- 1Department of Biomedical Engineering, University of Wisconsin–Madison, Madison, WI, United States
- 2Morgridge Institute for Research, Madison, WI, United States
- 3Department of Human Oncology, University of Wisconsin–Madison, Madison, WI, United States
- 4Department of Pediatrics, University of Wisconsin–Madison, Madison, WI, United States
Macrophages are dynamic immune cells that govern both normal tissue function and disease progression. However, standard methods to measure heterogeneity in macrophage function within tissues require tissue excision and fixation, which limits our understanding of diverse macrophage function in vivo. Two-photon microscopy of the endogenous metabolic co-enzymes NAD(P)H and flavin adenine dinucleotide (FAD) (metabolic autofluorescence imaging) enables dynamic imaging of mouse models in vivo. Here, we demonstrate metabolic autofluorescence imaging to assess cell-level macrophage heterogeneity in response to normal and cancerous tissue microenvironments in vivo. NAD(P)H and FAD fluorescence intensities and lifetimes were measured for both tissue-resident macrophages in mouse ear dermis and tumor-associated macrophages in pancreatic flank tumors. Metabolic and spatial organization of macrophages were determined by performing metabolic autofluorescence imaging and single macrophage segmentation in mice engineered for macrophage-specific fluorescent protein expression. Tumor-associated macrophages exhibited decreased optical redox ratio [NAD(P)H divided by FAD intensity] compared to dermal macrophages, indicating that tumor-associated macrophages are more oxidized than dermal macrophages. The mean fluorescence lifetimes of NAD(P)H and FAD were longer in dermal macrophages than in tumor-associated macrophages, which reflects changes in NAD(P)H and FAD protein-binding activities. Dermal macrophages had greater heterogeneity in optical redox ratio, NAD(P)H mean lifetime, and FAD mean lifetime compared to tumor-associated macrophages. Similarly, standard markers of macrophage phenotype (CD206 and CD86) assessed by immunofluorescence revealed greater heterogeneity in dermal macrophages compared to tumor-associated macrophages. Ultimately, metabolic autofluorescence imaging provides a novel tool to assess tissue-specific macrophage behavior and cell-level heterogeneity in vivo in animal models.
Introduction
Macrophages serve several roles in tissue maintenance and inflammatory response in both normal and diseased tissues (Gordon and Plüddemann, 2017). There is limited understanding of functional differences in macrophages across these tissue sites and of macrophage involvement in tissue dysfunction. Additionally, macrophage plasticity results in multiple unique functional phenotypes, presenting further challenges for assessing macrophage function (Shapouri-Moghaddam et al., 2018). Standard functional assays (e.g., flow cytometry, PCR, ELISA, and histology) require destructive sample preparation, which limit assessment of macrophage behavior in vivo (Chattopadhyay et al., 2014). Non-destructive methods of monitoring macrophage function are needed to better understand macrophage plasticity and the role of macrophages in regulating tissue homeostasis and disease pathogenesis.
Tissue niche conditions, such as cytokine/chemokine secretion, nutrient availability, and collagen organization, drive macrophages to adopt various functional phenotypes (Epelman et al., 2014; Lavin et al., 2014; Sridharan et al., 2019; Sapudom et al., 2020).Generally, tissue-resident macrophages serve to protect against invading pathogens and promote tissue repair, independent of tissue origin (Gordon and Plüddemann, 2017). Previous studies have shown that functional gene expression differs between macrophages originating from distinct tissue sites, and these macrophages can be functionally reprogrammed upon trafficking to other tissues (Lavin et al., 2014). Additionally, tumor-infiltrating macrophages are specifically directed to promote tumor progression by signaling tumor cell proliferation, motility, and angiogenesis while suppressing further immune recruitment (De Palma and Lewis, 2013). However, comparisons between distinct macrophage populations across normal and diseased tissue sites are limited due to constraints of functional assays (De Palma and Lewis, 2013; Panni et al., 2013).
Changes in macrophage phenotype and function have been directly correlated to their metabolic demands (Stout et al., 2005; De Palma and Lewis, 2013; Panni et al., 2013). Macrophages undergo metabolic switching between oxidative and glycolytic metabolism to fuel anti- or pro-inflammatory processes, respectively (Diskin and Pålsson-McDermott, 2018). Energy demands of tissue-resident macrophages are regulated by the tissue microenvironment, though characterization of macrophage metabolic profiles across specific tissues are limited (Caputa et al., 2019). Previous studies suggest that tissue-resident macrophages require stable upregulation of both glycolysis and oxidative phosphorylation to promote survival and proliferation in tissue (Van Den Bossche et al., 2015). Tumor-infiltrating macrophages are primarily characterized by an anti-inflammatory phenotype relying on fatty acid oxidation and oxidative phosphorylation (Panni et al., 2013). Conversely, highly glycolytic macrophage populations have also been observed invading tumors, demonstrating metabolic heterogeneity associated with tumor-infiltrating macrophages (Vitale et al., 2019). Tools for monitoring macrophage metabolic changes in vivo may better visualize this heterogeneity and inform on cell-level behavior of macrophage populations and interactions with the tissue microenvironment.
Two-photon microscopy of reduced nicotinamide adenine dinucleotide (phosphate) (NAD(P)H) and oxidized flavin adenine dinucleotide (FAD), or “metabolic autofluorescence imaging,” monitors metabolic activity on a single-cell level. NAD(P)H and FAD are an electron donor and acceptor, respectively, involved in metabolic reactions across numerous pathways (Chance et al., 1979; Walsh et al., 2013; Blacker and Duchen, 2016). NAD(P)H and FAD fluorescence intensities report on their respective abundance within cells (Chance et al., 1979; Blacker and Duchen, 2016). Furthermore, the optical redox ratio, defined as the ratio of fluorescence intensity of NAD(P)H to FAD, provides a quantitative measurement of the relative oxidation-reduction state of individual cells (Lakowicz et al., 1992; Georgakoudi and Quinn, 2012; Walsh et al., 2013; Blacker and Duchen, 2016). The fluorescence lifetimes of free and protein-bound NAD(P)H and FAD are distinct and can be recovered with a bi-exponential decay model (Lakowicz et al., 1992; Georgakoudi and Quinn, 2012; Quinn et al., 2013; Walsh et al., 2013). Therefore, fluorescence lifetime imaging microscopy (FLIM) of NAD(P)H and FAD reflects the protein-binding activities of these metabolic co-enzymes in the cell. Previous studies have demonstrated that in vivo tumor-associated macrophages have high FAD intensities that can discriminate these macrophages from other cell types (Szulczewski et al., 2016; Li and Liu, 2018). However, tissue-specific macrophage metabolism and the metabolic heterogeneity of macrophages within a tissue have not been thoroughly characterized in vivo due to a lack of appropriate tools. NAD(P)H and FAD autofluorescence have been previously used to distinguish metabolically diverse cell sub-populations (Kilarski et al., 2013; Ghesquière et al., 2014; Tong et al., 2015; Walsh and Skala, 2015; Alfonso-García et al., 2016; Shah et al., 2017; Heaster et al., 2018, 2020; You et al., 2018; Jones et al., 2020; Li et al., 2020; Smokelin et al., 2020), which supports the use of metabolic autofluorescence imaging to investigate macrophage heterogeneity in vivo. This study demonstrates that metabolic autofluorescence imaging can quantify metabolic heterogeneity between macrophages within normal and cancerous mouse tissues in vivo.
