- 1Laboratory of Movement Analysis and Measurement, EPFL, Lausanne, Switzerland
- 2Institute of Sport Sciences, University of Lausanne, Lausanne, Switzerland
- 3Swiss Olympic Medical Center, Sport Medicine Unit, Division of Physical Medicine and Rehabilitation, Lausanne University Hospital, Lausanne, Switzerland
- 4Gait Up S.A., Lausanne, Switzerland
- 5Huma Therapeutics Ltd., London, United Kingdom
Comprehensive monitoring of performance is essential for swimmers and swimming coaches to optimize the training. Regardless of the swimming technique, the swimmer passes various swimming phases from wall to wall, including a dive into the water or wall push-off, then glide and strokes preparation and finally, swimming up to the turn. The coach focuses on improving the performance of the swimmer in each of these phases. The purpose of this study was to assess the potential of using a sacrum-worn inertial measurement unit (IMU) for performance evaluation in each swimming phase (wall push-off, glide, stroke preparation and swimming) of elite swimmers in four main swimming techniques (i.e. front crawl, breaststroke, butterfly and backstroke). Nineteen swimmers were asked to wear a sacrum IMU and swim four one-way 25 m trials in each technique, attached to a tethered speedometer and filmed by cameras in the whole lap as reference systems. Based on the literature, several goal metrics were extracted from the instantaneous velocity (e.g. average velocity per stroke cycle) and displacement (e.g. time to reach 15 m from the wall) data from a tethered speedometer for the swimming phases, each one representing the goodness of swimmer’s performance. Following a novel approach, that starts from swimming bout detection and continues until detecting the swimming phases, the IMU kinematic variables in each swimming phase were extracted. The highly associated variables with the corresponding goal metrics were detected by LASSO (least absolute shrinkage and selection operator) variable selection and used for estimating the goal metrics with a linear regression model. The selected kinematic variables were relevant to the motion characteristics of each phase (e.g. selection of propulsion-related variables in wall push-off phase), providing more interpretability to the model. The estimation reached a determination coefficient (R2) value more than 0.75 and a relative RMSE less than 10% for most goal metrics in all swimming techniques. The results show that a single sacrum IMU can provide a wide range of performance-related swimming kinematic variables, useful for performance evaluation in four main swimming techniques.
Introduction
Swimming coaches seek comprehensive monitoring of performance to develop and refine a competition model for their top athletes. During a competition, the swimmer goes through several swimming phases from wall to wall, including a dive into the water or wall push-off, then glide and strokes preparation and finally swimming up to the turn at the end of the lap and repeating the same sequence in the next lap. Therefore, to have a comprehensive performance evaluation, studies have focused on various swimming phases, since the swimmers aim to master all of them (Mooney et al., 2016). As the principal goal of a swimmer is to reduce the swimming time by increasing the velocity, performance evaluation goal metrics in different phases are based on time records and velocity. Flight distance (Ruschel et al., 2007), time to 15 m (Vantorre et al., 2010), average velocity per stroke (Dadashi et al., 2015), swimming phase average velocity (Mason and Cossor, 2000), turn time (5 m before to 10 m after the wall) (Mooney et al., 2016) or lap time are examples of common goal metrics.
Recently, wearable IMUs (inertial measurement unit) have been used more for swimming motion analysis in all competitive swimming techniques (Guignard et al., 2017b), because of the challenges of video-based systems application in aquatic environments (Callaway et al., 2010). They are used in a multitude of studies for variable extraction in various swimming phases, such as start (Vantorre et al., 2014), swimming (Davey et al., 2008), and turn (Slawson et al., 2012). Novel orientation analysis algorithms made it possible to estimate the 3-dimensional orientation of IMU with high accuracy by fusing accelerometer, gyroscope and magnetometer data (Madgwick et al., 2011). This approach is implemented in swimming for inter-segmental coordination assessment (Guignard et al., 2017a), posture recognition (Wang et al., 2019) and intra-stroke velocity (Worsey et al., 2018). In another study, a new analysis approach is proposed and trunk elevation, body balance, and body rotation are used as new indices for swimming analysis (Félix et al., 2019; Morouço et al., 2020). Considering the significance of phase related kinematic variables, we have recently proposed a macro-micro approach for swimming analysis using IMUs (Hamidi Rad et al., 2021). In our approach, swimming bouts, laps and swimming technique are detected in macro analysis. Afterwards in micro level, each lap is segmented into swimming phases of wall push-off (Push), glide (Glid), strokes preparation (StPr), swimming (Swim) and turn (Turn) from wall to wall. In the next level of micro analysis, the kinematic variables within each swimming phase (micro variables) are extracted from IMU data.
These studies show there is still a substantial undiscovered potential for kinematic variable extraction with IMUs in swimming analysis. However, the association between the swimming kinematic variables extracted by IMU and the above-mentioned goal metrics is still unclear. Furthermore, as the variables provided by the IMU are claimed to be associated with the swimmers’ performance, they can be used for estimating the goal metrics of performance evaluation. As a result, the relationship between IMU kinematic variables and goal metrics is yet to be studied to prove IMU potential not only for swimming kinematic variable extraction, but also for performance evaluation and training optimization.
