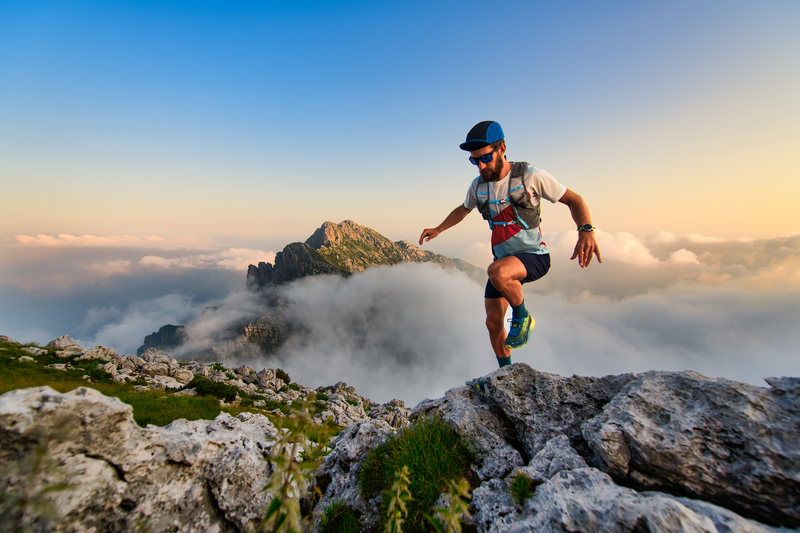
95% of researchers rate our articles as excellent or good
Learn more about the work of our research integrity team to safeguard the quality of each article we publish.
Find out more
ORIGINAL RESEARCH article
Front. Bioeng. Biotechnol. , 03 December 2020
Sec. Synthetic Biology
Volume 8 - 2020 | https://doi.org/10.3389/fbioe.2020.604041
This article is part of the Research Topic Facing the Upcoming of Multidrug-Resistant and Extensively Drug-Resistant Bacteria: Novel Antimicrobial Therapies (NATs) View all 11 articles
Antimicrobial resistance remains a great threat to global health. In response to the World Health Organizations’ global call for action, nature has been explored for novel and safe antimicrobial candidates. To date, fish have gained recognition as potential source of safe, broad spectrum and effective antimicrobial therapeutics. The use of computational methods to design antimicrobial candidates of industrial application has however, been lagging behind. To fill the gap and contribute to the current fish-derived antimicrobial peptide repertoire, this study used Support Vector Machines algorithm to fish out fish-antimicrobial peptide-motif candidates encrypted in 127 peptides submitted at the Antimicrobial Peptide Database (APD3), steered by their physico-chemical characteristics (i.e., positive net charge, hydrophobicity, stability, molecular weight and sequence length). The best two novel antimicrobial peptide-motifs (A15_B, A15_E) with the lowest instability index (−28.25, −22.49, respectively) and highest isoelectric point (pI) index (10.48 for each) were selected for further analysis. Their 3D structures were predicted using I-TASSER and PEP-FOLD servers while ProSA, PROCHECK, and ANOLEA were used to validate them. The models predicted by I-TASSER were found to be better than those predicted by PEP-FOLD upon validation. Two I-TASSER models with the lowest c-score of −0.10 and −0.30 for A15_B and A15_E peptide-motifs, respectively, were selected for docking against known bacterial-antimicrobial target-proteins retrieved from protein databank (PDB). Carbapenam-3-carboxylate synthase (PDB ID; 4oj8) yielded the lowest docking energy (−8.80 and −7.80 Kcal/mol) against motif A15_B and A15_E, respectively, using AutoDock VINA. Further, in addition to Carbapenam-3-carboxylate synthase, these peptides (A15_B and A15_E) were found to as well bind to membrane protein (PDB ID: 1by3) and Carbapenem synthetase (PDB: 1q15) when ClusPro and HPEPDOCK tools were used. The membrane protein yielded docking energy scores (DES): −290.094, −270.751; coefficient weight (CW): −763.6, 763.3 for A15_B and A15_E) whereas, Carbapenem synthetase (PDB: 1q15) had a DES of −236.802, −262.75 and a CW of −819.7, −829.7 for peptides A15_B and A15_E, respectively. Motif A15_B of amino acid positions 2–19 in Pleurocidin exhibited the strongest in silico antimicrobial potentials. This segment could be a good biological candidate of great application in pharmaceutical industries as an antimicrobial drug candidate.