Methods
Mouse Breeding, Inoculation, and Surgery
Csf1r-HBEGF/mCherry and Lyz2-Cre mice were obtained from Jackson Labs and bred to generate immunocompetent progeny with mCherry-expressing macrophages (Schreiber et al., 2013). The reporter mCherry was chosen to avoid spectral overlap with autofluorescence of NAD(P)H and FAD, and to confirm the identity of in vivo macrophages. Intravital imaging of the mouse ear dermis was performed by adapting previously established protocols (n = 4 mice) (Li et al., 2012; Kilarski et al., 2013). Prior to imaging, depilatory cream was applied for ∼2 min to the dorsal side of the ear of isoflurane-anesthetized mice to remove artifacts from hair. Depilatory cream was removed quickly with a moistened cotton swab to remove cream and loose hair and the area was thoroughly cleaned to prevent chemical skin burn and avoid inflammation (Figure 1A). Additional application of PBS was performed to provide extra hydration of the cleared area. Subdermal tumors were engrafted in the same mice used for ear dermis imaging by injecting 2.4 × 106 Panc02 pancreatic adenocarcinoma cells per 0.1 mL into the flanks of each mouse (n = 4). Panc02 cells used for subdermal injections were obtained from the NCI and were cultured in standard RPMI1640 (Gibco) + 10% FBS + 1% penicillin:streptomycin at 37°C and 5% CO2. Imaging commenced when tumors reached ∼100 mm3. Immediately prior to tumor imaging, skin flap surgery exposed flank tumors. Mice were anesthetized with isoflurane, then the skin around the tumor was cut and separated from the body cavity so that the tumor laid flat on the imaging stage while connected to the vasculature (Conway et al., 2017) (Figure 1B). Mice were placed on a specialized microscope stage for imaging (Figure 1C). For dermal imaging, a glass coverslip insert was used with PBS for tissue coupling and surgical tape to secure the tissue. For tumor imaging, an imaging window insert was used with surgical tape to secure skin flap tumors.
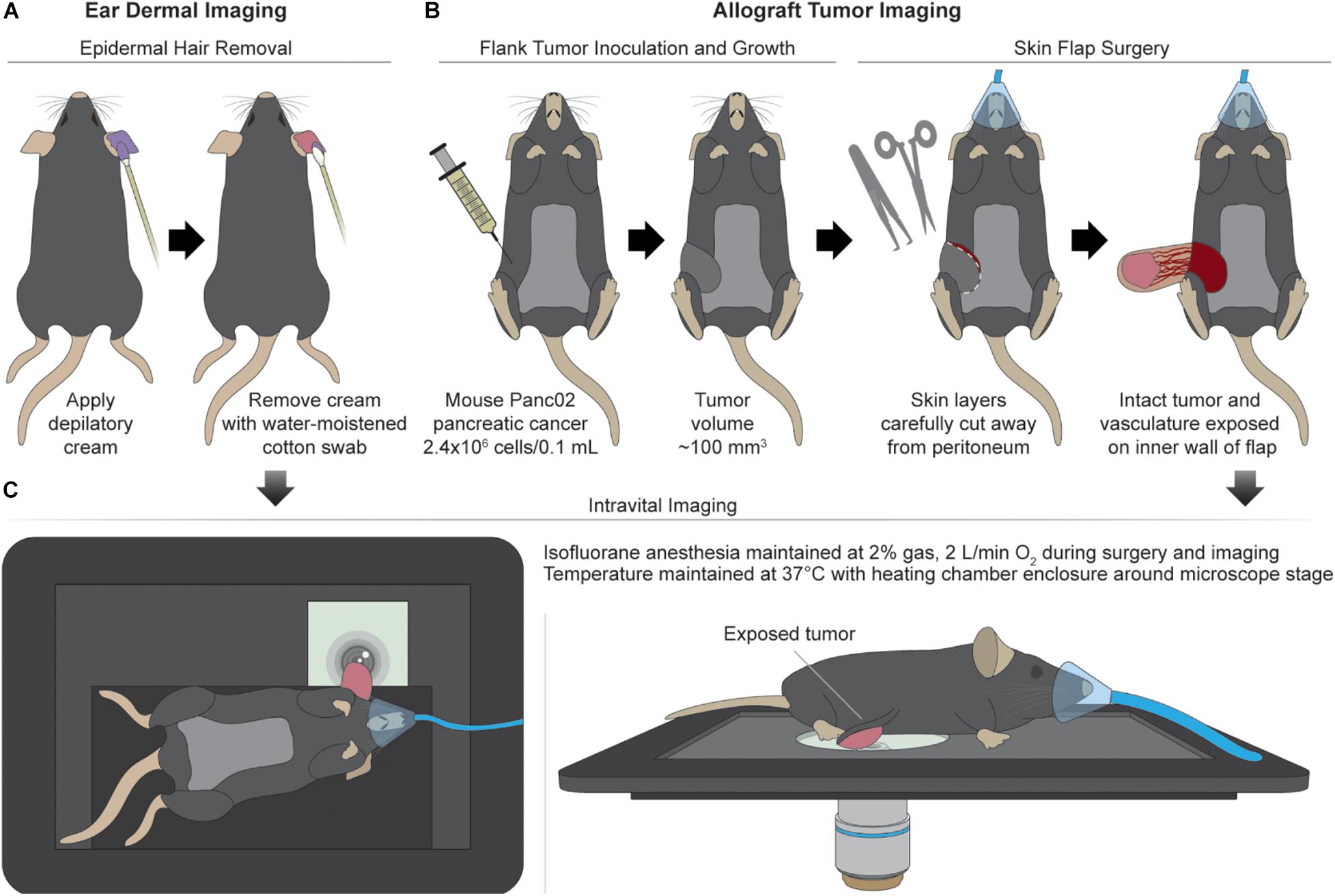
Figure 1. Mouse surgical preparation and intravital imaging setups. (A) Ear dermal imaging technique. Mouse is anesthetized and depilatory cream is applied for ∼2 min to the ventral side of the mouse ear to remove hair as a source of imaging artifact. Depilated area is cleaned with water-soaked cotton swab. (B) Allograft generation and imaging technique. Mouse is inoculated subdermally with 2.4 × 106 Panc02 pancreatic cancer cells (per 0.1 mL) in the flank and allowed to grow to a volume of ∼100 mm3. After reaching target volume, mouse is anesthetized for surgical exposure of tumor. Superficial incisions are made into skin surrounding the tumor to form a flap separable from the peritoneum and body cavity. The tumor is exposed by peeling the flap away from the body while remaining intact and vascularized. (C) Intravital imaging procedures. For ear dermal imaging, mouse is placed on microscope stage designed with inlay to support mouse body and imaging window on which the cleared ear skin is overlaid. For tumor imaging, mouse is placed on microscope stage and skin flap with attached tumor is overlaid for imaging. Anesthesia and temperature are regulated and maintained during all imaging procedures.
Intravital Fluorescence Lifetime Imaging
Autofluorescence images were captured with a custom-built multi-photon microscope (Bruker) using an ultrafast femtosecond laser (InSight DS+, Spectra Physics). Fluorescence lifetime measurements were performed using time-correlated single photon counting electronics (Becker & Hickl). Fluorescence emission was detected using bandpass filters of 466/40 nm (NAD(P)H), 514/30 nm (FAD), and 590/45 nm (mCherry) prior to detection with GaAsP photomultiplier tubes (Hamamatsu). All three fluorophores were concurrently excited using a previously reported wavelength mixing approach (Mahou et al., 2012; Stringari et al., 2017). Briefly, the laser source tuned to 750 nm (NAD(P)H excitation) was delayed and collimated with the secondary laser line fixed at 1,041 nm (mCherry excitation) for spatial and temporal overlap at each raster-scanned focal point (2-color excitation of FAD with 750 nm + 1041 nm). All images were acquired with a 40X/1.13 NA water-immersion objective (Nikon) at 512 × 512 pixel resolution and an optical zoom of two restricting the field of view (150 μm × 150 μm) to the area of laser overlap. A daily fluorescence standard measurement was collected by imaging a YG fluorescent bead (Polysciences Inc.) and verifying the measured lifetime with reported lifetime values. NAD(P)H and FAD intensity and lifetime volumes (z-depths ranging from 20 to 75 μm, 5 μm z-steps) were acquired to sample metabolic behavior of macrophages across 3–5 fields of view and multiple depths within each tissue. The fluorescence lifetimes of free and protein-bound NAD(P)H and FAD are distinct, and these lifetimes along with their weights can be recovered with a two-exponential fit function. Therefore, fluorescence lifetime data for both NAD(P)H and FAD was fit to the following bi-exponential decay in SPCImage: I(t) = α1e−t/τ1 + α2e−t/τ2 + C. For NAD(P)H, τ1 corresponds to the free lifetime, τ2 corresponds to the protein-bound lifetime, and the weights (α1, α2; α1 + α2 = 1) correspond to the proportion of free and protein-bound NAD(P)H, respectively (Lakowicz et al., 1992; Georgakoudi and Quinn, 2012; Blacker and Duchen, 2016; Datta et al., 2020). Conversely for FAD, τ1 corresponds to the protein-bound lifetime and τ2 corresponds to the free lifetime (Ramanujam, 2000; Datta et al., 2020). Decays within a 3 × 3 pixel area were binned to improve signal-to-noise ratio. An instrument response function was measured with second harmonic generation (900 nm excitation) from urea crystals for input into the decay fit procedure. The following fluorescence lifetime components were calculated from the fitted model: τ1, τ2, α1, and α2 for both NAD(P)H and FAD. Mean fluorescence lifetimes (τm) were calculated as τm = τ1α1 + τ2α2.