The main objective of this study is to find the association between swimming kinematics extracted using a sacrum-worn IMU and goal metrics in different swimming phases. We hypothesized that the micro variables extracted from IMU data are associated with the goal metrics used for performance evaluation, regardless of the swimming technique. Following the macro-micro approach for swimming analysis (Hamidi Rad et al., 2021), within each swimming phase (Push, Glid, StPr and Swim), we selected the kinematic variables that are highly associated with goal metrics. We then used the selected kinematics to estimate the goal metrics. Using the underlying model we can explains how kinematics determine the performance.
Materials and Methods
Measurement Setup and Protocol
Nineteen elite swimmers took part in this study, whose attributes are shown in Table 1. They were informed of the procedure and gave their written consent prior to participation. This study was approved by the EPFL human research ethics committee (HREC, No: 050/2018). One IMU (Physilog® IV, GaitUp, CH.) was attached to swimmer’s sacrum, using waterproof band (Tegaderm, 3M Co., USA). The sensor contained a 3D gyroscope (±2000 °/s) and 3D accelerometer (±16 g), with a sampling rate of 500 Hz (Figure 1). A functional calibration was performed after sensor installation with simple movements in land (upright standing and squats) before the measurement to make the data independent of sensor placement on swimmer’s body (Dadashi et al., 2013). During the measurements, the swimmers were asked to perform four one-way trials in each swimming technique (i.e. front crawl, breaststroke, butterfly, backstroke) with a progressive velocity (70–100%) in a 25 m indoor pool, starting with wall push-off inside water. The trials were separated with 1-min rests, and the total duration of the measurement was around 1 hour per swimmer.

TABLE 1. Statistics of the study participants. All variables are presented as mean ± standard deviation.
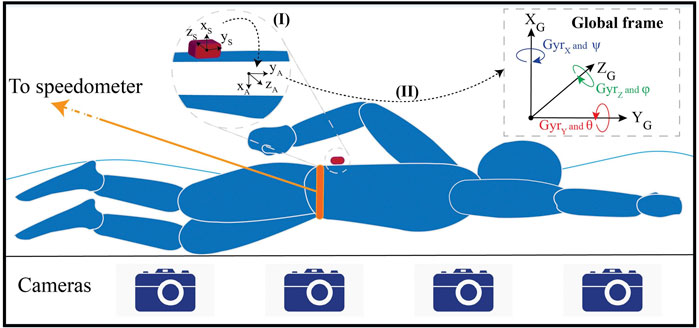
FIGURE 1. Measurement setup including one IMU attached to the sacrum, four cameras to capture the whole lap and tethered speedometer to record swimmer’s displacement and velocity. IMU data is transferred from sensor frame (x,y,z)S, first to anatomical frame (x,y,z)A using functional calibration (I), and then to the global frame (X,Y,Z)G using the gradient-descend based optimization algorithm (II). The global axes of acceleration, angular velocity and angles are displayed in the figure.
Two systems were used as references in our study to validate the goal metrics estimated by the IMU. The first one was a set of four 2-D cameras (GoPro Hero 7 Black, GoPro Inc., US) used for detecting the swimming phases. The cameras synchronized with the IMU, using the LED light of a push-button (Hamidi Rad et al., 2021) were attached to the pool wall (distributed along the length of the pool) to videotape all the lap from wall to wall underwater with a 60 Hz rate (Figure 1). The second reference system was a tethered speedometer (SpeedRT®, ApLab, Rome, Italy), attached with a belt to the waist of the swimmer. The speedometer calculated the displacement and velocity of the swimmer at a rate of 100 Hz and was used for finding the reference values of goal metrics in different swimming phases. As the speedometer was installed on the starting block above the swimmer’s level, it caused a parallax problem (Le Sage et al., 2011). Since the device level difference with respect to the still pool water was known (62 ± 1 cm), the velocity projection along the swimming direction was separated as the forward velocity of the swimmer.
Performance Evaluation
The general flowchart for performance evaluation is outlined in Figure 2. The algorithm includes three parts: 1) IMU data preparation 2) phase detection and phase-based micro variables extraction, 3) kinematic variable selection and goal metrics estimation. IMU data preparation aims to transfer the data to the global frame to achieve the true motion data of swimmer’s sacrum. Then we divided each lap into four phases of Push, Glid, StPr and Swim by camera or IMU (Hamidi Rad et al., 2021). In order to observe the error induced by IMU-based phase detection, the rest of the analysis was done once with swimming phases detected by cameras and once by the IMU for comparison, the results of which are illustrated in supplementary materials. Using the data in global frame (acceleration (
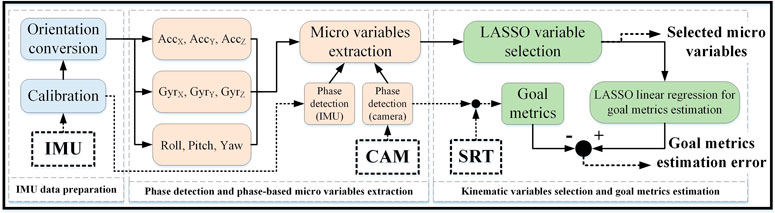
FIGURE 2. Flowchart of the performance evaluation algorithm. IMU data preparation including IMU calibration and expressing data in the global frame (left), phase detection by cameras (CAM) or IMU calibrated data and micro variable extraction from IMU data in global frame (middle) and variable selection from micro variables and the goal metrics estimation (right). The actual goal metrics are defined and extracted from the velocity and displacement data by tethered speedometer (SRT) during swimming phases separated by the cameras (CAM).