Infections caused by drug resistant bacteria remain one of the leading causes of death worldwide (Martín-Rodríguez et al., 2016), as the potential of conventional antibiotics to combat such microbial infections fall (Tillotson and Zinner, 2017). Over 700,000 lives are lost to antimicrobial resistance annually and the number is projected to increase (O’Neill, 2014). The rate at which these microorganisms develop resistance has outpaced the rate of production of the current class of antibiotics in spite of the immense attempts by pharmaceutical industries for new antibiotics, thereby complicating the overall efforts (Huttner et al., 2013).
Several attempts like phage therapy (Moghadam et al., 2020), anti-biofilms agents (Pletzer and Hancock, 2016; Hamayeli et al., 2019), and the use of phytochemicals (Manuel et al., 2012) have been pipelined to prevent antimicrobial resistance. Antimicrobial peptides also known as host defensive proteins (HDPs) biologics are gradually gaining ground as far as countering multiple drug resistance is concerned (Fox, 2013). A case to note is Tyrothricin; the first peptide antibiotic to be clinically used in humans (Dubos, 1939). Since its discovery over six decades ago, no record of resistance has been reported against Tyrothricin (Atiye et al., 2014). Similarly, polymixin B and Colistin are among the only standing antibiotics for the treatment of multiple drug resistant bacteria including the notorious Acinetobacter baumanni, Pseudomonas aeruginosa, and Klebsiella pneumoniae as the last line antibiotics (Falagas and Kasiakou, 2005). Their ability to withstand resistance has been attributed to their non-specific mechanism of action, multiple target sites and presence of rare D-amino acids (Ageitos and Villa, 2016). They classically conform to the first mode of action by interfering with bacterial peptidoglycan cell wall biogenesis to ease cell membrane disruption (Sujeet et al., 2018; Hao et al., 2019) and as ligands for bacterial intracellular targets (Mahlapuu et al., 2016). Most antimicrobial peptides have generally recognized as safe (GRAS) status (Hancock and Scott, 2000), with little or no toxicity (Wang S. et al., 2016). These good attributes have led to an intensified search for novel peptide antibiotics from diverse forms of life.
Fish are capable of producing antimicrobial peptides of various classes including defensins, cathelicidins, hepcidins, histone-derived peptides, and piscidins (Masso-silva and Diamond, 2014; Kumar et al., 2018). These fish derived antimicrobial peptides are active against both fish and human pathogens (Hayek et al., 2013; Huan et al., 2020; Tiralongo et al., 2020). However, their low stability coupled with insufficient information about their structures has limited their pharmaceutical applicability (Okella et al., 2018), since information on protein structure and biological (motif) interaction are key for determining the stability of any active protein (Vaidya et al., 2018). Antimicrobial activity of peptides greatly relies on amino acid composition, structure and their physicochemical properties (Kêska and Stadnik, 2017). There are numerous experimentally validated fish-derived antimicrobial peptides. However, insights into the amino acid composition, peptide structure and the target interactions with motifs in these antimicrobial peptides are lacking and present a gap that needs to be understood. This gap can however be filled through the use of in silico approaches. In this study we report findings of motif design, target identification and target interactions with putative antimicrobial peptide motif derived from fish.
This was an in silico study setup involving fishing out novel antimicrobial peptide motifs encrypted in 127 fish antimicrobial peptides on Antimicrobial Peptide Databases. Potential antimicrobial peptide motifs were then selected based on their physicochemical characteristics like hydrophobicity, stability, and molecular weight/size as well as sequence length. The best two antimicrobial peptide candidate-motifs were designed for their putative antimicrobial leads and docked against the known antimicrobial protein-targets to predict their potential mode of action.
Out of the 127 existing antimicrobial peptide (AMP) sequences, a total of 24 naturally occurring peptides (<100 amino acid residues) of fish origin (Table 1), with well characterized antimicrobial activity were retrieved from Antimicrobial Peptide Database (APD3) using fish as the source organism at http://aps.unmc.edu/AP/tools.php (Retrieved on May 19th, 2019) (Wang G. et al., 2016).
To generate and identify potential antimicrobial peptide motifs, the retrieved sequences in FASTA file format were subjected to web-based Support Vector Machines (SVMs) algorithm based tool of Collection of Anti-Microbial Peptides (CAMPR3) server (May, 2019)1 (Waghu et al., 2016). The generated motifs were then screened based on several physiochemical parameters (Torrent et al., 2012a). The choice of the physiochemical parameters took into account that of the already existing polycationic and amphipathic AMPs; Amino acid length (18 residues), positive net charge (+4 to +6), hydrophobicity (40 and 60%) and isoelectric point of up to 10 (Wang S. et al., 2016; Hincapié et al., 2018). Helical wheels for the generated motif sequences were determined using HeliQuest server2 at 18 amino acid window and one turn size (Gautier et al., 2008), so as to come up with cationic and hydrophobic amino acids, hydrophobicity and hydrophobic moment among other characteristics of the potential motifs (Torrent et al., 2012b). Furthermore, the instability of the putative peptides was checked using an ExPASy tool; ProtParam3, where an instability index above zero implies it’s an unstable peptide.
