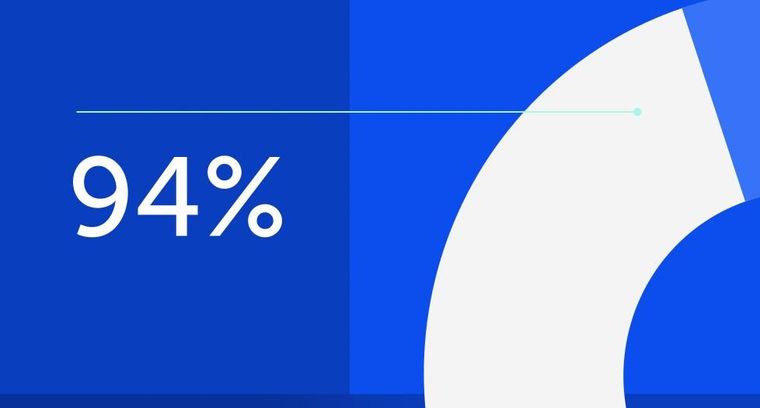
94% of researchers rate our articles as excellent or good
Learn more about the work of our research integrity team to safeguard the quality of each article we publish.
Find out more
MINI REVIEW article
Front. Bioeng. Biotechnol., 14 January 2022
Sec. Synthetic Biology
Volume 9 - 2021 | https://doi.org/10.3389/fbioe.2021.826479
This article is part of the Research TopicMolecular Engineering of Sensory Mechanisms in Bacteria for Biosensing Technologies and Novel Tools for Microbial EngineeringView all 7 articles
The multifaceted and heterogeneous nature of depression presents challenges in pinpointing treatments. Among these contributions are the interconnections between the gut microbiome and neurological function termed the gut-brain axis. A diverse range of microbiome-produced metabolites interact with host signaling and metabolic pathways through this gut-brain axis relationship. Therefore, biosensor detection of gut metabolites offers the potential to quantify the microbiome’s contributions to depression. Herein we review synthetic biology strategies to detect signals that indicate gut-brain axis dysregulation that may contribute to depression. We also highlight future challenges in developing living diagnostics of microbiome conditions influencing depression.
The bidirectional nature of the gut-brain axis links the gut microbiota with psychological behaviors (Mayer, 2011; Cryan and Dinan, 2012). Within this gut-brain axis, metabolites produced by the microbiota alter the host immune, metabolic and neural pathways that contribute to behavioral changes (Zheng et al., 2016). The interconnections between gut microbiota metabolites and host behavior are evidenced by human fecal transplantation experiments into mice (De Palma et al., 2017). These studies suggest that microbiota composition leads to dysregulation of the gut-brain axis, which has been proposed to influence depression (Pinto-Sanchez et al., 2017; Kelly et al., 2019). Several in-depth reviews have examined the rich interconnections with the gut-brain axis (Rutsch et al., 2020; Margolis et al., 2021; Morais et al., 2021). Here we introduce connections between the microbiome and mental health that present opportunities for synthetic biology (Figure 1).
FIGURE 1. Monitoring depression with biosensors through the gut-brain axis. Engineered biosensors might be used for monitoring alterations in gut metabolism that could impact depression. Gut inflammation plays a role in mental disorders. Nitric oxide (NO) and tetrathionate (S4O62-) present inflammation biomarkers that the engineered bacterial sensor can detect. Gut microbiota can alter the metabolites. For example, tryptophan metabolites and short-chain fatty acids (SCFAs) are associated with human behavior problems. The signals indicating mental disease can be coupled with general signals in the digestion system, such as pH and O2 level. The specificity of biosensors can be improved through multi-input logic gates. The icons of the brain, gut, and microbes are adapted from ‘Brain (lateral view)’ “Intestines” and “Spirillum (flagella)” by BioRender.com (2021). Retrieved from https://app.biorender.com/biorender-templates.
A current diagnostic test to quantify biomarkers may involve samples from cerebral spinal fluid and blood analyzed by techniques such as high-performance liquid chromatography (HPLC), mass spectrometry (MS), and nuclear magnetic resonance (NMR) (Haroon et al., 2020; Du et al., 2021; Pu et al., 2021). In contrast, synthetic biology offers next-generation diagnostic strategies through the engineering of probiotic bacteria. Early successes of the synthetic biology approaches are in clinical trials targeting metabolic diseases and cancers (McNerney et al., 2021). These strategies have been reviewed (Song et al., 2019; Barra et al., 2020; Lazar and Tabor, 2021; McNerney et al., 2021), including the engineering of probiotics to produce synthetic metabolites and therapeutic proteins (Kang et al., 2020). Collectively, these technologies have enabled the sensing of tumors and the release of therapeutics (Chien et al., 2017).
We are now poised to leverage these new synthetic biology tools to study, diagnose, and treat mental health disorders. Indeed, psychobiotics, including Bifidobacterium and Lactobacillus families (Mayer et al., 2014) have been studied to positively influence the gut-brain axis (Sarkar et al., 2016), and exhibited improved mental health (Dinan et al., 2013). Altered gut microbiota profiling was detected in patients, and probiotic treatments were shown to have promising improvement for depression (Kelly et al., 2019; Flux and Lowry, 2020). As a chassis for biosensing technologies, both bacteria and bacteriophages present opportunities to characterize the gut-brain axis.
On the one hand, bacteriophages can be engineered as biosensors to target specific bacteria within the microbiota (Bhardwaj et al., 2017). With specificity towards selected bacterial species, bacteriophages have been proposed as therapeutic vectors (Sulakvelidze et al., 2001; Summers, 2001), and technologies using bacteriophages are being evaluated in clinical trials (Lu and Koeris, 2011; Lenneman et al., 2021). Notably, protein engineering strategies upon the T3 phage tail fiber protein to engineer host-range specificity (Yehl et al., 2019). Bacteriophages thus present significant opportunities to track specific bacterial species that have been associated with mental health.
In a complementary manner, bacteria can be engineered as biosensors that detect the collective metabolic output from microbes and hosts. Emerging evidence of the gut-brain axis suggests a qualitative correlation between metabolite levels in the gut and the brain. With further development of technologies, biosensor surveillance of gut metabolites may lead to non-invasive, precise, personalized medicine for mental health-associated diseases (Zmora et al., 2018). Moreover, biosensors have the potential to quantitively score conditions within the gut that contribute to mental health. Sensing microbe species and metabolites collectively might help distinguish primary contributors to the changes in metabolite levels. However, the biosensor applications will be challenged by the specificity of metabolite signals, the dynamic range of signals, promoter leakiness, and potential crosstalk with other pathways. This minireview focuses on the current state and future challenges of bacterial biosensors that can monitor the impact of the microbiota upon the gut-brain axis.
