- 1Movement Control Rehabilitation Laboratory, Stevens Institute of Technology, Hoboken, NJ, United States
- 2Department of Biomedical Engineering, Stevens Institute of Technology, Hoboken, NJ, United States
This study investigated how modifications in the display of a computer trace under user control of grasp forces can co-modulate agency (perception of control) and performance of grasp on rigid and compliant surfaces. We observed positive correlation (p < 0.01) between implicit agency, measured from time-interval estimation for intentional binding, and grasp performance, measured by force-tracking error, across varying control modes for each surface type. The implications of this work are design directives for cognition-centered device interfaces for rehabilitation of grasp after neurotraumas such as spinal cord and brain injuries while considering if grasp interaction is rigid or compliant. These device interfaces should increase user integration to virtual reality training and powered assistive devices such as exoskeletons and prostheses. The modifications in control modes for this study included changes in force magnitude, addition of mild noise, and a measure of automation. Significant differences (p < 0.001) were observed for each surface type across control modes with metrics for implicit agency, performance, and grasp control efficiency. Explicit agency, measured from user survey responses, did not exhibit significant variations in this study, suggesting implicit measures of agency are needed for identifying co-modulation with grasp performance. Grasp on the compliant surface resulted in greater dependence of performance on agency and increases in agency and performance with the addition of mild noise. Noise in conjunction with perceived freedom at a flexible surface may have amplified visual feedback responses. Introducing automation in control decreased agency and performance for both surfaces, suggesting the value in continuous user control of grasp. In conclusion, agency and performance of grasp can be co-modulated across varying modes of control, especially for compliant grasp actions. Future studies should consider reliable measures of implicit agency, including physiological recordings, to automatically adapt rehabilitation interfaces for better cognitive engagement and to accelerate functional outcomes.
Introduction
The healthy hand is capable of exquisite grasp force control in manipulating objects during activities of daily living (Hubbard et al., 2009). Following neuromuscular traumas, such as spinal cord or brain injury, it is critical to rehabilitate grasp function for maintaining quality of life. Rehabilitation often involves physical therapy with repetitive task practice to reformulate neuromotor connections (Shepherd, 2001). Advanced physical therapy may employ engaging platforms such as virtual reality (VR) (Sveistrup, 2004) or robotics (Saleh et al., 2017). Powered assistive devices may also be employed to restore function as with powered exoskeletons (Lucas et al., 2004; Heo et al., 2012; Nataraj and van den Bogert, 2017) or neuroprostheses that activate sensorimotor pathways (Marasco et al., 2018; Schofield et al., 2019) of the hand. The primary objective with assistive or rehabilitative technologies is to enhance control of the hand and increase functional ability to perform manual tasks. Improved motor control may be enacted from training the person to move better independently or with the assistance of a powered device. Regardless of the rehabilitation approach, the person should be cognitively engaged and integrated with the therapeutic platform or the assistive device (Moore and Fletcher, 2012; Nataraj, 2017; Nataraj et al., 2020a,b,c). Improved perception of involvement and control of movement should better ensure continued participation and positive functional outcomes (Doyle, 2002; Behrman et al., 2005).
Despite intuitive relation between cognitive integration to movement and greater functional performance, this concept has not been systematically investigated nor incorporated in standard rehabilitation protocols. True innovation in neuromotor rehabilitation would include methods that optimize user-device movement abilities while increasing user cognition of movement. Systematically identifying agency, perception of control, and adapting device control accordingly may produce more effective, cognition-driven rehabilitation. Methods that leverage cognitive factors, such as agency, may accelerate functional gains and increase clinical retention of such methods and devices, which depends on user perception of utility (Childress, 1973; Phillips and Zhao, 1993; Hughes et al., 2014).
Sense of agency, or the perception of control, has been studied in experimental constructs that relate actions to consequences (Moore and Haggard, 2008; Moore, 2016). These studies have investigated modulation of agency with external cues (Moore et al., 2009; Khalighinejad et al., 2017) and the existence of agency within human machine interfaces (Evans et al., 2015; Le Goff et al., 2018). Agency is naturally implicated with rehabilitation through perception of neuromuscular action and related functional consequences (Moore and Obhi, 2012). Agency contributes to the performance of functional movements such as reaching (Nataraj et al., 2020c,d) and is impaired in the presence of neurological disorders (Jeannerod, 2009; Ritterband-Rosenbaum et al., 2012). Agency can also be compromised during the use of powered assistive devices, such as exoskeletons (Hartigan et al., 2015) or sensorimotor prostheses (Antfolk et al., 2013; Hebert et al., 2013), due to distortions in embodiment (Kilteni et al., 2012; Caspar et al., 2015). It remains unclear how agency is related to functional performance of grasping, and how agency and performance may be modulated with varying levels of control. Establishing the connection between agency and grasp could inspire the development of rehabilitation platforms that leverage agency for more effective control of grasp. These platforms would utilize agency to maximize classical performance objectives such as minimal effort or better movement tracking (Nataraj and van den Bogert, 2017).
Implicit measures of agency may be best utilized for adapting rehabilitation paradigms for grasp since they are less prone to conscious response bias (Wegner, 2003; Saito et al., 2015) compared to explicit measures of agency, which require survey-type responses (Moore et al., 2012). Indirect markers of agency, such as intentional binding, may better explain underlying feelings of control that are sensitive to sensory cues (Moore and Fletcher, 2012) and during impaired function as with neuropathological grasp (Delevoye-Turrell et al., 2002). Intentional binding indicates how coupled in time one perceives a voluntary action to an expected sensory consequence (Haggard et al., 2002; Moore and Obhi, 2012). Time-interval estimation between action and consequence has become a standard to implicitly infer agency via intentional binding. In the seminal work (Haggard et al., 2002), participants judged the time duration between an action (keypress) and sensory consequence (sound tone). A perceptual shift toward time compression was observed when the action was voluntary (high agency) versus an involuntary twitch (low agency) from transcranial magnetic stimulation. Intentional binding has since been used to explore agency in various contexts, including the influence of sensorimotor processes on agency from internal predictions and external outcomes (Moore and Haggard, 2008; Frith and Haggard, 2018).