Due to the shortness of the peptide sequences (<30 amino acids) coupled with the absence of their experimentally attained structure for templates, the three dimensional structure of putative peptide-motifs were predicted using the Iterative Threading Assembly Refinement (I-TASSER) server4 (Yang and Zhang, 2015). The peptides were modeled using protein templates identified by Local Meta-Threading Server (LOMETS) from the Protein Data Bank (PDB) library. LOMETS uses multiple threading approaches to align the query protein amino acid sequence against the PDB5. Template proteins with the highest sequence identity and lowest Z-score were used in the modeling exercise (Table 2). The best models were identified based on their c-scores. This score is calculated based on the significance of threading template alignments and the convergence parameters of the structure assembly simulations. It ranges from -5 to 2, where a lower score value indicates a highly confident model while the higher indicates the reverse. The peptide 3D structure prediction exercise was cross-validated using a web-based de novo peptide structure prediction tool, PEP-FOLD v3.56 (Thévenet et al., 2012). Briefly the query peptide amino acid sequences in FASTA format were used as the input file sequences. The algorithm was set to run 100 simulations and the output models were ranked based on sOPEP energies of individual model, where the lower the energy the better the model. The best models for both peptides A15_A and A15_B from the two peptide structure prediction tools (I-TASSER and PEP-FOLD v3.5) were then analyzed for their quality. Validation of these peptides structure was carried out in three phases; First by using Protein Structure Analysis (ProSA) web-server7 (Wiederstein and Sippl, 2007) which predicts the query protein z-score, local model quality, and residue energy. The Z-score indicates the model quality by comparing the query protein z-score against the z-score of experimentally validated proteins available in the protein data bank (PDB). In the second phase, PROCHECK was then used to measure the stereo-chemical properties of the modeled peptide-motifs (Laskowski et al., 1993), and finally, Atomic Non-Local Environment Assessment (ANOLEA) web server8 was used to calculate the energy of the query protein and evaluate their heavy atomic Non-Local Environment (NLE) in each molecule (Melo et al., 1997).
To identify the most probable target-proteins of the motifs, all the approved antibiotic targets in the DrugBank database (Law et al., 2014) at https://www.drugbank.ca/targets were fished using key words; target and antibiotics. The receptor proteins alongside their identities were later retrieved from Protein Data Bank (PDB) library.
The docking exercise was carried out on the top two potential AMP motifs against known protein drug targets. Docking was carried-out using the AutoDock VINA (Trott and Olson, 2019) on the DINC 2.0 Web server9 (Antunes et al., 2017). The docking was validated using two docking tools; Hierarchical flexible Peptide Docking (HPEPDOCK) and ClusPro (Kozakov et al., 2017; Zhou et al., 2018) for optimized protein-peptide interaction. HPEPDOCK predicts the protein-peptide interaction using the hierarchical algorithm between the protein and the peptide 3D structure while ClusPro performs a global docking procedure in four folds, motif-based prediction based on peptide conformation, rigid-body docking, scoring based on structural clustering; and final structure minimization. Briefly, the 3D structures of both the receptor protein (retrieved from PDB) and the modeled 3D peptide structures were the input files for both docking tools. Both ClusPro and HPEPDOCK docking were performed onto their respective web servers10 ,11.
A total of 127 fish derived peptide sequences were retrieved out of which, 24 peptide sequences were qualified (Table 1). The average peptide-amino acid length was 32 residues (ranging from 15–69 residues). 20% of the retrieved peptide-sequences belonged to the cathelcidin family with 45.8% not reported. The target organisms of the retrieved peptides ranged from bacteria to yeast and fungi.
A total of 361 peptide-motif sequences were designed from the qualified sequences which had suitable physico-chemical properties viz. mean hydrophobicity (Hm) greater than 0.3 (based on Fauchere and Pliska scale) (Fauchere and Pliska, 1983), net charge of + 4 and above, low instability index below zero, high antimicrobial probability were qualified. Seven peptide-motifs (Table 3), from which two peptide-motifs (A15_B and A15_E) with the highest stability (least instability index −28.25, −22.49, respectively) and highest antimicrobial probability (0.982) were selected for docking studies. Both peptides were found to be from the sequence of Pleurocidin; an AMP secreted by a winter flounder fish, P. americanus located between amino acids 2–19 and 5–22, respectively.