In the third part of this approach, we used the extracted phase-based micro variables to estimate the goal metrics. First, LASSO (least absolute shrinkage and selection operator) variable selection is used to rank and select the micro variables with higher importance (Fonti and Belitser, 2017). Using the speedometer and camera data, several goal metrics are extracted on the velocity and displacement of the swimmer in different swimming phases. These goal metrics are representatives of how well the swimmer performed in the corresponding phase. Finally, we used the selected micro variables to estimate the goal metrics. The principal outputs of this analysis are the selected variables and the error of using them for goal metrics estimation.
IMU Data Preparation
First, the data was calibrated for offset, scale and non-orthogonality (Ferraris et al., 1995). As explained in Measurement Setup and Protocol, a functional calibration is also performed before each measurement trial. The goal of this calibration is to transform the data from sensor frame
Where
Phase-Based Micro Variables
For IMU-based detection of swimming phases, we used a macro-micro approach in our previous study, started from swimming bouts detection down to lap segmentation into swimming phases (Hamidi Rad et al., 2021). Using the acceleration, angular velocity and orientation data in global frame, various kinematic variables based on motion biomechanics in every swimming phase are defined. As frequently discussed in the literature, fast swimming depends on 1) the ability to generate high propulsive forces, 2) the ability to keep the correct posture for reducing the drag, while 3) swimming with the highest efficiency (Toussaint and Truijens, 2005). Therefore, knowledge of the propulsion, posture and efficiency is relevant to optimize swimming performance. We related the extracted micro variables to one of these three categories (Table 2). We also added a fourth group for the variables related to the durations and rates of motion, which did not fit into the previous three categories. For example stroke rate in Swim phase which is not necessarily a sign of high or low propulsion, good or bad posture and high or low efficiency but it is widely used for performance evaluation (Siirtola et al., 2011; Beanland et al., 2014).
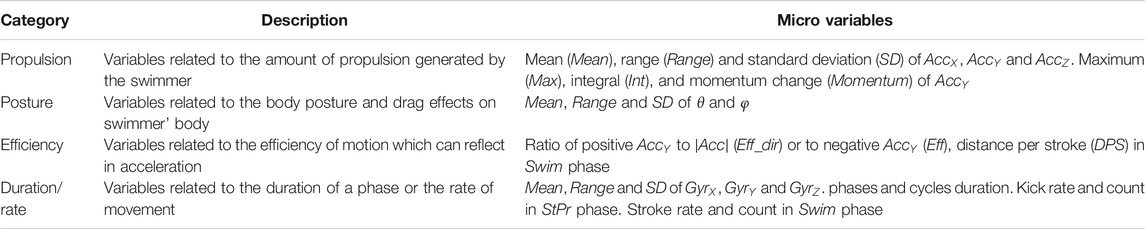
TABLE 2. Categories and description of the phase-based micro variable defined on IMU data in global frame. The name of the functions used for micro variables extraction are abbreviated in parentheses.
We extracted the micro variables by extremum detection, integration or calculation of the average, range and standard deviation of the signal. The variables defined per stroke in Swim phase need a cycle separation algorithm. For front crawl and backstroke, the duration between the two successive positive peaks on the longitudinal angular velocity in anatomical frame (
Goal Metrics
We extracted eight goal metrics from the tethered speedometer data i.e. the velocity and displacement of the swimmer, from wall to wall within the swimming phases detected on the cameras (Figure 3).
1. Push maximum velocity: the highest velocity during the lap is generated at start, as the swimmer can reach a velocity much greater than other swimming phases (Shimadzu et al., 2008). During Push phase, the maximum velocity reached is used to assess wall push-off (Stamm et al., 2013). We use this value as the goal metric for Push phase.
2. Glid end velocity: the velocity decreases during Glid phase because of water drag. The swimmer should keep the streamlined horizontal posture and start StPr phase at the right time before losing too much velocity (Vantorre et al., 2014). So we considered the velocity of the swimmer at the end of Glid phase as the goal metric for this phase.
3. StPr average velocity: the average velocity of the swimmer during StPr lower limbs actions is shown to have a negative correlation with 15-m time of the swimmer (Cossor and Mason, 2001). We used it as the goal metric for StPr phase.
During Swim phase, the performance of the swimmer can be studied per cycle or in the whole phase. Thus two goal metrics are defined in this phase:
4. Swim—average velocity per cycle: the average velocity of the swimmer per cycle provides valuable information of swimmer’s performance in every cycle (Dadashi et al., 2015).
5. Swim—average velocity of Swim phase: for looking at all the cycles together, the average velocity of the whole Swim phase is used as the second goal metric for this phase.
We also used three more goal metrics based on the literature, which include more than one phase.
6.
7.
8. Lap average velocity: considering all the phases together, average velocity of the lap (determined by lap time) is the final goal metric, displaying the overall performance of the swimmer in all phases (Davey et al., 2008; Mooney et al., 2016).
