- 1Department of Oncology and Metabolism, Mellanby Centre for Bone Research, University of Sheffield, Sheffield, United Kingdom
- 2INSIGNEO Institute for in Silico Medicine, University of Sheffield, Sheffield, United Kingdom
- 3School of Engineering, University of Greenwich, Chatham Maritime, United Kingdom
Biological tissues are complex hierarchical materials, difficult to characterise due to the challenges associated to the separation of scale and heterogeneity of the mechanical properties at different dimensional levels.
The Digital Volume Correlation approach is the only image-based experimental approach that can accurately measure internal strain field within biological tissues under complex loading scenarios. In this minireview examples of DVC applications to study the deformation of musculoskeletal tissues at different dimensional scales are reported, highlighting the potential and challenges of this relatively new technique.
The manuscript aims at reporting the wide breath of DVC applications in the past 2 decades and discuss future perspective for this unique technique, including fast analysis, applications on soft tissues, high precision approaches, and clinical applications.
1 Introduction
Understanding the mechanical properties of musculoskeletal tissues and how they deform under load is fundamental to assess the effect of diseases and to optimise treatments. For example, the bone risk of fracture is associated with its local ability to resist cracking, which is associated with its local deformation (i.e., if a bone is compressed beyond 1% or stretched beyond 0.7% (Bayraktar et al., 2004) local permanent deformation and damage will occur that will lead to fracture if not healed).
However, the mechanical properties of biological tissues are complex and driven by their heterogeneous structures, which are built in spatial hierarchical arrangements. For most engineering materials, where the mechanical properties can be usually characterised evaluating only two dimensional levels (e.g., the macrostructure and the grain in metals), it is relatively easy to estimate the deformation of the structure under complex loading using homogenisation techniques. In such cases, application of contact sensors such as strain gauges are enough to measure the strain under load and simple interpolation functions can be used to accurately estimate the field of deformation. For biological tissues this is not possible. In fact, heterogeneity of the structures requires a much more detailed assessment of the local strains, hundreds or thousands of (micro)sensors would be needed to characterise the deformation of the tissue, which is not feasible nor practical. Therefore, Digital Image Correlation (DIC) has been developed to perform superficial full-field measurements of deformation (Palanca et al., 2016a). However, the complex internal structural patterns of biological tissues do not allow for simple extrapolation of the strains measured at the surface to infer internal strains. Therefore, another technique has been developed: Digital Volume Correlation (DVC, originally called “texture correlation” in (Bay, 1995) for assessing the 3D deformation of trabecular bone).
DVC requires the acquisition of 3D images of the musculoskeletal tissue in its undeformed and deformed configurations, and the application of image-processing correlation algorithms to track the heterogeneous displacement and strain fields induced by the external load. Time-lapsed three-dimensional (3D) micro-computed tomography (microCT) imaging of specimens under loading (also referred to as in situ mechanical testing) has enabled the study of deformation and failure mechanisms in bone and biomaterials (Bay, 1995; Nazarian et al., 2005). With DVC the displacement and strain fields can then be evaluated in 4Ds (across space and time/load level) (Roberts et al., 2014; Grassi and Isaksson, 2015).
Several DVC approaches have been developed and used to characterise the deformation in different musculoskeletal tissues, at different dimensional scales. It is clear that DVC has huge potential to unravel the mechanism of mechanical deformation within biological tissues, as highlighted by the increasing number of studies using this technique in the past 20 years: since the early 2000s more than 150 papers have been published (Search Pubmed: “Digital Volume Correlation”), of which approximately 110 are related to musculoskeletal tissues. Nevertheless, a number of challenges and potential pitfalls associated with in situ mechanical testing [e.g., see recent review (Dall'Ara et al., 2022)] and the application of image correlation algorithms have to be addressed in order to obtain, report and interpret DVC results in a robust way.
The goal of this mini-review is to briefly report examples of DVC applications for musculoskeletal tissues, to highlight open challenges, and to report recent developments that have the potential of further improving its applicability in musculoskeletal research.
2 Digital volume correlation approaches and applications
2.1 Local vs. global approaches
Two main families of DVC approaches (local and global) have been developed and are currently available as commercial software, freeware or customised solutions/services. In both cases probably the most important parameters for obtaining the best displacement and strain measurements with DVC are the microstructural heterogeneity of the studied tissue (i.e., distribution of features within the image domain) and the quality of the input images (i.e., signal to noise ratio).
Local approaches are based on the subdivision of the image into smaller sub-volumes and the spatial correlation of metrics computed in each of the sub-volumes of the undeformed and deformed image independently. Interpolation (e.g., tri-linear) is used to calculate displacements of voxels located between nodes. The final displacement is therefore a 3D full-field average displacement of the pattern within that sub-volume between reference and deformed volume. From the field of resultant displacement vectors for each sub-volume, the field of strain components is computed (e.g., using a centered finite difference scheme). Different parameters (i.e., correlation function, subvolume size, overlap, interpolation, etc.,) affect the precision and accuracy of the algorithm, as demonstrated for bone applications (Dall׳Ara et al., 2017). Different correlation metrics have been used, the most common being direct correlation (DC) and fast-Fourier-transform (FFT), which was found to be faster but less accurate than DC for bone applications (Palanca et al., 2016b). This approach can be quite accurate in predicting local deformations but there may be regions where the correlation is low. Therefore, it is fundamental to examine the correlation map (distribution of coefficients that provide the confidence of the local correlation) and trust the results only in the highly correlated sub-volumes. Different threshold values for local correlation have been used in the literature for different applications in musculoskeletal tissues and biomaterials. While a threshold of 60% can be considered acceptable, correlation values above 80% should be considered for optimal applications. Moreover, while for local approaches the correlation and accuracy in strain measurement is usually good within the specimen’s domain and it is acceptable close to the border, large errors are usually found outside the specimen, where little information is available. Therefore, a masking approach to remove the effect of potential noise outside the specimen is recommended (Palanca et al., 2016b). In any case, local DVC parameters should be optimised for the different applications, in function of the quality of the input images, the heterogeneity of the features visible in the images, and the level of deformation the tissue is subjected to.
Global approaches are based on the minimisation of the difference of the deformed image and the registered undeformed image when a continuous displacement field is applied. The registration equations are solved in a grid of points (e.g., regular or heterogeneous, hexahedral or tetrahedral) called nodes. Interpolation (e.g., tri-linear) within the cell of the grid is used to calculate the displacements from the nodal values. Usually a smoothing of the displacement field is applied to avoid high gradients of displacement that would lead to localised unrealistically high strains. Finally, the displacement field is differentiated into a strain field (e.g., using the finite element or finite difference methods). The global approach is usually associated to lower errors compared to the local approaches, when using the same spatial resolution (Dall׳Ara et al., 2017). This is mainly due to the choice of the regularization process through the smoothing of the displacement field during its calculation. As the object is considered a continuum, one of the advantages of the global approach is the possibility of integrating it with finite element models to account for local differences in stiffness (see Section 3.5) (Madi et al., 2013).
Local and Global DVC approaches can be also used jointly on the same given image dataset. A recent example was applied to evaluate the hydro-mechanical behaviour in natural materials, where the combined use of both approaches improved the physical interpretation of displacement and strain fields for such a complex mechanical environment (Stavropoulou et al., 2020).
Independently from the employed DVC approach, correlation parameters and in particular the sub-volume shape and size should be optimised to obtain the best spatial resolution of the approach while keeping the average (or local) error below an acceptable threshold for that specific application (Madi et al., 2013; Dall'Ara et al., 2014; Boulanaache et al., 2020). For example, the accuracy and precision of the DVC has been found to increase by increasing the size of the sub-volume, and therefore decreasing the measurement resolution following a power law (Dall׳Ara et al., 2017).
