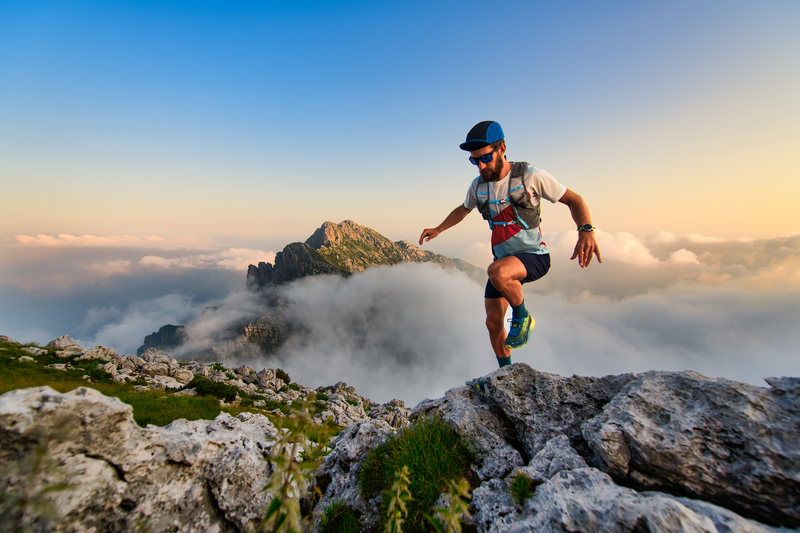
94% of researchers rate our articles as excellent or good
Learn more about the work of our research integrity team to safeguard the quality of each article we publish.
Find out more
REVIEW article
Front. Bioeng. Biotechnol. , 16 September 2021
Sec. Tissue Engineering and Regenerative Medicine
Volume 9 - 2021 | https://doi.org/10.3389/fbioe.2021.732130
This article is part of the Research Topic Biofabrication and Organs-on-Chips: Becoming More Automated and Realistic View all 7 articles
In the last decades, biomedical research has significantly boomed in the academia and industrial sectors, and it is expected to continue to grow at a rapid pace in the future. An in-depth analysis of such growth is not trivial, given the intrinsic multidisciplinary nature of biomedical research. Nevertheless, technological advances are among the main factors which have enabled such progress. In this review, we discuss the contribution of two state-of-the-art technologies–namely biofabrication and organ-on-a-chip–in a selection of biomedical research areas. We start by providing an overview of these technologies and their capacities in fabricating advanced in vitro tissue/organ models. We then analyze their impact on addressing a range of current biomedical challenges. Ultimately, we speculate about their future developments by integrating these technologies with other cutting-edge research fields such as artificial intelligence and big data analysis.
At its onset, at the end of the 1980s, the paramount goal of tissue engineering was to manufacture ex vivo cellularized substitutes to restore, maintain, or improve tissue functions in vivo, which could, ultimately, be used as building blocks for the production of whole functional organs. Although the latter is still almost exclusively the subject of science-fiction stories, researchers in the last three decades have made enormous progress in the fabrication of advanced substitutes capable of recapitulating specific functions of tissues and organs. As a result of these advancements, the application areas of tissue engineering have considerably broadened from the simple manufacturing of tissue substitutes towards being an answer to today’s main biomedical challenges, including drug development, disease, and tissue/organ modeling, and precision medicine. To better understand the progress achieved and the current trends, one should look backward and briefly analyze how the field has been established and evolved, paying particular attention to the technological advances that have been progressively introduced to meet the biological and clinical needs (Hubbell, 1995; Kim et al., 2000; Kaul and Ventikos, 2015).
Originally, tissue engineering found its driving force in the materials science community despite its intrinsic multidisciplinary nature. Pioneers of the field fabricated what can be termed the first generation of tissue-engineered substitutes, mainly aimed at discovering the cross-talk among materials, scaffold architecture, biological cues, and cells (Hollister, 2005; Vallet-Regí et al., 2007; Santos et al., 2010). In particular, the very introduction of three-dimensional (3D) materials for cell culture—generally referred to as scaffolds—generated a great wave of enthusiasm in the field as, for the first time, researchers demonstrated that the in vitro cellular responses could be significantly improved compared to standard two-dimensional (2D) cell cultures in plates (Knight and Przyborski, 2015; Souza et al., 2018). In the first studies, researchers had adapted prior existing technologies, such as solvent casting, particulate leaching, freeze-drying, gas foaming, and electrospinning to the manufacturing of biocompatible, highly porous 3D scaffolds (Guarino and Ambrosio, 2010; Raeisdasteh Hokmabad et al., 2017; Parham et al., 2020). 3D cell culture triggered a multitude of studies that originated invaluable information regarding cell attachment cues and cell-cell interactions, cytocompatibility of materials, and most importantly, identified the challenges faced. At the same time, the promising results captured the attention of researchers with a more biological background, laying the basis for the rapid implementation of such new 3D systems within their research pipeline.
Such a research phase continued for almost a decade until the end of the 1990s. During this time, the field advanced steadily on multiple fronts. In particular, researchers noticed that the methods for scaffold manufacturing were not entirely adequate to ensure neither overall control over macro- and micro-architecture of the 3D scaffolds nor cell distribution throughout them (Figure 1). As a direct consequence of these two issues, the repeatability of the experiments was relatively low, with the impossibility of comparing the results produced in different studies. This ignited the development of a plethora of different techniques for scaffold fabrication and the adaptation/redesign of old methods to the new needs. The peak in controlling scaffold architecture was finally reached at the end of the 1990s with the introduction of the 3D printing technologies (Mironov et al., 2003, 2009; Campbell and Weiss, 2007; Berthiaume et al., 2011). Such technologies enabled the fabrication of pre-determined 3D structures with unprecedented resolution, accuracy, and reproducibility. However, the fabricated 3D materials still required to be seeded with cells, and thus, all the issues connected with cell distribution and overall experiment repeatability were unmet. At the same time, researchers realized that culturing cells in 3D was not enough to attain tissular organization and, in order to move forward, it was essential to guide cells to form a functional tissue—i.e., a macroscopic structure that exhibits a histoarchitecture and functionalities comparable to the target tissue.
FIGURE 1. Tissue engineering evolution: (A) from Petri dishes to biofabrication and organ-on-a-chip. (B) Milestones in bioprinting in the 21st century (Kwon et al., 2000; Landers et al., 2002; Wilson Jr and Boland, 2003; Dhariwala et al., 2004; Jakab et al., 2008; Norotte et al., 2009; Wu et al., 2011; Skardal et al., 2012; Nakayama, 2013; Hinton et al., 2015; Gao et al., 2015; Kang et al., 2016; Retting et al., 2016; D O’Connell et al., 2016; Gladman et al., 2016; Lee J. H. et al., 2017; Pyo et al., 2017, 2017; Bernal et al., 2019; Skylar-Scott et al., 2019; Grigoryan et al., 2019, 2021; Jodat et al., 2020; Chen et al., 2020; Urciuolo et al., 2020; Brassard et al., 2021; Maharjan et al., 2021; Markert et al., 2021; De Santis et al., 2021). (C) Milestones in Organ-on-Chip in the 21st century (Huh et al., 2010; Nakao et al., 2011; Franco and Gerhardt, 2012; Jang et al., 2013; Nguyen et al., 2013; Wei-Xuan et al., 2013; Bersini et al., 2014; Rigat-Brugarolas et al., 2014; Torisawa et al., 2014; Choi et al., 2015; Park et al., 2015; Miller and Shuler, 2016; Rosa et al., 2016; Wufuer et al., 2016; Zhang et al., 2017a, 2021; Lind et al., 2017; Vernetti et al., 2017; Wikswo et al., 2017; Ingber et al., 2018; Lee et al., 2018; Marturano-Kruik et al., 2018; Achberger et al., 2019; Park et al., 2019; Chou et al., 2020; Marx and Ramme, 2020; Park et al., 2020; Varghese et al., 2020; Si et al., 2021).
At the beginning of this century, a new research era started having as the main focus the development of functional tissue models. This research line rapidly grew due to the increasing evidence of the inadequacy of animal models in predicting human responses, especially regarding drug safety and therapeutic efficacy. The aspiration to develop functional tissue models introduced new challenges to be faced that, in turn, established a new set of technological and biological needs.
Fabricating a macroscopic, 3D cellularized structure with biomimetic features requires precise control at the microscale over the spatial distribution of different cell types, matrix composition, and physicochemical stimuli. Without such control, cells would organize in a quasi-random manner with minimal functionalities and histoarchitectures far from the native ones (Figure 1). In this context, two groups of technologies have recently been established: biofabrication and organ-on-a-chip (Zhang and Radisic, 2017; Moroni et al., 2018a). Biofabrication technologies—which include bioprinting and bioassembly approaches—can be considered an evolution of 3D printing technologies specifically designed for the direct deposition of a bioink—i.e., a physiological solution containing living cells and matrix precursors—or cell-containing building blocks (Groll et al., 2016). On the other hand, organ-on-a-chip systems have been developed as a side branch of microfluidics. These systems comprise micro-channels and chambers where cells can be cultured under extremely controlled conditions, enabling the recapitulation of tissue/organ multi-cellular architectures, tissue-tissue interfaces, physicochemical microenvironments, and vascular perfusion of the body (Bhatia and Ingber, 2014).
In this review, we intend to outline the recent biotechnological advancements obtained using biofabrication and organ-on-a-chip technologies and provide an insight into how these two technologies can be used complementarily to assist biomedical research from bench to bedside.
Biofabrication and organ-on-a-chip technologies have the potential to revolutionize and boost biomedical research in the next few decades, eventually enabling the development of extremely accurate and functional in vitro tissues, organs, and disease models. In order to achieve this ambitious goal, it is paramount to recapitulate the microenvironment and the 3D spatial distribution of cells and extracellular matrix (ECM), ensuring native-like functionality both at the single-cell and tissue/organ levels. Currently, we are still far from fully addressing these challenges, and both biofabrication and organ-on-a-chip technologies suffer from some limits. Nevertheless, given their peculiar advantages, each of these technologies is finding concrete applications in different branches of biomedical research, becoming complementary rather than alternative to one another. Comparison charts of salient features for the two technologies are shown in Figure 2. For instance, biofabrication strategies are very suitable for regenerative medicine purposes through the manufacturing of biological constructs of clinically relevant size characterized by multi-scale, biomimetic architectures. On the other hand, organ-on-a-chip systems represent ideal platforms for higher-throughput studies such as those dealing with drug screening or toxicology. Moreover, they can be employed for studying the impact of various physiological stimuli on the cellular phenotype. In the next two paragraphs, we will provide the readers more insights regarding these two cutting-edge approaches.
FIGURE 2. Biofabrication vs organ-on-a-chip. Multi-feature comparisons between biofabrication and organ-on-a-chip technologies highlighting their advantages, limitations, and challenges. Features are classified using a 1–5 scale with 1 meaning very limited and 5 well-suitable (e.g., operator dependency: 1 highly operator dependent, 5 not operator dependent).
The extensive knowledge and advancements made in the medical, biological, and biotechnological fields have highlighted the limitations of conventional 2D cell cultures in the last decades. The increasing scientific needs demand the design and generation of advanced biomimetic 3D cellular and tissue models. The implementation of 3D manufacturing and materials science in biomedical developments have revealed relatively newer and more complex aspects, such as tissue microenvironments, cell-cell or cell-matrix interactions, and, most importantly, in recapitulating in vitro tissue/organ functionality. As anticipated in the introduction, biofabrication technologies have been developed in the last two decades as an extension of the 3D printing strategies for traditional scaffold fabrication. Since then, the field has greatly evolved, and nowadays, the term biofabrication groups together several additive manufacturing strategies. According to a recent publication, biofabrication is defined as the automated generation of biologically functional products with a structural organization from living cells, bioactive molecules, biomaterials, cell aggregates such as micro-tissues or hybrid cell-material constructs through bioprinting or bioassembly and subsequent tissue maturation processes (Groll et al., 2016). Following this definition, biofabrication strategies are primarily divided into bioprinting and bioassembly technologies. While bioprinting enables the 3D spatial arrangement of cells, biomaterials, and biologically active factors, bioassembly facilitates the modular fabrication of bio-structures using cell-containing units (Moroni et al., 2018b; Dalton et al., 2020). However, this primary classification is further ramified, and one can find several other sub-categories for both bioprinting and bioassembly (Figure 3). A thorough description of all these strategies is out of the scope of this review. Nevertheless, to help the readers going through the following sections, we will provide a brief overview of the most common biofabrication approaches, highlighting the differences in terms of cell/material deposition strategies and the quality of bio-substitutes fabricated.
FIGURE 3. Biofabrication strategies. Schematic representation of the various biofabrication methods employed for scaffold manufacturing divided into bioprinting and bioassembly strategies.
Bioprinting modalities can be sorted into three main groups: droplet-, fiber-, and voxel-based bioprinting (Gudapati et al., 2016; Ozbolat and Hospodiuk, 2016; Hong et al., 2018; Vijayavenkataraman et al., 2018). Droplet-based bioprinting is a non-contact printing process that focuses on the precise sequential positioning of cells in a single bioink drop (Angelopoulos et al., 2020). Droplet formation can be achieved through inkjet or drop-on-demand printing (DOD) (Derby, 2010). In inkjet systems, droplets are generated using thermal (Cui et al., 2010; Solis et al., 2019) or piezoelectric (Hewes et al., 2017; Masaeli et al., 2020) effect; in contrast, in DOD platforms, bioink drops are ejected onto a substrate using a laser beam (Kingsley et al., 2019) or acoustic waves (Foresti et al., 2018; Swaminathan et al., 2019). Fiber-based bioprinting is the most popular bioprinting approach, and in this frame, bioinks are loaded in disposable cartridges and extruded pneumatically or mechanically on sterile substrates. Extrusion systems are mainly based on the generation of continuous hydrogel filaments, which are generally solidified through photocuring (Maiullari et al., 2018), enzymatic (Tijore et al., 2018), ionic, (Kingsley et al., 2019), or thermal (Swaminathan et al., 2019) crosslinking. Lately, thanks to extensive research in the synthesis of photoresponsive biocompatible materials, voxel-based bioprinting systems, such as stereolithography (SLA) and digital light processing (DLP), are becoming increasingly popular. The main difference between these two systems is that SLA systems use a laser beam that moves from point to point to cure the resin, while in DLP systems, the UV light source is stationary, curing a complete layer of resin at a time (Hribar et al., 2014; Miao et al., 2018; Bernal et al., 2019).
The crucial point for bioprinting is choosing the proper bioprinting strategy and designing a biocompatible, instructive, and supportive bioink for the desired application (Gungor-Ozkerim et al., 2018; Ji and Guvendiren, 2021). Gelation kinetics, viscoelastic properties of biomaterial inks, and fabrication time directly influence the resolution, printing fidelity, and cell viability in the bioprinted constructs (Malda et al., 2013). For instance, low-viscosity bioinks are suitable for droplet-based bioprinting as the low-viscosity favors droplet formation at the printhead. On the contrary, high-viscosity bioinks are generally more suitable for fiber-based bioprinting, where the high viscosity of the bioink prevents the breaking and collapse of the deposited filaments during/after printing. Regardless of viscosity, for voxel-based printing, the use of photoresponsive, fast-curing bioinks is crucial to obtain high resolution, cell viability, and shape fidelity.
Alongside 3D bioprinting, bioassembly strategies are the other pillar of biofabrication. Bioassembly is defined as the fabrication of hierarchical constructs with a prescribed 2D or 3D organization through an automated assembly of pre-formed cell-containing units (Groll et al., 2016). One of the most sophisticated bioassembly approaches up to date can be considered as the organoids. These complex and highly trackable constructs are mainly inspired by embryogenic organogenesis, and the research based on organoids unravels the current challenges regarding tissue formation, cell-cell interactions, and tissue functionality (Takebe and Wells, 2019). Bioassembly approaches can be divided as well into three main categories according to the type of cell building block: spheroid-, cell-sheet-, and cell-fibers-based methods (Morimoto et al., 2015).
Strategies based on spheroids rely on the deposition through a nozzle or the assembly using external forces–such as aspiration or magnetic force–of pre-formed spheroids (Tseng et al., 2015; Ayan et al., 2020). Alternatively, pre-formed spheroids can also be assembled in 3D using arrays of tiny needles, a procedure known as the Kenzan method. Upon deposition, adjacent tissue spheroids undergo self-assembly forming larger tissue-engineered constructs (Murata et al., 2020).
Besides spheroid constructs, temperature-responsive culture plates (Shimizu et al., 2002; Sekine et al., 2013) have been used to obtain multi-cellular stratified cell-sheet building blocks. The temperature-responsive culture plates have been designed by grafting the polymer poly (N-isopropyl acrylamide) (p-NIPAM) to ordinary tissue culture dishes. Briefly, these temperature-responsive culture plates are relatively hydrophobic and cell-adherent under standard culture conditions. However, upon temperature reduction below the polymer’s lower critical solution temperature, the polymer surface becomes hydrophilic and swells, triggering the spontaneous detachment of the cell layer (Yang et al., 2005). Cell-fiber constructs consist of cell-seeded or cell-laden hydrogel fibers in which cells, after a certain culturing time, organize themselves into densely packed cell cords. For such applications, fibers are generally manufactured by molding (Shimizu et al., 2015) or laminar flow extrusion (Lee et al., 2009; Skylar-Scott et al., 2019; Watanabe et al., 2020). The plethora of biofabrication methods developed so far has generated extensive knowledge, and nowadays, first-generation functional models for several tissues and organs can be manufactured (Figure 4). Nevertheless, these models are in their infancy, still missing some key features needed to introduce disrupting systems in biomedical research truly.
FIGURE 4. Engineered tissue and organ models manufactured using biofabrication strategies. A selection of representative and advanced studies carried out with biofabrication systems: 3D printing of layered brain-like structures reproduced from ref. (Lozano et al., 2015), hybrid 3D bioprinting of retina reproduced from ref. (Shi et al., 2017), engineering in vitro air-blood barrier reproduced from ref. (Horváth et al., 2015), 3D printing of complex biological structures of the heart reproduced from ref. (Hinton et al., 2015), multi vascular networks and functional intravascular topologies reproduced from ref. (Grigoryan et al., 2019), bioprinting of multiscale hepatic lobules reproduced from ref. (Kang et al., 2020), bioprinting of 3D convoluted renal proximal tubules reproduced from ref. (Homan et al., 2016), microfluidic-enhanced 3D bioprinting of aligned myoblast-laden hydrogels reproduced from ref. (Costantini et al., 2017), 3D bioprinting using scaffold-free approach reproduced from ref. (Pourchet et al., 2017).
Recently, a wave of excitement has spread among the tissue engineering community following the development of new cell culture platforms, the so-called organ-on-a-chip. An organ-on-a-chip is a microfluidic cell culture device that contains continuously perfused and/or actuated (generally negative pressure-actuated) micro-chambers/channels populated by living cells arranged to simulate tissue- and organ-level physiology.
