- 1Department of Orthodontics and Dentofacial Orthopedics, University Hospital, LMU Munich, Munich, Germany
- 2Department of Conservative Dentistry and Periodontology, University Hospital, LMU Munich, Munich, Germany
- 3Department of Oral and Maxillofacial Plastic Surgery, Martin-Luther-University Halle-Wittenberg, Halle (Saale), Germany
Orthodontic tooth movement is based on the remodeling of tooth-surrounding tissues in response to mechanical stimuli. During this process, human periodontal ligament cells (hPDLCs) play a central role in mechanosensing and mechanotransduction. Various in vitro models have been introduced to investigate the effect of tension on hPDLCs. They provide a valuable body of knowledge on how tension influences relevant genes, proteins, and metabolites. However, no systematic review summarizing these findings has been conducted so far. Aim of this systematic review was to identify all related in vitro studies reporting tension application on hPDLCs and summarize their findings regarding force parameters, including magnitude, frequency and duration. Expression data of genes, proteins, and metabolites was extracted and summarized. Studies’ risk of bias was assessed using tailored risk of bias tools. Signaling pathways were identified by protein-protein interaction (PPI) networks using STRING and GeneAnalytics. According to our results, Flexcell Strain Unit® and other silicone-plate or elastic membrane-based apparatuses were mainly adopted. Frequencies of 0.1 and 0.5 Hz were predominantly applied for dynamic equibiaxial and uniaxial tension, respectively. Magnitudes of 10 and 12% were mostly employed for dynamic tension and 2.5% for static tension. The 10 most commonly investigated genes, proteins and metabolites identified, were mainly involved in osteogenesis, osteoclastogenesis or inflammation. Gene-set enrichment analysis and PPI networks gave deeper insight into the involved signaling pathways. This review represents a brief summary of the massive body of knowledge in this field, and will also provide suggestions for future researches on this topic.
Introduction
Orthodontic treatment aims to align malpositioned teeth towards a functional optimal position by application of an appropriate force (Wichelhaus, 2017). This force leads to bone resorption in direction of the movement (“compressive side”) and bone formation on the opposite side (“tension side”). Orthodontic tooth movement (OTM) is therefore based on the controlled stimulation of bone remodeling by application of external force (“orthodontic force”). At the cellular level, OTM is based on remodeling processes in the periodontal ligament (PDL) and the alveolar bone (Cho et al., 2010; Chang et al., 2017). Placed between the teeth and the surrounding alveolar bone, the PDL is a heterogeneous connective tissue, that is composed of several different cell populations including but not limited to fibroblasts, macrophages, stem cells and endothelial cells (Marchesan et al., 2011). In the context of in vitro experiments, the term “PDL fibroblast” should be used carefully. Cells described as “PDL fibroblast” are commonly isolated from the middle third of the tooth root. For cell isolation either the “explant” or the “digestion” technique is employed (for further details: see discussion). Yet, both techniques will result in a heterogeneous mixture of different cell types (Marchesan et al., 2011).
The PDL is essential for maintaining the homeostasis and integrity of the tooth supporting tissue (Ren et al., 2015; Wu et al., 2019b) and plays a pivotal role in coping with physiological forces that occur during routine activities, i.e. speaking or mastication and non-physiological external forces (Pavasant and Yongchaitrakul, 2011). Involution and atrophy of PDL is induced by lack of recurring mechanical stimuli (Cohn, 1965), while exposure against excessive forces will impair the subtle balance between osteogenesis and osteoclastogenesis, ultimately leading to the disintegration and loss of the osseous tooth support (Nogueira et al., 2014a). Therapeutic mechanical force applied onto teeth is mediated to the alveolar bone via the PDL thereby inducing bone remodeling and OTM (Vansant et al., 2018). Periodontal ligament cells (PDLCs) play a vital role in the transduction of mechanical force to biological signals, achieving the balance between bone formation and resorption (Kook and Lee, 2012; Li et al., 2019). PDLCs can be activated in response to periodontal ligament injury followed by proliferation, migration and synthesis of new matrix components (Krishnan and Davidovitch, 2006). Potentially, PDLCs can differentiate into cementoblasts or osteoblasts and are involved in the repair and the regeneration of the periodontal tissues (Pavasant and Yongchaitrakul, 2011).
Due to the complex structure of the periodontium and to evaluate inter and intracellular signaling pathways, in vitro models have been established to simulate the two major mechanical stimuli occurring during OTM (Yang et al., 2015; Janjic et al., 2018; Vansant et al., 2018): tension and compression. The main working principles of these setups can be summarized as approaches in which tension is applied via substrate deformation, whereas compression is mainly applied via weight, hydrostatic pressure, or centrifugation (Yang et al., 2015). In vitro compression models were recently summarized (Janjic et al., 2018) and the underlying molecular signal transduction has been shown by numerous in vitro experiments (Baumert et al., 2004; Shi et al., 2019a; Shi et al., 2019b; Janjic Rankovic et al., 2020). Based on these reports various molecular pathways involved in OTM have been identified, including but not limited to genes and proteins which are related to osteogenesis, osteoclastogenesis, inflammation and apoptosis (Yang et al., 2015; Janjic et al., 2018; Vansant et al., 2018). To study the effect of tension on PDLCs, different in vitro models have been designed to apply continuous (“static”) or intermitted (“dynamic”) tension force along one principal axis (“uniaxial”) or along all axes in all directions (“equibiaxial”) of a cell (definition according to Lee and von Recum, 2015)(Figure 1). However, the force parameters in terms of dynamic and static tension used show enormous heterogeneity, depending on the specific objectives of the experiments.
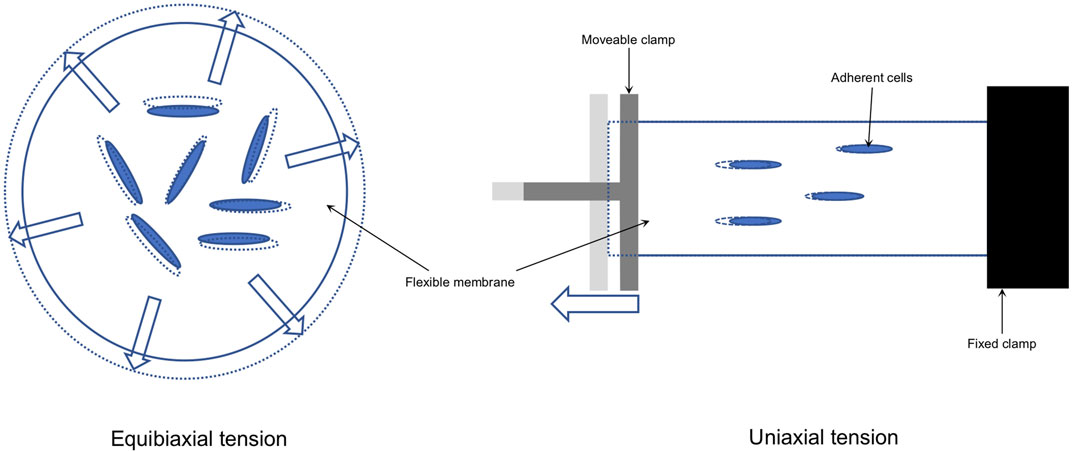
FIGURE 1. Tension is applied to adherent cells growing on a flexible surface (e.g. a. silicon membrane; solid lines) by elongation (dotted lines) of that surface either with equal forces acting in all directions in the same way (equibiaxial) or equal forces acting in one principle axis (uniaxial).
Therefore, this systematic review aimed to summarize the data on different in vitro tension models applied to human PDLCs (hPDLCs) as well as the effect of tension on the expression of genes and proteins. Specifically, this systematic review aimed 1) to identify all relevant studies applying tension on hPDLCs e.g. to simulate orthodontic force or other clinically relevant forces; 2) to make an assessment of the methodological and reporting quality of the included studies; 3) to summarize the biological and force parameters, and the commonly adopted methods for detecting biological regulation; and 4) to identify the most frequently investigated genes/proteins and their regulation, as well as the biological processes and pathways that might be affected by tension in hPDLCs.
Materials and Methods
This systematic review was conducted following the “Preferred Reporting Items for Systematic Reviews and Meta-Analyses” (PRISMA) guidelines (Moher et al., 2009). The protocol of this systematic review was finalized before data collection. A registration in the PROSPERO database was not possible, since only in vitro studies were included.
Eligibility Criteria
Inclusion criteria were defined in accordance with the “P.I.C.O.” framework (Schardt et al., 2007):
• P(atient): human periodontal ligament cells (hPDLCs) or human periodontal ligament derived stem cells (hPDLSCs);
• I(ntervention): in vitro static and dynamic tension (e.g. to simulate orthodontic force or other clinically relevant forces);
• C(ontrol): human periodontal ligament cells (hPDLCs) or human periodontal ligament derived stem cells (hPDLSCs) not subjected to mechanical force;
• O(utcome): force parameters (i.e. apparatus, force duration, force magnitude, frequency of force exposure) and regulation of gene, protein and/or metabolite expression in response to tension.
The following exclusion criteria were applied:
• In vivo studies
• In vitro studies not applying tension on hPDLCs or hPDLSCs
• Reviews
• Studies not reporting quantitative data on gene or protein expression
• Application of force other than tension or the specific type of force is undefined
• 3D model
• Co-culture
• Articles not published in English
Search Strategy and Study Selection
The search strategy considered keywords concerning the specific objectives of the studies, the force applied and the cells exposed to experimental force and were summarized in Table 1. PubMed search was completed on 31-01-2020 and the results were imported into EndNote® X9.3.1 (Clarivate Analytics, Philadelphia, Pennsylvania, United States).
First, unrelated studies were excluded after reading titles and abstracts according to the eligibility criteria defined. Then, full texts of the remaining studies were acquired. After full text reading, articles not fulfilling the eligibility criteria were excluded (Supplementary Table S1), and those in accordance with the inclusion criteria were used for data extraction (Supplementary Table S2). Any disagreements or uncertainties during both steps were discussed with two other review authors (U.B. and M.J.R.) until agreement was achieved.
Risk of Bias Assessment (Definition and Table for Assessment)
Risk of bias of the included in vitro studies was assessed using the methods described by Vansant et al. (2018) and Samuel et al. (2016). Methodological risk of bias was evaluated using 15 criteria and reporting risk of bias using 10 criteria (Supplementary Table S3). Each criterion was scored “low risk of bias” (“+”), “high risk of bias” (“−“), “incomplete or unclear risk of bias” (“?”) or “not applicable” (“n.a.”) based on the low risk of bias definitions given in Supplementary Table S3 for both, reporting and methodological quality. Low risk of bias (“LoB”) and if necessary high risk of bias (“HoB”) of the different criteria were defined according to the information provided in the aforementioned publications, citations therein and the following additional sources: “OHAT Risk of Bias Rating Tool for Human and Animal Studies” and “Biophysical Journal’s Guidelines for the Reproducibility in Biophysics Research” (details in Supplementary Table S3). To simplify data entry during the assessment, data sheets for both risk of bias assessments were developed (Supplementary Table S3).
All included articles were scored by two authors (C.S. and M.J.R.). Any disagreement was discussed internally until consensus was achieved. The results of the risk of bias assessment were recorded and summarized in predefined tables (Supplementary Tables S4.1, S4.2).
Data Extraction
After final selection of relevant studies, the following information on experimental design and outcome were extracted and summarized: reference (author, year, journal); cells used (age/gender of donors, tooth type, isolation method, cell culture passages and cell density used in the experiments); force applied (“dynamic”/”static” and “equibiaxial”/”uniaxial” force application; its duration, frequency of exposure, magnitude, and the device used); genes analyzed (official gene symbol if applicable) with reference to force application and the methods applied to measure their expression. “Gene or analyte investigated”, “Cells used” and the details on the “Force apparatus” were extracted using the original phrases from the studies and recorded in Supplementary Table S2. In addition, gene expression patterns including peak expression and the reported units were recorded; fold changes and ratios were calculated, if applicable.
Information Related to Genes and Proteins
Specificity of primers used in PCR reactions was verified with Primer-BLAST (https://www.ncbi.nlm.nih.gov/tools/primer-blast/). All genes were reported using their official gene symbol according to the HUGO Gene Nomenclature Committee (HGNC; URL: https://www.genenames.org). For protein data, antibody or ELISA specificity was verified using information provided within the studies and the information given by the suppliers. If possible, official gene symbols according to HGNC were applied. If antibody specificity was not sufficient, e.g. no discrimination between isoforms or gene variants, antibody targets were recorded according to the manufacturer given in that publication.
The expression patterns of genes and/or proteins were described using specific terms (Figure 2) and numerical data describing specific maxima and minima (stars in Figure 2) was collected. Data was directly acquired from the publications itself or extracted from graphs using Engauge Digitizer Software version 10.12 (URL: https://markummitchell.github.io/engauge-digitizer). To allow comparisons between gene or protein expression data from different sources, ratios (experimental condition vs control) or fold changes were calculated if not given by the authors. Gene expression was reported as “fold change” (FC), if its calculation was done either according to Livak and Schmittgen (2001) or similar sources or was clearly described as “2−ΔΔCt” or “ΔΔCt”. Gene expression was designated as “ratio”, if the control was “defined as 1”, otherwise it was described as “relative change” (“rel”). Results reported from more than one donor were listed separately.
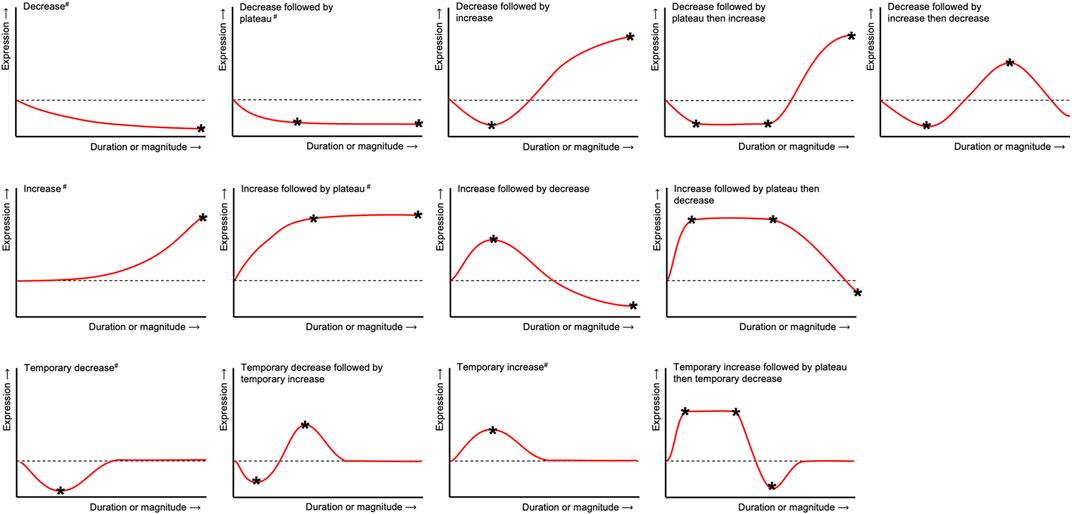
FIGURE 2. Terms used to describe the general expression patterns in hPDLCs after tension application for each gene, protein or metabolite included herein. Numerical data describing specific maxima and minima (*) was collected and reported in Supplementary Tables S2, S7. Expression patterns labeled with a double cross (#) were mapped on the protein-protein interaction networks constructed from gene lists shown in Figure 5.
