- 1Department of Chemical Engineering and Applied Chemistry, University of Toronto, Toronto, ON, Canada
- 2Institute of Biomedical Engineering, University of Toronto, Toronto, ON, Canada
Global warming and uneven distribution of fossil fuels worldwide concerns have spurred the development of alternative, renewable, sustainable, and environmentally friendly resources. From an engineering perspective, biosynthesis of fatty acid-derived chemicals (FACs) is an attractive and promising solution to produce chemicals from abundant renewable feedstocks and carbon dioxide in microbial chassis. However, several factors limit the viability of this process. This review first summarizes the types of FACs and their widely applications. Next, we take a deep look into the microbial platform to produce FACs, give an outlook for the platform development. Then we discuss the bottlenecks in metabolic pathways and supply possible solutions correspondingly. Finally, we highlight the most recent advances in the fast-growing model-based strain design for FACs biosynthesis.
Introduction
Increasing consumption of petroleum-derived products leads to increasing atmospheric carbon dioxide (CO2) levels and global warming (Sperry et al., 2019). Furthermore, the uneven distribution and unsustainability of fossil resources have motivated engineers to seek alternative sustainable solutions (Raslavičius et al., 2014; Chen et al., 2020). Compared with the traditional strategies to convert plant oils and animal fats into biodiesel, microbial synthesis of fuels, and chemicals presents several advantages. Firstly, feedstocks can be shifted from edible plant oils and animal fats to non-edible biomass feedstocks, especially CO2. Secondly, due to the flexibility of pathways in microbial chassis, a large diversity of bioproducts can be produced in microbial cell factories. Among these bioproducts, fatty acid-derived chemicals (FACs) have attracted significant attention, because fatty acids (FAs) are essential metabolites in all organisms. FAs and their biosynthetic/catabolic intermediates can be used as precursors for a large diversity of FACs, which have an unprecedented wide application range: biofuels, pharmaceuticals, feed additives, and others. Thirdly, bioproducts are green alternatives to petroleum-based fuels, given the capacity of net-zero greenhouse gas emissions. Microbial chassis must be extensively designed and engineered to produce FACs at high titer, rate and yield from various substrates. Recent successes in model-based strain design have speed-up the Design-Build-Test-Learn (DBTL) cycle in metabolic engineering (Carbonell et al., 2018; Hamedirad et al., 2019; Opgenorth et al., 2019). Although FACs biosynthesis has been reviewed from different angles (Marella et al., 2018; Liu and Li, 2020), the purpose of this review is to update the most recent advances in this fast-developing field, with an emphasis on possible synthetic microbial chassis and computational modeling for biosynthesis of FACs.
Types and Applications of Fatty Acid-Derived Chemicals
With accelerating concerns over climate change and the environmental impact of conventional production methods, interest in the renewable microbial production of chemicals have grown (Liu and Nielsen, 2019; Cho et al., 2020; Li M. et al., 2020; Sgobba and Wendisch, 2020; Wu et al., 2020). Among these chemicals, FACs are of particular interest due to their various applications in biofuels, detergents, medicines, industrial lubricants, bioplastics, emulsifiers, food and feed additives, and others (Supplementary Table 1; Richardson and Mcallister, 1945; Geller and Goodrum, 2004; Bellou et al., 2016; Jiang W. et al., 2018; Li G. et al., 2020; Verma et al., 2020; Zerhusen et al., 2020). Different end groups and lengths of FACs lead to different physical and chemical properties, which in turn lead to different practical applications (Figure 1). In general, FACs can be mainly classified into free fatty acids (FFA), fatty alcohols, alka(e)nes, and fatty acid esters (FAEs) (Steen et al., 2010). Most naturally occurring FACs have an unbranched chain within the range of C3 to C28. Based on the chain length, they are generally classified into short-chain (≤ 6), medium-chain (7–12), long-chain (13–20), and very-long-chain (> 20) (Schönfeld and Wojtczak, 2016). However, the definitions can vary from one study to another (Rodriguez-Moya and Gonzalez, 2015).
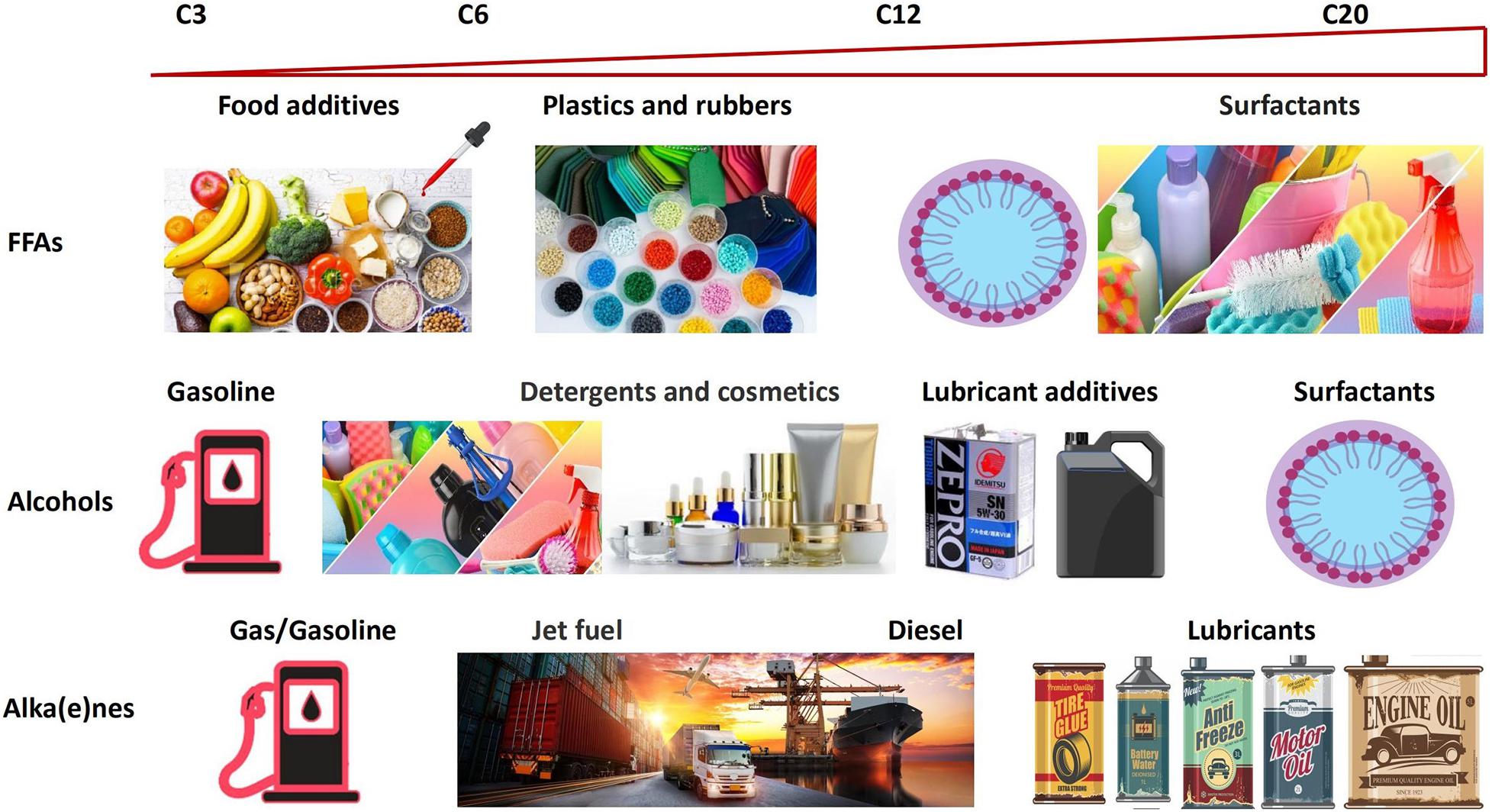
Figure 1. Overview of types and applications of FACs. FACs have a wide application range based on their various chemical properties caused by different end groups and chain lengths.
Fatty acids, one of the most studied FACs, are n-alkyl carboxylic acids with an aliphatic chain. Short-chain fatty acids play an important role in human health. For example, butyric acid can be used in food and pharmaceutical industries (Jiang L. et al., 2018). Most free FFAs are converted into biofuels, or consumer products (Leber et al., 2015; Marella et al., 2018; Sathesh-Prabu et al., 2019). Fatty alcohols have hydroxyl groups attached to the aliphatic chain. Short-chain alcohols, such as 1-propanol and 1-butanol, can be used as gasoline or fuel additives (Guo et al., 2019; Zhou et al., 2019). Alcohols with a chain length of C8–C10 are important materials to produce detergents, lubricants, cosmetics, pharmaceuticals, and plastics. Those in C12–C14 range are used as lubricant additives, and those in C16–C18 range are used for drug delivery and defoamers (Dong and Mumper, 2006; Zheng et al., 2012). Among these, C11–C14 alcohols, the key intermediates for surfactants production, represent 55% of the market share (Fillet and Adrio, 2016). Alkanes are saturated hydrocarbons with the general chemical formula CnH2n+2, while alkenes are unsaturated hydrocarbons containing at least one C–C double bond. Alka(e)nes are an important class of FACs because of their high similarity to petroleum-derived fuels. Depending on the chain length, alka(e)nes have different applications, including drop-in fuels in gasoline (C3–C9), jet fuel (C8–C16), diesel (C10–C18), and lubricants (C16–C30) (Kang et al., 2017). In addition to the FACs mentioned above, there are other important fatty acid-based chemicals, such as fatty acid alkyl esters (FAAEs) and branched FACs that are used for certain applications due to their specific properties (Röttig et al., 2010; Ngo et al., 2013; Gupta et al., 2015; Teo et al., 2015; Bentley et al., 2016; Jiang et al., 2017; Shrestha and Yamamoto, 2018; Singh and Choudhury, 2018). For examples, branched fatty alcohol 4-methyl-pentanol is a common brake fluids (Shrestha and Yamamoto, 2018; Supplementary Table 1).