Tryptophan is metabolized into three downstream pathways: 5-hydroxytryptamine, kynurenine, and indole pathways (Hubbard et al., 2015; Agus et al., 2018; Gheorghe et al., 2019; Kaur et al., 2019; Benech et al., 2021; Modoux et al., 2021). Variations in how the gut microbiota metabolizes tryptophan might play a role in depression (Kelly et al., 2016). Indole functions as an interkingdom signaling molecule (Lee et al., 2015) through stimulating the aryl hydrocarbon receptor (AhR) pathway (Agus et al., 2018; Liu et al., 2021). Metagenomic analysis of enzymes that produce indole (i.e., TnaA) suggests that gut microbiota from different individuals has a varied capacity to synthesize indole, leading to variations in indole within humans (Jaglin et al., 2018). Other studies in mice under chronic mild stress observed that indole production correlated with changes in mice behavior (Mir et al., 2020), while overproduction of indole-induced anxiety-like behavior in rats (Jaglin et al., 2018). In contrast, studies in mice have shown that indole stimulation of the AhR regulates neurogenesis in the adult hippocampus, a critical step in recovering from depression (Wei et al., 2021). Thus, maintenance of indole levels appears critical for proper gut-brain axis regulation (Jaglin et al., 2018; Mir et al., 2020).
Protein engineering of the tryptophan repressor protein could enable rapid detection of tryptophan-derived metabolites. A fluorescence resonance energy transfer (FRET) sensor for the signaling molecule indole-3-acetic acid (I3AA) was reported with such a protein engineering approach. The sensor was designed by altering the specificity of the E. coli tryptophan repressor for I3AA binding (Herud-Sikimić et al., 2021). The I3AA FRET sensor was tested for tracking dynamic fluctuations of I3AA signals in plants. The same strategy can be applied to engineer FRET biosensors for other indole metabolites. Moreover, future preclinical studies applying similar FRET biosensors may enable three-dimension mapping of indole metabolism along the digestive tract.
Other than indole, indole-3-aldehyde (I3A) may also activate the AhR receptor and regulate central nervous system inflammation (Rothhammer et al., 2016). To detect I3A, a biosensor was engineered based on signaling parts from a bacterial two-component system (TCS) (Figure 2Ai). The engineered histidine kinase is a hybrid protein carrying an I3A-sensing Per-Arnt-Sim (PAS) domain (Wang et al., 2021). The optimization within the promotor region improved the dynamic range and resulted in ∼35 fold change in mCherry signal upon sensing I3A. The engineered E. coli selectively sense I3A over other indole metabolites. However, at higher concentrations, the I3A sensor can respond to indole and I3AA. This I3A-sensing biosensor raises the potential of developing biosensors to investigate the impact of indole metabolites on mental health disorders.
FIGURE 2. Input and output modules for biosensors. (A) Input modules for detecting potential depression-associated signals. POI: protein of interest. (i–ii) Two-component systems (TCSs) that were used in biosensors. (i) I3A-HK/FixJ: an engineered sensor for indole-3-aldehyde (I3A). (ii) TtrSR: tetrathionate sensor (iii–v) Transcription factors and sigma factors that have been used as biosensor parts. (iii) NorR: nitric oxide sensing regulator. (iv) FNR: fumarate and nitrate reduction regulatory protein. (v) CadC: a membrane-bound transcriptional regulator. (B) Output modules for biosensors (i–iv). Outputs for in situ detection. (i) Essential genes are used as outputs. asd: aspartate-semialdehyde dehydrogenase; glms: glucosamine-6-phosphate synthase. Both asd and glms encoded proteins are involved in Gram-negative bacterial cell wall biosynthesis. In the engineered S. Typhimurium ELH1301 Δasd Δglms strain, asd and glms can be induced once sensing the signals. The compensation of the essential genes will increase the cell population in the designated environment. (ii) Split fluorescent protein is used as output for the signal. The ligand binding to the sensing domain induces a conformational change, which leads to the reunion of domains from a split fluorescent protein. The reunited fluorescent protein generates detectable signals. (iii) The fluorescence resonance energy transfer (FRET) is used for output detection. The ligand binding to the sensing domain induces a conformational change, which leads to the FRET for detectable output fluorescent signal. (iv) The operon for gas vesicles can serve as the output for a biosensor. The expressed proteins can assemble into gas vesicles, allowing ultrasound signals from tissue in depth. (v,vi) Outputs for delayed detection. (v) Recombinases can irreversibly invert gene of interest (GOI) on the DNA upon detecting signals. The readout can be measured through DNA sequencing for complex circuits. (vi) The toggle circuit controls the GOI as a memory module. The icon of the gut is adapted from “Intestines” by BioRender.com (2021). Retrieved from https://app.biorender.com/biorender-templates.
The microbiota may also stimulate gut inflammation (Miller and Raison, 2016), which plays a role in depression (Liu et al., 2020). The immune system signals to the brain through inflammatory cytokines (Flux and Lowry, 2020). Recent research found that inflammation can increase the permeability of the blood-brain barrier (Varatharaj and Galea, 2017) to allow cytokines to cross. At the same time, microbes can modulate gut-associated lymphoid tissue, resulting in various cytokines (Desbonnet et al., 2010; Rudzki and Szulc, 2018). Therefore, quantification of inflammation may assist in developing treatment regimens for depression.
Tetrathionate is a small molecule signal indicating inflammation (Winter et al., 2010). Host cells convert the toxic hydrogen sulfide generated from bacteria to the thiosulphate (Levitt et al., 1999), which interacts with reactive oxygen species generated during gut inflammation to form the tetrathionate (Winter et al., 2010). Salmonella typhimurium sense the tetrathionate by TCS TtrSR, which regulates tetrathionate reductase (TtrBCA) in the tetrathionate respiration (Hensel et al., 1999). The E. coli Nissle 1917 was successfully engineered to carry a TtrSR for detecting tetrathionate in the inflamed mice gut (Figure 2Aii) (Daeffler et al., 2017). The Silver lab further applied this tetrathionate sensing system to regulate a memory toggle switch within the mouse gut commensal E. coli NGF-1 strain. Their studies demonstrated that the bacterial sensor with the memory circuit maintained and recorded tetrathionate signals after 160 days in mice gut (Riglar et al., 2017).
The nitric oxide (NO) also serves as an inflammatory biomarker (Kimura et al., 1997; Sharma et al., 2007). NO is a water-soluble gaseous small molecule synthesized from l-arginine by nitric oxide synthases (NOSs) in mammalian cells. The expression of inducible nitric oxide synthase (iNOS) is stimulated by inflammation in epithelial cells. Therefore, the upregulation of NO by iNOS under inflammation indicates that NO may serve as an informative biomarker of depression.
There are two major regulators, NorR (nitric oxide sensing regulator) and NsrR (nitric oxide-sensitive transcriptional repressor), that mediate the bacterial response to NO stress (Spiro, 2007; Stern and Zhu, 2014). NorR directly binds NO and regulates genes for NO detoxification in E. coli and V. cholerae (Figure 2Aiii) (D'Autréaux et al., 2005; Stern et al., 2012). To leverage these natural NO-sensing parts, NorR was coupled to the regulation of a DNA recombinase, which allows for the permanent recording of NO signals associated with gut inflammation tested in explant cultures of mouse ileum (Archer et al., 2012). For optimizing NO biosensors, a positive feedback loop was introduced to fine-tune the level of NorR in E. coli Nissle 1917 (Chen et al., 2021). With a positive feedback loop, the NO also induces the NorR expression, and the increase of activated NorR provides the biosensor with a larger dynamic range.
The reporting of symptoms related to inflammation can vary from person to person, which may complicate the clinical correlation of digestive inflammation with a neurological disorder. The development of NO and tetrathionate biosensors offers new tools to correlate the earliest stages of inflammation with depression-associated gut-brain axis dysregulation.