Among the defined goal metrics, Push maximum velocity is calculated with a peak detection algorithm in Push phase. The rest of the goal metrics only rely on the beginning or end of swimming phases, which are already obtained by phase detection.
Association Between Micro Variables and Goal Metrics
After extracting the micro variables from IMU and goal metrics from speedometer and camera data, we look for association between every goal metric with the micro variables of its corresponding phase or phases. For example, Push maximum velocity is associated with Push phase micro variables. For goal metrics involving more than one phase, such as
To identify the variables with higher significance, we ran a variable selection algorithm. In the first step, we normalized each variable and removed the multicollinearity between them using variance inflation factors (VIF) (Mansfield and Helms, 1982). LASSO variable selection is then applied over the variables related to each goal metric, to select the ones of higher importance. LASSO is a forward-looking variable selectin method for regression, which improves both the estimation accuracy and the interpretability of the model (Muthukrishnan and Rohini, 2017). It ranks the variables and allocates a wight to each one based on their significance in the regression model. Among the selected variables, we neglected the ones with a relative weight less than 5% because of their less important role. Moreover, to quantify the contribution of each category to the regression model, we summed the relative weights of variables from each category (propulsion, posture, efficiency and duration/rate).
Once the significant variables were identified, we utilized them to estimate the goal metrics by a LASSO regression model with leave-one-out cross-validation to avoid overfitting (Berrar, 2018). The cross validated determination coefficient (
Results
A sample size analysis based on a previous study (Dadashi et al., 2012) that used the same speedometer and measurement protocol for velocity estimation is performed. Considering a power of 80% (β = 0.2) and 95% (α = 0.05) confidence interval, we reached a sample size of 64 for this study. Since the models are generated using the data from all swimmers pooled together, the number of observations used to estimate all goal metrics, except for average velocity of the cycle in Swim phase was 76 samples. The overall number of cycles used for estimating the average velocity per cycle in Swim phase was 1,166, 627, 695 and 1,052 for front crawl, breaststroke, butterfly and backstroke respectively.
Goal Metrics Estimation
The cross-validated values (R2, RMSE and the relative RMSE in percent) of LASSO regression model used for estimating the corresponding goal metric are reported in Table 3 for each goal metric. Table 3 shows that LASSO regression model fits the data with an R2 value more than 0.75 for most goal metrics in all swimming techniques. The RMSE of the regression are less than 0.15
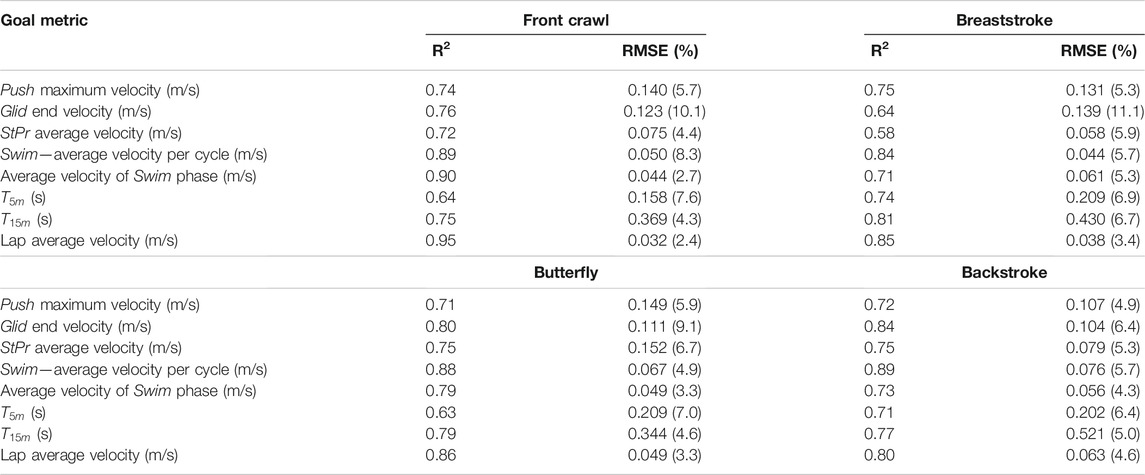
TABLE 3. The results of evaluating LASSO regression for goal metrics estimation. The determination coefficient (R2) and root mean square of error (RMSE) and the relative RMSE (in %) of regression are reported for each swimming technique.
Micro-Variables Selection
The selected variables for each goal metric estimation during front crawl technique are listed in Table 4. Same tables for other swimming techniques are brought in supplementary materials (Supplementary Tables A2–A4). Among acceleration axes,
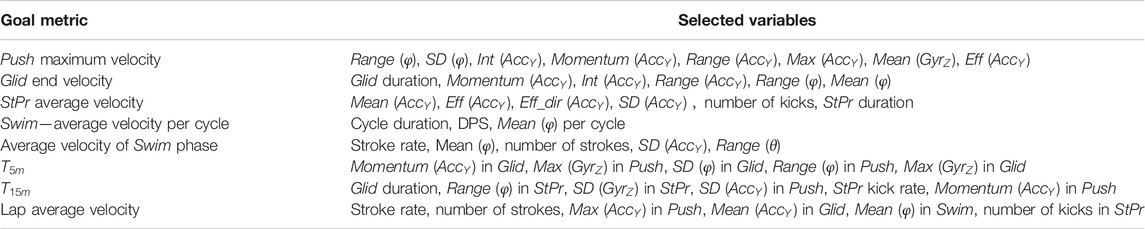
TABLE 4. The selected variables for estimating each goal metric for front crawl technique, written in the order of relative weights. The variables are written in the order of their relative weights. For the abbreviated name of functions, see Table 2.