2.2 Applications on different musculoskeletal tissues
DVC approaches applied to CT, MRI, microCT, or Synchrotron microCT (SR-microCT; with standard absorption or phase contrast modalities) have been used to analyse the deformation patterns in different native musculoskeletal tissues at different dimensional scales. These studies have reported exhaustive measurements of the internal deformation in tissues under physiological or failure loading scenarios, enabling a much more detailed understanding of the loading and failure mechanisms of the complex hierarchical musculoskeletal tissues and their integration with biomaterials.
While reporting a comprehensive list of every study that used DVC to study the deformation in musculoskeletal tissues is not the goal of this mini-review, examples are reported in Table 1. Several organs and tissues have been studied, including for example: vertebral bodies (Hosseini et al., 2014; Hussein et al., 2018; Palanca et al., 2021), proximal femur (Ridzwan et al., 2018; Ryan et al., 2020; Martelli et al., 2021), implanted scapula (Boulanaache et al., 2020), osteochondral plugs (Tozzi et al., 2020), intervertebral disc (IVD) (Disney et al., 2019; Tavana et al., 2020a), dentin (Lu et al., 2019), bone-biomaterial (Tozzi et al., 2014; Danesi et al., 2016; Joffre et al., 2017; Pena Fernandez et al., 2019; Rapagna et al., 2019), trabecular bone (Zwahlen et al., 2015; Turunen et al., 2020; Yan et al., 2020), cortical bone (Christen et al., 2012), subchondral bone (Madi et al., 2020).
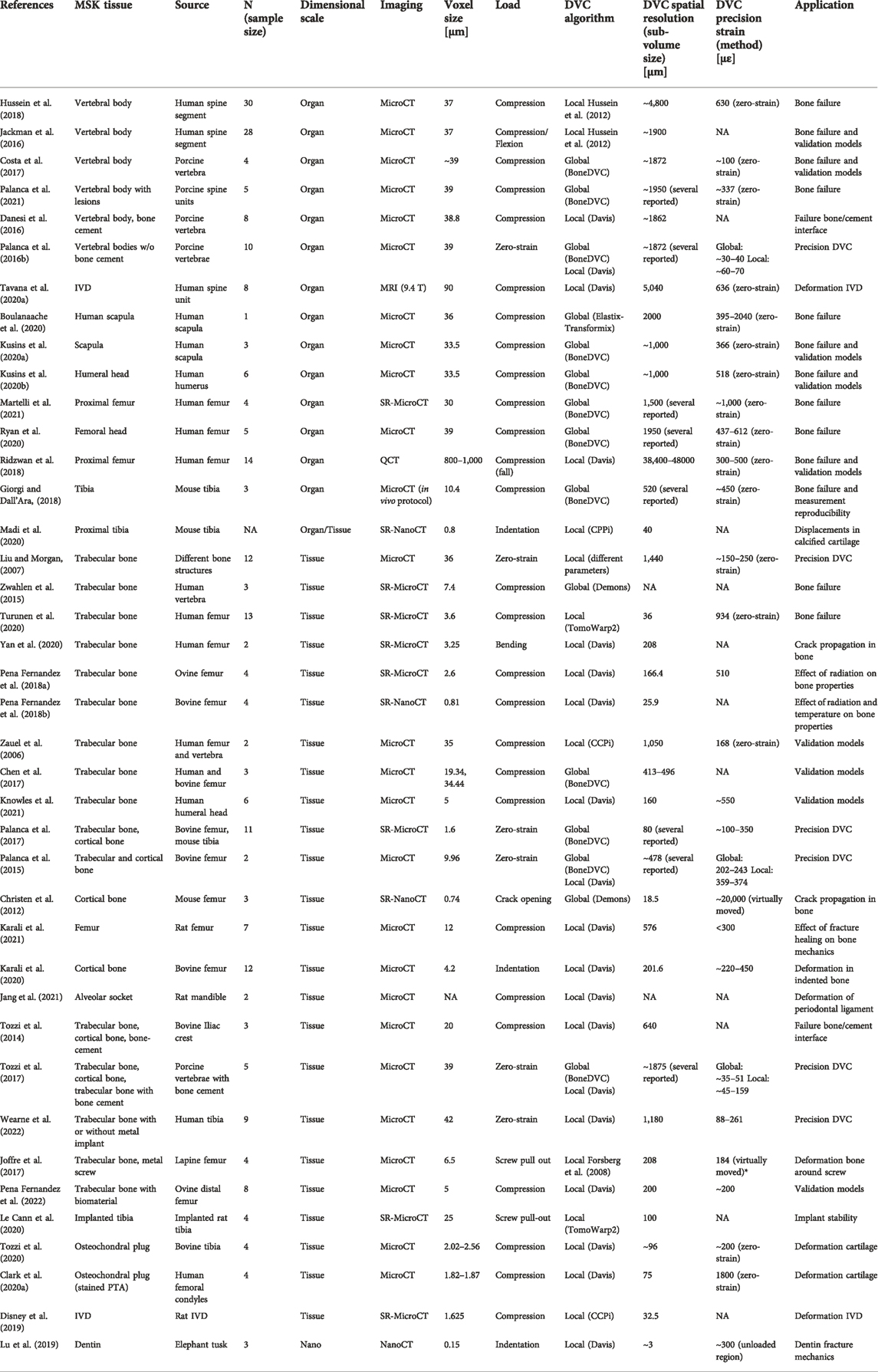
TABLE 1. Examples of applications of DVC at different dimensional levels to evaluate the deformation of different musculoskeletal tissues. While the goal of this minireview is not to report every study where DVC was used on musculoskeletal tissues, the reported examples provide an exhaustive overview of different applications.
While these assessments are performed mainly in hard tissues and biomaterials that do not need contrast enhancing in CT images, for soft tissues (e.g., cartilage, IVD) staining techniques have been applied to improve the visualisation of microstructural features and, therefore, improve the assessment of the local deformation using DVC (Section 3.2).
Depending on the size of the studied specimen (field of view, FOV) and the resolution of the biomedical image used for the DVC analyses, assessments of the deformation at different dimensional scales can be performed, spanning from the organ to the nano level (Figure 1). For example, bone analyses on large portions of the organ can be performed using clinical CT (Ridzwan et al., 2018), on biopsies with microCT (Pena Fernandez et al., 2018a), and on the bulk tissue with SR-microCT (Lu et al., 2019). Nevertheless, it should be noted that we need to accept a compromise between the size of the FOV and the spatial resolution of the DVC approach. This is due to two typical inverse relationships between the size of the FOV and the image resolution, and between the spatial resolution of the DVC and its accuracy (Dall'Ara et al., 2014). However, a recent study on the deformation of the human proximal femur has shown the feasibility of acquiring a large FOV at high resolution with SR-microCT and, therefore, to evaluate the failure mechanism of a large portion of organ with a relatively good DVC spatial resolution (Martelli et al., 2021). Nevertheless, it should be noted that in that case longer scanning time is needed, which is associated with potentially high ionising radiation, which may affect the bone mechanical properties and its deformation (Section 3.4). In that study the DVC approach was applied at the organ level (whole proximal femur) and at the tissue level (femoral head). First DVC applied to the proximal femur using subsampled images (120 μm) to reduce the DVC calculation time. Afterwards, more detailed analyses on the region where the strains localised (femoral head) was performed with the full resolution images (30 μm). This multi-scale application of the DVC approach could be expanded in the future. In fact, some of the current imaging techniques (e.g., Zeiss Xradia Versa X-ray microscopes with Scout-and-Zoom option) enable a multiscale scan of the same specimens, starting from a low-resolution scan of the whole object, and then zooming into interesting regions for high-resolution scans. So far, this approach has been used to highlight differences on the residual strain distribution in cortical bone after cyclic loading at different dimensional scales. By increasing the resolution from 5 µm to 2 µm for the multiscale microCT imaging finer details of the cortical canal network and osteocyte lacunae were revealed. This resulted in improved DVC spatial resolution from 320 µm to 96 µm, which led to different internal strain distributions for the overall scan when compared to the zoom-in region (Pena Fernandez et al., 2020a).