The spatial heterogeneity of microbial populations across the digestive tract is challenging to detect in live organisms (Donaldson et al., 2016). This spatial heterogeneity is rooted in the variation of metabolite producers, metabolite sinks, and diffusion from the production site to the detection site. This requires approaches to resolve metabolite spatial heterogeneity. The mapping of signals across the digestive tract requires landmark signals that can report on the address of detected signals. Two parameters that vary throughout the digestive tract tissues are oxygen (O2) and pH (Carreau et al., 2011; Aoi and Marunaka, 2014; De Santis and Singer, 2015). Oxygen concentration decreases from tissue to lumen. Also, the small intestine has a higher O2 level and lower pH, while the large intestine has a lower O2 level and higher pH. Coupling O2 sensing (Figure 2Aiv) and pH sensing (Figure 2Av) with the ability to sense biomarker metabolites would enable the spatiotemporal sensing capabilities needed for engineered bacteria to target diseases precisely.
Recently, work from the Tal Danino group engineered bacteria to carry a AND circuit of hypoxia and lactate sensors (Chien et al., 2021). The AND gate is built based on two transcriptional regulators. The fumarate and nitrate reduction regulatory protein (FNR) senses O2 level (Figure 2Aiv). FNR was engineered to regulate asd, an essential gene that encodes an aspartate-semialdehyde dehydrogenase for the lysine, threonine, and methionine biosynthesis. In tandem, the l-lactate dehydrogenase operon regulatory protein (LldR) senses lactic acid. LldR was engineered to regulate glms, another essential gene that encodes a glucosamine-6-phosphate synthase for the biosynthesis of building blocks for the bacterial cell wall. Therefore, bacterial replication is only permitted when both essential genes are expressed when the environmental O2 and lactic acid are in the designated range for the S. Typhimurium ELH1301 Δasd Δglms. S. Typhimurium was used in cancer therapy because its population increases and accumulates around tumors. However, S. Typhimurium can also localize to the liver and spleen (Hoffman, 2016). Because hypoxia and high lactate are unique signals in tumor environments, in mice experiments, these hypoxia-lactate sensing S. Typhimurium will improve safety with increased tumor specificity compared to the O2-only and lactate-only sensing strains. Similar genetic circuits might be applied for identified tissue microenvironments in which the O2 levels and pH are dramatically different (Pereira and Berry, 2017; Singhal and Shah, 2020). When sensing O2 and pH coupled with depression biomarker sensors, biosensors with complex circuits may identify specific microbial niches that produce depression-associated biomarkers.
Microbial communities can be spatially and compositionally heterogeneous and change with maturation. One strategy discussed above is the regulation of bacterial growth to defined niches. Based on signal sensing, outputs can control the expression of essential genes like asd and glms discussed above (Figure 2Bi). A second approach applies imaging technologies to map how biomarker signals change with time. Such approaches may have utility in the preclinical studies to connect gut-brain axis dysregulation with depression symptoms. The split fluorescent proteins (Figure 2Bii) and FRET (Figure 2Biii) have been developed as detecting approaches. The binding of signaling molecules to the FRET biosensor results in conformational changes that alter the distance between the donor and acceptor fluorophores leading to changes in the FRET signal.
In the I3AA FRET biosensor we discussed above (Herud-Sikimić et al., 2021), the binding of I3AA to the repressor induces structural changes that can be detected by FRET. A similar FRET strategy was applied to histidine kinase, the protein that senses environmental signals in the bacterial TCS (Duvall and Childers, 2020). In this example, the Caulobacter crescentus kinase CckA was engineered to contain both donor and acceptor fluorescent proteins. The authors demonstrated that the engineered histidine kinase successfully responds to cyclic-di-GMP. This FRET-based approach could potentially be applied to the I3A-sensing (Wang et al., 2021) and the tetrathionate-sensing histidine kinases (Daeffler et al., 2017) to spatially and temporally map these signals within microbial populations.
A third strategy has utilized circular permuted GFP, whose fluorescence is sensitive to ligand binding. This strategy has been recently applied to engineer a sensor for GABA (γ-aminobutyric acid) (Marvin et al., 2019), a major inhibitory neurotransmitter of the central nervous system (CNS). The biosensor design included inserting a circularly permuted fluorescent protein into the Pseudomonas fluorescens GABA binding protein Pf622. This GABA biosensor was applied to imaging experiments in mice and zebrafish. Bacterial gut commensals have also been found to have the enzymatic capacity to decarboxylate glutamate to generate GABA (Pokusaeva et al., 2017; Strandwitz et al., 2019). Therefore, future development of GABA biosensors applied towards microbial communities will be insightful.
Along the longitudinal and transverse axes in the gut, distinct habitats create a heterogeneous distribution of microbes and their metabolites (Tropini et al., 2017). While engineered biosensors would enable spatiotemporal sensing capabilities, visualization of bacteria in the gut will be required for resolving spatial heterogeneity of microbial populations. Fluorescence imaging approaches are limited in application as light cannot penetrate deeply into tissues. Ultrasound detection methods have been developed to track signals deep within tissues (Figure 2Biv). These technologies leverage gas vesicles (GVs), protein nanostructures found from diverse bacteria and archaea (Walsby, 1994; Pfeifer, 2012). GVs are filled with gas and thus contribute to buoyancy. The unique features of GVs allow for the scattering of sound waves (Shapiro et al., 2014). Therefore, GVs present opportunities for detecting signals by ultrasound, which allow deep penetrant imaging with a <100 µM spatial resolution. This technology has been developed with engineered GV genes as acoustic reporter genes (ARG). Furthermore, their expression in bacteria and detection by ultrasound have been demonstrated (Bourdeau et al., 2018).
Genetic engineering has tuned the sensitivity of GVs to varied acoustic pressure (Figure 2Biv) (Shapiro et al., 2014). Therefore, distinct GVs can be distinguished by their sensitivity to acoustic pressure (Lakshmanan et al., 2016; Hurt et al., 2021) to monitor two signals simultaneously. The ARGs have been engineered with improved expression (Lakshmanan et al., 2017; Hurt et al., 2021) and various acoustic properties (Bourdeau et al., 2018; Hurt et al., 2021) in different cell types, such as E. coli and Salmonella typhimurium (Bourdeau et al., 2018). The engineered bacteria with ARGs were also tested deep inside a mammalian host within the tumors (Hurt et al., 2021) and gastrointestinal tract (Bourdeau et al., 2018). ARGs thus present maturing diagnostic technologies for real-time reporting of gut-brain axis dysregulation.
While FRET biosensors offer real-time monitoring, the longevity of these signals is limited by the protein’s half-life, which can span over the time frame of ∼30 min to 20–200 h (Eden et al., 2011; Schwanhäusser et al., 2011). Longer-term storage of signals has utilized recombinases, enzymes that invert the orientation of a piece of DNA (Figure 2Bv). The recombinases-based genetic circuit allows recording in the form of altered DNA upon transient exposure to signal. The recorded information in DNA can be recalled later by PCR, which allows the engineered biosensor to maintain a long-term memory (Yang et al., 2014).