Microbial Chassis for Biosynthesis of FACs
Theoretically speaking, any microorganism can be used as a microbial chassis for biosynthesis of FACs, because fatty acid metabolic pathways exist in all living cells. Currently, most researches are devoted to model organisms such as Escherichia coli and Saccharomyces cerevisiae (Liu et al., 2016; Hu et al., 2019; Kim and Park, 2019; Yang et al., 2020). In our opinion, there are some other promising microorganisms, which have not been explored. In this review, microorganisms are classified into four groups including chemoautotroph, photoautotroph, heterotrophic prokaryotes, and heterotrophic eukaryotes. Their advantages, disadvantages, and the promising synthetic community strategy for microbial production of FACs will be discussed in detail (Figure 2).
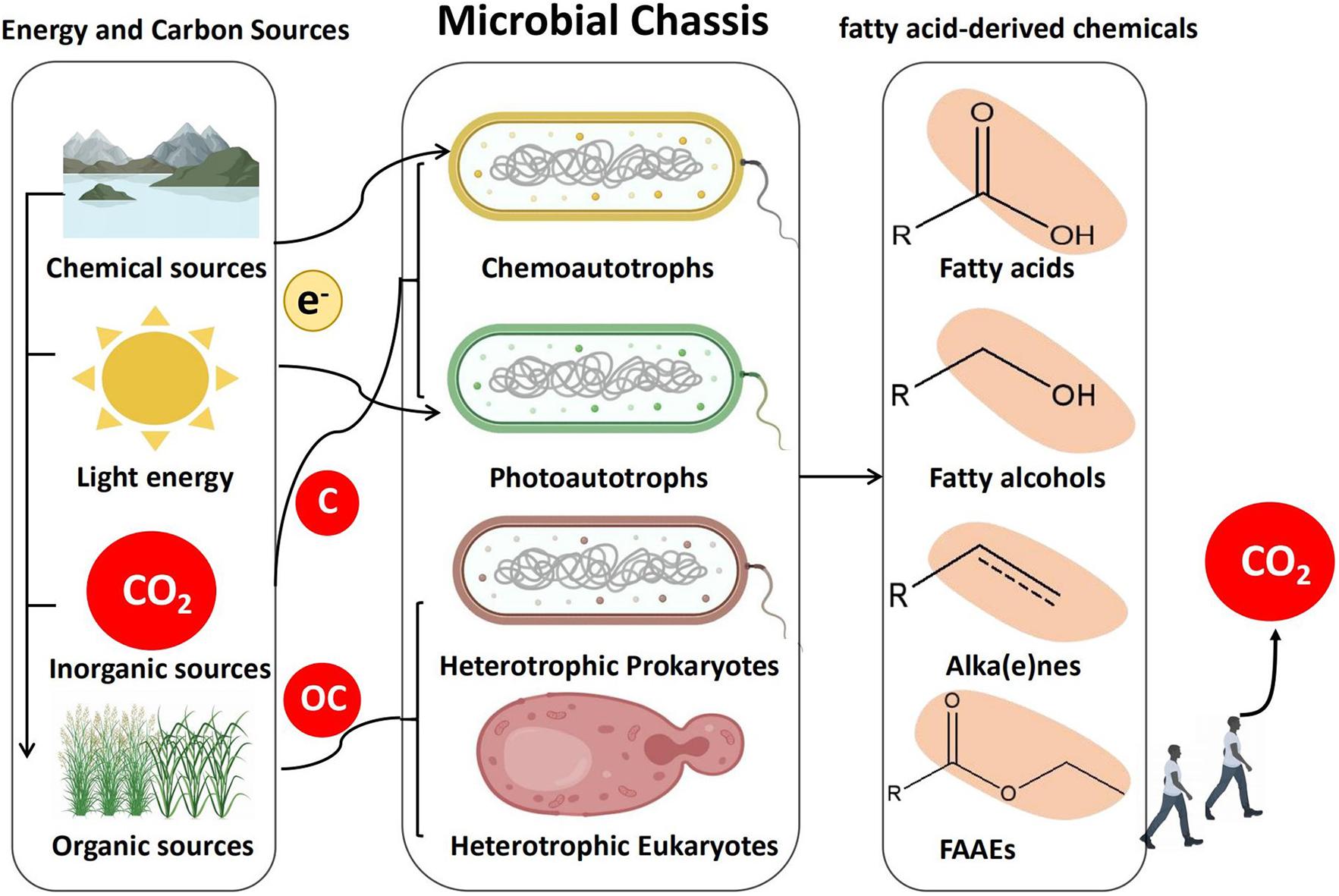
Figure 2. Strategies for production of FACs in microbial chassis. Autotrophs and heterotrophs synthesize FACs from CO2 and organic carbon (OC), respectively. Either platforms have their own advantages and limitations. Autotroph-heterotroph consortia represent a promising platform for microbial production of FACs.
Chemoautotrophs are organisms that can synthesize their own organic molecules through the fixation of carbon dioxide. Energy required for this process comes from the oxidation of inorganic molecules such as iron, sulfur, or magnesium (Thakur et al., 2018). Though research efforts on chemoautotrophic bacteria have started to gain attention, the application of chemoautotrophs at an industrial scale is still challenging, due to their slow growth pattern and the limited applicable genetic engineering tools. Cupriavidus necator, which has one of the highest growth rates among natural autotrophic bacteria, was successfully used to produce FACs from CO2. However, the autotrophic production level of FACs was much lower compared to heterotrophic production on fructose (Crépin et al., 2016). Another chemolithotrophic oleaginous bacterium, Rhodococcus opacus, was engineered to produce fatty acids and fuels as high as 50.2 g/L, however this was carried out under heterotrophic condition with glucose as carbon source (Kim et al., 2019). Recently some chemoautotrophs were observed to utilize electricity as energy resources for biosynthesis, which make them promising microbial chassis (Geelhoed and Stams, 2011). Photoautotrophic microorganisms are cells that capture light energy to fix carbon. Among these microorganisms, cyanobacteria are the most studied because it is easy to genetically modify (Liu et al., 2011; Tan et al., 2011; Wang et al., 2013), and biosynthesis of FACs has already been proven feasible in them (Liu et al., 2011; Eungrasamee et al., 2019). For examples, an important Omega−3 fatty acid was produced by overexpression of desaturase desA and desB in Synechococcus sp. PCC 7002 (Santos-Merino et al., 2018), and fatty alcohols were also successfully produced in photosynthesis-driven cyanobacteria (Tan et al., 2011; Yunus and Jones, 2018). By overexpressing acyl-acyl carrier protein reductase (AAR) and aldehyde decarbonylase (AD), metabolically engineered cyanobacterium, Nostoc punctiforme, produced alkanes at levels up to 12% of their cell dry weight (Peramuna et al., 2015). In the case of the heterotrophs, both heterotrophic prokaryotes and eukaryotes have been widely used for FACs biosynthesis (Wang et al., 2010; Rutter and Rao, 2016; Wu et al., 2017; Xin et al., 2017; McNeil and Stuart, 2018; Zhou et al., 2018; Wu et al., 2019). E. coli, the most commonly used heterotrophic prokaryote, was engineered to produce free fatty acids at a titer of up to 21.5 g/L (Xiao et al., 2016). The most commonly used heterotrophic eukaryote, Saccharomyces cerevisiae was designed and engineered to produce 33.4 g/L of extracellular free fatty acids (Yu et al., 2018). Some oleaginous heterotrophic eukaryotes, such as Yarrowia lipolytica and Aureobasidium pullulans show great potential for FACs biosynthesis, since just with simple adjustment they can reach much higher titers than the engineered S. cerevisiae (Xu et al., 2016; Xin et al., 2017). Although these microbial platforms have their own advantages, they also have their own limitations. For example, autotrophs can synthesize FACs from CO2 via using solar, chemical and electric energy. Due to the abundance of CO2 in the atmosphere and its role in driving global climate change, CO2-assimilating microbes represent a unique and promising type of microbial chassis for FACs biosynthesis. However, autotrophs normally have limited growth rates and genetic engineering tools, resulting in difficulties to engineer metabolic pathways to produce specific FACs. Comparatively, many genetic engineering tools have been developed for fast-growing and metabolically versatile heterotrophs. But using organic carbon substrates makes them less environmental friendliness. Therefore, a platform combining different types of microorganism is required for more economical, environmental, and efficient microbial factories. Fortunately, recent advances in synthetic biology have made synthetic microbial communities possible (Johns et al., 2016; Tang, 2019; Liu et al., 2020). There are microalgae-microalgae, microalgae and bacteria, microalgae and molds communities constructed for FACs production (Magdouli et al., 2016). Ideally, a microbial community system could use autotrophs to fix CO2 from the atmosphere and subsequently transfer the organic products to heterotrophs for FACs biosynthesis. One such system has already been reported, a Synechococcus elongates-Pseudomonas putida consortium was constructed to produce bioplastic (PHA, polyhydroxyakanotate) (García-Jiménez et al., 2018). Another microalgae-yeast co-culture was isolated from wastewater and identified to contain a number of microalgae and yeast species, which was also successfully used for fatty acid methyl esters production (Suastes-Rivas et al., 2019). From our perspective, these autotroph-heterotroph communities have the potential to produce specific FACs from CO2. However, the slow rates of CO2 fixation in autotrophs seriously affect their practical applications. We believe that direct evolution of these synthetic communities could be a promising solution to overcome this limitation (Chang et al., 2020).
Systems Engineering Strategies for Biosynthetic Pathway Optimization
The metabolic pathway for FACs biosynthesis can be broadly divided into three steps: initiation, elongation and termination. It starts with the conversion of feed materials to the universal precursor acetyl-CoA through various conversion pathways. The most common pathway for the synthesis of acetyl-CoA is through glycolysis, which converts glucose into pyruvate, then can be decarboxylated to produce acetyl-CoA. However, the decarboxylation of pyruvate loses a carbon equivalent, thus limits the theoretical carbon yield, and constrains the commercialization potential. Fortunately, a non-oxidative pathway was built up to produce stoichiometric amounts of acetyl-CoA from hexose, pentose and triose phosphates without carbon loss (Bogorad et al., 2013). Another intriguing alternative to sugars is the potential to produce acetyl-CoA from one-carbon resources such as CO2 and formate (Lu et al., 2019). After external carbon sources being converted into acetyl-CoA, it can be directly used as initiation blocks or transformed to propionyl-CoA, acetyl-acyl carrier protein (ACP), and propionyl-ACP for initiation. The initiation pattern determines the odd or even carbon chain of the produced FACs (Supplementary Figure 1; Dellomonaco et al., 2011; Park et al., 2020; Zhang et al., 2020).