Time-interval estimation methods for implicit measurements of agency are well posed for rehabilitation training. These methods can quantify agency trial-to-trial and are classically used with sensory feedback experiments. These experiments are similar to motor rehabilitation protocols employing external reward and sensory cues through VR (Sveistrup, 2004; Saleh et al., 2017). Any programmable interface for rehabilitation training or assistive device tuning can potentially adapt parameters for greater agency. Parameters include feedback gains (Nataraj et al., 2010, 2012b; De Havas et al., 2018) or customized settings within training environments (Velazquez et al., 2008). Systematic and computational approaches to adapt user training through agency would readily apply to any advanced rehabilitation platform (VR, robotics) or powered assistive devices such as exoskeletons (Farris et al., 2013) and neuroprostheses (Nataraj et al., 2012a,b; Marasco et al., 2018). The objective of agency-based rehabilitation would be to leverage perception of control for more effective user performance of functional tasks involving hand grasp. However, it remains unclear if varying control modes can effectively co-modulate agency and performance of grasp.
In this study, we hypothesized that agency was positively related to performance of a grasp force task. To test this hypothesis, we varied the control of a grasp force trace that participants visually tracked to match a target ramp. Error to the ramp served as the primary performance metric. We sought to observe potential covariation of agency and grasp force performance across various control modes. The testing environment utilized a force-sensitive pinch apparatus to record forces that were visually projected under the terms of each control mode. Completion of the ramp signified an “action” to be coupled to sensory “consequences” (visual and sound events) from which users estimated lapsed time intervals to implicitly infer agency via intentional binding. Each control mode defined the speed the force trace would move proportional to grasp force and if there existed a measure of noise or assisted automation. These control modes are consistent with parameters commonly adapted for powered devices such as setpoints for speed (Blaya and Herr, 2004; Wege et al., 2005), noise mitigation (Taylor et al., 2002; Agostini and Knaflitz, 2012), and automated assistance (Ronsse et al., 2011). These parameters can be tuned ad hoc (Terenzi, 1998) or identified through optimization of mechanical performance (e.g., effort, tracking) in a model system (Davoodi et al., 2007; Nataraj and van den Bogert, 2017). This study may newly inspire a cognitive basis from which to adapt such parameters in the rehabilitation of grasp performance.
The protocol in this grasp study was repeated for both a rigid and compliant surface. Compliance has been extensively considered for the object being grasped (Friedman and Flash, 2007; Nataraj et al., 2015) and in the design of robotic hands (Kazemi et al., 2012) that better mimic natural human grasp. As such, we sought to investigate how compliance may additionally affect the covariation of agency and performance across control modes. We hypothesized that compliant surfaces may induce higher agency due to the freedom to express more dexterous manipulation. Ultimately, we were able to observe how specific control modes may uniquely affect agency and performance of grasp against a rigid and compliant surface.
Materials and Methods
The core experimental task involved participants controlling a visible trace to dynamically track a target ramp through precision pinch (index finger and thumb) grasp loading onto a force-sensitive pinch apparatus (Figure 1). Performance and agency were assessed across a variety of control modes for the force trace. An initial control mode, specified as “Baseline,” translated grasp loads to changes in trace height at a fixed gain. This gain was ∼2 vertical inches on the screen per 1 N total force applied. Total force was computed as the sum of the 3D (x-y-z) force vector of the index finger to that of the thumb, or:
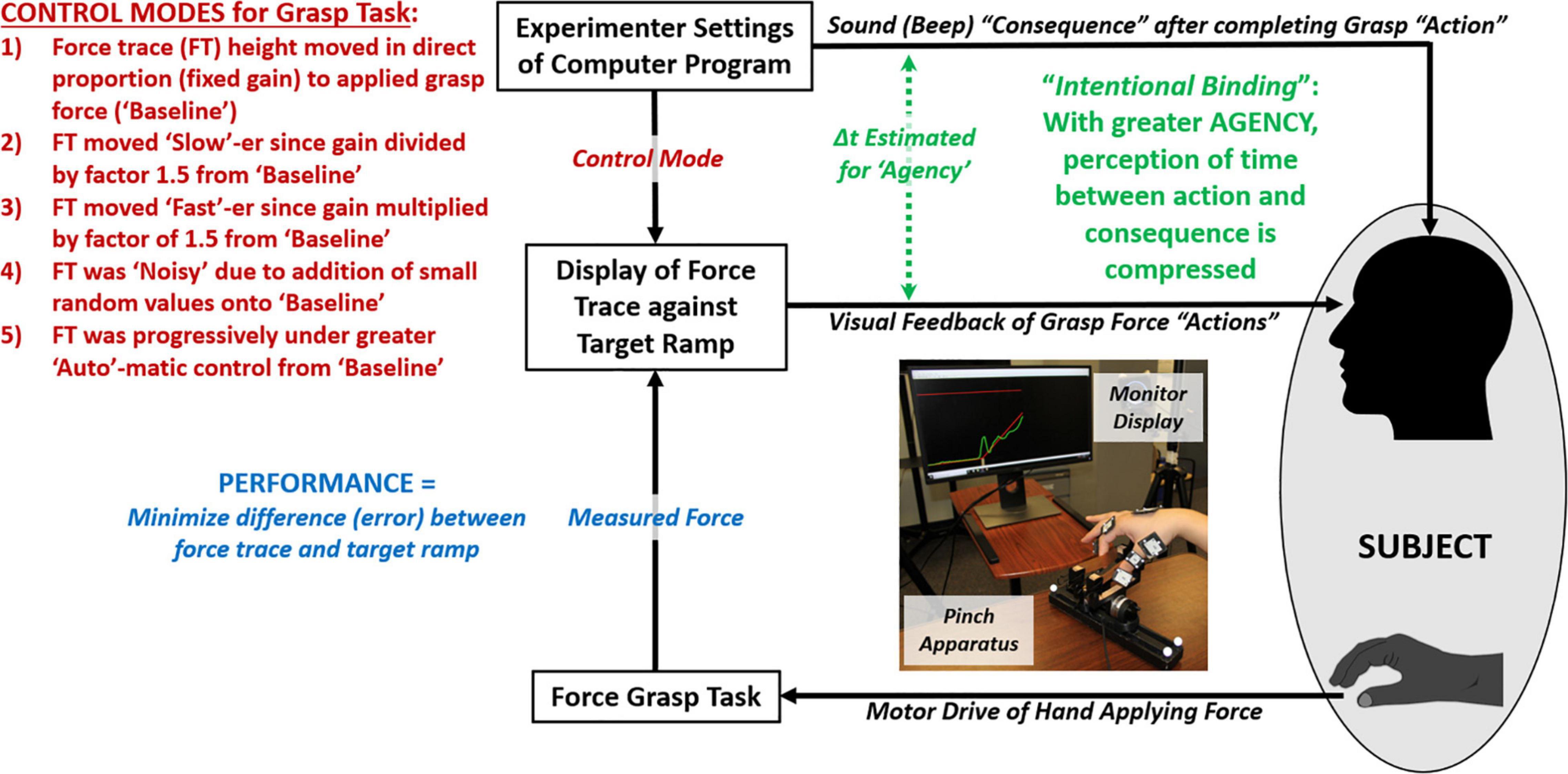
Figure 1. Flow diagram of experiment of participant performing grasp force task to visually trace a target ramp under varying control modes while assessing performance and agency.