As an alternative, the toggle switch-like circuit can also record and store signals for a long time (Figure 2Bvi). A toggle switch is a bistable gene-regulatory network (Gardner et al., 2000). The two repressors mutually repress the expression of the other in a toggle switch, which flips between the two states. In a biosensor, the expression of one repressor is induced by the signal. The induction of one repressor turns and keeps the toggle switch into one of the states. Thus, the biosensor will sense and memorize the signal and can be read out through the analysis of samples such as stools.
While good initial progress has been made in identifying biosensor input and output strategies, several steps will be needed to apply these biosensors to study mental health. This includes validating and tuning current biosensors for metabolite surveillance within the mouse and human gut microbiome environments. For example, studies will be needed to quantify and compare biomarker signal levels under health and disease conditions, and this may require engineering biosensors’ IC50 for needed sensitivity. Beyond measurement validation, the metabolic landscape within the mouse gut contains multiple sources and sinks. The flux of gut metabolites may be variably directed towards other non-depression-associated pathways. Therefore, preclinical studies in mice correlating biosensor readout with depression symptoms and metabolite levels in the brain will be needed.
Moreover, while FDA approved the first cell therapy in 2017 (Braendstrup et al., 2020), the and microbial sensors have been tested in a pig, biosensors remain to be deployed in humans (Mimee et al., 2018). Regarding the use of microbial biosensors, ensuring biosafety is another challenge. To this end, bacterial pathogenicity, survival, and the risk of mutation should be minimized and under strict control (McNerney et al., 2021).
Another major challenge for cell-based diagnostics and therapeutics is how to target diseases of interest specifically (Cubillos-Ruiz et al., 2021). Currently known signals are associated with a wide range of diseases for the microbial biosensors discussed above. For example, indole(s) and indole-producing bacteria are correlated with inflammatory bowel diseases (IBD), metabolic diseases, neurodegenerative diseases, and cancers (Lee et al., 2015). In addition, many mental health diseases share overlapping symptoms reported by patients (Ronald et al., 2008; Grisanzio et al., 2018; Plana-Ripoll et al., 2019), which present challenges in the deconvolution of many diseases correlated with variations in the microbiome. This suggests a need for additional biomarkers with improved specificity to distinguish depression from other mental health disorders. Alternatively, with only low to moderate specificity biomarkers, strategies to detect a suite of biomarkers may provide another route to increase specificity.
As an example of integrating multiple cues, hypoxia-lactate sensing S. Typhimurium has increased tumor specificity (Chien et al., 2021). Recent work demonstrated that living cells that sense up to three signals precisely recognize targeted cancer (Williams et al., 2020). New studies will be needed to demonstrate how integrated sensing of multiple cues correlates with mental health disease states. Towards the goal of signal integration, there has been success in both protein-based (Ho et al., 2021; Vishweshwaraiah et al., 2021) and transcriptional logic gates to enhance specificity by processing multiple inputs (Cubillos-Ruiz et al., 2021).
In bacteria, TCSs exhibit diverse mechanisms to integrate and process multiple signals. TCSs process multi-inputs through kinase networks. Crosstalks between multiple kinases increase the signal integration capabilities of those networks (Francis and Porter, 2019). In addition, kinase signaling pathways integrate signals through tandems sensors where a single histidine kinase has more than one sensory domain. Interestingly, natural (Fang et al., 2015; Mann et al., 2016) and synthetically engineered (Moglich et al., 2010) tandem sensors were found integrating signals as logic gates. The design principles for how tandem sensor structure encodes logic remain poorly developed. We envision that the rapid development in machine learning-based protein structure prediction (Baek et al., 2021; Tunyasuvunakool et al., 2021) and engineering (Yang et al., 2019) will increase our capabilities to design and understand the mechanisms of tandem sensor processing.
While progress has been made towards biosensors for indole metabolites, inflammation, pH, and oxygen, new biosensors for many biomarkers of mental health issues will be needed. Notably, we currently lack well-characterized microbial biosensors for kynurenine metabolites. Another key family of depression-associated metabolites is short-chain fatty acids (SCFAs). By fermentation of unabsorbed foods within our digestive tract, the microbiota produces SCFAs (Dalile et al., 2019) that include acetate, butyrate, and propionate (Erny et al., 2015; Kelly et al., 2019). These SCFAs impact the central nervous system through orphan G protein-coupled receptors (GPCRs) (Dalile et al., 2019) and histone deacetylases (HDACs), which impact depression and other mental diseases (Waldecker et al., 2008; Soliman and Rosenberger, 2011). These results suggest the need to develop bacterial biosensors for SCFAs as biomarkers of gut-brain axis dysregulation.
The characterization of the I3A and tetrathionate sensing histidine kinases highlights the potential sensing capabilities of bacterial TCS. We hypothesize that microbes colonized in the gut provide an extensive treasure trove of sensory domains. Furthermore, gut microbes constantly respond to metabolites fluctuation at the colonized location. Therefore, characterization of poorly studied TCSs in the genome of gut microbes may reveal new sensing interchangeable parts to construct biosensors.
JW and WSC wrote the manuscript. All authors contributed to the manuscript and approved the submitted version.
Start-up funds to WC provided by the University of Pittsburgh.
The authors declare that the research was conducted in the absence of any commercial or financial relationships that could be construed as a potential conflict of interest.
All claims expressed in this article are solely those of the authors and do not necessarily represent those of their affiliated organizations, or those of the publisher, the editors and the reviewers. Any product that may be evaluated in this article, or claim that may be made by its manufacturer, is not guaranteed or endorsed by the publisher.