Born from the convergence of various biomedical research fields and microfluidics, organ-on-a-chips have aroused in the last two decades a growing interest in the scientific community for their potential in generating faithful and high-content reproduction of functional tissue units.
Organ-on-a-chips are generally manufactured using conventional soft-lithographic approaches, and, as one could expect, the first-choice material used for their fabrication is polydimethylsiloxane (PDMS) thanks to its moldability, biocompatibility, gas diffusion properties, and optical transparency, the latter representing a key feature enabling live monitoring of cells (Bhagat et al., 2007; Hongbin et al., 2009). More recently, 3D printing technologies—in particular SLA systems—are emerging as an alternative to soft-lithography for the rapid prototyping of organ-on-a-chips (Amin et al., 2016; Park et al., 2018). The reasons for that are numerous, including the reduction of costs associated with organ-on-a-chip manufacturing, ease of use, and the possibility to create more intricate, fully 3D microfluidic devices in a single step.
Organ-on-a-chips have been previously categorized for their functions, methods of fabrication, and tissue-specific applications (Ahadian et al., 2018; Wu et al., 2020). Here, we would like to propose a different classification meter based on the geometrical characteristics of organ-on-a-chip units. When analyzed closely, these can be classified into three major categories according to their intended purpose: micro-units, macro units, and dynamic units. Organ-on-a-chip micro units—such as microwells, traps, and posts—are mainly designed to provide stimuli or confined cells at a small cluster or even at the single-cell level. Organ-on-a-chip macro units can be described as channel networks and cross-communication channels, where multiple cell types can reproduce the systemic interaction and response of several tissue models. Dynamic units, instead, indicate all those components that can be actively deformed, inducing tailored stimuli fundamental to study the mechano-responses of cells/tissues under investigation and better recapitulating the actual in vivo conditions (Figure 5).
FIGURE 5. Organ-on-a-chip geometrical units. Different geometrical and structural organ-on-a-chip elements are grouped in three major categories: micro, macro, and dynamic units. While micro units provide stimuli or confined cells at a small cluster or single-cell level, macro and dynamic units are employed to recapitulate specific systemic functions. Such division has been proposed for the sake of presentation, however, organ-on-a-chip units can be designed to possess multiple elements belonging to different groups.
By recapitulating the multi-cellular architectures, tissue-tissue interfaces, physicochemical microenvironments, and vascular perfusion of the body, these devices produce levels of tissue and organ functionality that are not possible to achieve neither with conventional 2D or 3D culture systems nor with animals or human patients. They also enable high-resolution, real-time imaging and in vitro analysis of biochemical, genetic, and metabolic activities of living cells in a functional tissue- and organ context (Jackson and Lu, 2016). Moreover, organ-on-a-chip microsystems are particularly suitable for higher throughput screening, such as drug testing. Nowadays, the latter application is particularly under exploitation. If successful, it should be especially valuable for studying molecular mechanisms of action, prioritization of lead candidates, toxicity testing, and biomarker identification (Paul et al., 2010).
To date, a great variety of organs and tissues have been recapitulated into organ-on-a-chip-based systems, including liver (Nakao et al., 2011), kidney (Jang and Suh, 2010), intestine (Kim et al., 2016), lung (Douville et al., 2011), heart (Grosberg et al., 2011), muscle (Grosberg et al., 2012), vessel (Song and Munn, 2011), and bone marrow (Torisawa et al., 2014), up to complex models of body-on-a-chip (Esch et al., 2011) (Figure 6). However, despite the critical breakthroughs achieved in the field, a pressing unmet question that continues to emerge is the data-supported evidence of its actual benefits compared to existing conventional models or well-established tissue engineering approaches (Mastrangeli et al., 2019b). For instance, the advantages of the inclusion of dynamic perfusion and in situ stimulation in organ-on-a-chip are becoming evident only recently and more substantial readout correlations with clinical and human physiological behavior are still needed (Del Rio et al., 2017; Vernetti et al., 2017).
FIGURE 6. Engineered tissues and organs manufactured using the organ-on-a-chip technology. A selection of the representative and advanced studies carried out with organ-on-a-chip systems: blood-brain-barrier-on-a-chip reproduced from ref. (Vatine et al., 2019), retina-on-a-chip reproduced from ref. (Achberger et al., 2019), small airway-on-a-chip reproduced from ref. (Benam et al., 2016), liver-on-a-chip reproduced from ref. (Jang et al., 2019), vascularized and perfused organ-on-a-chip platform reproduced from ref. (Phan et al., 2017), tubuloids of human adult kidney-on-a-chip reproduced from ref. (Schutgens et al., 2019), heart-on-a-chip reproduced from ref. (Qian et al., 2017), skeletal muscle-on-a-chip reproduced from ref. (Agrawal et al., 2017), small intestine-on-a-chip reproduced from ref. (Kasendra et al., 2018), full-thickness human skin-on-a-chip reproduced from ref. (Sriram et al., 2018), on-chip recapitulation of clinical bone marrow reproduced from ref. (Chou et al., 2020).
Since their establishment, biofabrication and organ-on-a-chip technologies have evolved notably and nowadays represent the most promising approaches to overcome the restrictions of conventional biological and medical research methods. Together with these techniques, biomedical research has greatly advanced and branched into a multitude of sub-fields that reflect its intrinsic, complex heterogeneity. In this context, biofabrication and organ-on-a-chips are now called to face and possibly address relevant biomedical challenges such as 1) the manufacturing of spare tissues/organs to fight the shortage of organs’ donors, 2) the fabrication of reliable physiological and pathophysiological in vitro tissue/organ models to replace animal testing and to unravel new disease markers and mechanisms, 3) the reduction of costs connected with new drug development, and 4) the formulation of precision medicine therapeutic protocols. Indeed, these are long-term challenges, and still, many years will pass before one could actually solve them. Nevertheless, biofabrication (Table 1) and organ-on-a-chips systems (Table 2) have already contributed and, more importantly, are expected to further contribute significantly in the near future to address them. Below, we will briefly report and analyze the impact of these technologies in a selection of relevant biomedical challenges, including tissue engineering and regenerative medicine, disease models for precision medicine, drug development and testing drug kinetics, and in the growing field of omics.
TABLE 1. Representative studies based on biofabrication technologies reported for the selected biomedical applications.
TABLE 2. Representative studies based on organ-on-a-chip technologies reported for the selected biomedical applications.
Organ and tissue failures due to chronic or genetic diseases, trauma, or infection, represent a major medical issue worldwide, which nowadays can be effectively treated exclusively through organ transplantation. Such surgical operations are extremely invasive, and the placement of a heterologous organ often triggers a severe immune response in the host that causes organ rejection. TERM—two interdisciplinary research fields that combine engineering and life science principles—were proposed during the 1990s to replace, repair, or regenerate human tissues or organs with the ultimate goal of reestablishing normal tissue functions (Langer and Vacanti, 1993).
In the last decade, 3D biofabrication techniques and organ-on-a-chip have served to this goal as complementary approaches. Whereas organ-on-a-chip methodologies have generally provided some inputs to TERM at the cellular level (i.e., micro-tissue level), biofabrication technologies have been successfully tested for the fabrication of larger-scale constructs up to, in few cases, human-sized organs (Kang et al., 2016; Mirdamadi et al., 2020). However, the biofabricated structures, despite their realistic shapes and dimensions, exhibit very limited functionalities, thus being unsuitable directly for clinical applications.
As one could easily infer, fabricating artificial organs is an extremely complex task, and, up to date, it is still difficult to precisely identify and foresee all the actions that may be required for this process. Nevertheless, few fundamental steps will be needed, including 1) dedicated protocols for stem cell identification and expansion, 2) definition of blueprints for target organs/tissues—i.e., complete sets of instructions for automated assembly of the artificial organ/tissue, 3) development of post-printing protocols for engineered organ maturation. Below, we provide a concise description of these three key points and the challenges behind them.
Manufacturing engineered autologous tissues require billions to trillions of cells. At the cellular level, the living matter of an engineered tissue should have two intrinsic characteristics, cellular diversity—i.e., the range of different cell types that constitute a tissue—and self-renewal—i.e., perpetuating the cell pool throughout life. Thus, selecting a sustainable, appropriate autologous cell source as the starting point and establishing standardized scaled-up cell expansion processes represent pivotal milestones. Currently, stem cells, including embryonic stem cells (ESCs), adult stem cells (AdSCs), and induced pluripotent stem cells (iPSCs), are the sole remedy to guarantee cellular diversity and self-renewal for TERM applications as terminally differentiated cells tend to lose their proliferative and self-renewal potential rapidly when intensively expanded in vitro (Brown et al., 2013).
On the contrary, stem cells can undergo asymmetric divisions—i.e., one stem cell can divide into a progenitor daughter cell while the other one stays in a self-renewal state. Whereas self-renewal of stem cells is significantly advantageous in populating engineered tissue constructs, this phenomenon can jeopardize the sustainability of stem cell expansion processes (Shahriyari and Komarova, 2013). Hence, uncontrolled proliferation and differentiation of stem cells have to be strictly monitored during the expansion, and their phenotypic stability should be certified. Consequently, scaled-up expansion of stem cells would require substantial advancements in the media recipes, culturing devices, and techniques. One way to stabilize these processes can be the automation of the main cell culture variables. Alternatively, new culture conditions and media recipes could guarantee stable expansion, preserving the self-renewal capability and phenotypic stability of stem cells.
The process of designing a blueprint starts with deciphering the anatomical architectural complexity. Currently, the macro architecture of patient-specific tissue substitutes can be unprecedentedly designed using computed tomography (CT) and magnetic resonance imaging (MRI) data (Hosny et al., 2018). However, achieving biomimetic matrix composition and cellular-level complexity of the intact human organs is a daunting task, at first due to the lack of scalable technologies to image human organs at the cellular level. Although the standard histological techniques provide invaluable information of 2D sections, creating a 3D reconstruction of a whole organ from 2D histological sections could be very complicated or impossible because of the numerous tissue section distortions, besides being extremely time-consuming. In this context, some innovative approaches have recently been proposed to better decipher the anatomical complexity of matrix composition and diversity of cell populations (Menden et al., 2020; Zhao et al., 2020). One of these approaches focuses on developing deconvolution algorithms that can estimate different cell fractions and infer cell type-specific gene expressions in a tissue sample (Menden et al., 2020). These algorithms present valuable information regarding cell diversity. However, these algorithms do not provide any information about the spatial distribution of such cell populations. The latter information can be attained using a complementary method based on a 3D deep tissue immunofluorescence staining strategy recently introduced. This method—named SHANEL—involves clearing the tissue with a zwitterionic detergent (CHAPS (3-[(3-cholami- dopropyl)dimethylammonio]-1-propanesulfonate) and then labeling it with a 3D deep-tissue antibody-staining protocol, attaining precise 3D spatial information of specific cell populations (Zhao et al., 2020).
Alongside these prescient studies to resolve the limitations in large organ design, significant success has been achieved by implementing the bottom-up tissue engineering approach in the manufacturing of tissue/organ building blocks—i.e., miniaturized constructs generally having a volume up to 1 cm3—which exhibit histoarchitectures and functions close to the native targeted tissue/organ. For instance, researchers have recently succeeded, to some degrees, in fabricating tissue-specific building blocks by recapitulating the architectures and functions of the human liver lobules (Ma et al., 2016; Gori et al., 2020), the dynamic microenvironment of the alveolar-capillary unit (Huh, 2015), the parallel organization of myofiber bundles (Costantini et al., 2021), and the primary human small intestine (Kasendra et al., 2018; Shin et al., 2019). Despite the diversity in their structural and cellular complexity, the conjoined outcomes of these studies indicate that effective architectural guidance provides crosstalk among the cell populations and enhances the morphogenesis of the desired tissue. The preferential alignment of cells through contact guidance along with the topographical features actively impacts polarization, aggregation, and, as a result, differentiation and maturation of the cells. Consequently, in defined architectures, cells can sense organ size and functionality, initiating a cross-talk to terminally differentiate, stop proliferation, and yet, retain a minimal level of stemness for homeostasis and potential for regeneration (dos Santos and Liberali, 2019).
Housing billions to trillions of stem cells in anatomically legit architectures and directing them into full-size, functional engineered organs require standardized differentiation protocols and dynamic microenvironments. Inspired from the industrial bioprocesses, bioreactors have been adapted to TERM applications for subjecting 3D engineered tissues to various physiologically relevant stimuli, such as mechanical stresses, nutrient/cytokine gradients, and culturing conditions. An interplay between these physiological stimuli and the cellular components is the key element for ensuring their maturation into full-size, functional engineered organs. As nutrients, oxygen, and their transport mechanisms hold paramount importance for the development of tissue structures and functions, up to date, different bioreactor designs with circulatory system approximation have been proposed to address the difficulties for sufficient nutrient/oxygen supplies through engineered tissue building blocks (Kasendra et al., 2018). Alongside the sufficient nutrient supplies, such reactors have also enhanced tissue maturation as a result of the creating gradients of growth factors, cytokines, changing ECM directionality, and effective degradation of the matrix.
Although these bioreactors can satisfy the needs of long-term cultures in certain aspects, the nutrient diffusion through full organ thickness is still limited without a functional vasculature network. Introducing endothelial cells and a mechanism of angiogenic growth factor release are the current strategies to induce vascularization in engineered tissues. Nevertheless, creating fully vascularized constructs with capillary-size, perfusable, tubular constructs is significantly demanding mainly due to the limited resolution of current biofabrication techniques alongside the extensive phenotypic and zonal heterogeneity of endothelial cells. While various bioreactors have been designed to improve diffusion or vascularization, some bioreactor layouts have also evolved towards introducing physiological stimulation to tissue constructs. In long-term tissue culture, the dynamic state is favorable as it introduces a degree of stress that impacts the performance of engineered organs (Selden and Fuller, 2018). The applied mechanical stresses influence the mechano-sensing properties of the cytoskeleton proteins that play a critical role in representing physiological stimulation effects on cell behaviors. As an example, smooth muscle—endothelial cell co-cultures may transform into arteries-like functionality when subjected to blood pressure loads. However, these co-cultures may exhibit heart valve-like functionality when they are subjected to alternating pressure gradients (Ayoub et al., 2016). Regarding musculoskeletal tissues, bioreactors designed to mimic mechanical stretching and electrical stimulation will provide a good model for studying the functional maturation of tissue constructs (Parrish et al., 2019). As physiological stimulation is required to sustain homeostasis, cell polarity, plasticity, and tissue maturation, surely, at different stages of development, engineered tissues might require different regimes of physiological conditioning due to the maturation of the engineered tissue, changes in the cell population densities and the accumulation of extracellular matrix.
In medicine, signs and symptoms are the core of the diagnostic approach to specify and explain the disease state as well as to establish a prognosis and a therapeutic approach. Nevertheless, the outcome may vary among the patient groups due to their genetic, phenotypical, and psychosocial characteristics. Hence, the recent clinical data on individual patient differences and the developments in biomedical research have propounded the idea of precision medicine. Precision medicine mainly focuses on the treatments that distinguish the individual patients’ needs based on their genetic, clinical, phenotypic, psychosocial idiosyncrasy from other patients with similar clinical representation. The precision medicine hypothesis resides on the premise that stratified disease subgroups can be better treated through precise and validated genetic and phenotypic recognition (Ashley, 2016). There is no steady-state endpoint where precise medical care is provided to the patients; hence, each stratification can be just the interim result of the whole process. The cycles in stratification imply that the prognosis and treatments in patient subgroups become more and more effective with ongoing efforts. For instance, the discovery of CYP2C19 gene’s role in some drug cardiotoxicity—e.g., Terodiline, Clopidogrel, Desipramine—and specific drug efficacies for cancer patients with certain mutations—such as the case of effective taxane chemotherapy for BRCA1 mutation breast cancer patient subgroup—can be considered as some distinctive victories of precision medicine (Wang et al., 2013; Asif et al., 2016; Balasubramaniam et al., 2017). Indeed, precision medicine is not a need or a unique tool for all diseases. Currently, precision medicine is finding promising applications in some medical fields, such as cancer, neurodegenerative and rare diseases, to assure effective treatments and bench-to-bedside transition of these treatments for patient subgroups with similar epigenetic profiles. Despite the major premise of precision medicine, the field requires enhancements in two main aspects for its clinical translation. Specifically, collecting and analyzing the data to stratify the patient subgroups is considered the first main hurdle of precision medicine. Currently, cluster analysis of differently expressed genes is being used to define the patient subgroups as well as to discover the underlying pathological mechanism and a potential target for therapeutic development. Nonetheless, this technique requires full data sets on the genetic, clinical, phenotypic, psychosocial idiosyncrasies of the patients, which are not always obtainable (Kaur and Chupp, 2019). Moreover, handling the out-of-range data that are below or above the detection or threshold limits also restricts the success of cluster analysis. The second aspect to be improved consists of how well the patient subgroups respond to the proposed treatment. Longitudinal assessments—i.e., short-term assessments of treatment efficacy, recurring assessments over a period of time to reinforce treatments, iterative disease remission testing, and follow-up assessments to ensure successful treatment—reflecting the disease courses and planning safe clinical trials are substantial for precision medicine’s clinical translation. Since there is a gap between the data collection and establishing the clinical translation of the targeted treatments, 3D engineered tissue models, and disease-on-a-chip platforms have been proposed to bridge this gap. The use of engineered tissues and disease-on-a-chip can offer new platforms that can model the molecular and cellular disease phenotypes, develop correlated data for the cluster analysis and present longitudinal assessments of therapeutic responses on the bench for clinical translation.
Cancer is an epigenetic disease caused by mutations to genes that control the way our cells function, especially how they grow and divide. The epigenetic changes that cause cancer can be inherited or can arise during a person’s lifetime due to damage to DNA caused by specific environmental exposures. Over the years, the clinical findings have indicated genetic differences among cancer patients with the same diagnosis. Thus, precision medicine has gained increasing interest, potentially being the next frontier in cancer research (Friedman et al., 2015). Up to date, patient subgroups with non-small cell lung cancers due to the mutation in the anaplastic lymphoma kinase (ALK), BRCA mutant-ovary cancer, breast cancer with human epidermal growth factor receptor 2 (HER2) overexpression have already benefited from precision medicine with targeted therapies (Vogel et al., 2002; Neff et al., 2017; Qin and Gadgeel, 2017).