In terms of elongation, fatty acid synthesis (FAS) and reverse beta-oxidation (RBO) pathways are the two identified routes for FACs biosynthesis. Although the four serial steps including condensation, reduction, dehydration, and reduction are similar in both FAS and RBO pathways (Figure 3), the iterative feeding strategies are different. In FAS pathway, acetyl-CoA was transferred into malonyl-ACP before being fed into the elongation cycle, while acetyl-CoA was directly fed into the elongation cycle in RBO pathway (Figure 3). FAS has been most widely studied and engineered to produce free fatty acids, alcohols, esters, and alkanes (Liu et al., 2016, 2018; Wenning et al., 2017; Yunus and Jones, 2018). However, RBO is widely accepted as the promising pathway for several reasons. Firstly, one ATP will be saved via the RBO pathway, as acetyl-CoA can be directly fed into the elongation cycle, while for elongation in FAS, acetyl-CoA must first be converted to malonyl-CoA via an ATP-consuming acetyl-CoA carboxylase. Secondly, most reductases from the FAS pathway have been shown to prefer NADPH as cofactors (Ratledge, 2004; Handke et al., 2011; Javidpour et al., 2014). In contrast, reductases from the RBO routes are mostly NADH-dependent (Lian and Zhao, 2014; Sheppard et al., 2016; Kim and Gonzalez, 2018). Since it has been demonstrated that cell has relatively high NADH/NAD+ ratio under anaerobic condition (De Graef et al., 1999), RBO will be benefited in the anaerobic biosynthesis of FACs. Thirdly, RBO pathway is dependent on the universal CoA molecule, while FAS pathway is dependent on organism specific A, making RBO pathway more transferable in target microorganisms. Recently, it was reported that with the exception of condensation step, the remaining enzymes for other steps in FAS pathway: 3-ketoacyl-ACP reductase (FabG), 3-hydroxyacylACP dehydratase (FabZ), and enoyl-ACP reductase identified (FabI) can carry out similar conversions as in RBO in E. coli (Vick et al., 2015; Clomburg et al., 2018). It was reported that some of these enzymes show preference for acyl-ACP intermediates, such as fabZ from E. coli (Tsuge et al., 2003). These findings present both opportunities and challenges. On one hand, it offers the potential to employ FAS enzymes on various acyl-CoA intermediates, which can greatly expand the range of FACs produced by RBO. On the other hand, it will lead to promiscuous activity and make it difficult to produce specific FACs, as intracellular substrates will be automatically used by the endogenous FAS enzymes, which results in impure and unwanted products. Though both of FAS and RBO pathways can be used to produce FACs with different chain lengths, FAS pathway is preferred for long-chain FACs production, because it naturally has high efficiency; while for short-chain FACs, the RBO pathway is favored because it is easier to control product lengths than FAS pathway (Sheppard et al., 2016).
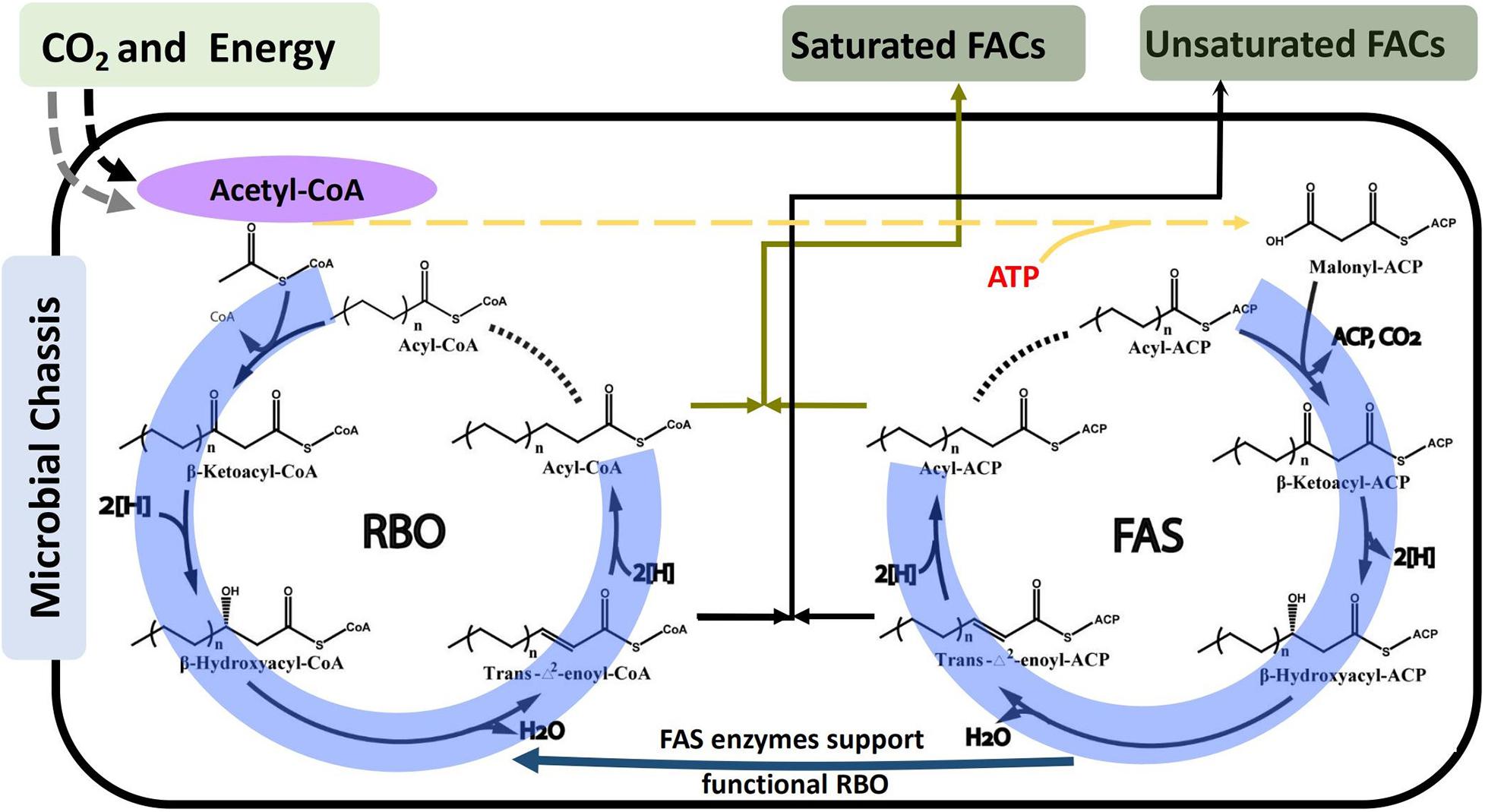
Figure 3. Schematic of metabolic pathways for FACs biosynthesis via Reverse Beta Oxidation (RBO) and Fatty Acid Synthesis (FAS). The entry of CO2 and energy to acetyl-CoA depends on the type of microbial chassis selected, as shown in Figure 2. The catalytic reaction cycles for both RBO and FAS include the same four serial steps: condensation, reduction, dehydration, and reduction. The major difference is that RBO employs a non-decarboxylative condensation mechanism to incorporate acetyl-CoA while FAS utilizes a decarboxylative condensation mechanism to incorporate malonyl-ACP.
The termination step, which releases fatty acyl-CoA or fatty acyl-ACP from the elongation cycle, is the most important and widely investigated step, as it determines the types of FACs produced by microbial cell factories. For each type of FACs, there are multiple options for termination. For example, fatty alkenes and fatty alcohols can be generated from fatty acids, fatty acyl-ACPs and fatty acyl-CoAs (Liu et al., 2016; Liu and Li, 2020). Alkanes can be converted from fatty aldehydes by aldehyde decarbonylase or from fatty acids by photodecarboxylase (Eser et al., 2011; Sorigué et al., 2017). Even though numerous terminal pathway options have been found, it is still the major bottleneck for FACs biosynthesis for the following reasons: First, production of FACs other than FFAs is not efficient. According to our knowledge, the highest titer of mixed long chain FFAs (C14–C22) is 50.2 g/L using an oleaginous bacterium Rhodococcus opacus PD630 (Kim et al., 2019), while the highest titers of fatty alcohols and alka(e)nes is 12.5 and 2.54 g/L, respectively (Fatma et al., 2018). Considering the same upstream pathway, the low titers of fatty alcohols and alka(e)nes are perhaps caused by the low efficiency of enzymes in the termination step. Second, enzymes in terminal step naturally prefer longer chain substrates. Although great efforts have been made for short chain substrates, the problem is far from being resolved (Khara et al., 2013; Gajewski et al., 2017). Hence, screening and engineering of enzymes that prefer short chain substrates should be an important area of research.
Model-Assisted Design for Biosynthesis of FACs
Model-assisted design has shown to be successful in metabolic engineering (Teusink and Smid, 2006; Fatma et al., 2018; Ferreira et al., 2019; Das et al., 2020; Figure 4). Increasing information in databases, such as KEGG, BioCyc, BRENDA, MetRxn, and SEED (Shin et al., 2013; Long et al., 2015; Delépine et al., 2018; Choi et al., 2019), makes it possible to develop organism-specific reaction networks, de novo pathway predictions and even retrosynthetic design of metabolic pathways for non-natural chemicals (Medema et al., 2012; Tabei et al., 2016; Biz et al., 2019; Garcia and Trinh, 2019a). Model-assisted design facilitates efficient Design-Build-Test-Learn (DBTL) cycle, avoiding costly trial and error approaches (Long et al., 2015; Choi et al., 2019). There are two basic metabolic analysis algorithms for model-guided design in metabolic engineering: Flux balance analysis (FBA) and Elementary mode analysis (EMA) (Mahadevan et al., 2002; Klamt and Gilles, 2004; Machado and Herrgård, 2015). FBA uses linear optimization to find a set of reaction fluxes that satisfy both an objective function and a set of constraints limiting the solution space of the network representing a given growth condition (Orth et al., 2010). EMA calculates all the solutions with minimal support in the network that satisfy the steady state and other constraints (Trinh et al., 2009). Each solution in EMA is called an elementary mode (EM) and describes the topology of the metabolic network, which is useful in determining its properties and for rational design (Trinh et al., 2009). Most computational tools are derived from EMA or FBA for more specific purposes (Rodrigo et al., 2008; Trinh et al., 2009; Campodonico et al., 2014; Long et al., 2015; Garcia and Trinh, 2019b, 2020; Jiang et al., 2020).