Agus, A., Planchais, J., and Sokol, H. (2018). Gut Microbiota Regulation of Tryptophan Metabolism in Health and Disease. Cell Host & Microbe 23, 716–724. doi:10.1016/j.chom.2018.05.003
Aoi, W., and Marunaka, Y. (2014). Importance of pH Homeostasis in Metabolic Health and Diseases: Crucial Role of Membrane Proton Transport. Biomed. Res. Int. 2014, 598986. doi:10.1155/2014/598986
Archer, E. J., Robinson, A. B., and Süel, G. M. (2012). Engineered E. coli that Detect and Respond to Gut Inflammation through Nitric Oxide Sensing. ACS Synth. Biol. 1, 451–457. doi:10.1021/sb3000595
Baek, M., DiMaio, F., Anishchenko, I., Dauparas, J., Ovchinnikov, S., Lee, G. R., et al. (2021). Accurate Prediction of Protein Structures and Interactions Using a Three-Track Neural Network. Science 373, 871–876. doi:10.1126/science.abj8754
Barra, M., Danino, T., and Garrido, D. (2020). Engineered Probiotics for Detection and Treatment of Inflammatory Intestinal Diseases. Front. Bioeng. Biotechnol. 8, 265. doi:10.3389/fbioe.2020.00265
Benech, N., Rolhion, N., and Sokol, H. (2021). Tryptophan Metabolites Get the Gut Moving. Cell Host & Microbe 29, 145–147. doi:10.1016/j.chom.2021.01.009
Bhardwaj, N., Bhardwaj, S. K., Mehta, J., Kim, K.-H., and Deep, A. (2017). MOF-bacteriophage Biosensor for Highly Sensitive and Specific Detection of Staphylococcus aureus. ACS Appl. Mater. Inter. 9, 33589–33598. doi:10.1021/acsami.7b07818
Bourdeau, R. W., Lee-Gosselin, A., Lakshmanan, A., Farhadi, A., Kumar, S. R., Nety, S. P., et al. (2018). Acoustic Reporter Genes for Noninvasive Imaging of Microorganisms in Mammalian Hosts. Nature 553, 86–90. doi:10.1038/nature25021
Braendstrup, P., Levine, B. L., and Ruella, M. (2020). The Long Road to the First FDA-Approved Gene Therapy: Chimeric Antigen Receptor T Cells Targeting CD19. Cytotherapy 22, 57–69. doi:10.1016/j.jcyt.2019.12.004
Carreau, A., Hafny-Rahbi, B. E., Matejuk, A., Grillon, C., and Kieda, C. (2011). Why Is the Partial Oxygen Pressure of Human Tissues a Crucial Parameter? Small Molecules and Hypoxia. J. Cel Mol Med 15, 1239–1253. doi:10.1111/j.1582-4934.2011.01258.x
Chen, X. J., Wang, B., Thompson, I. P., and Huang, W. E. (2021). Rational Design and Characterization of Nitric Oxide Biosensors in E. coli Nissle 1917 and Mini SimCells. ACS Synth. Biol. 10, 2566–2578. doi:10.1021/acssynbio.1c00223
Chien, T., Doshi, A., and Danino, T. (2017). Advances in Bacterial Cancer Therapies Using Synthetic Biology. Curr. Opin. Syst. Biol. 5, 1–8. doi:10.1016/j.coisb.2017.05.009
Chien, T., Harimoto, T., Kepecs, B., Gray, K., Coker, C., Hou, N., et al. (2021). Enhancing the Tropism of Bacteria via Genetically Programmed Biosensors. Nat. Biomed. Eng. 29. doi:10.1038/s41551-021-00772-3
Cryan, J. F., and Dinan, T. G. (2012). Mind-altering Microorganisms: the Impact of the Gut Microbiota on Brain and Behaviour. Nat. Rev. Neurosci. 13, 701–712. doi:10.1038/nrn3346
Cubillos-Ruiz, A., Guo, T., Sokolovska, A., Miller, P. F., Collins, J. J., Lu, T. K., et al. (2021). Engineering Living Therapeutics with Synthetic Biology. Nat. Rev. Drug Discov. 20, 941–960. doi:10.1038/s41573-021-00285-3
D'Autréaux, B., Tucker, N. P., Dixon, R., and Spiro, S. (2005). A Non-haem Iron centre in the Transcription Factor NorR Senses Nitric Oxide. Nature 437, 769–772. doi:10.1038/nature03953
Daeffler, K. N. M., Galley, J. D., Sheth, R. U., Ortiz‐Velez, L. C., Bibb, C. O., Shroyer, N. F., et al. (2017). Engineering Bacterial Thiosulfate and Tetrathionate Sensors for Detecting Gut Inflammation. Mol. Syst. Biol. 13, 923. doi:10.15252/msb.20167416
Dalile, B., Van Oudenhove, L., Vervliet, B., and Verbeke, K. (2019). The Role of Short-Chain Fatty Acids in Microbiota-Gut-Brain Communication. Nat. Rev. Gastroenterol. Hepatol. 16, 461–478. doi:10.1038/s41575-019-0157-3
De Palma, G., Lynch, M. D. J., Lu, J., Dang, V. T., Deng, Y., Jury, J., et al. (2017). Transplantation of Fecal Microbiota from Patients with Irritable Bowel Syndrome Alters Gut Function and Behavior in Recipient Mice. Sci. Transl. Med. 9. doi:10.1126/scitranslmed.aaf6397
De Santis, V., and Singer, M. (2015). Tissue Oxygen Tension Monitoring of Organ Perfusion: Rationale, Methodologies, and Literature Review. Br. J. Anaesth. 115, 357–365. doi:10.1093/bja/aev162
Desbonnet, L., Garrett, L., Clarke, G., Kiely, B., Cryan, J. F., and Dinan, T. G. (2010). Effects of the Probiotic Bifidobacterium Infantis in the Maternal Separation Model of Depression. Neuroscience 170, 1179–1188. doi:10.1016/j.neuroscience.2010.08.005
Dinan, T. G., Stanton, C., and Cryan, J. F. (2013). Psychobiotics: A Novel Class of Psychotropic. Biol. Psychiatry 74, 720–726. doi:10.1016/j.biopsych.2013.05.001
Donaldson, G. P., Lee, S. M., and Mazmanian, S. K. (2016). Gut Biogeography of the Bacterial Microbiota. Nat. Rev. Microbiol. 14, 20–32. doi:10.1038/nrmicro3552
Du, Y., Wei, J., Zhang, Z., Yang, X., Wang, M., Wang, Y., et al. (2021). Plasma Metabolomics Profiling of Metabolic Pathways Affected by Major Depressive Disorder. Front. Psychiatry 12. doi:10.3389/fpsyt.2021.644555
Duvall, S. W., and Childers, W. S. (2020). Design of a Histidine Kinase FRET Sensor to Detect Complex Signal Integration within Living Bacteria. ACS Sens. 5, 1589–1596. doi:10.1021/acssensors.0c00008
Eden, E., Geva-Zatorsky, N., Issaeva, I., Cohen, A., Dekel, E., Danon, T., et al. (2011). Proteome Half-Life Dynamics in Living Human Cells. Science 331, 764–768. doi:10.1126/science.1199784
Erny, D., Hrabě de Angelis, A. L., Jaitin, D., Wieghofer, P., Staszewski, O., David, E., et al. (2015). Host Microbiota Constantly Control Maturation and Function of Microglia in the CNS. Nat. Neurosci. 18, 965–977. doi:10.1038/nn.4030
Fang, F., Lin, Y.-H., Pierce, B. D., and Lynn, D. G. (2015). ARhizobium radiobacterHistidine Kinase Can Employ Both Boolean and and OR Logic Gates to Initiate Pathogenesis. Chembiochem 16, 2183–2190. doi:10.1002/cbic.201500334