Nevertheless, clinical translation of precision medicine in cancer research is often hampered due to the cost of genomic testing, inadequate phenotypic and psychosocial patient information, and lack of longitudinal assessment of therapeutic responses. In this frame, biofabricated 3D models and tumor-on-a-chip platforms have been proposed for understanding the cancer progression and efficient bench-to-bed translation of precision medicine. Whereas 3D biofabrication strategies have been able to recapitulate the tumor microenvironment complexity by patterning tissue-specific bioinks and fabricate physiologically relevant tissue constructs with high spatiotemporal control over the 3D structures, tumor-on-a-chip platforms mostly aim to recreate significant features of the tumor physiology at molecular and cellular levels (Yesil-Celiktas et al., 2018). For instance, tumor-on-a-chip platforms have provided unique microenvironments to simulate the overall tumor pathologies (Wang Z. et al., 2019; Wei et al., 2019; Mencattini et al., 2020). In this scenario, single-cell tumor-on-a-chip platforms have been employed to study the complex composition of tumors. These platforms have been used to visualize rare cancer cells in bulk tumors, with the final aim to interpret tumor heterogeneity comprehensively, detect circulating molecules, and widen the current know-how of cancer genomics (Saadatpour et al., 2015; Tsoucas and Yuan, 2017).
Conversely, multiple-cell-type tumor-on-a-chip platforms (e.g., cancer, endothelial, stromal, and immune cells) have been used to investigate the specific crosstalk between different cell populations in the tumor microenvironments such as signaling cascades, tumor angiogenesis, and metastasis pathways (Kolesky et al., 2014; Grolman et al., 2015; Sharifi et al., 2020; Cabon et al., 2021). Alternatively, 3D-bioprinted compartmented multiple-cell constructs have been used to create paracrine loops for studying cancer cell extravasation into the bloodstream and analyze metastatic progression through trans-endothelial migration (Kolesky et al., 2014; Wang et al., 2018c; Li J. et al., 2020). Without a doubt, both the 3D biofabrication and the tumor-on-a-chip platforms have advanced the in vitro cancer research up to a new level by providing stable longitudinal real-time monitoring, as well as dynamic microenvironment to discover cancer and metastasis mechanisms which can be used in precision medicine for stratifying sub-groups and planning safe therapeutic strategies for patients. Most likely, these platforms will progressively become key tools for precision medicine application in cancer research in the near future, possibly with the convergence of the inputs from other fields, such as omics, systems biology, and bioinformatics.
A rare disease is defined as a condition that affects fewer than 200,000 people globally. The major problems associated with rare diseases are the lack of understanding of the disease mechanisms and the very small patient population that prohibits efficient clinical trials to identify effective therapeutic approaches. These issues make rare diseases a focus point of precision medicine. Recently, with the development of iPSCs and CRISPR technologies, establishing relevant mutations on patient-specific cells has become a common practice that could be helpful to understand rare disease mechanisms in 2D cell culture. These results should then be translated into suitable biofabricated or disease-on-a-chip systems to further increase their accuracy and reliability.
Although the research in engineered models of rare diseases is at its preliminary state, there have already been successful pilot studies. For instance, various multi-organ-on-a-chip platforms based on iPSCs and CRISPR technology have been proposed to induce specific mutations in an engineered cell population to study the autoimmune response and to understand the complex mechanism of autoimmune diseases, such as type I diabetes, rheumatoid arthritis, and celiac disease (de Mello et al., 2019). Since the severity of autoimmune diseases varies considerably amongst individuals, the potential to model combinations of genetic, environmental, and cellular components makes multi-organ-on-a-chip platforms uniquely able to determine the most relevant factors in disease progression for specific patients. For rare autoimmune diseases, where the incidence is too low to gather essential data on affected individuals and shared risk factors, multi-organ-on-a-chip systems can potentially be engineered with modules representing a patient’s own somatic and immune cells to determine the disease cause and to identify or test relevant therapeutics as a precision medicine application.
Along with the multi-organ-on-a-chip studies for autoimmune diseases, rare multifocal motor neuropathies have also been recently studied to understand the disease mechanisms at the cellular level. Hence, a human-based neuromuscular junction (NMJ) model has been suggested for congenital myasthenic syndrome, which commonly arises from mutations in one of the acetylcholine receptors encoding genes. The described 3D co-culture model provided a robust method to investigate adult human NMJ development and, for the first time, the adult forms of neuromuscular diseases in vitro (Bakooshli et al., 2019). Indeed, the use of engineered rare disease models is still in its infancy; nevertheless, the premise of these platforms has already started to play a pivotal role in streamlining the precision medicine processes.
Neurodegeneration, the slow and progressive dysfunction and loss of neurons and axons in the central nervous system, is the primary pathological feature of acute and chronic neurodegenerative conditions. As neurodegeneration may occur due to genetic or phenotypic idiosyncrasies, it is of great interest in precision medicine. Although the neurodegenerative diseases may vary significantly in their clinical and pathological characteristics, at the molecular level, they share a fundamental pathogenic mechanism termed the seeded aggregation of disease-specific proteins. TDP-43 protein accumulation in amyotrophic lateral sclerosis, beta-amyloid accumulation in Alzheimer’s disease, and huntingtin protein accumulation in Huntingtin disease can be considered as the most common examples (Wegorzewska and Baloh, 2011; Park et al., 2015; Virlogeux et al., 2018). Alongside identifying disease-related protein accumulation, the interaction between the neurons and vascular cells has been established as a critical player in regulating the neuronal function and fate of neurodegenerative disease. Despite the recent progress—i.e., discovering the mutations, misfolded proteins, and neurovascular interaction—most of the human neurodegenerative conditions remain poorly understood, as the currently available models based on the transgenic mouse, rat, and non-human primate models, are unsatisfactory to reveal the interplay of genetic and environmental factors in many cases. Thus, 3D-biofabricated and disease-on-a-chip systems have been proposed as alternatives to create more realistic human neurodegenerative disease models. Recent studies have shown that by employing these systems, it is possible to obtain results comparable with the current gold-standard in vivo and 2D in vitro disease models. In few cases, these new platforms have even outperformed such standards, identifying the synergetic effects of genetic and environmental factors, neuroinflammation, and production of proinflammatory cytokines. For instance, the most recent achievements in the design of neurodegenerative disease models are the development of neural network-on-a-chip models and a corticostriatal network-on-a-chip for studying Alzheimer’s disease, Huntingtin disease, and amyotrophic lateral sclerosis (Wegorzewska and Baloh, 2011; Park et al., 2015; Virlogeux et al., 2018). Without a doubt, these platforms cannot recreate the higher-level cognitive processing of a human brain or the full impact of neurodegeneration on the body. Yet, these tunable platforms hold great potential in precision medicine for deciphering the molecular and cellular neuro-pathologies by integrating genetic and phenotypic idiosyncrasies into the disease models.
The 1990s can be considered a golden era in the pharmaceutical industry as the discovery of several blockbuster drugs generated maximal profits for the pharmaceutical sector. However, in the last two decades, the increased regulatory scrutiny in drug safety has decelerated the fast growth resulting in reduced revenues (Krumholz et al., 2007). Moreover, key patent expirations between 2010 and 2014 caused a further decline in product proceeds, which reduced the budget spent on drug development (Khanna, 2012). The reduced R&D expenditures have tumbled the discovery of potential revenue-generating innovative drugs, creating a financially vicious circle.
In recent years, reports from regulatory agencies have indicated that only less than 7% of phase I drugs have been launched to market. Hence, the pharmaceutical industry has taken significant measures to increase the bench-to-market turnover of phase I drugs. Low bench-to-market turnover and high cost of the clinical trials have diverted pharmaceutical research into a more precise and accurate assessment of new drugs at the pre-clinical stage (Paul et al., 2010). Therefore, extensive efforts have been put into developing new screening methodologies for the pre-clinical stage, mimicking the reality of clinical trials alongside drug discovery. Thus, only the safest and most efficient new drugs will be qualified for clinical trials in a fast and financially feasible manner.
Drug safety is the primary concern of clinical drug attrition. Unfortunately, at the pre-clinical stage, overlooking the population variance and uncorrelated in vivo toxicity may jeopardize the drug’s success during clinical trials. At the clinical trial period of a new drug, ADME (absorption, distribution, metabolism, and excretion) profiles, dosing, side effects, toxicity, and interaction with different drugs should be evaluated considering the population variability. 3D biofabrication and organ-on-a-chip systems are already employed for quasi-in vivo toxicity testing subsidiary to animal studies. Alongside their use in toxicity testing, the pharmaceutical industry, ethics committees, and lawmakers have also started to debate whether these systems can be better predictive models to explore therapeutic efficiency (Ingber, 2020). Whereas 3D biofabrication approaches aim to contribute to drug testing research, up to date, organ-on-a-chip technologies have been mostly applied and even commercialized for toxicology testing at the pre-clinical level. Parallel to the benchwork, these systems have also been proposed for designing precise and accurate data sets for in silico libraries as machine learning became widely used in the field of computer-aided drug discovery and development of new drugs (Rifaioglu et al., 2019).
The current pre-clinical in vivo testing is inadequate to satisfy population diversity in the majority of the cases, and often the absence of toxicity in animal models, do not correlate with a similar lack of toxicity in humans (Bailey and Balls, 2019). Up to date, different organ-on-a-chip devices and 3D-biofabricated tissue models such as blood vessels (Grigoryan et al., 2019), bone marrow (Chou et al., 2020), the gut epithelium (Madden et al., 2018), lung (Chou et al., 2020), liver (Nguyen et al., 2016), ocular compartment (Achberger et al., 2019), kidney epithelium (Homan et al., 2016), skin (Madden et al., 2018), and reproductive organ (Xiao et al., 2017) have been reported to recapitulate the structural and functional complexity of human organs to study tissue-specific toxicity and overcome the drawbacks in the animal testing. One of the main advantages of organ-on-a-chip systems is the possibility of using human cells derived from different donors to attain toxicity information related to diverse human populations. Thanks to such developments, some commercialized tissue-specific organ-on-a-chip devices have even started to become a standardized procedure for toxicity testing for several research groups. Indeed, designing functional tissue models to investigate organ-specific toxicity of drugs is beneficial; nonetheless, organ-specific requirements are not sufficient to provide a full understanding of drug safety. Thus, the proposed systems should enable the incorporation of fundamental physiological responses regarding ADME profiles, immunity, endocrinal effects, gut-microbiome interactions, effects on reproductive organs, and real-world multi pathology. Therefore, multi-organ-on-a-chip systems have been widely investigated, aiming at validating the functionality of each organ in the multi-organ-on-a-chip separately. Without a doubt, up to date, these studies have demonstrated, on the one hand, a high potential for the use of functionally coupled multi-organ-on-a-chip for drug assessments and, on the other hand, that highlighting the pertinent need to overcome existing challenges. First, these systems cannot be considered as an exact mimic of the entire in vivo effects but as a more predictive and biologically relevant assay for the drug discovery cascade. The main challenges in integrating organ-on-a-chips into multi-organ-on-a-chips are the optimization of custom cell culture medium formulations for each organ and developing of perfusion of the nutrients and the oxygen throughout the overall multi-organ-on-a-chip. Concurrently, thorough assessments in proper scaling of organ-on-a-chip models, implementation of real-time evaluation, acquiring renewable cell sources, developing chemical, mechanical, and electrical cues for missing organ systems, structural and functional validation of multi-organ-on-a-chip platforms required for the translation of these systems.
Despite the in vivo testing is currently the gold standard to explore therapeutic efficiency, qualitative and quantitative high-resolution analysis of diverse biological processes has not been possible in animal models. Thus, the demand for 3D-biofabricated tissues and organ-on-a-chip devices is significantly increasing thanks to their key advantages of enabling direct real-time or end-point analysis. These systems can reveal the collective and independent interaction of an organ’s different tissue components with new therapeutics and unveil the mechanism of drug action and their efficacy at the molecular level (Benam et al., 2016; Knowlton and Tasoglu, 2016; Srivastava et al., 2016; Song et al., 2020; Si et al., 2021). However, small culture volumes and low cell numbers in these platforms often give rise to technical issues associated with detection sensitivity and specificity. Research in the field is trying to overcome these technical issues by finding a focal point between what needs to be measured—the biomarkers—and which analytical method should be used in the detection limits for high specificity and sensitivity (Li and Tian, 2018). Biomarkers are specific molecules that can be objectively measured and evaluated as an indicator of a normal biological process, a pathological process, or a biological response to a therapeutic intervention. Precise identification of application-specific biomarkers is crucially important; hence, the collection and handling of all samples and all test performance should be conducted in a standardized manner. Therefore, analytical techniques, such as enzyme-linked immunosorbent assays (ELISA) (Luan et al., 2017; Gjorevski et al., 2020), liquid chromatography coupled with mass spectrometry (LC-MS) (Mao et al., 2018), and polymerase chain reactions (PCR) (Phan et al., 2017) as well as micro-total analysis systems (µTAS) (Fantuzzo et al., 2018), have been integrated within organ-on-a-chip platforms, showing high-resolution biochemical analysis for commercialized drugs and new therapeutic agents with substantially reduced sample volume requirements (Yesil-Celiktas et al., 2018).
Since the quantitative structure-activity relationship (QSAR) concept has been proposed back in the 1960s, QSAR-based models have been used in the lead-optimization step of drug discovery to assess various drug properties such as enzymatic reactions of drugs, drug-target interactions, and drug toxicity (Marx et al., 2020). Prospering and clinically coherent predictions of in silico tools have substantially contributed to drug screening and identifying potential new drug leads. Moreover, recently, with the integration of machine learning methods to develop QSAR models, extraction of non-parametric and non-linear relationships from datasets has been expedited. Consequently, these developments have accelerated the analysis of ineffective compounds and enabled the development of in silico models with better predictive performance. Indeed, the increased predictive values will serve for patient benefit and the reduction of in vivo testing. A predictive model’s power strongly relies on experimental data, and obtaining reliable experimental datasets can only be possible through automated and standardized experimental protocols. In this context, organ-on-a-chip platforms are extensively trying to meet machine learning platforms’ needs at all levels of their life cycle, training, and feedback loops. Ultimately, these systems are envisioned to create in silico libraries and a new level of bio-virtuality by bridging human in vitro and in silico models.
Omic sciences attempt to comprehensively study the complex interactions between molecules in the different systems biology layers. With the progress and development of new postgenomic technologies, omics studies are becoming increasingly prevalent and more accessible to diverse disciplines. In order to succeed in the omic investigation, the correct design of the experimental part is crucial. Omic approaches, based on a holistic view of molecules, can improve the validity of preclinical predictions for drug response, which is essential to patient survival, and at the same time would reduce the cost of clinical practices. The existing technologies are differentiated by the specific target they will detect, and the leading omic techniques can be classified as genomics, transcriptomics, proteomics, and metabolomics. Whereas the analysis of gene expressions is the primary concern of genomics, the entire set of transcripts, including coding and non-coding RNAs, are the subjects of transcriptomics. Additionally, proteomics and metabolomics are involved in investigating the whole set of proteins and the large-scale study of metabolites of a cell, tissue, or organ.
The modern evolution of omic practices has brought the resolution of analysis at the single-cell level. The refinement of technologies has moved the challenge towards studying small quantities of molecules contained in each cell (Chappell et al., 2018). To date, omic analyses have allowed us to reconstruct networks and pathways that have been used to extrapolate the functional interactions between genes, proteins, and metabolites. Furthermore, single-cell approaches have highlighted the heterogeneity among cells within populations previously considered homogeneous (Vemuri and Aristidou, 2005; Bodein et al., 2020; Xing et al., 2020). Unfortunately, many preclinical omic data were generated within 2D biological systems and thus are affected by the lack of contribution of the extracellular microenvironment, the parenchymal and vascular compartments, and the tissue-tissue interface. Therefore, only a few of them have generated new algorithms with an adequate prognostic capacity to be used in medical practices (Sontheimer-Phelps et al., 2019; Canzler et al., 2020). Consequently, the combined use of high-throughput omic methods with 3D biofabrication or organ-on-a-chip technologies could represent an opportunity to validate new drug targets and develop new personalized therapeutic strategies.
As mentioned above, several in vitro organ and 3D tumor models have already been generated, and their deep characterizations are progressively implemented by emerging multi-omic technologies (Agarwal et al., 2017; Trujillo-de Santiago et al., 2019). However, to date, mostly microtissues cultured within OOC systems have been used as inputs for omic analyses—which are generally performed off-chip—while no significant steps have been reported in the integration of biofabricated samples and omics approaches. In particular, OOCs coupled with omics have been developed based on the profiling of the metabolome in microfluidic bioartificial organs with the ability to identify toxicity markers in vitro (Shintu et al., 2012; Lee D. W. et al., 2017; Jellali et al., 2018). For example, Wang and colleagues created an excellent human organ-on-a-chip system integrating, for the first time, cell engineering as well as drug metabolism and metabolomics to imitate complex human physiology and multi-organ interconnections (Wang X. et al., 2019). With this system, the metabolomics of ‘tolcapone,’ a medicine used to treat the symptoms of Parkinson’s disease, has been able to generate complete metabolite profiles, highly representative of the human system. More recently, Ndiaye et al. presented a ChipFilter Proteolysis (CFP) microfluidic platform as a proteomics bioreactor for the miniaturization of protein extraction steps for bottom-up proteomics approaches. This system allows the combination of a molecular filtration membrane in PDMS microchip, using soft lithography and replica molding, promoting efficient protein retention and proteolysis on the membrane, and reducing significantly the time for proteome analysis and the amount of the samples if compared with membrane-based commercial ultracentrifugation cartridges (Ndiaye et al., 2020).
Furthermore, integration with microfluidic platforms could further allow the assessment of in situ cytotoxicity and the dynamic drug-transport and -delivery behavior from nanocarriers in the same system (Zhang et al., 2017b; Chen et al., 2018, 2021). The dizzying expansion of these technologies has, however, seen the need to re-isolate the cells once they have done their job within a complex bioprinted or microfluidic system. To date, methods for isolating single-cells in a format compatible with single-cell omic experiments include laser acquisition microdissection (Emmert-Buck et al., 1996), manual micromanipulation (Hu et al., 2016), patch-clamp method (Bussard et al., 2016), and Raman tweezers (Snook et al., 2009). However, the high amount of work does not guarantee a high percentage of isolated cells used in the experimental system, sometimes tens and sometimes hundreds per study. Therefore, increasing the number of cells profiled is the right strategy for overcoming the noise that is intrinsic in single-cell measurements. In the past few years, microfluidics-based chips have been established to increase the number of cells profiled, reducing the experimental costs and coupling the tissue engineering approaches to the next-generation sequencing, a currently preferred method for omics analyses. To date, several microfluidic devices have been adapted to address concerns of productivity and cost in single-cell preparation and analysis. The main method couples microfluidic channels with pressure-control valves (Whitesides, 2006; Fan et al., 2011). Other innovative techniques for detecting single-cells and their components are based on using a microfluidic apparatus that is able to capture them in an inert carrier oil or using arrays of nanoliter-scale wells (nano-wells) in which, by gravity, cells or cellular components are seeded at low densities to reach a single element per well (Prakadan et al., 2017). Each of these approaches can be used to establish the interconnection between single cells, capture their specific products, and retain their components upon lysis. Importantly, given their small size, these features can be used to process many single cells in a compact physical space, reducing reagent requirements (and thus costs) and increasing analyte concentrations and thus assay efficiency when limited kinetically or by background. These innovative systems that focus on cellular output once intercellular dynamics are exhausted, have significantly improved the performance of single-cell omic studies, enabling the parallel processing of thousands of cells. The platforms developed so far not only exploit the experience of providing realistic physiological models, but also significantly contribute to reducing the costs for the preparation of molecular libraries for single-cell approaches by reducing the reaction volumes to the scale of nano/picoliters (Esch et al., 2015).