The I-TASSER modeling returned five models for each modeled peptide motif (A15_B and A15_E),while the PEP-FOLD prediction returned 10 models. The best I-TASSER models had a negative c-score. I-TASSER Model-1 for both peptides (A15_B and A15_E) had the best c-score of -0.10 and -0.03, respectively (Table 4). On the other hand PEP-FOLD model1 for both peptides (A15_B and A15_E) were recognized as the best model with the lowest sOPEP energy of −25.1325 and −25.4534 and Apollo predicted melting temperature (tm) score of 0.703 and 0.714, respectively. The Model1_A15_B and Model1_A15_E for both I-TASSER and PEP-FOLD were characterized as the best models from both tools, thus selected for model structure analysis.
Table 4. Top 5 output peptide structure prediction models from i-TASSER, PEP-FOLD, and their model evaluation.
The Ramachandran plot analysis indicates that I-TASSER Model1_A15_E had 13 residues in the most favorable region and 1 in the additional allowed region. None of the Model1_A15_E peptide-motif residues were in the disallowed region. Similarly, I-TASSER Model1_A15_B had 12 residues in the most favorable region, 1 in the additional allowed region with none in disallowed region (Figure 1; Laskowski et al., 1993). On the other hand, PEP-FOLD model1_A15_B had 13 residues in the favorable region while PEP-FOLD model1 A15_E had 14 residues in the favorable region. In addition, a cross-validation with ProSA, showed that I-TASSER models had a z-score of -1.5, -1.27 against A15_B and A15_E, while a z-score of -1.44, -1.5 were observed for PEP-FOLD A_15_B and A_15_E models, respectively. All model z-scores were in the same range with the z-score of experimentally validated proteins, thus considered to be accurate. Likewise, ANOLEA showed that majority of I-TASSER models (33.3 and 44.5% for model A15_B and A15_E, respectively) had amino acid residues of the peptide chain in a favorable energy environment (with low energy-scores) (Figure 2) while PEP-FOLD model A15_B and A15_E had 22.2 and 55.7% of amino acid residues with low energy. I-TASSER model1 for both A15_B and A15_E show to be the best peptide structures and they were selected for docking exercise.
Figure 1. I-TASSER predicted peptide 3D structure homology models and their Ramachandran validation plots. (A) A15_B peptide-motif, (B) Ramachandran plot for A15_B peptide-motif, (C) A15_E peptide-motif, (D) Ramachandran plot for A15_E peptide-motif. Peptide-motif A15_B had 12 amino acids sequences in the allowed region while peptide-motif A15_E had 13 amino acid in the favorable region. Both peptide-motifs had no amino acid sequence in the disallowed region. The cartons were rendered in Edu PyMOL.
Figure 2. I-TASSER predicted peptide 3D structure ANOLEA and ProSA validation plots. (A) Peptide A15_B ANOLEA energy score, (B) Peptide A15_B ProSA z-score, (C) Peptide A15_E ANOLEA energy score, (D) Peptide A15_B ProSA z-score. ANOLEA validation showed that 33.3 and 44.5% of peptide A15_B and A15_E had their amino acid residues in the favorable regions (low energy scores highlighted in red). Peptide motifs A15_B and A15_E had z-scores of −1.5 and −1.27, respectively, and were within the normal z-score of experimentally validated proteins. The ANOLEA plots were generated in R using latticeExtra package.
A total of 28 targets were fished from the DrugBank database, out of which 18 had experimentally determined structures deposited at PDB (Table 5). Majority of the structures (83.3%) were determined using X-ray diffraction with only one structure (C-1027) determined using solution Nuclear Magnetic Resonance (NMR).
Docking exercise with AutoDock VINA revealed that both peptide-motifs (A15_B and A15_E) were able to bind with low docking energies (ranging from -8.80 to -5.80 Kcal/mol) indicating their fairly high affinity with the selected antimicrobial target protein (Table 6). The best docking energy, however, was observed against vancosaminyl transferase protein (PDB ID; 1rrv, docking energy (DE); −8.20, −7.60 Kcal/mol), Beta-hexosaminidase protein (PDB ID; 4g46 DE; −7.90, −7.70 Kcal/mol), membrane protein (PDB: 1by3 DE; −7.3, −7.3), and carbapenam protein (PDB ID; 4oj8 DE; −8.80, −7.80 Kcal/mol) against peptide-motif A15_B and A15_E, respectively (Table 6). The affinity of peptide-motifs A15_B and A15_E was highest within chains of the target proteins (PDB ID 1rrv, 4g6c, and 4oj8). Docking validation with HPEPDOCK shows that membrane protein (PDB: 1by3) and Carbapenem synthetase had the highest docking potential to peptide A15_B and A15_E with a docking energy score of −290.094, −270.751 against protein 1by3 and −236.802, −262.75 against 1q15, respectively. Likewise, docking with ClusPro further indicated that membrane protein and carbapenem synthetase had the highest chance to bind to peptide A15_B and A15_E with a coefficient weight of −763.6, −763.3 against protein 1by3 and −819.7, −829.7 against peptide A15_B and A15_E, respectively. Carbapenam synthetase (PDB ID; 4oj8) which had the lowest docking energy against the two peptides was found to be among the targets with lowest docking energies scores of (-221.657 and −196.952) against peptide A15_B and A15_E using HPEPDOCK. However, this protein had the lowest coefficient weight score of −681.2 and −66.8 against peptide A15_B and A15_E using ClusPro, respectively. Peptide motif A15_B which had the lowest instability index (highest stability) also showed a relatively higher binding affinity than its counterpart A15_E (Table 6) in all the 3 docking methods, except with protein 1by3 where peptide A15_E had a lower docking energy score than A15_B using HPEPDOCK.