Flux, M. C., and Lowry, C. A. (2020). Finding Intestinal Fortitude: Integrating the Microbiome into a Holistic View of Depression Mechanisms, Treatment, and Resilience. Neurobiol. Dis. 135, 104578. doi:10.1016/j.nbd.2019.104578
Francis, V. I., and Porter, S. L. (2019). Multikinase Networks: Two-Component Signaling Networks Integrating Multiple Stimuli. Annu. Rev. Microbiol. 73, 199–223. doi:10.1146/annurev-micro-020518-115846
Gardner, T. S., Cantor, C. R., and Collins, J. J. (2000). Construction of a Genetic Toggle Switch in Escherichia coli. Nature 403, 339–342. doi:10.1038/35002131
Gheorghe, C. E., Martin, J. A., Manriquez, F. V., Dinan, T. G., Cryan, J. F., and Clarke, G. (2019). Focus on the Essentials: Tryptophan Metabolism and the Microbiome-Gut-Brain axis. Curr. Opin. Pharmacol. 48, 137–145. doi:10.1016/j.coph.2019.08.004
Grisanzio, K. A., Goldstein-Piekarski, A. N., Wang, M. Y., Rashed Ahmed, A. P., Samara, Z., and Williams, L. M. (2018). Transdiagnostic Symptom Clusters and Associations with Brain, Behavior, and Daily Function in Mood, Anxiety, and Trauma Disorders. JAMA Psychiatry 75, 201–209. doi:10.1001/jamapsychiatry.2017.3951
Haroon, E., Welle, J. R., Woolwine, B. J., Goldsmith, D. R., Baer, W., Patel, T., et al. (2020). Associations Among Peripheral and central Kynurenine Pathway Metabolites and Inflammation in Depression. Neuropsychopharmacol. 45, 998–1007. doi:10.1038/s41386-020-0607-1
Hensel, M., Hinsley, A. P., Nikolaus, T., Sawers, G., and Berks, B. C. (1999). The Genetic Basis of Tetrathionate Respiration in Salmonella typhimurium. Mol. Microbiol. 32, 275–287. doi:10.1046/j.1365-2958.1999.01345.x
Herud-Sikimić, O., Stiel, A. C., Kolb, M., Shanmugaratnam, S., Berendzen, K. W., Feldhaus, C., et al. (2021). A Biosensor for the Direct Visualization of Auxin. Nature 592, 768–772. doi:10.1038/s41586-021-03425-2
Ho, T. Y. H., Shao, A., Lu, Z., Savilahti, H., Menolascina, F., Wang, L., et al. (2021). A Systematic Approach to Inserting Split Inteins for Boolean Logic Gate Engineering and Basal Activity Reduction. Nat. Commun. 12, 2200. doi:10.1038/s41467-021-22404-9
Hoffman, R. M. (2016). Tumor-targeting Salmonella typhimurium A1-R: An Overview. Methods Mol. Biol. 1409, 1–8. doi:10.1007/978-1-4939-3515-4_1
Hubbard, T. D., Murray, I. A., and Perdew, G. H. (2015). Indole and Tryptophan Metabolism: Endogenous and Dietary Routes to Ah Receptor Activation. Drug Metab. Dispos. 43, 1522–1535. doi:10.1124/dmd.115.064246
Hurt, R. C., Buss, M. T., Wong, K., Sawyer, D. P., Swift, M. B., Dutka, P., et al. (2021). Genomically Mined Acoustic Reporter Genes Enable On-Demand In Vivo Monitoring of Tumor-Homing Bacteria. bioRxiv 2004, 2026.441537. doi:10.1101/2021.04.26.441537
Jaglin, M., Rhimi, M., Philippe, C., Pons, N., Bruneau, A., Goustard, B., et al. (2018). Indole, a Signaling Molecule Produced by the Gut Microbiota, Negatively Impacts Emotional Behaviors in Rats. Front. Neurosci. 12, 216. doi:10.3389/fnins.2018.00216
Kang, M., Choe, D., Kim, K., Cho, B.-K., and Cho, S. (2020). Synthetic Biology Approaches in the Development of Engineered Therapeutic Microbes. Ijms 21, 8744. doi:10.3390/ijms21228744
Kaur, H., Bose, C., and Mande, S. S. (2019). Tryptophan Metabolism by Gut Microbiome and gut-brain-axis: An In Silico Analysis. Front. Neurosci. 13. doi:10.3389/fnins.2019.01365
Kelly, J. R., Borre, Y., O' Brien, C., Patterson, E., El Aidy, S., Deane, J., et al. (2016). Transferring the Blues: Depression-Associated Gut Microbiota Induces Neurobehavioural Changes in the Rat. J. Psychiatr. Res. 82, 109–118. doi:10.1016/j.jpsychires.2016.07.019
Kelly, J. R., Keane, V. O., Cryan, J. F., Clarke, G., and Dinan, T. G. (2019). Mood and Microbes. Gastroenterol. Clin. North America 48, 389–405. doi:10.1016/j.gtc.2019.04.006
Kimura, H., Miura, S., Shigematsu, T., Ohkubo, N., Tsuzuki, Y., Kurose, I., et al. (1997). Increased Nitric Oxide Production and Inducible Nitric Oxide Synthase Activity in Colonic Mucosa of Patients with Active Ulcerative Colitis and Crohn's Disease. Dig. Dis. Sci. 42, 1047–1054. doi:10.1023/a:1018849405922
Lakshmanan, A., Farhadi, A., Nety, S. P., Lee-Gosselin, A., Bourdeau, R. W., Maresca, D., et al. (2016). Molecular Engineering of Acoustic Protein Nanostructures. ACS Nano 10, 7314–7322. doi:10.1021/acsnano.6b03364
Lakshmanan, A., Lu, G. J., Farhadi, A., Nety, S. P., Kunth, M., Lee-Gosselin, A., et al. (2017). Preparation of Biogenic Gas Vesicle Nanostructures for Use as Contrast Agents for Ultrasound and MRI. Nat. Protoc. 12, 2050–2080. doi:10.1038/nprot.2017.081
Lazar, J. T., and Tabor, J. J. (2021). Bacterial Two-Component Systems as Sensors for Synthetic Biology Applications. Curr. Opin. Syst. Biol., 28, 100398i: doi:10.1016/j.coisb.2021.100398
Lee, J.-H., Wood, T. K., and Lee, J. (2015). Roles of Indole as an Interspecies and Interkingdom Signaling Molecule. Trends Microbiol. 23, 707–718. doi:10.1016/j.tim.2015.08.001
Lenneman, B. R., Fernbach, J., Loessner, M. J., Lu, T. K., and Kilcher, S. (2021). Enhancing Phage Therapy through Synthetic Biology and Genome Engineering. Curr. Opin. Biotechnol. 68, 151–159. doi:10.1016/j.copbio.2020.11.003
Levitt, M. D., Furne, J., Springfield, J., Suarez, F., and DeMaster, E. (1999). Detoxification of Hydrogen Sulfide and Methanethiol in the Cecal Mucosa. J. Clin. Invest. 104, 1107–1114. doi:10.1172/jci7712
Liu, J.-R., Miao, H., Deng, D.-Q., Vaziri, N. D., Li, P., and Zhao, Y.-Y. (2021). Gut Microbiota-Derived Tryptophan Metabolism Mediates Renal Fibrosis by Aryl Hydrocarbon Receptor Signaling Activation. Cell. Mol. Life Sci. 78, 909–922. doi:10.1007/s00018-020-03645-1
Liu, R. T., Rowan-Nash, A. D., Sheehan, A. E., Walsh, R. F. L., Sanzari, C. M., Korry, B. J., et al. (2020). Reductions in Anti-inflammatory Gut Bacteria Are Associated with Depression in a Sample of Young Adults. Brain Behav. Immun. 88, 308–324. doi:10.1016/j.bbi.2020.03.026
Lu, T. K., and Koeris, M. S. (2011). The Next Generation of Bacteriophage Therapy. Curr. Opin. Microbiol. 14, 524–531. doi:10.1016/j.mib.2011.07.028