In the last two decades, biomedical research has greatly benefited from introducing highly sophisticated methods to fabricate more advanced in vitro models of tissues and organs. Organ-on-chips and biofabrication technologies are two great examples of them. These technologies are effectively driving a revolution in several areas of biomedical research. In this review, we have initially provided a brief overview of the broad set of available biofabrication and organ-on-a-chip technologies. This is followed by analyses of how they have been exploited by researchers in few research scenarios, which include the fabrication of functional, implantable tissue/organ substitutes, the development of platforms for modelling diseases or drug testing/screening, and the integration of omic analyses. As described in the text, the progress made is relevant; however, we are still far from translating these solutions into actual clinical scenarios due to unmet technical challenges and lack of fundamental knowledge of the biological processes involved (Hoffman et al., 2019).
In the context of tissue engineering and regenerative medicine, biofabrication technologies have affirmed themselves as the gold standard enabling to recapitulate architectural complexity and dimensions of human organs. The advantages offered by these technologies are numerous, including automated generation of 3D, biologically relevant tissues with high precision and repeatability. The 3D structures biofabricated so far, despite their realistic shapes and dimensions, still exhibit limited functionalities, thus being unsuitable directly for clinical applications. As one could easily infer, fabricating artificial tissues/organs is a highly complex task, and, up to date, it is still difficult to precisely identify and foresee all the actions required for this process. Typical current approximations entail the number and type of cells, their 3D spatial distribution, and the presence of a functional vasculature and innervation. The latter features are required to supply nutrients to each cell in the construct and, depending on the target tissue, support a proper integration with the host nervous system upon construct grafting in vivo. Among the possible challenges, those connected with the scaling-up processes to manufacture an idoneous number of cells will most likely need in the future a thorough revision and improvement to fulfil the stringent requirements of GMP production. Additionally, researchers will need to understand better the relations between bioink properties and the fate of embedded cells. So far, in fact, the focus has been mainly directed to formulate bioinks that would enable high printing resolution and support preliminary tissue maturation. Last but not least, on the bench to clinical translation side, designing mechanically stable, biocompatible, and financially feasible constructs can be considered the utmost concern.
Regarding the complex field of disease modelling, biofabricated constructs and OOC platforms significantly complement each other to develop an understanding of disease mechanisms and precision medicine. Indeed, biofabricated and OOC disease models are not a need or a unique tool for all diseases, and currently, these platforms are finding promising applications in some medical fields, such as cancer, neurodegenerative and rare diseases, to assure effective treatments and bench-to-bedside transition of these treatments for patient subgroups with similar epigenetic profiles. For instance, tumor-on-chip platforms have taken particular attention in pediatric oncology, where they could be used to test tissues harvested from patients as an alternative to risky first-in-human studies within pediatric populations. Alternatively, on the rare disease front, pioneering models for rare disorders, e.g., Barth syndrome, progeria, Timothy syndrome, and hereditary hemorrhagic telangiectasia, cystic fibrosis, have been suggested for clinical trial implementation and therapeutic development (Wang et al., 2014; Hinson et al., 2015; Atchison et al., 2017; Ribas et al., 2017; Shik Mun et al., 2019). Moreover, since the outbreak of the COVID-19 pandemic, such platforms have also been exploited to understand the effects of the SARS-CoV-2 virus on respiratory tissues, underlining once again their great potential (Si et al., 2021; Zhang et al., 2021). Overall, biofabricated and OOC disease modeling platforms are still facing some challenges with their integration into clinical applications. From the biomimicry perspective, embodying immune and endocrine responses in such platforms is still a significantly complex and daunting task. Moreover, physiochemically relevant disease modeling platforms that are standardized and user-friendly have not been fully achieved yet, apart from few commercialized examples (Nawroth et al., 2020; Apostolou et al., 2021; Fengler et al., 2021; Vormann et al., 2021).
In drug development, surely, OOC platforms and biofabricated tissues cannot be considered as an exact mimic of the entire in vivo effects but as a more predictive and biologically relevant assay for the drug discovery cascade. More specifically, to understand and evaluate the efficacy of the drugs and their ADME profile, developing multi-organ systems—i.e., miniaturized chips embedding various organ/tissue-specific compartments—has become a necessary requirement. The main challenges in developing such multi-organ platforms entail optimizing universal cell culture medium formulations to simultaneously support all organ/tissue compartments, acquiring renewable cell sources, and developing biomimetic perfusion of the nutrients/oxygen throughout the whole system. Additionally, to achieve a structurally and functionally validated multi-organ-on-a-chips, a thorough assessments in proper scaling of organ-on-a-chip models, implementation of real-time evaluation, and development of chemical, mechanical, and electrical cues for missing organ systems stand as crucial requirements.
Finally, the combined use—although off-chip—of omic approaches with OOCs has led to new insights in providing realistic physiological models and significantly reducing the costs for the preparation of molecular libraries for single-cell approaches by reducing the reaction volumes to the scale of nano/picoliters. These innovative systems that focus on cellular output have significantly improved the performance of single-cell omic studies, enabling the parallel processing of thousands of cells cultured in such a tailored microenvironments.
All in all, OOC platforms and biofabrication systems are largely considered the way-to-go strategies to develop advance tissue/organ models and, most likely, will continue to play a key role in the next decades.
Undoubtedly, in the last few years, biofabrication and organ-on-a-chip strategies have had a significant, positive impact on biomedical research through the fabrication of increasingly refined 3D tissue/organ models. While these first successes are a matter of fact, it is not trivial to foresee how these technologies will further develop over the next one or two decades and how their outputs will be eventually translated from the realm of research to meaningful clinical applications. Nevertheless, these systems are nowadays affected by some common limitations which should be necessarily addressed in the near future. Given the inherent complexity of advanced, in vitro tissue model manufacturing, these limits do not have clear boundaries, being often intertwined one to the other.
First, the variability of the experiments among different batches and systems should be drastically reduced through a thorough standardization of the whole process. Both biofabrication and organ-on-a-chip systems, in fact, lack any specific guideline that should help researchers in developing regulatory-approvable products and, only recently have researchers started to define common roadmaps to address standardization issues (Mastrangeli et al., 2019a; Sun et al., 2020). Such standardization should affect all the aspects of these biotech strategies, including material and cell selection, isolation and purification, bio-construct manufacturing, processing and characterizations, culturing protocols, and bio-construct post-processing. The first step towards this direction has been lately made by the United States Food and Drug Administration (FDA) in its predictive toxicology roadmap where organ-on-a-chip models have been identified as new promising approaches to develop innovative toxicology methods and have been adopted in FDA laboratories to assess their capacities (Food and Drug Administration, 2017). As pointed out in the FDA document, the acceptance of any new methods will require sufficient convincing data as well as continuous dialogue and feedback among all relevant stakeholders from development to implementation, including, in particular, validation and acceptance by regulatory authorities. Reasonably, the implementation of organ-on-a-chip platforms by a regulatory agency should promote and accelerate standardization of this technology at different levels.
The second challenge that should be addressed in the near future consists of the increase in the reliability and robustness of the manufactured models. These aspects represent a key point for the adoption of these technologies in clinically relevant contexts. To improve tissue model reliability and robustness, a more thorough and deeper characterization of the inputs, outputs, and models themselves and their cross-impacts will be needed. This will require the simultaneous analysis of tens up to hundreds of variables/parameters at the same time with the generation of extremely large volumes of data. Performing data analytics of such voluminous data sets is generally complex, and new in silico tools should be specifically developed in collaboration with mathematicians, statisticians, and bioinformaticians. Big data analysis, deep learning, and artificial intelligence methods have already been effectively applied and implemented in many industrial and research fields and technologies, and it is reasonable to foresee that soon they might also be integrated with the most advanced biotech systems for organ/tissue modeling (Galan et al., 2020; Xu and Ye, 2020; Zhou et al., 2020). Of note, generating these datasets, at least in the near future, is expected to be time-consuming and costly and, therefore, impractical at the single laboratory or small start-up levels. A possible solution to this economic problem could be establishing research clusters/networks where scientists could tackle this challenge through a common effort.
The third issue that affects both current biofabrication and organ-on-a-chip models is their oversimplified nature and thus poor functional behavior, primarily due to persisting technological limitations and lack of fundamental knowledge. By definition, a model is a simplified version of the actual tissue/organ found in vivo. However, as demonstrated in numerous studies, a proper recapitulation of the tissue microenvironment is essential to obtain in vitro functionalities comparable to native tissues/organs. To this end, the main focus of the research community had been to recreate static microenvironments, which are still far from dynamic native ones. In this regard, recently, the integration of 3D printing technology and smart shape-memory materials has created a great wave of enthusiasm for developing physiologically more relevant tissue models, establishing a new research field termed 4D printing. With the introduction of a fourth dimension, i.e., ‘time,’ the 4D printing technology allows both spatial and temporal control over the fabricated constructs, better mimicking dynamic tissue responses towards certain natural stimuli (Tamay et al., 2019; Hann et al., 2020). Though this technology is still in its infancy, it has already succeeded in placing a landmark in the sphere of biomedical research, holding promising prospects for further advancements in the near future.
Besides vascularization, innervation, spatially defined cell distribution, ECM composition, biochemical and electro-mechanical stimulation, and inter-organ cross-talks still remain as pertinent challenges and are hardly observed in the currently available biofabricated or organ-on-a-chip models. In order to overcome these limits, a great deal of work will surely be needed in different research domains, ranging from biomaterials and biochemistry to bioengineering, cell and developmental biology, and bioinformatics.
Finally, it is foreseen for the near future convergence of these two sets of technologies that would eventually enable the manufacturing of cellularized OOC systems using additive manufacturing platforms (Knowlton et al., 2016; Yi et al., 2017; Mi et al., 2018a; Carvalho et al., 2021). To date, this research area—i.e., 3D printing of microfluidic devices—is growing at an impressive pace following the tremendous technological advancements in 3D printing, which enable to simultaneously manipulate multiple materials (bio- and non-bio materials) at high printing resolution. Specifically, researchers have developed few strategies to combine OOC and 3D bioprinting: 1) the use of 3D printing systems to manufacture structures for OOC replica molding, 2) bioprinting of micro-tissues within pre-fabricated OOC, and 3) one-step fabrication of the cellularized OOC. The convergence of these technologies is expected to merge their intrinsic advantages, thus providing more customizable and controllable microenvironments for the bioprinted tissues, which should eventually lead to more realistic tissue/organ models. However, there is still some work ahead—especially for the more attractive case of one-step fabrication of cellularized OOC—due to some material (for instance, the optical properties of the printed OOC are still unsatisfactory) and technological limitations.
In conclusion, biofabrication and organ-on-a-chip methods represent the most promising technologies nowadays available to advance biomedical research and clearly will play a key role in academic and industrial research during the next two decades. As pointed out in this review, these technologies have already found applications in almost all branches of biomedical research, being also the catalyst for the establishment of as many promising start-up companies. Roadmaps have been set to guide the future development of both research fields, and, hopefully, regulatory agencies worldwide will soon accelerate the redaction of guidelines to implement these systems into more relevant clinical scenarios.
NC, DP and MC have structured the concept of the manuscript and have investigated the related current literature discussed in this manuscript. Authors NC, DP, FM, EF, AP, MV, CG and MC have contributed to the writing of the original manuscript. MC and DP have equally contributed to the creation and presentation of visual art in the manuscript. All authors participated in reviewing and editing the manuscript. MC, the corresponding author, has supervised the overall work for the manuscript.
This study was supported by the National Science Centre—Poland (NCN) within the POLONEZ 3 fellowship number 2016/23/P/NZ1/03604 which has received funding from the European Union’s Horizon 2020 research and innovation programme under the Marie Skłodowska-Curie grant agreement No. 665778. This study is also supported by the National Science Centre Poland (NCN) within PRELUDIUM 19 Project No. 2020/37/N/ST5/03272 to NC. This work was also supported by the National Science Centre (NCN) under the Grant No.: UMO-2016/23/Z/ST8/04375 (BIOMEMBRANE project (M-ERA.NET2 project 4246)).
The authors declare that the research was conducted in the absence of any commercial or financial relationships that could be construed as a potential conflict of interest.
All claims expressed in this article are solely those of the authors and do not necessarily represent those of their affiliated organizations, or those of the publisher, the editors and the reviewers. Any product that may be evaluated in this article, or claim that may be made by its manufacturer, is not guaranteed or endorsed by the publisher.
Achberger, K., Probst, C., Haderspeck, J., Bolz, S., Rogal, J., Chuchuy, J., et al. (2019). Merging Organoid and Organ-On-A-Chip Technology to Generate Complex Multi-Layer Tissue Models in a Human Retina-On-A-Chip Platform. Elife 8, e46188. doi:10.7554/eLife.46188
Afshar Bakooshli, M., Lippmann, E. S., Mulcahy, B., Iyer, N., Nguyen, C. T., Tung, K., et al. (2019). A 3d Culture Model of Innervated Human Skeletal Muscle Enables Studies of the Adult Neuromuscular junction. Elife 8, 1–29. doi:10.7554/eLife.44530
Agarwal, P., Wang, H., Sun, M., Xu, J., Zhao, S., Liu, Z., et al. (2017). Microfluidics Enabled Bottom-Up Engineering of 3D Vascularized Tumor for Drug Discovery. ACS Nano 11, 6691–6702. doi:10.1021/acsnano.7b00824
Agrawal, G., Aung, A., and Varghese, S. (2017). Skeletal Muscle-On-A-Chip: an In Vitro Model to Evaluate Tissue Formation and Injury. Lab. Chip 17, 3447–3461. doi:10.1039/C7LC00512A
Ahadian, S., Civitarese, R., Bannerman, D., Mohammadi, M. H., Lu, R., Wang, E., et al. (2018). Organ-On-A-Chip Platforms: A Convergence of Advanced Materials, Cells, and Microscale Technologies. Adv. Healthc. Mater. 7, 1–53. doi:10.1002/adhm.201800734
Aleman, J., George, S. K., Herberg, S., Devarasetty, M., Porada, C. D., Skardal, A., et al. (2019). Deconstructed Microfluidic Bone Marrow On‐A‐Chip to Study Normal and Malignant Hemopoietic Cell-Niche Interactions. Small 15, 1902971. doi:10.1002/smll.201902971
Ali, M., Pr, A. K., Yoo, J. J., Zahran, F., Atala, A., and Lee, S. J. (2019). A Photo‐Crosslinkable Kidney ECM‐Derived Bioink Accelerates Renal Tissue Formation. Adv. Healthc. Mater. 8, 1800992. doi:10.1002/adhm.201800992
Amin, R., Knowlton, S., Hart, A., Yenilmez, B., Ghaderinezhad, F., Katebifar, S., et al. (2016). 3D-printed Microfluidic Devices. Biofabrication 8, 022001. doi:10.1088/1758-5090/8/2/022001
Angelopoulos, I., Allenby, M. C., Lim, M., and Zamorano, M. (2020). Engineering Inkjet Bioprinting Processes toward Translational Therapies. Biotechnol. Bioeng. 117, 272–284. doi:10.1002/bit.27176
Apostolou, A., Panchakshari, R. A., Banerjee, A., Manatakis, D. V., Paraskevopoulou, M. D., Luc, R., et al. (2021). A Novel Microphysiological colon Platform to Decipher Mechanisms Driving Human Intestinal Permeability. Cell. Mol. Gastroenterol. Hepatol. S2352-345X (21), 00145–154. doi:10.1016/j.jcmgh.2021.07.004
Ashley, E. A. (2016). Towards Precision Medicine. Nat. Rev. Genet. 17, 507–522. doi:10.1038/nrg.2016.86
Asif, H. M., Sultana, S., Ahmed, S., Akhtar, N., and Tariq, M. (2016). HER-2 Positive Breast Cancer - a Mini-Review. Asian Pac. J. Cancer Prev. 17, 1609–1615. doi:10.7314/apjcp.2016.17.4.1609
Atchison, L., Zhang, H., Cao, K., and Truskey, G. A. (2017). A Tissue Engineered Blood Vessel Model of Hutchinson-Gilford Progeria Syndrome Using Human iPSC-Derived Smooth Muscle Cells. Sci. Rep. 7, 1–12. doi:10.1038/s41598-017-08632-4
Ayan, B., Heo, D. N., Zhang, Z., Dey, M., Povilianskas, A., Drapaca, C., et al. (2020). Aspiration-assisted Bioprinting for Precise Positioning of Biologics. Sci. Adv. 6, eaaw5111. doi:10.1126/sciadv.aaw5111
Ayoub, S., Ferrari, G., Gorman, R. C., Gorman, J. H., Schoen, F. J., and Sacks, M. S. (2016). Heart Valve Biomechanics and Underlying Mechanobiology. Compr. Physiol. 6, 1743–1780. doi:10.1002/cphy.c150048
Bailey, J., and Balls, M. (2019). Recent Efforts to Elucidate the Scientific Validity of Animal-Based Drug Tests by the Pharmaceutical Industry, Pro-testing Lobby Groups, and Animal Welfare Organisations. BMC Med. Ethics 20, 1–7. doi:10.1186/s12910-019-0352-3
Balasubramaniam, S., Beaver, J. A., Horton, S., Fernandes, L. L., Tang, S., Horne, H. N., et al. (2017). FDA Approval Summary: Rucaparib for the Treatment of Patients with Deleterious BRCA Mutation-Associated Advanced Ovarian Cancer. Clin. Cancer Res. 23, 7165–7170. doi:10.1158/1078-0432.ccr-17-1337
Beaurivage, C., Naumovska, E., Chang, Y., Elstak, E., Nicolas, A., Wouters, H., et al. (2019). Development of a Gut-On-A-Chip Model for High Throughput Disease Modeling and Drug Discovery. Ijms 20, 5661. doi:10.3390/ijms20225661
Benam, K. H., Villenave, R., Lucchesi, C., Varone, A., Hubeau, C., Lee, H.-H., et al. (2016). Small Airway-On-A-Chip Enables Analysis of Human Lung Inflammation and Drug Responses In Vitro. Nat. Methods 13, 151–157. doi:10.1038/nmeth.3697
Bernal, P. N., Delrot, P., Loterie, D., Li, Y., Malda, J., Moser, C., et al. (2019). Volumetric Bioprinting of Complex Living‐Tissue Constructs within Seconds. Adv. Mater. 31, 1904209. doi:10.1002/adma.201904209
Bersini, S., Jeon, J. S., Dubini, G., Arrigoni, C., Chung, S., Charest, J. L., et al. (2014). A Microfluidic 3D In Vitro Model for Specificity of Breast Cancer Metastasis to Bone. Biomaterials 35, 2454–2461. doi:10.1016/j.biomaterials.2013.11.050
Berthiaume, F., Maguire, T. J., and Yarmush, M. L. (2011). Tissue Engineering and Regenerative Medicine: History, Progress, and Challenges. Annu. Rev. Chem. Biomol. Eng. 2, 403–430. doi:10.1146/annurev-chembioeng-061010-114257
Bhagat, A. A. S., Jothimuthu, P., and Papautsky, I. (2007). Photodefinable Polydimethylsiloxane (PDMS) for Rapid Lab-On-A-Chip Prototyping. Lab. Chip 7, 1192–1197. doi:10.1039/b704946c
Bhatia, S. N., and Ingber, D. E. (2014). Microfluidic Organs-On-Chips. Nat. Biotechnol. 32, 760–772. doi:10.1038/nbt.2989
Bodein, A., Scott-Boyer, M.-P., Perin, O., Le Cao, K.-A., and Droit, A. (2020). Interpretation of Network-Based Integration from Multi-Omics Longitudinal Data. bioRxiv.