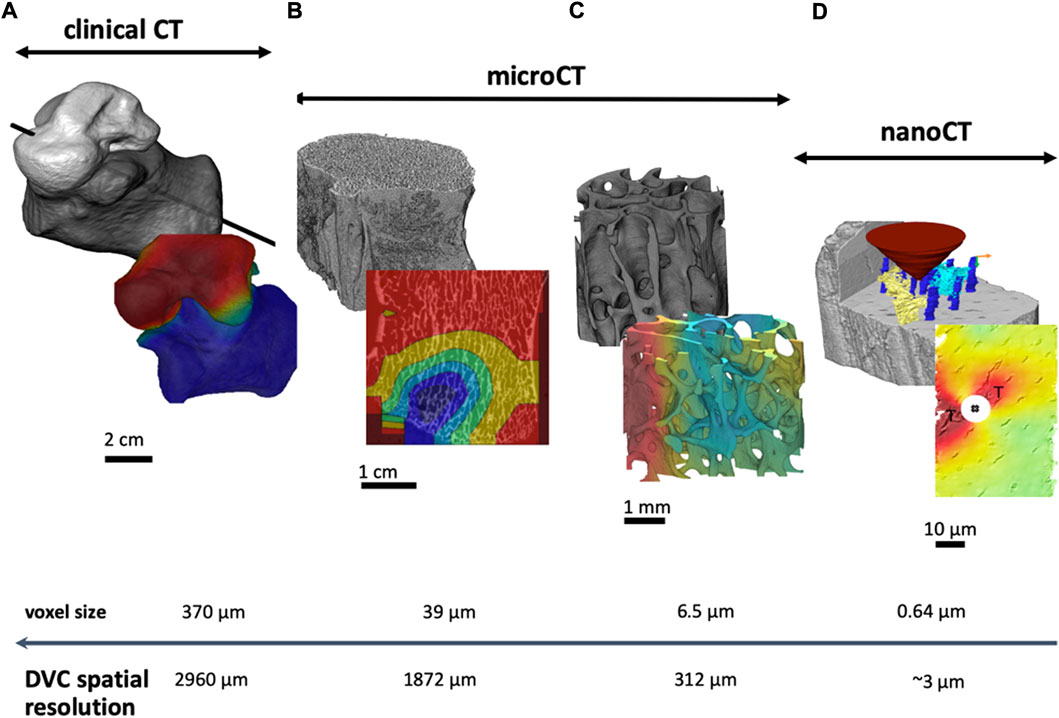
FIGURE 1. Multi-scale approach based on data acquired from different systems from clinical CT to nanoCT. According to the image source and voxel size, the spatial resolution of the measurement enables coarser or finer DVC evaluation. This is very important when planning an experiment to ensure reliable measurements. References: (A) (Pena Fernandez et al., 2020b); (B) (Tozzi et al., 2016); (C) (Pena Fernandez et al., 2021); (D) (Lu et al., 2019).
2.3 A unique approach to study complex deformation and validate computational models
While standard DVC requires time-lapsed imaging, and therefore slow step-wise loading, it is the only experimental technique that can measure the localization of complex deformations within the heterogeneous tissues. This fundamental feature of DVC enables the comprehensive study of the relationship between the tissue’s microstructure and its mechanical competence (Pena Fernandez et al., 2021), highlighting the effect of local defects or gradients in structure properties (e.g., density, orientation). Nevertheless, it should be noted that the assessment at low dimensional scales can also be done with a combination of high-resolution imaging (e.g., SR-microCT) and highly accurate DVC approaches that allow for a reasonably low sub-volume size.
DVC can also be used to study the deformation associated with the internal material interfaces [e.g., bone and calcified cartilage (Madi et al., 2020), bone and cartilage (Tozzi et al., 2020), bone and biomaterials (Joffre et al., 2017)]. The assessment of the localization of the deformation around the tissue-biomaterial interface can be used to optimise the design of medical devices or to study the integration of biomaterials and native tissue, especially when applied to material collected from animal studies (Pena Fernandez et al., 2019).
While DVC measurements require complex in situ mechanical testing and (usually) high resolution imaging, which may not be applicable in most in vivo conditions, the experimental assessment of local displacement and strain fields can be used to validate computational models based on laboratory or clinical images. In fact, DVC has been first used to validate the predictions of micro-Finite Element (microFE) models of trabecular bone (biopsy level) generated from microCT images (Zauel et al., 2006; Chen et al., 2017). Further validation has been performed to validate microFE models of more complex structures such as vertebral bodies with (Palanca et al., 2022) or without (Costa et al., 2017) lesions, and the mouse tibia (Oliviero et al., 2018). Recently, more complex models of trabecular bone with heterogeneous material properties (Knowles et al., 2021) or material nonlinearities (Pena Fernandez et al., 2022) have also been validated with DVC data. Finally, the DVC has also been used to validate the outputs of homogenised FE models based on clinical CT images including the human proximal femur (Ridzwan et al., 2018), scapula (Kusins et al., 2020a), humeral head (Kusins et al., 2020b), and vertebrae (Jackman et al., 2016).
3 Open challenges and current/future developments
Despite the steep growth of DVC applications in musculoskeletal research and bioengineering over the past 10 years, there are still some aspects that require further attention and research to fully exploit the technique for a variety of tissues, imaging modalities and loading conditions. This section will also suggest some potential avenues to empower/enrich DVC analysis for future work in this area.
3.1 Faster measurements and analysis
Conventional in situ microCT mechanics is performed in a stepwise manner (time-lapsed testing) where each loading is followed by a “holding period” to perform a full tomographic acquisition, which is less affected by moving artifacts due to stress relaxation. This is particularly important for all biomaterials as they exhibit a certain extent of viscoelasticity increasing from hard to soft tissues. To such extent, DVC analysis based on time-lapsed tests cannot be used to capture the physiological strain mechanisms in biological materials, as their time-dependent response may alter the local measured strains. In response to this need, major efforts have been made to perform fast imaging and time-resolved in situ SR-microCT experiments to capture the complex deformation and local strain-damage relationship of bone and biomaterials (Pena Fernandez et al., 2021), which can be further extended to soft tissues and hard-soft interfaces showing higher viscoelasticity and time-dependent mechanical behaviour. A possible route to potentially improve speed and efficiency of DVC is by implementing, for example, Projection-based DVC measurements (P-DVC), which has the advantage of high temporal resolution and a continuous loading (Jailin et al., 2019). The method has been used so far to evaluate engineering materials with fairly resolvable features and would be interesting to see its applicability to complex biological structures. Another way forward to improve efficiency in DVC performance is via its integration with machine learning strategies. Deep learning has already proven a powerful tool to classify bone tissue deformation stages to fracture using high-resolution SR-microCT data (Shen et al., 2021). Deep learning-based measurements have been recently introduced as an evolution of 2D DIC (Boukhtache et al., 2021) or for a DVC application (Duan and Huang, 2022), which in both cases was shown to greatly reduce computational complexity of the analysis and therefore improving efficiency. This area has a huge potential to improve measurement and generate more advanced models for understanding and prediction of musculoskeletal tissue mechanics.