Other control modes were modifications from “Baseline” that involved changes in gain magnitude (and required peak force), addition of noise, or inclusion of automation. Participants were asked to maximize tracking performance in matching the force trace to a target ramp. Additionally, participants were required to apply a peak force that exceeded the top of the ramp to complete grasp “action” for each trial. This action would subsequently initiate a sound beep as “consequence” from which participants estimated the time-interval between action and consequence to assess agency. Based on intentional binding, the more subjects underestimate the time-interval, i.e., compress their perception of time, they exhibit greater agency in coupling their actions to related consequences.
Participants
A total of 16 able-bodied volunteers (12 male, 4 female, 21 ± 3 years) were recruited to participate in this study. A power analysis for ANOVA at 95% suggested that seven-participant samples would show significant differences (α = 0.05) in agency and grasp force performance across the tested control modes. Only right-handed participants were tested for right-hand grasp to avoid effects of hand dominance. All participants had normal or corrected-to-normal vision and did not report nor demonstrate a history of disease, injury or complications involving cognition or upper extremity function. All participants signed an informed consent form for this study approved by the Stevens Institutional Review Board.
Equipment (Hardware and Software)
A custom pinch apparatus (Figure 2) was constructed utilizing two 6-DOF load cells (Mini40, ATI Industrial Automation, Apex, NC, United States). The designated locations for applying pinch force included a surface for the index finger in parallel to a surface for the thumb. Both locations could accommodate surfaces as either a metal bar (rigid surface) or an elastic band (compliant surface). The band was set to provide approximately constant compliance of 1.5 N/cm normal to the surface. Data was acquired on a multi-input/output data acquisition system (PXIe-6363 with BNC interface, National Instruments, Austin, TX, United States). Force data was sampled at 100 Hz and processed in software developed in Simulink (Mathworks, Natick, MA, United States). The force trace was displayed in real-time on a 27-inch monitor (Dell P2717H).
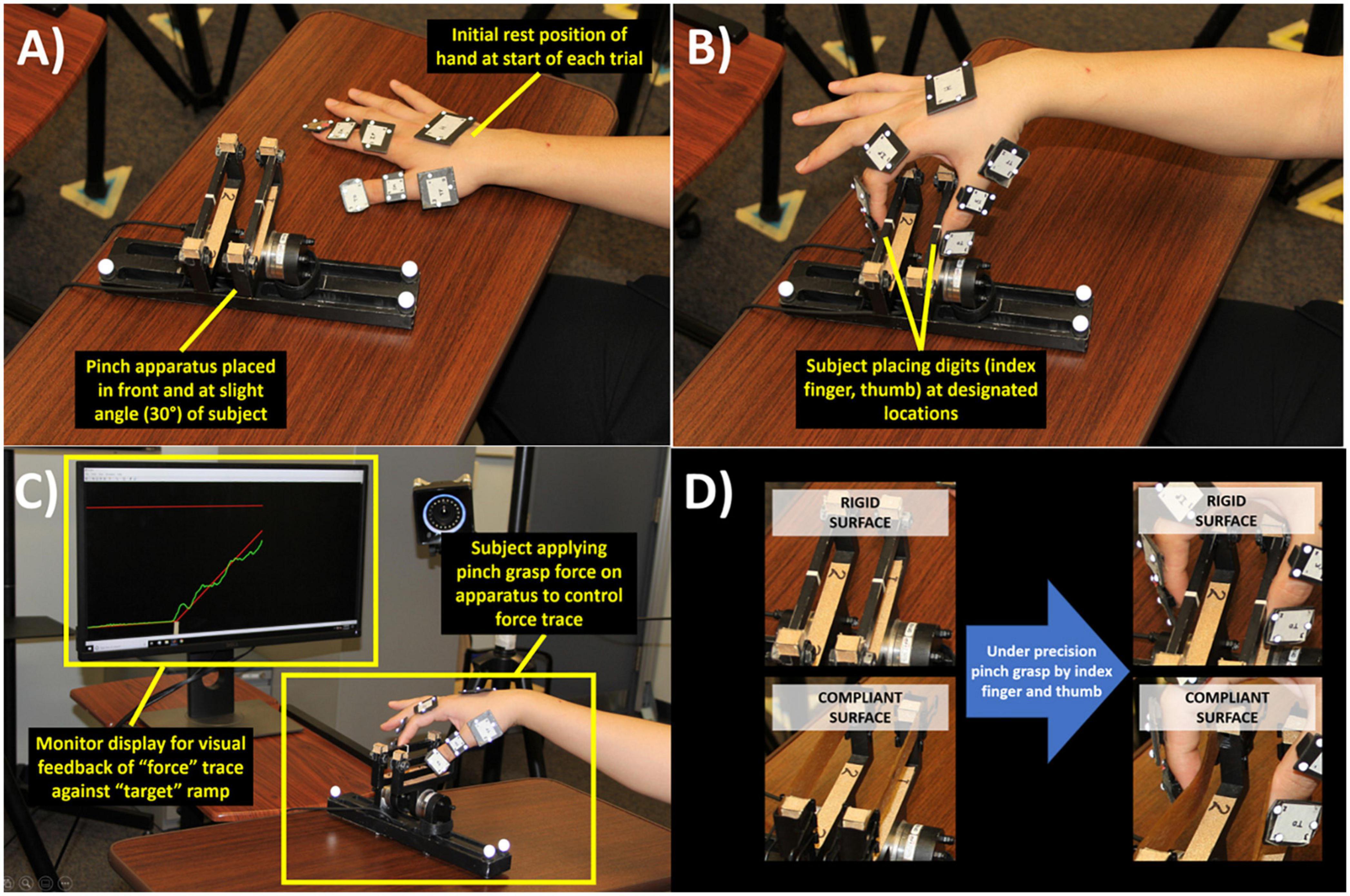
Figure 2. Participant applied grasp force to pinch apparatus each trial. (A) Participant hand initially palm-down on table to start each trial. (B) After trial began, participant moved hand to contact index finger and thumb on designated locations of grasp surfaces on pinch apparatus. (C) Participant progressively applied grasp force to control height (up-down) of force trace to match ramp as trial progressed. (D) Protocol repeated for rigid and compliant grasp surfaces.