Mann, T. H., Seth Childers, W., Blair, J. A., Eckart, M. R., and Shapiro, L. (2016). A Cell Cycle Kinase with Tandem Sensory PAS Domains Integrates Cell Fate Cues. Nat. Commun. 7, 11454. doi:10.1038/ncomms11454
Margolis, K. G., Cryan, J. F., and Mayer, E. A. (2021). The Microbiota-Gut-Brain axis: From Motility to Mood. Gastroenterology 160, 1486–1501. doi:10.1053/j.gastro.2020.10.066
Marvin, J. S., Shimoda, Y., Magloire, V., Leite, M., Kawashima, T., Jensen, T. P., et al. (2019). A Genetically Encoded Fluorescent Sensor for In Vivo Imaging of GABA. Nat. Methods 16, 763–770. doi:10.1038/s41592-019-0471-2
Mayer, E. A. (2011). Gut Feelings: the Emerging Biology of Gut-Brain Communication. Nat. Rev. Neurosci. 12, 453–466. doi:10.1038/nrn3071
Mayer, E. A., Knight, R., Mazmanian, S. K., Cryan, J. F., and Tillisch, K. (2014). Gut Microbes and the Brain: Paradigm Shift in Neuroscience. J. Neurosci. 34, 15490–15496. doi:10.1523/JNEUROSCI.3299-14.2014
McNerney, M. P., Doiron, K. E., Ng, T. L., Chang, T. Z., and Silver, P. A. (2021). Theranostic Cells: Emerging Clinical Applications of Synthetic Biology. Nat. Rev. Genet. 22, 730–746. doi:10.1038/s41576-021-00383-3
Miller, A. H., and Raison, C. L. (2016). The Role of Inflammation in Depression: from Evolutionary Imperative to Modern Treatment Target. Nat. Rev. Immunol. 16, 22–34. doi:10.1038/nri.2015.5
Mimee, M., Nadeau, P., Hayward, A., Carim, S., Flanagan, S., Jerger, L., et al. (2018). An Ingestible Bacterial-Electronic System to Monitor Gastrointestinal Health. Science 360, 915–918. doi:10.1126/science.aas9315
Mir, H.-D., Milman, A., Monnoye, M., Douard, V., Philippe, C., Aubert, A., et al. (2020). The Gut Microbiota Metabolite Indole Increases Emotional Responses and Adrenal Medulla Activity in Chronically Stressed Male Mice. Psychoneuroendocrinology 119, 104750. doi:10.1016/j.psyneuen.2020.104750
Modoux, M., Rolhion, N., Mani, S., and Sokol, H. (2021). Tryptophan Metabolism as a Pharmacological Target. Trends Pharmacol. Sci. 42, 60–73. doi:10.1016/j.tips.2020.11.006
Möglich, A., Ayers, R. A., and Moffat, K. (2010). Addition at the Molecular Level: Signal Integration in Designed Per-ARNT-Sim Receptor Proteins. J. Mol. Biol. 400, 477–486. doi:10.1016/j.jmb.2010.05.019
Morais, L. H., Schreiber, H. L., and Mazmanian, S. K. (2021). The Gut Microbiota-Brain axis in Behaviour and Brain Disorders. Nat. Rev. Microbiol. 19, 241–255. doi:10.1038/s41579-020-00460-0
Pereira, F. C., and Berry, D. (2017). Microbial Nutrient Niches in the Gut. Environ. Microbiol. 19, 1366–1378. doi:10.1111/1462-2920.13659
Pfeifer, F. (2012). Distribution, Formation and Regulation of Gas Vesicles. Nat. Rev. Microbiol. 10, 705–715. doi:10.1038/nrmicro2834
Pinto-Sanchez, M. I., Hall, G. B., Ghajar, K., Nardelli, A., Bolino, C., Lau, J. T., et al. (2017). Probiotic Bifidobacterium Longum NCC3001 Reduces Depression Scores and Alters Brain Activity: A Pilot Study in Patients with Irritable Bowel Syndrome. Gastroenterology 153, 448–459. e448. doi:10.1053/j.gastro.2017.05.003
Plana-Ripoll, O., Pedersen, C. B., Holtz, Y., Benros, M. E., Dalsgaard, S., de Jonge, P., et al. (2019). Exploring Comorbidity within Mental Disorders Among a Danish National Population. JAMA Psychiatry 76, 259–270. doi:10.1001/jamapsychiatry.2018.3658
Pokusaeva, K., Johnson, C., Luk, B., Uribe, G., Fu, Y., Oezguen, N., et al. (2017). GABA-producingBifidobacterium Dentiummodulates Visceral Sensitivity in the Intestine. Neurogastroenterol. Motil. 29, e12904. doi:10.1111/nmo.12904
Pu, J., Liu, Y., Zhang, H., Tian, L., Gui, S., Yu, Y., et al. (2021). An Integrated Meta-Analysis of Peripheral Blood Metabolites and Biological Functions in Major Depressive Disorder. Mol. Psychiatry 26, 4265–4276. doi:10.1038/s41380-020-0645-4
Riglar, D. T., Giessen, T. W., Baym, M., Kerns, S. J., Niederhuber, M. J., Bronson, R. T., et al. (2017). Engineered Bacteria Can Function in the Mammalian Gut Long-Term as Live Diagnostics of Inflammation. Nat. Biotechnol. 35, 653–658. doi:10.1038/nbt.3879
Ronald, A., Simonoff, E., Kuntsi, J., Asherson, P., and Plomin, R. (2008). Evidence for Overlapping Genetic Influences on Autistic and ADHD Behaviours in a Community Twin Sample. J. Child. Psychol. Psychiat 49, 535–542. doi:10.1111/j.1469-7610.2007.01857.x
Rothhammer, V., Mascanfroni, I. D., Bunse, L., Takenaka, M. C., Kenison, J. E., Mayo, L., et al. (2016). Type I Interferons and Microbial Metabolites of Tryptophan Modulate Astrocyte Activity and central Nervous System Inflammation via the Aryl Hydrocarbon Receptor. Nat. Med. 22, 586–597. doi:10.1038/nm.4106
Rudzki, L., and Szulc, A. (2018). "Immune Gate" of Psychopathology-The Role of Gut Derived Immune Activation in Major Psychiatric Disorders. Front. Psychiatry 9. doi:10.3389/fpsyt.2018.00205
Rutsch, A., Kantsjö, J. B., and Ronchi, F. (2020). The Gut-Brain axis: How Microbiota and Host Inflammasome Influence Brain Physiology and Pathology. Front. Immunol. 11. doi:10.3389/fimmu.2020.604179
Sarkar, A., Lehto, S. M., Harty, S., Dinan, T. G., Cryan, J. F., and Burnet, P. W. J. (2016). Psychobiotics and the Manipulation of Bacteria-Gut-Brain Signals. Trends Neurosciences 39, 763–781. doi:10.1016/j.tins.2016.09.002
Schwanhäusser, B., Busse, D., Li, N., Dittmar, G., Schuchhardt, J., Wolf, J., et al. (2011). Global Quantification of Mammalian Gene Expression Control. Nature 473, 337–342. doi:10.1038/nature10098
Shapiro, M. G., Goodwill, P. W., Neogy, A., Yin, M., Foster, F. S., Schaffer, D. V., et al. (2014). Biogenic Gas Nanostructures as Ultrasonic Molecular Reporters. Nat. Nanotech 9, 311–316. doi:10.1038/nnano.2014.32
Sharma, J. N., Al-Omran, A., and Parvathy, S. S. (2007). Role of Nitric Oxide in Inflammatory Diseases. Inflammopharmacol 15, 252–259. doi:10.1007/s10787-007-0013-x
Singhal, R., and Shah, Y. M. (2020). Oxygen Battle in the Gut: Hypoxia and Hypoxia-Inducible Factors in Metabolic and Inflammatory Responses in the Intestine. J. Biol. Chem. 295, 10493–10505. doi:10.1074/jbc.REV120.011188
