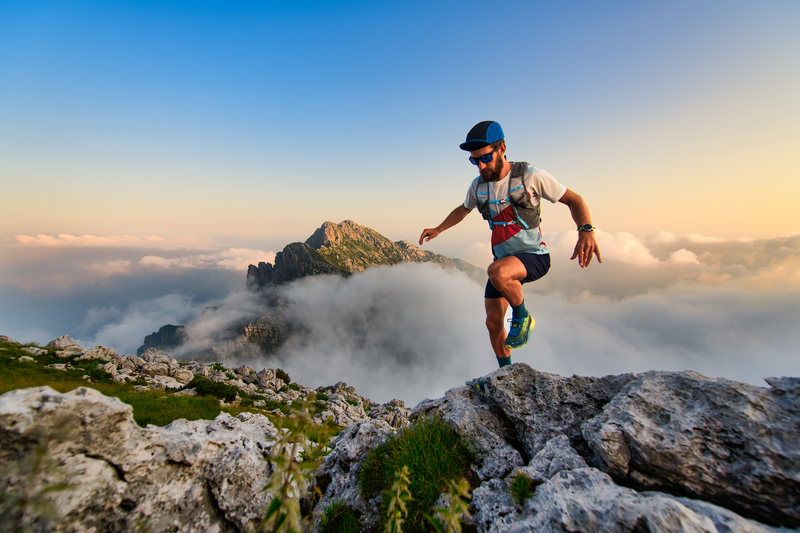
94% of researchers rate our articles as excellent or good
Learn more about the work of our research integrity team to safeguard the quality of each article we publish.
Find out more
DATA REPORT article
Front. Bioeng. Biotechnol. , 27 January 2021
Sec. Industrial Biotechnology
Volume 8 - 2020 | https://doi.org/10.3389/fbioe.2020.607176
This article is part of the Research Topic Bio-Based Compound Production and Their Innovative Industrial Applications View all 10 articles
Plant biomass is an important feedstock for the production of biofuel and other value-added chemicals in biorefinery industry (Rosales-Calderon and Arantes, 2019). In theory, a ton of cellulose could be converted to ~170 gallons of bioethanol, and thus the global cellulosic biomasses (including corn stover, rice straw and wood residues) can potentially be used to produce over 167 billion gallons of bioethanol annually (Murdock et al., 2019). Despite its great potential, the challenges of biomass utilization lie in the current uncompetitive production cost when compared to that of fossil fuel. Feedstock prices, raw material transportation, and production process, especially pretreatment, saccharification, and fermentation, are major factors of the high capital costs in the production of biofuels (Lynd et al., 2008; Mithra and Padmaja, 2017; Zoghlami and Paës, 2019). Unlike other bottlenecks, the pretreatment and saccharification processes can be biologically catalyzed by enzymes, especially from microorganisms, providing one of the most environmentally and economically sustainable solutions (Wang and Sun, 2010; Wang et al., 2012). Evidently, it has already been shown that genetic modification of a cellulase producing microbe, Trichoderma reesei, could provide a low-cost on-site enzyme production in Brazil (Zhuang et al., 2007), where the genetically engineered T. reesei could efficiently utilize local biomasses such as soybean hulls and molasse.
Cellulolytic enzymes from filamentous fungi such as Aspergillus (de Vries et al., 2000, 2017), Neurospora (Campos Antoniêto et al., 2017), Penicillium (Gusakov and Sinitsyn, 2012), and Trichoderma (Novy et al., 2019) have been screened for cellulose-, hemicellulose-, and other polysaccharide-degrading enzyme activities, and successfully applied in lignocellulose conversion. Among the cellulase-producing fungi, Aspergillus aculeatus is one of the most important models for optimization of cellulolytic enzymes due to its versatile metabolism and ability to grow on a wide range of substrates and under various conditions (Jayani et al., 2005; Poonsrisawat et al., 2014; Kunitake et al., 2015; Zhao et al., 2020). Different types of enzymes from A. aculeatus have already been used effectively by us and others, in the productions of animal feed (Saxena et al., 2019), paper (Jayani et al., 2005), cotton fabric (Abdulrachman et al., 2017), and bioethanol (Poonsrisawat et al., 2014). To explore new microorganisms for industrial applications, we have previously screened over 1,300 isolates from our institutional depository known as “Thailand Bioresource Research Center (TBRC, www.tbrcnetwork.org),” and the fungus A. aculeatus strain “BCC199” (also known as TBRC277 in our repository) came out as one of the most promising cellulolytic enzyme producers in our collection, based on its cellulolytic enzyme activity on saccharification of different types of biomass (Suwannarangsee et al., 2012, 2014). Random mutagenesis had already been applied for further industrial development (Champreda et al., 2019), leading to an increment of cellulolytic activity up to 2-folds. However, such strategies are time-consuming and not yet able to sufficiently ameliorate the enzyme activity to the level that is economically feasible for industrial production and utilization.