Brassard, J. A., Nikolaev, M., Hübscher, T., Hofer, M., and Lutolf, M. P. (2021). Recapitulating Macro-Scale Tissue Self-Organization through Organoid Bioprinting. Nat. Mater. 20, 22–29. doi:10.1038/s41563-020-00803-5
Brown, P. T., Handorf, A. M., Jeon, W. B., and Li, W. J. (2013). Stem Cell-Based Tissue Engineering Approaches for Musculoskeletal Regeneration. Cpd 19, 3429–3445. doi:10.2174/13816128113199990350
Bussard, K. M., Mutkus, L., Stumpf, K., Gomez-Manzano, C., and Marini, F. C. (2016). Tumor-associated Stromal Cells as Key Contributors to the Tumor Microenvironment. Breast Cancer Res. 18, 84. doi:10.1186/s13058-016-0740-2
Cabon, L., Petropolis, D., Tien-Street, W., Ng, P. R., Grant, H. S., Karalis, K., et al. (2021). Human Immunocompetent Organs-On-Chips Platforms Allow Safety Profiling of Tumor-Targeted T-Cell Bispecific Antibodies. bioRxiv.
Campbell, P. G., and Weiss, L. E. (2007). Tissue Engineering with the Aid of Inkjet Printers. Expert Opin. Biol. Ther. 7, 1123–1127. doi:10.1517/14712598.7.8.1123
Canzler, S., Schor, J., Busch, W., Schubert, K., Rolle-Kampczyk, U. E., Seitz, H., et al. (2020). Prospects and Challenges of Multi-Omics Data Integration in Toxicology. Arch. Toxicol. 94 (2), 371–388. doi:10.1007/s00204-020-02656-y
Carvalho, V., Gonçalves, I., Lage, T., Rodrigues, R. O., Minas, G., Teixeira, S. F. C. F., et al. (2021). 3D Printing Techniques and Their Applications to Organ-On-A-Chip Platforms: A Systematic Review. Sensors 21, 3304. doi:10.3390/s21093304
Chappell, L., Russell, A. J. C., and Voet, T. (2018). Single-Cell (Multi)omics Technologies. Annu. Rev. Genom. Hum. Genet. 19, 15–41. doi:10.1146/annurev-genom-091416-035324
Chen, X., Zhang, Y. S., Zhang, X., and Liu, C. (2021). Organ-on-a-chip Platforms for Accelerating the Evaluation of Nanomedicine. Bioactive Mater. 6, 1012–1027. doi:10.1016/j.bioactmat.2020.09.022
Chen, Y., Gao, D., Wang, Y., Lin, S., and Jiang, Y. (2018). A Novel 3D Breast-Cancer-On-Chip Platform for Therapeutic Evaluation of Drug Delivery Systems. Analytica Chim. Acta 1036, 97–106. doi:10.1016/j.aca.2018.06.038
Chen, Y., Zhang, J., Liu, X., Wang, S., Tao, J., Huang, Y., et al. (2020). Noninvasive In Vivo 3D Bioprinting. Sci. Adv. 6, eaba7406. doi:10.1126/sciadv.aba7406
Choi, Y., Hyun, E., Seo, J., Blundell, C., Kim, H. C., Lee, E., et al. (2015). A Microengineered Pathophysiological Model of Early-Stage Breast Cancer. Lab. Chip 15, 3350–3357. doi:10.1039/c5lc00514k
Chou, D. B., Frismantas, V., Milton, Y., David, R., Pop-Damkov, P., Ferguson, D., et al. (2020). On-chip Recapitulation of Clinical Bone Marrow Toxicities and Patient-specific Pathophysiology. Nat. Biomed. Eng. 4, 394–406. doi:10.1038/s41551-019-0495-z
Chung, M., Lee, S., Lee, B. J., Son, K., Jeon, N. L., and Kim, J. H. (2018). Wet-AMD on a Chip: Modeling Outer Blood-Retinal Barrier In Vitro. Adv. Healthc. Mater. 7, 1700028. doi:10.1002/adhm.201700028
Costantini, M., Testa, S., Fornetti, E., Fuoco, C., Sanchez Riera, C., Nie, M., et al. (2021). Biofabricating Murine and Human Myo-Substitutes for Rapid Volumetric Muscle Loss Restoration. EMBO Mol. Med. 13, e12778. doi:10.15252/emmm.202012778
Costantini, M., Testa, S., Mozetic, P., Barbetta, A., Fuoco, C., Fornetti, E., et al. (2017). Microfluidic-enhanced 3D Bioprinting of Aligned Myoblast-Laden Hydrogels Leads to Functionally Organized Myofibers In Vitro and In Vivo. Biomaterials 131, 98–110. doi:10.1016/j.biomaterials.2017.03.026
Cubo, N., Garcia, M., del Cañizo, J. F., Velasco, D., and Jorcano, J. L. (2016). 3D Bioprinting of Functional Human Skin: Production and In Vivo Analysis. Biofabrication 9, 015006. doi:10.1088/1758-5090/9/1/015006
Cui, X., Dean, D., Ruggeri, Z. M., and Boland, T. (2010). Cell Damage Evaluation of thermal Inkjet Printed Chinese Hamster Ovary Cells. Biotechnol. Bioeng. 106, 963–969. doi:10.1002/bit.22762
Dalton, P. D., Woodfield, T. B. F., Mironov, V., and Groll, J. (2020). Advances in Hybrid Fabrication toward Hierarchical Tissue Constructs. Adv. Sci. 7, 1902953. doi:10.1002/advs.201902953
de Mello, C. P. P., Rumsey, J., Slaughter, V., and Hickman, J. J. (2019). A Human-On-A-Chip Approach to Tackling Rare Diseases. Drug Discov. Today 24, 2139–2151. doi:10.1016/j.drudis.2019.08.001
De Santis, M. M., Alsafadi, H. N., Tas, S., Bölükbas, D. A., Prithiviraj, S., Da Silva, I. A. N., et al. (2021). Extracellular‐Matrix‐Reinforced Bioinks for 3D Bioprinting Human Tissue. Adv. Mater. 33, 2005476. doi:10.1002/adma.202005476
Del Rio, D., Zimetti, F., Caffarra, P., Tassotti, M., Bernini, F., Brighenti, F., et al. (2017). The Gut Microbial Metabolite Trimethylamine-N-Oxide Is Present in Human Cerebrospinal Fluid. Nutrients 9, 1053. doi:10.3390/nu9101053
Derby, B. (2010). Inkjet Printing of Functional and Structural Materials: Fluid Property Requirements, Feature Stability, and Resolution. Annu. Rev. Mater. Res. 40, 395–414. doi:10.1146/annurev-matsci-070909-104502
Dhariwala, B., Hunt, E., and Boland, T. (2004). Rapid Prototyping of Tissue-Engineering Constructs, Using Photopolymerizable Hydrogels and Stereolithography. Tissue Eng. 10, 1316–1322. doi:10.1089/1076327042500256
Douville, N. J., Zamankhan, P., Tung, Y.-C., Li, R., Vaughan, B. L., Tai, C.-F., et al. (2011). Combination of Fluid and Solid Mechanical Stresses Contribute to Cell Death and Detachment in a Microfluidic Alveolar Model. Lab. Chip 11, 609–619. doi:10.1039/c0lc00251h
Emmert-Buck, M. R., Bonner, R. F., Smith, P. D., Chuaqui, R. F., Zhuang, Z., Goldstein, S. R., et al. (1996). Laser Capture Microdissection. Science 274, 998–1001. doi:10.1126/science.274.5289.998
Esch, E. W., Bahinski, A., and Huh, D. (2015). Organs-on-chips at the Frontiers of Drug Discovery. Nat. Rev. Drug Discov. 14, 248–260. doi:10.1038/nrd4539
Esch, M. B., King, T. L., and Shuler, M. L. (2011). The Role of Body-On-A-Chip Devices in Drug and Toxicity Studies. Annu. Rev. Biomed. Eng. 13, 55–72. doi:10.1146/annurev-bioeng-071910-124629
Fan, H. C., Wang, J., Potanina, A., and Quake, S. R. (2011). Whole-genome Molecular Haplotyping of Single Cells. Nat. Biotechnol. 29, 51–57. doi:10.1038/nbt.1739
Fantuzzo, J. A., Mirabella, V. R., Hart, R. P., Pang, Z. P., and Zahn, J. D. (2018). “Development of a High-Throughput Micro-neurocircuitry Platform for Drug Screening Studies,” in 22nd International Conference on Miniaturized Systems for Chemistry and Life Sciences, MicroTAS 2018 (Chemical and Biological Microsystems Society), 287–290.
Fengler, S., Kurkowsky, B., Kaushalya, S. K., Roth, W., Denner, P., and Fava, E. (2021). Human iPSC-Derived Brain Endothelial Microvessels in a Multi-Well Format Enable Permeability Screens of Anti-inflammatory Drugs. bioRxiv.
Foresti, D., Kroll, K. T., Amissah, R., Sillani, F., Homan, K. A., Poulikakos, D., et al. (2018). Acoustophoretic Printing. Sci. Adv. 4, eaat1659. doi:10.1126/sciadv.aat1659
Franco, C., and Gerhardt, H. (2012). Blood Vessels on a Chip. Nature 488, 465–466. doi:10.1038/488465a
Friedman, A. A., Letai, A., Fisher, D. E., and Flaherty, K. T. (2015). Precision Medicine for Cancer with Next-Generation Functional Diagnostics. Nat. Rev. Cancer 15, 747–756. doi:10.1038/nrc4015
Galan, E. A., Zhao, H., Wang, X., Dai, Q., Huck, W. T. S., and Ma, S. (2020). Intelligent Microfluidics: The Convergence of Machine Learning and Microfluidics in Materials Science and Biomedicine. Matter 3, 1893–1922. doi:10.1016/j.matt.2020.08.034
Gao, Q., He, Y., Fu, J.-z., Zhong, A., and Liu, L. (2015). Coaxial Nozzle-Assisted 3D Bioprinting with Built-In Microchannels for Nutrients Delivery. Biomaterials 61, 203–215. doi:10.1016/j.biomaterials.2015.05.031
Gjorevski, N., Avignon, B., Gérard, R., Cabon, L., Roth, A. B., Bscheider, M., et al. (2020). Neutrophilic Infiltration in Organ-On-A-Chip Model of Tissue Inflammation. Lab. Chip 20, 3365–3374. doi:10.1039/d0lc00417k
Gori, M., Giannitelli, S. M., Torre, M., Mozetic, P., Abbruzzese, F., Trombetta, M., et al. (2020). Biofabrication of Hepatic Constructs by 3D Bioprinting of a Cell-Laden Thermogel: An Effective Tool to Assess Drug-Induced Hepatotoxic Response. Adv. Healthc. Mater. 9 (21), e2001163. doi:10.1002/adhm.202001163
Grigoryan, B., Sazer, D. W., Avila, A., Albritton, J. L., Padhye, A., Ta, A. H., et al. (2021). Development, Characterization, and Applications of Multi-Material Stereolithography Bioprinting. Sci. Rep. 11, 3171–3213. doi:10.1038/s41598-021-82102-w
Grigoryan, B., Paulsen, S. J., Corbett, D. C., Sazer, D. W., Fortin, C. L., Zaita, A. J., et al. (2019). Multivascular Networks and Functional Intravascular Topologies within Biocompatible Hydrogels. Science 364, 458–464. doi:10.1126/science.aav9750
Groll, J., Boland, T., Blunk, T., Burdick, J. A., Cho, D.-W., Dalton, P. D., et al. (2016). Biofabrication: Reappraising the Definition of an Evolving Field. Biofabrication 8, 013001. doi:10.1088/1758-5090/8/1/013001
Grolman, J. M., Zhang, D., Smith, A. M., Moore, J. S., and Kilian, K. A. (2015). Rapid 3D Extrusion of Synthetic Tumor Microenvironments. Adv. Mater. 27, 5512–5517. doi:10.1002/adma.201501729
Grosberg, A., Alford, P. W., McCain, M. L., and Parker, K. K. (2011). Ensembles of Engineered Cardiac Tissues for Physiological and Pharmacological Study: Heart on a Chip. Lab. Chip 11, 4165–4173. doi:10.1039/c1lc20557a
Grosberg, A., Nesmith, A. P., Goss, J. A., Brigham, M. D., McCain, M. L., and Parker, K. K. (2012). Muscle on a Chip: In Vitro Contractility Assays for Smooth and Striated Muscle. J. Pharmacol. Toxicol. Methods 65, 126–135. doi:10.1016/j.vascn.2012.04.001
Guarino, V., and Ambrosio, L. (2010). Temperature-driven Processing Techniques for Manufacturing Fully Interconnected Porous Scaffolds in Bone Tissue Engineering. Proc. Inst. Mech. Eng. H 224, 1389–1400. doi:10.1243/09544119JEIM744
Gudapati, H., Dey, M., and Ozbolat, I. (2016). A Comprehensive Review on Droplet-Based Bioprinting: Past, Present and Future. Biomaterials 102, 20–42. doi:10.1016/j.biomaterials.2016.06.012
Gungor-Ozkerim, P. S., Inci, I., Zhang, Y. S., Khademhosseini, A., and Dokmeci, M. R. (2018). Bioinks for 3D Bioprinting: an Overview. Biomater. Sci. 6, 915–946. doi:10.1039/c7bm00765e
Hann, S. Y., Cui, H., Nowicki, M., and Zhang, L. G. (2020). 4D Printing Soft Robotics for Biomedical Applications. Additive Manufacturing 36, 101567. doi:10.1016/j.addma.2020.101567
Heinrich, M. A., Bansal, R., Lammers, T., Zhang, Y. S., Michel Schiffelers, R., and Prakash, J. (2019). 3D‐Bioprinted Mini‐Brain: A Glioblastoma Model to Study Cellular Interactions and Therapeutics. Adv. Mater. 31, 1806590. doi:10.1002/adma.201806590
Hewes, S., Wong, A. D., and Searson, P. C. (2017). Bioprinting Microvessels Using an Inkjet Printer. Bioprinting 7, 14–18. doi:10.1016/j.bprint.2017.05.002
Hinson, J. T., Chopra, A., Nafissi, N., Polacheck, W. J., Benson, C. C., Swist, S., et al. (2015). Titin Mutations in iPS Cells Define Sarcomere Insufficiency as a Cause of Dilated Cardiomyopathy. Science 349, 982–986. doi:10.1126/science.aaa5458
Hinton, T. J., Jallerat, Q., Palchesko, R. N., Park, J. H., Grodzicki, M. S., Shue, H.-J., et al. (2015). Three-dimensional Printing of Complex Biological Structures by Freeform Reversible Embedding of Suspended Hydrogels. Sci. Adv. 1, e1500758. doi:10.1126/sciadv.1500758
Hoffman, T., Khademhosseini, A., and Langer, R. (2019). Chasing the Paradigm: Clinical Translation of 25 Years of Tissue Engineering. Tissue Eng. A 25, 679–687. doi:10.1089/ten.tea.2019.0032
Hollister, S. J. (2005). Porous Scaffold Design for Tissue Engineering. Nat. Mater 4 (7), 518–524. doi:10.1038/nmat1421
Homan, K. A., Kolesky, D. B., Skylar-Scott, M. A., Herrmann, J., Obuobi, H., Moisan, A., et al. (2016). Bioprinting of 3D Convoluted Renal Proximal Tubules on Perfusable Chips. Sci. Rep. 6, 34845. doi:10.1038/srep34845
Hong, N., Yang, G.-H., Lee, J., and Kim, G. (2018). 3D Bioprinting and its In Vivo Applications. J. Biomed. Mater. Res. 106, 444–459. doi:10.1002/jbm.b.33826
Hongbin, Y., Guangya, Z., Siong, C. F., Shouhua, W., and Feiwen, L. (2009). Novel Polydimethylsiloxane (PDMS) Based Microchannel Fabrication Method for Lab-On-A-Chip Application. Sensors Actuators B: Chem. 137, 754–761. doi:10.1016/j.snb.2008.11.035
Horton, R. E., Yadid, M., McCain, M. L., Sheehy, S. P., Pasqualini, F. S., Park, S.-J., et al. (2016). Angiotensin II Induced Cardiac Dysfunction on a Chip. PLoS One 11, e0146415. doi:10.1371/journal.pone.0146415
Horváth, L., Umehara, Y., Jud, C., Blank, F., Petri-fink, A., Rothen-rutishauser, B., et al. (2015). Engineering an In Vitro Air-Blood Barrier by 3D Bioprinting. Sci. Rep. 5, 1–8. doi:10.1038/srep07974
Hosny, A., Keating, S. J., Dilley, J. D., Ripley, B., Kelil, T., Pieper, S., et al. (2018). From Improved Diagnostics to Presurgical Planning: High-Resolution Functionally Graded Multimaterial 3D Printing of Biomedical Tomographic Data Sets. 3D Printing and Additive Manufacturing 5, 103–113. doi:10.1089/3dp.2017.0140
Hribar, K. C., Soman, P., Warner, J., Chung, P., and Chen, S. (2014). Light-assisted Direct-Write of 3D Functional Biomaterials. Lab. Chip 14, 268–275. doi:10.1039/C3LC50634G
Hu, P., Zhang, W., Xin, H., and Deng, G. (2016). Single Cell Isolation and Analysis. Front. Cel Dev. Biol. 4, 116. doi:10.3389/fcell.2016.00116
Hubbell, J. A. (1995). Biomaterials in Tissue Engineering. Nat. Biotechnol. 13, 565–576. doi:10.1038/nbt0695-565
Huh, D. (2015). A Human Breathing Lung-On-A-Chip. Ann. ATS 12, S42–S44. doi:10.1513/annalsats.201410-442mg
Huh, D., Matthews, B. D., Mammoto, A., Montoya-Zavala, M., Hsin, H. Y., and Ingber, D. E. (2010). Reconstituting Organ-Level Lung Functions on a Chip. Science 328, 1662–1668. doi:10.1126/science.1188302
Ingber, D. E. (2020). Is it Time for Reviewer 3 to Request Human Organ Chip Experiments Instead of Animal Validation Studies? Adv. Sci. 7, 2002030. doi:10.1002/advs.202002030
Ingber, D. E., Parker, K. K., Hamilton, G. A., and Bahinski, A. (2018). Organ Chips and Uses Thereof. IFI CLAIMS Patent Services.