Geodesic Reconstruction
Individual cell masks were segmented using Geodesic Reconstruction (MorphoLibJ, Fiji) (Legland et al., 2016) depicted in Figure 2A and described as follows: (1) mCherry lifetime images were thresholded based on reported lifetimes (1.3–1.5 ns) to exclude any non-specific fluorescence in the red fluorescence emission channel (Gu et al., 2013; Penjweini et al., 2018; Štefl et al., 2020), (2) thresholded images were designated as marker images for identifying mCherry+ cells, (3) FAD intensity images were designated as mask images based on high FAD signal associated with macrophages (Szulczewski et al., 2016), (4) the marker image was overlaid on the mask image and was iteratively dilated until all connected component objects in the mask image were intersected (i.e., idempotency), and (5) cell outlines were then determined from the reconstructed masks. Intensity and lifetime components for each pixel within resulting masks were averaged in CellProfiler to obtain single-cell measurements. Agreement between geodesic reconstruction and manual segmentation were confirmed by Dice coefficients (Zou et al., 2004) of representative images (Supplementary Figure 1).
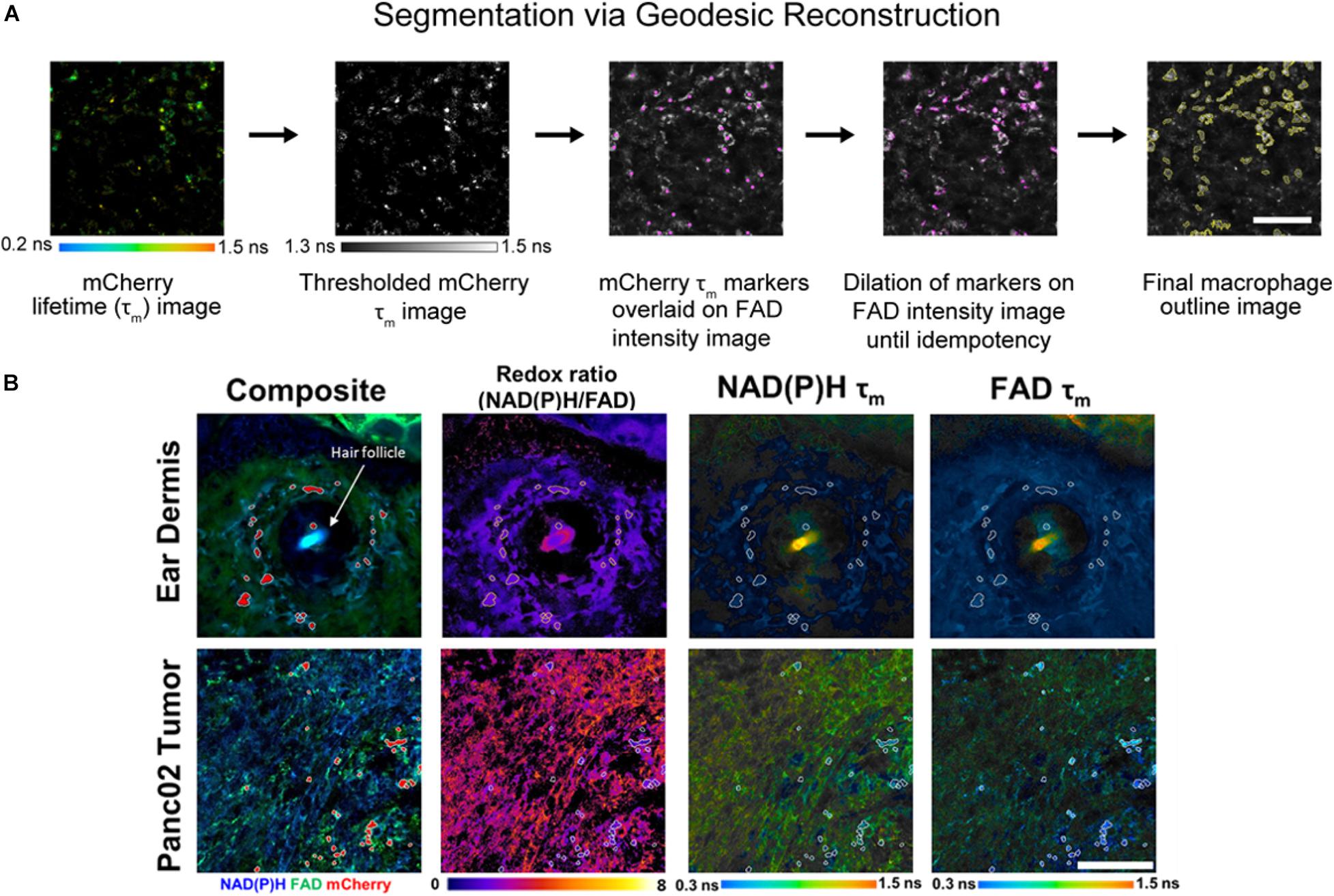
Figure 2. In vivo metabolic autofluorescence imaging and segmentation of macrophages in tumor and dermal tissue. (A) Geodesic reconstruction for segmenting mCherry+ macrophages. Lifetime images of mCherry were thresholded [1.3–1.5 ns, based on reported mCherry lifetime values (Gu et al., 2013; Penjweini et al., 2018; Štefl et al., 2020)] to exclude any non-specific fluorescence in the red emission channel. Thresholded images were used as a marker image to identify mCherry+ cells. FAD intensity images were used as mask images based on previous evidence of high FAD intensity in macrophages (Szulczewski et al., 2016). The marker image was overlaid on the mask image and iteratively dilated until all connected component objects in the mask image were intersected (idempotency). Cell outlines were then determined from the reconstructed masks. Scale bar = 125 μm. (B) Representative fluorescence images of mouse ear dermis and Panc02 pancreatic tumors. Composite intensity images visualize tissue-infiltrating macrophages (mCherry, red) with co-localized NAD(P)H (blue) and FAD (green) autofluorescence. Autofluorescence intensity and lifetime images demonstrate qualitative differences in the optical redox ratio [intensity of NAD(P)H divided by intensity of FAD], NAD(P)H mean lifetime (τm), and FAD τm. Ear dermis images depict a hair follicle (white arrow), which consistently exhibited increased accumulation of macrophages. Conversely, random distribution of macrophages was observed across pancreatic tumor images. Scale bar = 125 μm.
Immunofluorescence Staining and Imaging
Following the final in vivo imaging session for each mouse (post-dermis and tumor imaging), animals were euthanized and both tumors and ear dermis were excised for immunofluorescence staining of macrophage abundance and phenotype. Excised tissues were formalin fixed and paraffin-embedded for antibody staining with a panel of fluorescent markers (CD86, CD206, and mCherry). Sections were taken 5 μm apart through the first 100 μm from the tissue surface to sample from similar tissue areas as measured with two-photon imaging. Embedded sections were deparaffinized and hydrated prior to antigen retrieval and placement in blocking solution. Next, primary antibodies were sequentially applied upon removal of blocking solution at the following dilutions and incubation times: CD86 – 1:100 for 30 min, mCherry – 1:500 for 10 min, and CD206 – 1:1,000 for 5 min. Secondary antibodies were then added following each primary antibody incubation using rat and rabbit secondary antibodies. The following staining dyes were added after secondary antibody washes at 1:100 dilution for 10 min: CD86 – Opal-dye 520, mCherry – Opal-dye 570, CD206 – Opal-dye 620. Finally, stained sections were incubated in DAPI for 5 min at room temperature for nuclear labeling and mounted on coverslips for imaging. Imaging was performed at 20× using a Vectra multispectral imaging system (PerkinElmer) and a spectral library was generated to separate spectral curves for each of the fluorophores. Resulting images were analyzed using Nuance and inForm software (PerkinElmer).