Here, we have sequenced and comprehensively analyzed the genomes and transcriptomes of A. aculeatus strain “BCC199” (TBRC277) and two additional strains representing different levels of cellulase activities. The three genomes were sequenced and assembled at higher coverages, as compared to the A. aculeatus genomes publicly available. Transcriptome of each strain were also constructed to facilitate transcript annotation. Both types of omic data sets not only provide the highest quality reference genomes and annotations of the cellulolytic enzyme-producing fungus A. aculeatus, which can be used to investigate distinct genomic features of the fungus that may play the role in cellulolytic activity.
The genomes of three A. aculeatus strains with different cellulase enzyme activities (see Table 1—Enzymatic activities, and Methods), including BCC56535 (the strain representing “low” cellulase activity), BCC199 (representing “moderate” activity), and HUT2365 (representing “high” activity), were completely sequenced in-house using the PacBio RS II platform (Pacific Biosciences, USA), giving the total circular consensus sequences of 345.3–463.3 Mb, equivalent to the genome coverages of 9.3X-12.1X (Table 1—Genome sequencing and analyses, see Supplementary Figure 1 for the analytical process). The final genome sizes after assembly were between 36.0 and 37.7 Mb, similar to the size of the most closely-related publicly available genome A. aculeatus ATCC16872 (36.01 Mb) (https://mycocosm.jgi.doe.gov/Aspac1/Aspac1.home.html), and with consistent GC contents of 49.4–50.8%.
To improve the annotation and functional analyses of the newly sequenced A. aculeatus genomes, we constructed multiple reference transcriptomes of each strain using RNA-seq, and obtained the averages of 47.69, 47.31, and 71.61 M clean reads from BCC56535, BCC199, and HUT2365, respectively (Table 1 - Transcriptome sequencing and Supplementary Table 3). De novo transcriptome assembly followed by integrating the gene models with those from genome annotation (see Methods and Supplementary Figure 1), yielded comparable genomic features among the three A. aculeatus genomes, including number of genes (11,000–12,000 genes), GC contents, as well as genomic and functional contents, when compared against publicly available databases such as NCBI's non-redundant database (Coordinators, 2014), enzyme commission (Webb, 1990), and Gene Ontology (Carbon et al., 2009) (Supplementary Table 2). Two of the newly sequenced genomes, BCC199 and HUT2365, are of higher quality than the closely-related reference genomes publicly available, A. aculeatus ATCC16872 and A. niger CBS513.88, in terms of the largest contig sizes and N50 (Supplementary Figure 2).
We identified 14,618 orthologs and singletons and the vast majority (~97% in each strain) could be assigned with at least one known function based on the Uniport Blast hit (Supplementary Figure 3). Among these predicted genes, 9,294 (64%) have orthologs in all three strains (Figure 1A). Between 417–429 predicted genes (3.5–4%) of each strain were classified into one of the carbohydrate-active enzyme families (CAZy; Cantarel et al., 2009) (Supplementary Table 2); whereas the total of 280 genes were assigned as Glycosyl Hydrolase (GH) genes and 195 (~70%) of these are common in our three strains (Figure 1B). Focusing on the cellulase-relating genes (comprising GH5, 6, 7, and AA9 families), the vast majority (18 out 22 genes with these GH or AA9 functions, ~82%) are commonly found in the three strains (Figure 1C). Importantly, none of these families is uniquely present or absent in any particular strain (Supplementary Table 4). However, we noted that one cellulose-degrading gene, endo-1,4-glucanase gene (Acu12065_1: GH5), was exclusively found in the A. aculeatus HUT2365 (Figure 1C) and potentially be one of the candidate genes contributing to the higher cellulase activity in A. aculeatus HUT2365. The Acu12065_1 gene contains a signal peptide (1–17 amino acids), GH5 protein domain (58–245 amino acids), and conserved catalytic domain (157–166 amino acids) with one active site, E164 (Supplementary Figure 4).
Figure 1. Gene annotation and prediction of biological function. Venn diagram showing the core orthologous and unique genes of the model A. aculeatus strains. (A) All predicted genes, (B) Glycoside Hydrolase (GH) families, and (C) Cellulose-degrading enzymes (GH5, 6, 7 and AA9 families). The genome of each strain possesses a unique putative gene: endo-1,4- glucanase gene (Acu12065_1) in HUT2365, 1,3-beta-glucosidase gene (Acu00225_3) in BCC56535, and AA9 domain-containing gene (Acu10321_1) in BCC199. (D,E) Phylogenetic relationships between studied A. aculeatus strains and other seven Aspergilli. Maximum likelihood phylogeny was constructed by using (D) the concatenation of housekeeping genes, translation elongation factor 1 alpha, ubiquitin-conjugating enzyme, and beta-tubulin, and (E) the concatenation of cellulose-degrading enzymes, GH5, 6, 7, and AA9. The percentages of reproducible trees in which the associated taxa clustered together in the bootstrap test (from 1,000 replicates) are shown next to the branches. (F) Sequence variations between the strains—e.g., multiple alignments, SNP positions in relation to functional genomic positions of glycoside hydrolase protein domain (in brown block), and active sites (shown by pink), predicted by HMMER 3.0 (Potter et al., 2018). (G) Example of a differentially expressed gene, a putative sugar transporter (Acu07785_1), which was uniquely over-expressed in all three replicates of HUT2365 transcriptomes.