Jackson, E. L., and Lu, H. (2016). Three-dimensional Models for Studying Development and Disease: Moving on from Organisms to Organs-On-A-Chip and Organoids. Integr. Biol. 8, 672–683. doi:10.1039/c6ib00039h
Jakab, K., Norotte, C., Damon, B., Marga, F., Neagu, A., Besch-Williford, C. L., et al. (2008). Tissue Engineering by Self-Assembly of Cells Printed into Topologically Defined Structures. Tissue Eng. Part A 14, 413–421. doi:10.1089/tea.2007.0173
Jalili-Firoozinezhad, S., Gazzaniga, F. S., Calamari, E. L., Camacho, D. M., Fadel, C. W., Bein, A., et al. (2019). A Complex Human Gut Microbiome Cultured in an Anaerobic Intestine-On-A-Chip. Nat. Biomed. Eng. 3, 520–531. doi:10.1038/s41551-019-0397-0
Jang, K.-J., Mehr, A. P., Hamilton, G. A., McPartlin, L. A., Chung, S., Suh, K.-Y., et al. (2013). Human Kidney Proximal Tubule-On-A-Chip for Drug Transport and Nephrotoxicity Assessment. Integr. Biol. 5, 1119–1129. doi:10.1039/c3ib40049b
Jang, K.-J., and Suh, K.-Y. (2010). A Multi-Layer Microfluidic Device for Efficient Culture and Analysis of Renal Tubular Cells. Lab. Chip 10, 36–42. doi:10.1039/b907515a
Jang, K. J., Otieno, M. A., Ronxhi, J., Lim, H. K., Ewart, L., Kodella, K. R., et al. (2019). Reproducing Human and Cross-Species Drug Toxicities Using a Liver-Chip. Sci. Transl. Med. 11, eaax5516. doi:10.1126/scitranslmed.aax5516
Jellali, R., Gilard, F., Pandolfi, V., Legendre, A., Fleury, M.-J., Paullier, P., et al. (2018). Metabolomics-on-a-chip Approach to Study Hepatotoxicity of DDT, Permethrin and Their Mixtures. J. Appl. Toxicol. 38, 1121–1134. doi:10.1002/jat.3624
Ji, S., and Guvendiren, M. (2021). Complex 3D Bioprinting Methods. APL Bioeng. 5, 11508. doi:10.1063/5.0034901
Jia, W., Gungor-Ozkerim, P. S., Zhang, Y. S., Yue, K., Zhu, K., Liu, W., et al. (2016). Direct 3D Bioprinting of Perfusable Vascular Constructs Using a Blend Bioink. Biomaterials 106, 58–68. doi:10.1016/j.biomaterials.2016.07.038
Jodat, Y. A., Kiaee, K., Vela Jarquin, D., De la Garza Hernández, R. L., Wang, T., Joshi, S., et al. (2020). A 3D‐Printed Hybrid Nasal Cartilage with Functional Electronic Olfaction. Adv. Sci. 7, 1901878. doi:10.1002/advs.201901878
Kamei, K.-i., Kato, Y., Hirai, Y., Ito, S., Satoh, J., Oka, A., et al. (2017). Integrated Heart/cancer on a Chip to Reproduce the Side Effects of Anti-cancer Drugs In Vitro. RSC Adv. 7, 36777–36786. doi:10.1039/c7ra07716e
Kang, D., Hong, G., An, S., Jang, I., Yun, W. S., Shim, J. H., et al. (2020). Bioprinting of Multiscaled Hepatic Lobules within a Highly Vascularized Construct. Small 16, 1905505. doi:10.1002/smll.201905505
Kang, H.-W., Lee, S. J., Ko, I. K., Kengla, C., Yoo, J. J., and Atala, A. (2016). A 3D Bioprinting System to Produce Human-Scale Tissue Constructs with Structural Integrity. Nat. Biotechnol. 34, 312–319. doi:10.1038/nbt.3413
Kasendra, M., Tovaglieri, A., Sontheimer-Phelps, A., Jalili-Firoozinezhad, S., Bein, A., Chalkiadaki, A., et al. (2018). Development of a Primary Human Small Intestine-On-A-Chip Using Biopsy-Derived Organoids. Sci. Rep. 8, 2871. doi:10.1038/s41598-018-21201-7
Kaul, H., and Ventikos, Y. (2015). On the Genealogy of Tissue Engineering and Regenerative Medicine. Tissue Eng. B: Rev. 21, 203–217. doi:10.1089/ten.teb.2014.0285
Kaur, R., and Chupp, G. (2019). Phenotypes and Endotypes of Adult Asthma: Moving toward Precision Medicine. J. Allergy Clin. Immunol. 144, 1–12. doi:10.1016/j.jaci.2019.05.031
Khanna, I. (2012). Drug Discovery in Pharmaceutical Industry: Productivity Challenges and Trends. Drug Discov. Today 17, 1088–1102. doi:10.1016/j.drudis.2012.05.007
Kim, B.-S., Baez, C. E., and Atala, A. (2000). Biomaterials for Tissue Engineering. World J. Urol. 18, 2–9. doi:10.1007/s003450050002
Kim, B. S., Gao, G., Kim, J. Y., and Cho, D. W. (2019). 3D Cell Printing of Perfusable Vascularized Human Skin Equivalent Composed of Epidermis, Dermis, and Hypodermis for Better Structural Recapitulation of Native Skin. Adv. Healthc. Mater. 8, 1801019. doi:10.1002/adhm.201801019
Kim, H. J., Li, H., Collins, J. J., and Ingber, D. E. (2016). Contributions of Microbiome and Mechanical Deformation to Intestinal Bacterial Overgrowth and Inflammation in a Human Gut-On-A-Chip. Proc. Natl. Acad. Sci. USA 113, E7–E15. doi:10.1073/pnas.1522193112
Kim, J. H., Kim, I., Seol, Y.-J., Ko, I. K., Yoo, J. J., Atala, A., et al. (2020). Neural Cell Integration into 3D Bioprinted Skeletal Muscle Constructs Accelerates Restoration of Muscle Function. Nat. Commun. 11, 1025. doi:10.1038/s41467-020-14930-9
Kim, J. H., Seol, Y.-J., Ko, I. K., Kang, H.-W., Lee, Y. K., Yoo, J. J., et al. (2018). 3D Bioprinted Human Skeletal Muscle Constructs for Muscle Function Restoration. Sci. Rep. 8, 12307. doi:10.1038/s41598-018-29968-5
Kingsley, D. M., Roberge, C. L., Rudkouskaya, A., Faulkner, D. E., Barroso, M., Intes, X., et al. (2019). Laser-based 3D Bioprinting for Spatial and Size Control of Tumor Spheroids and Embryoid Bodies. Acta Biomater. 95, 357–370. doi:10.1016/j.actbio.2019.02.014
Knight, E., and Przyborski, S. (2015). Advances in 3D Cell Culture Technologies Enabling Tissue-like Structures to Be Created In Vitro. J. Anat. 227, 746–756. doi:10.1111/joa.12257
Knowlton, S., and Tasoglu, S. (2016). A Bioprinted Liver-On-A-Chip for Drug Screening Applications. Trends Biotechnol. 34, 681–682. doi:10.1016/j.tibtech.2016.05.014
Knowlton, S., Yenilmez, B., and Tasoglu, S. (2016). Towards Single-step Biofabrication of Organs on a Chip via 3D Printing. Trends Biotechnol. 34, 685–688. doi:10.1016/j.tibtech.2016.06.005
Kolesky, D. B., Truby, R. L., Gladman, A. S., Busbee, T. A., Homan, K. A., and Lewis, J. A. (2014). 3D Bioprinting of Vascularized, Heterogeneous Cell-Laden Tissue Constructs. Adv. Mater. 26, 3124–3130. doi:10.1002/adma.201305506
Krumholz, H. M., Ross, J. S., Presler, A. H., and Egilman, D. S. (2007). What Have We Learnt from Vioxx? BMJ 334, 120–123. doi:10.1136/bmj.39024.487720.68
Kwon, O. H., Kikuchi, A., Yamato, M., Sakurai, Y., and Okano, T. (2000). Rapid Cell Sheet Detachment from Poly(N-Isopropylacrylamide)-Grafted Porous Cell Culture Membranes. J. Biomed. Mater. Res. 50, 82–89. doi:10.1002/(sici)1097-4636(200004)50:1<82:aid-jbm12>3.0.co;2-7
Landers, R., Hübner, U., Schmelzeisen, R., and Mülhaupt, R. (2002). Rapid Prototyping of Scaffolds Derived from Thermoreversible Hydrogels and Tailored for Applications in Tissue Engineering. Biomaterials 23, 4437–4447. doi:10.1016/S0142-9612(02)00139-4
Langer, R., and Vacanti, J. (1993). Tissue Engineering. Science 260, 920–926. doi:10.1126/science.8493529
Lasli, S., Kim, H. J., Lee, K., Suurmond, C. A. E., Goudie, M., Bandaru, P., et al. (2019). A Human Liver‐on‐a‐Chip Platform for Modeling Nonalcoholic Fatty Liver Disease. Adv. Biosys. 3, 1900104. doi:10.1002/adbi.201900104
Lee, A., Hudson, A. R., Shiwarski, D. J., Tashman, J. W., Hinton, T. J., Yerneni, S., et al. (2019a). 3D Bioprinting of Collagen to Rebuild Components of the Human Heart. Science 365, 482–487. doi:10.1126/science.aav9051
Lee, D. W., Ha, S. K., Choi, I., and Sung, J. H. (2017a). 3D Gut-Liver Chip with a PK Model for Prediction of First-Pass Metabolism. Biomed. Microdevices 19, 100. doi:10.1007/s10544-017-0242-8
Lee, H., Chae, S., Kim, J. Y., Han, W., Kim, J., Choi, Y., et al. (2019b). Cell-printed 3D Liver-On-A-Chip Possessing a Liver Microenvironment and Biliary System. Biofabrication 11, 025001. doi:10.1088/1758-5090/aaf9fa
Lee, J. H., Protze, S. I., Laksman, Z., Backx, P. H., and Keller, G. M. (2017b). Human Pluripotent Stem Cell-Derived Atrial and Ventricular Cardiomyocytes Develop from Distinct Mesoderm Populations. Cell Stem Cell 21, 179–194. doi:10.1016/j.stem.2017.07.003
Lee, K. H., Shin, S. J., Park, Y., and Lee, S.-H. (2009). Synthesis of Cell-Laden Alginate Hollow Fibers Using Microfluidic Chips and Microvascularized Tissue-Engineering Applications. Small 5, 1264–1268. doi:10.1002/smll.200801667
Lee, K. K., McCauley, H. A., Broda, T. R., Kofron, M. J., Wells, J. M., and Hong, C. I. (2018). Human Stomach-On-A-Chip with Luminal Flow and Peristaltic-like Motility. Lab. Chip 18, 3079–3085. doi:10.1039/c8lc00910d
Li, J., Parra-Cantu, C., Wang, Z., and Zhang, Y. S. (2020a). Improving Bioprinted Volumetric Tumor Microenvironments In Vitro. Trends Cancer 6 (9), 745–756. doi:10.1016/j.trecan.2020.06.002
Li, X., and Tian, T. (2018). Recent Advances in an Organ-On-A-Chip: Biomarker Analysis and Applications. Anal. Methods 10, 3122–3130. doi:10.1039/c8ay00970h
Li, Y. C., Jodat, Y. A., Samanipour, R., Zorzi, G., Zhu, K., Hirano, M., et al. (2020b). Toward a Neurospheroid Niche Model: Optimizing Embedded 3D Bioprinting for Fabrication of Neurospheroid Brain-like Co-culture Constructs. Biofabrication 13, 15014. doi:10.1088/1758-5090/abc1be
Lin, N. Y. C., Homan, K. A., Robinson, S. S., Kolesky, D. B., Duarte, N., Moisan, A., et al. (2019). Renal Reabsorption in 3D Vascularized Proximal Tubule Models. Proc. Natl. Acad. Sci. USA 116, 5399–5404. doi:10.1073/pnas.1815208116
Lind, J. U., Busbee, T. A., Valentine, A. D., Pasqualini, F. S., Yuan, H., Yadid, M., et al. (2017). Instrumented Cardiac Microphysiological Devices via Multimaterial Three-Dimensional Printing. Nat. Mater. 16, 303–308. doi:10.1038/nmat4782
Lozano, R., Stevens, L., Thompson, B. C., Gilmore, K. J., Gorkin, R., Stewart, E. M., et al. (2015). 3D Printing of Layered Brain-like Structures Using Peptide Modified Gellan Gum Substrates. Biomaterials 67, 264–273. doi:10.1016/j.biomaterials.2015.07.022
Luan, Q., Cahoon, S., Wu, A., Bale, S. S., Yarmush, M., and Bhushan, A. (2017). A Microfluidic In-Line ELISA for Measuring Secreted Protein under Perfusion. Biomed. Microdevices 19, 101. doi:10.1007/s10544-017-0244-6
Ma, X., Qu, X., Zhu, W., Li, Y.-S., Yuan, S., Zhang, H., et al. (2016). Deterministically Patterned Biomimetic Human iPSC-Derived Hepatic Model via Rapid 3D Bioprinting. Proc. Natl. Acad. Sci. USA 113, 2206–2211. doi:10.1073/pnas.1524510113
Madden, L. R., Nguyen, T. V., Garcia-Mojica, S., Shah, V., Le, A. V., Peier, A., et al. (2018). Bioprinted 3D Primary Human Intestinal Tissues Model Aspects of Native Physiology and ADME/Tox Functions. IScience 2, 156–167. doi:10.1016/j.isci.2018.03.015
Maharjan, S., Alva, J., Cámara, C., Rubio, A. G., Hernández, D., Delavaux, C., et al. (2021). Symbiotic Photosynthetic Oxygenation within 3D-Bioprinted Vascularized Tissues. Matter 4, 217–240. doi:10.1016/j.matt.2020.10.022
Maiullari, F., Costantini, M., Milan, M., Pace, V., Chirivì, M., Maiullari, S., et al. (2018). A Multi-Cellular 3D Bioprinting Approach for Vascularized Heart Tissue Engineering Based on HUVECs and iPSC-Derived Cardiomyocytes. Sci. Rep. 8, 13532. doi:10.1038/s41598-018-31848-x
Malda, J., Visser, J., Melchels, F. P., Jüngst, T., Hennink, W. E., Dhert, W. J. A., et al. (2013). 25th Anniversary Article: Engineering Hydrogels for Biofabrication. Adv. Mater. 25, 5011–5028. doi:10.1002/adma.201302042
Mao, S., Li, W., Zhang, Q., Zhang, W., Huang, Q., and Lin, J.-M. (2018). Cell Analysis on Chip-Mass Spectrometry. Trac Trends Anal. Chem. 107, 43–59. doi:10.1016/j.trac.2018.06.019
Markert, M. L., Gupton, S. E., and McCarthy, E. A. (2021). Experience with Cultured Thymus Tissue in 105 Children. J. Allergy Clin. Immunol. S0091-6749 (21), 01056–1063. doi:10.1016/j.jaci.2021.06.028
Marsano, A., Conficconi, C., Lemme, M., Occhetta, P., Gaudiello, E., Votta, E., et al. (2016). Beating Heart on a Chip: a Novel Microfluidic Platform to Generate Functional 3D Cardiac Microtissues. Lab. Chip 16, 599–610. doi:10.1039/c5lc01356a
Marturano-Kruik, A., Nava, M. M., Yeager, K., Chramiec, A., Hao, L., Robinson, S., et al. (2018). Human Bone Perivascular Niche-On-A-Chip for Studying Metastatic Colonization. Proc. Natl. Acad. Sci. USA 115, 1256–1261. doi:10.1073/pnas.1714282115
Marx, U., Akabane, T., Andersson, T. B., Baker, E., Beilmann, M., Beken, S., et al. (2020). Biology-inspired Microphysiological Systems to advance Patient Benefit and Animal Welfare in Drug Development. ALTEX 37 (3), 365–394. doi:10.14573/altex.2001241
Marx, U., and Ramme, A. (2020). Novel Multi-Organ-Chips Establishing Differentiation of Ipsc-Derived Cells into Organ Equivalents. Spreenhagen, Berlin: TissUse GmbH.