Protocol
Participant Preparation
After arriving to the laboratory, participants were re-informed about consent and had their right-hand size measured. Hand size was measured as the maximum spread distance from tip of thumb to tip of index finger. The average hand size was 15 ± 1 cm. For each participant, the distance between the index and thumb pinch surfaces was set at one-third of their hand size. Each participant was seated with chair height adjusted so the grasping hand could be table-supported with shoulders comfortably level. The pinch apparatus was kept in place on the table surface with double-side adhesive tape. The apparatus was positioned directly in front of the participant midline and oriented 30-degrees so that the index finger surface was comfortably forward and leftward to the thumb while grasping (Figure 2). The distance between the participant and the apparatus was set for a comfortable reach when grasping. The monitor displaying the force trace and ramp was placed approximately at participant eye level at a distance 1.5 m from the head.
Force Grasp Task
The experimenter cued the participant to the start of each trial, at which time, the participant would move the hand from rest, palm-side down on the table, to place their index finger and thumb near, without contact, designated locations on grasp surfaces of the apparatus. Each trial with data capture was 10 s. At the start of each trial (ttrial = 0 s), the participant began to see real-time tracing of three lines. All three lines moved horizontally at a constant speed of 2.35 inches per second, computed as screen width divided by total trial time. The three lines (Figure 3) included: (1) force trace (green line) – the force trace height (upward vertical displacement) was under participant control and moved at fixed gain proportional to total grasp force. The force trace was additionally modified depending on the control mode applied. (2) target performance trace (red line) – this target trace was initially flat at height coincident with the force trace when no grasp forces were present. This target trace transitioned to a positive linear slope (ramp) from ttrial = 3 s to ttrial = 7 s. The ramp height grew at slope of ∼2 inches/sec over the 4-s ramp period. The bottom of the ramp coincided with zero force and the top with the target maximum force. (3) target action trace (red line) – this target trace was flat throughout the trial and remained at a height coincident with the target maximum force. This target maximum force was the same for all subjects since grasp forces were relatively low with maximum force around 5N. Variations in the maximum force were based on the specific control mode (described under “Varying control modes”). This target trace would meet the performance trace at the top of the ramp (ttrial = 7 s).
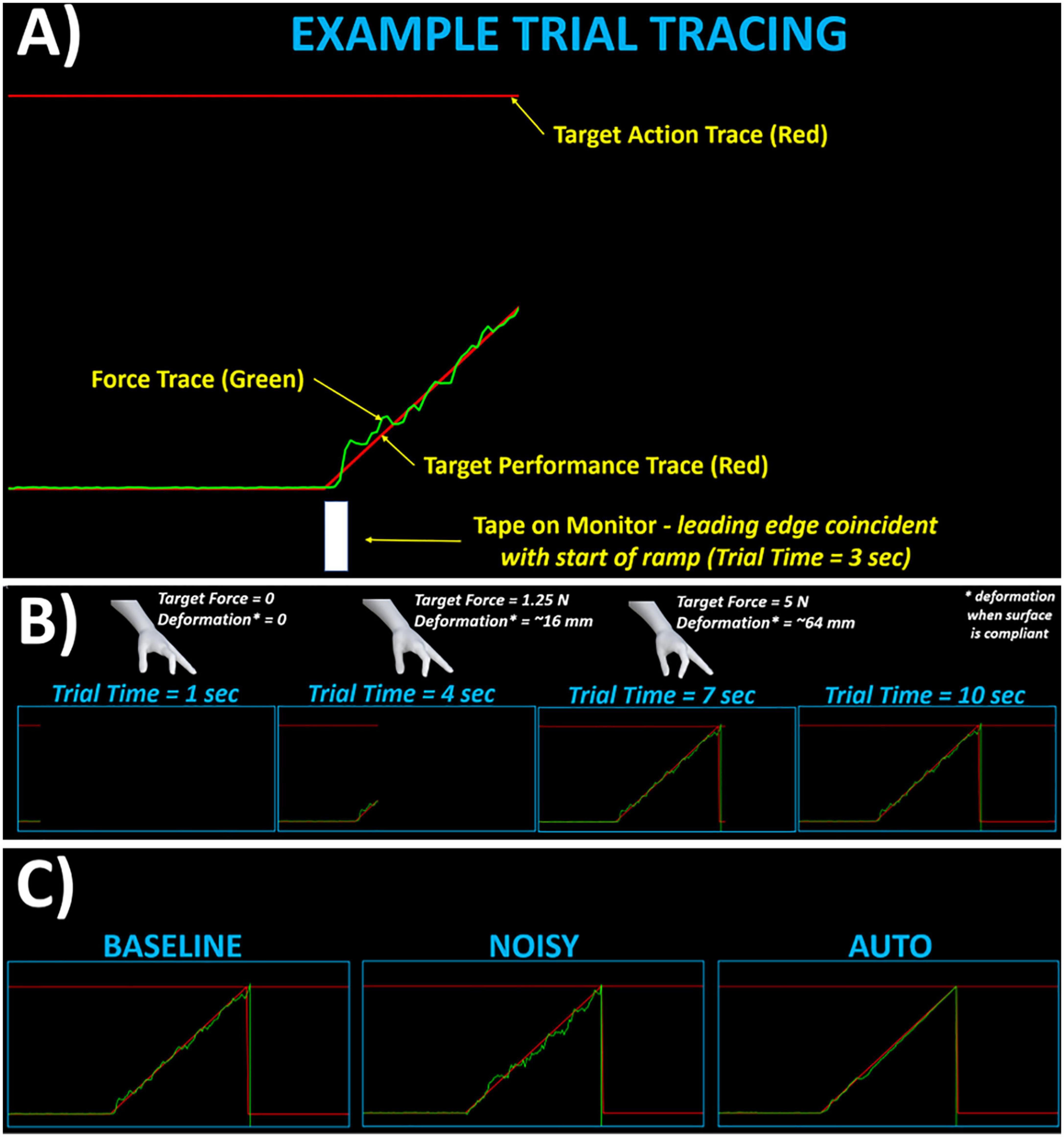
Figure 3. Snapshots of real-time tracings shown for various trial cases. (A) Example depiction of force and target (action, performance) traces shown during ramp portion of trial. (B) Tracing shown at various progressive instances across the 10-s trial. Also shown are depictions of respective grasp postures and force-deformation moduli assuming a “compliant” grasp surface. (C) Representative final traces shown for trials with “Baseline”, “Noisy”, and “Auto” control modes.