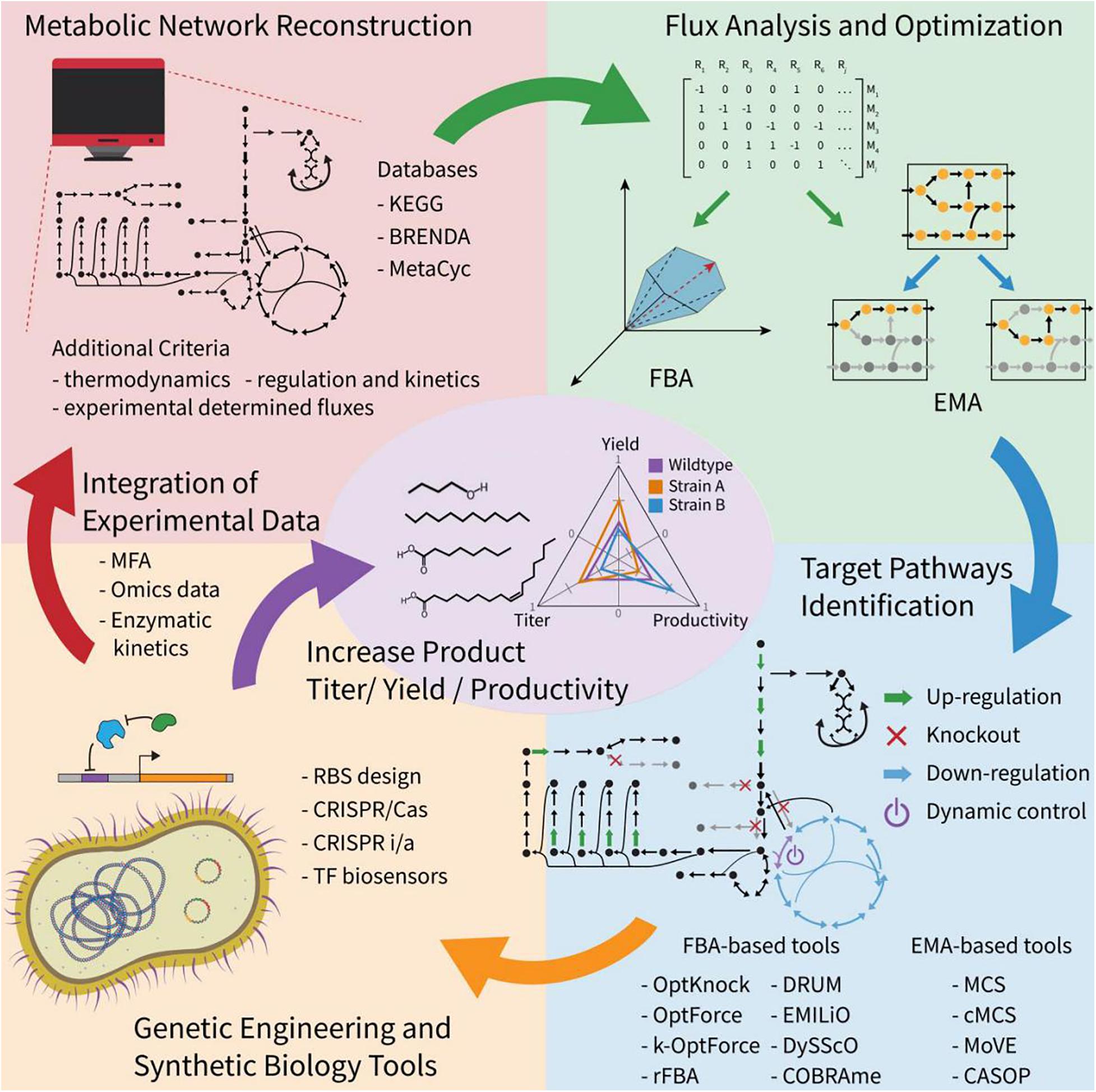
Figure 4. Overview of computational model-assisted design algorithms. Computational methods have been developed to identify pathways to enable production of desired compounds using known or de novo metabolic pathways. Additional host metabolic strategies, including up/down-regulation, knockout, and dynamic control can be designed using a variety of constraint-based modeling algorithms.
Methods based on FBA, such as OptKnock, OptStrain, OptForce, dFBA, DySScO DynamicME, and COBRAme have been developed for strain engineering purposes, to identify a set of genetic interventions to increase the production of target compounds (Mahadevan et al., 2002; Burgard et al., 2003; Pharkya et al., 2004; Ranganathan et al., 2010; Zhuang et al., 2013; Lloyd et al., 2018; Yang et al., 2019). The two most used design programs based on FBA are OptKnock and OptForce. OptKnock is the first bi-level optimization framework for strain design, which can identify optimal reaction deletion strategies that couple cellular growth with the production of a target metabolite (Burgard et al., 2003). A successful case of OptKnock algorithm application is a growth-coupled strategy designed for biofuel production in Synechocystis, and it shows that lowering the ATP/NADPH ratio in the cell is a promising strategy for biosynthesis of fatty alcohols and alkanes (Shabestary and Hudson, 2016). OptForce is a framework predicting genetic interventions such as overexpression and repression based on the comparison of an initial metabolic status and the desired overproduction goal (Ranganathan et al., 2010). It can prioritize the interventions according to their effects on the increment of the production, making it possible to start with the modifications that would have higher impact on the process. The OptForce algorithm has been successfully used for strategy design in E. coli for fatty acids production. Moreover, it can predict less intuitive interventions, such as the redirection of the flux through the Entner-Doudoroff pathway to produce NADPH and induce a growth arrest limiting the ATP production (Ranganathan et al., 2012; Tee et al., 2014). Recently, another study applied OptForce for the production of octanoic acid, achieving high selectivity (>70%) and an extracellular concentration up to 1 g/L of free octanoic acid in minimal medium via fed-batch culture (Liu et al., 2018). A metabolic model was constructed for long-chain alkane and alcohol production based on FBA analysis, and the engineered strain produced the maximum titers of hydrocarbons (Fatma et al., 2018). Besides, recently breakthroughs have been made in visualizing genome-scale metabolic flux networks, which improved understanding of the predicted solutions (Chazalviel et al., 2018; Hari and Lobo, 2020).
EMA-based tools have been used for strain engineering by applying the concept of minimal cut sets (MCS) (Klamt and Gilles, 2004). Constrained MCS (cMCS) was developed to remove limitations in MCS, where many solutions also eliminated growth (Hädicke and Klamt, 2011). Using cMCS, researchers identified sets of reactions to eliminate and enhanced the production of ethanol and isobutanol in Clostridium thermocellum and cyanobacteria, respectively (Erdrich et al., 2014; Thompson and Trinh, 2017). There has been interest in dynamic control strategies, which can dynamically regulate of flux through metabolite sensor, inducer, temperature, light and cell density (Lalwani et al., 2018; Liu et al). These provide the option to prioritize growth or production in a two-stage process, which can lead to higher yields, productivities and titers of FACs (Zhang et al., 2012; Lalwani et al., 2018; Raj et al., 2020). To accelerate the strain engineering process for enhanced chemical production, MODCELL and MODCELL 2 frameworks were developed for rapid generation of optimal production strains by systematically assembling a modular cell with an exchangeable production module (Trinh et al., 2015; Garcia and Trinh, 2019c). Moreover, MoVE, a newly developed tool based on MCS, can identify genetic interventions that allow the transition between growth and production states for dynamic control of the metabolism (Venayak et al., 2018).
Once engineering strategies are obtained from computational modeling, there are plenty of synthetic biology tools available to implement the suggested metabolic engineering interventions. For instance, CRISPR-based technologies make it possible to perform multiple knockouts, inhibitions, or activations of designed sets (Behler et al., 2018; Kaczmarzyk et al., 2018; Reis et al., 2019). Significant improvements have been achieved in FACs biosynthesis using model-based strain design strategies (Matsuda et al., 2011; Shabestary and Hudson, 2016; Fatma et al., 2018; Yu et al., 2018), however, there remain challenges to be addressed in future studies. For example, there is still a lack of methods to integrate large amounts of data into genome-scale models and provide user-friendly tools that allow users with no programming experience to exploit the potential of genome-scale metabolic models for rational design. In conclusion, model-based strain engineering is still in an early stage and its application has been limited to few chemical targets and tools. We expect that the development of novel user-friendly computational models can enable increased adoption of such tools for various types of FACs production.
Conclusion
The ongoing reliance on fossil fuels of human society is driving elevated atmospheric CO2 and increasing global temperatures, thereby escalating the risk of widespread environmental disasters in the near future. We anticipate that microbial synthesis of products from CO2, which can provide chemicals with near-zero net greenhouse gas emissions, will play as a game-changer in the future (Ediger, 2019). Great progress has been made in the areas of enzyme engineering, metabolic engineering, and model-assisted engineering to assist microbial production of FACs (Cao et al., 2016; Herman and Zhang, 2016; Kim et al., 2016; Zhou et al., 2016; Fatma et al., 2018; Marella et al., 2018; Kim and Park, 2019; Liu and Nielsen, 2019; Lynch et al., 2019). However, the present-day microbial cell factories still have major challenges to overcome, such as controlling the length and types of released FACs and improving the conversion efficiency via RBO. We expect that directed enzyme evolution and rational enzyme engineering will contribute to the production of target FACs through the RBO pathway. Recently, there are some machine learning-based algorithms developed for computational protein design, which can also be used in enzyme engineering (Masso and Vaisman, 2008; Fang, 2019; Zu Belzen et al., 2019). In addition, new methods for design and build of synthetic microorganism communities can contribute to the construction of novel microbial platforms, which combine carbon-fixing autotrophs with heterotrophs for efficient FACs biosynthesis with net-zero greenhouse gas emissions.