Information Related to Force
The frequency of force exposure used in all dynamic tension studies was reported as “Hz” if possible. If frequency was reported in other units, conversion was done according to the information given. Any inconsistencies were resolved by discussion between the authors. Information about the force apparatuses was either directly collected from the publications, by searching the manufacturer information or Google® patent search (URL: https://patents.google.com) if applicable. Equibiaxial and uniaxial tension (Figure 1) were distinguished depending on the direction of the force applied in relation to the hPDLCs using information contained in the publications and citation chaining. The information was defined “unclear” or “incomplete”, if insufficient information was given that was even not resolved after communication with the manufacturer.
Summary Statistics
A summary of statistics on the force apparatuses was prepared from a unified list based on “apparatus”, followed by sorting according to the force type (i.e. “dynamic”/“static” and “equibiaxial”/“uniaxial”). Afterwards, publications using the same type of apparatus were combined into the same category for further analysis. A summary statistic on the different force parameters was compiled from a unified list of publications based on tension type and tension frequency. From each publication reporting dynamic tension application, maximum duration of force exposure and mainly adopted magnitude were summarized for the same frequency. Studies utilizing static tension were classified first by magnitude followed by maximum force duration. Replicates derived from the same study were removed and all analytes were ranked according to abundance.
Gene-List and Protein-Protein Interaction Network Analysis
Based on the complete lists of examined genes, differential expressed gene (DEG) lists were compiled according to the following criteria: the gene was identified unequivocally and changes in gene expression due to force application were reported using the terms defined in Figure 2. Depending on force application, the genes were assigned either to the “dynamic” or to the “static” gene list. Both DEG lists were used to generate protein-protein-interaction (PPI) networks and for gene list enrichment (Figure 3B).
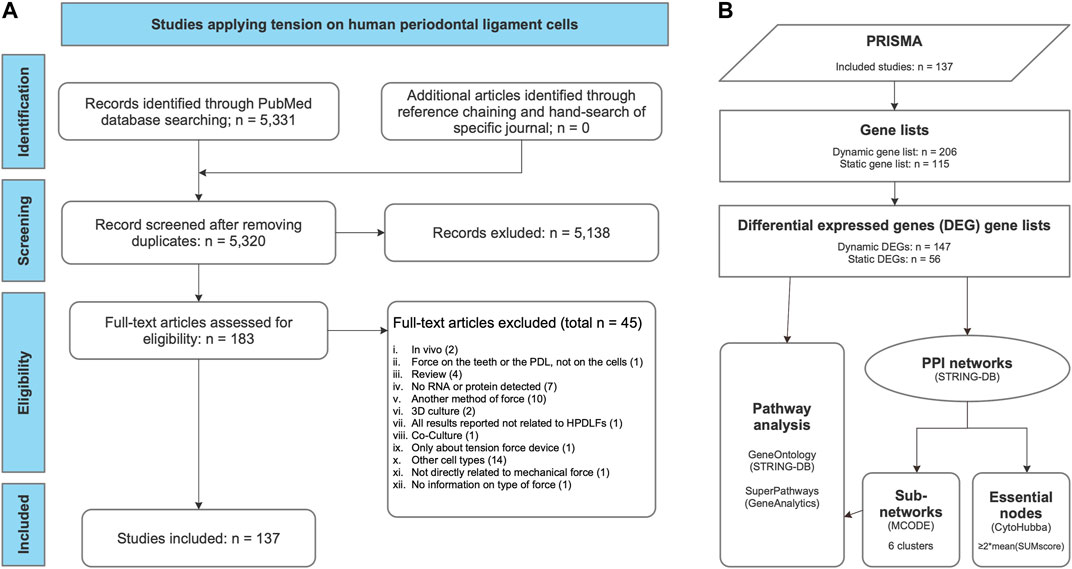
FIGURE 3. Workflows applied in this systematic review. (A) PRISMA flow diagram for the whole process of study selection according to Moher et al. (2009). (B) Gene set enrichment and network analysis of gene lists derived from the review process. Gene lists were compiled, listing the examined genes, proteins or metabolites studied either after dynamic or static tension application (Table 3, Supplementary Table S2). From these, differential expressed gene (DEG) lists were generated according to the specified criteria (Table 4). For each of these two DEG lists (dynamic DEG, static DEG) protein-protein interaction (PPI) networks were constructed using STRING-DB (Szklarczyk et al., 2019) (Figures 5A,B) and pathway analysis was conducted quering “GeneOntology/Biologcal Process” (Ashburner et al., 2000) and GeneAnalytics’ “SuperPath” databases (Ben-Ari Fuchs et al., 2016) (Supplementary Table S8). Subnetworks were identified in both PPI networks using MCODE (Bader and Hogue, 2003) (Table 4; Figures 5C,D) and essential nodes, so called “hub genes”, using cytoHubba (Chin et al., 2014) (Table 5; Figure 5).
To predict potential interactions between the DEGs at the protein level, PPI networks were constructed querying the “Search Tool for the Retrieval of Interacting Genes/Proteins” database (STRING-DB) (v11.0; URL: https://string-db.org) (Szklarczyk et al., 2019) using the stringApp plugin version 1.5.1 (Doncheva et al., 2019) with Cytoscape version 3.8.0 (Shannon et al., 2003; Su et al., 2014). A minimum required combined score of 0.7 was applied, i.e. only high confidence interactions were included in the predicted networks. Cluster analysis and cluster visualization of both “dynamic” and “static” PPI networks was done applying the “Molecular Complex Detection” (MCODE) algorithm (Bader and Hogue, 2003) as implemented in the clusterMaker2 app version 1.3.1 (Morris et al., 2011) with default settings and “fluff” activated. Hub genes, i.e. essential genes in a network, were identified with cytoHubba version 0.1 (Chin et al., 2014) using default settings. This plugin applies eleven different local and global topological methods to the nodes of a given network. For each node, a total score was calculated based on these eleven measures. A node was considered as a hub node, if its total score was at least twofold higher than the mean total score of all nodes of that particular network. Networks were visualized with Cytoscape version 3.8.0.
StringApp was also applied for gene list enrichment using the “GeneOntology/Biological Process” database (Ashburner et al., 2000). The “SuperPaths” database was analyzed online with “GeneAnalytics” (version 4.14 Build 1; URL: https://ga.genecards.org) (Ben-Ari Fuchs et al., 2016). To increase specificity, results from both databases were filtered according to the proportion of query genes in relation to the number of background genes of the specific database entry and a cut-off of ≥0.05 (i.e. 5 %) was applied. In all cases the ten most significant terms or pathways were reported. Individual gene set enrichment was applied to 1) the two complete networks and 2) each identified cluster, using “GeneAnalytics” and stringApp.
Results
Study Selection
The whole process of study selection was summarized in the PRISMA flow diagram (Figure 3A) (Moher et al., 2009). The applied search strategy identified 5,331 publications. No additional articles were identified through reference chaining or hand-search of specific journals. After removing 11 duplicates, 5,320 studies were left, of which 5,138 publications were excluded after title and abstract reading according to the defined criteria. Afterwards, 182 publications were assessed by full-text reading, of which 45 were excluded according to the exclusion criteria defined previously (Figure 3A; Supplementary Table S1).
Risk of Bias
Methodological quality was assessed using 15 criteria (Supplementary Table S3). The criteria “Randomization”, “Blinding of researchers”, and “Blinding of outcome assessors” were not applicable to in vitro studies. The results of the other risk of bias criteria showed a large variability. “Sample size determination” and “Statistical analysis” were mostly assessed as “high risk of bias” (“HoB”). “Accounting for confounding variables”, “Optimal time window used” and “Test organism/system” were found to have a high level of “incomplete or unclear risk of bias”. With reference to the remaining criteria, most of the studies were assessed as “low risk of bias” (“LoB”) (Figure 4; details in Supplementary Table S4.1).
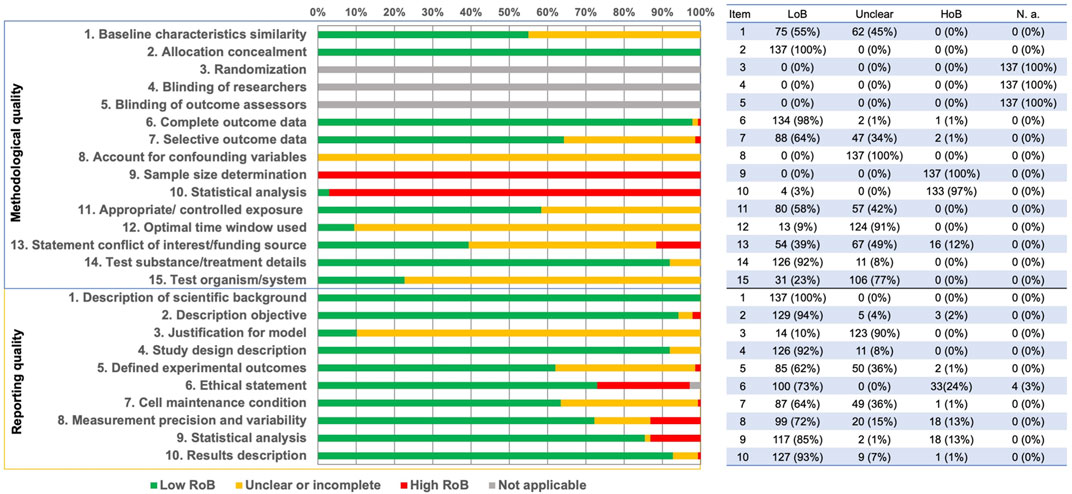
FIGURE 4. Summary of the risk of bias assessments for methodological (upper panel) and reporting quality risk of bias (lower panel). The tabulated data on the right reported frequency and percentage [n(%)] for each item according to its scoring: “LoB” – low risk of bias, “Unclear” – unclear or incomplete, “HoB” – high risk of bias, “N.a.” – not applicable.
The reporting quality of the publications was higher in comparison to the methodological quality, since more studies were classified as “LoB” (Figure 4, Supplementary Table S4.2). Only the criterion “Justification for model” was found to have a large percent of “Incomplete or unclear risk of bias” (Figure 4; details in Supplementary Table S4.2).
Tension Characteristics
In 30 out of 137 qualified studies (∼22%) static tension was applied, whereas 103 out of 137 included studies (∼75%) focused on the effect of dynamic tension (Supplementary Table S5). Direct comparison between static and dynamic tension was conducted in three studies (∼2%) (Papadopoulou et al., 2017; Wada et al., 2017; Memmert et al., 2020). One study did not clearly define the specific force type applied (Supplementary Table S5).
Devices for Tension Application
Regarding the type of apparatus, all included studies were identified as either equibiaxial or uniaxial (Figure 1). Various apparatuses were used in these studies either for equibiaxial or uniaxial tension (Table 2; Tables S5.1 and S5.2 in Supplementary Table S5).
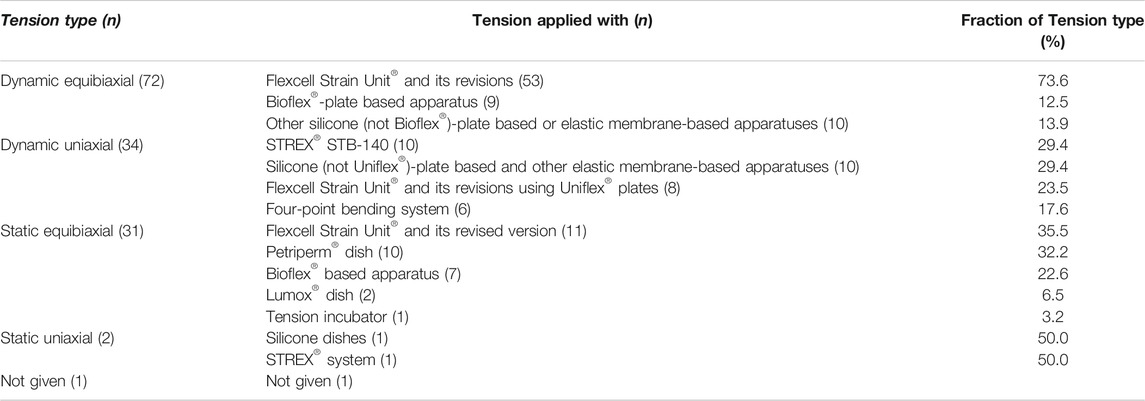
TABLE 2. Summary statistics according to tension type and apparatus. Since some publications applied two tension types, the total number given here is larger (n = 140) than the number of studies identified (n = 137).
Dynamic equibiaxial tension: Fifty three studies applied dynamic equibiaxial tension using the Flexcell Strain Unit (Flexcell® International Corporation, Burlington, NC, United States) and its revisions (FX-2000, FX-3000, FX-4000, FX-5000) (Banes et al., 1985). This system employs tension to cells seeded on elastic silicone membranes fixed in special 6-well plates (Bioflex® plates; Flexcell® International Corporation) by application of a vacuum below the flexible membrane. In nine studies, Bioflex® plates were used together with individually constructed devices, including the “CESTRA” (Deschner et al., 2007) and the “Cell Extender” devices (Wada et al., 2017) (Table 2; Supplementary Table S5.1 in Supplementary Table S5). Ten studies used different non-Bioflex®-plate based silicone or elastic membrane-based apparatuses including the “Cell Strain Unit (CSU)” (Hao et al., 2009), which was used in eight studies.
Dynamic uniaxial tension: Application of dynamic uniaxial tension using the “STB-140” system (STREX® Inc., Osaka, Japan) was described in ten publications. The Flexcell Strain Unit® in combination with Uniflex® culture plates (Flexcell® International Corporation) was adopted in eight studies. Non Bioflex®-plate based silicone and other elastic membrane-based apparatus were adopted in ten studies. Four-point bending systems were employed in six studies (Table 2; Supplementary Table S5.2 in Supplementary Table S5).
Static tension: To apply static equibiaxial tension, the Flexcell Strain Unit and its revisions (FX-3000™, FX-4000™, FX-5000™) were adopted in eleven studies. Apparatuses based on the Petriperm™ dish were used in ten studies, in which the dish was deformed by the weight placed onto a spheroidal template. Bioflex®-based devices were used in seven studies, Lumox® culture dishes were adopted in two and the “tension incubator” in one study (Table 2; Supplementary Table S5.3 in Supplementary Table S5). Static uniaxial tension was applied in two studies (Table 2; Supplementary Table S5.4 in Supplementary Table S5): one with the STREX system and the other via a “silicone dishes based in-house designed device” with a moving clamp (Papadopoulou et al., 2017).
In summary, dynamic tension was more frequently investigated than static tension, and equibiaxial tension was more commonly adopted than uniaxial tension, both with a ratio ∼3:1.
Force Magnitude and Duration in Equibiaxial and Uniaxial Tension
Dynamic equibiaxial tension was applied using frequencies between 0.005–1 Hz, with 0.1 Hz used in the majority of the studies. The maximum magnitude most frequently adopted varied between 1–24% of which 10 and 12% were more commonly applied. The mainly adopted duration of force exposure varied from 1 h to 6 days, using 48 and 72 h in the majority of studies (Supplementary Table S5.5).
For dynamic uniaxial tension frequencies between 0.005 – 1 Hz were used. The most frequently adopted frequency was 0.5 Hz. The magnitude varied between 0.2 and 33%, with magnitudes of 10 and 12% being most frequently applied. Force duration ranged from 1 h to 7 days and 48 h was the most frequent one (Supplementary Table S5.6).
Static equibiaxial tension was applied with force magnitudes varying between 0.28 and 35%, mimicking physiological or pathological mechanical force. The most frequent magnitude was 2.5%. Force duration varied between 0.5 h and 15 days, whereby 12 h was the most commonly used one (Supplementary Table S5.7).
For static uniaxial tension, magnitudes of 5%, 8 and 10% were applied for a maximum of 12, 3, and 12 h, respectively (Supplementary Table S5.8).