Masaeli, E., Forster, V., Picaud, S., Karamali, F., Nasr-Esfahani, M. H., and Marquette, C. (2020). Tissue Engineering of Retina through High Resolution 3-dimensional Inkjet Bioprinting. Biofabrication 12, 025006. doi:10.1088/1758-5090/ab4a20
Mastikhina, O., Moon, B.-U., Williams, K., Hatkar, R., Gustafson, D., Mourad, O., et al. (2020). Human Cardiac Fibrosis-On-A-Chip Model Recapitulates Disease Hallmarks and Can Serve as a Platform for Drug Testing. Biomaterials 233, 119741. doi:10.1016/j.biomaterials.2019.119741
Mastrangeli, M., Millet, S., Millet, S., and van den Eijnden-van Raaij, J. (2019b). Organ-on-chip in Development: Towards a Roadmap for Organs-On-Chip. Atlex 36 (4), 650–668. doi:10.14573/altex.1908271
Mastrangeli, M., Millet, S., Mummery, C., Loskill, P., Braeken, D., Eberle, W., et al. (2019a). Building Blocks for a European Organ-On-Chip Roadmap. Altex 36, 481–492. doi:10.14573/altex.1905221
Mencattini, A., De Ninno, A., Mancini, J., Businaro, L., Martinelli, E., Schiavoni, G., et al. (2020). High-throughput Analysis of Cell-Cell Crosstalk in Ad Hoc Designed Microfluidic Chips for Oncoimmunology Applications. Methods Enzymol. 632, 479–502. doi:10.1016/bs.mie.2019.06.012
Menden, K., Marouf, M., Oller, S., Dalmia, A., Magruder, D. S., Kloiber, K., et al. (2020). Deep Learning-Based Cell Composition Analysis from Tissue Expression Profiles. Sci. Adv. 6, eaba2619. doi:10.1126/sciadv.aba2619
Mi, S., Du, Z., Xu, Y., and Sun, W. (2018a). The Crossing and Integration between Microfluidic Technology and 3D Printing for Organ-On-Chips. J. Mater. Chem. B. 6, 6191–6206. doi:10.1039/c8tb01661e
Mi, S., Yi, X., Du, Z., Xu, Y., and Sun, W. (2018b). Construction of a Liver Sinusoid Based on the Laminar Flow on Chip and Self-Assembly of Endothelial Cells. Biofabrication 10, 025010. doi:10.1088/1758-5090/aaa97e
Miao, S., Cui, H., Nowicki, M., Xia, L., Zhou, X., Lee, S. J., et al. (2018). Stereolithographic 4D Bioprinting of Multiresponsive Architectures for Neural Engineering. Adv. Biosys. 2, 1800101. doi:10.1002/adbi.201800101
Miller, P. G., and Shuler, M. L. (2016). Design and Demonstration of a Pumpless 14 Compartment Microphysiological System. Biotechnol. Bioeng. 113, 2213–2227. doi:10.1002/bit.25989
Mirdamadi, E., Tashman, J. W., Shiwarski, D. J., Palchesko, R. N., and Feinberg, A. W. (2020). FRESH 3D Bioprinting a Full-Size Model of the Human Heart. ACS Biomater. Sci. Eng. 6, 6453–6459. doi:10.1021/acsbiomaterials.0c01133
Mironov, V., Boland, T., Trusk, T., Forgacs, G., and Markwald, R. R. (2003). Organ Printing: Computer-Aided Jet-Based 3D Tissue Engineering. Trends Biotechnol. 21, 157–161. doi:10.1016/S0167-7799(03)00033-7
Mironov, V., Visconti, R. P., Kasyanov, V., Forgacs, G., Drake, C. J., and Markwald, R. R. (2009). Organ Printing: Tissue Spheroids as Building Blocks. Biomaterials 30, 2164–2174. doi:10.1016/j.biomaterials.2008.12.084
Morimoto, Y., Hsiao, A. Y., and Takeuchi, S. (2015). Point-, Line-, and Plane-Shaped Cellular Constructs for 3D Tissue Assembly. Adv. Drug Deliv. Rev. 95, 29–39. doi:10.1016/j.addr.2015.09.003
Moroni, L., Boland, T., Burdick, J. A., De Maria, C., Derby, B., Forgacs, G., et al. (2018a). Biofabrication: a Guide to Technology and Terminology. Trends Biotechnol. 36, 384–402. doi:10.1016/j.tibtech.2017.10.015
Moroni, L., Burdick, J. A., Highley, C., Lee, S. J., Morimoto, Y., Takeuchi, S., et al. (2018b). Biofabrication Strategies for 3D In Vitro Models and Regenerative Medicine. Nat. Rev. Mater. 3, 21–37. doi:10.1038/s41578-018-0006-y
Moura Rosa, P., Gopalakrishnan, N., Ibrahim, H., Haug, M., and Halaas, Ø. (2016). The Intercell Dynamics of T Cells and Dendritic Cells in a Lymph Node-On-A-Chip Flow Device. Lab. Chip 16, 3728–3740. doi:10.1039/c6lc00702c
Murata, D., Arai, K., and Nakayama, K. (2020). Scaffold‐Free Bio‐3D Printing Using Spheroids as "Bio‐Inks" for Tissue (Re‐)Construction and Drug Response Tests. Adv. Healthc. Mater. 9 (15), 1901831. doi:10.1002/adhm.201901831
Nakao, Y., Kimura, H., Sakai, Y., and Fujii, T. (2011). Bile Canaliculi Formation by Aligning Rat Primary Hepatocytes in a Microfluidic Device. Biomicrofluidics 5, 22212. doi:10.1063/1.3580753
Nakayama, K. (2013). “In Vitro biofabrication of Tissues and Organs,” in Biofabrication (Elsevier), 1–21. doi:10.1016/b978-1-4557-2852-7.00001-9
Nawroth, J. C., Lucchesi, C., Cheng, D., Shukla, A., Ngyuen, J., Shroff, T., et al. (2020). A Microengineered Airway Lung Chip Models Key Features of Viral-Induced Exacerbation of Asthma. Am. J. Respir. Cel Mol. Biol. 63, 591–600. doi:10.1165/rcmb.2020-0010ma
Ndiaye, M. M., Ta, H. P., Chiappetta, G., and Vinh, J. (2020). On-chip Sample Preparation Using a ChipFilter Coupled to nanoLC-MS/MS for Bottom-Up Proteomics. J. Proteome Res. 19 (7), 2654–2663. doi:10.1021/acs.jproteome.9b00832
Neff, R. T., Senter, L., and Salani, R. (2017). BRCA Mutation in Ovarian Cancer: Testing, Implications and Treatment Considerations. Ther. Adv. Med. Oncol. 9, 519–531. doi:10.1177/1758834017714993
Nguyen, D.-H. T., Stapleton, S. C., Yang, M. T., Cha, S. S., Choi, C. K., Galie, P. A., et al. (2013). Biomimetic Model to Reconstitute Angiogenic Sprouting Morphogenesis In Vitro. Proc. Natl. Acad. Sci. 110, 6712–6717. doi:10.1073/pnas.1221526110
Nguyen, D. G., Funk, J., Robbins, J. B., Crogan-Grundy, C., Presnell, S. C., Singer, T., et al. (2016). Bioprinted 3D Primary Liver Tissues Allow Assessment of Organ-Level Response to Clinical Drug Induced Toxicity In Vitro. PLoS One 11, e0158674. doi:10.1371/journal.pone.0158674
Norotte, C., Marga, F. S., Niklason, L. E., and Forgacs, G. (2009). Scaffold-free Vascular Tissue Engineering Using Bioprinting. Biomaterials 30, 5910–5917. doi:10.1016/j.biomaterials.2009.06.034
O'Connell, C. D., Di Bella, C., Thompson, F., Augustine, C., Beirne, S., Cornock, R., et al. (2016). Development of the Biopen: a Handheld Device for Surgical Printing of Adipose Stem Cells at a Chondral Wound Site. Biofabrication 8, 015019. doi:10.1088/1758-5090/8/1/015019
Ozbolat, I. T., and Hospodiuk, M. (2016). Current Advances and Future Perspectives in Extrusion-Based Bioprinting. Biomaterials 76, 321–343. doi:10.1016/j.biomaterials.2015.10.076
Parham, S., Kharazi, A. Z., Bakhsheshi-Rad, H. R., Ghayour, H., Ismail, A. F., Nur, H., et al. (2020). Electrospun Nano-Fibers for Biomedical and Tissue Engineering Applications: A Comprehensive Review. Materials 13, 2153. doi:10.3390/ma13092153
Park, J., Lee, B. K., Jeong, G. S., Hyun, J. K., Lee, C. J., and Lee, S.-H. (2015). Three-dimensional Brain-On-A-Chip with an Interstitial Level of Flow and its Application as an In Vitro Model of Alzheimer's Disease. Lab. Chip 15, 141–150. doi:10.1039/c4lc00962b
Park, J. Y., Jang, J., and Kang, H.-W. (2018). 3D Bioprinting and its Application to Organ-On-A-Chip. Microelectronic Eng. 200, 1–11. doi:10.1016/j.mee.2018.08.004
Park, S. R., Kim, S. R., Lee, J. W., Park, C. H., Yu, W. J., Lee, S. J., et al. (2020). Development of a Novel Dual Reproductive Organ on a Chip: Recapitulating Bidirectional Endocrine Crosstalk between the Uterine Endometrium and the Ovary. Biofabrication 13, 15001. doi:10.1088/1758-5090/abbd29
Park, T. E., Mustafaoglu, N., Herland, A., Hasselkus, R., Mannix, R., FitzGerald, E. A., et al. (2019). Hypoxia-enhanced Blood-Brain Barrier Chip Recapitulates Human Barrier Function and Shuttling of Drugs and Antibodies. Nat. Commun. 10, 2621–2712. doi:10.1038/s41467-019-10588-0
Parrish, J., Lim, K., Zhang, B., Radisic, M., and Woodfield, T. B. F. (2019). New Frontiers for Biofabrication and Bioreactor Design in Microphysiological System Development. Trends Biotechnol. 37, 1327–1343. doi:10.1016/j.tibtech.2019.04.009
Paul, S. M., Mytelka, D. S., Dunwiddie, C. T., Persinger, C. C., Munos, B. H., Lindborg, S. R., et al. (2010). How to Improve R&D Productivity: the Pharmaceutical Industry's Grand challenge. Nat. Rev. Drug Discov. 9, 203–214. doi:10.1038/nrd3078
Petrosyan, A., Cravedi, P., Villani, V., Angeletti, A., Manrique, J., Renieri, A., et al. (2019). A Glomerulus-On-A-Chip to Recapitulate the Human Glomerular Filtration Barrier. Nat. Commun. 10, 3656. doi:10.1038/s41467-019-11577-z
Phan, D. T. T., Wang, X., Craver, B. M., Sobrino, A., Zhao, D., Chen, J. C., et al. (2017). A Vascularized and Perfused Organ-On-A-Chip Platform for Large-Scale Drug Screening Applications. Lab. Chip 17, 511–520. doi:10.1039/c6lc01422d
Pourchet, L. J., Thepot, A., Albouy, M., Courtial, E. J., Boher, A., Blum, L. J., et al. (2017). Human Skin 3D Bioprinting Using Scaffold-free Approach. Adv. Healthc. Mater. 6, 1601101. doi:10.1002/adhm.201601101
Prakadan, S. M., Shalek, A. K., and Weitz, D. A. (2017). Scaling by Shrinking: Empowering Single-Cell 'omics' with Microfluidic Devices. Nat. Rev. Genet. 18, 345–361. doi:10.1038/nrg.2017.15
Pyo, S.-H., Wang, P., Hwang, H. H., Zhu, W., Warner, J., and Chen, S. (2017). Continuous Optical 3D Printing of green Aliphatic Polyurethanes. ACS Appl. Mater. Inter. 9, 836–844. doi:10.1021/acsami.6b12500
Qian, F., Huang, C., Lin, Y.-D., Ivanovskaya, A. N., O'Hara, T. J., Booth, R. H., et al. (2017). Simultaneous Electrical Recording of Cardiac Electrophysiology and Contraction on Chip. Lab. Chip 17, 1732–1739. doi:10.1039/c7lc00210f
Qin, A., and Gadgeel, S. (2017). The Current Landscape of Anaplastic Lymphoma Kinase (ALK) in Non-small Cell Lung Cancer: Emerging Treatment Paradigms and Future Directions. Targ Oncol. 12, 709–718. doi:10.1007/s11523-017-0526-1
Raeisdasteh Hokmabad, V., Davaran, S., Ramazani, A., and Salehi, R. (2017). Design and Fabrication of Porous Biodegradable Scaffolds: a Strategy for Tissue Engineering. J. Biomater. Sci. Polym. Edition 28, 1797–1825. doi:10.1080/09205063.2017.1354674
Retting, K. N., O’neill, C. M., Nguyen, D. L. G., and Presnell, S. C. (2016). Engineered Three-Dimensional Skin Tissues, Arrays Thereof, and Methods of Making the Same. Patentscope.
Ribas, J., Zhang, Y. S., Pitrez, P. R., Leijten, J., Miscuglio, M., Rouwkema, J., et al. (2017). Biomechanical Strain Exacerbates Inflammation on a Progeria‐on‐a‐Chip Model. Small 13, 1603737. doi:10.1002/smll.201603737
Rifaioglu, A. S., Atas, H., Martin, M. J., Cetin-Atalay, R., Atalay, V., and Doğan, T. (2019). Recent Applications of Deep Learning and Machine Intelligence on In Silico Drug Discovery: Methods, Tools and Databases. Brief. Bioinform. 20, 1878–1912. doi:10.1093/bib/bby061
Rigat-Brugarolas, L. G., Elizalde-Torrent, A., Bernabeu, M., De Niz, M., Martin-Jaular, L., Fernandez-Becerra, C., et al. (2014). A Functional Microengineered Model of the Human Splenon-On-A-Chip. Lab. Chip 14, 1715–1724. doi:10.1039/c3lc51449h
Saadatpour, A., Lai, S., Guo, G., and Yuan, G.-C. (2015). Single-cell Analysis in Cancer Genomics. Trends Genet. 31, 576–586. doi:10.1016/j.tig.2015.07.003
Santos, E., Zarate, J., Orive, G., Hernández, R. M., and Pedraz, J. L. (2010). Biomaterials in Cell Microencapsulation. Adv. Exp. Med. Biol. 670, 5–21. doi:10.1007/978-1-4419-5786-3_2
Schutgens, F., Rookmaaker, M. B., Margaritis, T., Rios, A., Ammerlaan, C., Jansen, J., et al. (2019). Tubuloids Derived from Human Adult Kidney and Urine for Personalized Disease Modeling. Nat. Biotechnol. 37, 303–313. doi:10.1038/s41587-019-0048-8
Sekine, H., Shimizu, T., Sakaguchi, K., Dobashi, I., Wada, M., Yamato, M., et al. (2013). In Vitro fabrication of Functional Three-Dimensional Tissues with Perfusable Blood Vessels. Nat. Commun. 4, 1399–1410. doi:10.1038/ncomms2406
Selden, C., and Fuller, B. (2018). Role of Bioreactor Technology in Tissue Engineering for Clinical Use and Therapeutic Target Design. Bioengineering 5, 32. doi:10.3390/bioengineering5020032
Serena, E., Zatti, S., Zoso, A., Lo Verso, F., Tedesco, F. S., Cossu, G., et al. (2016). Skeletal Muscle Differentiation on a Chip Shows Human Donor Mesoangioblasts' Efficiency in Restoring Dystrophin in a Duchenne Muscular Dystrophy Model. Stem Cells Transl. Med. 5, 1676–1683. doi:10.5966/sctm.2015-0053
Shahriyari, L., and Komarova, N. L. (2013). Symmetric vs. Asymmetric Stem Cell Divisions: an Adaptation against Cancer? PLoS One 8, e76195. doi:10.1371/journal.pone.0076195
Sharifi, F., Yesil-Celiktas, O., Kazan, A., Maharjan, S., Saghazadeh, S., Firoozbakhsh, K., et al. (2020). A Hepatocellular Carcinoma-Bone Metastasis-On-A-Chip Model for Studying Thymoquinone-Loaded Anticancer Nanoparticles. Bio-des. Manuf. 3, 189–202. doi:10.1007/s42242-020-00074-8
Shi, P., Edgar, T. Y. S., Yeong, W. Y., and Laude, A. (2017). Hybrid Three-Dimensional (3D) Bioprinting of Retina Equivalent for Ocular Research. Int. J. Bioprint 3, 008. doi:10.18063/IJB.2017.02.008
Shik Mun, K., Arora, K., Huang, Y., Yang, F., Yarlagadda, S., Ramananda, Y., et al. (2019). Patient-derived Pancreas-On-A-Chip to Model Cystic Fibrosis-Related Disorders. Nat. Commun. 10 (1), 3124. doi:10.1038/s41467-019-11178-w
Shimizu, K., Araki, H., Sakata, K., Tonomura, W., Hashida, M., and Konishi, S. (2015). Microfluidic Devices for Construction of Contractile Skeletal Muscle Microtissues. J. Biosci. Bioeng. 119, 212–216. doi:10.1016/j.jbiosc.2014.07.003
Shimizu, T., Yamato, M., Isoi, Y., Akutsu, T., Setomaru, T., Abe, K., et al. (2002). Fabrication of Pulsatile Cardiac Tissue Grafts Using a Novel 3-dimensional Cell Sheet Manipulation Technique and Temperature-Responsive Cell Culture Surfaces. Circ. Res. 90, e40–e48. doi:10.1161/hh0302.105722
Shin, W., Hinojosa, C. D., Ingber, D. E., and Kim, H. J. (2019). Human Intestinal Morphogenesis Controlled by Transepithelial Morphogen Gradient and Flow-dependent Physical Cues in a Microengineered Gut-On-A-Chip. Iscience 15, 391–406. doi:10.1016/j.isci.2019.04.037
Shintu, L., Baudoin, R., Navratil, V., Prot, J.-M., Pontoizeau, C., Defernez, M., et al. (2012). Metabolomics-on-a-chip and Predictive Systems Toxicology in Microfluidic Bioartificial Organs. Anal. Chem. 84, 1840–1848. doi:10.1021/ac2011075
Si, L., Bai, H., Rodas, M., Cao, W., Oh, C. Y., Jiang, A., et al. (2021). A Human-Airway-On-A-Chip for the Rapid Identification of Candidate Antiviral Therapeutics and Prophylactics. Nat. Biomed. Eng. 5 (8), 815–829. doi:10.1038/s41551-021-00718-9
Skardal, A., Mack, D., Kapetanovic, E., Atala, A., Jackson, J. D., Yoo, J., et al. (2012). Bioprinted Amniotic Fluid-Derived Stem Cells Accelerate Healing of Large Skin Wounds. Stem Cells Transl. Med. 1, 792–802. doi:10.5966/sctm.2012-0088
Skylar-Scott, M. A., Uzel, S. G. M., Nam, L. L., Ahrens, J. H., Truby, R. L., Damaraju, S., et al. (2019). Biomanufacturing of Organ-specific Tissues with High Cellular Density and Embedded Vascular Channels. Sci. Adv. 5, eaaw2459. doi:10.1126/sciadv.aaw2459
Snook, R. D., Harvey, T. J., Correia Faria, E., and Gardner, P. (2009). Raman Tweezers and Their Application to the Study of Singly Trapped Eukaryotic Cells. Integr. Biol. 1, 43–52. doi:10.1039/b815253e
Solis, L. H., Ayala, Y., Portillo, S., Varela-Ramirez, A., Aguilera, R., and Boland, T. (2019). Thermal Inkjet Bioprinting Triggers the Activation of the VEGF Pathway in Human Microvascular Endothelial Cells In Vitro. Biofabrication 11, 045005. doi:10.1088/1758-5090/ab25f9
Song, J. W., and Munn, L. L. (2011). Fluid Forces Control Endothelial Sprouting. Proc. Natl. Acad. Sci. 108, 15342–15347. doi:10.1073/pnas.1105316108
Song, Y., Su, X., Firouzian, K. F., Fang, Y., Zhang, T., and Sun, W. (2020). Engineering of Brain-like Tissue Constructs via 3D Cell-Printing Technology. Biofabrication 12, 035016. doi:10.1088/1758-5090/ab7d76
Sontheimer-Phelps, A., Hassell, B. A., and Ingber, D. E. (2019). Modelling Cancer in Microfluidic Human Organs-On-Chips. Nat. Rev. Cancer 19, 65–81. doi:10.1038/s41568-018-0104-6
Souza, A. G., Silva, I. B. B., Campos-Fernandez, E., Barcelos, L. S., Souza, J. B., Marangoni, K., et al. (2018). Comparative Assay of 2D and 3D Cell Culture Models: Proliferation, Gene Expression and Anticancer Drug Response. Cpd 24, 1689–1694. doi:10.2174/1381612824666180404152304
Sriram, G., Alberti, M., Dancik, Y., Wu, B., Wu, R., Feng, Z., et al. (2018). Full-thickness Human Skin-On-Chip with Enhanced Epidermal Morphogenesis and Barrier Function. Mater. Today 21, 326–340. doi:10.1016/j.mattod.2017.11.002
Srivastava, S., Pasipanodya, J. G., Ramachandran, G., Deshpande, D., Shuford, S., Crosswell, H. E., et al. (2016). A Long-Term Co-perfused Disseminated tuberculosis-3D Liver Hollow Fiber Model for Both Drug Efficacy and Hepatotoxicity in Babies. EBioMedicine 6, 126–138. doi:10.1016/j.ebiom.2016.02.040
Sun, W., Starly, B., Daly, A. C., Burdick, J. A., Groll, J., Skeldon, G., et al. (2020). The Bioprinting Roadmap. Biofabrication 12, 022002. doi:10.1088/1758-5090/ab5158
Swaminathan, S., Hamid, Q., Sun, W., and Clyne, A. M. (2019). Bioprinting of 3D Breast Epithelial Spheroids for Human Cancer Models. Biofabrication 11, 025003. doi:10.1088/1758-5090/aafc49
Sydney Gladman, A., Matsumoto, E. A., Nuzzo, R. G., Mahadevan, L., and Lewis, J. A. (2016). Biomimetic 4D Printing. Nat. Mater. 15, 413–418. doi:10.1038/nmat4544
Takebe, T., and Wells, J. M. (2019). Organoids by Design. Science 364, 956–959. doi:10.1126/science.aaw7567
Tamay, D. G., Dursun Usal, T., Alagoz, A. S., Yucel, D., Hasirci, N., and Hasirci, V. (2019). 3D and 4D Printing of Polymers for Tissue Engineering Applications. Front. Bioeng. Biotechnol. 7, 164. doi:10.3389/fbioe.2019.00164
Thacker, V. V., Dhar, N., Sharma, K., Barrile, R., Karalis, K., and McKinney, J. D. (2020). A Lung-On-Chip Model of Early Mycobacterium tuberculosis Infection Reveals an Essential Role for Alveolar Epithelial Cells in Controlling Bacterial Growth. Elife 9, e59961. doi:10.7554/eLife.59961
Tijore, A., Behr, J.-M., Irvine, S. A., Baisane, V., and Venkatraman, S. (2018). Bioprinted Gelatin Hydrogel Platform Promotes Smooth Muscle Cell Contractile Phenotype Maintenance. Biomed. Microdevices 20, 32. doi:10.1007/s10544-018-0274-8
Torisawa, Y.-s., Spina, C. S., Mammoto, T., Mammoto, A., Weaver, J. C., Tat, T., et al. (2014). Bone Marrow-On-A-Chip Replicates Hematopoietic Niche Physiology In Vitro. Nat. Methods 11, 663–669. doi:10.1038/nmeth.2938
Trujillo-de Santiago, G., Flores-Garza, B. G., Tavares-Negrete, J. A., Lara-Mayorga, I. M., González-Gamboa, I., Zhang, Y. S., et al. (2019). The Tumor-On-Chip: Recent Advances in the Development of Microfluidic Systems to Recapitulate the Physiology of Solid Tumors. Materials 12, 2945. doi:10.3390/ma12182945
Tseng, H., Gage, J. A., Shen, T., Haisler, W. L., Neeley, S. K., Shiao, S., et al. (2015). A Spheroid Toxicity Assay Using Magnetic 3D Bioprinting and Real-Time mobile Device-Based Imaging. Sci. Rep. 5, 13987. doi:10.1038/srep13987
Tsoucas, D., and Yuan, G.-C. (2017). Recent Progress in Single-Cell Cancer Genomics. Curr. Opin. Genet. Dev. 42, 22–32. doi:10.1016/j.gde.2017.01.002
Urciuolo, A., Poli, I., Brandolino, L., Raffa, P., Scattolini, V., Laterza, C., et al. (2020). Intravital Three-Dimensional Bioprinting. Nat. Biomed. Eng. 4, 901–915. doi:10.1038/s41551-020-0568-z
Vallet-Regí, M., Balas, F., and Arcos, D. (2007). Mesoporous Materials for Drug Delivery. Angew. Chem. Int. Ed. 46, 7548–7558. doi:10.1002/anie.200604488
Varghese, S., Aung, A., and Lim, H. L. (2020). Microfluidic Assisted Perfusion Devices. Patentscope.