The performance objective of the participant was to apply grasp forces to match, as best to their ability, the green force trace with the target performance trace. The participant was cued as to when the ramp would start by a piece of tape at the monitor base whose starting edge was coincident to ttrial = 3 s. To successfully complete the trial and fulfill the agency objective, the participant needed to ensure the force trace crossed (contacted) the target action trace near (within 1 s) the top of the ramp. The crossing served as completion of the grasp “action” that then triggered a subsequent sound beep as “consequence” after some time-interval. The beep was moderately pitched with duration of 100 msec. The beep occurred at some time-interval between 100 and 1000 msec. The participant was asked to verbally estimate the time-interval to the best of their abilities after each beep. The participant was previously instructed that the time-interval was anywhere from 100 to 1000 msec in denominations of 100 msec. The actual time-intervals were always 100, 300, 500, 700, or 900 msec.
Varying Control Modes
All participants performed a block of trials of the grasp task for each of five different control modes, which were randomly presented. As previously described, the control modes examined in this study considered modifications in gain, addition of mild noise, and automation. The control modes were as follows:
(1) Baseline – The force trace magnitude (height) moved in direct proportion to the total grasp force applied at a gain of 2 in/N. The target maximum force associated with the top of the ramp at the end of 4-s period was 5 N. This control mode served as the template from which other control modes were modified.
(2) Slow – The force trace magnitude moved at a speed slower than “Baseline” for a given grasp load. Specifically, the gain was divided by 1.5 (reduced to 1.33 in/N) and the target maximum force consequently became 7.5 N. The participant needed to apply 50% more force on average than Baseline to accurately track the target ramp.
(3) Fast – The force trace magnitude moved at a speed faster than “Baseline” for a given grasp load. Specifically, the gain was multiplied by 1.5 (increased to 3 in/N) and the target maximum force consequently became 3.33 N. The participant needed to apply 33% less force on average than Baseline to accurately track the target ramp.
(4) Noisy – The force trace moved at the same speed as “Baseline” but was visually infected by mild noise. A small random value between (−0.5N, +0.5N) was added to each displayed instance of the force trace. This noise-level produced visible tremor that was noticeable but not distracting nor challenging in performing the grasp task.
(5) Auto – The force trace was progressively (linear with time) under greater automatic control. At the start-time of the ramp (tramp = 0, ttrial = 3 s), the participant controlled the force trace just as in “Baseline.” Over the 4-s ramp period, the force trace with “Auto” control (FTauto) was a weighted average between the participant’s force trace with “Baseline” control (FTbase) and an optimal trace (FTopt) that perfectly matches the ramp. The displayed force trace for “Auto” was given as: . At tramp = 4 s, the force trace was guaranteed to match the top of the ramp and simultaneously match the target action line. This automated case was akin to user initiation of movement to trigger device assistance and auto-complete the movement (Farris et al., 2013).
Experimental Testing Blocks
Participants would perform a block of 20 consecutive trials for each of the five control modes. The first three trials of every block were “practice” with the time-interval between grasp action completion and the beep fixed at 1000 msec. The participant was aware these practice trials served to gain familiarity with the control mode and to re-calibrate their internal reference of a 1000 msec time-interval. The remaining 17 trials were used for agency and performance assessment with randomly presented time-intervals ranging from 100 to 900 msec with Gaussian distribution. Each participant was given up to 5 min between blocks to rest and complete a survey to rate their explicit subjective experience for the completed control mode. The trial-blocks for each of the control modes were conducted first for the rigid surface and then repeated for the compliant surface.
Surveys
For each trial-block, the participant was presented with a 1-statement survey to express their subjective perception of the control mode presented. Participants were asked to rate, on a 5-point Likert scale (−2 = strongly disagree, −1 = disagree, 0 = neutral, +1 = agree, +2 = strongly agree), to what extent the observed force trace movements reflected their intentions. The survey responses served as an explicit, or conscious, measure of agency (Moore et al., 2012) for comparison to the implicit measurements of agency.
Data and Statistical Analysis
There were four data variables serving as the primary metrics in this study as follows:
(1) Implicit agency (msec) was the underestimation in time-interval between “action” (completion of force ramp) and “consequence” (delayed sound beep) to signify greater intentional binding. This measurement was taken once with each trial.
(2) Performance (N–1) was the inverse of the grasp force error during the 4-s ramp period to signify greater force tracking. Each measurement was taken as the mean error per trial.
(3) Control efficiency (sec2/N2) was the normalization of performance by force acceleration (N/sec2) to signify the error per unit acceleration effort to make corrections in tracking a constant velocity ramp. This variable was computed concurrently with performance.
(4) Explicit agency (Likert scale) was the survey response score on subjective perception of control mode. This measurement was done once after each block of trials.
The analyses that were performed on the above metrics are as follows:
Analysis 1: A linear regression was applied to performance (y-axis) and implicit agency (x-axis) data to assess the dependence of performance on agency for each surface in the aggregate (across all control modes, subjects). The F-statistic and p-value were computed to refute the null hypothesis that the slope coefficient was equal to zero and suggest significant dependence of performance on implicit agency. ANCOVA was performed to assess significant difference in slopes between compliant and rigid surfaces and to determine if significant slopes were observable within each control mode, not just in the aggregate.
Analysis 2: We performed a repeated-measures two-way ANOVA (factors for surface-type and control mode) for each metric to observe main effects due to each factor and potential interactions between factors. For significant factors, post hoc pairwise comparisons were done with Bonferonni correction for multiple comparisons. For multiple comparisons, all reported p-values are scaled according to the number of comparisons such that first-level significance is always p < 0.05. Post hoc comparisons allowed for observation of specific simple effects to be considered for each pair of control modes within surface type.
Analysis 3: Finally, the mean variability (standard deviation) in the force profile in each of the three directional dimensions was compared between rigid and compliant surfaces to indicate the presence of any surface-unique directional sensitivities during grasp.
Results
Analysis 1
The aggregate dependence of performance on agency across participant-averages for control modes is shown for each grasp surface in Figure 4. For both surfaces, there was a positive relationship between performance and agency indicated by a non-zero (p < 0.001) regression slope, however, the regression fit to both data sets was low (R2 < 0.20). Dependence of performance on agency was greater (increased slope) for the compliant surface. The increased slope with compliant surface grasp was confirmed with an ANCOVA comparison (Table 1B, p < 0.01). ANCOVA did not reveal significant slope dependence within control modes (Table 1A). ANCOVA did demonstrate significant differences in the intercept parameters both within control modes and in the aggregate across surfaces (Tables 1C,D). Combined slope and intercept results suggest that independent regressions for control modes are parallel but different, and that intercept differences across control modes drive differences in aggregate slope.