Genes, Proteins and Metabolites Analyzed
Relevant data on 205 genes, proteins or metabolites in relation to dynamic tension application to hPDLCs was extracted from 104 publications (Table 3). Static tension was applied in 33 publications and expression profiles of 115 different genes, proteins or metabolites related to force application were determined (Table 3). Genes or proteins that were not clearly assigned to a specific gene symbol due to ambiguities in the reported PCR primers or antibodies used in western blot or ELISA procedures were also included (e.g. “COL1A1/COL1A2” or “MAPK3/MAPK1”).
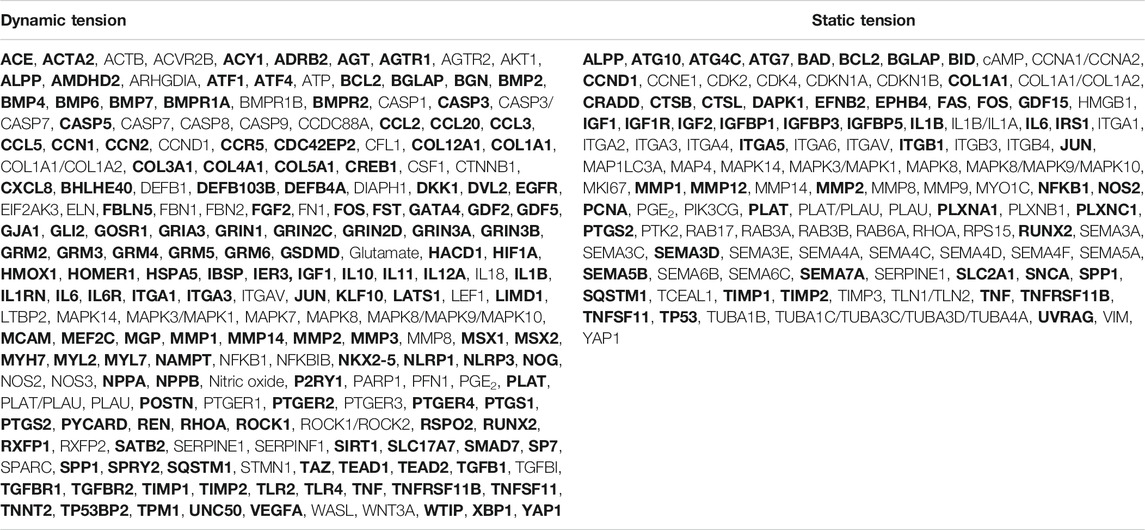
TABLE 3. Genes, proteins and metabolites analyzed in the included studies. Differential expressed genes (DEGs) used for the subsequent gene list enrichment analysis are given in bold.
The most commonly investigated 10 genes or metabolites in these studies were (in descending order): runt-related transcription factor 2 (RUNX2), alkaline phosphatase (ALPP; also known as ALP), bone gamma-carboxy glutamic acid-containing protein (BGLAP; also known as osteocalcin), interleukin 1β (IL1B), prostaglandin-endoperoxide synthase 2 (PTGS2; also known as COX2), tumor necrosis factor-alpha receptor superfamily member 11B (TNFRSF11B; also known as osteoprotegerin, OPG), TNF superfamily member 11 (TNFSF11; also known as RANKL), collagen Iα1 (COL1A1), prostaglandin E2 (PGE2) and Osterix (SP7; identical with OSX) (Supplementary Table S6). Their expression profiles and the corresponding force-related information were summarized in Supplementary Table S7.
Gene List and Protein-Protein Interaction Network Analysis
Gene list analysis was done as described (Figure 3B). The gene list compiled from studies on dynamic forces contained 206 genes, proteins or analytes, of which 147 (∼71.4%) were identified as differential expressed genes (DEG) as defined above. Of 115 entries from the gene list related to studies applying static forces, 56 (∼48.7%) were identified as DEGs (Table 3).
Protein-protein interaction (PPI) networks were generated using both gene lists with STRING-DB as described, and network statistics for both networks were calculated (Figure 5). For each gene node shown in Figure 5 the number of studies (node size) and expression pattern(s) were depicted: most of the genes included in the “dynamic” network showed (Figure 5A) an upregulation in gene expression after dynamic tension application. Some genes were downregulated only (e.g. ATF1, BMPR2, TGFBR1 and TGFBR2) whereas a few genetic loci were reported to be either up- or down regulated (e.g. ALPP, COL1A1, CXCL8, IL1B, or IL10). In contrast, gene expression of the majority of genes included in the “static” PPI network was either up- or downregulated depending on the particular study (Figure 5B). Up- and downregulation was reported for three genes (IL6, IGF, TNFSF11) by different studies. Interestingly, 12 genes from the “dynamic” DEG list (Figure 5A) and 4 genes from the “static” DEG list (Figure 5B) were not included in their particular PPI networks (Table 4) including ALPP, which is one of the 10 most frequently investigated genes or metabolites here.
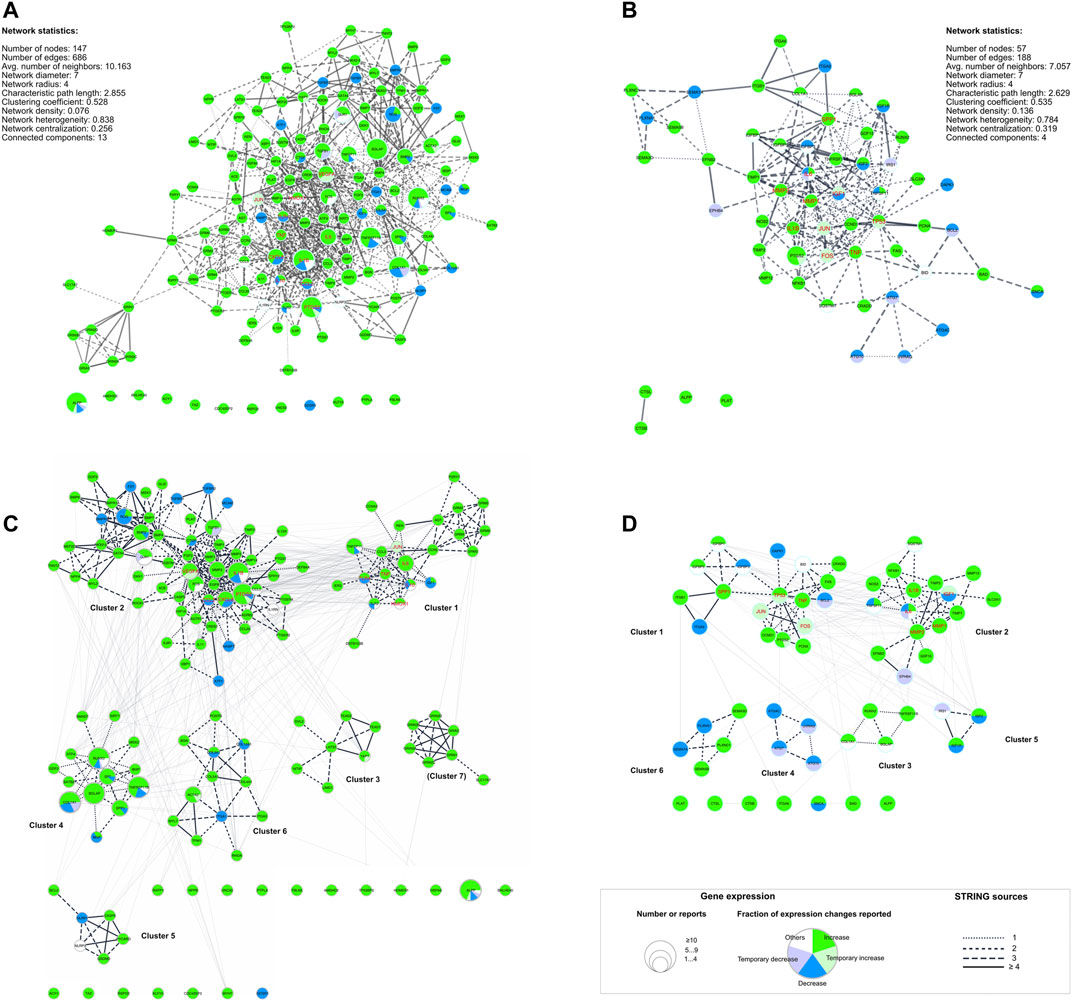
FIGURE 5. Dynamic (A, C) and static (B, C) protein-protein-interaction (PPI) networks generated using differential expressed genes (DEG) lists (Table 4). (A, B) PPI networks including basic network statistics for each were generated using STRING-DB. (C, D) show the same networks as in (A, B) but herein, the identified subnetworks are emphasized and labeled (Table 4, Supplementary Table S8). The legend applies to all four networks: The node size corresponds to the number of reports identified for the respective underlying gene. The edges’ line style depicts the number of STRING sources for the given connection. Additionally, hub genes identified with cytoHubba (Chin et al., 2014) were colored red (Table 5). For the complete networks (A, B) and each of the subnetworks (C, D) pathway analysis was applied (Supplementary Table S8).
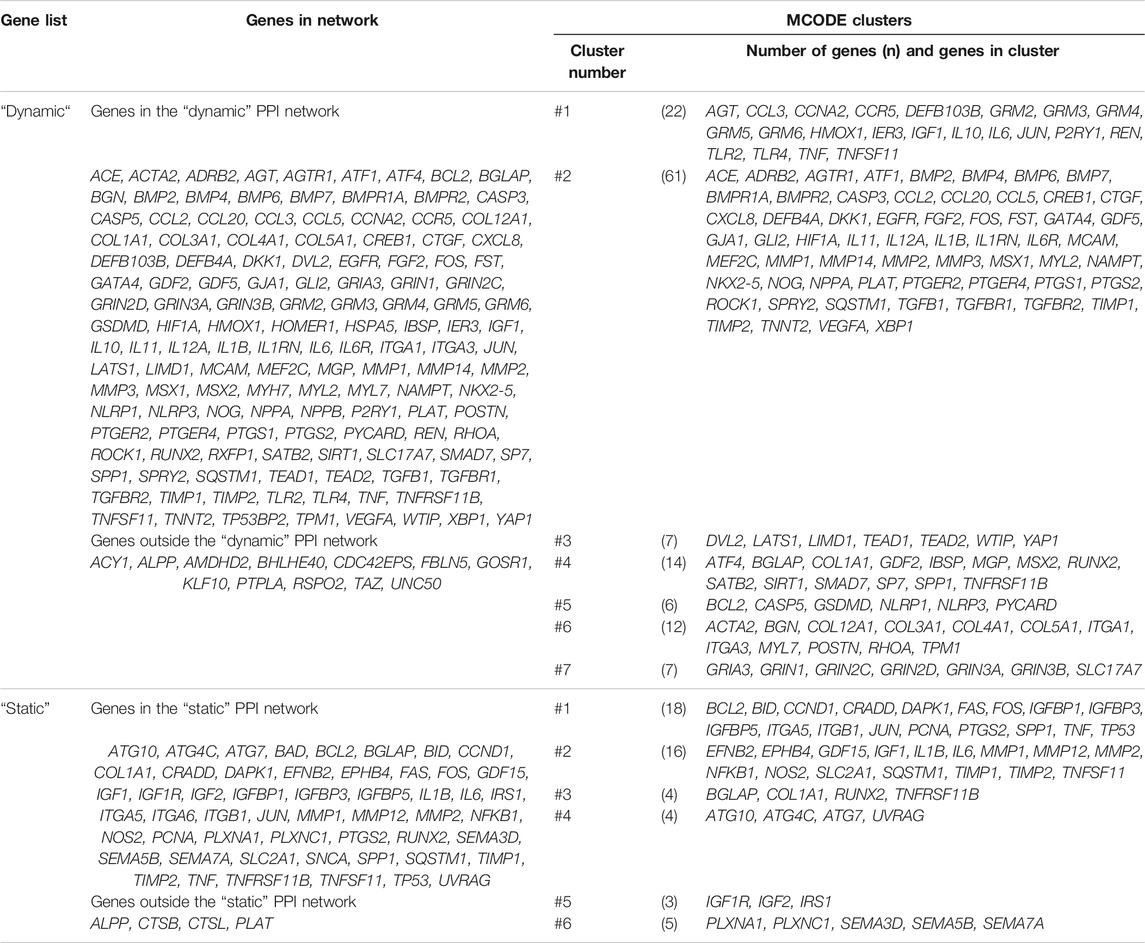
TABLE 4. Differential expressed genes (DEGs) from the “dynamic” and “static” gene lists and their affiliation to the “dynamic” and “static” protein-protein interaction networks and one of the MCODE clusters. The corresponding networks and clusters were depicted in Figure 5.
Biological processes and signaling pathways involved in the regulation of gene expression after dynamic and static tension application on hPDLCs were analyzed querying the “Biological Process” subsection of GeneOntology (GO) and GeneAnalytics’ SuperPathway database. The top 10 enriched terms from the “dynamic” and “static” DEG lists were ranked according to log10(FDR) for GO or the SuperPathway’s score, respectively. Only GO terms containing at least 5% of the genes from the particular DEG list (ratio ≥0.05) were considered (Supplementary Table S8).
Dynamic DEG list. The most significant GO terms describing biological processes were related to “responses to endogenous stimuli” (ratio: 0.05/log10(FDR) = 36.16; 0.05/30.14), the action of growth factors (0.09/30.14; 0.09/28.91), ossification (0.15/29.10) or differentiation (0.06/27.36) and motility/movement (0.06/27.80; 0.06/27.77; 0.06/27.54) (Supplementary Table S8.1). SuperPathways analysis revealed high scores of the more general ERK (ratio: 0.05/score: 115.97), Akt (0.06/96.02), and PAK (0.06/95.94) signaling pathways (Supplementary Table S8.2). Lower scores but higher ratios were attributed to “Interleukin-4 and 13 signaling” (0.18/70.16) and the “Hippo signaling pathway” (0.14/66.29). Nevertheless, “Lung Fibrosis” SuperPathway (0.27/70.05) was also part of the “top 10 list” of enriched terms.
Static DEG list. The most significant GO terms describing biological processes driven by genes from the “static” DEG list were ossification (0.06/10.08), responses to mechanical stimuli (0.05/8.97; 0.10/8.40), regulation by glucocorticoids (0.06/8.91; 0.07/7.99), positive regulation of small molecule metabolic processes (0.07/8.21), and apoptotic signaling pathways (0.09/7.88; 0.19/7.54) (Supplementary Table S8.3). SuperPathways related to apoptosis (0.15/76.37) and autophagy (0.07/62.14; 0.03/53.00) but also to ERK signaling (0.02/62.14), cell adhesion/ECM remodeling (0.20/61.73) and interleukin-4 and 13 signaling (0.11/56.77) were significantly enriched (Supplementary Table S8.4 in Supplementary Table S8).
To identify highly connected gene clusters within each network, MCODE clustering was performed. Seven different clusters were identified in the dynamic PPI network (Figure 5C; Table 4) and six clusters in the static PPI network (Figure 5D; Table 4). Cluster #7 of the “dynamic” network consisted of seven genes, which were included in this DEG list based on one study only (Fujihara et al., 2010) (Figure 5C). This cluster was not further analyzed. All other clusters were re-analyzed concerning GO/Biological Process terms and SuperPathway enrichment (Supplementary Table S8). The same ordering and ratio cut-off were applied as above.
Generally, the identified clusters showed a higher ratio of included genes than the whole network (Supplementary Table S8) independent of the database used. For example, cluster #1 from the dynamic network was described significantly as “adenylate cyclase-inhibiting G protein-coupled glutamate receptor signaling pathway”-related (0.56/8.97). Cluster #3 from the same network was described significantly by the “ionotropic glutamate receptor signaling pathway” (0.24/14.10), but also by “excitatory chemical synaptic transmission” (0.38/6.97), whereas cluster #4 was dominated by terms related to bone mineralization and remodeling, e.g. “osteoblast differentiation” (0.10/12.50) and development (0.22/6.80), or “regulation of bone resorption” (0.08/4.09). In general, an increase in specificity (i.e. higher ratio) was also observed for SuperPathways enrichment (Supplementary Tables S8.2,S8.4 in Supplementary Table S8).