3.2 Digital volume correlation of soft tissues
The use of DVC to extract local mechanical properties of musculoskeletal soft tissues and hard-soft interfaces is indeed very important and attractive. To this remit a few considerations are needed, particularly in terms of the imaging techniques/modalities used to acquire tissues under loading and consequently the ability of DVC to correlate those image patterns and output displacement/strain. One of the main aspects is in relation to how imaging setup and sample preparation could resolve the texture needed to run DVC. This can be done by employing imaging techniques that are historically developed and refined for soft biomaterials (e.g., confocal microscopy, second/third harmonic generation) where DVC has been used, for example, in strain measurements of the extracellular matrix (Roeder et al., 2004), tendons (Khodabakhshi et al., 2013), tendon cell-induced extracellular matrix deformations (Fung et al., 2018), a variety of other soft materials (Mac Donald and Ravichandran, 2019) and even thin sections of bone (Wentzell et al., 2016). Optical coherence tomography (OCT) combined with DVC is also a promising technique to investigate soft tissues. OCT-based DVC applications include measurement of displacement/strain fields in porcine aortic tissues (Acosta Santamaría et al., 2018) and biomechanical strains in human ocular tissue of patients affected by glaucoma (Czerpak et al., 2022). Its further implementation in musculoskeletal tissues such as articular cartilage and tendon/ligament would surely contribute to advance understanding of their biomechanics. Another image modality that was recently explored in combination to DVC is magnetic resonance imaging (MRI). MRI-based DVC application has been successfully used to obtain the full-field strain in human intervertebral disc (IVD) ex vivo (Tavana et al., 2020b). However, due to the limitations in volume imaged with confocal or availability/cost/resolution of MRI, the main input image for DVC is still from X-ray CT which is inherently less suitable to resolve soft tissues. To overcome this problem two main solutions are typically used to enhance structural features for DVC by either employing in-line phase-contrast imaging (achievable in SR-microCT like setups) or staining of the tissues. Interesting applications of DVC in soft tissues from phase-contrast SR-microCT images include the visualisation of microstructural deformation (Disney et al., 2019) and localised measurement of fibre-level orientation, curvature and strain of native intact intervertebral disc (Disney et al., 2022). Phase-contrast has been also exploited in laboratory microCT to improve contrast in native articular cartilage and mineralized tissue to quantify residual strain distribution at the cartilage-bone interface (Tozzi et al., 2020). Unfortunately, phase-contrast in situ mechanics in laboratory systems expose tissue to long periods of irradiation, which could affect its integrity and consequent measurements as will be further discussed in Section 3.5. Another route to enhance CT contrast in soft tissues is via radiopaque staining (e.g., iodine potassium iodide, phosphotungstic acid). A recent study relied on previously developed staining protocols (Clark et al., 2020b) to resolve chondrocyte distribution in human articular cartilage and measure full-field displacement and strain using DVC (Clark et al., 2021), but once again, most staining procedures can alter both tissue morphology and mechanical properties (Buytaert et al., 2014). New generation of staining agents with the potential of better preserving tissue integrity (de Bournonville et al., 2020) could address the degradation problem; however, even in such case, issues related to heterogeneity of tissue contrast due to staining penetration/power can notably reduce DVC ability to measure reliable and reproducible local deformations.
3.3 Increase the digital volume correlation accuracy/precision and validation
The accuracy and precision of DVC approaches have been comprehensively assessed using repeated microCT scans (Dall׳Ara et al., 2017; Liu and Morgan, 2007) or virtually deformed repeated microCT scans (Comini et al., 2019; Ryan et al., 2020), which consider the input image noise in contrast with the virtually moved or deformed images. While these assessments are required to provide a level of confidence of the DVC measurements, which are extremely dependent from the signal-to-noise ratio in the input images, the heterogeneity of the tested material, and the DVC parameters, these approaches are based on homogeneous (usually zero-strain fields) or simple (usually affine deformations) strain fields, which are unrealistic for most biological applications. Therefore, future tests of accuracy based on realistic heterogeneous deformations (e.g., from finite element model simulations) would provide a better assessment of local DVC errors.
From the standard assessment of DVC uncertainties it has been demonstrated for different bone structures that the precision increases by decreasing the spatial resolution of the DVC and that the precision depends on the image quality of the input images and in particular on how many features are clearly identified in them. Combining these properties it becomes clear that in order to increase the spatial resolution of the DVC input images with higher resolution and signal-to-noise ratio are required: for example the spatial resolution of the DVC increases of 13 times when applied to SR-microCT images (voxel size 1.6 μm) of the mouse tibia compared to in vivo microCT images (resolution 10.4 μm) of the same object. Nevertheless, while high-resolution images can be obtained using high-resolution laboratory microCT scanners or SR-microCT facilities, it should be noted that these imaging modalities are likely to perturb the mechanical competence of the scanned biological tissue (see Section 3.4).
3.4 Reducing invasiveness of X-ray imaging
It is established that prolonged X-ray exposure may severely alter the structural integrity and mechanical properties of musculoskeletal tissues, particularly in high-flux setups such as SR-microCT as extensively reported in a recent study (Dall'Ara et al., 2022). Briefly, as for SR-irradiated bone the degradation of the tissue and its mechanical properties is due to the alteration of collagen cross-linking (Barth et al., 2010; Barth et al., 2011), in soft tissues like articular cartilage degradation of mechanical properties has been instead associated with the decline in proteoglycan synthesis (Cicek, 2016). DVC has played a vital contribution in this area by further evaluating the effect of SR-microCT radiation on trabecular bone (Pena Fernandez et al., 2018a) and proposing strategies to mitigate this issue by reducing temperature in the in situ experiments (Pena Fernandez et al., 2018b). Despite such great efforts, more research is needed to clearly identify the mechanism of radiation-induced tissue degeneration and achieve the best compromise between image quality, number of in situ steps during the experiment and potential damage. All this is inevitably reflecting on the DVC analysis from such images and it is important to properly plan future studies where this potential issue is carefully evaluated, contextualising the DVC-based measurements with at least proper estimations of the radiation exposure on the specimens, to avoid reporting DVC results which may be affected by the invasiveness of the imaging modality.
3.5 Integrating mechanics in digital volume correlation inputs
The DVC algorithm usually ignores the local mechanical properties of the studied objects. In fact, the image correlation is completely unaware of the local material properties of the imaged object, but can only take into account for the features that affect the local porosity or anisotropy, if visible in the acquired input images. Nevertheless, the deformation of the considered tissue is also a function of those material properties that do not directly affect the image taken at a certain dimensional scale. For example, the material properties of the extracellular matrix of bone may be different from specimen to specimen (e.g., healthy tissue vs. tissue from osteogenesis imperfecta patients, which are more fragile for a molecular mutation in the collagen), even in specimens with similar bone mineral density and microstructure. While some DVC algorithms have been integrated with finite element solvers to consider the mechanical heterogeneity of the studied structures and back calculate their constitutive behaviour [i.e., the parameters of the chosen constitutive law), so far this approach has been used only for engineered materials (e.g., metamaterials (Valmalle et al., 2022)]. Applications to biological tissues with hierarchical organisation of the structure and the local heterogeneity in material properties due to local differences in mineralization (e.g., bone) or organisation of fibres (e.g., tendons, intervertebral disc), would require fitting complex constitutive laws and require further development.