The overall contribution of each category in estimating the goal metrics is illustrated in Figure 4 for all four swimming techniques. It is observable that propulsion category plays an important role in Push phase, while posture-related variables are more selected in Glid phase. StPr phase is less affected by efficiency compared to other categories. Efficiency and propulsion categories are both significant in determining the average velocity per cycle in Swim phase. Duration/rate category is dominant in estimating average velocity of Swim phase and lap average velocity.
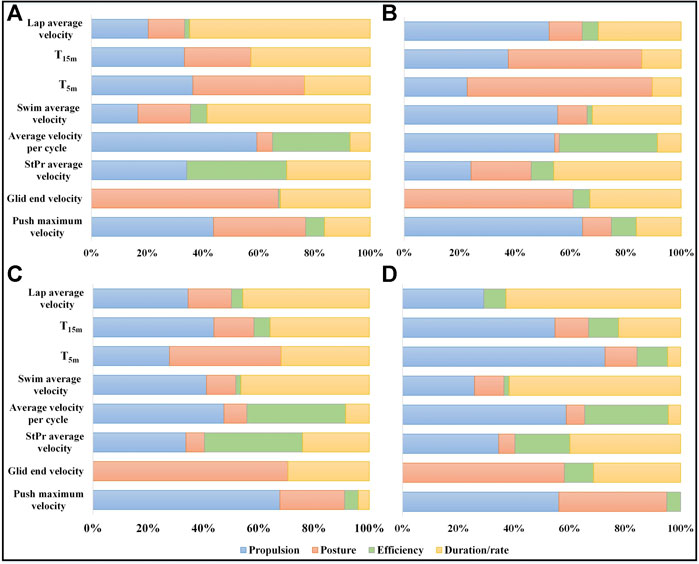
FIGURE 4. Variable categories contribution to goal metrics estimation for front crawl (A), breaststroke (B), butterfly (C) and backstroke (D). The contribution of each category (propulsion: blue, posture: orange, efficiency: green, duration/rate: yellow) is represented in percent for estimating the corresponding goal metric. The results are based on the variables with higher than 5% relative weight in LASSO variable selection.
Discussion
In this research, we studied the association between IMU micro variables and the performance evaluation goal metrics found by camera and speedometer during the swimming phases from wall to wall in four main swimming techniques. The obtained results confirmed our hypothesis that micro variables extracted from a single IMU placed at sacrum within each phase are associated with the corresponding goal metrics used generally for performance evaluation. As a result, using a single IMU would be enough for performance evaluation in main swimming techniques. Micro variables, showing strong association with the goal metrics, were identified thanks to LASSO variable selection and used for predicting the goal metrics.
Goal Metrics Estimation
The selected kinematic variables within each swimming phase were used for estimating the corresponding goal metrics (Table 3). Estimating the Push maximum velocity and Glid end velocity showed similar results in different swimming techniques, as the two initial phases are the same for them (only for backstroke, the swimmer has a supine posture). The relative RMSE is the highest for Glid end velocity estimation (11%) because this goal metric has the lowest value in the whole lap. In the StPr phase, the average velocity shows a high amount of variability among the swimmers, and determination coefficient (i.e. the proportion of the variance of the true goal metric value explained by the regression model) is relatively lower for it (less than 0.8 in all techniques) compared to other goal metrics in all techniques, because a linear model is not efficient enough in reflecting the variation of this goal metric, and a non-linear model might estimate it better.
Average velocity per cycle is estimated in all techniques with a determination coefficient more than 0.84 and an RMSE less than 0.076 m/s and relative error less than 6%. However, estimating the average velocity of the whole Swim phase achieved poorer results (R2 of 0.71–0.90 in different techniques). As estimating each cycle average velocity is more accurate in all techniques, the average value of all cycles in Swim phase can also be used for estimating Swim phase average velocity. The regression models for estimating
Micro Variables Selection
As shown in Table 4 and Figure 4 during the Push phase, the kinematic variables related to
StPr phase has the highest amount of velocity variation on speedometer data and the average velocity during this phase is related to a combination of forward acceleration, accelerating efficiency, number of kicks and phase duration. Two types of efficiency-related variables are selected for this phase. Eff (
For Swim phase goal metrics, the average velocity per stroke is mainly associated with the duration of each cycle and the DPS. The Mean (
With an overall observation on Figure 4, it is noted that the dominant categories in swimming phases are in line with the swimming phase biomechanics. Push phase asks for high propulsion, and Glid phase is more about keeping the right posture to avoid the drag. StPr phase is a combination of propulsion, posture and efficiency. Since the variable selection algorithm chooses the best variables for goal metric estimation, the variables which have the strongest relationship with the goal metrics are selected. As a result, we cannot assert that the rest of the variables are of no importance in swimming. For example, the DPS and cycle duration were dominant in estimating the average velocity per cycle in Swim phase, while no one can ignore the importance of orientation-related variables (e.g.