Statistical Analysis
Mann–Whitney statistical tests for non-parametric, unpaired comparisons were performed to assess differences in metabolic autofluorescence variables in dermal and tumor tissues. Results are represented as violin plots showing mean ± 95% CI or stacked bar plots showing mean ± SEM plotted in GraphPad. Heatmaps representing percent coefficient of variation calculations (measurement standard deviation divided by measurement mean multiplied by 100) for each autofluorescence variable per tissue type were also generated in GraphPad.
Results
Representative images of optical redox ratio, NAD(P)H τm, and FAD τm depict qualitative differences in the metabolic activity and spatial organization of dermal macrophages and tumor-infiltrating macrophages (Figure 2B). Macrophages (mCherry+ cells segmented with geodesic reconstruction) localize around hair follicles in the ear dermis, consistent with previous observations (Palero et al., 2007). Conversely, tumor-associated macrophages are randomly distributed across the tissue (Figure 2B). These results indicate that metabolic autofluorescence imaging can visualize metabolic differences in macrophages in vivo with single-cell resolution across multiple tissue types.
Using the composite imaging and single cell segmentation shown in Figure 2, quantitative analyses of the full data set for all mice enables comparisons of tumor and dermal macrophage autofluorescence in vivo (Figure 3). Tumor-infiltrating macrophages exhibited a small but significant decrease in optical redox ratio compared to macrophages within dermal tissue (Figure 3A), consistent with a metabolic preference for oxidative phosphorylation (Qian and Pollard, 2010; Panni et al., 2013). Furthermore, dermal macrophages displayed higher NAD(P)H τm and FAD τm compared to tumor-infiltrating macrophages (Figures 3B,C). The distributions of redox and lifetime values represented by the violin plots reveal notable metabolic heterogeneity in macrophages within both tissue types (Figures 3A–C). Dermal macrophages demonstrate greater metabolic heterogeneity compared to tumor-associated macrophages, as shown in the violin plots and coefficients of variation for all autofluorescence variables (Figure 3D). Macrophage variability is highest for the optical redox ratio, regardless of tissue type (CV: 30.37% – tumor; 108.05% – ear dermis). Ultimately, these quantitative trends illustrate the sensitivity of metabolic autofluorescence imaging to heterogeneous macrophage populations in vivo.
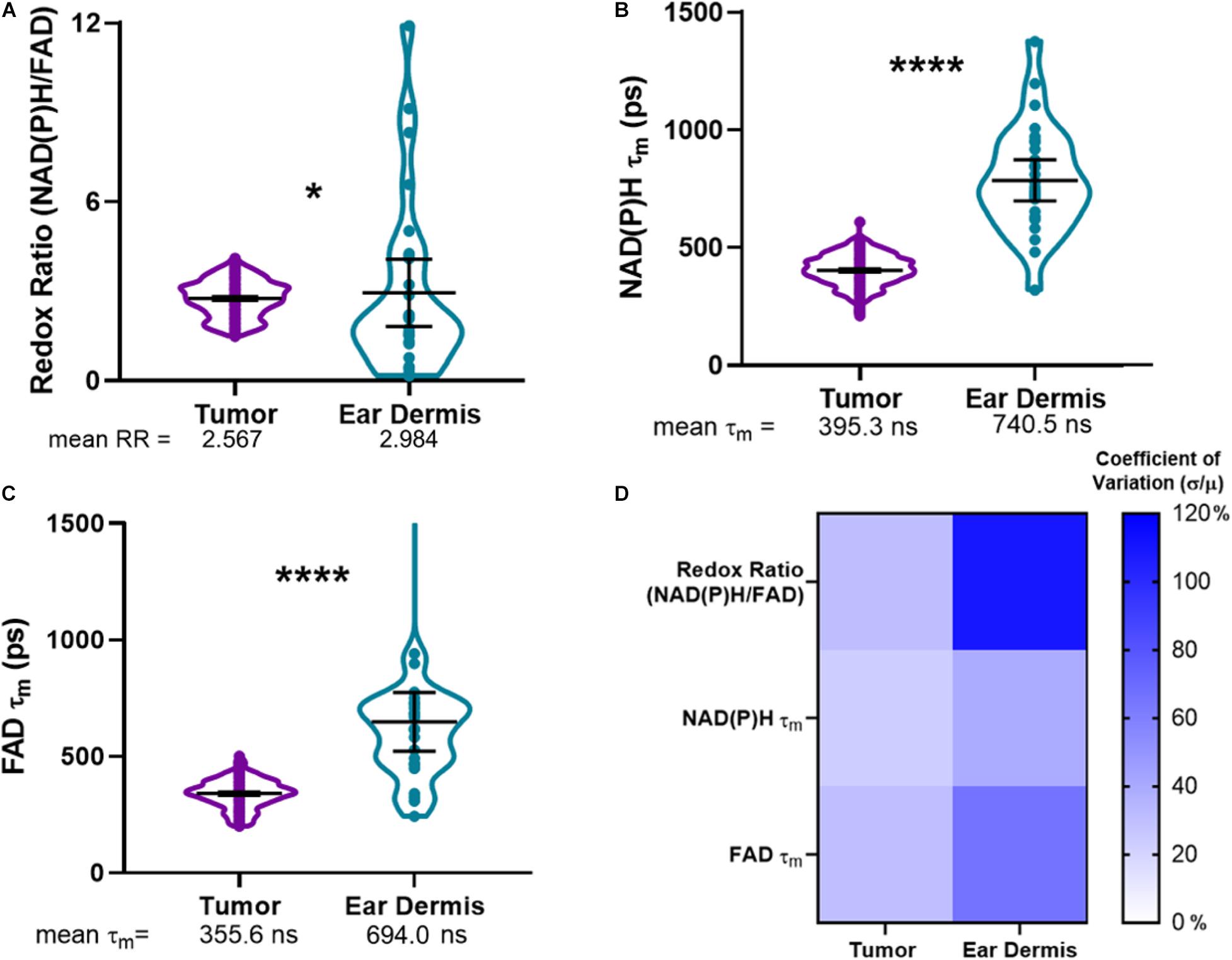
Figure 3. Metabolic autofluorescence resolves differences between tumor and dermal macrophages. (A–C) Violin plots show the full distributions of optical redox ratio (RR), NAD(P)H τm, and FAD τm measured from individual macrophages in tumor and dermal tissues. Mean ± 95% confidence intervals for each measurement are represented by thick black lines and error bars, respectively, overlaid on the violin plots. (A) Quantitative single-cell analysis of redox ratio reveals that tumor-infiltrating macrophages are more oxidized than non-malignant dermal macrophages (2.567 ± 0.53 tumor RR vs. 2.984 ± 0.905 dermis RR). Additionally, mean lifetimes (τm) of (B) NAD(P)H and (C) FAD are lower in tumor-infiltrating macrophage populations compared to non-malignant dermal macrophages. (*, ****p < 0.05, 0.0001; n = 4 mice, 836 cells – tumor; 52 cells – ear dermis). (D) Heatmap of coefficients of variation for autofluorescence variables demonstrate macrophage heterogeneity across both tissues, with the greatest variation observed across ear dermis macrophages. Percent coefficients of variation were calculated as the measurement standard deviation (σ) divided by the measurement mean (μ) multiplied by 100 for each variable and tissue type.