Moreover, standard DVC analysis can only provide information about displacement and strain fields, with no information about the internal loads and stresses in the microstructure. DVC has been extensively used in the last decade to validate the displacement and strain outputs of FE models built from the undeformed images, both at the microstructural (microFE) (Palanca et al., 2022) and continuum (Kusins et al., 2020a) levels for bone. The validated FE models have then the potential to evaluate stresses in the musculoskeletal tissue, using the DVC data only to assign the proper boundary conditions to the FE models. Nevertheless, this approach has not been applied yet to study the local stresses in musculoskeletal tissues.
3.6 Clinical applications of digital volume correlation
A very important implementation of DVC is in the mechanical interpretation of clinical imaging. The technique was used to quantify the 3D full-field displacement and measure the helical axis of the subtalar joint in vivo during inversion-eversion in full weight-bearing clinical CT, providing a better understanding of the relative motion at the subtalar joint under physiological loading (Pena Fernandez et al., 2020b). MRI-based DVC was also used to assess in vivo strain uncertainties in the human talus, showing its potential to measure relevant levels of in vivo bone strain and to be used for a range of clinical applications (Tavana et al., 2020c). Another interesting application is the digital tomosynthesis (DTS) based DVC, which was employed to calculate full-field vertebral displacement maps, and in turn stiffness, using in vivo images acquired in both standing and standing-with-weight (8 kg) configurations (Oravec et al., 2019). Altogether, such use of DVC is very promising and can provide valuable insights in clinical diagnostics and surgical planning.
4 Conclusion
The goal of this minireview was to highlight the strengths, limitations and potential of the DVC approach to study the deformation of musculoskeletal tissues. Considering the limited space, the comprehensive review of every DVC study on musculoskeletal tissues was not in the remit of this manuscript. The authors hope that the reader will find this review useful and stimulating to engage with the usage of DVC for musculoskeletal research, to tackle current challenges and to further develop this approach in the musculoskeletal field and beyond.
Author contributions
All authors listed have made a substantial, direct, and intellectual contribution to the work and approved it for publication.
Funding
The Funding from the EPSRC (Frontier Grant Multisim EP/K03877X/1 and EP/S032940/1, and EP/P015778/1).
Acknowledgments
ED would like to acknowledge funding from the EPSRC (Frontier Grant Multisim EP/K03877X/1 and EP/S032940/1, and EP/P015778/1).
Conflict of interest
The authors declare that the research was conducted in the absence of any commercial or financial relationships that could be construed as a potential conflict of interest.
Publisher’s note
All claims expressed in this article are solely those of the authors and do not necessarily represent those of their affiliated organizations, or those of the publisher, the editors and the reviewers. Any product that may be evaluated in this article, or claim that may be made by its manufacturer, is not guaranteed or endorsed by the publisher.
References
Acosta Santamaría, V. A., Flechas García, M., Molimard, J., and Avril, S. (2018). Three-dimensional full-field strain measurements across a whole porcine aorta subjected to tensile loading using optical coherence tomography–digital volume correlation. Front. Mech. Eng. 4, 3. doi:10.3389/fmech.2018.00003
Barth, H. D., Launey, M. E., Macdowell, A. A., Ager, J. W., and Ritchie, R. O. (2010). On the effect of X-ray irradiation on the deformation and fracture behavior of human cortical bone. Bone 46, 1475–1485. doi:10.1016/j.bone.2010.02.025
Barth, H. D., Zimmermann, E. A., Schaible, E., Tang, S. Y., Alliston, T., and Ritchie, R. O. (2011). Characterization of the effects of x-ray irradiation on the hierarchical structure and mechanical properties of human cortical bone. Biomaterials 32, 8892–8904. doi:10.1016/j.biomaterials.2011.08.013
Bay, B. K. (1995). Texture correlation: a method for the measurement of detailed strain distributions within trabecular bone. J. Orthop. Res. 13, 258–267. doi:10.1002/jor.1100130214
Bayraktar, H. H., Morgan, E. F., Niebur, G. L., Morris, G. E., Wong, E. K., and Keaveny, T. M. (2004). Comparison of the elastic and yield properties of human femoral trabecular and cortical bone tissue. J. Biomechanics 37, 27–35. doi:10.1016/s0021-9290(03)00257-4
Boukhtache, S., Abdelouahab, K., Berry, F., Blaysat, B., Grédiac, M., and Sur, F. (2021). When deep learning meets digital image correlation. Opt. Lasers Eng. 136, 106308. doi:10.1016/j.optlaseng.2020.106308
Boulanaache, Y., Becce, F., Farron, A., Pioletti, D. P., and Terrier, A. (2020). Glenoid bone strain after anatomical total shoulder arthroplasty: In vitro measurements with micro-CT and digital volume correlation. Med. Eng. Phys. 85, 48–54. doi:10.1016/j.medengphy.2020.09.009
Buytaert, J., Goyens, J., De Greef, D., Aerts, P., and Dirckx, J. (2014). Volume shrinkage of bone, brain and muscle tissue in sample preparation for micro-CT and light sheet fluorescence microscopy (LSFM). Microsc. Microanal. 20, 1208–1217. doi:10.1017/s1431927614001329
Chen, Y., Dall Ara, E., Sales, E., Manda, K., Wallace, R., Pankaj, P., et al. (2017). Micro-CT based finite element models of cancellous bone predict accurately displacement once the boundary condition is well replicated: A validation study. J. Mech. Behav. Biomed. Mat. 65, 644–651. doi:10.1016/j.jmbbm.2016.09.014
Christen, D., Levchuk, A., Schori, S., Schneider, P., Boyd, S. K., and Muller, R. (2012). Deformable image registration and 3D strain mapping for the quantitative assessment of cortical bone microdamage. J. Mech. Behav. Biomed. Mat. 8, 184–193. doi:10.1016/j.jmbbm.2011.12.009
Cicek, E. (2016). Effect of X-ray irradiation on articular cartilage mechanical properties. Acta Phys. Pol. A 129, 200–202. doi:10.12693/aphyspola.129.200
Clark, J. N., Heyraud, A., Tavana, S., Al-Jabri, T., Tallia, F., Clark, B., et al. (2020). Exploratory full-field mechanical analysis across the osteochondral tissue-biomaterial interface in an ovine model. Mater. (Basel) 13, 3911. doi:10.3390/ma13183911
Clark, J. N., Garbout, A., Ferreira, S. A., Javaheri, B., Pitsillides, A. A., Rankin, S. M., et al. (2020). Propagation phase-contrast micro-computed tomography allows laboratory-based three-dimensional imaging of articular cartilage down to the cellular level. Osteoarthr. Cartil. 28, 102–111. doi:10.1016/j.joca.2019.10.007