Author Contributions
YL and RM conceived of the idea. YL, MB, JC, EH, and AK wrote the manuscript. YL, JC, EH, MB, and RM contributed to revising. All authors contributed to the article and approved the submitted version.
Funding
This work was supported by the Ontario Ministry of Research and Innovation.
Conflict of Interest
The authors declare that the research was conducted in the absence of any commercial or financial relationships that could be construed as a potential conflict of interest.
Supplementary Material
The Supplementary Material for this article can be found online at: https://www.frontiersin.org/articles/10.3389/fbioe.2021.613322/full#supplementary-material
Supplementary Figure 1 | Initiation and feed in blocks in Reverse Beta Oxidation (RBO) (A) and Fatty Acid Synthesis (FAS) (B) pathways. Each turn of the FAS and RBO cycles leads to the addition of two carbons through incorporation of malonyl-ACP or acetyl-CoA, respectively. Consequently, the initiation blocks control over whether the produced FACs have odd or even carbon chains.
References
Behler, J., Vijay, D., Hess, W. R., and Akhtar, M. K. (2018). CRISPR-based technologies for metabolic engineering in cyanobacteria. Trends Biotechnol. 36, 996–1010. doi: 10.1016/j.tibtech.2018.05.011
Bellou, S., Triantaphyllidou, I.-E., Aggeli, D., Elazzazy, A. M., Baeshen, M. N., and Aggelis, G. (2016). Microbial oils as food additives: recent approaches for improving microbial oil production and its polyunsaturated fatty acid content. Curr. Opin. Biotechnol. 37, 24–35. doi: 10.1016/j.copbio.2015.09.005
Bentley, G. J., Jiang, W., Guamán, L. P., Xiao, Y., and Zhang, F. (2016). Engineering Escherichia coli to produce branched-chain fatty acids in high percentages. Metab. Eng. 38, 148–158. doi: 10.1016/j.ymben.2016.07.003
Biz, A., Proulx, S., Xu, Z., Siddartha, K., Indrayanti, A. M., and Mahadevan, R. (2019). Systems biology based metabolic engineering for non-natural chemicals. Biotechnol. Adv. 37:107379. doi: 10.1016/j.biotechadv.2019.04.001
Bogorad, I. W., Lin, T.-S., and Liao, J. C. (2013). Synthetic non-oxidative glycolysis enables complete carbon conservation. Nature 502, 693–697. doi: 10.1038/nature12575
Burgard, A. P., Pharkya, P., and Maranas, C. D. (2003). Optknock: a bilevel programming framework for identifying gene knockout strategies for microbial strain optimization. Biotechnol. Bioeng. 84, 647–657. doi: 10.1002/bit.10803
Campodonico, M. A., Andrews, B. A., Asenjo, J. A., Palsson, B. O., and Feist, A. M. (2014). Generation of an atlas for commodity chemical production in Escherichia coli and a novel pathway prediction algorithm. GEM Path. Metab. Eng. 25, 140–158. doi: 10.1016/j.ymben.2014.07.009
Cao, Y.-X., Xiao, W.-H., Zhang, J.-L., Xie, Z.-X., Ding, M.-Z., and Yuan, Y.-J. (2016). Heterologous biosynthesis and manipulation of alkanes in Escherichia coli. Metab. Eng. 38, 19–28. doi: 10.1016/j.ymben.2016.06.002
Carbonell, P., Jervis, A. J., Robinson, C. J., Yan, C., Dunstan, M., Swainston, N., et al. (2018). An automated Design-Build-Test-Learn pipeline for enhanced microbial production of fine chemicals. Commun. Biol. 1, 1–10. doi: 10.1007/978-3-319-31421-1_214-1
Chang, C.-Y., Vila, J. C., Bender, M., Li, R., Mankowski, M. C., Bassette, M., et al. (2020). Top-down engineering of complex communities by directed evolution. bioRxiv [Preprint]. doi: 10.1101/2020.07.24.214775v2
Chazalviel, M., Frainay, C., Poupin, N., Vinson, F., Merlet, B., Gloaguen, Y., et al. (2018). MetExploreViz: web component for interactive metabolic network visualization. Bioinformatics 34, 312–313. doi: 10.1093/bioinformatics/btx588
Chen, Y., Banerjee, D., Mukhopadhyay, A., and Petzold, C. J. (2020). Systems and synthetic biology tools for advanced bioproduction hosts. Curr. Opin. Biotechnol. 64, 101–109. doi: 10.1016/j.copbio.2019.12.007
Cho, I. J., Choi, K. R., and Lee, S. Y. (2020). Microbial production of fatty acids and derivative chemicals. Curr. Opin. Biotechnol. 65, 129–141. doi: 10.1016/j.copbio.2020.02.006
Choi, K. R., Jang, W. D., Yang, D., Cho, J. S., Park, D., and Lee, S. Y. (2019). Systems metabolic engineering strategies: integrating systems and synthetic biology with metabolic engineering. Trends Biotechnol. 37, 817–837. doi: 10.1016/j.tibtech.2019.01.003
Clomburg, J. M., Contreras, S. C., Chou, A., Siegel, J. B., and Gonzalez, R. (2018). Combination of type II fatty acid biosynthesis enzymes and thiolases supports a functional β-oxidation reversal. Metab. Eng. 45, 11–19. doi: 10.1016/j.ymben.2017.11.003
Crépin, L., Lombard, E., and Guillouet, S. E. (2016). Metabolic engineering of Cupriavidus necator for heterotrophic and autotrophic alka (e) ne production. Metab. Eng. 37, 92–101. doi: 10.1016/j.ymben.2016.05.002
Das, M., Patra, P., and Ghosh, A. (2020). Metabolic engineering for enhancing microbial biosynthesis of advanced biofuels. Renewab. Sustain. Energy Rev. 119:109562. doi: 10.1016/j.rser.2019.109562
De Graef, M. R., Alexeeva, S., Snoep, J. L., and De Mattos, M. J. T. (1999). The steady-state internal redox state (NADH/NAD) reflects the external redox state and is correlated with catabolic adaptation in Escherichia coli. J. Bacteriol. 181, 2351–2357. doi: 10.1128/jb.181.8.2351-2357.1999
Delépine, B., Duigou, T., Carbonell, P., and Faulon, J.-L. (2018). RetroPath2.0: a retrosynthesis workflow for metabolic engineers. Metab. Eng. 45, 158–170. doi: 10.1016/j.ymben.2017.12.002
Dellomonaco, C., Clomburg, J. M., Miller, E. N., and Gonzalez, R. (2011). Engineered reversal of the β-oxidation cycle for the synthesis of fuels and chemicals. Nature 476, 355–359. doi: 10.1038/nature10333
Dong, X., and Mumper, R. (2006). The metabolism of fatty alcohols in lipid nanoparticles by alcohol dehydrogenase. Drug Dev. Ind. Pharm. 32, 973–980. doi: 10.1080/03639040600640006
Ediger, V. Ş (2019). An integrated review and analysis of multi-energy transition from fossil fuels to renewables. Energy Proc. 156, 2–6. doi: 10.1016/j.egypro.2018.11.073
Erdrich, P., Knoop, H., Steuer, R., and Klamt, S. (2014). Cyanobacterial biofuels: new insights and strain design strategies revealed by computational modeling. Microb. Cell Fact. 13:128.
Eser, B. E., Das, D., Han, J., Jones, P. R., and Marsh, E. N. G. (2011). Oxygen-independent alkane formation by non-heme iron-dependent cyanobacterial aldehyde decarbonylase: investigation of kinetics and requirement for an external electron donor. Biochemistry 50, 10743–10750. doi: 10.1021/bi2012417
Eungrasamee, K., Miao, R., Incharoensakdi, A., Lindblad, P., and Jantaro, S. (2019). Improved lipid production via fatty acid biosynthesis and free fatty acid recycling in engineered Synechocystis sp. PCC 6803. Biotechnol. Biofuels 12:8.
Fang, J. (2019). A critical review of five machine learning-based algorithms for predicting protein stability changes upon mutation. Briefings Bioinform. 21, 1285–1292. doi: 10.1093/bib/bbz071
Fatma, Z., Hartman, H., Poolman, M. G., Fell, D. A., Srivastava, S., Shakeel, T., et al. (2018). Model-assisted metabolic engineering of Escherichia coli for long chain alkane and alcohol production. Metab. Eng. 46, 1–12. doi: 10.1016/j.ymben.2018.01.002
Ferreira, R., Skrekas, C., Hedin, A., Sánchez, B. J., Siewers, V., Nielsen, J., et al. (2019). Model-assisted fine-tuning of central carbon metabolism in yeast through dCas9-based regulation. ACS Synth. Biol. 8, 2457–2463. doi: 10.1021/acssynbio.9b00258
Fillet, S., and Adrio, J. L. (2016). Microbial production of fatty alcohols. World J. Microbiol. Biotechnol. 32:152.
Gajewski, J., Pavlovic, R., Fischer, M., Boles, E., and Grininger, M. (2017). Engineering fungal de novo fatty acid synthesis for short chain fatty acid production. Nat. Commun. 8, 1–8.
Garcia, S., and Trinh, C. T. (2019a). Comparison of multi-objective evolutionary algorithms to solve the modular cell design problem for novel biocatalysis. Processes 7:361. doi: 10.3390/pr7060361
Garcia, S., and Trinh, C. T. (2019b). Modular design: implementing proven engineering principles in biotechnology. Biotechnol. Adv. 37:107403. doi: 10.1016/j.biotechadv.2019.06.002
Garcia, S., and Trinh, C. T. (2019c). Multiobjective strain design: a framework for modular cell engineering. Metab. Eng. 51, 110–120. doi: 10.1016/j.ymben.2018.09.003
Garcia, S., and Trinh, C. T. (2020). Harnessing natural modularity of metabolism with goal attainment optimization to design a modular chassis cell for production of diverse chemicals. ACS Synth. Biol. 9, 1665–1681. doi: 10.1021/acssynbio.9b00518
García-Jiménez, B., García, J. L., and Nogales, J. (2018). FLYCOP: metabolic modeling-based analysis and engineering microbial communities. Bioinformatics 34, i954–i963.