Essential nodes (i.e. hub genes) in both networks were identified with cytoHubba. Within the network derived from the dynamic DEG list, eleven hub genes were identified (Table 5: IL6, CXCL8, IL1B, TNF, CCL2, IL10, VEGFA, HMOX1, JUN, PTGS2, TLR4; red labeled nodes in Figures 5A,C). Altogether, ten hub genes were identified in the network generated from the static DEG list (Table 5: IL6, IGF1, TP53, TNF, MMP2, JUN, FOS, IL1B, MMP1, SPP1; red labeled nodes in Figures 5B,D). In both networks, the hub genes were either located in cluster #1 or #2 of the particular network.
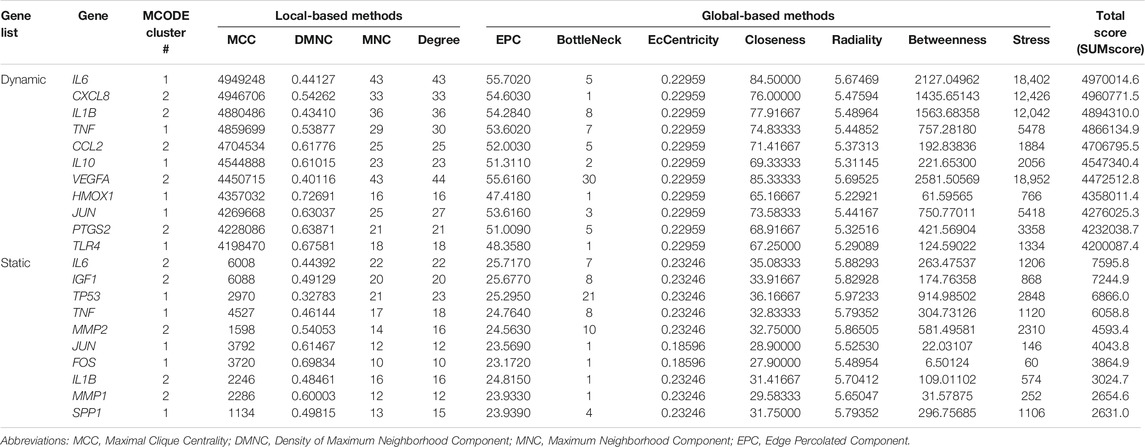
TABLE 5. Top hub genes identified in both STRING networks derived from the “dynamic” (n = 147) and “static” (n = 57) tension DEG lists. Different score measures were calculated by cytoHubba (Chin et al., 2014). The total score cut-off was set to ≥ 2*mean total score of each network being 361511.0 for the “dynamic” network and 1209.0 for the “static” network. In both lists the hub genes were sorted in descending order according to the total score. These hub genes were colored red in Figure 5.
The expression of five of eleven hub genes (VEGFA, JUN, TNF, IL6, HMOX1) included into the “dynamic” PPI network was upregulated by dynamic tension forces. For the remaining six hub genes both up- and downregulation of gene expression was reported. For eight out of ten hub genes (SPP1, JUN, TP53, TNF, FOS, IL1B, MMP1 and MMP2) upregulated expression was observed after exposure to static tension forces, and for the remaining two genetic loci (IL6 and IGF1) both up- and downregulated expression was reported.
Discussion
This systematic review aimed to identify and analyze studies applying tension forces to human periodontal ligament cells (hPDLCs) and to delineate the impact of different force parameters on the expression of relevant genes. Risk of bias assessment was conducted by application of published criteria using clear definitions. The commonly investigated genes, proteins, and metabolites were summarized and analyzed by gene enrichment and pathway analysis.
Commonly Used Force Apparatuses
To apply tension type of force on hPDLCs in vitro three different major groups of apparatuses were identified here: 1) commercially available systems. i.e. the Flexcell® Tension System or the STREX® Cell Stretching System, 2) self-designed apparatuses using commercially available components (e.g. Bioflex® or Uniflex® plates, petriPERM® or Lumox® dishes) as a central part of a stretching device, and 3) solely self-constructed apparatuses. Irrespective of the particular design, cells were grown on a flexible surface undergoing cyclic (or static) equibiaxial or uniaxial stretching in all devices.
According to this review, the Flexcell® Tension System was the most widely adopted apparatus for equibiaxial tension using Bioflex®, and uniaxial tension using Uniflex® cell culture plates. In various reports the mechanical characteristics of these elastic membranes used to apply tension to adherent cells have been studied and limitations of this method have been reported. Specifically, several studies reported a heterogeneous strain distribution within the surface used for cell cultivation and a considerable interference of other types of force (e.g. compression and shear stress) (Gilbert et al., 1994; Vande Geest et al., 2004; Matheson et al., 2006). In the Flexcell® Tension system using Bioflex® plates without a biaxial loading post, equibiaxial strain was mostly focused in the center of the membrane, whereas almost pure uniaxial strain was found at the rigid rim of the well (Gilbert et al., 1994). We consider this setup to be similar to several self-designed apparatuses using Bioflex® plates. As such, we propose, that the heterogeneous strain distribution might also apply to these setups. In contrast, in Flexcell Tension systems using Bioflex® plates with a “biaxial loading post” the constant biaxial strain region was located in the membrane area on the post, whereas off-post large radial strain was produced (Vande Geest et al., 2004). Application of uniaxial strain with Flexcell’s Uniflex® culture plates using an uniaxial loading post results in almost uniform strain distribution in the membrane area on-post (Matheson et al., 2006). Irregular strain distribution might occur, anyhow, comparing longitudinal and transverse orientation together with a certain amount of compressive strain (Matheson et al., 2006). As a consequence of the heterogenous strain distribution, only some of the cells receive the desired mechanical stimulation (Matsuda et al., 1998) and thus gene expression reflects the average of all cells in the examined area. Irrespective of the not entirely standardized strain parameters, the tension systems identified herein have proven to be efficient models to mimic the in vivo mechanical microenvironment to investigate the related biological reactions on the cellular level. In 2017, the “BioFlex® Cell Seeder” (Flexcell Inc.) was introduced to the market to be used in combination with the BioFlex cell culture plates. Limiting cell growth within a defined area providing uniform strain distribution increases the reproducibility of cell seeding and exposure against mechanical cues. However, none of the included studies reported the application of this device.
The PDL comprises different types of collagen, including type I (about 80%), type III (15%), type V, type VI, and type XII collagen (Berkovitz et al., 2009, pp. 179–180). Most frequently BioFlex plates pre-coated with collagen type I were used, but pronectin coating was also reported (Jacobs et al., 2013). Other silicone-based membranes were used together with coatings of gelatin (Tantilertanant et al., 2019a; b) or collagen type I and/or fibronectin (Konstantonis et al., 2014; Papadopoulou et al., 2019). Further studies did not report any coating, particularly when using PetriPerm or Lumox dishes. Diercke et al. (2011) applied a combination of collagen type-I and fibronectin to coat the PetriPerm dishes. Commonly coatings were applied to increase the biocompatibility of the membrane surface.
Most recently, three-dimensional (3D) cell culture studies have reached increasing significance since they allow for a better simulation of the cells’ extracellular matrix (ECM) and in vivo microenvironment. Providing structural support and signal transduction to the cells, ECM is involved in various biological processes, including cell migration, proliferation, differentiation and intercellular communication (Dieterle et al., 2021). For simulation matrices made from collagen, polylactic-co-glycolic acid (PLGA) and hydrophilically modified poly-L-lactide (PLLA) are used in cytomechanics (Yang et al., 2015; Janjic et al., 2018). Though 2D coating resembles the in vivo situation more than tissue culture plastic alone, differences in porosity, microarchitecture and local rigidity in comparison to 3D substrates effect cell migration (Doyle et al., 2015) and mechanotransduction (Yang et al., 2015).
Interestingly, only two studies reported on the effects of tension force applied to cells within 3D scaffolds (Von den Hoff, 2003; Ku et al., 2009). The first one focused on the assessment of tension force exerted to the substrate by the cells (Von den Hoff, 2003). With only one qualified study (Ku et al., 2009) a comparison between 2D and 3D cell culture setups seemed not conclusive. Yang et al. (2015) emphasized that 3D cell culture techniques are yet not standardized and suggested to establish tissue specific scaffolds along with the identification of appropriate cell densities. The insufficient knowledge on 3D scaffolds might explain its infrequent use to study the effect of tension force on hPDLCs.
Rationale for Force Parameters
Tension type of force was applied either statically or dynamically on hPDLCs. As such, the selection of the relevant model parameters (cell type, type of force and its duration, magnitude and frequency) was mostly based on the purpose of the specific study, being either the simulation of a clinical situation (occlusal forces or OTM), or to investigate the force-related expression of specific genes, group of genes or pathways. Due to the general objectives of the studies analyzed herein, the selection of cell type and type of force seems plausible. The remaining parameters were selected according to: 1) in vivo evidence from animal models simulating OTM or measurement of bite force in human subjects (e.g. He et al., 2004; Fujihara et al., 2010; Li et al., 2013; Li et al., 2014; Ren et al., 2015; Tantilertanant et al., 2019a); 2) finite element analysis to study the biomechanical behaviour of the PDL (e.g. Howard et al., 1998; Kletsas et al., 2002; Konstantonis et al., 2014; Chen et al., 2015); 3) in vitro studies defining an “optimal” window to study the expression of specific genes or pathways (e.g. Basdra et al., 1996; Hao et al., 2009; Huelter-Hassler et al., 2017; Hülter-Hassler et al., 2017).
Force duration: Continuous exposure to a stretching force is considered as an appropriate surrogate for in vivo forces applied by fixed appliances during OTM (Ziegler et al., 2010; Steinberg et al., 2011). As such, time intervals for application were chosen reflecting different stages in OTM or other clinically relevant conditions (Ziegler et al., 2010; Goto et al., 2011; Steinberg et al., 2011). According to our results, the maximum force duration varied between 0.5 h and 15 days. The most commonly used force duration in dynamic tension experiments were either 48 h or 72 h, and static tension was most commonly applied for 12 h. Under experimental in vitro conditions the maximum application time is, in fact, limited by the feeding intervals of the cells and the apparatus used. With static tension, cell proliferation should be considered as an influencing factor – especially during long-term force application. Cells undergoing cell division might transiently loose contact to the stretched surface. After reattachment to the already deformed surface they might not be further subjected to stretching force.
Force magnitude: Similar to force duration, force magnitude is a relevant experimental parameter for simulation of both, dynamic and static tension forces. Based on the studies considered herein, force magnitude selection was again mostly related to the objective of the study: 1) expression of a specific gene in response to tension force application allowing to define the dynamic range along with the range of optimum forces (e.g. Long et al., 2002; Agarwal et al., 2003), and 2) to simulate a clinical situation (e.g. Long et al., 2002; Li et al., 2014). In most studies, 10% dynamic tension was applied to mimic the physiologic conditions of occlusal force or OTM (e.g. Fujihara et al., 2010; Li et al., 2014; Ren et al., 2015). This force magnitude was based on in vivo studies, which either focused on tissue remodeling and tooth movement after exposure to different levels of orthodontic force (King et al., 1991; Gonzales et al., 2008), or studies on tooth mobility in response to different force levels (Mühlemann, 1954; Mühlemann and Zander, 1954). Several studies (Howard et al., 1998; Kletsas et al., 2002; Konstantonis et al., 2014; Chen et al., 2015) took results from finite element analysis into consideration to select the force magnitude best corresponding with the real clinical situation (Andersen et al., 1991; Natali et al., 2004; Dong-Xu et al., 2011).
Force frequency: To apply dynamic tension different frequencies were adopted. Selection of appropriate force frequencies mostly relied on two different rationales: 1) experience from previous in vitro studies either using similar setups or defining appropriate frequency ranges to study the expression of specific genes (Yamaguchi et al., 1994; Long et al., 2002; Ma et al., 2015; e.g.; Ren et al., 2015; Memmert et al., 2020), and 2) deduction from the real clinical situation, e.g. tooth contact rates during sleep (He et al., 2004) or an average masticatory cycle (Tantilertanant et al., 2019a). In this context various estimates of contact rates were reported so far, e.g. contacts ranging from 17.2 to 104.3 contacts/h (Yamashita et al., 1993), a mean frequency of masticatory cycle of ∼70 rpm (Pini et al., 2002), or 1.5–2 Hz (Woda et al., 2006).
According to our review, the most commonly used frequency was 0.1 Hz for dynamic equibiaxial tension and 0.5 Hz for dynamic uniaxial tension. Although the real frequency of tension applied on the PDL cells during OTM yet remains unknown (Long et al., 2002; Padial-Molina et al., 2013; Wang Y. et al., 2019), the clinical and technical evidence summarized in this review will provide clues for design of related experiments.
Most Frequently Investigated Genes, Proteins and Metabolites
To identify the most relevant mechanical responses during OTM, we focused on the top 10 most frequently investigated genes, proteins and metabolites: RUNX2, ALPP, BGLAP, IL1B, PTG2, TNFRSF11B, TNFSF11, COL1A1, PGE2 and SP7 (Supplementary Table S6). According to their functional contribution we grouped these genes into three categories: genes related to osteogenesis (RUNX2, SP7, ALPP, BGLAP, COL1A1), osteoclastogenesis (TNFRSF11B, TNFSF11), and inflammation (IL1B, PTGS2, PGE2).
Osteogenesis
RUNX2 regulation is an integral and central part in the development and remodeling not only of osseous tissue but also of the periodontal ligament (Ziros et al., 2008). PDL cells are capable of differentiation into osteoblasts or cementoblasts in response to mechanical stimulation (Ziros et al., 2002). RUNX2 upregulation was reported in 19/26 (73%) studies using either RT-qPCR or both RT-qPCR and western blotting. Decrease, temporary changes or other types of regulation were found in the remaining studies (7/16, ∼27%). Although several studies revealed partially contradictory results, it was well-supported that RUNX2 expression increased within the first 12 h of tension application.
Another essential transcription factor in the osteogenic pathway acting downstream of RUNX2 is SP7 (also known as Osterix), which belongs to the zinc finger-containing transcription factor SP family (Tang et al., 2012; Li et al., 2013; Li et al., 2015). Significant gene and/or protein upregulation of SP7 in response to tension was reported in 9/10 (90%) of the relevant studies, whereas only one study reported downregulation of gene expression and temporary upregulation of the corresponding protein (Li et al., 2013). The authors concluded, that this difference might be due to complex regulation mechanisms and modifications occurring during its transcription and translation (Li et al., 2013).
Alkaline phosphatase (ALPP) also plays a crucial role in the initiation of osteogenic differentiation and bone remodeling (Chen et al., 2014). In all of the 24 relevant studies, ALPP gene expression was determined using either semiquantitative or quantitative PCR. ALPP protein was quantified in cell lysates using western blots, ELISA or enzyme activity assays. Significant upregulation in response to tension <12% was reported in 17/24 (71%) of the studies, mostly during the first 12 h of force application.
As the most abundant non-collagenous bone-matrix protein, bone gamma-carboxy glutamic acid-containing protein (BGLAP; osteocalcin) is a late marker of osteoblast differentiation and mineralization (Chen et al., 2014). Significant upregulation of BGLAP in response to tension was reported in 16/18 (89%) of the studies. In contrast, one study reported a slight decrease in BGLAP gene expression and it was assumed that an enhanced cell proliferation of young osteoblasts might be responsible (Jacobs et al., 2013). Another study described a transient upregulation of BGLAP following 3 h of exposure against tension force (Qin and Hua, 2016).