We further investigated the phylogenetic relationships of the cellulose-degrading enzyme sequences (GH5, 6, 7, and AA9, Figure 1E), compared to those of selected housekeeping genes (Figure 1D). All three A. aculeatus strains and the publicly available ATCC16872 are closely related based on the housekeeping references, although BCC199 and HUT2365 appeared slightly closer to one another (Figure 1D). However, based on the cellulose-degrading protein sequences, our moderate-cellulase-activity BCC199 strain diverges the most among the three, and thus there is no clear evidence that the high cellulase activity seen in HUT2365 can be accounted for by the overall divergence of the protein sequences (Figure 1E). On the contrary, we observed several single nucleotide polymorphisms (SNPs) within the protein domains and some are in proximity to the active sites (Figure 1F, Supplementary Figures 5, 6), suggesting that small genomic variations such as point mutations in the cellulolytic genes between the strains might also play a role in their different cellulase activities.
In addition to the genomic data, our transcriptomics of the three A. aculeatus strains cultivated on plant biomass as the sole carbon source, can be used to investigate the expression of cellulolytic genes and their facilitating genes, that may contribute to the cellulose-degrading activities. Figure 1G provides an example of a highly expressed putative sugar transporter (Acu07785_1), which is unique to HUT2365. Indeed, we observed this and other Major Facilitator Superfamily (MFS) genes (Nogueira et al., 2020) being transcribed at higher levels in the high-cellulase-activity HUT2365 than in the other strains. As shown in Neurospora crassa (Wang et al., 2017) and T. reesei (Zhang et al., 2013), modifications of genes encoding glucose transporters greatly affected the cellulase production. These newly constructed transcriptomic data, together with the genomes and gene models described above, not only provide an important resource for genetic engineering of the fungal strains for industrial applications, but also serve as high-quality references for future characterization of the fungi and other related species and their genomic evolution.
The three A. aculeatus strains of interest: BCC199, BCC56535, and HUT2365, were cultivated on potato dextrose agar (PDA; Difco Laboratories, USA) at 25°C for 4 days, before spore collection. The spores were harvested by washing PDA plate with 10 ml of sterile water containing 1% Triton X-100 (Fischer Scientific, USA). In order to express cellulases (endoglucanases, exoglucanases, and β-D-glucosidases), the fungi have to be cultivated in a medium containing cellulose fiber, such as wheat bran and soybean. Therefore, fungal spores were then cultivated into 50 ml of wheat bran (3% w/v) and soybean (2% w/v) medium, to the final concentration of 107 per ml. The fungal strains were cultured for 72 h in an aerobic cultivation condition at 30°C with 150 rpm in Innova 44R (Eppendorf AG, Germany). To collect cells and crude enzymes in the broth, fungal cells were separated from the whole broth by centrifugation (12,000 × g). The resulting supernatant was used in enzymatic assay. The cells were kept at −80°C for further genomic DNA and RNA isolations.
Total cellulase activity was measured with No. 1 Whatman filter paper (FPase activity) (Miller et al., 1960). For the major cellulose polymer degradation, endoglucanase activity was measured with carboxymethylcellulose (CMCase activity) (Miller et al., 1960). Briefly, the reaction mixture contained 20 uL of crude supernatant, 30 uL of 100 mM (pH 5.5) sodium acetate buffer and 50 uL of substrate to the 0.5% (w/v) final concentration in the final volume of 1 ml. The reaction was incubated for 60 min (for FPase assay) and for 10 min (for CMCase assay). The reaction was stopped by adding 100 uL of DNS. The enzymatic activities were calculated based on a corresponding standard containing glucose. One unit (U) of enzyme activity was defined as the amount of enzyme needed to liberate 1 umol of reducing sugars per minute. All samples were analyzed in triplicates and the mean values were calculated and reported.