Geelhoed, J. S., and Stams, A. J. (2011). Electricity-assisted biological hydrogen production from acetate by Geobacter sulfurreducens. Environ. Sci. Technol. 45, 815–820. doi: 10.1021/es102842p
Geller, D. P., and Goodrum, J. W. (2004). Effects of specific fatty acid methyl esters on diesel fuel lubricity. Fuel 83, 2351–2356. doi: 10.1016/j.fuel.2004.06.004
Guo, X., Zhang, R. M., Gao, L. G., Zhang, X., and Xu, X. (2019). Computational kinetics of the hydrogen abstraction reactions of n-propanol and iso-propanol by OH radical. Phys. Chem. Chem. Phys. 21, 24458–24468. doi: 10.1039/c9cp04809j
Gupta, C., Prakash, D., and Gupta, S. (2015). A biotechnological approach to microbial based perfumes and flavours. J. Microbiol. Exp. 3, 11–18.
Hädicke, O., and Klamt, S. (2011). Computing complex metabolic intervention strategies using constrained minimal cut sets. Metab. Eng. 13, 204–213. doi: 10.1016/j.ymben.2010.12.004
Hamedirad, M., Chao, R., Weisberg, S., Lian, J., Sinha, S., and Zhao, H. (2019). Towards a fully automated algorithm driven platform for biosystems design. Nat. Commun. 10, 1–10.
Handke, P., Lynch, S. A., and Gill, R. T. (2011). Application and engineering of fatty acid biosynthesis in Escherichia coli for advanced fuels and chemicals. Metab. Eng. 13, 28–37. doi: 10.1016/j.ymben.2010.10.007
Hari, A., and Lobo, D. (2020). Fluxer: A Web Application to Compute, Analyze and Visualize Genome-Scale Metabolic Flux Networks. Baltimore, MD: UMBC Faculty Collection.
Herman, N. A., and Zhang, W. (2016). Enzymes for fatty acid-based hydrocarbon biosynthesis. Curr. Opin. Chem. Biol. 35, 22–28. doi: 10.1016/j.cbpa.2016.08.009
Hu, Y., Zhu, Z., Nielsen, J., and Siewers, V. (2019). Engineering Saccharomyces cerevisiae cells for production of fatty acid-derived biofuels and chemicals. Open Biol. 9:190049. doi: 10.1098/rsob.190049
Javidpour, P., Pereira, J. H., Goh, E.-B., Mcandrew, R. P., Ma, S. M., Friedland, G. D., et al. (2014). Biochemical and structural studies of NADH-dependent FabG used to increase the bacterial production of fatty acids under anaerobic conditions. Appl. Environ. Microbiol. 80, 497–505. doi: 10.1128/aem.03194-13
Jiang, L., Fu, H., Yang, H. K., Xu, W., Wang, J., and Yang, S.-T. (2018). Butyric acid: applications and recent advances in its bioproduction. Biotechnol. Adv. 36, 2101–2117. doi: 10.1016/j.biotechadv.2018.09.005
Jiang, S., Wang, Y., Kaiser, M., and Krasnogor, N. (2020). NIHBA: a network interdiction approach for metabolic engineering design. Bioinformatics 36, 3482–3492. doi: 10.1093/bioinformatics/btaa163
Jiang, W., Gu, P., and Zhang, F. (2018). Steps towards ‘drop-in’biofuels: focusing on metabolic pathways. Curr. Opin. Biotechnol 53, 26–32. doi: 10.1016/j.copbio.2017.10.010
Jiang, W., Qiao, J. B., Bentley, G. J., Liu, D., and Zhang, F. (2017). Modular pathway engineering for the microbial production of branched-chain fatty alcohols. Biotechnol. Biofuels 10:244.
Johns, N. I., Blazejewski, T., Gomes, A. L., and Wang, H. H. (2016). Principles for designing synthetic microbial communities. Curr. Opin. Microbiol. 31, 146–153. doi: 10.1016/j.mib.2016.03.010
Kaczmarzyk, D., Cengic, I., Yao, L., and Hudson, E. P. (2018). Diversion of the long-chain acyl-ACP pool in Synechocystis to fatty alcohols through CRISPRi repression of the essential phosphate acyltransferase PlsX. Metab. Eng. 45, 59–66. doi: 10.1016/j.ymben.2017.11.014
Kang, M.-K., Zhou, Y. J., Buijs, N. A., and Nielsen, J. (2017). Functional screening of aldehyde decarbonylases for long-chain alkane production by Saccharomyces cerevisiae. Microb. Cell Fact. 16:74.
Khara, B., Menon, N., Levy, C., Mansell, D., Das, D., Marsh, E. N. G., et al. (2013). Production of propane and other short-chain alkanes by structure-based engineering of ligand specificity in aldehyde-deformylating oxygenase. ChemBioChem 14, 1204–1208. doi: 10.1002/cbic.201300307
Kim, H. M., Chae, T. U., Choi, S. Y., Kim, W. J., and Lee, S. Y. (2019). Engineering of an oleaginous bacterium for the production of fatty acids and fuels. Nat. Chem. Biol. 15, 721–729. doi: 10.1038/s41589-019-0295-5
Kim, S., Cheong, S., Chou, A., and Gonzalez, R. (2016). Engineered fatty acid catabolism for fuel and chemical production. Curr. Opin. Biotechnol. 42, 206–215. doi: 10.1016/j.copbio.2016.07.004
Kim, S., and Gonzalez, R. (2018). Selective production of decanoic acid from iterative reversal of β-oxidation pathway. Biotechnol. Bioeng. 115, 1311–1320. doi: 10.1002/bit.26540
Kim, S.-K., and Park, Y.-C. (2019). Biosynthesis of ω-hydroxy fatty acids and related chemicals from natural fatty acids by recombinant Escherichia coli. Appl. Microbiol. Biotechnol. 103, 191–199. doi: 10.1007/s00253-018-9503-6
Klamt, S., and Gilles, E. D. (2004). Minimal cut sets in biochemical reaction networks. Bioinformatics 20, 226–234. doi: 10.1093/bioinformatics/btg395
Lalwani, M. A., Zhao, E. M., and Avalos, J. L. (2018). Current and future modalities of dynamic control in metabolic engineering. Curr. Opin. Biotechnol. 52, 56–65. doi: 10.1016/j.copbio.2018.02.007
Leber, C., Polson, B., Fernandez-Moya, R., and Da Silva, N. A. (2015). Overproduction and secretion of free fatty acids through disrupted neutral lipid recycle in Saccharomyces cerevisiae. Metab. Eng. 28, 54–62. doi: 10.1016/j.ymben.2014.11.006
Li, G., Huang, D., Sui, X., Li, S., Huang, B., Zhang, X., et al. (2020). Advances in microbial production of medium-chain dicarboxylic acids for nylon materials. React. Chem. Eng. 5, 221–238. doi: 10.1039/c9re00338j
Li, M., Hou, F., Wu, T., Jiang, X., Li, F., Liu, H., et al. (2020). Recent advances of metabolic engineering strategies in natural isoprenoid production using cell factories. Nat. Product Rep. 37, 80–99. doi: 10.1039/c9np00016j
Lian, J., and Zhao, H. (2014). Reversal of the β-oxidation cycle in Saccharomyces cerevisiae for production of fuels and chemicals. ACS Synth. Biol. 4, 332–341. doi: 10.1021/sb500243c
Liu, D., Mao, Z., Guo, J., Wei, L., Ma, H., Tang, Y., et al. (2018). Construction, model-based analysis, and characterization of a promoter library for fine-tuned gene expression in Bacillus subtilis. ACS Synth. Biol. 7, 1785–1797. doi: 10.1021/acssynbio.8b00115
Liu, K., and Li, S. (2020). Biosynthesis of fatty acid-derived hydrocarbons: perspectives on enzymology and enzyme engineering. Curr. Opin. Biotechnol. 62, 7–14. doi: 10.1016/j.copbio.2019.07.005
Liu, X., Sheng, J., and Curtiss Iii, R. (2011). Fatty acid production in genetically modified cyanobacteria. Proc. Natl. Acad. Sci. U.S.A. 108, 6899–6904. doi: 10.1073/pnas.1103014108
Liu, Y., Chen, J., Crisante, D., Lopez, J. M. J., and Mahadevan, R. (2020). Dynamic cell programming with quorum sensing-controlled CRISPRi circuit. ACS Synth. Biol. 9, 1284–1291. doi: 10.1021/acssynbio.0c00148
Liu, Y., Chen, S., Chen, J., Zhou, J., Wang, Y., Yang, M., et al. (2016). High production of fatty alcohols in Escherichia coli with fatty acid starvation. Microb. Cell Fact. 15:129.
Liu, Y., and Nielsen, J. (2019). Recent trends in metabolic engineering of microbial chemical factories. Curr. Opin. Biotechnol. 60, 188–197. doi: 10.1016/j.copbio.2019.05.010
Lloyd, C. J., Ebrahim, A., Yang, L., King, Z. A., Catoiu, E., O’brien, E. J., et al. (2018). COBRAme: a computational framework for genome-scale models of metabolism and gene expression. PLoS Comput. Biol. 14:e1006302. doi: 10.1371/journal.pcbi.1006302
Long, M. R., Ong, W. K., and Reed, J. L. (2015). Computational methods in metabolic engineering for strain design. Curr. Opin. Biotechnol. 34, 135–141. doi: 10.1016/j.copbio.2014.12.019
Lu, X., Liu, Y., Yang, Y., Wang, S., Wang, Q., Wang, X., et al. (2019). Constructing a synthetic pathway for acetyl-coenzyme A from one-carbon through enzyme design. Nat. Commun. 10, 1–10.
Lynch, M., Louie, M., Copley, S., Spindler, E., Prather, B., Lipscomb, M., et al. (2019). Microorganisms and Methods for The Production of Fatty Acids and Fatty Acid Derived Products. Google Patents. Wayzata, MN: Cargill, Inc.