This study shows that a single sacrum IMU can provide kinematic variables relevant to the performance of the swimmer, in different techniques and phases for performance evaluation without using complex instrumentation such as speedometers or cameras. This offers new tools for training, where for example output of the IMU can be transferred to a mobile application for coaches and swimmers to easily follow the progress of the swimmers. Although using wearables induces more drag on swimmer body (Magalhaes et al., 2015), it needs extremely less effort than cameras for preparation and use, and it overcomes many of the limits of video-based systems (Callaway et al., 2010). The kinematic variables that were found dominant in our study were already analyzed using IMU of video-based methods but their relationship with the goal metrics were not studied. Swimmer’s posture during Push and Glid (Pereira et al., 2015), Glid duration (Guimaraes and Hay, 1985), StPr kicking rate (Shimojo et al., 2014), Swim stroke rate (Beanland et al., 2014) or DPS (Bächlin et al., 2008) are examples of the micro variables that were found relevant to performance, and we also found them significant in this study.
Both male and female swimmers were included for generating the results of this study to have a larger, more variant dataset. Comparing the swimmers due to their individual differences is out of the scope of our study. The estimations are done over all swimming velocities so the results are valid for 70–100 percent of swimmers’ paces. The synchronization error between the three systems of IMU, cameras and speedometer is a source of error in this study. Since tethered speedometer was used as reference in this study, the measurements were done over one-way trials without turn and turn motion is not evaluated. In this study, we used linear regression to have interpretable models highlighting the main variables correlated to the goal metrics. More complex non-linear models could be used if the goal is more accurate prediction of goal metrics.
Conclusion
Using the IMU data, we extracted numerous kinematic variables related to propulsion, posture, efficiency and duration/rate of motion in four main swimming phases, associated with the goal metrics defined over velocity and time of swimming in each swimming phase. These kinematic variables were biomechanically interpretable and were able to predict the goal metrics using LASSO linear regression. The generated models fit the data with an R2 value more than 0.75 for most goal metrics. The RMSE of the regression were less than 0.15
Data Availability Statement
The raw data supporting the conclusion of this article will be made available to qualified researcher, without undue reservation.
Ethics Statement
The studies involving human participants were reviewed and approved by the EPFL human research ethics committee (HREC, No: 050/2018). The patients/participants provided their written informed consent to participate in this study.
Author Contributions
MH, KA, VG, FM, and FC designed and conceptualized the study and contributed to the analysis and interpretation of the data. MH carried out the measurements and designed the algorithms. FD supervised the study and KA co-advised it. MH drafted the manuscript, and all other authors revised it critically. All authors confirmed the final version and concurred to be responsible for all aspects of this study.
Funding
This project has received funding from the European Union’s Horizon 2020 research and innovation program under the Marie Skłodowska-Curie grant agreement No. 754354.
Conflict of Interest
Author FM was employed by Gait Up S.A. and author FD was employed by HUMA Therapeudics Ltd.
The remaining authors declare that the research was conducted in the absence of any commercial or financial relationships that could be construed as a potential conflict of interest.
Publisher’s Note
All claims expressed in this article are solely those of the authors and do not necessarily represent those of their affiliated organizations, or those of the publisher, the editors and the reviewers. Any product that may be evaluated in this article, or claim that may be made by its manufacturer, is not guaranteed or endorsed by the publisher.
Acknowledgments
We would like to thank all the swimmers who participated in this study and their coaches, especially Benjamin Paris, Jean-Christophe Sarnin, Adrien Perez, Thibaut Lefevre and Matthieu Balanch for their help in data collection and critical appraise of the results from a coaching perspective.
Supplementary Material
The Supplementary Material for this article can be found online at: https://www.frontiersin.org/articles/10.3389/fbioe.2021.793302/full#supplementary-material
References
Bächlin, M., Förster, K., Schumm, J., Breu, D., Germann, J., and Tröster, G. (2008). “An Automatic Parameter Extraction Method for the 7×50 m Stroke Efficiency,” in 2008 Third International Conference on Pervasive Computing and Applications ICPCA08, Alexandria, Egypt, 442–447. doi:10.1109/ICPCA.2008.4783628
Beanland, E., Main, L. C., Aisbett, B., Gastin, P., and Netto, K. (2014). Validation of GPS and Accelerometer Technology in Swimming. J. Sci. Med. Sport 17, 234–238. doi:10.1016/j.jsams.2013.04.007
Berrar, D. (2019). “Cross-validation,” in Encyclopedia of Bioinformatics and Computational Biology: ABC of Bioinformatics (Academic Pres), 1–3, 542–545. doi:10.1016/B978-0-12-809633-8.20349-X
Callaway, A. J., Cobb, J. E., and Jones, I. (2009). A Comparison of Video and Accelerometer Based Approaches Applied to Performance Monitoring in Swimming. Int. J. Sports Sci. Coach. 4, 139–153. doi:10.1260/1747-9541.4.1.139
Cossor, J. M., and Mason, B. R. (2001). “Swim Start Performances at the Sydney 2000 Olympic Games,” in International Society of Biomechanics in Sports: XIX International Symposium (University of San Francisco), 70–74.