Table 6. Docking energies and score of ligand A15_B, A15_E against the Antimicrobial target proteins using Autodock Vina, HPEPDOCK, ClusPro.
The present study demonstrates that an online Support Vector Machines (SVMs) algorithm effectively localizes motifs of potentially best antimicrobial activity within a peptide. This technique is vital in enhancing the antimicrobial activity of peptides especially on resistant strains including Pseudomonas aeruginosa (Torrent et al., 2012c). The strength of this study is hinged on its ability to generate very many peptide fragments and being able to systematically sieve them based on their physicochemical parameters to arrive at the best candidates. However, the number of peptide templates used was small 24 (0.77%) compared to a total of 3,105 antimicrobial peptides in the antimicrobial peptides database (accessed on 01.08.2019). This is due to the fact that this study focuses only on “experimentally validated” peptides even so, only 127 fish antimicrobial peptides are present at the database.
Out of the 361 peptide motifs generated, the most active with the highest in silico antimicrobial probability of 0.982 (A15_B and A15_E) were both from Pleurocidin; an AMP secreted by flatfish, Pleuronectes americanus that largely inhabits soft muddy to moderately hard bottoms of marine waters. Even so, motif A15_B proved to be much more stable (instability index −28.25), rendering it the best fragment designed. When docked with AutoDock VINA, A15_B continued as the best designed peptide motif yielding the highest binding energy (−8.80 Kcal/mol) and highest number of hydrogen bond interactions (3) on Carbapenam-3-carboxylate synthase target. This indicates the motif (A15_B) binds spontaneously onto Carbapenam-3-carboxylate synthase target without consuming energy (Meng et al., 2011). Moreover, docking with HPEPDOCK and ClusPro further indicated that Carbapenam synthetase protein (PDB: 1Q15) alongside a Membrane proteins (PDB: 1by3) and Carbapenam-3-caboxylate protein (PDB: 4oj8) are among the proteins with highest binding potentials to peptide motif A15_B. However, Carbapenam-3-caboxylate protein yielded the least Docking energy when compared to the Membrane proteins and carbapenam synthetase and Carbapenam synthetase protein. Carbapenam-3-carboxylate synthase is responsible for the biosynthesis of the naturally occurring β-lactam antibiotics in bacteria (Stapon et al., 2003). The enzyme catalyzes the ATP-dependent formation of (3S,5S)-carbapenam-3-carboxylate from (2S,5S)-5-carboxymethylproline in Pectobacterium carotovorum (Gerratana et al., 2003). Therefore, the binding of the designed peptide motif A15_B is likely to activate Carbapenam-3-carboxylate synthase to synthesis amass of natural antibiotic that destroys the bacteria (Samantha et al., 2007), a phenomenon that can be explored for novel therapeutics. However, being a novel motif on amino acids of positions 2–19 of Pleurocidin, this study could hardly access preceding studies to match the complex binding affinity.
An important but unanswered question is how these peptides can be optimized for a good platform particularly in drug discovery where the nature and properties of potential hits can be understood specifically on how best they can be modified into useful leads as antimicrobials in the fight against drug resistance. Ultimately, efforts are underway for better ways to handle such small fragments on benches to ascertain the in vitro and in vivo efficacy in low resource facilities.
This study revealed that the motifs (A15_B) of amino acid positions 2-19 in Pleurocidin secreted by a winter flounder fish, Pleuronectes americanus as the best antimicrobial potentials. This segment is among the promising biological candidates that could be of great application in pharmaceutical and nutraceutical industries as virtual tools show great potentials in drug development even in the absence of large investment laboratory equipment. However, further studies focused on synthesized peptides would be helpful.
The original contributions presented in the study are included in the article/supplementary material, further inquiries can be directed to the corresponding author/s.