Soliman, M. L., and Rosenberger, T. A. (2011). Acetate Supplementation Increases Brain Histone Acetylation and Inhibits Histone Deacetylase Activity and Expression. Mol. Cel Biochem. 352, 173–180. doi:10.1007/s11010-011-0751-3
Song, Y., Rampley, C. P. N., Chen, X., Du, F., Thompson, I. P., and Huang, W. E. (2019). “Application of Bacterial Whole-Cell Biosensors in Health,” in Handbook of Cell Biosensors. Editor G. Thouand (Springer International Publishing), 1–17. doi:10.1007/978-3-319-47405-2_136-1
Spiro, S. (2007). Regulators of Bacterial Responses to Nitric Oxide. FEMS Microbiol. Rev. 31, 193–211. doi:10.1111/j.1574-6976.2006.00061.x
Stern, A. M., Hay, A. J., Liu, Z., Desland, F. A., Zhang, J., Zhong, Z., et al. (2012). The NorR Regulon Is Critical for Vibrio cholerae Resistance to Nitric Oxide and Sustained Colonization of the Intestines. MBio 3, e00013–12. doi:10.1128/mBio.00013-12
Stern, A. M., and Zhu, J. (2014). “An Introduction to Nitric Oxide Sensing and Response in Bacteria,” in Advances in Applied Microbiology. Editors S. Sariaslani, and G. M. Gadd (Academic Press), 187–220. doi:10.1016/B978-0-12-800261-2.00005-0
Strandwitz, P., Kim, K. H., Terekhova, D., Liu, J. K., Sharma, A., Levering, J., et al. (2019). GABA-modulating Bacteria of the Human Gut Microbiota. Nat. Microbiol. 4, 396–403. doi:10.1038/s41564-018-0307-3
Sulakvelidze, A., Alavidze, Z., and Morris, J. G. (2001). Bacteriophage Therapy. Antimicrob. Agents Chemother. 45, 649–659. doi:10.1128/aac.45.3.649-659.2001
Summers, W. C. (2001). Bacteriophage Therapy. Annu. Rev. Microbiol. 55, 437–451. doi:10.1146/annurev.micro.55.1.437
Tropini, C., Earle, K. A., Huang, K. C., and Sonnenburg, J. L. (2017). The Gut Microbiome: Connecting Spatial Organization to Function. Cell Host & Microbe 21, 433–442. doi:10.1016/j.chom.2017.03.010
Tunyasuvunakool, K., Adler, J., Wu, Z., Green, T., Zielinski, M., Žídek, A., et al. (2021). Highly Accurate Protein Structure Prediction for the Human Proteome. Nature 596, 590–596. doi:10.1038/s41586-021-03828-1
Varatharaj, A., and Galea, I. (2017). The Blood-Brain Barrier in Systemic Inflammation. Brain Behav. Immun. 60, 1–12. doi:10.1016/j.bbi.2016.03.010
Vishweshwaraiah, Y. L., Chen, J., Chirasani, V. R., Tabdanov, E. D., and Dokholyan, N. V. (2021). Two-input Protein Logic Gate for Computation in Living Cells. Nat. Commun. 12, 6615. doi:10.1038/s41467-021-26937-x
Waldecker, M., Kautenburger, T., Daumann, H., Busch, C., and Schrenk, D. (2008). Inhibition of Histone-Deacetylase Activity by Short-Chain Fatty Acids and Some Polyphenol Metabolites Formed in the colon. J. Nutr. Biochem. 19, 587–593. doi:10.1016/j.jnutbio.2007.08.002
Wang, J., Zhang, C., and Childers, W. S. (2021). A Biosensor for Detection of Indole Metabolites. ACS Synth. Biol. 10, 1605–1614. doi:10.1021/acssynbio.1c00090
Wei, G. Z., Martin, K. A., Xing, P. Y., Agrawal, R., Whiley, L., Wood, T. K., et al. (2021). Tryptophan-metabolizing Gut Microbes Regulate Adult Neurogenesis via the Aryl Hydrocarbon Receptor. Proc. Natl. Acad. Sci. USA 118, e2021091118. doi:10.1073/pnas.2021091118
Williams, J. Z., Allen, G. M., Shah, D., Sterin, I. S., Kim, K. H., Garcia, V. P., et al. (2020). Precise T Cell Recognition Programs Designed by Transcriptionally Linking Multiple Receptors. Science 370, 1099–1104. doi:10.1126/science.abc6270
Winter, S. E., Thiennimitr, P., Winter, M. G., Butler, B. P., Huseby, D. L., Crawford, R. W., et al. (2010). Gut Inflammation Provides a Respiratory Electron Acceptor for Salmonella. Nature 467, 426–429. doi:10.1038/nature09415
Yang, K. K., Wu, Z., and Arnold, F. H. (2019). Machine-learning-guided Directed Evolution for Protein Engineering. Nat. Methods 16, 687–694. doi:10.1038/s41592-019-0496-6
Yang, L., Nielsen, A. A. K., Fernandez-Rodriguez, J., McClune, C. J., Laub, M. T., Lu, T. K., et al. (2014). Permanent Genetic Memory with >1-byte Capacity. Nat. Methods 11, 1261–1266. doi:10.1038/nmeth.3147
Yehl, K., Lemire, S., Yang, A. C., Ando, H., Mimee, M., Torres, M. D. T., et al. (2019). Engineering Phage Host-Range and Suppressing Bacterial Resistance through Phage Tail Fiber Mutagenesis. Cell 179, 459–469. e459. doi:10.1016/j.cell.2019.09.015
Zheng, P., Zeng, B., Zhou, C., Liu, M., Fang, Z., Xu, X., et al. (2016). Gut microbiome remodeling induces depressive-like behaviors through a pathway mediated by the host’s metabolism. Mol. Psychiatry 21, 786–796.
Keywords: depression, gut-brain axis, biosensors, metabolites, synthetic biology
Citation: Wang J and Childers WS (2022) The Future Potential of Biosensors to Investigate the Gut-Brain Axis. Front. Bioeng. Biotechnol. 9:826479. doi: 10.3389/fbioe.2021.826479
Received: 30 November 2021; Accepted: 28 December 2021;
Published: 14 January 2022.
Edited by:
Bernardo Franco, University of Guanajuato, MexicoReviewed by:
Kiran Veer Sandhu, University College Cork, IrelandCopyright © 2022 Wang and Childers. This is an open-access article distributed under the terms of the Creative Commons Attribution License (CC BY). The use, distribution or reproduction in other forums is permitted, provided the original author(s) and the copyright owner(s) are credited and that the original publication in this journal is cited, in accordance with accepted academic practice. No use, distribution or reproduction is permitted which does not comply with these terms.
*Correspondence: W. Seth Childers, d3NjaGlsZEBwaXR0LmVkdQ==
Disclaimer: All claims expressed in this article are solely those of the authors and do not necessarily represent those of their affiliated organizations, or those of the publisher, the editors and the reviewers. Any product that may be evaluated in this article or claim that may be made by its manufacturer is not guaranteed or endorsed by the publisher.
Research integrity at Frontiers
Learn more about the work of our research integrity team to safeguard the quality of each article we publish.