Dadashi, F., Crettenand, F., Millet, G. P., and Aminian, K. (2012). Front-Crawl Instantaneous Velocity Estimation Using a Wearable Inertial Measurement Unit. Sensors 12, 12927–12939. doi:10.3390/s121012927
Dadashi, F., Crettenand, F., Millet, G. P., Seifert, L., Komar, J., and Aminian, K. (2013). Automatic Front-Crawl Temporal Phase Detection Using Adaptive Filtering of Inertial Signals. J. Sports Sci. 31, 1251–1260. doi:10.1080/02640414.2013.778420
Dadashi, F., Millet, G. P., and Aminian, K. (2015). A Bayesian Approach for Pervasive Estimation of Breaststroke Velocity Using a Wearable IMU. Pervasive Mobile Comput. 19, 37–46. doi:10.1016/j.pmcj.2014.03.001
Davey, N., Anderson, M., and James, D. A. (2008). Validation Trial of an Accelerometer‐based Sensor Platform for Swimming. Sports Techn. 1, 202–207. doi:10.1080/19346182.2008.9648474
De Jesus, K., De Jesus, K., Figueiredo, P., Gonçalves, P., Pereira, S., Vilas-Boas, J. P., et al. (2011). Biomechanical Analysis of Backstroke Swimming Starts. Int. J. Sports Med. 32, 546–551. doi:10.1055/s-0031-1273688
Félix, E. R., Silva, B. H., Olstad, P. L., Cabri, J., and Correia, P. L. (2019). SwimBIT: A Novel Approach to Stroke Analysis during Swim Training Based on Attitude and Heading Reference System (AHRS). Sports 7, 238. doi:10.3390/sports7110238
Ferraris, F., Grimaldi, U., and Parvis, M. (1995). Procedure for Effortless In-Field Calibration of Three-axis Rate Gyros and Accelerometers. Sensors Mater. 311.
Fonti, V., and Belitser, E. (2017). Feature Selection Using LASSO. VU Amsterdam Res. Pap. Bus. Anal., 1–25.
Guignard, B., Rouard, A., Chollet, D., Ayad, O., Bonifazi, M., Dalla Vedova, D., et al. (2017a). Perception and Action in Swimming: Effects of Aquatic Environment on Upper Limb Inter-segmental Coordination. Hum. Move. Sci. 55, 240–254. doi:10.1016/j.humov.2017.08.003
Guignard, B., Rouard, A., Chollet, D., and Seifert, L. (2017b). Behavioral Dynamics in Swimming: The Appropriate Use of Inertial Measurement Units. Front. Psychol. 8, 383. doi:10.3389/fpsyg.2017.00383
Guimaraes, A. C. S., and Hay, J. G. (1985). A Mechanical Analysis of the Grab Starting Technique in Swimming. J. Appl. Biomech. 1, 25–35. doi:10.1123/ijsb.1.1.25
Hamidi Rad, M., Gremeaux, V., Dadashi, F., and Aminian, K. (2021). A Novel Macro-Micro Approach for Swimming Analysis in Main Swimming Techniques Using IMU Sensors. Front. Bioeng. Biotechnol. 8, 1–16. doi:10.3389/fbioe.2020.597738
Le Sage, T., Bindel, A., Conway, P. P., Justham, L. M., Slawson, S. E., and West, A. A. (2011). Embedded Programming and Real-Time Signal Processing of Swimming Strokes. Sports Eng. 14, 1–14. doi:10.1007/s12283-011-0070-7
Madgwick, S. O. H., Harrison, A. J. L., and Vaidyanathan, R. (2011). “Estimation of IMU and MARG Orientation Using a Gradient Descent Algorithm,” in 2011 IEEE International Conference on Rehabilitation Robotics, Zurich, Switzerland. doi:10.1109/ICORR.2011.5975346
Magalhaes, F. A. d., Vannozzi, G., Gatta, G., and Fantozzi, S. (2015). Wearable Inertial Sensors in Swimming Motion Analysis: a Systematic Review. J. Sports Sci. 33, 732–745. doi:10.1080/02640414.2014.962574
Mansfield, E. R., and Helms, B. P. (1982). Detecting Multicollinearity. Am. Statistician 36, 158–160. doi:10.1080/00031305.1982.10482818
Mason, B., and Cossor, J. (2000). “What Can We Learn from Competition Analysis at the 1999 Pan Pacific Swimming Championships,” in International Symposium on Biomechanics in Sports Applied programme, Canberra, Australia, 75–82.
Mooney, R., Corley, G., Godfrey, A., Quinlan, L., and ÓLaighin, G. (2016). Inertial Sensor Technology for Elite Swimming Performance Analysis: A Systematic Review. Sensors 16, 18. doi:10.3390/s16010018
Morouço, P., Pinto, J., Félix, E., Correia, P. L., Humana, F. D. M., Lisboa, U. D., et al. (2020). “Development of a Low- Cost Imu for Swimmers’ Evaluation,” in International Conference of Biomechanics in Sports, 952–955.
