (2020). Structural and mechanical remodeling of the cytoskeleton maintains tensional homeostasis in 3D microtissues under acute dynamic stretch. Sci. Rep. 10, 7696. doi:10.1038/s41598-020-64725-7
Walter, F. R., Valkai, S., Kincses, A., Petneházi, A., Czeller, T., Veszelka, S., et al. (2016). A versatile lab-on-a-chip tool for modeling biological barriers. Sensors Actuators, B Chem. 222, 1209–1219. doi:10.1016/j.snb.2015.07.110
Wang, N., Naruse, K., Stamenovic, D., Fredberg, J. J., Mijailovich, S. M., Toric-Norrelykke, I. M., et al. (2001). Mechanical behavior in living cells consistent with the tensegrity model. Proc. Natl. Acad. Sci. U. S. A. 98, 7765–7770. doi:10.1073/pnas.141199598
Wang, E. Y., Rafatian, N., Zhao, Y., Lee, A., Lai, B. F. L., Lu, R. X., et al. (2019). Biowire model of interstitial and focal cardiac fibrosis. ACS Cent. Sci. 5, 1146–1158. doi:10.1021/acscentsci.9b00052
Wang, C., Koo, S., Park, M., Vangelatos, Z., Hoang, P., Conklin, B. R., et al. (2020). Cardiac microtissues: Maladaptive contractility of 3D human cardiac microtissues to mechanical nonuniformity (Adv. Healthcare mater. 8/2020). Adv. Healthc. Mat. 9, 2070024. doi:10.1002/adhm.202070024
Wu, J. Z., Herzog, W., and Epstein, M. (1998). Evaluation of the finite element software ABAQUS for biomechanical modelling of biphasic tissues. J. Biomechanics 31, 165–169. doi:10.1016/s0021-9290(97)00117-6
Wu, Q., Liu, J., Wang, X., Feng, L., Wu, J., Zhu, X., et al. (2020). Organ-on-a-chip: Recent breakthroughs and future prospects. Biomed. Eng. OnLine 19, 9. doi:10.1186/s12938-020-0752-0
Xie, R., Korolj, A., Liu, C., Song, X., Lu, R. X. Z., Zhang, B., et al. (2020). h-FIBER: Microfluidic topographical hollow fiber for studies of glomerular filtration barrier. ACS Cent. Sci. 6, 903–912. doi:10.1021/acscentsci.9b01097
Xu, F., Zhao, R., Liu, A. S., Metz, T., Shi, Y., Bose, P., et al. (2015). A microfabricated magnetic actuation device for mechanical conditioning of arrays of 3D microtissues. Lab. Chip 15, 2496–2503. doi:10.1039/c4lc01395f
Yoshie, H., Koushki, N., Kaviani, R., Tabatabaei, M., Rajendran, K., Dang, Q., et al. (2018). Traction force screening enabled by compliant PDMS elastomers. Biophysical J. 114, 2194–2199. doi:10.1016/j.bpj.2018.02.045
Yoshie, H., Koushki, N., Molter, C., Siegel, P. M., Krishnan, R., and Ehrlicher, A. J. (2019). High throughput traction force microscopy using PDMS reveals dose-dependent effects of transforming growth factor-β on the epithelial-to-mesenchymal transition. J. Vis. Exp., 10.3791/59364. doi:10.3791/59364
Young, E. W. K., and Moraes, C. (2015). Patients are a virtue: advances in microengineered systems for clinical applications. Integr. Biol. 7, 962–966. doi:10.1039/c5ib90031j
Zhang, X., and Greenleaf, J. F. (2007). Estimation of tissue’s elasticity with surface wave speed. J. Acoust. Soc. Am. 122, 2522–2525. doi:10.1121/1.2785045
Zhang, Y., Aleman, J., Shin, S. R., Kilic, T., Kim, D., Mousavi Shaegh, S. A., et al. (2017a). Multisensor-integrated organs-on-chips platform for automated and continual in situ monitoring of organoid behaviors. Proc. Natl. Acad. Sci. U. S. A. 114, E2293–E2302. doi:10.1073/pnas.1612906114
Zhang, Y., Wei, F., Poh, Y-C., Jia, Q., Chen, J., Chen, J., et al. (2017b). Interfacing 3D magnetic twisting cytometry with confocal fluorescence microscopy to image force responses in living cells. Nat. Protoc. 12, 1437–1450. doi:10.1038/nprot.2017.042
Zhang, B., Korolj, A., Lai, B. F. L., and Radisic, M. (2018). Link to external site this link will open in a new windowAdvances in organ-on-a-chip engineering. Nat. Rev. Mat. 3, 257–278. doi:10.1038/s41578-018-0034-7
Zhang, S., Skinner, D., Joshi, P., Criado-Hidalgo, E., Yeh, Y-T., Lasheras, J. C., et al. (2019). Quantifying the mechanics of locomotion of the schistosome pathogen with respect to changes in its physical environment. J. R. Soc. Interface 16, 20180675. doi:10.1098/rsif.2018.0675
Zhao, R., Boudou, T., Wang, W-G., Chen, C. S., and Reich, D. H. (2013). Decoupling cell and matrix mechanics in engineered microtissues using magnetically actuated microcantilevers. Adv. Mat. 25, 1699–1705. doi:10.1002/adma.201203585
Zhao, Y., Rafatian, N., Feric, N. T., Cox, B., Aschar-Sobbi, R., Wang, E. Y., et al. (2019). A platform for generation of chamber-specific cardiac tissues and disease modeling. Cell 176, 913–927. doi:10.1016/j.cell.2018.11.042
Keywords: mechanobiology, organ-on-a-chip, biomechanics, tissue engineering, sensors
Citation: Morales IA, Boghdady C-M, Campbell BE and Moraes C (2022) Integrating mechanical sensor readouts into organ-on-a-chip platforms. Front. Bioeng. Biotechnol. 10:1060895. doi: 10.3389/fbioe.2022.1060895
Received: 03 October 2022; Accepted: 05 December 2022;
Published: 16 December 2022.
Edited by:
Jinglong Tang, Qingdao University, ChinaReviewed by:
Yandong Chen, National Center for Nanoscience and Technology (CAS), ChinaDong Wang, Qingdao University, China
Copyright © 2022 Morales, Boghdady, Campbell and Moraes. This is an open-access article distributed under the terms of the Creative Commons Attribution License (CC BY). The use, distribution or reproduction in other forums is permitted, provided the original author(s) and the copyright owner(s) are credited and that the original publication in this journal is cited, in accordance with accepted academic practice. No use, distribution or reproduction is permitted which does not comply with these terms.
*Correspondence: Christopher Moraes, Y2hyaXMubW9yYWVzQG1jZ2lsbC5jYQ==