Immunofluorescence was used to confirm the heterogeneity observed with in vivo autofluorescence imaging (Figure 4). Collectively, dermal tissue yielded much fewer total macrophages (mCherry+ cells) than tumor tissue, supporting the fewer number of macrophages imaged in the dermis compared to the tumor with in vivo autofluorescence imaging (Figure 4A). Macrophages positive for CD206, commonly associated with M2-like macrophages that prefer oxidative metabolism were abundant in tumors (Figure 4A). Significant CD206 staining was also observed for dermal macrophages but yielded much sparser expression than tumor-associated macrophages (Figure 4A). These CD206+mCherry+ macrophages (yellow, Figure 4B inlay) were also more prevalent surrounding hair follicles (white arrows), similar to the localization observed in metabolic autofluorescence images. Despite notable CD86 expression in tumor-associated macrophages, limited numbers of CD86+mCherry+ macrophages (purple, Figure 4B inlay) were detected across either tissue type. These qualitative observations are apparent in the quantification of relative proportions of CD86+ mCherry+ or CD206+ mCherry+ cells from immunofluorescence images (Figure 4C and Supplementary Figure 2). Tumor-infiltrating macrophages were observed to be primarily CD206+ (52.2% of mCherry+ cells vs. 8.5% CD86+ mCherry+ cells, p < 0.0001; Figure 4C), while dermal macrophages exhibited a more heterogenous mixture of CD206+ and CD86+ phenotypes (35.9% of CD206+ mCherry+ cells vs. 20.6% CD86+ mCherry+ cells, p > 0.05; Figure 4C). Note that some mCherry+ cells do not stain for CD86 or CD206 [e.g., non-M1/M2-type macrophages (Aras and Zaidi, 2017)], so the total percentage in Figure 4C does not reach 100%. Overall, immunofluorescence staining of excised mouse ear dermis and tumors confirmed that heterogeneous macrophage phenotypes contribute to the heterogenous macrophage autofluorescence in both dermal and tumor tissues. These immunofluorescence data also support greater heterogeneity in dermal macrophage phenotype compared to tumor macrophage phenotype, consistent with autofluorescence imaging.
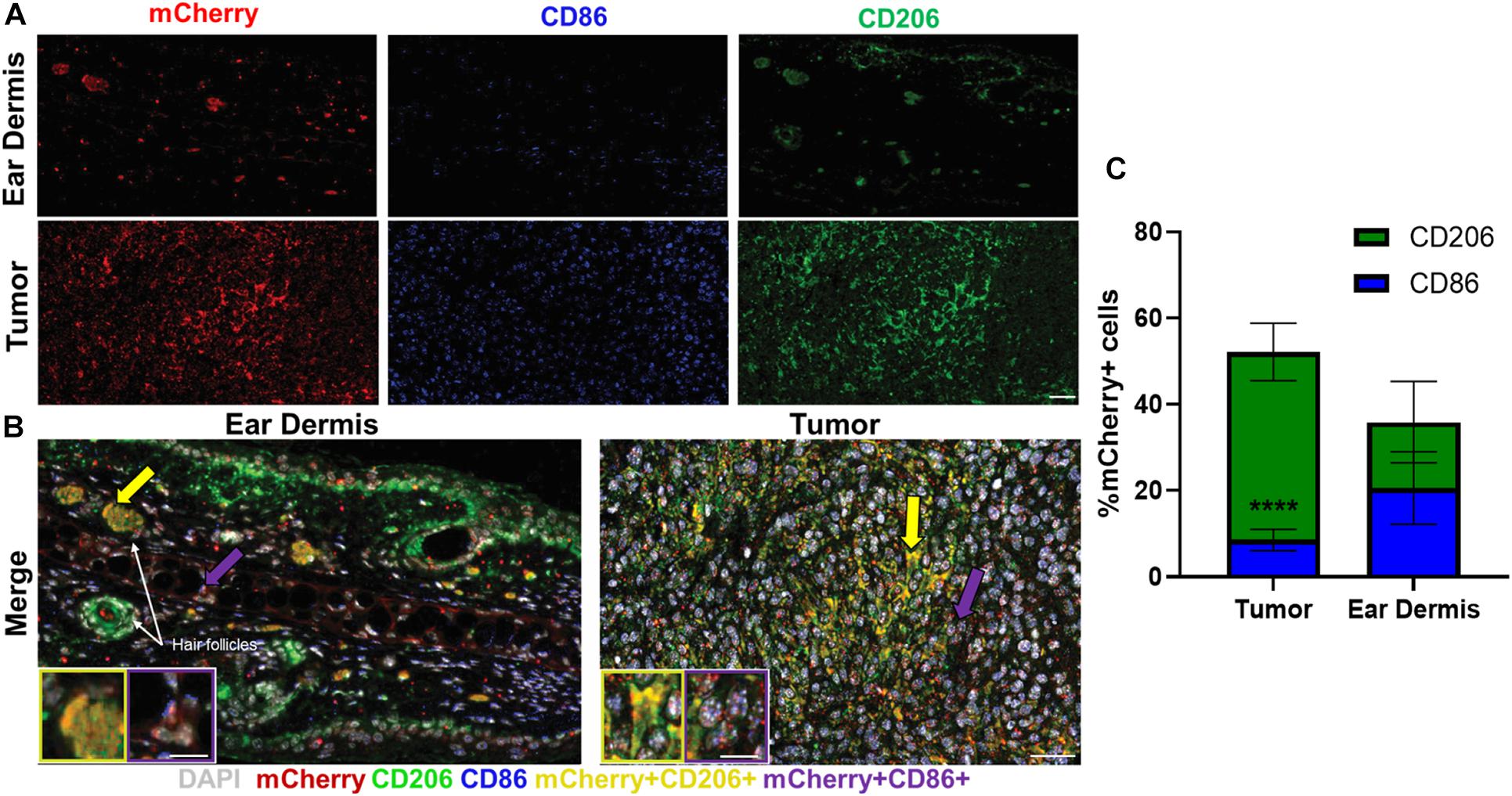
Figure 4. Immunofluorescence staining reflects heterogeneity in macrophage phenotypes across tissue types. Representative immunofluorescence images of resident macrophages in mouse ear dermis and infiltrating macrophages within mouse Panc02 pancreatic tumors. (A) Images of individual mCherry (red), CD86 (blue), and CD206 (green) channels visualize distribution of macrophage-specific markers in ear dermis (top) and tumor (bottom). (B) Merged images identify multiple macrophage phenotypes within both tissues. Inlays represent magnifications of cell areas indicated by yellow (left inlay) and purple (right inlay) arrows in original merged images. DAPI – nuclei, gray; CD86/mCherry co-localization – M1-like macrophage, purple; CD206/mCherry co-localization – M2-like macrophage, yellow. Purple arrows point to M1-like staining; yellow arrows point to M2-like staining. Scale bar, inlays = 15 microns, composite image = 50 microns. (C) Quantification of CD206+ mCherry+ macrophage and CD86+ mCherry+ macrophage proportions for both tissue types reveals a greater ratio of CD206+ to CD86+ macrophages infiltrating in tumor tissue than dermal tissue (****p < 0.0001 CD206+ vs. CD86+), demonstrating greater macrophage heterogeneity in dermal tissue (mean ± SEM; n = 4 mice, 22 ear dermis FOVs, 24 tumor FOVs).
Discussion
This study establishes metabolic autofluorescence imaging as an effective approach for non-destructive, in vivo assessment of macrophage metabolism across normal and cancerous tissue in immunocompetent mouse models. Distinct tissue microenvironments have been previously shown to alter macrophage phenotype and function, providing innate perturbation of macrophage metabolism in vivo (Stout et al., 2005). Mouse ear dermis served as an optimal site to measure non-diseased tissue for comparison with engrafted tumors in a single animal, without the need for multiple surgeries. Autofluorescence imaging enabled quantitative assessment of metabolic heterogeneity within infiltrating macrophage populations across these tissues. Standard immunofluorescence confirmed the observations from metabolic autofluorescence imaging of macrophages within both dermal and tumor tissues. Collectively, these findings support the use of in vivo metabolic autofluorescence imaging to study relationships between macrophage behavior and the local tissue microenvironment.