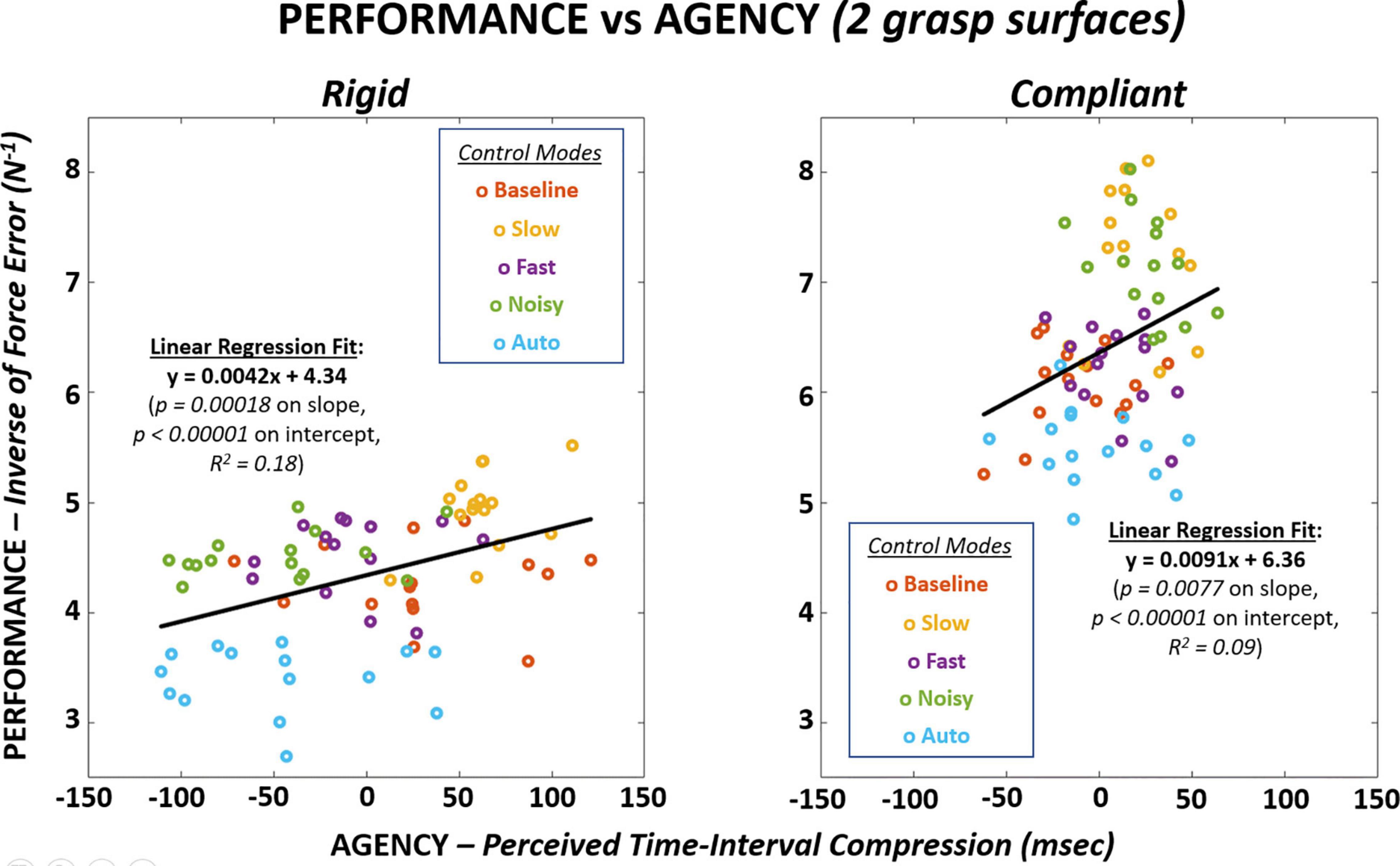
Figure 4. Performance of precision pinch grasp displayed against implicit agency across control modes on both rigid (LEFT) and compliant (RIGHT) grasp surfaces. Each point indicates a participant-average for that control mode and surface. Performance positively measured as inverse of average tracking error to target force ramp. Implicit agency positively measured as underestimation of time-intervals between completion of ramp task and subsequent sound beep.
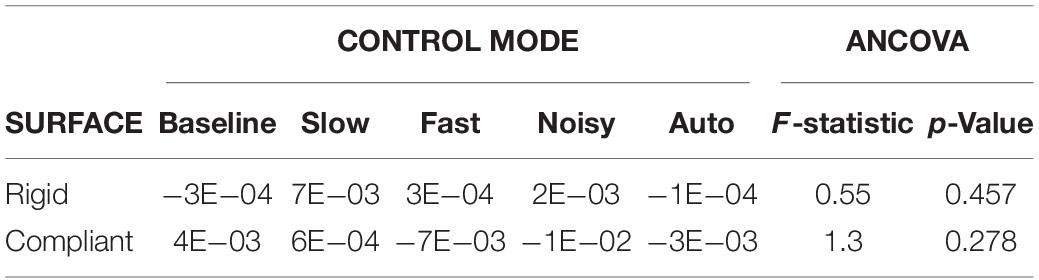
Table 1A. Linear regression results on slope (N–1 msec–1) for each control mode and ANCOVA results across control modes for each surface.
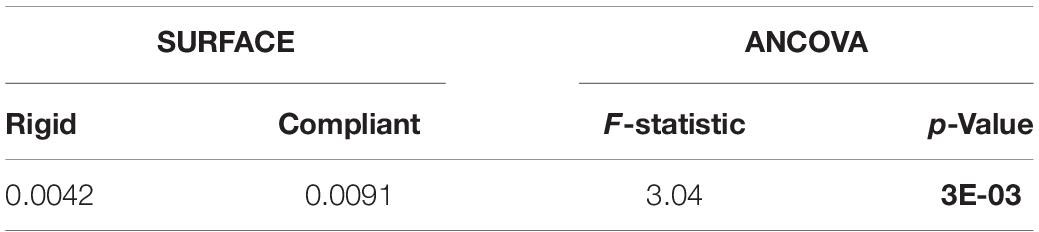
Table 1B. Linear regression results on slope (N–1 msec–1) in total (pooled) for each surface and ANCOVA results across both surfaces.