Genomic DNA (gDNA) samples of the three fungal strains grown in potato dextrose broth (PDB) were extracted using Wizard® genomic DNA purification kit (Promega, USA). The extracted gDNA was quality-checked using NanoDrop spectrophotometer (Thermo Scientific, USA) and agarose gel electrophoresis (Al Samarrai and Schmid, 2000). Purified gDNA was used to construct the DNA sequencing libraries, according to the PacBio RSII protocol (Pacific Bioscience, USA). Genome assembly was done using the SMRT Analysis Software version 2.3.0 (http://www.pacb.com/productsand-services/analytical-software/smrt-analysis/) with the following steps (Supplementary Figure 1): extracting subreads by bash5tools (https://github.com/PacificBiosciences/pbh5tools) with the default parameters [min read length = 500 bp, minimum polymerase read quality (QV) = 0.8]; correcting subreads by HGAP3 (Chin et al., 2013) with seed coverage = 30X; performing de novo assembly using HGAP3, with assembly target coverage = 40X. The assembled genomes were further polished by Quiver (https://github.com/PacificBiosciences/GenomicConsensus), using the default parameters (max divergence percentage = 30, minimum anchor size = 12). The contiguity of assembled sequences was evaluated by comparing the newly assembled genomes to those of publicly available A. niger CBS513.88 and A. aculeatus ATCC16872, using QUAST (Gurevich et al., 2013). The completeness of genome assembly and annotation was also assessed using the Benchmarking Universal Single-Copy Orthologs (BUSCO, version 4.1.2) pipeline (Simão et al., 2015), using the “Fungal set” (odb10) in the OrthoDB database (Zdobnov et al., 2017) as a reference. AUGUSTUS (Stanke et al., 2006), a Hidden Markov Model (HMM)-based gene prediction program, was employed to predict and locate the positions of genes (including start-stop codons, exons, and introns) in the three fungal genomes sequenced. A. terreus was used as the pre-trained model, as it was the most closely related reference genome available in the database.
In addition to genome sequencing, we also analyzed the transcriptomes of the three A. aculeatus strains to improve the gene annotation. Fungal mycelium, cultured for 72 h in an aerobic cultivation condition at 30°C, was used to extract RNA following the Tri Reagent® protocol (MRC, USA). On-column DNase digestion kit (Qiagen, Germany) was used to further remove DNA traces. Then, the RNA was subjected to quantity and quality assessments by Advances Analyzer™ automated CS system (Advanced Analytical Technologies Inc., USA).
RNA-seq library preparation and sequencing were performed by Novogene (Beijing, China). In short, ribosomal RNA was degraded from 10 μg of total RNA using the Ribominus™ Eukaryotic kit (Life Technologies, USA). The RNA was enriched using the Absolutely mRNA Purification kit (Agilent Technologies, USA) and further quantified by BioAnalyzer 2100 (Agilent Technologies, USA) before cDNA library construction. The cDNA libraries were constructed using the NEBNext Ultra RNA Library Prep Kit for Illumina (NEB, USA). Illumina paired-end adapters and barcode sequences were ligated onto the cDNA fragments. The quality and quantity of the libraries were then assessed, before being sequenced using the Illumina HiSeq2000 platform (Illumina, USA). Quality control (QC) of raw reads were done using FastQC (http://www.bioinformatics.babraham.ac.uk/projects/fastqc/) and the adapters were trimmed using FastP (Chen et al., 2018) (see also Supplementary Figure 1). Reads after QC were assembled using Trinity (version 2014-07-17) (Grabherr et al., 2011). The assembled RNA-seq reads were then used as inputs for gene prediction, which were done using BRAKER (Hoff et al., 2015) and MAKER (Cantarel et al., 2008).
EVidenceModeler (Haas et al., 2008) was used to combine the set of gene predictions from AUGUSTUS, BRAKER, and MAKER, where the gene models from the three programs were equally weighted. OrthoFinder (Emms and Kelly, 2019) was used to identify and cluster orthologous genes of the three fungal strains, and their putative functions were assessed using UniProt (The UniProt Consortium, 2019), InterProScan (Jones et al., 2014), and CAZy databases (Cantarel et al., 2009). We also performed additional manual reciprocal BLAST to find the best hits between the strains, to refine the orthologous genes, especially within the multi-copy gene clusters. We set stringent criteria for assigning a gene function to an orthologous group as follows: 1) when the genes from at least 2 out of 3 strains were assigned to the same function; 2) when none of the hits from the 3 strains agreed, we took the one with the highest BLAST score as the representative function of the orthologous group with (Supplementary Table 5).
For the predicted proteins, BLASTP (Wheeler et al., 2006) was used to align the protein sequences against the UniProtKB database Release 2019_08, using E-value cut off = 1e-6. Putative protein functions were also obtained using InterproScan (Jones et al., 2014), by matching amino acid sequences against Pfam (Finn et al., 2014), TIGRFAMs (Haft et al., 2012), SMART (Letunic et al., 2015), Prosite (Sigrist et al., 2012), and Gene3D (Lewis et al., 2018). CAZYmes Analysis Toolkit (CAT) (Cantarel et al., 2009) was employed to assess the enzymatic activities based on the similarity of amino acid sequences and or protein domain structures. Identification of enzyme active sites and protein domain was performed using HMMER 3.0 (Potter et al., 2018) (http://hmmer.org). To further assess the functional genes in biological pathways, KOALA (KEGG Orthology And Links Annotation, Kanehisa et al., 2016) was also used. Transcription levels were computed separately for each strain based on the newly assembled genomes and gene annotations. HISAT2 (Kim et al., 2019) and StringTie (Pertea et al., 2016) were used to estimate the transcript per million (TPM) values, as previously described (Cortijo et al., 2017).