The combination of intravital autofluorescence imaging and reporter mouse models ensures that the cells of interest (i.e., macrophages) can be accurately identified in vivo. Here, we used a previously established macrophage reporter model generated from crossbreeding Lyz2-Cre mice and mice harboring a floxed mCherry under the Csfr1 promoter (Schreiber et al., 2013; Hoffmann and Ponik, 2020). These mice express mCherry restricted to blood-circulating monocytes and those that have infiltrated into tissue becoming macrophages (Das et al., 2015). Previous studies injected fluorescently labeled antibodies into tissues to identify macrophages and then co-registered autofluorescence signals within these antibody-labeled macrophages in vivo (Szulczewski et al., 2016). This approach was limited by issues with in vivo antibody delivery, non-specific antibody binding, and spectral overlap between the fluorescent label and FAD autofluorescence. The use of fluorescent reporter mice in the current study provided macrophage-specific labels while circumventing these issues of antibody delivery and non-specific binding. The mCherry reporter used in the current study also did not interfere with NAD(P)H and FAD autofluorescence, similarly observed in previous in vivo autofluorescence studies (Hoffmann and Ponik, 2020; Miskolci et al., 2020). Identifying macrophage-specific autofluorescence features without fluorescent reporters remains challenging to due to substantial macrophage heterogeneity in vivo. However, this model could serve as a basis for cultivating reference in vivo datasets to train machine learning classifiers for unlabeled macrophages (Rostam et al., 2017; Christiansen et al., 2018; Pavillon et al., 2018). Overall, the combination of macrophage-specific mCherry reporter mice and two-photon autofluorescence imaging provide a convenient method to monitor macrophage behavior in vivo.
Macrophages traffic to specific tissues and undergo phenotypic switching in response to tissue-secreted biochemical signals (Aloysius et al., 2020). Dermal macrophages comprise a large proportion of cells (∼60%) in the mouse ear dermis, primarily of glycolytic, M1-like phenotype (Dupasquier et al., 2004; Italiani and Boraschi, 2014). The increased optical redox ratio of dermal macrophages reflects this abundant functional phenotype in the mouse dermis (Figure 3A). The increased optical redox ratio and FAD τm observed for dermal macrophages (Figures 3A,C) are also consistent with increased optical redox ratio and FAD τm in previous studies of 2D mouse macrophage cell lines stimulated to glycolytic, M1-like phenotypes (compared to naïve and M2-like macrophages) (Heaster et al., 2020). Similarly, macrophages are highly abundant in mouse pancreatic tumors (Sindrilaru et al., 2011; Ino et al., 2013). However, tumors commonly promote immunosuppressive function and oxidative metabolism in infiltrating macrophages to promote tumor survival, associated with M2 phenotype and function (Qian and Pollard, 2010). This reported preference for oxidative metabolism in tumor-infiltrating macrophages agrees with our measured decrease in optical redox ratio and FAD τm in tumor-infiltrating macrophages compared to dermal macrophages (Figures 3A,C). Our autofluorescence measurements in pancreatic tumor-infiltrating macrophages (Figures 2, 3) are also comparable to previous studies that show low NAD(P)H τm and high FAD intensity in tumor-infiltrating macrophages in vivo (Szulczewski et al., 2016). Small differences in absolute lifetime values reported here and in the previous in vivo autofluorescence study (Szulczewski et al., 2016) may be attributed to differences in tumor site (breast vs. pancreatic cancer) and tumor model (spontaneous vs. engrafted), which are known to alter cell autofluorescence (Heaster et al., 2020). Overall, these results demonstrate that metabolic autofluorescence imaging can detect heterogenous macrophage populations across normal and cancerous tissue based on unique macrophage metabolic activities.
Finally, phenotypic and functional heterogeneity captured from metabolic autofluorescence was confirmed with immunofluorescence staining of known macrophage markers. Diversity in metabolic autofluorescence was observed for both tumor-infiltrating macrophages and dermal macrophages (Figure 3D). Immunofluorescence confirmed this diversity, as mixed CD206 and CD86 expression was observed for both tumor-infiltrating macrophages and dermal macrophages (Figure 4). This heterogeneity is consistent with previous studies of tumor-associated macrophage diversity measured with flow cytometry in this tumor model (Biswas and Mantovani, 2012; Urs, 2019). Similarly, previous studies have measured diverse macrophage populations from immunofluorescence characterization of mouse skin comparable to the CD86/CD206 staining observed for ear dermis tissue (Sindrilaru et al., 2011; Lee and Prisby, 2017). This agreement with immunofluorescence supports autofluorescence imaging of NAD(P)H and FAD as a label-free in vivo method to monitor spatial and temporal variations in both macrophage metabolism and phenotype.
Here, we have shown that intravital metabolic autofluorescence imaging can resolve dynamic macrophage function within native tissue microenvironments, confirmed with fluorescent reporter mouse models. This provides an attractive method to assess macrophage behavior throughout disease progression and in response to treatment, which could aid in new drug development to target macrophages in cancer, wound healing, and a variety of other diseases. While autofluorescence FLIM alone is not sufficient to specify a biological mechanism, the in vivo macrophage imaging techniques described in this paper can be used to guide independent measurements of metabolism. The methods described here are also amenable to time-course measurements of macrophage movement and autofluorescence over hours and days within the same animal in vivo. Longer-term studies require alternative procedures (e.g., specialized vital regulation and imaging windows) though could provide further insight into the evolution of macrophage function in vivo (Ingman et al., 2006; Szulczewski et al., 2016; Entenberg et al., 2017; Dawson et al., 2021). These methods could also identify novel approaches to alter tissue state to influence macrophage function. Ultimately, this imaging approach could elucidate the dynamic role of tissue-specific macrophage populations in vivo.
Data Availability Statement
The raw data supporting the conclusions of this article will be made available by the authors, without undue reservation.
Ethics Statement
The animal study was reviewed and approved by Institutional Animal Care and Use Committee, the University of Wisconsin–Madison.
Author Contributions
TH designed the experiments, collected the data, designed and performed the analysis, interpreted the data, generated the figures, and drafted the manuscript for this study. AH assisted in performing the analysis, interpreting the data, and editing the manuscript. PS and MC helped to design the experiments and analysis, interpreted the data, and edited the manuscript. All authors contributed to the article and approved the submitted version.
Funding
This work was funded by the National Science Foundation Graduate Research Fellowship (DGE-1256259 to TH); the National Science Foundation (CBET-1642287); Stand Up to Cancer (SU2C-AACR-IG-08-16 and SU2C-AACR-PS-18); and the National Institutes of Health (R01 CA185747, R01 CA205101, R01 CA211082, and R35 CA197078).
Conflict of Interest
The authors declare that the research was conducted in the absence of any commercial or financial relationships that could be construed as a potential conflict of interest.
Acknowledgments
We would like to thank Karla Esbona, Vishal Chanana, Rebecca Baus, and the University of Wisconsin Translational Research Initiatives in Pathology laboratory (TRIP), supported by the UW Department of Pathology and Laboratory Medicine, UWCCC (P30 CA014520) and the Office of The Director-NIH (S10OD023526) for use of its facilities and services. We further acknowledge Steve Trier, David Inman, Rupsa Datta, Alexander Rakhmilevich, and Anna Hoefges for practical guidance and insightful discussions. We also thank Matt Stefely for assistance in figure formatting.
Supplementary Material
The Supplementary Material for this article can be found online at: https://www.frontiersin.org/articles/10.3389/fbioe.2021.644648/full#supplementary-material
References
Alfonso-García, A., Smith, T. D., Datta, R., Luu, T. U., Gratton, E., Potma, E. O., et al. (2016). Label-free identification of macrophage phenotype by fluorescence lifetime imaging microscopy. J. Biomed. Opt. 21:46005.