HO, SO, and CN designed and implemented the study. JA, CA, HI, FF, JN, CK, CB, PO, HO, AM, JG, and KK performed the experiments and data analysis. All authors participated in writing and proofreading the manuscript and approved the final manuscript for publication.
This work was supported by the Pharm-Biotechnology and Traditional Medicine Center (PHARMBIOTRAC) under Grant number PH/2019/SG/03.
The authors declare that the research was conducted in the absence of any commercial or financial relationships that could be construed as a potential conflict of interest.
Ageitos, J. M., and Villa, T. G. (2016). Antimicrobial peptides (AMPs): ancient compounds that represent novel weapons in the fight against bacteria. Biochem. Pharmacol. 133, 117–138. doi: 10.1016/j.bcp.2016.09.018
Antunes, D. A., Moll, M., Devaurs, D., Jackson, K. R., Lizée, G., and Kavraki, L. E. (2017). DINC 2.0: a new protein-peptide docking webserver using an incremental approach. Cancer Res. 77, e55–e57. doi: 10.1158/0008-5472.CAN-17-0511
Atiye, S., Le, T., and Kretschmar, M. (2014). Decade-long use of the antimicrobial peptide combination tyrothricin does not pose a major risk of acquired resistance with gram-positive bacteria and Candida spp. Pharmazie 69, 2–5. doi: 10.1691/ph.2014.4686
Dubos, J. R. (1939). Studies on a bactericidal agent extracted from a soil Bacillus: I. preparation of the agent. its activity in vitro. J. Exp. Med. 70, 1–10.
Falagas, M. E., and Kasiakou, S. K. (2005). Colistin: the revival of polymyxins for the management of multidrug-resistant gram-negative bacterial infections. Infect. Dis. 40, 1333–1342.
Fauchere, J.-L., and Pliska, V. (1983). Hydrophobic parameters II of amino acid side-chains from the partitioning of N-acetyl-amino acid amides. Eur. J. Med. Chem. 18, 369–375.
Fox, J. L. (2013). Antimicrobial peptides stage a comeback. Nat. Biotechnol. 31, 379–382. doi: 10.1038/nbt.2572
Gautier, R., Douguet, D., Antonny, B., and Drin, G. (2008). HELIQUEST: a web server to screen sequences with specific α-helical properties. Bioinformatics 24, 2101–2102. doi: 10.1093/bioinformatics/btn392
Gerratana, B., Stapon, A., and Townsend, C. A. (2003). Inhibition and alternate substrate studies on the mechanism of carbapenam synthetase from Erwinia carotovora. Biochemistry 42, 7836–7847. doi: 10.1021/bi034361d
Hamayeli, H., Hassanshahian, M., and Askari Hesni, M. (2019). The antibacterial and antibiofilm activity of sea anemone (Stichodactyla haddoni) against antibiotic-resistant bacteria and characterization of bioactive metabolites. Int. Aquat. Res. 11, 85–97. doi: 10.1007/s40071-019-0221-1
Hancock, R. E., and Scott, M. G. (2000). The role of antimicrobial peptides in animal defenses. Proc. Natl. Acad. Sci. U.S.A. 97, 8856–8861. doi: 10.1073/pnas.97.16.8856
Hao, A., Guan, Z., and Lee, S. (2019). Visualizing conformation transitions of the Lipid II fl ippase MurJ. Nat. Commun. 10:1736. doi: 10.1038/s41467-019-09658-0
Hayek, S. A., Gyawali, R., and Ibrahim, S. A. (2013). “Antimicrobial natural products,” in Microbial Pathogens and Strategies for Combating Them: Science, Technology and Education, ed. A. Méndez-Vilas (Greensboro: North Carolina Agricultural and Technical State University), 910–921.
Hincapié, O., Giraldo, P., and Orduz, S. (2018). In silico design of polycationic antimicrobial peptides active against Pseudomonas aeruginosa and Staphylococcus aureus. Antonie Van Leeuwenhoek Int. J. Gen. Mol. Microbiol. 111, 1871–1882. doi: 10.1007/s10482-018-1080-2
Huan, Y., Kong, Q., Mou, H., and Yi, H. (2020). Antimicrobial peptides: classification, design, application and research progress in multiple fields. Front. Microbiol. 11:582779. doi: 10.3389/fmicb.2020.582779
Huttner, A., Harbarth, S., Carlet, J., Cosgrove, S., Goossens, H., and Holmes, A. (2013). Antimicrobial resistance: a global view from the 2013 World healthcare-associated infections forum. Antimicrob. Resist. Infect. Control 2, 1–13.