The extracellular matrix (ECM) of the periodontal ligament mainly consists of fibrillar collagens, among which type I collagen accounts for ∼75% (Kaku and Yamauchi, 2014). The latter is composed of alpha-1 (COL1A1) and alpha-2 (COL1A2) type I collagen chains. COL1A1 is confirmed to be essential for bone remodeling and osteoblastic differentiation in response to tension during OTM (Birkedal-Hansen et al., 1977; Jacobs et al., 2013). Significant upregulation of protein expression was found in 8/13 (62%) of related studies after exposure to ≤12% of tension. Contradicting expression patterns have been reported, which might be due to the heterogeneity of the PDL cells used, digestion of COL1A1 by MMP1 (Nemoto et al., 2010), or the inhibitory effect of IL1β and TNFα on COL1A1 gene expression (Sun et al., 2017). Although inconsistencies were partially found, upregulation of COL1A1 in response to tension was confirmed in the majority of the studies considered here.
Osteoclastogenesis
Bone remodeling is primarily regulated by a closely interrelated system of receptors and mediators including TNFSF11 (receptor activator of nuclear factor kappa ligand; RANKL), its cellular receptor, receptor activator of NF-kappaB (RANK), and TNFRSF11B (osteoprotegerin, OPG) ultimately maintaining the balance between osteogenesis and osteoclastogenesis (Krishnan et al., 2015). As a decoy receptor for RANKL, OPG suppresses the binding between RANKL and RANK and thus inhibits osteoclastogenesis and bone resorption (Liao and Hua, 2013).
Upregulation of OPG gene expression and/or protein synthesis was reported in 11/14 (79%) of the included studies. Due to the heterogeneity of force parameters, the correlation between gene expression, force duration and/or force magnitude can only partly be defined. The regulation of RANKL expression showed large variability in comparison to OPG: only 57% (8/14) of the relevant studies reported an upregulation of both gene and protein expression after force application, whereas the remaining studies showed inconsistent expression patterns.
It is commonly accepted, that an increased RANKL/OPG ratio favours osteoclastogenesis, meaning an upregulation of RANKL in parallel with a downregulation/lower induction of OPG (Boyce and Xing, 2007). The RANKL/OPG ratio was reported in three studies included into this review (Nogueira et al., 2014b; Konstantonis et al., 2014; Jacobs et al., 2015). Two of these studies identified a decreasing RANKL/OPG ratio compatible with a more intensive bone formation after exposure to minor tension forces (≤10%): a temporary decrease of the RANKL/OPG ratio was found by Jacobs et al. (2015) using RT-qPCR, whereas Liao and Hua (2013) reported an increasing OPG/RANKL ratio for both, gene and protein expression. Higher tension forces (20%) were reported to induce an increased RANKL/OPG ratio at both gene and protein levels indicating net bone resorption (Nogueira et al., 2014b).
Inflammation
The response of the PDL to mechanical stress has been characterized as an aseptic transitory inflammatory process, which is regulated by various mediators, including cytokines and chemokines (Lee et al., 2012). Interleukin 1β (IL1B) is an upstream cytokine involved in many inflammatory processes (Lee et al., 2012) and in osteoclast formation, differentiation and activation (Long et al., 2001). Upregulation was reported in 75% (12/16) of the relevant studies depending on the particular duration and magnitude of force application. A reduced expression or inconsistent expression patterns during exposure to forces of various magnitudes and durations were reported in the remaining 4/16 (25%) studies. These differences have been mainly attributed to the particular magnitude of tension: a lower magnitude attenuates the inflammatory response, while a higher one elicits inflammation (Long et al., 2001).
Prostaglandin-endoperoxide synthase 2 (PTGS2; also known as COX2) is known as a key regulator enzyme of the eicosanoid biosynthesis pathway and is thus also involved in prostaglandin E2 (PGE2) synthesis (Nogueira et al., 2014b). Amongst others, the activity of PTGS2 and the synthesis of PGE2 is particularly amplified by pro-inflammatory stimuli including IL1B (Nogueira et al., 2014b). PGE2 mediates bone resorption under physiological and pathological conditions, and is centrally involved in both, the response of periodontal tissue to mechanical stress and the pathogenesis of periodontitis (Shimizu et al., 1998). Herein, PTGS2 gene expression and PGE2 synthesis were reported in 14 and 12 of the included studies, respectively. Of these studies, 9 focused on PTGS2 and PGE2, among which 8/9 studies (∼89%) found an increasing transcription of the PTGS2 gene and/or PGE2 concentration after mechanical stimulation correlating with force duration and force magnitude. The data of the remaining studies revealed mixed expression patterns of PTGS2 and PGE2 activity after force exposure, which might be attributable to anti-inflammatory effects of lower tension forces (Long et al., 2002). Taken together PTGS2 expression and PGE2 production is induced by the exposure of cell cultures to tensions forces and it is positively correlated to force duration and force magnitude.
Reasons for Heterogeneity of Genes/Proteins/Metabolites Regulation
Considering the list of the most commonly considered genes, inconsistencies between gene and protein expression were found in several reports. The observed non-proportional relationship between gene expression and protein activity can be attributed to the time lag between transcription and translation. This time lag might be prolonged by post-transcriptional processing and degradation of the transcripts, as well as post-translational modifications like phosphorylations (e.g. Li et al., 2013; Ren et al., 2015) or proteolytic cleavage (e.g. Wang et al., 2013; Zhuang et al., 2019). The experimental heterogeneity identified among different studies reporting force-related expression of the same genes can be attributed to: 1) donor-related issues (e.g. age of donor), 2) hPDLC isolation-related issues, 3) cell culture of hPDLCs (e.g. cell culture medium, passage number), 4) reference gene selection in (s)qPCR experiments, and 5) heterogeneity of force parameters. In addition, the pooling of cells from different donors (Stoddart et al., 2012), the seeding density of cells and thus the amount of confluency and the different cell culture media used might have caused heterogeneity of results.
All studies included herein isolated hPDLCs from teeth that have been removed due to orthodontic reasons. When considering all studies the age of donors ranged from 8 to 40 years, but within each study the donors had the same age. This is even more important, since the phenotype of hPDLCs, specifically the proliferation rate, osteogenic potential or in vitro life span clearly depends on the age of the donor (Marchesan et al., 2011). Moreover, force-related gene expression has been reported to be significantly dependent on the age of the donor (Mayahara et al., 2007). Accordingly, many studies reported considerable functional inconsistencies between different cell samples most likely caused by the biological heterogeneity among different donors (Monnouchi et al., 2011; Yuda et al., 2015; Papadopoulou et al., 2017; Arima et al., 2019). For experimental simulation of the age, several studies focusing on cellular senescence simply used different passage numbers, supposing a correlation between the passage number and the age. Thus, they designated passage numbers ranging from 3 to 7 as “young” and those ranging from 18 to 24 as “old” or “senescent” (Shimizu et al., 1997; Abiko et al., 1998; Ohzeki et al., 1999; Miura et al., 2000; Konstantonis et al., 2014). Generally, passage numbers of hPDLCs used in the included studies ranged between 2 and 15 (Supplementary Table S2). A maximum passage number of 20 was reported in a study using limited dilution cloning (Long et al., 2001). Differences in morphology and biological activity between early and late passages were reported, with the early passages resembling fibroblasts characteristic of original tissue more closely (Marchesan et al., 2011). Therefore, the use of early passage (passage ≤7) of primary culture is recommended to maintain most of the original cell phenotype (Marchesan et al., 2011).
Exclusively all studies analyzed herein applied tension force to human periodontal ligament cells (hPDLCs). These cells were commonly isolated from the middle third of the roots from teeth removed due to mostly orthodontic reasons using two different cell isolation techniques, i.e. the “explant” (Brunette et al., 1976; Somerman et al., 1988) or the “digestion” technique (Brunette et al., 1976; Seo et al., 2004). Different terms and abbreviations were used to identify these cells, including “hPDLF”, hPDL-fibroblasts”, “hPDL fibroblasts”, “hPDLC”, “hPDLCs”, “hPDL cells”, “hPDLSCs” and “hPDLS cells”. In order to identify and include all relevant studies on this topic into this review, the search strategy considered all terms and abbreviations identified. In all cases, PDL tissue derived from premolars or third molars. Both isolation techniques unequivocally result in a heterogeneous mixture of different cell types (Yamaguchi et al., 2002; Marchesan et al., 2011), though the “digestion” technique was shown to result in a cell population enriched with mesenchymal stem cells (Seo et al., 2004). Therefore, hPDLCs should be regarded as a heterogeneous cell population consisting of cells originating from different lineages. Most recently, in-vitro cell type verification was increasingly used including several studies on hPDLCs (Marchesan et al., 2011), that were also reported in some of the included studies herein, i.e. flow cytometric analysis of cell surface markers (Wang H. et al., 2019; Wu et al., 2019a), osteogenic potential as reflected by ALP staining and/or Ca2+ deposition (Jacobs et al., 2018; Wang H. et al., 2019), cell type specific gene expression pattern (Memmert et al., 2019), and immunohistochemical staining of vimentin and cytokeratin (Sun et al., 2017; Yu et al., 2018; Wang Y. et al., 2019).
Careful reference gene selection is essential to overcome variations in RT-qPCR experiments and to enhance comparability between various studies. In order to reduce the risk of bias in qPCR experiments, the “Minimum Information for Publication of Quantitative Real-Time PCR Experiments (MIQE)” guidelines were established, with reference gene selection being one of the most crucial steps in RT-qPCR establishment (Bustin et al., 2009). As such, the MIQE guidelines cover some of the reporting and methodology-related risk of bias criteria considered in the present review, directly or indirectly related to RT-qPCR. According to the present systematic review, GAPDH or ACTB were the most frequently adopted reference genes for qPCR experiments. The rationale for reference gene selection was only rarely stated. Only recently, MIQE reporting gained more attention (Janjic Rankovic et al., 2020). Several studies evaluated reference gene selection specifically focusing on hPDLCs in different areas of dentistry (Kirschneck et al., 2017; Setiawan et al., 2019; Nazet et al., 2020).
The heterogeneity of force parameters and the limitations of several experimental set-ups as discussed previously might also have considerable impact on the consistency and comparability between studies as included herein. Additionally, optimal force duration, magnitude and frequency mainly depend on the experimental design and the specific objectives of the study.
In Silico Analysis of Gene Lists
To gain additional insights into the biological processes and pathways regulated by dynamic or static tension forces, gene-set enrichment analysis and protein-protein interaction (PPI) network construction were applied. Moreover, the most influential genes and sub-networks were identified in both PPI networks. The gene lists were created from the studies identified in this systematic review. To increase specificity, only those genes showing clear force-dependent expression were included.
Gene-set enrichment analysis: In general, gene-set enrichment analysis is applied to expression data of individual genes obtained by techniques like microarrays, next-generation sequencing or proteomics (Hutchins, 2014; Mooney and Wilmot, 2015). As such, the gene list contains ranking data (e.g. confidence scores, fold changes or similar quantitative information) or is unranked (Hung, 2013; Haw et al., 2020). Both types are then used for over-representation and/or gene-set enrichment analysis to identify relevant signaling and/or regulation pathways. To increase specificity of the gene lists, gene expression data was restricted to the criteria specified in Materials and Method. Additionally, protein expression data was excluded due to the heterogeneity of the methods used for quantification (quantification via western blotting or ELISA vs enzyme activities) and the specificity of some of the antibodies used in the immunoassays. The results of gene enrichment and the pathway analysis showed a close relationship with osteogenesis, osteoclastogenesis and apoptosis. These findings were consistent with the reporting of the relevant studies identified.
The search strategy used herein identified 18 reports applying tension to hPDLCs with subsequent microarray or RNA-seq analysis: 15 studies applied dynamic tension and 3 static tension. All studies reported the most significantly up- and down-regulated genes applying different cut-offs. A re-analysis was not possible, since full (raw) data was publicly not available. Nevertheless, data from these studies was included if qualified reanalysis using (s)qPCR or protein expression was reported additionally.
Protein-Protein Interaction Networks
Complementary to pathway enrichment analysis, protein-protein interaction networks were generated for dynamic and static tension gene sets using the “Search Tool for the Retrieval of Interacting Genes/Proteins” database (STRING-DB), which incorporates regularly updated data from different biological pathway and scientific literature databases (Szklarczyk et al., 2019). As such, STRING-DB not only contains data on experimental derived PPI, but also functional annotation from literature and computational predictions. For each individual PPI pair a confidence score is given (range: 0–1), with higher scores are “meant to express an approximate confidence, […], of the association being true, given all the available evidence” (Szklarczyk et al., 2019; p. D608). Both networks were analyzed regarding subclusters and most-influential nodes (i.e. hub genes) and 7 (dynamic) and 6 (static) subclusters were identified. Subsequent pathway enrichment analysis showed a more specific enrichment of GO/Biological Process terms and GeneAnalytics SuperPathways.
We applied a confidence score cut-off ≥ 0.700, thus only high confidence interactions were included. Interestingly, in both networks several differentially expressed genes were not integrated, including alkaline phosphatase (ALPP), being one of the most frequently analyzed genes/proteins identified herein. With the application of a confidence score cut-off of ≥0.400, thus including medium confidence interactions, ALPP was integrated into dynamic network due to co-mentioning in PubMed abstracts with bone sialoprotein 2 (IBSP; score: 0.407), osteopontin (SPP1; 0.438), bone morphogenetic protein 2 (BMP2; 0.434), RUNX2 (0.495), and osteocalcin (BGLAP; 0.627). GOSR1, CDC42EP2, UNC50, ACY1, and AMDHD2 were still not integrated. Application of the same threshold to the static network would integrate all nodes including ALPP (ALPP – RUNX2: 0.495; ALPP – BGLAP: 0.627; ALPP – SPP1: 0.438).
Interestingly, cluster #7 of the dynamic network consisted of nodes, that were contributed to the gene list based on one study (Fujihara et al., 2010). This finding demonstrates the limits of the approach applied: both lists of differentially expressed genes were compiled based on publications identified in our search strategy. The more specific an individual study deals with a specific aspect, the more specific it will be described by gene-list enrichment analysis and network cluster analysis. As such, measures were taken, to reduce this impact: 1) the genes included in our gene lists were those only with reports on changes in gene expression due to mechanical stimulation. 2) Additionally, cut-offs were applied to GO, pathway enrichment and PPI network construction, to exclude incorporation of data too general, that means very general biological processes with thousands of genes involved.
Meta-Analysis
Initially, a meta-analysis of the ten most frequently analyzed genes or metabolites was intended to supplement the findings. Unfortunately, due to heterogeneity of the experimental conditions, including force parameters, cell culture, reference gene selection in RT-qPCR experiments and incomplete reporting especially concerning the statistical unit, this was not further considered.
Several identified studies showed that not only gene and protein expression is regulated by tension application, but also post-translational modifications like proteolytic cleavage, activation by GTP-binding, phosphorylations and protein translocation between nucleus and cytoplasm, or cytoplasm and extracellular space. Regulation of second messengers like cAMP (Ngan et al., 1990) and metabolites like glutamate (Fujihara et al., 2010), NOx (Pelaez et al., 2017) and ATP (Tantilertanant et al., 2019a) are also effected by tension application, as well as microRNA and long non-coding RNAs (Chen et al., 2016). Epigenetic effects on gene expression also should be taken into account, since several genes discussed herein like COL1A1 (Kaku and Yamauchi, 2014) and RUNX2 (Montecino et al., 2015) are known to be under epigenetic control (Francis et al., 2019).