Aloysius, A., Saxena, S., and Seifert, A. W. (2020). Metabolic regulation of innate immune cell phenotypes during wound repair and regeneration. Curr. Opin. Immunol. 68, 72–82. doi: 10.1016/j.coi.2020.10.012
Aras, S., and Zaidi, M. R. (2017). TAMeless traitors: macrophages in cancer progression and metastasis. Br. J. Cancer 117, 1583–1591. doi: 10.1038/bjc.2017.356
Biswas, S. K., and Mantovani, A. (2012). Orchestration of metabolism by macrophages. Cell Metab. 15, 432–437. doi: 10.1016/j.cmet.2011.11.013
Blacker, T. S., and Duchen, M. R. (2016). Investigating mitochondrial redox state using NADH and NADPH autofluorescence. Free Radic. Biol. Med. 100, 53–65. doi: 10.1016/j.freeradbiomed.2016.08.010
Caputa, G., Castoldi, A., and Pearce, E. J. (2019). Metabolic adaptations of tissue-resident immune cells. Nat. Immunol. 20, 793–801. doi: 10.1038/s41590-019-0407-0
Chance, B., Schoener, B., Oshino, R., Itshak, F., and Nakase, Y. (1979). Oxidation-reduction ratio studies of mitochondria in freeze-trapped samples. NADH and flavoprotein fluorescence signals. J. Biol. Chem. 254, 4764–4771. doi: 10.1016/s0021-9258(17)30079-0
Chattopadhyay, P. K., Gierahn, T. M., Roederer, M., and Love, J. C. (2014). Single-cell technologies for monitoring immune systems. Nat. Immunol. 15, 128–135. doi: 10.1038/ni.2796
Christiansen, E. M., Yang, S. J., Ando, D. M., Javaherian, A., Skibinski, G., Lipnick, S., et al. (2018). In silico labeling: predicting fluorescent labels in unlabeled images. Cell 173, 792–803. doi: 10.1016/j.cell.2018.03.040
Conway, J. R. W., Warren, S. C., and Timpson, P. (2017). Context-dependent intravital imaging of therapeutic response using intramolecular FRET biosensors. Methods 128, 78–94. doi: 10.1016/j.ymeth.2017.04.014
Das, A., Datta, S., Abas, M., Chaffee, S., Sen, C. K., and Roy, S. (2015). Monocyte and macrophage plasticity in tissue repair and regeneration. Am. J. Path 185, 2596–2606. doi: 10.1016/j.ajpath.2015.06.001
Datta, R., Heaster, T. M., Sharick, J. T., Gillette, A. A., and Skala, M. C. (2020). Fluorescence lifetime imaging microscopy: fundamentals and advances in instrumentation, analysis, and applications. J. Biomed. Opt. 25:071203.
Dawson, C. A., Mueller, S. N., Lindeman, G. J., Rios, A. C., and Visvader, J. E. (2021). Intravital microscopy of dynamic single-cell behavior in mouse mammary tissue. Nat. Protoc. 1–29.
De Palma, M., and Lewis, C. E. (2013). Macrophage regulation of tumor responses to anticancer therapies. Cancer Cell 23, 277–286. doi: 10.1016/j.ccr.2013.02.013
Diskin, C., and Pålsson-McDermott, E. M. (2018). Metabolic modulation in macrophage effector function. Front. Immunol. 9:270. doi: 10.3389/fimmu.2018.00270
Dupasquier, M., Stoitzner, P., van Oudenaren, A., Romani, N., and Leenen, P. J. M. (2004). Macrophages and dendritic cells constitute a major subpopulation of cells in the mouse dermis. J. Invest. Dermatol. 123, 876–879. doi: 10.1111/j.0022-202x.2004.23427.x
Entenberg, D., Pastoriza, J. M., Oktay, M. H., Voiculescu, S., Wang, Y., Sosa, M. S., et al. (2017). Time-lapsed, large-volume, high-resolution intravital imaging for tissue-wide analysis of single cell dynamics. Methods 128, 65–77. doi: 10.1016/j.ymeth.2017.07.019
Epelman, S., Lavine, K. J., and Randolph, G. J. (2014). Origin and functions of tissue macrophages. Immunity 41, 21–35. doi: 10.1016/j.immuni.2014.06.013
Georgakoudi, I., and Quinn, K. P. (2012). Optical imaging using endogenous contrast to assess metabolic state. Annu. Rev. Biomed. Eng. 14, 351–367. doi: 10.1146/annurev-bioeng-071811-150108
Ghesquière, B., Wong, B. W., Kuchnio, A., and Carmeliet, P. (2014). Metabolism of stromal and immune cells in health and disease. Nature 511, 167–176. doi: 10.1038/nature13312
Gordon, S., and Plüddemann, A. (2017). Tissue macrophages: heterogeneity and functions. BMC Biol. 15:53. doi: 10.1186/s12915-017-0392-4
Gu, L., Hall, D. J., Qin, Z., Anglin, E., Joo, J., Mooney, D. J., et al. (2013). In vivo time-gated fluorescence imaging with biodegradable luminescent porous silicon nanoparticles. Nat. Comm. 4:2326.
Heaster, T. M., Humayun, M., Yu, J., Beebe, D. J., and Skala, M. C. (2020). Autofluorescence imaging of 3D tumor-macrophage microscale cultures resolves spatial and temporal dynamics of macrophage metabolism. Can. Res. 80, 5408–5423.
Heaster, T. M., Walsh, A. J., Zhao, Y., Hiebert, S. W., and Skala, M. C. (2018). Autofluorescence imaging identifies tumor cell-cycle status on a single-cell level. J. Biophotonics 11, 1–15.
Hoffmann, E. J., and Ponik, S. M. (2020). Biomechanical contributions to macrophage activation in the tumor microenvironment. Front. Oncol. 10:787. doi: 10.3389/fonc.2020.00787
Ingman, W. V., Wyckoff, J., Gouon-Evans, V., Condeelis, J., and Pollard, J. W. (2006). Macrophages promote collagen fibrillogenesis around terminal end buds of the developing mammary gland. Dev. Dynam. 235, 3222–3229. doi: 10.1002/dvdy.20972
Ino, Y., Yamazaki-Itoh, R., Shimada, K., Iwasaki, M., Kosuge, T., Kanai, Y., et al. (2013). Immune cell infiltration as an indicator of the immune microenvironment of pancreatic cancer. Br. J. Cancer 108, 914–923. doi: 10.1038/bjc.2013.32
Italiani, P., and Boraschi, D. (2014). From monocytes to M1/M2 macrophages: phenotypical vs. functional differentiation. Front. Immunol. 5:514. doi: 10.3389/fimmu.2014.00514
Jones, J. D., Ramser, H. E., Woessner, A. E., Veves, A., and Quinn, K. P. (2020). Quantifying age-related changes in skin wound metabolism using in vivo multiphoton microscopy. Adv. Wound Care 9, 90–102. doi: 10.1089/wound.2019.1030
Kilarski, W. W., Güç, E., Teo, J. C., Oliver, S. R., Lund, A. W., and Swartz, M. A. (2013). Intravital immunofluorescence for visualizing the microcirculatory and immune microenvironments in the mouse ear dermis. PloS One 8:e57135. doi: 10.1371/journal.pone.0057135
Lakowicz, J. R., Szmacinski, H., Nowaczyk, K., and Johnson, M. L. (1992). Fluorescence lifetime imaging of free and protein-bound NADH. Proc. Natl. Acad. Sci. U. S. A. 89, 1271–1275. doi: 10.1073/pnas.89.4.1271
Lavin, Y., Winter, D., Blecher-Gonen, R., David, E., Keren-Shaul, H., Merad, M., et al. (2014). Tissue-resident macrophage enhancer landscapes are shaped by the local microenvironment. Cell 159, 1312–1326. doi: 10.1016/j.cell.2014.11.018
Lee, S., and Prisby, R. (2017). Pro-inflammatory cytokines and the marrow microenvironment. FASEB J. 31:679.4-679.4.
Legland, D., Arganda-Carreras, I., and Andrey, P. (2016). MorphoLibJ: integrated library and plugins for mathematical morphology with ImageJ. Bioinformatics 32, 3532–3534. doi: 10.1093/bioinformatics/btw413
Li, J. L., Goh, C. C., Keeble, J. L., Qin, J. S., Roediger, B., Jain, R., et al. (2012). Intravital multiphoton imaging of immune responses in the mouse ear skin. Nat. Prot. 7:221. doi: 10.1038/nprot.2011.438
Li, Y., He, Y., Miao, K., Zheng, Y., Deng, C., and Liu, T. M. (2020). Imaging of macrophage mitochondria dynamics in vivo reveals cellular activation phenotype for diagnosis. Theranostics 10, 2897. doi: 10.7150/thno.40495
Li, Y., and Liu, T. M. (2018). Discovering macrophage functions using in vivo optical imaging techniques. Front. Immunol. 9:502. doi: 10.3389/fimmu.2018.00502
Mahou, P., Zimmerley, M., Loulier, K., Matho, K. S., Labroille, G., Morin, X., et al. (2012). Multicolor two-photon tissue imaging by wavelength mixing. Nat. methods 9, 815–818. doi: 10.1038/nmeth.2098
Miskolci, V., Tweed, K. E., Lasarev, M. R., Britt, E. C., McDougal, C. E., Walsh, A. J., et al. (2020). In vivo fluorescence lifetime imaging captures metabolic changes in macrophages during wound responses in zebrafish. bioRxiv [Preprint] doi: 10.1101/2020.06.16.153361
Palero, J. A., de Bruijn, H. S., van der Ploeg van den Heuvel, A., Sterenborg, H. J. C. M., and Gerritsen, H. C. (2007). Spectrally resolved multiphoton imaging of in vivo and excised mouse skin tissues. Biophys J. 93, 992–1007. doi: 10.1529/biophysj.106.099457
Panni, R. Z., Linehan, D. C., and DeNardo, D. G. (2013). Targeting tumor-infiltrating macrophages to combat cancer. Immunotherapy 5, 1075–1087. doi: 10.2217/imt.13.102
Pavillon, N., Hobro, A. J., Akira, S., and Smith, N. I. (2018). Noninvasive detection of macrophage activation with single-cell resolution through machine learning. Proc. Natl. Acad. Sci. U.S.A. 115, E2676–E2685.