Kêska, P., and Stadnik, J. (2017). Antimicrobial peptides of meat origin-an in silico and in vitro analysis. Protein Pept. Lett. 24, 165–173. doi: 10.2174/092986652366616122
Kozakov, D., Hall, D. R., Xia, B., Porter, K. A., Padhorny, D., Yueh, C., et al. (2017). The ClusPro web server for protein-protein docking. Nat. Protoc. 12, 255–278. doi: 10.1038/nprot.2016.169.The
Kumar, P., Kizhakkedathu, J. N., and Straus, S. K. (2018). Antimicrobial peptides: diversity, mechanism of action and strategies to improve the activity and biocompatibility in vivo. Biomolecules 8:4. doi: 10.3390/biom8010004
Laskowski, R. A., MacArthur, M., Thornton, J., and Moss, D. (1993). PROCHECK: a program to check the stereochemical quality of protein structures. J. Appl. Crystallogr. 26, 283–291. doi: 10.1107/S0021889892009944
Law, V., Knox, C., Djoumbou, Y., Jewison, T., Guo, A. C., Liu, Y., et al. (2014). DrugBank 4.0: shedding new light on drug metabolism. Nucleic Acids Res. 42, 1091–1097. doi: 10.1093/nar/gkt1068
Mahlapuu, M., Håkansson, J., Ringstad, L., and Björn, C. (2016). Antimicrobial peptides: an emerging category of therapeutic agents. Front. Cell Infect. Microbiol. 6:194. doi: 10.3389/fcimb.2016.00194
Manuel, S., Madalena, L., and Lúcia, C. S. (2012). ““Phytochemicals against drug-resistant microbes,” in Dietary Phytochemicals and Microbes, ed. A. K. Patra (Porto: Springer Science), 185–205. doi: 10.1007/978-94-007-3926-0
Martín-Rodríguez, A. J., Quezada, H., Becerril, G., Fuente-nuñez, C., and De Castillo-juarez, I. (2016). Recent advances in novel antibacterial development. Front. Clin. Drug Res. Anti Infect. 2, 3–61. doi: 10.2174/9781681081533116020003
Masso-silva, J. A., and Diamond, G. (2014). Antimicrobial peptides from fish. Pharmaceuticals 7, 265–310. doi: 10.3390/ph7030265
Melo, F., Devos, D., Depiereux, E., and Feytmans, E. (1997). ANOLEA: a www server to assess protein structures. Proc. Int. Conf. Intell. Syst. Mol. Biol. 5, 187–190.
Meng, M.-Y., Hong-Xing, Z., Mihaly, M., and Meng, C. (2011). Molecular docking: a powerful approach for structure-based drug discovery. Curr. Comput. Aided Drug Discov. 7, 146–157. doi: 10.1038/jid.2014.371
Moghadam, M. T., Amirmozafari, N., Shariati, A., Hallajzadeh, M., Mirkalantari, S., Khoshbayan, A., et al. (2020). How phages overcome the challenges of drug resistant bacteria in clinical infections. Infect. Drug Resist. 13, 45–61. doi: 10.2147/IDR.S234353
Okella, H., Aber, J., Kevin, T. K., Kato, C. D., and Ogwang, P. E. (2018). Fish mucus: a neglected reservoir for antimicrobial peptides. Asian J. Pharm. Res. Dev. 6, 6–11.
O’Neill, J. (2014). Antimicrobial Resistance: Tackling a Crisis for the Health and Wealth of Nations. London: Wellcome Trust.
Pletzer, D., and Hancock, R. E. W. (2016). Antibiofilm peptides: potential as Broadspectrum agents. J. Bacteriol. 198, 2572–2578. doi: 10.1128/JB.00017-16
Samantha, O., Arnett, B. G., and Craig, A. T. (2007). Rate-Limiting steps and role of active site lys443 in the mechanism of carbapenam synthetase. Biochemistry 46, 9337–9347.