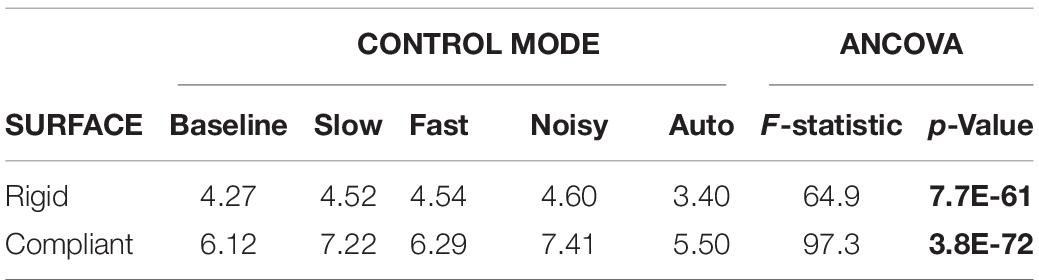
Table 1C. Linear regression results on intercept (N–1) for each control mode and ANCOVA results across control modes for each surface.
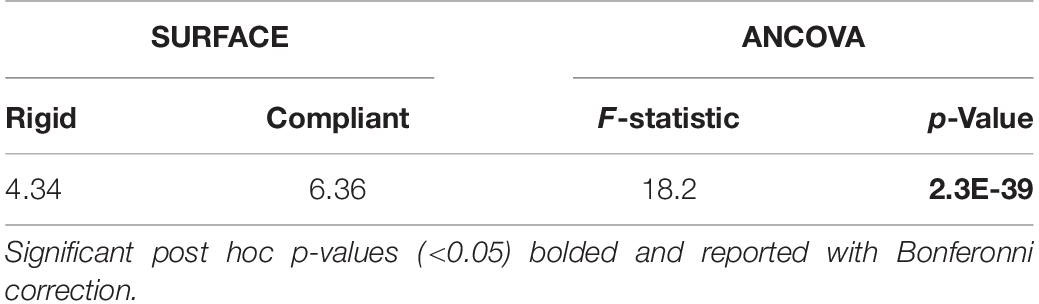
Table 1D. Linear regression results on intercept (N–1) in total (pooled) for each surface and ANCOVA results across both surfaces.
Analysis 2
The two-way (factors of control mode, surface) ANOVA results for each of the primary metrics are shown in Table 2. A significant difference (p < 0.001) was observed with control mode for implicit agency, performance, and control efficiency. A significant difference (p < 0.001) was observed with surface for performance and control efficiency. In each case, the interaction term was significant and required an investigation of simple effects (i.e., hold one factor constant) and post hoc pairwise comparisons.
Several significant pairwise differences (p < 0.0001) were observed in post hoc across control modes for both implicit agency (Figure 5 TOP and Tables 3A–C) and performance (Figure 5 BOTTOM and Tables 3D–F). In the presence of significant interaction between surface and control mode, unique variations were observed across control modes based on surface for both implicit agency and performance. Performance was universally greater for the compliant surface than rigid surface. For the rigid surface, the highest agency and performance with significant pairwise differences (p < 0.0001) were observed for the “Slow” control mode. For the compliant surface, the highest agency and performance with significant pairwise differences (p < 0.001) were observed for the “Slow” and “Noisy” control modes.
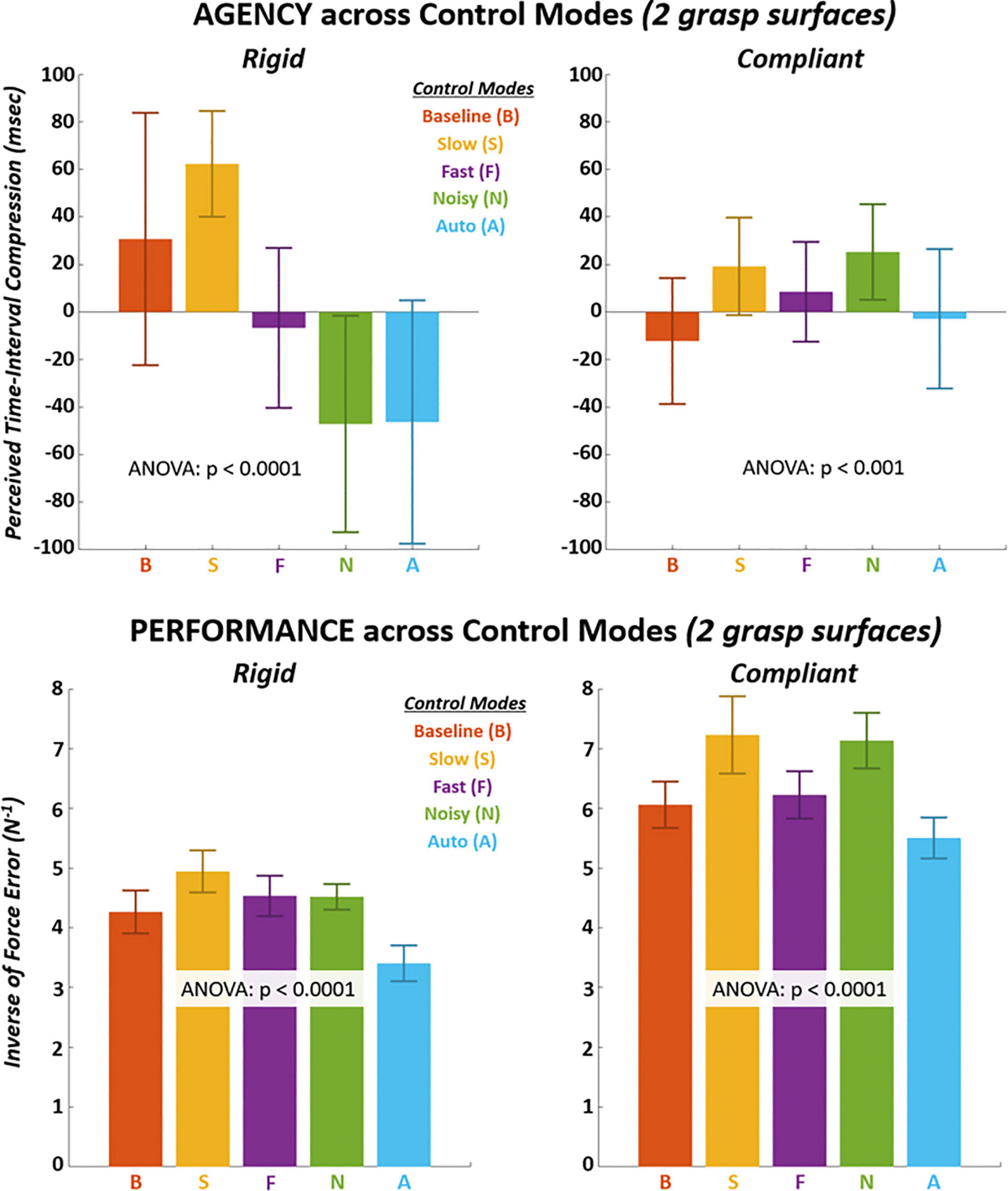
Figure 5. TOP – Mean implicit agency shown for each control mode on each grasp surface. Implicit agency positively measured as underestimation of time-intervals between completion of ramp task and subsequent sound beep. BOTTOM – Mean performance shown for each control mode on each grasp surface. Performance positively measured as inverse of average tracking error to target force ramp.