To investigate the phylogenetic relationship of the Aspergillus strains based on their housekeeping (translation elongation factor 1 alpha, ubiquitin conjugating enzyme and beta-tubulin) and cellulolytic genes (AA9, GH5, 6, and 7), we constructed phylogenetic trees using the MEGA X software (Kumar et al., 2018). The evolutionary history of the genes was inferred using the maximum likelihood method with a bootstrapping test of 1,000 replicates.
The datasets presented in this study can be found in online repositories. The names of the repository/repositories and accession number(s) can be found at: https://www.ncbi.nlm.nih.gov/sra, PRJNA659152; https://www.ncbi.nlm.nih.gov/geo/, GSE157700.
LE, VCham, VChar, and DC conceived the overall study. AP, WP, ST, CS, and SS performed the experiments. WM and SC analyzed the data. VChar and DC wrote the manuscript with input from all authors. All authors read and approved the final manuscript.
This work was supported by the National Science and Technology Development Agency (NSTDA), National Center for Genetic Engineering and Biotechnology (BIOTEC), Thailand, under the Grant P-18-52106 to DC and VChar; and the Thailand Research Fund (TRF) Grant for New Researcher (MRG6080235) and Faculty of Science, Mahidol University to VChar.
The authors declare that the research was conducted in the absence of any commercial or financial relationships that could be construed as a potential conflict of interest.
The Supplementary Material for this article can be found online at: https://www.frontiersin.org/articles/10.3389/fbioe.2020.607176/full#supplementary-material
Abdulrachman, D., Thongkred, P., Kocharin, K., Nakpathom, M., Somboon, B., Narumol, N., et al. (2017). Heterologous expression of Aspergillus aculeatus endo-polygalacturonase in Pichia pastoris by high cell density fermentation and its application in textile scouring. BMC Biotechnol. 17:15. doi: 10.1186/s12896-017-0334-9
Al Samarrai, T., and Schmid, J. (2000). A simple method for extraction of fungal genomic DNA. Lett. Appl. Microbiol. 30, 53–56. doi: 10.1046/j.1472-765x.2000.00664.x
Campos Antoniêto, A. C., Ramos Pedersoli, W., dos Santos Castro, L., da Silva Santos, R., da Silva Cruz, A. H., Nogueira, K. M. V., et al. (2017). Deletion of pH regulator pac-3 affects cellulase and xylanase activity during sugarcane bagasse degradation by Neurospora crassa. PLoS ONE 12:e0169796. doi: 10.1371/journal.pone.0169796
Cantarel, B. L., Coutinho, P. M., Rancurel, C., Bernard, T., Lombard, V., and Henrissat, B. (2009). The Carbohydrate-Active EnZymes database (CAZy): an expert resource for glycogenomics. Nucleic Acids Res. 37(Suppl. 1), D233–D238. doi: 10.1093/nar/gkn663
Cantarel, B. L., Korf, I., Robb, S. M., Parra, G., Ross, E., Moore, B., et al. (2008). MAKER: an easy-to-use annotation pipeline designed for emerging model organism genomes. Genome Res. 18, 188–196. doi: 10.1101/gr.6743907
Carbon, S., Ireland, A., Mungall, C. J., Shu, S., Marshall, B., Lewis, S., et al. (2009). AmiGO: online access to ontology and annotation data. Bioinformatics 25, 288–289. doi: 10.1093/bioinformatics/btn615
Champreda, V., Suwannarangsri, S., Arnthong, J., Siamphan, C., Poonsrisawat, A., Ketsub, N., et al. (2019). Mutant Strain Aspergillus aculeatus for Producing Cellulase and Xylanase and Preparation Method Thereof. World Patent WO2018226171A3, filed May 30, 2018 and issued March 3, 2019. Bangkok. Available online at: https://patentimages.storage.googleapis.com/a0/ab/ba/010f019f291ba8/WO2018226171A3.pdf
Chen, S., Zhou, Y., Chen, Y., and Gu, J. (2018). fastp: an ultra-fast all-in-one FASTQ preprocessor. Bioinformatics 34, i884–i890. doi: 10.1093/bioinformatics/bty560