Machado, D., and Herrgård, M. J. (2015). Co-evolution of strain design methods based on flux balance and elementary mode analysis. Metab. Eng. Commun. 2, 85–92. doi: 10.1016/j.meteno.2015.04.001
Magdouli, S., Brar, S. K., and Blais, J.-F. (2016). Co-culture for lipid production: advances and challenges. Biomass Bioenergy 92, 20–30. doi: 10.1016/j.biombioe.2016.06.003
Mahadevan, R., Edwards, J. S., and Doyle Iii, F. J. (2002). Dynamic flux balance analysis of diauxic growth in Escherichia coli. Biophys. J. 83, 1331–1340. doi: 10.1016/s0006-3495(02)73903-9
Marella, E. R., Holkenbrink, C., Siewers, V., and Borodina, I. (2018). Engineering microbial fatty acid metabolism for biofuels and biochemicals. Curr. Opin. Biotechnol. 50, 39–46. doi: 10.1016/j.copbio.2017.10.002
Masso, M., and Vaisman, I. I. (2008). Accurate prediction of stability changes in protein mutants by combining machine learning with structure based computational mutagenesis. Bioinformatics 24, 2002–2009. doi: 10.1093/bioinformatics/btn353
Matsuda, F., Furusawa, C., Kondo, T., Ishii, J., Shimizu, H., and Kondo, A. (2011). Engineering strategy of yeast metabolism for higher alcohol production. Microb. Cell Fact. 10:70. doi: 10.1186/1475-2859-10-70
McNeil, B. A., and Stuart, D. T. (2018). Optimization of C16 and C18 fatty alcohol production by an engineered strain of Lipomyces starkeyi. J. Ind. Microbiol. Biotechnol. 45, 1–14. doi: 10.1007/s10295-017-1985-1
Medema, M. H., Van Raaphorst, R., Takano, E., and Breitling, R. (2012). Computational tools for the synthetic design of biochemical pathways. Nat. Rev. Microbiol. 10, 191–202. doi: 10.1038/nrmicro2717
Ngo, H. L., Dunn, R. O., and Hoh, E. (2013). C18-unsaturated branched-chain fatty acid isomers: characterization and physical properties. Eur. J. Lipid Sci. Technol. 115, 676–683. doi: 10.1002/ejlt.201200323
Opgenorth, P., Costello, Z., Okada, T., Goyal, G., Chen, Y., Gin, J., et al. (2019). Lessons from two design–build–test–learn cycles of dodecanol production in Escherichia coli aided by machine learning. ACS Synth. Biol. 8, 1337–1351. doi: 10.1021/acssynbio.9b00020
Orth, J. D., Thiele, I., and Palsson, B. Ø (2010). What is flux balance analysis? Nat. Biotechnol. 28, 245–248. doi: 10.1038/nbt.1614
Park, Y.-K., Ledesma-Amaro, R., and Nicaud, J.-M. (2020). De novo biosynthesis of odd-chain fatty acids in Yarrowia lipolytica enabled by modular pathway engineering. Front. Bioeng. Biotechnol. 7:484. doi: 10.3389/fbioe.2019.00484
Peramuna, A., Morton, R., and Summers, M. (2015). Enhancing alkane production in cyanobacterial lipid droplets: a model platform for industrially relevant compound production. Life 5, 1111–1126. doi: 10.3390/life5021111
Pharkya, P., Burgard, A. P., and Maranas, C. D. (2004). OptStrain: a computational framework for redesign of microbial production systems. Genome Res. 14, 2367–2376. doi: 10.1101/gr.2872004
Raj, K., Venayak, N., and Mahadevan, R. (2020). Phenotypic design choices for enhanced two-stage microbial chemical production processes. bioRxiv [Preprint]. doi: 10.1101/803023v2
Ranganathan, S., Suthers, P. F., and Maranas, C. D. (2010). OptForce: an optimization procedure for identifying all genetic manipulations leading to targeted overproductions. PLoS Comput. Biol. 6:e1000744. doi: 10.1371/journal.pcbi.1000744
Ranganathan, S., Tee, T. W., Chowdhury, A., Zomorrodi, A. R., Yoon, J. M., Fu, Y., et al. (2012). An integrated computational and experimental study for overproducing fatty acids in Escherichia coli. Metab. Eng. 14, 687–704. doi: 10.1016/j.ymben.2012.08.008
Raslavičius, L., Keršys, A., Mockus, S., Keršienė, N., and Starevičius, M. (2014). Liquefied petroleum gas (LPG) as a medium-term option in the transition to sustainable fuels and transport. Renew. Sustain. Energy Rev. 32, 513–525. doi: 10.1016/j.rser.2014.01.052
Ratledge, C. (2004). Fatty acid biosynthesis in microorganisms being used for single cell oil production. Biochimie 86, 807–815. doi: 10.1016/j.biochi.2004.09.017
Reis, A. C., Halper, S. M., Vezeau, G. E., Cetnar, D. P., Hossain, A., Clauer, P. R., et al. (2019). Simultaneous repression of multiple bacterial genes using nonrepetitive extra-long sgRNA arrays. Nat. Biotechnol. 37, 1294–1301. doi: 10.1038/s41587-019-0286-9
Richardson, A. S., and Mcallister, W. H. (1945). Detergent Composition. Google Patents EP19980947649. Wayzata, MN: Cargill, Inc.
Rodrigo, G., Carrera, J., Prather, K. J., and Jaramillo, A. (2008). DESHARKY: automatic design of metabolic pathways for optimal cell growth. Bioinformatics 24, 2554–2556. doi: 10.1093/bioinformatics/btn471
Rodriguez-Moya, M., and Gonzalez, R. (2015). Proteomic analysis of the response of Escherichia coli to short-chain fatty acids. J. Proteom. 122, 86–99. doi: 10.1016/j.jprot.2015.03.033
Röttig, A., Wenning, L., Bröker, D., and Steinbüchel, A. (2010). Fatty acid alkyl esters: perspectives for production of alternative biofuels. Appl. Microbiol. Biotechnol. 85, 1713–1733. doi: 10.1007/s00253-009-2383-z
Rutter, C. D., and Rao, C. V. (2016). Production of 1-decanol by metabolically engineered Yarrowia lipolytica. Metab. Eng. 38, 139–147. doi: 10.1016/j.ymben.2016.07.011
Santos-Merino, M., Garcillán-Barcia, M. P., and De La Cruz, F. (2018). Engineering the fatty acid synthesis pathway in Synechococcus elongatus PCC 7942 improves omega-3 fatty acid production. Biotechnol. Biofuels 11:239.
Sathesh-Prabu, C., Shin, K. S., Kwak, G. H., Jung, S.-K., and Lee, S. K. (2019). Microbial production of fatty acid via metabolic engineering and synthetic biology. Biotechnol. Bioprocess Eng. 24, 1–18.
Schönfeld, P., and Wojtczak, L. (2016). Short-and medium-chain fatty acids in energy metabolism: the cellular perspective. J. Lipid Res. 57, 943–954. doi: 10.1194/jlr.r067629
Sgobba, E., and Wendisch, V. F. (2020). Synthetic microbial consortia for small molecule production. Curr. Opin. Biotechnol. 62, 72–79. doi: 10.1016/j.copbio.2019.09.011
Shabestary, K., and Hudson, E. P. (2016). Computational metabolic engineering strategies for growth-coupled biofuel production by synechocystis. Metab. Eng. Commun. 3, 216–226. doi: 10.1016/j.meteno.2016.07.003
Sheppard, M. J., Kunjapur, A. M., and Prather, K. L. (2016). Modular and selective biosynthesis of gasoline-range alkanes. Metab. Eng. 33, 28–40. doi: 10.1016/j.ymben.2015.10.010
Shin, J. H., Kim, H. U., Kim, D. I., and Lee, S. Y. (2013). Production of bulk chemicals via novel metabolic pathways in microorganisms. Biotechnol. Adv. 31, 925–935. doi: 10.1016/j.biotechadv.2012.12.008
Shrestha, K., and Yamamoto, S. (2018). Tractor Hydraulic Fluid Compositions. Google Patents. Wayzata, MN: Cargill, Inc.
Singh, N., and Choudhury, B. (2018). Potential of Lentibacillus sp. NS12IITR for production of lipids with enriched branched-chain fatty acids for improving biodiesel properties along with hydrocarbon co-production. Extremophiles 22, 865–875. doi: 10.1007/s00792-018-1043-6
Sorigué, D., Legeret, B., Cuiné, S., Blangy, S., Moulin, S., Billon, E., et al. (2017). An algal photoenzyme converts fatty acids to hydrocarbons. Science 357, 903–907. doi: 10.1126/science.aan6349
Sperry, J. S., Venturas, M. D., Todd, H. N., Trugman, A. T., Anderegg, W. R., Wang, Y., et al. (2019). The impact of rising CO2 and acclimation on the response of US forests to global warming. Proc. Natl. Acad. Sci. U.S.A. 116, 25734–25744. doi: 10.1073/pnas.1913072116
Steen, E. J., Kang, Y., Bokinsky, G., Hu, Z., Schirmer, A., Mcclure, A., et al. (2010). Microbial production of fatty-acid-derived fuels and chemicals from plant biomass. Nature 463:559. doi: 10.1038/nature08721
Suastes-Rivas, J. K., Hernández-Altamirano, R., Mena-Cervantes, V. Y., Valdez-Ojeda, R., Toledano-Thompson, T., Tovar-Gálvez, L. R., et al. (2019). Efficient production of fatty acid methyl esters by a wastewater-isolated microalgae-yeast co-culture. Environ. Sci. Pollut. Res. 27, 28490–28499. doi: 10.1007/s11356-019-07286-1
Tabei, Y., Yamanishi, Y., and Kotera, M. (2016). Simultaneous prediction of enzyme orthologs from chemical transformation patterns for de novo metabolic pathway reconstruction. Bioinformatics 32, i278–i287.
Tan, X., Yao, L., Gao, Q., Wang, W., Qi, F., and Lu, X. (2011). Photosynthesis driven conversion of carbon dioxide to fatty alcohols and hydrocarbons in cyanobacteria. Metab. Eng. 13, 169–176. doi: 10.1016/j.ymben.2011.01.001
Tee, T. W., Chowdhury, A., Maranas, C. D., and Shanks, J. V. (2014). Systems metabolic engineering design: fatty acid production as an emerging case study. Biotechnol. Bioeng. 111, 849–857. doi: 10.1002/bit.25205
Teo, W. S., Ling, H., Yu, A.-Q., and Chang, M. W. (2015). Metabolic engineering of Saccharomyces cerevisiae for production of fatty acid short-and branched-chain alkyl esters biodiesel. Biotechnol. Biofuels 8:177.