Clark, J. N., Tavana, S., Clark, B., Briggs, T., Jeffers, J. R. T., and Hansen, U. (2021). High resolution three-dimensional strain measurements in human articular cartilage. J. Mech. Behav. Biomed. Mat. 124, 104806. doi:10.1016/j.jmbbm.2021.104806
Comini, F., Palanca, M., Cristofolini, L., and Dall'Ara, E. (2019). Uncertainties of synchrotron microCT-based digital volume correlation bone strain measurements under simulated deformation. J. Biomech. 86, 232–237. doi:10.1016/j.jbiomech.2019.01.041
Costa, M. C., Tozzi, G., Cristofolini, L., Danesi, V., Viceconti, M., and Dall'Ara, E. (2017). Micro Finite Element models of the vertebral body: Validation of local displacement predictions. PLoS One 12, e0180151. doi:10.1371/journal.pone.0180151
Czerpak, C. A., Kashaf, M. S., Zimmerman, B. K., Quigley, H. A., and Nguyen, T. D. (2022). The strain response to intraocular pressure decrease in the lamina cribrosa of glaucoma patients. Ophthalmol. Glaucoma. https://www.sciencedirect.com/science/article/abs/pii/S258941962200120X?via%3Dihub. doi:10.1016/j.ogla.2022.07.005
Dall׳Ara, E., Peña-Fernández, M., Palanca, M., Giorgi, M., Cristofolini, L., and Tozzi, G. (2017). Precision of digital volume correlation approaches for strain analysis in bone imaged with micro-computed tomography at different dimensional levels. Front. Mater. 4, 31. doi:10.3389/fmats.2017.00031
Dall'Ara, E., Barber, D., and Viceconti, M. (2014). About the inevitable compromise between spatial resolution and accuracy of strain measurement for bone tissue: a 3D zero-strain study. J. Biomech. 47, 2956–2963. doi:10.1016/j.jbiomech.2014.07.019
Dall'Ara, E., Bodey, A. J., Isaksson, H., and Tozzi, G. (2022). A practical guide for in situ mechanical testing of musculoskeletal tissues using synchrotron tomography. J. Mech. Behav. Biomed. Mat. 133, 105297. doi:10.1016/j.jmbbm.2022.105297
Danesi, V., Tozzi, G., and Cristofolini, L. (2016). Application of digital volume correlation to study the efficacy of prophylactic vertebral augmentation. Clin. Biomech. (Bristol, Avon. 39, 14–24. doi:10.1016/j.clinbiomech.2016.07.010
de Bournonville, S., Vangrunderbeeck, S., Ly, H. G. T., Geeroms, C., De Borggraeve, W. M., Parac-Vogt, T. N., et al. (2020). Exploring polyoxometalates as non-destructive staining agents for contrast-enhanced microfocus computed tomography of biological tissues. Acta Biomater. 105, 253–262. doi:10.1016/j.actbio.2020.01.038
Disney, C. M., Eckersley, A., McConnell, J. C., Geng, H., Bodey, A. J., Hoyland, J. A., et al. (2019). Synchrotron tomography of intervertebral disc deformation quantified by digital volume correlation reveals microstructural influence on strain patterns. Acta Biomater. 92, 290–304. doi:10.1016/j.actbio.2019.05.021
Disney, C. M., Mo, J., Eckersley, A., Bodey, A. J., Hoyland, J. A., Sherratt, M. J., et al. (2022). Regional variations in discrete collagen fibre mechanics within intact intervertebral disc resolved using synchrotron computed tomography and digital volume correlation. Acta Biomater. 138, 361–374. doi:10.1016/j.actbio.2021.10.012
Duan, X., and Huang, J. (2022). Deep learning-based digital volume correlation. Extreme Mech. Lett. 53, 101710. doi:10.1016/j.eml.2022.101710
Forsberg, F., Mooser, R., Arnold, M., Hack, E., and Wyss, P. (2008). 3D micro-scale deformations of wood in bending: Synchrotron radiation μCT data analyzed with digital volume correlation. J. Struct. Biol. X. 164, 255–262. doi:10.1016/j.jsb.2008.08.004
Fung, A. K., Paredes, J. J., and Andarawis-Puri, N. (2018). Novel image analysis methods for quantification of in situ 3-D tendon cell and matrix strain. J. Biomech. 67, 184–189. doi:10.1016/j.jbiomech.2017.11.030
Giorgi, M., and Dall'Ara, E. (2018). Variability in strain distribution in the mice tibia loading model: A preliminary study using digital volume correlation. Med. Eng. Phys. 62, 7–16. doi:10.1016/j.medengphy.2018.09.001
Grassi, L., and Isaksson, H. (2015). Extracting accurate strain measurements in bone mechanics: A critical review of current methods. J. Mech. Behav. Biomed. Mat. 50, 43–54. doi:10.1016/j.jmbbm.2015.06.006
Hosseini, H. S., Clouthier, A. L., and Zysset, P. K. (2014). Experimental validation of finite element analysis of human vertebral collapse under large compressive strains. J. Biomech. Eng. 136 (4), 041006. https://asmedigitalcollection.asme.org/biomechanical/article/136/4/041006/371035/Experimental-Validation-of-Finite-Element-Analysis. doi:10.1115/1.4026409
Hussein, A. I., Barbone, P. E., and Morgan, E. F. (2012). Digital volume correlation for study of the mechanics of whole bones. Procedia IUTAM 4, 116–125. doi:10.1016/j.piutam.2012.05.013
Hussein, A. I., Louzeiro, D. T., Unnikrishnan, G. U., and Morgan, E. F. (2018). Differences in trabecular microarchitecture and simplified boundary conditions limit the accuracy of quantitative computed tomography-based finite element models of vertebral failure. J. Biomech. Eng. 140. doi:10.1115/1.4038609
Jackman, T. M., Hussein, A. I., Curtiss, C., Fein, P. M., Camp, A., De Barros, L., et al. (2016). Quantitative, 3D visualization of the initiation and progression of vertebral fractures under compression and anterior flexion. J. Bone Min. Res. 31, 777–788. doi:10.1002/jbmr.2749
Jailin, C., Buljac, A., Bouterf, A., Hild, F., and Roux, S. (2019). “Projection-based measurement and identification,” in SEM 2019, Reno, NV, June 2019. https://hal.archives-ouvertes.fr/hal-02132459/document
Jang, A., Wang, B., Ustriyana, P., Gansky, S. A., Maslenikov, I., Useinov, A., et al. (2021). Functional adaptation of interradicular alveolar bone to reduced chewing loads on dentoalveolar joints in rats. Dent. Mat. 37, 486–495. doi:10.1016/j.dental.2020.12.003
Joffre, T., Isaksson, P., Procter, P., and Persson, C. (2017). Trabecular deformations during screw pull-out: a micro-CT study of lapine bone. Biomech. Model. Mechanobiol. 16, 1349–1359. doi:10.1007/s10237-017-0891-9
Karali, A., Kao, A. P., Meeson, R., Roldo, M., Blunn, G. W., and Tozzi, G. (2020). Full-field strain of regenerated bone tissue in a femoral fracture model. J. Microsc. 285, 156–166. doi:10.1111/jmi.12937
Karali, A., Kao, A. P., Zekonyte, J., Blunn, G., and Tozzi, G. (2021). Micromechanical evaluation of cortical bone using in situ XCT indentation and digital volume correlation. J. Mech. Behav. Biomed. Mat. 115, 104298. doi:10.1016/j.jmbbm.2020.104298
Khodabakhshi, G., Walker, D., Scutt, A., Way, L., Cowie, R. M., and Hose, D. R. (2013). Measuring three-dimensional strain distribution in tendon. J. Microsc. 249, 195–205. doi:10.1111/jmi.12009
Knowles, N. K., Kusins, J., Columbus, M. P., Athwal, G. S., and Ferreira, L. M. (2021). Experimental DVC validation of heterogeneous micro finite element models applied to subchondral trabecular bone of the humeral head. J. Orthop. Res. 40, 2039–2047. doi:10.1002/jor.25229