Summary
In this systematic review we summarized relevant information about tension application on hPDLCs in vitro and assessed potential reporting and methodology-associated risk of bias related to this issue. Due to the enormous variety of apparatus in both, dynamic and static tension experiments, it is not possible to universally define optimum force parameters including force magnitude, duration and frequency. However, clinically relevant parameters were identified, that can be used as a reference for in vitro studies. Taken together, quantitative and qualitative information on mechanical stimulated gene and protein regulation and a comprehensive network analysis have provided more clear insights into the mechanisms involved in the OTM.
Future studies should focus on the comparison of dynamic and static tension. There is also a need to elucidate the differences between the application of equibiaxial and uniaxial tension in more detail, to develop an optimal in vitro model for the simulation of orthodontic force, and to provide more reliable evidence for clinical treatment.
Data Availability Statement
The original contributions presented in the study are included in the article/Supplementary Material. Further inquiries can be directed to the corresponding author.
Author Contributions
Conceptualization: UB, CS, MJR; Methodology: UB, CS, MJR, SO; Formal analysis and investigation: CS, MJR, UB; Writing-original draft preparation: CS, MJR, UB; Writing-review and editing: CS, MJR, UB, SO, MF, AW; Resources: UB, CS, MJR, AW; Supervision: UB.
Funding
CS was supported by a grant from the China Scholarship Council (CSC File No 201809370043).
Conflict of Interest
The authors declare that the research was conducted in the absence of any commercial or financial relationships that could be construed as a potential conflict of interest.
Publisher’s Note
All claims expressed in this article are solely those of the authors and do not necessarily represent those of their affiliated organizations, or those of the publisher, the editors and the reviewers. Any product that may be evaluated in this article, or claim that may be made by its manufacturer, is not guaranteed or endorsed by the publisher.
Acknowledgments
The authors wish to thank Dipl.-Ing. Thomas Stocker (Biomechanics Laboratory, Department of Orthodontics and Dentofacial Orthopedics, University Hospital, LMU Munich) for helpful discussions during the preparation of the manuscript.
Supplementary Material
The Supplementary Material for this article can be found online at: https://www.frontiersin.org/articles/10.3389/fbioe.2021.695053/full#supplementary-material
References
Abiko, Y., Shimizu, N., Yamaguchi, M., Suzuki, H., and Takiguchi, H. (1998). Effect of Aging on Functional Changes of Periodontal Tissue Cells. Ann. Periodontol. 3 (1), 350–369. doi:10.1902/annals.1998.3.1.350
Agarwal, S., Long, P., Al, S., Piesco, N., Shree, A., and Gassner, R. (2003). A central Role for Nuclear factor‐κB Pathway in the Antiinflammatory and Proinflammatory Actions of Mechanical Strain. FASEB J. 17 (8), 1–15. doi:10.1096/fj.02-0901fje
Andersen, K. L., Pedersen, E. H., and Melsen, B. (1991). Material Parameters and Stress Profiles within the Periodontal Ligament. Am. J. Orthod. Dentofacial Orthopedics 99 (5), 427–440. doi:10.1016/s0889-5406(05)81576-8
Arima, M., Hasegawa, D., Yoshida, S., Mitarai, H., Tomokiyo, A., Hamano, S., et al. (2019). R-spondin 2 Promotes Osteoblastic Differentiation of Immature Human Periodontal Ligament Cells through the Wnt/β-Catenin Signaling Pathway. J. Periodont Res. 54 (2), 143–153. doi:10.1111/jre.12611
Ashburner, M., Ball, C. A., Blake, J. A., Botstein, D., Butler, H., Cherry, J. M., et al. (2000). Gene Ontology: Tool for the Unification of Biology. Nat. Genet. 25 (1), 25–29. doi:10.1038/75556
Bader, G. D., and Hogue, C. W. (2003). An Automated Method for Finding Molecular Complexes in Large Protein Interaction Networks. BMC Bioinformatics 4, 2. doi:10.1186/1471-2105-4-2
Banes, A. J., Gilbert, J., Taylor, D., and Monbureau, O. (1985). A New Vacuum-Operated Stress-Providing Instrument that Applies Static or Variable Duration Cyclic Tension or Compression to Cells In Vitro. J. Cell Sci. 75, 35–42. doi:10.1242/jcs.75.1.35
Basdra, E. K., Kohl, A., and Komposch, G. (1996). Mechanische Dehnung von Desmodontalfibroblasten - eine Untersuchung zur zytoskelettalen Beteiligung. J. Orofac Orthop/Fortschr Kieferorthop 57 (1), 24–30. doi:10.1007/BF02189045
Baumert, U., Golan, I., Becker, B., Hrala, B. P., Redlich, M., Roos, H. A., et al. (2004). Pressure Simulation of Orthodontic Force in Osteoblasts: a Pilot Study. Orthod. Craniofac. Res. 7 (1), 3–9. doi:10.1046/j.1601-6335.2003.00270.x
Ben-Ari Fuchs, S., Lieder, I., Stelzer, G., Mazor, Y., Buzhor, E., Kaplan, S., et al. (2016). GeneAnalytics: An Integrative Gene Set Analysis Tool for Next Generation Sequencing, RNAseq and Microarray Data. OMICS: A J. Integr. Biol. 20 (3), 139–151. doi:10.1089/omi.2015.0168
Berkovitz, B. K. B., Holland, G. R., and Moxham, B. J. (2009). Oral Anatomy, Histology and Embryology. 4th Ed. Edinburgh: Mosby.
Birkedal-Hansen, H., Butler, W. T., and Taylor, R. E. (1977). Proteins of the Periodontium. Calcif. Tissue Res. 23 (1), 39–44. doi:10.1007/BF02012764
Boyce, B. F., and Xing, L. (2007). Biology of RANK, RANKL, and Osteoprotegerin. Arthritis Res. Ther. 9 (Suppl. 1), S1. doi:10.1186/ar2165
Brunette, D. M., Melcher, A. H., and Moe, H. K. (1976). Culture and Origin of Epithelium-like and Fibroblast-like Cells from Porcine Periodontal Ligament Explants and Cell Suspensions. Arch. Oral Biol. 21 (7), 393–400. doi:10.1016/0003-9969(76)90001-7
Bustin, S. A., Benes, V., Garson, J. A., Hellemans, J., Huggett, J., Kubista, M., et al. (2009). The MIQE Guidelines: Minimum Information for Publication of Quantitative Real-Time PCR Experiments. Clin. Chem. 55 (4), 611–622. doi:10.1373/clinchem.2008.112797
Chang, M., Lin, H., Fu, H., Wang, B., Han, G., and Fan, M. (2017). MicroRNA-195-5p Regulates Osteogenic Differentiation of Periodontal Ligament Cells under Mechanical Loading. J. Cell. Physiol. 232 (12), 3762–3774. doi:10.1002/jcp.25856
Chen, N., Sui, B. D., Hu, C. H., Cao, J., Zheng, C. X., Hou, R., et al. (2016). microRNA-21 Contributes to Orthodontic Tooth Movement. J. Dent. Res. 95 (12), 1425–1433. doi:10.1177/0022034516657043
Chen, Y.-J., Shie, M.-Y., Hung, C.-J., Wu, B.-C., Liu, S.-L., Huang, T.-H., et al. (2014). Activation of Focal Adhesion Kinase Induces Extracellular Signal-Regulated Kinase-Mediated Osteogenesis in Tensile Force-Subjected Periodontal Ligament Fibroblasts but Not in Osteoblasts. J. Bone Miner. Metab. 32 (6), 671–682. doi:10.1007/s00774-013-0549-3
Chen, Y., Mohammed, A., Oubaidin, M., Evans, C. A., Zhou, X., Luan, X., et al. (2015). Cyclic Stretch and Compression Forces Alter microRNA-29 Expression of Human Periodontal Ligament Cells. Gene 566 (1), 13–17. doi:10.1016/j.gene.2015.03.055
Chin, C.-H., Chen, S.-H., Wu, H.-H., Ho, C.-W., Ko, M.-T., and Lin, C.-Y. (2014). cytoHubba: Identifying Hub Objects and Sub-networks from Complex Interactome. BMC Syst. Biol. 8 (Suppl. 4), S11. doi:10.1186/1752-0509-8-S4-S11
Cho, J.-H., Lee, S.-K., Lee, J.-W., and Kim, E.-C. (2010). The Role of Heme Oxygenase-1 in Mechanical Stress- and Lipopolysaccharide-Induced Osteogenic Differentiation in Human Periodontal Ligament Cells. The Angle Orthodontist 80 (4), 740–747. doi:10.2319/091509-520.1
Cohn, S. A. (1965). Disuse Atrophy of the Periodontium in Mice. Arch. Oral Biol. 10 (6), 909–919. doi:10.1016/0003-9969(65)90084-1
Deschner, J., Rath-Deschner, B., Reimann, S., Bourauel, C., Götz, W., Jepsen, S., et al. (2007). Regulatory Effects of Biophysical Strain on Rat TMJ Discs. Ann. Anat. - Anatomischer Anzeiger 189 (4), 326–328. doi:10.1016/j.aanat.2007.02.004
Diercke, K., Kohl, A., Lux, C. J., and Erber, R. (2011). Strain-dependent Up-Regulation of Ephrin-B2 Protein in Periodontal Ligament Fibroblasts Contributes to Osteogenesis during Tooth Movement. J. Biol. Chem. 286 (43), 37651–37664. doi:10.1074/jbc.M110.166900
Dieterle, M. P., Husari, A., Steinberg, T., Wang, X., Ramminger, I., and Tomakidi, P. (2021). From the Matrix to the Nucleus and Back: Mechanobiology in the Light of Health, Pathologies, and Regeneration of Oral Periodontal Tissues. Biomolecules 11 (6), 824. doi:10.3390/biom11060824
Doncheva, N. T., Morris, J. H., Gorodkin, J., and Jensen, L. J. (2019). Cytoscape StringApp: Network Analysis and Visualization of Proteomics Data. J. Proteome Res. 18 (2), 623–632. doi:10.1021/acs.jproteome.8b00702
Dong-Xu, L., Hong-Ning, W., Chun-Ling, W., Hong, L., Ping, S., and Xiao, Y. (2011). Modulus of Elasticity of Human Periodontal Ligament by Optical Measurement and Numerical Simulation. The Angle Orthodontist 81 (2), 229–236. doi:10.2319/060710-311.1
Doyle, A. D., Carvajal, N., Jin, A., Matsumoto, K., and Yamada, K. M. (2015). Local 3D Matrix Microenvironment Regulates Cell Migration through Spatiotemporal Dynamics of Contractility-dependent Adhesions. Nat. Commun. 6, 8720. doi:10.1038/ncomms9720
Francis, M., Pandya, M., Gopinathan, G., Lyu, H., Ma, W., Foyle, D., et al. (2019). Histone Methylation Mechanisms Modulate the Inflammatory Response of Periodontal Ligament Progenitors. Stem Cells Develop. 28 (15), 1015–1025. doi:10.1089/scd.2019.0125
Fujihara, C., Yamada, S., Ozaki, N., Takeshita, N., Kawaki, H., Takano-Yamamoto, T., et al. (2010). Role of Mechanical Stress-Induced Glutamate Signaling-Associated Molecules in Cytodifferentiation of Periodontal Ligament Cells. J. Biol. Chem. 285 (36), 28286–28297. doi:10.1074/jbc.M109.097303
Gilbert, J. A., Weinhold, P. S., Banes, A. J., Link, G. W., and Jones, G. L. (1994). Strain Profiles for Circular Cell Culture Plates Containing Flexible Surfaces Employed to Mechanically Deform Cells In Vitro. J. Biomech. 27 (9), 1169–1177. doi:10.1016/0021-9290(94)90057-4
Gonzales, C., Hotokezaka, H., Yoshimatsu, M., Yozgatian, J. H., Darendeliler, M. A., and Yoshida, N. (2008). Force Magnitude and Duration Effects on Amount of Tooth Movement and Root Resorption in the Rat Molar. Angle Orthod. 78 (3), 502–509. doi:10.2319/052007-240.1
Goto, K. T., Kajiya, H., Nemoto, T., Tsutsumi, T., Tsuzuki, T., Sato, H., et al. (2011). Hyperocclusion Stimulates Osteoclastogenesis via CCL2 Expression. J. Dent. Res. 90 (6), 793–798. doi:10.1177/0022034511400742
Hao, Y., Xu, C., Sun, S.-y., and Zhang, F.-q. (2009). Cyclic Stretching Force Induces Apoptosis in Human Periodontal Ligament Cells via Caspase-9. Arch. Oral Biol. 54 (9), 864–870. doi:10.1016/j.archoralbio.2009.05.012
Haw, R., Loney, F., Ong, E., He, Y., and Wu, G. (2020). Perform Pathway Enrichment Analysis Using ReactomeFIViz. Methods Mol. Biol., 2074, 165–179. doi:10.1007/978-1-4939-9873-9_13
He, Y., Macarak, E. J., Korostoff, J. M., and Howard, P. S. (2004). Compression and Tension: Differential Effects on Matrix Accumulation by Periodontal Ligament Fibroblasts In Vitro. Connect. Tissue Res. 45 (1), 28–39. doi:10.1080/03008200490278124
Howard, P. S., Kucich, U., Taliwal, R., and Korostoff, J. M. (1998). Mechanical Forces Alter Extracellular Matrix Synthesis by Human Periodontal Ligament Fibroblasts. J. Periodontal Res. 33 (8), 500–508. doi:10.1111/j.1600-0765.1998.tb02350.x
Huelter-Hassler, D., Tomakidi, P., Steinberg, T., and Jung, B. A. (2017). Orthodontic Strain Affects the Hippo-Pathway Effector YAP Concomitant with Proliferation in Human Periodontal Ligament Fibroblasts. Eur. J. Orthod. 39 (3), 251–257. doi:10.1093/ejo/cjx012
Hülter-Hassler, D., Wein, M., Schulz, S. D., Proksch, S., Steinberg, T., Jung, B. A., et al. (2017). Biomechanical Strain-Induced Modulation of Proliferation Coincides with an ERK1/2-independent Nuclear YAP Localization. Exp. Cell Res. 361 (1), 93–100. doi:10.1016/j.yexcr.2017.10.006
Hung, J.-H. (2013). Gene Set/Pathway Enrichment Analysis. Methods Mol. Biol. 939, 201–213. doi:10.1007/978-1-62703-107-3_13
Hutchins, J. R. A. (2014). What's that Gene (Or Protein)? Online Resources for Exploring Functions of Genes, Transcripts, and Proteins. MBoC 25 (8), 1187–1201. doi:10.1091/mbc.E13-10-0602
Jacobs, C., Grimm, S., Ziebart, T., Walter, C., and Wehrbein, H. (2013). Osteogenic Differentiation of Periodontal Fibroblasts Is Dependent on the Strength of Mechanical Strain. Arch. Oral Biol. 58 (7), 896–904. doi:10.1016/j.archoralbio.2013.01.009
Jacobs, C., Schramm, S., Dirks, I., Walter, C., Pabst, A., Meila, D., et al. (2018). Mechanical Loading Increases Pro-inflammatory Effects of Nitrogen-Containing Bisphosphonate in Human Periodontal Fibroblasts. Clin. Oral Invest. 22 (2), 901–907. doi:10.1007/s00784-017-2168-1
Jacobs, C., Walter, C., Ziebart, T., Dirks, I., Schramm, S., Grimm, S., et al. (2015). Mechanical Loading Influences the Effects of Bisphosphonates on Human Periodontal Ligament Fibroblasts. Clin. Oral Invest. 19 (3), 699–708. doi:10.1007/s00784-014-1284-4
Janjic, M., Docheva, D., Trickovic Janjic, O., Wichelhaus, A., and Baumert, U. (2018). In Vitro Weight-Loaded Cell Models for Understanding Mechanodependent Molecular Pathways Involved in Orthodontic Tooth Movement: A Systematic Review. Stem Cells Int. 2018, 1–17. doi:10.1155/2018/3208285
Janjic Rankovic, M., Docheva, D., Wichelhaus, A., and Baumert, U. (2020). Effect of Static Compressive Force on In Vitro Cultured PDL Fibroblasts: Monitoring of Viability and Gene Expression over 6 Days. Clin. Oral Invest. 24 (7), 2497–2511. doi:10.1007/s00784-019-03113-6
Kaku, M., and Yamauchi, M. (2014). Mechano-regulation of Collagen Biosynthesis in Periodontal Ligament. J. Prosthodontic Res. 58 (4), 193–207. doi:10.1016/j.jpor.2014.08.003
King, G. J., Keeling, S. D., McCoy, E. A., and Ward, T. H. (1991). Measuring Dental Drift and Orthodontic Tooth Movement in Response to Various Initial Forces in Adult Rats. Am. J. Orthod. Dentofacial Orthopedics 99 (5), 456–465. doi:10.1016/s0889-5406(05)81579-3
Kirschneck, C., Batschkus, S., Proff, P., Köstler, J., Spanier, G., and Schröder, A. (2017). Valid Gene Expression Normalization by RT-qPCR in Studies on hPDL Fibroblasts with Focus on Orthodontic Tooth Movement and Periodontitis. Sci. Rep. 7 (1), 14751. doi:10.1038/s41598-017-15281-0
Kletsas, D., Basdra, E. K., and Papavassiliou, A. G. (2002). Effect of Protein Kinase Inhibitors on the Stretch-Elicited C-Fos and C-Jun Up-Regulation in Human PDL Osteoblast-like Cells. J. Cell. Physiol. 190 (3), 313–321. doi:10.1002/jcp.10052
Konstantonis, D., Papadopoulou, A., Makou, M., Eliades, T., Basdra, E., and Kletsas, D. (2014). The Role of Cellular Senescence on the Cyclic Stretching-Mediated Activation of MAPK and ALP Expression and Activity in Human Periodontal Ligament Fibroblasts. Exp. Gerontol. 57, 175–180. doi:10.1016/j.exger.2014.05.010
Kook, S.-H., and Lee, J.-C. (2012). Tensile Force Inhibits the Proliferation of Human Periodontal Ligament Fibroblasts through Ras-P38 MAPK Up-Regulation. J. Cell. Physiol. 227 (3), 1098–1106. doi:10.1002/jcp.22829
Krishnan, V., and Davidovitch, Z. e. (2006). Cellular, Molecular, and Tissue-Level Reactions to Orthodontic Force. Am. J. Orthod. Dentofacial Orthopedics 129 (4), 461–469. doi:10.1016/j.ajodo.2005.10.007
Krishnan, V., Viecilli, R. F., and Davidovitch, Z. e. (2015). “Cellular and Molecular Biology behind Orthodontic Tooth Movement,” in Biological Mechanisms of Tooth Movement. Editors V. Krishnan, and Z. Davidovitch. 2nd ed (Chichester, UK: Wiley), 30–50. doi:10.1002/9781118916148.ch3
Ku, S.-J., Chang, Y.-I., Chae, C.-H., Kim, S.-G., Park, Y.-W., Jung, Y.-K., et al. (2009). Static Tensional Forces Increase Osteogenic Gene Expression in Three-Dimensional Periodontal Ligament Cell Culture. BMB Rep. 42 (7), 427–432. doi:10.5483/bmbrep.2009.42.7.427
Lee, E. L., and von Recum, H. A. (2015). “Mechanical Conditioning,” in Molecular, Cellular, and Tissue Engineering. Editors J.D. Bronzino, and D.R. Peterson. 4th ed (Boca Raton: CRC Press), 53-51–53-24.