Chin, C.-S., Alexander, D. H., Marks, P., Klammer, A. A., Drake, J., Heiner, C., et al. (2013). Nonhybrid, finished microbial genome assemblies from long-read SMRT sequencing data. Nat. Methods 10:563. doi: 10.1038/nmeth.2474
Coordinators, N. R. (2014). Database resources of the national center for biotechnology information. Nucleic Acids Res. 42, D7–D17. doi: 10.1093/nar/gkt1146
Cortijo, S., Charoensawan, V., Brestovitsky, A., Buning, R., Ravarani, C., Rhodes, D., et al. (2017). Transcriptional regulation of the ambient temperature response by H2A.Z nucleosomes and HSF1 transcription factors in arabidopsis. Mol. Plant 10, 1258–1273. doi: 10.1016/j.molp.2017.08.014
de Vries, R. P., Kester, H. C., Poulsen, C. H., Benen, J. A., and Visser, J. (2000). Synergy between enzymes from Aspergillus involved in the degradation of plant cell wall polysaccharides. Carbohydr. Res. 327, 401–410. doi: 10.1016/S0008-6215(00)00066-5
de Vries, R. P., Riley, R., Wiebenga, A., Aguilar-Osorio, G., Amillis, S., Uchima, C. A., et al. (2017). Comparative genomics reveals high biological diversity and specific adaptations in the industrially and medically important fungal genus Aspergillus. BMC Genome Biol. 18:28. doi: 10.1186/s13059-017-1151-0
Emms, D. M., and Kelly, S. (2019). OrthoFinder: phylogenetic orthology inference for comparative genomics. Genome Biol. 20, 1–14. doi: 10.1186/s13059-019-1832-y
Finn, R. D., Bateman, A., Clements, J., Coggill, P., Eberhardt, R. Y., Eddy, S. R., et al. (2014). Pfam: the protein families database. Nucleic Acids Res. 42, D222–D230. doi: 10.1093/nar/gkt1223
Grabherr, M. G., Haas, B. J., Yassour, M., Levin, J. Z., Thompson, D. A., Amit, I., et al. (2011). Trinity: reconstructing a full-length transcriptome without a genome from RNA-Seq data. Nat. Biotechnol. 29:644. doi: 10.1038/nbt.1883
Gurevich, A., Saveliev, V., Vyahhi, N., and Tesler, G. (2013). QUAST: quality assessment tool for genome assemblies. Bioinformatics 29, 1072–1075. doi: 10.1093/bioinformatics/btt086
Gusakov, A. V., and Sinitsyn, A. P. (2012). Cellulases from Penicillium species for producing fuels from biomass. Biofuels 3, 463–477. doi: 10.4155/bfs.12.41
Haas, B. J., Salzberg, S. L., Zhu, W., Pertea, M., Allen, J. E., Orvis, J., et al. (2008). Automated eukaryotic gene structure annotation using EVidenceModeler and the Program to Assemble Spliced Alignments. Genome Biol. 9:R7. doi: 10.1186/gb-2008-9-1-r7
Haft, D. H., Selengut, J. D., Richter, R. A., Harkins, D., Basu, M. K., and Beck, E. (2012). TIGRFAMs and genome properties in 2013. Nucleic Acids Res. 41, D387–D395. doi: 10.1093/nar/gks1234
Hoff, K. J., Lange, S., Lomsadze, A., Borodovsky, M., and Stanke, M. (2015). BRAKER1: unsupervised RNA-Seq-based genome annotation with GeneMark-ET and AUGUSTUS. Bioinformatics 32, 767–769. doi: 10.1093/bioinformatics/btv661
Jayani, R. S., Saxena, S., and Gupta, R. (2005). Microbial pectinolytic enzymes: a review. Process Biochem. 40, 2931–2944. doi: 10.1016/j.procbio.2005.03.026
Jones, P., Binns, D., Chang, H.-Y., Fraser, M., Li, W., McAnulla, C., et al. (2014). InterProScan 5: genome-scale protein function classification. Bioinformatics 30, 1236–1240. doi: 10.1093/bioinformatics/btu031
Kanehisa, M., Sato, Y., and Morishima, K. (2016). BlastKOALA and GhostKOALA: KEGG tools for functional characterization of genome and metagenome sequences. J. Mol. Biol. 428, 726–731. doi: 10.1016/j.jmb.2015.11.006
Kim, D., Paggi, J. M., Park, C., Bennett, C., and Salzberg, S. L. (2019). Graph-based genome alignment and genotyping with HISAT2 and HISAT-genotype. Nat. Biotechnol. 37, 907–915. doi: 10.1038/s41587-019-0201-4
Kumar, S., Stecher, G., Li, M., Knyaz, C., and Tamura, K. (2018). MEGA X: molecular evolutionary genetics analysis across computing platforms. Mol. Biol. Evol. 35, 1547–1549. doi: 10.1093/molbev/msy096