Muthukrishnan, R., and Rohini, R. (2016). “LASSO: A Feature Selection Technique,” in 2016 IEEE International Conference on Advances in Computer Applications (ICACA), Coimbatore, India, 18–20. doi:10.1109/ICACA.2016.7887916
Pereira, S. M., Ruschel, C., Hubert, M., Machado, L., Roesler, H., Fernandes, R. J., et al. (2015). Kinematic, Kinetic and Emg Analysis of Four Front Crawl Flip Turn Techniques. J. Sports Sci. 33, 2006–2015. doi:10.1080/02640414.2015.1026374
Psycharakis, S. G., and Sanders, R. H. (2010). Body Roll in Swimming: A Review. J. Sports Sci. 28, 229–236. doi:10.1080/02640410903508847
Ruschel, C., Araujo, L., Pereira, S. M., and Roesler, H. (2007). “Kinematical Analysis of the Swimming Start : Block, Flight and Underwater Phases,” in XXV ISBS Symp, Ouro Preto, Brazil, 385–388.
Shimadzu, H., Shibata, R., and Ohgi, Y. (2008). Modelling Swimmers' Speeds over the Course of a Race. J. Biomech. 41, 549–555. doi:10.1016/j.jbiomech.2007.10.007
Shimojo, H., Sengoku, Y., Miyoshi, T., Tsubakimoto, S., and Takagi, H. (2014). Effect of Imposing Changes in Kick Frequency on Kinematics during Undulatory Underwater Swimming at Maximal Effort in Male Swimmers. Hum. Move. Sci. 38, 94–105. doi:10.1016/j.humov.2014.09.001
Siirtola, P., Laurinen, P., Roning, J., and Kinnunen, H. (2011). “Efficient Accelerometer-Based Swimming Exercise Tracking,” in 2011 IEEE Symposium on Computational Intelligence and Data Mining (CIDM), Paris, France, 156–161. doi:10.1109/CIDM.2011.5949430
Slawson, S. E., Justham, L. M., Conway, P. P., Le-Sage, T., and West, A. A. (2012). Characterizing the Swimming Tumble Turn Using Acceleration Data. Proc. Inst. Mech. Eng. P: J. Sports Eng. Techn. 226, 3–15. doi:10.1177/1754337111428395
Stamm, A., James, D. A., Burkett, B. B., Hagem, R. M., and Thiel, D. V. (2013). Determining Maximum Push-Off Velocity in Swimming Using Accelerometers. Proced. Eng. 60, 201–207. doi:10.1016/j.proeng.2013.07.067
Truijens, M., and Toussaint, H. (2005). Biomechanical Aspects of Peak Performance in Human Swimming. Anim. Biol. 55, 17–40. doi:10.1163/1570756053276907
Toussaint, H. M. (2002). “Biomechanics of Propulsion and Drag in Front Crawl Swimming,” in 20 International Symposium on Biomechanics in Sports, 2002, Cáceres, Spain, 13–22. Available at: https://ojs.ub.uni-konstanz.de/cpa/article/viewFile/613/538%0Ahttp://scholar.google.com/scholar?hl=en&btnG=Search&q=intitle:Biomechanics+of+propulsion+and+drag+in+front+crawl+swimming#5.
Vantorre, J., Seifert, L., Fernandes, R. J., Boas, J. P. V., and Chollet, D. (2010). Kinematical Profiling of the Front Crawl Start. Int. J. Sports Med. 31, 16–21. doi:10.1055/s-0029-1241208
Vantorre, J., Chollet, D., and Seifert, L. (2014). Biomechanical Analysis of the Swim-Start: A Review. J. Sports Sci. Med. 13, 223–231.
Wang, Z., Shi, X., Wang, J., Gao, F., Li, J., Guo, M., et al. (2019). “Swimming Motion Analysis and Posture Recognition Based on Wearable Inertial Sensors,” in 2019 IEEE International Conference on Systems, Man and Cybernetics (SMC), Bari, Italy, 3371–3376. doi:10.1109/smc.2019.8913847
Worsey, M. T. O., Pahl, R., Thiel, D. V., and Milburn, P. D. (2018). A Comparison of Computational Methods to Determine Intrastroke Velocity in Swimming Using IMUs. IEEE Sens. Lett. 2, 1–4. doi:10.1109/lsens.2018.2804893
Keywords: sports biomechanics, wearable sensor, swimming, performance evaluation, variable selection
Citation: Hamidi Rad M, Aminian K, Gremeaux V, Massé F and Dadashi F (2021) Swimming Phase-Based Performance Evaluation Using a Single IMU in Main Swimming Techniques. Front. Bioeng. Biotechnol. 9:793302. doi: 10.3389/fbioe.2021.793302
Received: 11 October 2021; Accepted: 22 November 2021;
Published: 07 December 2021.
Edited by:
Argyris G. Toubekis, National and Kapodistrian University of Athens, GreeceReviewed by:
Rodrigo Zacca, University of Porto, PortugalPedro Morouço, Polytechnic Institute of Leiria, Portugal
Copyright © 2021 Hamidi Rad, Aminian, Gremeaux, Massé and Dadashi. This is an open-access article distributed under the terms of the Creative Commons Attribution License (CC BY). The use, distribution or reproduction in other forums is permitted, provided the original author(s) and the copyright owner(s) are credited and that the original publication in this journal is cited, in accordance with accepted academic practice. No use, distribution or reproduction is permitted which does not comply with these terms.
*Correspondence: Mahdi Hamidi Rad, TWFoZGkuaGFtaWRpcmFkQGVwZmwuY2g=