Lee, S.-I., Park, K.-H., Kim, S.-J., Kang, Y.-G., Lee, Y.-M., and Kim, E.-C. (2012). Mechanical Stress-Activated Immune Response Genes via Sirtuin 1 Expression in Human Periodontal Ligament Cells. Clin. Exp. Immunol. 168 (1), 113–124. doi:10.1111/j.1365-2249.2011.04549.x
Li, L., Han, M.-x., Li, S., Xu, Y., and Wang, L. (2014). Hypoxia Regulates the Proliferation and Osteogenic Differentiation of Human Periodontal Ligament Cells under Cyclic Tensile Stress via Mitogen-Activated Protein Kinase Pathways. J. Periodontol. 85 (3), 498–508. doi:10.1902/jop.2013.130048
Li, L., Han, M., Li, S., Wang, L., and Xu, Y. (2013). Cyclic Tensile Stress during Physiological Occlusal Force Enhances Osteogenic Differentiation of Human Periodontal Ligament Cells via ERK1/2-Elk1 MAPK Pathway. DNA Cell Biol. 32 (9), 488–497. doi:10.1089/dna.2013.2070
Li, M., Zhang, C., and Yang, Y. (2019). Effects of Mechanical Forces on Osteogenesis and Osteoclastogenesis in Human Periodontal Ligament Fibroblasts. Bone Jt. Res. 8 (1), 19–31. doi:10.1302/2046-3758.81.Bjr-2018-0060.R1
Li, S., Zhang, H., Li, S., Yang, Y., Huo, B., and Zhang, D. (2015). Connexin 43 and ERK Regulate Tension-Induced Signal Transduction in Human Periodontal Ligament Fibroblasts. J. Orthop. Res. 33 (7), 1008–1014. doi:10.1002/jor.22830
Liao, C., and Hua, Y. (2013). Effect of Hydrogen Sulphide on the Expression of Osteoprotegerin and Receptor Activator of NF-Κb Ligand in Human Periodontal Ligament Cells Induced by Tension-Force Stimulation. Arch. Oral Biol. 58 (12), 1784–1790. doi:10.1016/j.archoralbio.2013.08.004
Livak, K. J., and Schmittgen, T. D. (2001). Analysis of Relative Gene Expression Data Using Real-Time Quantitative PCR and the 2−ΔΔCT Method. Methods 25 (4), 402–408. doi:10.1006/meth.2001.1262
Long, P., Hu, J., Piesco, N., Buckley, M., and Agarwal, S. (2001). Low Magnitude of Tensile Strain Inhibits IL-1β-dependent Induction of Pro-inflammatory Cytokines and Induces Synthesis of IL-10 in Human Periodontal Ligament Cells In Vitro. J. Dent. Res. 80 (5), 1416–1420. doi:10.1177/00220345010800050601
Long, P., Liu, F., Piesco, N. P., Kapur, R., and Agarwal, S. (2002). Signaling by Mechanical Strain Involves Transcriptional Regulation of Proinflammatory Genes in Human Periodontal Ligament Cells In Vitro. Bone 30 (4), 547–552. doi:10.1016/s8756-3282(02)00673-7
Ma, J., Zhao, D., Wu, Y., Xu, C., and Zhang, F. (2015). Cyclic Stretch Induced Gene Expression of Extracellular Matrix and Adhesion Molecules in Human Periodontal Ligament Cells. Arch. Oral Biol. 60 (3), 447–455. doi:10.1016/j.archoralbio.2014.11.019
Marchesan, J. T., Scanlon, C. S., Soehren, S., Matsuo, M., and Kapila, Y. L. (2011). Implications of Cultured Periodontal Ligament Cells for the Clinical and Experimental Setting: a Review. Arch. Oral Biol. 56 (10), 933–943. doi:10.1016/j.archoralbio.2011.03.003
Matheson, L., Jackfairbank, N., Maksym, G., Paulsanterre, J., and Labow, R. (2006). Characterization of the Flexcell Uniflex Cyclic Strain Culture System with U937 Macrophage-like Cells. Biomaterials 27 (2), 226–233. doi:10.1016/j.biomaterials.2005.05.070
Matsuda, N., Yokoyama, K., Takeshita, S., and Watanabe, M. (1998). Role of Epidermal Growth Factor and its Receptor in Mechanical Stress-Induced Differentiation of Human Periodontal Ligament Cells In Vitro. Arch. Oral Biol. 43 (12), 987–997. doi:10.1016/s0003-9969(98)00079-x
Mayahara, K., Kobayashi, Y., Takimoto, K., Suzuki, N., Mitsui, N., and Shimizu, N. (2007). Aging Stimulates Cyclooxygenase-2 Expression and Prostaglandin E2production in Human Periodontal Ligament Cells after the Application of Compressive Force. J. Periodontal Res. 42 (1), 8–14. doi:10.1111/j.1600-0765.2006.00885.x
Memmert, S., Damanaki, A., Weykopf, B., Rath-Deschner, B., Nokhbehsaim, M., Götz, W., et al. (2019). Autophagy in Periodontal Ligament Fibroblasts under Biomechanical Loading. Cell Tissue Res 378 (3), 499–511. doi:10.1007/s00441-019-03063-1
Memmert, S., Nogueira, A. V. B., Damanaki, A., Nokhbehsaim, M., Rath-Deschner, B., Götz, W., et al. (2020). Regulation of the Autophagy-Marker Sequestosome 1 in Periodontal Cells and Tissues by Biomechanical Loading. J. Orofac. Orthop. 81 (1), 10–21. doi:10.1007/s00056-019-00197-3
Miura, S., Yamaguchi, M., Shimizu, N., and Abiko, Y. (2000). Mechanical Stress Enhances Expression and Production of Plasminogen Activator in Aging Human Periodontal Ligament Cells. Mech. Ageing Develop. 112 (3), 217–231. doi:10.1016/s0047-6374(99)00095-0
Moher, D., Liberati, A., Tetzlaff, J., and Altman, D. G. (2009). Preferred Reporting Items for Systematic Reviews and Meta-Analyses: the PRISMA Statement. Open Med. 3 (3), e123–30. doi:10.1371/journal.pmed.1000097
Monnouchi, S., Maeda, H., Fujii, S., Tomokiyo, A., Kono, K., and Akamine, A. (2011). The Roles of Angiotensin II in Stretched Periodontal Ligament Cells. J. Dent. Res. 90 (2), 181–185. doi:10.1177/0022034510382118
Montecino, M., Stein, G., Stein, J., Zaidi, K., and Aguilar, R. (2015). Multiple Levels of Epigenetic Control for Bone Biology and Pathology. Bone 81, 733–738. doi:10.1016/j.bone.2015.03.013
Mooney, M. A., and Wilmot, B. (2015). Gene Set Analysis: A Step-by-step Guide. Am. J. Med. Genet. 168 (7), 517–527. doi:10.1002/ajmg.b.32328
Morris, J. H., Apeltsin, L., Newman, A. M., Baumbach, J., Wittkop, T., Su, G., et al. (2011). clusterMaker: a Multi-Algorithm Clustering Plugin for Cytoscape. BMC Bioinformatics 12, 436. doi:10.1186/1471-2105-12-436
Mühlemann, H. R. (1954). Tooth Mobility (V): Tooth Mobility Changes through Artificial Trauma. J. Periodontol. 25 (3), 202–208. doi:10.1902/jop.1954.25.3.202
Mühlemann, H. R., and Zander, H. A. (1954). Tooth Mobility (III): the Mechanism of Tooth Mobility. J. Periodontol. 25 (2), 128–137. doi:10.1902/jop.1954.25.2.128
Natali, A., Pavan, P., and Scarpa, C. (2004). Numerical Analysis of Tooth Mobility: Formulation of a Non-linear Constitutive Law for the Periodontal Ligament. Dental Mater. 20 (7), 623–629. doi:10.1016/j.dental.2003.08.003
Nazet, U., Schröder, A., Spanier, G., Wolf, M., Proff, P., and Kirschneck, C. (2020). Simplified Method for Applying Static Isotropic Tensile Strain in Cell Culture Experiments with Identification of Valid RT-qPCR Reference Genes for PDL Fibroblasts. Eur. J. Orthod. 42 (4), 359–370. doi:10.1093/ejo/cjz052
Nemoto, T., Kajiya, H., Tsuzuki, T., Takahashi, Y., and Okabe, K. (2010). Differential Induction of Collagens by Mechanical Stress in Human Periodontal Ligament Cells. Arch. Oral Biol. 55 (12), 981–987. doi:10.1016/j.archoralbio.2010.08.004
Ngan, P., Saito, S., Saito, M., Lanese, R., Shanfeld, J., and Davidovitch, Z. (1990). The Interactive Effects of Mechanical Stress and Interleukin-1β on Prostaglandin E and Cyclic AMP Production in Human Periodontal Ligament Fibroblasts In Vitro: Comparison with Cloned Osteoblastic Cells of Mouse (MC3T3-E1). Arch. Oral Biol. 35 (9), 717–725. doi:10.1016/0003-9969(90)90094-Q
Nogueira, A. V. B., Nokhbehsaim, M., Eick, S., Bourauel, C., Jäger, A., Jepsen, S., et al. (2014a). Regulation of Visfatin by Microbial and Biomechanical Signals in PDL Cells. Clin. Oral Invest. 18 (1), 171–178. doi:10.1007/s00784-013-0935-1
Nogueira, A. V. B., Nokhbehsaim, M., Eick, S., Bourauel, C., Jäger, A., Jepsen, S., et al. (2014b). Biomechanical Loading Modulates Proinflammatory and Bone Resorptive Mediators in Bacterial-Stimulated PDL Cells. Mediators Inflamm. 2014, 1–10. doi:10.1155/2014/425421
Ohzeki, K., Yamaguchi, M., Shimizu, N., and Abiko, Y. (1999). Effect of Cellular Aging on the Induction of Cyclooxygenase-2 by Mechanical Stress in Human Periodontal Ligament Cells. Mech. Ageing Develop. 108 (2), 151–163. doi:10.1016/s0047-6374(99)00006-8
Padial-Molina, M., Volk, S. L., Rodriguez, J. C., Marchesan, J. T., Galindo-Moreno, P., and Rios, H. F. (2013). Tumor Necrosis Factor-α andPorphyromonas gingivalisLipopolysaccharides Decrease Periostin in Human Periodontal Ligament Fibroblasts. J. Periodontol. 84 (5), 694–703. doi:10.1902/jop.2012.120078
Papadopoulou, A., Iliadi, A., Eliades, T., and Kletsas, D. (2017). Early Responses of Human Periodontal Ligament Fibroblasts to Cyclic and Static Mechanical Stretching. Eur. J. Orthod. 39 (3), cjw075–263. doi:10.1093/ejo/cjw075
Papadopoulou, A., Todaro, A., Eliades, T., and Kletsas, D. (2019). Effect of Hyperglycaemic Conditions on the Response of Human Periodontal Ligament Fibroblasts to Mechanical Stretching. Eur. J. Orthod. 41 (6), 583–590. doi:10.1093/ejo/cjz051
Pavasant, P., and Yongchaitrakul, T. (2011). Role of Mechanical Stress on the Function of Periodontal Ligament Cells. Periodontol 56 (1), 154–165. doi:10.1111/j.1600-0757.2010.00374.x
Pelaez, D., Acosta Torres, Z., Ng, T. K., Choy, K. W., Pang, C. P., and Cheung, H. S. (2017). Cardiomyogenesis of Periodontal Ligament-Derived Stem Cells by Dynamic Tensile Strain. Cell Tissue Res 367 (2), 229–241. doi:10.1007/s00441-016-2503-x
Pini, M., Wiskott, H. W. A., Scherrer, S. S., Botsis, J., and Belser, U. C. (2002). Mechanical Characterization of Bovine Periodontal Ligament. J. Periodontal Res. 37 (4), 237–244. doi:10.1034/j.1600-0765.2002.00344.x
Qin, J., and Hua, Y. (2016). Effects of Hydrogen Sulfide on the Expression of Alkaline Phosphatase, Osteocalcin and Collagen Type I in Human Periodontal Ligament Cells Induced by Tension Force Stimulation. Mol. Med. Rep. 14 (4), 3871–3877. doi:10.3892/mmr.2016.5680
Ren, D., Wei, F., Hu, L., Yang, S., Wang, C., and Yuan, X. (2015). Phosphorylation of Runx2, Induced by Cyclic Mechanical Tension via ERK1/2 Pathway, Contributes to Osteodifferentiation of Human Periodontal Ligament Fibroblasts. J. Cell. Physiol. 230 (10), 2426–2436. doi:10.1002/jcp.24972
Samuel, G. O., Hoffmann, S., Wright, R. A., Lalu, M. M., Patlewicz, G., Becker, R. A., et al. (2016). Guidance on Assessing the Methodological and Reporting Quality of Toxicologically Relevant Studies: A Scoping Review. Environ. Int. 92–93, 630–646. doi:10.1016/j.envint.2016.03.010
Schardt, C., Adams, M. B., Owens, T., Keitz, S., and Fontelo, P. (2007). Utilization of the PICO Framework to Improve Searching PubMed for Clinical Questions. BMC Med. Inform. Decis. Mak. 7, 16. doi:10.1186/1472-6947-7-16
Seo, B.-M., Miura, M., Gronthos, S., Mark Bartold, P., Batouli, S., Brahim, J., et al. (2004). Investigation of Multipotent Postnatal Stem Cells from Human Periodontal Ligament. The Lancet 364 (9429), 149–155. doi:10.1016/S0140-6736(04)16627-0
Setiawan, M., Jäger, A., and Konermann, A. (2019). The Stability of Different Housekeeping Genes in Human Periodontal Ligament Cells under Inflammatory Conditions. Ann. Anat. - Anatomischer Anzeiger 224, 81–87. doi:10.1016/j.aanat.2019.04.001
Shannon, P., Markiel, A., Ozier, O., Baliga, N. S., Wang, J. T., Ramage, D., et al. (2003). Cytoscape: a Software Environment for Integrated Models of Biomolecular Interaction Networks. Genome Res. 13 (11), 2498–2504. doi:10.1101/gr.1239303