Vatine, G. D., Barrile, R., Workman, M. J., Sances, S., Barriga, B. K., Rahnama, M., et al. (2019). Human iPSC-Derived Blood-Brain Barrier Chips Enable Disease Modeling and Personalized Medicine Applications. Cell Stem Cell 24, 995–1005. e6. doi:10.1016/j.stem.2019.05.011
Vemuri, G. N., and Aristidou, A. A. (2005). Metabolic Engineering in the -omics Era: Elucidating and Modulating Regulatory Networks. Microbiol. Mol. Biol. Rev. 69, 197–216. doi:10.1128/mmbr.69.2.197-216.2005
Vernetti, L., Gough, A., Baetz, N., Blutt, S., Broughman, J. R., Brown, J. A., et al. (2017). Functional Coupling of Human Microphysiology Systems: Intestine, Liver, Kidney Proximal Tubule, Blood-Brain Barrier and Skeletal Muscle. Sci. Rep. 7, 1–15. doi:10.1038/srep42296
Vijayavenkataraman, S., Yan, W.-C., Lu, W. F., Wang, C.-H., and Fuh, J. Y. H. (2018). 3D Bioprinting of Tissues and Organs for Regenerative Medicine. Adv. Drug Deliv. Rev. 132, 296–332. doi:10.1016/j.addr.2018.07.004
Virlogeux, A., Moutaux, E., Christaller, W., Genoux, A., Bruyère, J., Fino, E., et al. (2018). Reconstituting Corticostriatal Network On-A-Chip Reveals the Contribution of the Presynaptic Compartment to Huntington's Disease. Cel Rep. 22, 110–122. doi:10.1016/j.celrep.2017.12.013
Vogel, C. L., Cobleigh, M. A., Tripathy, D., Gutheil, J. C., Harris, L. N., Fehrenbacher, L., et al. (2002). Efficacy and Safety of Trastuzumab as a Single Agent in First-Line Treatment of HER2-Overexpressing Metastatic Breast Cancer. Jco 20, 719–726. doi:10.1200/jco.2002.20.3.719
Vormann, M. K., Vriend, J., Lanz, H. L., Gijzen, L., van den Heuvel, A., Hutter, S., et al. (2021). Implementation of a Human Renal Proximal Tubule on a Chip for Nephrotoxicity and Drug Interaction Studies. J. Pharm. Sci. 110, 1601–1614. doi:10.1016/j.xphs.2021.01.028
Wang, G., McCain, M. L., Yang, L., He, A., Pasqualini, F. S., Agarwal, A., et al. (2014). Modeling the Mitochondrial Cardiomyopathy of Barth Syndrome with Induced Pluripotent Stem Cell and Heart-On-Chip Technologies. Nat. Med. 20, 616–623. doi:10.1038/nm.3545
Wang, H.-F., Ran, R., Liu, Y., Hui, Y., Zeng, B., Chen, D., et al. (2018a). Tumor-Vasculature-on-a-Chip for Investigating Nanoparticle Extravasation and Tumor Accumulation. ACS Nano 12, 11600–11609. doi:10.1021/acsnano.8b06846
Wang, J., Wang, C., Xu, N., Liu, Z.-F., Pang, D.-W., and Zhang, Z.-L. (2019a). A Virus-Induced Kidney Disease Model Based on Organ-On-A-Chip: Pathogenesis Exploration of Virus-Related Renal Dysfunctions. Biomaterials 219, 119367. doi:10.1016/j.biomaterials.2019.119367
Wang, P., Li, X., Zhu, W., Zhong, Z., Moran, A., Wang, W., et al. (2018b). 3D Bioprinting of Hydrogels for Retina Cell Culturing. Bioprinting 12, e00029. doi:10.1016/j.bprint.2018.e00029
Wang, S.-C., Ho, I.-K., Tsou, H.-H., Liu, S.-W., Hsiao, C.-F., Chen, C.-H., et al. (2013). Functional Genetic Polymorphisms inCYP2C19Gene in Relation to Cardiac Side Effects and Treatment Dose in a Methadone Maintenance Cohort. OMICS: A J. Integr. Biol. 17, 519–526. doi:10.1089/omi.2012.0068
Wang, X., Cirit, M., Wishnok, J. S., Griffith, L. G., and Tannenbaum, S. R. (2019b). Analysis of an Integrated Human Multiorgan Microphysiological System for Combined Tolcapone Metabolism and Brain Metabolomics. Anal. Chem. 91, 8667–8675. doi:10.1021/acs.analchem.9b02224
Wang, Y., Shi, W., Kuss, M., Mirza, S., Qi, D., Krasnoslobodtsev, A., et al. (2018c). 3D Bioprinting of Breast Cancer Models for Drug Resistance Study. ACS Biomater. Sci. Eng. 4, 4401–4411. doi:10.1021/acsbiomaterials.8b01277
Wang, Y., Wang, L., Zhu, Y., and Qin, J. (2018d). Human Brain Organoid-On-A-Chip to Model Prenatal Nicotine Exposure. Lab. Chip 18, 851–860. doi:10.1039/c7lc01084b
Wang, Z., Lang, B., Qu, Y., Li, L., Song, Z., and Wang, Z. (2019c). Single-cell Patterning Technology for Biological Applications. Biomicrofluidics 13, 061502. doi:10.1063/1.5123518
Watanabe, T., Okitsu, T., Ozawa, F., Nagata, S., Matsunari, H., Nagashima, H., et al. (2020). Millimeter-thick Xenoislet-Laden Fibers as Retrievable Transplants Mitigate Foreign Body Reactions for Long-Term Glycemic Control in Diabetic Mice. Biomaterials 255, 120162. doi:10.1016/j.biomaterials.2020.120162
Wegorzewska, I., and Baloh, R. H. (2011). TDP-43-based Animal Models of Neurodegeneration: New Insights into ALS Pathology and Pathophysiology. Neurodegenerative Dis. 8, 262–274. doi:10.1159/000321547
Wei, C., Yang, C., Wang, S., Shi, D., Zhang, C., Lin, X., et al. (2019). Crosstalk between Cancer Cells and Tumor Associated Macrophages Is Required for Mesenchymal Circulating Tumor Cell-Mediated Colorectal Cancer Metastasis. Mol. Cancer 18, 64. doi:10.1186/s12943-019-0976-4
Wei-Xuan, L. I., Liang, G.-T., Wei, Y. A. N., Zhang, Q., Wei, W., Xiao-Mian, Z., et al. (2013). Artificial Uterus on a Microfluidic Chip. Chin. J. Anal. Chem. 41, 467–472. doi:10.1016/S1872-2040(13)60639-8
Wevers, N. R., Kasi, D. G., Gray, T., Wilschut, K. J., Smith, B., van Vught, R., et al. (2018). A Perfused Human Blood-Brain Barrier On-A-Chip for High-Throughput Assessment of Barrier Function and Antibody Transport. Fluids Barriers CNS 15, 23. doi:10.1186/s12987-018-0108-3
Whitesides, G. M. (2006). The Origins and the Future of Microfluidics. Nature 442, 368–373. doi:10.1038/nature05058
Wikswo, J. P., Samson, P. C., Block, F. E., Reiserer, R. S., Parker, K. K., McLean, J. A., et al. (2017). Integrated Human Organ-On-Chip Microphysiological Systems. IFI CLAIMS Patent Services.
Wilson, W. C., and Boland, T. (2003). Cell and Organ Printing 1: Protein and Cell Printers. Anat. Rec. 272A, 491–496. doi:10.1002/ar.a.10057
Wu, Q., Liu, J., Wang, X., Feng, L., Wu, J., Zhu, X., et al. (2020). Organ-on-a-chip: Recent Breakthroughs and Future Prospects. Biomed. Eng. Online 19, 9. doi:10.1186/s12938-020-0752-0
Wu, W., DeConinck, A., and Lewis, J. A. (2011). Omnidirectional Printing of 3D Microvascular Networks. Adv. Mater. 23, H178–H183. doi:10.1002/adma.201004625
Wufuer, M., Lee, G., Hur, W., Jeon, B., Kim, B. J., Choi, T. H., et al. (2016). Skin-on-a-chip Model Simulating Inflammation, Edema and Drug-Based Treatment. Sci. Rep. 6, 37471. doi:10.1038/srep37471
Xavier da Silveira dos Santos, A., and Liberali, P. (2019). From Single Cells to Tissue Self‐organization. FEBS J. 286, 1495–1513. doi:10.1111/febs.14694
Xiao, S., Coppeta, J. R., Rogers, H. B., Isenberg, B. C., Zhu, J., Olalekan, S. A., et al. (2017). A Microfluidic Culture Model of the Human Reproductive Tract and 28-day Menstrual Cycle. Nat. Commun. 8, 14584. doi:10.1038/ncomms14584
Xing, Q. R., Cipta, N. O., Hamashima, K., Liou, Y.-C., Koh, C. G., and Loh, Y.-H. (2020). Unraveling Heterogeneity in Transcriptome and its Regulation through Single-Cell Multi-Omics Technologies. Front. Genet. 11, 662. doi:10.3389/fgene.2020.00662
Xu, J., and Ye, J. (2020). Perspectives on Supercomputing and Artificial Intelligence Applications in Drug Discovery. Supercomput. Front. Innov., 7.
Yang, H., Sun, L., Pang, Y., Hu, D., Xu, H., Mao, S., et al. (2020). Three-dimensional Bioprinted Hepatorganoids Prolong Survival of Mice with Liver Failure. Gut 70 (3), 567–574. doi:10.1136/gutjnl-2019-319960
Yang, J., Yamato, M., Kohno, C., Nishimoto, A., Sekine, H., Fukai, F., et al. (2005). Cell Sheet Engineering: Recreating Tissues without Biodegradable Scaffolds. Biomaterials 26, 6415–6422. doi:10.1016/j.biomaterials.2005.04.061
Yang, X., Li, K., Zhang, X., Liu, C., Guo, B., Wen, W., et al. (2018). Nanofiber Membrane Supported Lung-On-A-Chip Microdevice for Anti-cancer Drug Testing. Lab. Chip 18, 486–495. doi:10.1039/c7lc01224a
Yesil-Celiktas, O., Hassan, S., Miri, A. K., Maharjan, S., Al-kharboosh, R., Quiñones-Hinojosa, A., et al. (2018). Mimicking Human Pathophysiology in Organ-On-Chip Devices. Adv. Biosys. 2, 1800109. doi:10.1002/adbi.201800109
Yi, H.-G., Lee, H., and Cho, D.-W. (2017). 3D Printing of Organs-On-Chips. Bioengineering 4, 10. doi:10.3390/bioengineering4010010
Zhang, B., Montgomery, M., Chamberlain, M. D., Ogawa, S., Korolj, A., Pahnke, A., et al. (2016). Biodegradable Scaffold with Built-In Vasculature for Organ-On-A-Chip Engineering and Direct Surgical Anastomosis. Nat. Mater. 15, 669–678. doi:10.1038/nmat4570
Zhang, B., and Radisic, M. (2017). Organ-on-A-chip Devices advance to Market. Lab. Chip 17, 2395–2420. doi:10.1039/c6lc01554a
Zhang, M., Wang, P., Luo, R., Wang, Y., Li, Z., Guo, Y., et al. (2021). Biomimetic Human Disease Model of SARS‐CoV‐2‐Induced Lung Injury and Immune Responses on Organ Chip System. Adv. Sci. 8, 2002928. doi:10.1002/advs.202002928
Zhang, X., Hong, S., Yen, R., Kondash, M., Fernandez, C. E., and Truskey, G. A. (2018). A System to Monitor Statin-Induced Myopathy in Individual Engineered Skeletal Muscle Myobundles. Lab. Chip 18, 2787–2796. doi:10.1039/c8lc00654g
Zhang, Y. S., Aleman, J., Shin, S. R., Kilic, T., Kim, D., Mousavi Shaegh, S. A., et al. (2017a). Multisensor-integrated Organs-On-Chips Platform for Automated and Continual In Situ Monitoring of Organoid Behaviors. Proc. Natl. Acad. Sci. USA 114 (12), E2293–E2302. doi:10.1073/pnas.1612906114
Zhang, Y. S., Zhang, Y.-N., and Zhang, W. (2017b). Cancer-on-a-chip Systems at the Frontier of Nanomedicine. Drug Discov. Today 22, 1392–1399. doi:10.1016/j.drudis.2017.03.011
Zhao, S., Todorov, M. I., Cai, R., Maskari, R. A., Steinke, H., Kemter, E., et al. (2020). Cellular and Molecular Probing of Intact Human Organs. Cell 180, 796–812. doi:10.1016/j.cell.2020.01.030
Zhou, X., Qu, M., Tebon, P., Jiang, X., Wang, C., Xue, Y., et al. (2020). Screening Cancer Immunotherapy: When Engineering Approaches Meet Artificial Intelligence. Adv. Sci. 7, 2001447. doi:10.1002/advs.202001447
Keywords: 3D biofabrication, organ-on-a-chip, tissue engineering, regenerative medicine, precision medicine, drug development
Citation: Celikkin N, Presutti D, Maiullari F, Fornetti E, Agarwal T, Paradiso A, Volpi M, Święszkowski W, Bearzi C, Barbetta A, Zhang YS, Gargioli C, Rizzi R and Costantini M (2021) Tackling Current Biomedical Challenges With Frontier Biofabrication and Organ-On-A-Chip Technologies. Front. Bioeng. Biotechnol. 9:732130. doi: 10.3389/fbioe.2021.732130
Received: 28 June 2021; Accepted: 31 August 2021;
Published: 16 September 2021.
Edited by:
Pedro Ferreira Costa, Biofabics, PortugalReviewed by:
Krasimir Vasilev, University of South Australia, AustraliaCopyright © 2021 Celikkin, Presutti, Maiullari, Fornetti, Agarwal, Paradiso, Volpi, Święszkowski, Bearzi, Barbetta, Zhang, Gargioli, Rizzi and Costantini. This is an open-access article distributed under the terms of the Creative Commons Attribution License (CC BY). The use, distribution or reproduction in other forums is permitted, provided the original author(s) and the copyright owner(s) are credited and that the original publication in this journal is cited, in accordance with accepted academic practice. No use, distribution or reproduction is permitted which does not comply with these terms.
*Correspondence: Marco Costantini, bWNvc3RhbnRpbmlAaWNoZi5lZHUucGw=
Disclaimer: All claims expressed in this article are solely those of the authors and do not necessarily represent those of their affiliated organizations, or those of the publisher, the editors and the reviewers. Any product that may be evaluated in this article or claim that may be made by its manufacturer is not guaranteed or endorsed by the publisher.
Research integrity at Frontiers
Learn more about the work of our research integrity team to safeguard the quality of each article we publish.