Kusins, J., Knowles, N., Ryan, M., Dall'Ara, E., and Ferreira, L. (2020). Full-field comparisons between strains predicted by QCT-derived finite element models of the scapula and experimental strains measured by digital volume correlation. J. Biomech. 113, 110101. doi:10.1016/j.jbiomech.2020.110101
Kusins, J., Knowles, N., Columbus, M., Oliviero, S., Dall'Ara, E., Athwal, G. S., et al. (2020). The application of digital volume correlation (DVC) to evaluate strain predictions generated by finite element models of the osteoarthritic humeral head. Ann. Biomed. Eng. 48, 2859–2869. doi:10.1007/s10439-020-02549-2
Le Cann, S., Tudisco, E., Tagil, M., Hall, S. A., and Isaksson, H. (2020). Bone damage evolution around integrated metal screws using X-ray tomography - in situ pullout and digital volume correlation. Front. Bioeng. Biotechnol. 8, 934. doi:10.3389/fbioe.2020.00934
Liu, L., and Morgan, E. F. (2007). Accuracy and precision of digital volume correlation in quantifying displacements and strains in trabecular bone. J. Biomech. 40, 3516–3520. doi:10.1016/j.jbiomech.2007.04.019
Lu, X., Fernandez, M. P., Bradley, R. S., Rawson, S. D., O'Brien, M., Hornberger, B., et al. (2019). Anisotropic crack propagation and deformation in dentin observed by four-dimensional X-ray nano-computed tomography. Acta Biomater. 96, 400–411. doi:10.1016/j.actbio.2019.06.042
Mac Donald, K., and Ravichandran, G. (2019). Confocal microscopy and digital volume correlation methods for intergranular force transmission experiments. Exp. Tech. 43, 457–468. doi:10.1007/s40799-018-0292-8
Madi, K., Tozzi, G., Zhang, Q. H., Tong, J., Cossey, A., Au, A., et al. (2013). Computation of full-field displacements in a scaffold implant using digital volume correlation and finite element analysis. Med. Eng. Phys. 35, 1298–1312. doi:10.1016/j.medengphy.2013.02.001
Madi, K., Staines, K. A., Bay, B. K., Javaheri, B., Geng, H., Bodey, A. J., et al. (2020). In situ characterization of nanoscale strains in loaded whole joints via synchrotron X-ray tomography. Nat. Biomed. Eng. 4, 343–354. doi:10.1038/s41551-019-0477-1
Martelli, S., Giorgi, M., Dall' Ara, E., and Perilli, E. (2021). Damage tolerance and toughness of elderly human femora. Acta Biomater. 123, 167–177. doi:10.1016/j.actbio.2021.01.011
Nazarian, A., Stauber, M., and Muller, R. (2005). Design and implementation of a novel mechanical testing system for cellular solids. J. Biomed. Mat. Res. 73, 400–411. doi:10.1002/jbm.b.30232
Oliviero, S., Giorgi, M., and Dall'Ara, E. (2018). Validation of finite element models of the mouse tibia using digital volume correlation. J. Mech. Behav. Biomed. Mat. 86, 172–184. doi:10.1016/j.jmbbm.2018.06.022
Oravec, D., Flynn, M. J., Zauel, R., Rao, S., and Yeni, Y. N. (2019). Digital tomosynthesis based digital volume correlation: A clinically viable noninvasive method for direct measurement of intravertebral displacements using images of the human spine under physiological load. Med. Phys. 46, 4553–4562. doi:10.1002/mp.13750
Palanca, M., Tozzi, G., Cristofolini, L., Viceconti, M., and Dall'Ara, E. (2015). Three-dimensional local measurements of bone strain and displacement: comparison of three digital volume correlation approaches. J. Biomech. Eng. 137 (7), 071006. https://asmedigitalcollection.asme.org/biomechanical/article/137/7/071006/442560/Three-Dimensional-Local-Measurements-of-Bone. doi:10.1115/1.4030174
Palanca, M., Tozzi, G., and Cristofolini, L. (2016). The use of digital image correlation in the biomechanical area: a review. Int. Biomech. 3, 1–21. doi:10.1080/23335432.2015.1117395
Palanca, M., Cristofolini, L., Dall'Ara, E., Curto, M., Innocente, F., Danesi, V., et al. (2016). Digital volume correlation can be used to estimate local strains in natural and augmented vertebrae: An organ-level study. J. Biomech. 49, 3882–3890. doi:10.1016/j.jbiomech.2016.10.018
Palanca, M., Bodey, A. J., Giorgi, M., Viceconti, M., Lacroix, D., Cristofolini, L., et al. (2017). Local displacement and strain uncertainties in different bone types by digital volume correlation of synchrotron microtomograms. J. Biomech. 58, 27–36. doi:10.1016/j.jbiomech.2017.04.007
Palanca, M., De Donno, G., and Dall'Ara, E. (2021). A novel approach to evaluate the effects of artificial bone focal lesion on the three-dimensional strain distributions within the vertebral body. PLoS One 16, e0251873. doi:10.1371/journal.pone.0251873
Palanca, M., Oliviero, S., and Dall'Ara, E. (2022). MicroFE models of porcine vertebrae with induced bone focal lesions: Validation of predicted displacements with digital volume correlation. J. Mech. Behav. Biomed. Mat. 125, 104872. doi:10.1016/j.jmbbm.2021.104872
Pena Fernandez, M., Cipiccia, S., Dall'Ara, E., Bodey, A. J., Parwani, R., Pani, M., et al. (2018). Effect of SR-microCT radiation on the mechanical integrity of trabecular bone using in situ mechanical testing and digital volume correlation. J. Mech. Behav. Biomed. Mat. 88, 109–119. doi:10.1016/j.jmbbm.2018.08.012
Pena Fernandez, M., Dall'Ara, E., Kao, A. P., Bodey, A. J., Karali, A., Blunn, G. W., et al. (2018). Preservation of bone tissue integrity with temperature control for in situ SR-MicroCT experiments. Mater. (Basel) 11, 2155. doi:10.3390/ma11112155
Pena Fernandez, M., Dall'Ara, E., Bodey, A. J., Parwani, R., Barber, A. H., Blunn, G. W., et al. (2019). Full-field strain analysis of bone-biomaterial systems produced by the implantation of osteoregenerative biomaterials in an ovine model. ACS Biomater. Sci. Eng. 5, 2543–2554. doi:10.1021/acsbiomaterials.8b01044
Pena Fernandez, M., Kao, A. P., Witte, F., Arora, H., and Tozzi, G. (2020). Low-cycle full-field residual strains in cortical bone and their influence on tissue fracture evaluated via in situ stepwise and continuous X-ray computed tomography. J. Biomech. 113, 110105. doi:10.1016/j.jbiomech.2020.110105
Pena Fernandez, M., Hoxha, D., Chan, O., Mordecai, S., Blunn, G. W., Tozzi, G., et al. (2020). Centre of rotation of the human subtalar joint using weight-bearing clinical computed tomography. Sci. Rep. 10, 1035. doi:10.1038/s41598-020-57912-z
Pena Fernandez, M., Kao, A. P., Bonithon, R., Howells, D., Bodey, A. J., Wanelik, K., et al. (2021). Time-resolved in situ synchrotron-microCT: 4D deformation of bone and bone analogues using digital volume correlation. Acta Biomater. 131, 424–439. doi:10.1016/j.actbio.2021.06.014
Pena Fernandez, M., Sasso, S. J., McPhee, S., Black, C., Kanczler, J., Tozzi, G., et al. (2022). Nonlinear micro finite element models based on digital volume correlation measurements predict early microdamage in newly formed bone. J. Mech. Behav. Biomed. Mat. 132, 105303. doi:10.1016/j.jmbbm.2022.105303