Stapon, A., Li, R., and Townsend, C. A. (2003). Synthesis of (3S,5R)-Carbapenam-3-carboxylic Acid and its role in carbapenem biosynthesis and the stereoinversion problem. J. Am. Chem. Soc. 125, 15746–15747. doi: 10.1021/ja037665w
Sujeet, K., Frederick, A. R., Alicia, G. M., and Natividad, R. (2018). The bacterial lipid II flippase MurJ functions by an alternating-access mechanism. J. Biol. Chem. 294, 981–990. doi: 10.1074/jbc.RA118.006099
Thévenet, P., Shen, Y., Maupetit, J., Guyon, F., Derreumaux, P., and Tufféry, P. (2012). PEP-FOLD: an updated de novo structure prediction server for both linear and disulfide bonded cyclic peptides. Nucleic Acids Res. 40, 288–293. doi: 10.1093/nar/gks419
Tillotson, G. S., and Zinner, S. H. (2017). Burden of antimicrobial resistance in an era of decreasing susceptibility. Expert Rev. Anti. Infect. Ther. 15, 663–676. doi: 10.1080/14787210.2017.1337508
Tiralongo, F., Messina, G., Lombardo, B. M., Longhitano, L., Volti, G. L., Tibullo, D., et al. (2020). Skin mucus of marine fish as a source for the development of antimicrobial agents. Front. Mar. Sci. 7:541853. doi: 10.3389/fmars.2020.541853
Torrent, M., Nogués, M. V., and Boix, E. (2012a). Discovering new in silico tools for antimicrobial peptide prediction. Curr. Drug Targets 13, 1148–1157. doi: 10.2174/138945012802002311
Torrent, M., Tommaso, P., Di Pulido, D., Nogués, M. V., Notredame, C., Boix, E., et al. (2012b). AMPA: an automated web server for prediction of protein antimicrobial regions. Bioinformatics 28, 130–131. doi: 10.1093/bioinformatics/btr604
Torrent, M., Victoria Nogues, M., and Boix, E. (2012c). Discovering new in silico tools for antimicrobial peptide prediction. Curr. Drug Targets 13, 1148–1157.
Trott, O., and Olson, A. J. (2019). Autodock vina: improving the speed and accuracy of docking. J. Comput. Chem. 31, 455–461. doi: 10.1002/jcc.21334.AutoDock
Vaidya, A., Nair Varun, S., Georrge, John, J., and Singh, S. P. (2018). Comparative analysis of thermophilic proteases. J. Life Sci. Bioinform. Pharm. Chem. Sci. 4, 65–91. doi: 10.26479/2018.0406.06
Waghu, F. H., Barai, R. S., Gurung, P., and Idicula-Thomas, S. (2016). CAMPR3: a database on sequences, structures and signatures of antimicrobial peptides. Nucleic Acids Res. 44, D1094–D1097. doi: 10.1093/nar/gkv1051
Wang, G., Li, X., and Wang, Z. (2016). APD3: the antimicrobial peptide database as a tool for research and education. Nucleic Acids Res. 44, 1087–1093. doi: 10.1093/nar/gkv1278
Wang, S., Zeng, X., Yang, Z., and Qiao, S. (2016). Antimicrobial peptides as potential alternatives to antibiotics in food animal industry. Int. J. Mol. Sci. 17, 1–12. doi: 10.3390/ijms17050603
Wiederstein, M., and Sippl, M. J. (2007). ProSA-web: interactive web service for the recognition of errors in three-dimensional structures of proteins. Nucleic Acids Res. 35, 407–410. doi: 10.1093/nar/gkm290
Yang, J., and Zhang, Y. (2015). I-TASSER server: new development for protein structure and function predictions. Nucleic Acids Res. 43, 174–181. doi: 10.1093/nar/gkv342
Keywords: antimicrobial, fish, peptides, putative, motifs
Citation: Okella H, Georrge JJ, Ochwo S, Ndekezi C, Koffi KT, Aber J, Ajayi CO, Fofana FG, Ikiriza H, Mtewa AG, Nkamwesiga J, Bassogog CBB, Kato CD and Ogwang PE (2020) New Putative Antimicrobial Candidates: In silico Design of Fish-Derived Antibacterial Peptide-Motifs. Front. Bioeng. Biotechnol. 8:604041. doi: 10.3389/fbioe.2020.604041
Received: 08 September 2020; Accepted: 09 November 2020;
Published: 03 December 2020.
Edited by:
Angel León-Buitimea, Autonomous University of Nuevo León, MexicoReviewed by:
Sheikh Arslan Sehgal, University of Okara, PakistanCopyright © 2020 Okella, Georrge, Ochwo, Ndekezi, Koffi, Aber, Ajayi, Fofana, Ikiriza, Mtewa, Nkamwesiga, Bassogog, Kato and Ogwang. This is an open-access article distributed under the terms of the Creative Commons Attribution License (CC BY). The use, distribution or reproduction in other forums is permitted, provided the original author(s) and the copyright owner(s) are credited and that the original publication in this journal is cited, in accordance with accepted academic practice. No use, distribution or reproduction is permitted which does not comply with these terms.
*Correspondence: Hedmon Okella, aG9rZWxsYUBzdGQubXVzdC5hYy51Zw==; John J. Georrge, am9obmpnZW9ycmdlQGdtYWlsLmNvbQ==
Disclaimer: All claims expressed in this article are solely those of the authors and do not necessarily represent those of their affiliated organizations, or those of the publisher, the editors and the reviewers. Any product that may be evaluated in this article or claim that may be made by its manufacturer is not guaranteed or endorsed by the publisher.
Research integrity at Frontiers
Learn more about the work of our research integrity team to safeguard the quality of each article we publish.