Kunitake, E., Kawamura, A., Tani, S., Takenaka, S., Ogasawara, W., Sumitani, J.-,i., et al. (2015). Effects of clbR overexpression on enzyme production in Aspergillus aculeatus vary depending on the cellulosic biomass-degrading enzyme species. Biosci. Biotechnol. Biochem. 79, 488–495. doi: 10.1080/09168451.2014.982501
Letunic, I., Doerks, T., and Bork, P. (2015). SMART: recent updates, new developments and status in 2015. Nucleic Acids Res. 43, D257–D260. doi: 10.1093/nar/gku949
Lewis, T. E., Sillitoe, I., Dawson, N., Lam, S. D., Clarke, T., Lee, D., et al. (2018). Gene3D: extensive prediction of globular domains in proteins. Nucleic Acids Res. 46, D435–D439. doi: 10.1093/nar/gkx1069
Lynd, L. R., Laser, M. S., Bransby, D., Dale, B. E., Davison, B., Hamilton, R., et al. (2008). How biotech can transform biofuels. Nat. Biotechnol. 26, 169–172. doi: 10.1038/nbt0208-169
Miller, G. L., Blum, R., Glennon, W. E., and Burton, A. L. (1960). Measurement of carboxymethylcellulase activity. Anal. Biochem. 1, 127–132. doi: 10.1016/0003-2697(60)90004-X
Mithra, M., and Padmaja, G. (2017). Strategies for enzyme saving during saccharification of pretreated lignocellulo-starch biomass: effect of enzyme dosage and detoxification chemicals. Heliyon 3:e00384. doi: 10.1016/j.heliyon.2017.e00384
Murdock, H. E., Gibb, D., André, T., Appavou, F., Brown, A., Epp, B., et al. (2019). Renewables 2019 Global Status Report. Paris: REN21. Available online at: https://www.ren21.net/wp-content/uploads/2019/05/gsr_2019_full_report_en.pdf
Nogueira, K. M. V., Mendes, V., Carraro, C. B., Taveira, I. C., Oshiquiri, L. H., Gupta, V. K., et al. (2020). Sugar transporters from industrial fungi: key to improving second-generation ethanol production. Renew. Sustain. Energy Rev. 131:109991. doi: 10.1016/j.rser.2020.109991
Novy, V., Nielsen, F., Seiboth, B., and Nidetzky, B. (2019). The influence of feedstock characteristics on enzyme production in Trichoderma reesei: a review on productivity, gene regulation and secretion profiles. Biotechnol. Biofuels 12:238. doi: 10.1186/s13068-019-1571-z
Pertea, M., Kim, D., Pertea, G. M., Leek, J. T., and Salzberg, S. L. (2016). Transcript-level expression analysis of RNA-seq experiments with HISAT, StringTie and Ballgown. Nat. Protoc. 11, 1650–1667. doi: 10.1038/nprot.2016.095
Poonsrisawat, A., Wanlapatit, S., Paemanee, A., Eurwilaichitr, L., Piyachomkwan, K., and Champreda, V. (2014). Viscosity reduction of cassava for very high gravity ethanol fermentation using cell wall degrading enzymes from Aspergillus aculeatus. Process Biochem. 49, 1950–1957. doi: 10.1016/j.procbio.2014.07.016
Potter, S. C., Luciani, A., Eddy, S. R., Park, Y., Lopez, R., and Finn, R. D. (2018). HMMER web server: 2018 update. Nucleic Acids Res. 46, W200–W204. doi: 10.1093/nar/gky448
Rosales-Calderon, O., and Arantes, V. (2019). A review on commercial-scale high-value products that can be produced alongside cellulosic ethanol. Biotechnol. Biofuels 12:240. doi: 10.1186/s13068-019-1529-1
Saxena, A., Verma, M., Singh, B., Sangwan, P., Yadav, A. N., Dhaliwal, H. S., et al. (2019). Characteristics of an acidic phytase from Aspergillus aculeatus APF1 for dephytinization of biofortified wheat genotypes. Appl. Biochem. Biotechnol. 191, 679–694. doi: 10.1007/s12010-019-03205-9
Sigrist, C. J., De Castro, E., Cerutti, L., Cuche, B. A., Hulo, N., Bridge, A., et al. (2012). New and continuing developments at PROSITE. Nucleic Acids Res. 41, D344–D347. doi: 10.1093/nar/gks1067
Simão, F. A., Waterhouse, R. M., Ioannidis, P., Kriventseva, E. V., and Zdobnov, E. M. (2015). BUSCO: assessing genome assembly and annotation completeness with single-copy orthologs. Bioinformatics 31, 3210–3212.