Shi, J., Baumert, U., Folwaczny, M., and Wichelhaus, A. (2019a). Influence of Static Forces on the Expression of Selected Parameters of Inflammation in Periodontal Ligament Cells and Alveolar Bone Cells in a Co-culture In Vitro Model. Clin. Oral Invest. 23 (6), 2617–2628. doi:10.1007/s00784-018-2697-2
Shi, J., Folwaczny, M., Wichelhaus, A., and Baumert, U. (2019b). Differences in RUNX 2 and P2 RX 7 Gene Expression between Mono‐ and Coculture of Human Periodontal Ligament Cells and Human Osteoblasts under Compressive Force Application. Orthod. Craniofac. Res. 22, 168–176. doi:10.1111/ocr.12307
Shimizu, N., Goseki, T., Yamaguchi, M., Iwasawa, T., Takiguchi, H., and Abiko, Y. (1997). In Vitro Cellular Aging Stimulates Interleukin-1β Production in Stretched Human Periodontal-Ligament-Derived Cells. J. Dent. Res. 76 (7), 1367–1375. doi:10.1177/00220345970760070601
Shimizu, N., Ozawa, Y., Yamaguchi, M., Goseki, T., Ohzeki, K., and Abiko, Y. (1998). Induction of COX-2 Expression by Mechanical Tension Force in Human Periodontal Ligament Cells. J. Periodontol. 69 (6), 670–677. doi:10.1902/jop.1998.69.6.670
Somerman, M. J., Archer, S. Y., Imm, G. R., and Foster, R. A. (1988). A Comparative Study of Human Periodontal Ligament Cells and Gingival Fibroblasts In Vitro. J. Dent. Res. 67 (1), 66–70. doi:10.1177/00220345880670011301
Steinberg, T., Ziegler, N., Alonso, A., Kohl, A., Müssig, E., Proksch, S., et al. (2011). Strain Response in Fibroblasts Indicates a Possible Role of the Ca2+-dependent Nuclear Transcription Factor NM1 in RNA Synthesis. Cell Calcium 49 (4), 259–271. doi:10.1016/j.ceca.2011.03.001
Stoddart, M., Richards, R. G., Richards, R., and Alini, M. (2012). In Vitro experiments with Primary Mammalian Cells: to Pool or Not to Pool? eCM 24, i–ii. doi:10.22203/ecm.v024a00
Su, G., Morris, J. H., Demchak, B., and Bader, G. D. (2014). Biological Network Exploration with Cytoscape 3. Curr. Protoc. Bioinformatics 47, 8 13 11–24. doi:10.1002/0471250953.bi0813s47
Sun, C., Liu, F., Cen, S., Chen, L., Wang, Y., Sun, H., et al. (2017). Tensile Strength Suppresses the Osteogenesis of Periodontal Ligament Cells in Inflammatory Microenvironments. Mol. Med. Rep. 16 (1), 666–672. doi:10.3892/mmr.2017.6644
Szklarczyk, D., Gable, A. L., Lyon, D., Junge, A., Wyder, S., Huerta-Cepas, J., et al. (2019). STRING V11: Protein-Protein Association Networks with Increased Coverage, Supporting Functional Discovery in Genome-wide Experimental Datasets. Nucleic Acids Res. 47 (D1), D607–D613. doi:10.1093/nar/gky1131
Tang, N., Zhao, Z., Zhang, L., Yu, Q., Li, J., Xu, Z., et al. (2012). Up-regulated Osteogenic Transcription Factors during Early Response of Human Periodontal Ligament Stem Cells to Cyclic Tensile Strain. Arch. Med. Sci. 3 (3), 422–430. doi:10.5114/aoms.2012.28810
Tantilertanant, Y., Niyompanich, J., Everts, V., Supaphol, P., Pavasant, P., and Sanchavanakit, N. (2019a). Cyclic Tensile Force Stimulates BMP9 Synthesis and In Vitro Mineralization by Human Periodontal Ligament Cells. J. Cell. Physiol. 234 (4), 4528–4539. doi:10.1002/jcp.27257
Tantilertanant, Y., Niyompanich, J., Everts, V., Supaphol, P., Pavasant, P., and Sanchavanakit, N. (2019b). Cyclic Tensile Force-Upregulated IL6 Increases MMP3 Expression by Human Periodontal Ligament Cells. Arch. Oral Biol. 107, 104495. doi:10.1016/j.archoralbio.2019.104495
Vande Geest, J. P., Di Martino, E. S., and Vorp, D. A. (2004). An Analysis of the Complete Strain Field within FlexercellTM Membranes. J. Biomech. 37 (12), 1923–1928. doi:10.1016/j.jbiomech.2004.02.022
Vansant, L., Cadenas De Llano-Pérula, M., Verdonck, A., and Willems, G. (2018). Expression of Biological Mediators during Orthodontic Tooth Movement: A Systematic Review. Arch. Oral Biol. 95, 170–186. doi:10.1016/j.archoralbio.2018.08.003
Von den Hoff, J. W. (2003). Effects of Mechanical Tension on Matrix Degradation by Human Periodontal Ligament Cells Cultured in Collagen Gels. J. Periodontal Res. 38 (5), 449–457. doi:10.1034/j.1600-0765.2003.00404.x
Wada, S., Kanzaki, H., Narimiya, T., and Nakamura, Y. (2017). Novel Device for Application of Continuous Mechanical Tensile Strain to Mammalian Cells. Biol. Open 6 (4), 518–524. doi:10.1242/bio.023671
Wang, H., Feng, C., Jin, Y., Tan, W., and Wei, F. (2019a). Identification and Characterization of Circular RNAs Involved in Mechanical Force‐induced Periodontal Ligament Stem Cells. J. Cell. Physiol. 234 (7), 10166–10177. doi:10.1002/jcp.27686
Wang, L., Pan, J., Wang, T., Song, M., and Chen, W. (2013). Pathological Cyclic Strain-Induced Apoptosis in Human Periodontal Ligament Cells through the RhoGDIα/Caspase-3/PARP Pathway. PLoS One 8 (10), e75973. doi:10.1371/journal.pone.0075973
Wang, Y., Hu, B., Hu, R., Tong, X., Zhang, M., Xu, C., et al. (2019b). TAZ Contributes to Osteogenic Differentiation of Periodontal Ligament Cells under Tensile Stress. J. Periodont Res. 55, 152–160. doi:10.1111/jre.12698
Wichelhaus, A. (2017). Orthodontic Therapy - Fundamental Treatment Concepts. New York: Georg Thieme.
Woda, A., Mishellany, A., and Peyron, M.-A. (2006). The Regulation of Masticatory Function and Food Bolus Formation. J. Oral Rehabil. 33 (11), 840–849. doi:10.1111/j.1365-2842.2006.01626.x
Wu, Y., Ou, Y., Liao, C., Liang, S., and Wang, Y. (2019). High-throughput Sequencing Analysis of the Expression Profile of microRNAs and Target Genes in Mechanical Force-Induced Osteoblastic/cementoblastic Differentiation of Human Periodontal Ligament Cells. Am. J. Transl. Res. 11 (6), 3398–3411.
Wu, Y., Zhuang, J., Zhao, D., and Xu, C. (2019). Interaction between Caspase‐3 and Caspase‐5 in the Stretch‐induced Programmed Cell Death in the Human Periodontal Ligament Cells. J. Cell. Physiol. 234 (8), 13571–13581. doi:10.1002/jcp.28035
Yamaguchi, M., Shimizu, N., Goseki, T., Shibata, Y., Takiguchi, H., Iwasawa, T., et al. (1994). Effect of Different Magnitudes of Tension Force on Prostaglandin E2 Production by Human Periodontal Ligament Cells. Arch. Oral Biol. 39 (10), 877–884. doi:10.1016/0003-9969(94)90019-1
Yamaguchi, N., Chiba, M., and Mitani, H. (2002). The Induction of C-Fos mRNA Expression by Mechanical Stress in Human Periodontal Ligament Cells. Arch. Oral Biol. 47 (6), 465–471. doi:10.1016/s0003-9969(02)00022-5
Yamashita, S., Ai, M., and Mizutani, H. (1993). A New Method for Measuring Nocturnal Tooth Contacts. J. Oral Rehabil. 20 (5), 525–532. doi:10.1111/j.1365-2842.1993.tb01639.x
Yang, L., Yang, Y., Wang, S., Li, Y., and Zhao, Z. (2015). In Vitro mechanical Loading Models for Periodontal Ligament Cells: from Two-Dimensional to Three-Dimensional Models. Arch. Oral Biol. 60 (3), 416–424. doi:10.1016/j.archoralbio.2014.11.012
Yu, W., Hu, B., Shi, X., Cao, Z., Ren, M., He, Z., et al. (2018). Nicotine Inhibits Osteogenic Differentiation of Human Periodontal Ligament Cells under Cyclic Tensile Stress through Canonical Wnt Pathway and α7 Nicotinic Acetylcholine Receptor. J. Periodont Res. 53 (4), 555–564. doi:10.1111/jre.12545
Yuda, A., Maeda, H., Fujii, S., Monnouchi, S., Yamamoto, N., Wada, N., et al. (2015). Effect of CTGF/CCN2 on Osteo/cementoblastic and Fibroblastic Differentiation of a Human Periodontal Ligament Stem/progenitor Cell Line. J. Cell. Physiol 230 (1), 150–159. doi:10.1002/jcp.24693
Zhuang, J., Wang, Y., Qu, F., Wu, Y., Zhao, D., and Xu, C. (2019). Gasdermin-d Played a Critical Role in the Cyclic Stretch-Induced Inflammatory Reaction in Human Periodontal Ligament Cells. Inflammation 42 (2), 548–558. doi:10.1007/s10753-018-0912-6
Ziegler, N., Alonso, A., Steinberg, T., Woodnutt, D., Kohl, A., Müssig, E., et al. (2010). Mechano-transduction in Periodontal Ligament Cells Identifies Activated States of MAP-Kinases P42/44 and P38-Stress Kinase as a Mechanism for MMP-13 Expression. BMC Cell Biol 11, 10. doi:10.1186/1471-2121-11-10
Ziros, P. G., Basdra, E. K., and Papavassiliou, A. G. (2008). Runx2: of Bone and Stretch. Int. J. Biochem. Cell Biol. 40 (9), 1659–1663. doi:10.1016/j.biocel.2007.05.024
Ziros, P. G., Gil, A.-P. R., Georgakopoulos, T., Habeos, I., Kletsas, D., Basdra, E. K., et al. (2002). The Bone-specific Transcriptional Regulator Cbfa1 Is a Target of Mechanical Signals in Osteoblastic Cells. J. Biol. Chem. 277 (26), 23934–23941. doi:10.1074/jbc.M109881200
Glossary
ALP Alkaline phosphatase
BGLAP Bone gamma-carboxy glutamic acid-containing protein
BMPs Bone morphogenetic proteins
COL I Collagen type I
DEG Differential expressed gene
ECM Extracellular matrix
FC Fold change
FGFs Fibroblast growth factors
GO GeneOntology
HoB High risk of bias
hPDLFs Human periodontal ligament fibroblasts
IL6 Interleukin 6
IL8 Interleukin 8
KEGG Kyoto Encyclopedia of Genes and Genomes
LoB Low risk of bias
MAPK Mitogen-activated protein kinase
MCODE Molecular Complex Detection
MIQE Minimum Information for Publication of Quantitative Real-Time PCR Experiments
n. a. Not applicable
OPG Osteoprotegerin
OTM Orthodontic tooth movement
PCR Polymerase chain reaction
PDL Periodontal ligament
PDLFs Periodontal ligament fibroblasts
PDLSCs Periodontal ligament stem cells
PGE2 Prostaglandin E2
PPI Protein-protein interaction
PRISMA Preferred Reporting Items for Systematic Reviews and Meta-Analyses
qPCR Quantitative polymerase chain reaction
RANK Receptor activator of NF-kappa B
RANKL Receptor activator of the nuclear factor kappa ligand
RT-qPCR Reverse transcriptase quantitative polymerase chain reaction
RT-sqPCR Reverse transcriptase semi-quantitative polymerase chain reaction
RUNX2 Runt-related transcription factor 2 (identical with CBFA1)
STRING-DB “Search Tool for the Retrieval of Interacting Genes/Proteins” database
TNFRSF11B Tumor necrosis factor-alpha receptor superfamily member 11B
TNFSF11 TNF superfamily member 11
WB Western blotting
Keywords: periodontal ligament fibroblasts, tension, tissue remodelling, orthodontic tooth movement, mechanical stress
Citation: Sun C, Janjic Rankovic M, Folwaczny M, Otto S, Wichelhaus A and Baumert U (2021) Effect of Tension on Human Periodontal Ligament Cells: Systematic Review and Network Analysis. Front. Bioeng. Biotechnol. 9:695053. doi: 10.3389/fbioe.2021.695053
Received: 21 April 2021; Accepted: 10 August 2021;
Published: 27 August 2021.
Edited by:
Georges Limbert, University of Southampton, United KingdomReviewed by:
Vincent Everts, Academic Centre for Dentistry Amsterdam, NetherlandsKlara Janjic, Medical University of Vienna, Austria
Copyright © 2021 Sun, Janjic Rankovic, Folwaczny, Otto, Wichelhaus and Baumert. This is an open-access article distributed under the terms of the Creative Commons Attribution License (CC BY). The use, distribution or reproduction in other forums is permitted, provided the original author(s) and the copyright owner(s) are credited and that the original publication in this journal is cited, in accordance with accepted academic practice. No use, distribution or reproduction is permitted which does not comply with these terms.
*Correspondence: Uwe Baumert, uwe.baumert@med.uni-muenchen.de