Rapagna, S., Berahmani, S., Wyers, C. E., van den Bergh, J. P. W., Reynolds, K. J., Tozzi, G., et al. (2019). Quantification of human bone microarchitecture damage in press-fit femoral knee implantation using HR-pQCT and digital volume correlation. J. Mech. Behav. Biomed. Mat. 97, 278–287. doi:10.1016/j.jmbbm.2019.04.054
Ridzwan, M. I. Z., Sukjamsri, C., Pal, B., van Arkel, R. J., Bell, A., Khanna, M., et al. (2018). Femoral fracture type can be predicted from femoral structure: A finite element study validated by digital volume correlation experiments. J. Orthop. Res. 36, 993–1001. doi:10.1002/jor.23669
Roberts, B. C., Perilli, E., and Reynolds, K. J. (2014). Application of the digital volume correlation technique for the measurement of displacement and strain fields in bone: a literature review. J. Biomech. 47, 923–934. doi:10.1016/j.jbiomech.2014.01.001
Roeder, B. A., Kokini, K., Robinson, J. P., and Voytik-Harbin, S. L. (2004). Local, three-dimensional strain measurements within largely deformed extracellular matrix constructs. J. Biomech. Eng. 126, 699–708. doi:10.1115/1.1824127
Ryan, M. K., Oliviero, S., Costa, M. C., Wilkinson, J. M., and Dall'Ara, E. (2020). Heterogeneous strain distribution in the subchondral bone of human osteoarthritic femoral heads, measured with digital volume correlation. Mater. (Basel) 13, 4619. doi:10.3390/ma13204619
Shen, S. C., Pena Fernandez, M., Tozzi, G., and Buehler, M. J. (2021). Deep learning approach to assess damage mechanics of bone tissue. J. Mech. Behav. Biomed. Mat. 123, 104761. doi:10.1016/j.jmbbm.2021.104761
Stavropoulou, E., Andò, E., Roubin, E., Lenoir, N., Tengattini, A., Briffaut, M., et al. (2020). Dynamics of water absorption in callovo-oxfordian claystone revealed with multimodal X-ray and neutron tomography. Front. Earth Sci. 8, 6. doi:10.3389/feart.2020.00006
Tavana, S., Clark, J. N., Prior, J., Baxan, N., Masouros, S. D., Newell, N., et al. (2020). Quantifying deformations and strains in human intervertebral discs using Digital Volume Correlation combined with MRI (DVC-MRI). J. Biomech. 102, 109604. doi:10.1016/j.jbiomech.2020.109604
Tavana, S., Masouros, S. D., Baxan, N., Freedman, B. A., Hansen, U. N., and Newell, N. (2020). The effect of degeneration on internal strains and the mechanism of failure in human intervertebral discs analyzed using digital volume correlation (DVC) and ultra-high field MRI. Front. Bioeng. Biotechnol. 8, 610907. doi:10.3389/fbioe.2020.610907
Tavana, S., Clark, J. N., Newell, N., Calder, J. D., and Hansen, U. (2020). In vivo deformation and strain measurements in human bone using digital volume correlation (DVC) and 3T clinical MRI. Mater. (Basel) 13, 5354. doi:10.3390/ma13235354
Tozzi, G., Zhang, Q. H., and Tong, J. (2014). Microdamage assessment of bone-cement interfaces under monotonic and cyclic compression. J. Biomech. 47, 3466–3474. doi:10.1016/j.jbiomech.2014.09.012
Tozzi, G., Danesi, V., Palanca, M., and Cristofolini, L. (2016). Elastic full-field strain analysis and microdamage progression in the vertebral body from digital volume correlation. Strain 52, 446–455. doi:10.1111/str.12202
Tozzi, G., Dall'Ara, E., Palanca, M., Curto, M., Innocente, F., and Cristofolini, L. (2017). Strain uncertainties from two digital volume correlation approaches in prophylactically augmented vertebrae: Local analysis on bone and cement-bone microstructures. J. Mech. Behav. Biomed. Mat. 67, 117–126. doi:10.1016/j.jmbbm.2016.12.006
Tozzi, G., Pena Fernandez, M., Davis, S., Karali, A., Kao, A. P., and Blunn, G. (2020). Full-field strain uncertainties and residuals at the cartilage-bone interface in unstained tissues using propagation-based phase-contrast XCT and digital volume correlation. Mater. (Basel) 13, 2579. doi:10.3390/ma13112579
Turunen, M. J., Le Cann, S., Tudisco, E., Lovric, G., Patera, A., Hall, S. A., et al. (2020). Sub-trabecular strain evolution in human trabecular bone. Sci. Rep. 10, 13788. doi:10.1038/s41598-020-69850-x
Valmalle, M., Vintache, A., Smaniotto, B., Gutmann, F., Spagnuolo, M., Ciallella, A., et al. (2022). Local–global DVC analyses confirm theoretical predictions for deformation and damage onset in torsion of pantographic metamaterial. Mech. Mater. 172, 104379. doi:10.1016/j.mechmat.2022.104379
Wearne, L. S., Rapagna, S., Taylor, M., and Perilli, E. (2022). Micro-CT scan optimisation for mechanical loading of tibia with titanium tibial tray: A digital volume correlation zero strain error analysis. J. Mech. Behav. Biomed. Mat. 134, 105336. doi:10.1016/j.jmbbm.2022.105336
Wentzell, S., Nesbitt, R. S., Macione, J., and Kotha, S. (2016). Measurement of lacunar bone strains and crack formation during tensile loading by digital volume correlation of second harmonic generation images. J. Mech. Behav. Biomed. Mat. 60, 148–156. doi:10.1016/j.jmbbm.2015.12.027
Yan, L., Cinar, A., Ma, S., Abel, R., Hansen, U., and Marrow, T. J. (2020). A method for fracture toughness measurement in trabecular bone using computed tomography, image correlation and finite element methods. J. Mech. Behav. Biomed. Mat. 109, 103838. doi:10.1016/j.jmbbm.2020.103838
Zauel, R., Yeni, Y. N., Bay, B. K., Dong, X. N., and Fyhrie, D. P. (2006). Comparison of the linear finite element prediction of deformation and strain of human cancellous bone to 3D digital volume correlation measurements. J. Biomech. Eng. 128, 1–6. doi:10.1115/1.2146001
Keywords: digital volume correlation, musculoskeletal tissues, biomaterials, in situ mechanics, bone
Citation: Dall’Ara E and Tozzi G (2022) Digital volume correlation for the characterization of musculoskeletal tissues: Current challenges and future developments. Front. Bioeng. Biotechnol. 10:1010056. doi: 10.3389/fbioe.2022.1010056
Received: 02 August 2022; Accepted: 20 September 2022;
Published: 04 October 2022.
Edited by:
Alessandra Carriero, City College of New York (CUNY), United StatesReviewed by:
Jeroen Eyckmans, Boston University, United StatesStephane Avril, MINES Saint-Etienne, Institut Mines-Télécom, France
Jerome Molimard, Centre Ingénierie et Santé, Ecole des Mines de Saint-Étienne, France
Elizabeth A. Zimmermann, McGill University, Canada
Copyright © 2022 Dall’Ara and Tozzi. This is an open-access article distributed under the terms of the Creative Commons Attribution License (CC BY). The use, distribution or reproduction in other forums is permitted, provided the original author(s) and the copyright owner(s) are credited and that the original publication in this journal is cited, in accordance with accepted academic practice. No use, distribution or reproduction is permitted which does not comply with these terms.
*Correspondence: Enrico Dall’Ara, ZS5kYWxsYXJhQHNoZWZmaWVsZC5hYy51aw==