Stanke, M., Keller, O., Gunduz, I., Hayes, A., Waack, S., and Morgenstern, B. (2006). AUGUSTUS: ab initio prediction of alternative transcripts. Nucleic Acids Res. 34(Suppl. 2), W435–W439. doi: 10.1093/nar/gkl200
Suwannarangsee, S., Arnthong, J., Eurwilaichitr, L., and Champreda, V. (2014). Production and characterization of multipolysaccharide degrading enzymes from Aspergillus aculeatus BCC199 for saccharification of agricultural residues. J. Microbiol. Biotechnol. 24, 1427–1437. doi: 10.4014/jmb.1406.06050
Suwannarangsee, S., Bunterngsook, B., Arnthong, J., Paemanee, A., Thamchaipenet, A., Eurwilaichitr, L., et al. (2012). Optimisation of synergistic biomass-degrading enzyme systems for efficient rice straw hydrolysis using an experimental mixture design. Bioresour. Technol 119, 252–261. doi: 10.1016/j.biortech.2012.05.098
The UniProt Consortium (2019). UniProt: a worldwide hub of protein knowledge. Nucleic Acids Res. 47, D506–D515. doi: 10.1093/nar/gky1049
Wang, B., Li, J., Gao, J., Cai, P., Han, X., and Tian, C. (2017). Identification and characterization of the glucose dual-affinity transport system in Neurospora crassa: pleiotropic roles in nutrient transport, signaling, and carbon catabolite repression. Biotechnol. Biofuels 10, 1–22. doi: 10.1186/s13068-017-0705-4
Wang, K., and Sun, R.-C. (2010). “Chapter 7.5 - biorefinery Straw for Bioethanol,” in Cereal Straw as a Resource for Sustainable Biomaterials and Biofuels (Amsterdam: Elsevier), 267–287. doi: 10.1016/B978-0-444-53234-3.00015-8
Wang, M., Li, Z., Fang, X., Wang, L., and Qu, Y. (2012). “Cellulolytic enzyme production and enzymatic hydrolysis for second-generation bioethanol production,” in Biotechnology in China III: Biofuels and Bioenergy, eds F. W. Bai, C. G. Liu, H. Huang, G. T. Tsao (Berlin; Heidelberg: Springer), 1–24. doi: 10.1007/978-3-642-28478-6
Webb, E. C. (1990). “Enzyme nomenclature,” in The Terminology of Biotechnology: A Multidisciplinary Problem, ed K. L. Loening (Berlin; Heidelberg: Springer), 51–60. doi: 10.1007/978-3-642-76011-2_6
Wheeler, D. L., Barrett, T., Benson, D. A., Bryant, S. H., Canese, K., Chetvernin, V., et al. (2006). Database resources of the national center for biotechnology information. Nucleic Acids Res. 35(Suppl. 1), D5–D12. doi: 10.1093/nar/gkj158
Zdobnov, E. M., Tegenfeldt, F., Kuznetsov, D., Waterhouse, R. M., Simao, F. A., Ioannidis, P., et al. (2017). OrthoDB v9. 1: cataloging evolutionary and functional annotations for animal, fungal, plant, archaeal, bacterial and viral orthologs. Nucleic Acids Res. 45, D744–D749. doi: 10.1093/nar/gkw1119
Zhang, W., Kou, Y., Xu, J., Cao, Y., Zhao, G., Shao, J., et al. (2013). Two major facilitator superfamily sugar transporters from Trichoderma reesei and their roles in induction of cellulase biosynthesis. J. Biol. Chem. 288, 32861–32872. doi: 10.1074/jbc.M113.505826
Zhao, L., Cheng, L., Deng, Y., Li, Z., Hong, Y., Li, C., et al. (2020). Study on rapid drying and spoilage prevention of potato pulp using solid-state fermentation with Aspergillus aculeatus. Bioresour. Technol. 296:122323. doi: 10.1016/j.biortech.2019.122323
Zhuang, J., Marchant, M. A., Nokes, S. E., and Strobel, H. J. (2007). Economic analysis of cellulase production methods for bio-ethanol. Appl. Eng. Agric. 23, 679–687. doi: 10.13031/2013.23659
Keywords: plant biomass degradation, cellulolytic enzyme activities, genome sequencing, transcriptome sequencing, de novo assembly
Citation: Mhuantong W, Charoensri S, Poonsrisawat A, Pootakham W, Tangphatsornruang S, Siamphan C, Suwannarangsee S, Eurwilaichitr L, Champreda V, Charoensawan V and Chantasingh D (2021) High Quality Aspergillus aculeatus Genomes and Transcriptomes: A Platform for Cellulase Activity Optimization Toward Industrial Applications. Front. Bioeng. Biotechnol. 8:607176. doi: 10.3389/fbioe.2020.607176
Received: 14 October 2020; Accepted: 31 December 2020;
Published: 27 January 2021.
Edited by:
Ligia R. Rodrigues, University of Minho, PortugalReviewed by:
Artur Ribeiro, University of Minho, PortugalCopyright © 2021 Mhuantong, Charoensri, Poonsrisawat, Pootakham, Tangphatsornruang, Siamphan, Suwannarangsee, Eurwilaichitr, Champreda, Charoensawan and Chantasingh. This is an open-access article distributed under the terms of the Creative Commons Attribution License (CC BY). The use, distribution or reproduction in other forums is permitted, provided the original author(s) and the copyright owner(s) are credited and that the original publication in this journal is cited, in accordance with accepted academic practice. No use, distribution or reproduction is permitted which does not comply with these terms.
*Correspondence: Varodom Charoensawan, dmFyb2RvbS5jaGFAbWFoaWRvbC5hYy50aA==; Duriya Chantasingh, ZHVyaXlhQGJpb3RlYy5vci50aA==
†These authors have contributed equally to this work
Disclaimer: All claims expressed in this article are solely those of the authors and do not necessarily represent those of their affiliated organizations, or those of the publisher, the editors and the reviewers. Any product that may be evaluated in this article or claim that may be made by its manufacturer is not guaranteed or endorsed by the publisher.
Research integrity at Frontiers
Learn more about the work of our research integrity team to safeguard the quality of each article we publish.