Teusink, B., and Smid, E. J. (2006). Modelling strategies for the industrial exploitation of lactic acid bacteria. Nat. Rev. Microbiol. 4, 46–56. doi: 10.1038/nrmicro1319
Thakur, I. S., Kumar, M., Varjani, S. J., Wu, Y., Gnansounou, E., and Ravindran, S. (2018). Sequestration and utilization of carbon dioxide by chemical and biological methods for biofuels and biomaterials by chemoautotrophs: opportunities and challenges. Bioresour. Technol. 256, 478–490. doi: 10.1016/j.biortech.2018.02.039
Thompson, R. A., and Trinh, C. T. (2017). Overflow metabolism and growth cessation in Clostridium thermocellum DSM1313 during high cellulose loading fermentations. Biotechnol. Bioeng. 114, 2592–2604. doi: 10.1002/bit.26374
Trinh, C. T., Liu, Y., and Conner, D. J. (2015). Rational design of efficient modular cells. Metab. Eng. 32, 220–231. doi: 10.1016/j.ymben.2015.10.005
Trinh, C. T., Wlaschin, A., and Srienc, F. (2009). Elementary mode analysis: a useful metabolic pathway analysis tool for characterizing cellular metabolism. Appl. Microbiol. Biotechnol. 81:813. doi: 10.1007/s00253-008-1770-1
Tsuge, T., Taguchi, K., and Doi, Y. (2003). Molecular characterization and properties of (R)-specific enoyl-CoA hydratases from Pseudomonas aeruginosa: metabolic tools for synthesis of polyhydroxyalkanoates via fatty acid ß-oxidation. Int. J. Biol. Macromol. 31, 195–205. doi: 10.1016/s0141-8130(02)00082-x
Venayak, N., Von Kamp, A., Klamt, S., and Mahadevan, R. (2018). MoVE identifies metabolic valves to switch between phenotypic states. Nat. Commun. 9, 1–9.
Verma, M. L., Kishor, K., Sharma, D., Kumar, S. and Sharma, K. D. (2020). “Microbial production of omega-3 polyunsaturated fatty acids,” in Biotechnological Production of Bioactive Compounds, eds M. L. Verma and A. Chandel, (Amsterdam: Elsevier), 293–326. doi: 10.1016/b978-0-444-64323-0.00010-2
Vick, J. E., Clomburg, J. M., Blankschien, M. D., Chou, A., Kim, S., and Gonzalez, R. (2015). Escherichia coli enoyl-acyl carrier protein reductase (FabI) supports efficient operation of a functional reversal of the β-oxidation cycle. Appl. Environ. Microbiol. 81, 1406–1416. doi: 10.1128/aem.03521-14
Wang, J.-P., Wu, L.-X., Xu, F., Lv, J., Jin, H.-J., and Chen, S.-F. (2010). Metabolic engineering for ethylene production by inserting the ethylene-forming enzyme gene (efe) at the 16S rDNA sites of Pseudomonas putida KT2440. Bioresour. Technol. 101, 6404–6409. doi: 10.1016/j.biortech.2010.03.030
Wang, W., Liu, X., and Lu, X. (2013). Engineering cyanobacteria to improve photosynthetic production of alka (e) nes. Biotechnol. Biofuels 6:69. doi: 10.1186/1754-6834-6-69
Wenning, L., Yu, T., David, F., Nielsen, J., and Siewers, V. (2017). Establishing very long-chain fatty alcohol and wax ester biosynthesis in Saccharomyces cerevisiae. Biotechnol. Bioeng. 114, 1025–1035. doi: 10.1002/bit.26220
Wu, J., Wang, Z., Duan, X., Zhou, P., Liu, P., Pang, Z., et al. (2019). Construction of artificial micro-aerobic metabolism for energy-and carbon-efficient synthesis of medium chain fatty acids in Escherichia coli. Metab. Eng. 53, 1–13. doi: 10.1016/j.ymben.2019.01.006
Wu, J., Zhang, X., Xia, X., and Dong, M. (2017). A systematic optimization of medium chain fatty acid biosynthesis via the reverse beta-oxidation cycle in Escherichia coli. Metab. Eng. 41, 115–124. doi: 10.1016/j.ymben.2017.03.012
Wu, X., Zha, J., and Koffas, M. A. (2020). Microbial production of bioactive chemicals for human health. Curr. Opin. Food Sci. 32, 9–16. doi: 10.1016/j.cofs.2019.12.007
Xiao, Y., Bowen, C. H., Liu, D., and Zhang, F. (2016). Exploiting nongenetic cell-to-cell variation for enhanced biosynthesis. Nat. Chem. Biol. 12:339. doi: 10.1038/nchembio.2046
Xin, F.-H., Zhang, Y., Xue, S.-J., Chi, Z., Liu, G.-L., Hu, Z., et al. (2017). Heavy oils (mainly alkanes) over-production from inulin by Aureobasidium melanogenum 9-1 and its transformant 88 carrying an inulinase gene. Renew. Energy 105, 561–568. doi: 10.1016/j.renene.2017.01.004
Xu, P., Qiao, K., Ahn, W. S., and Stephanopoulos, G. (2016). Engineering Yarrowia lipolytica as a platform for synthesis of drop-in transportation fuels and oleochemicals. Proc. Natl. Acad. Sci. U.S.A. 113, 10848–10853. doi: 10.1073/pnas.1607295113
Yang, D., Park, S. Y., Park, Y. S., Eun, H., and Lee, S. Y. (2020). Metabolic engineering of Escherichia coli for natural product biosynthesis. Trends Biotechnol. 38, 745–765. doi: 10.1016/j.tibtech.2019.11.007
Yang, L., Ebrahim, A., Lloyd, C. J., Saunders, M. A., and Palsson, B. O. (2019). DynamicME: dynamic simulation and refinement of integrated models of metabolism and protein expression. BMC Syst. Biol. 13:2. doi: 10.1186/s12918-018-0675-6
Yu, T., Zhou, Y. J., Huang, M., Liu, Q., Pereira, R., David, F., et al. (2018). Reprogramming yeast metabolism from alcoholic fermentation to lipogenesis. Cell 174, 1549.e14–1558.e14.
Yunus, I. S., and Jones, P. R. (2018). Photosynthesis-dependent biosynthesis of medium chain-length fatty acids and alcohols. Metab. Eng. 49, 59–68. doi: 10.1016/j.ymben.2018.07.015
Zerhusen, C., Bollmann, T., Gödderz, A., Fleischer, P., Glüsen, B., and Schörken, U. (2020). Microbial synthesis of nonionic long-chain sophorolipid emulsifiers obtained from fatty alcohol and mixed lipid feeding. Eur. J. Lipid Sci. Technol. 122:1900110. doi: 10.1002/ejlt.201900110
Zhang, F., Carothers, J. M., and Keasling, J. D. (2012). Design of a dynamic sensor-regulator system for production of chemicals and fuels derived from fatty acids. Nat. Biotechnol. 30:354. doi: 10.1038/nbt.2149
Zhang, L.-S., Liang, S., Zong, M.-H., Yang, J.-G., and Lou, W.-Y. (2020). Microbial synthesis of functional odd-chain fatty acids: a review. World J. Microbiol. Biotechnol. 36, 1–9.
Zheng, Y.-N., Li, L.-L., Liu, Q., Yang, J.-M., Wang, X.-W., Liu, W., et al. (2012). Optimization of fatty alcohol biosynthesis pathway for selectively enhanced production of C12/14 and C16/18 fatty alcohols in engineered Escherichia coli. Microb. Cell Fact. 11:65. doi: 10.1186/1475-2859-11-65
Zhou, A., Zhang, C., Li, Y., Li, S., and Yin, P. (2019). Effect of hydrogen peroxide additive on the combustion and emission characteristics of an n-butanol homogeneous charge compression ignition engine. Energy 169, 572–579. doi: 10.1016/j.energy.2018.12.076
Zhou, Y. J., Buijs, N. A., Zhu, Z., Qin, J., Siewers, V., and Nielsen, J. (2016). Production of fatty acid-derived oleochemicals and biofuels by synthetic yeast cell factories. Nat. Commun. 7, 1–9.
Zhou, Y. J., Hu, Y., Zhu, Z., Siewers, V., and Nielsen, J. (2018). Engineering 1-alkene biosynthesis and secretion by dynamic regulation in yeast. ACS Synth. Biol. 7, 584–590. doi: 10.1021/acssynbio.7b00338
Zhuang, K., Yang, L., Cluett, W. R., and Mahadevan, R. (2013). Dynamic strain scanning optimization: an efficient strain design strategy for balanced yield, titer, and productivity. DySScO strategy for strain design. BMC Biotechnol. 13:8. doi: 10.1186%2F1472-6750-13-8
Keywords: fatty acid-derived chemicals, microbial chassis, systems engineering, model-assisted design, review
Citation: Liu Y, Benitez MG, Chen J, Harrison E, Khusnutdinova AN and Mahadevan R (2021) Opportunities and Challenges for Microbial Synthesis of Fatty Acid-Derived Chemicals (FACs). Front. Bioeng. Biotechnol. 9:613322. doi: 10.3389/fbioe.2021.613322
Received: 02 October 2020; Accepted: 04 January 2021;
Published: 26 January 2021.
Edited by:
Dipesh Dhakal, University of Florida, United StatesReviewed by:
Zongbao K. Zhao, Chinese Academy of Sciences, ChinaDae-Hee Lee, Korea Research Institute of Bioscience and Biotechnology (KRIBB), South Korea
Copyright © 2021 Liu, Benitez, Chen, Harrison, Khusnutdinova and Mahadevan. This is an open-access article distributed under the terms of the Creative Commons Attribution License (CC BY). The use, distribution or reproduction in other forums is permitted, provided the original author(s) and the copyright owner(s) are credited and that the original publication in this journal is cited, in accordance with accepted academic practice. No use, distribution or reproduction is permitted which does not comply with these terms.
*Correspondence: Radhakrishnan Mahadevan, a3Jpc2huYS5tYWhhZGV2YW5AdXRvcm9udG8uY2E=