Penjweini, R., Andreoni, A., Rosales, T., Kim, J., Brenner, M. D., Sackett, D. L., et al. (2018). Intracellular oxygen mapping using a myoglobin-mCherry probe with fluorescence lifetime imaging. J. Biomed. Opt. 23:107001.
Qian, B. Z., and Pollard, J. W. (2010). Macrophage diversity enhances tumor progression and metastasis. Cell 141, 39–51. doi: 10.1016/j.cell.2010.03.014
Quinn, K. P., Sridharan, G. V., Hayden, R. S., Kaplan, D. L., Lee, K., and Georgakoudi, I. (2013). Quantitative metabolic imaging using endogenous fluorescence to detect stem cell differentiation. Sci. Rep. 3:3432.
Ramanujam, N. (2000). Fluorescence spectroscopy of neoplastic and non-neoplastic tissues. Neoplasia 2, 89–117. doi: 10.1038/sj.neo.7900077
Rostam, H. M., Reynolds, P. M., Alexander, M. R., Gadegaard, N., and Ghaemmaghami, A. M. (2017). Image based Machine Learning for identification of macrophage subsets. Sci. Rep. 7:3521.
Sapudom, J., Mohamed, W. K. E., Garcia-Sabaté, A., Alatoom, A., Karaman, S., Mahtani, N., et al. (2020). Collagen fibril density modulates macrophage activation and cellular functions during tissue repair. Bioengineering 7:33. doi: 10.3390/bioengineering7020033
Schreiber, H. A., Loschko, J., Karssemeijer, R. A., Escolano, A., Meredith, M. M., Mucida, D., et al. (2013). Intestinal monocytes and macrophages are required for T cell polarization in response to Citrobacter rodentium. J. Exp. Med. 210, 2025–2039. doi: 10.1084/jem.20130903
Shah, A. T., Heaster, T. M., and Skala, M. C. (2017). Metabolic imaging of head and neck cancer organoids. PLoS One 12:e0170415. doi: 10.1371/journal.pone.0170415
Shapouri-Moghaddam, A., Mohammadian, S., Vazini, H., Taghadosi, M., Esmaeili, S. A., and Mardani, F. (2018). Macrophage plasticity, polarization, and function in health and disease. J. Cell. Physiol. 233, 6425–6440. doi: 10.1002/jcp.26429
Sindrilaru, A., Peters, T., Wieschalka, S., Baican, C., Baican, A., Peter, H., et al. (2011). An unrestrained proinflammatory M1 macrophage population induced by iron impairs wound healing in humans and mice. J. Clin. Invest. 121, 985–997. doi: 10.1172/jci44490
Smokelin, I. S., Mizzoni, C., Erndt-Marino, J., Kaplan, D., and Georgakoudi, I. (2020). Optical changes in THP-1 macrophage metabolism in response to pro-and anti-inflammatory stimuli reported by label-free two-photon imaging. J. Biomed. Opt. 25:014512.
Sridharan, R., Cavanagh, B., Cameron, A. R., Kelly, D. J., and O’Brien, F. J. (2019). Material stiffness influences the polarization state, function and migration mode of macrophages. Acta Biomaterialia 89, 47–59. doi: 10.1016/j.actbio.2019.02.048
Štefl, M., Herbst, K., Rübsam, M., Benda, A., and Knop, M. (2020). Single-color fluorescence lifetime cross-correlation spectroscopy In Vivo. Biophys J. 119, 1359–1370. doi: 10.1016/j.bpj.2020.06.039
Stout, R. D., Jiang, C., Matta, B., Tietzel, I., Watkins, S. K., and Suttles, J. (2005). Macrophages sequentially change their functional phenotype in response to changes in microenvironmental influences. J. Immunol. 175, 342–349. doi: 10.4049/jimmunol.175.1.342
Stringari, C., Abdeladim, L., Malkinson, G., Mahou, P., Solinas, X., Lamarre, I., et al. (2017). Multicolor two-photon imaging of endogenous fluorophores in living tissues by wavelength mixing. Sci. Rep. 7:3792.
Szulczewski, J. M., Inman, D. R., Entenberg, D., Ponik, S. M., Aguirre-Ghiso, J., Castracane, J., et al. (2016). In Vivo visualization of stromal macrophages via label-free FLIM-based metabolite imaging. Sci. Rep. 6:25086.
Tong, P. L., Roediger, B., Kolesnikoff, N., Biro, M., Tay, S. S., Jain, R., et al. (2015). The skin immune atlas: three-dimensional analysis of cutaneous leukocyte subsets by multiphoton microscopy. J. Invest. Derm. 135, 84–93. doi: 10.1038/jid.2014.289
Van Den Bossche, J., Baardman, J., and De Winther, M. P. J. (2015). Metabolic characterization of polarized M1 and M2 bone marrow-derived macrophages using real-time extracellular flux analysis. J. Vis. Exp. 2015:53424.
Vitale, I., Manic, G., Coussens, L. M., Kroemer, G., and Galluzzi, L. (2019). Macrophages and metabolism in the tumor microenvironment. Cell Metab. 30, 36–50. doi: 10.1016/j.cmet.2019.06.001
Walsh, A. J., Cook, R. S., Manning, H. C., Hicks, D. J., Lafontant, A., Arteaga, C. L., et al. (2013). Optical metabolic imaging identifies glycolytic levels, subtypes, and early-treatment response in breast cancer. Cancer Res. 73, 6164–6174. doi: 10.1158/0008-5472.can-13-0527
Walsh, A. J., and Skala, M. C. (2015). Optical metabolic imaging quantifies heterogeneous cell populations. Biomed. Opt. Express 6, 559–573. doi: 10.1364/boe.6.000559
You, S., Tu, H., Chaney, E. J., Sun, Y., Zhao, Y., Bower, A. J., et al. (2018). Intravital imaging by simultaneous label-free autofluorescence-multiharmonic microscopy. Nat. Comm. 9:2125.
Keywords: fluorescence lifetime imaging, autofluorescence, cellular metabolism, intravital microscopy, macrophages
Citation: Heaster TM, Heaton AR, Sondel PM and Skala MC (2021) Intravital Metabolic Autofluorescence Imaging Captures Macrophage Heterogeneity Across Normal and Cancerous Tissue. Front. Bioeng. Biotechnol. 9:644648. doi: 10.3389/fbioe.2021.644648
Received: 21 December 2020; Accepted: 30 March 2021;
Published: 20 April 2021.
Edited by:
Hui Mao, Emory University, United StatesReviewed by:
Eunha Kim, Harvard Medical School, United StatesJeremy Teo, New York University Abu Dhabi, United Arab Emirates
Copyright © 2021 Heaster, Heaton, Sondel and Skala. This is an open-access article distributed under the terms of the Creative Commons Attribution License (CC BY). The use, distribution or reproduction in other forums is permitted, provided the original author(s) and the copyright owner(s) are credited and that the original publication in this journal is cited, in accordance with accepted academic practice. No use, distribution or reproduction is permitted which does not comply with these terms.
*Correspondence: Melissa C. Skala, bWNza2FsYUB3aXNjLmVkdQ==