Table 3A. Mean implicit agency (time-interval underestimation, msec) across control modes on rigid and compliant surfaces.

Table 3B. Post hoc comparisons (p-values) for implicit agency across control modes on rigid surface.

Table 3C. Post hoc comparisons (p-values) for implicit agency across control modes on compliant surface.

Table 3D. Mean performance (inverse force error, N–1) across control modes on rigid and compliant surfaces.

Table 3F. Post hoc comparisons (p-values) for performance across control modes on compliant surface.
Significant pairwise differences (p < 0.0001) were observed in control efficiency (Figure 6 TOP and Tables 4A–C) across control modes for both rigid and compliant surfaces. Similar to performance, there was significant interactions between surface and control mode for control efficiency such that unique variations in efficiency across control modes were observed for each surface. Furthermore, performance efficiency was also universally greater for the compliant surface. The lowest control efficiency was observed with “Auto” control mode for both surfaces and with multiple significant (p < 0.0001) pairwise differences. Significant differences (p < 0.05) were not observed for explicit agency (Figure 6 BOTTOM and Table 4D) except for the compliant surface which demonstrated one significant (p < 0.05) pairwise difference (“Baseline” greater than “Noisy”). As indicated from the two-way analysis, both surface effects and interaction with control modes were absent for explicit agency.
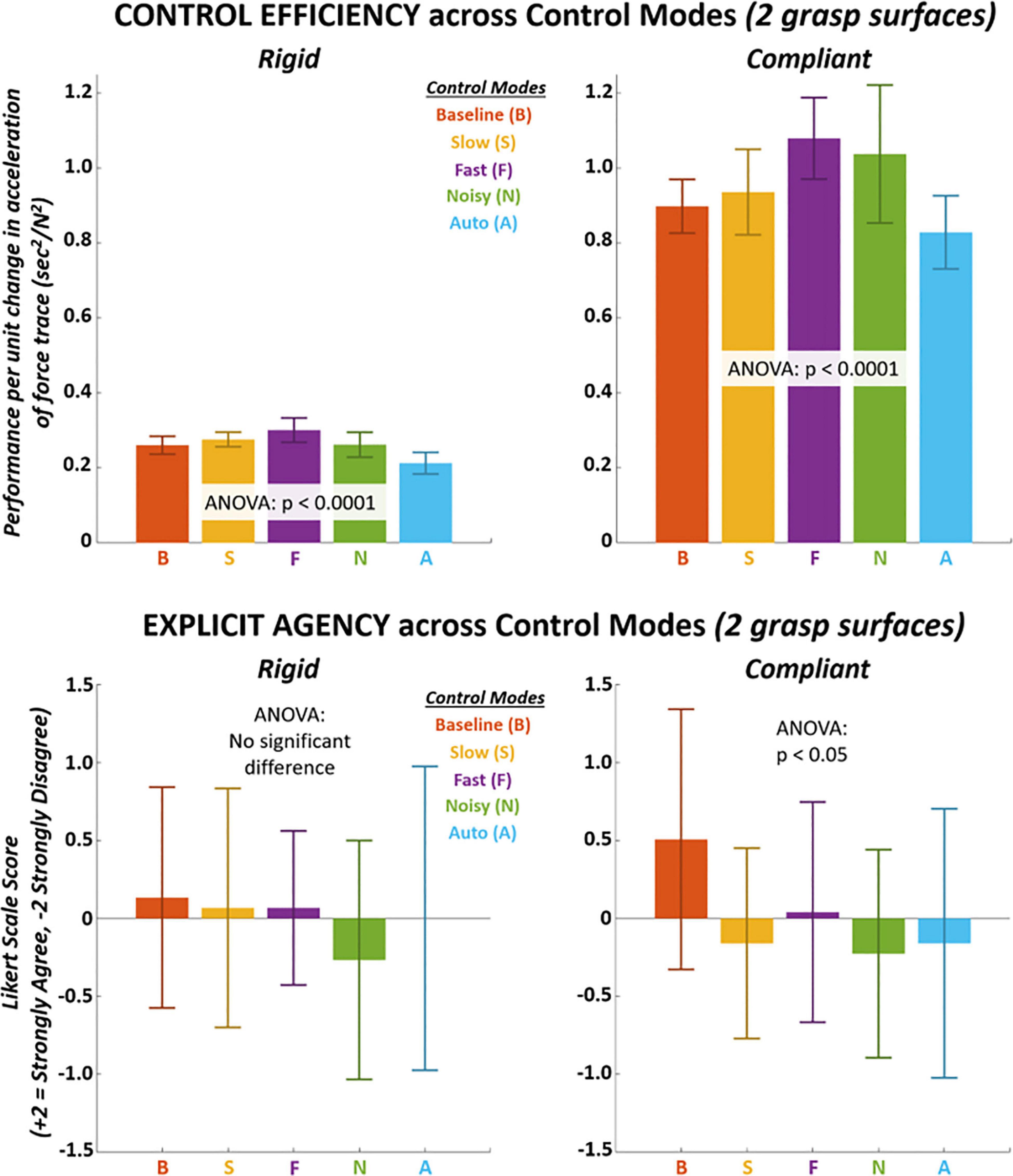
Figure 6. TOP – Mean control efficiency shown for each control mode on each grasp surface. Control efficiency measured as performance normalized by acceleration (2nd derivative) of force trace. BOTTOM – Mean explicit agency shown for each control mode on each grasp surface. Explicit agency measured from survey response along Likert scale: +2 strongly agree, +1 agree, 0 neutral, –1, disagree, –2 strongly disagree. Survey asked participant to what extent they agreed that force trace movements reflected their intended actions after a trial-block for each control mode.

Table 4B. Post hoc comparisons (p-values) for control efficiency across control modes on rigid surface.

Table 4C. Post hoc comparisons (p-values) for control efficiency across control modes on compliant surface.

Table 4D. Mean explicit agency (Likert scale: +2 strongly agree to −2 strongly disagree) across control modes on rigid and compliant surfaces.
The shifts in metrics from rigid to compliant surfaces across control modes are explicitly shown in Figure 7 and Table 5. significant differences (p < 0.001) were observed for all metrics except for explicit agency (p > 0.05). The largest shifts for implicit agency, performance, and efficiency were observed for the “Noisy” control mode.
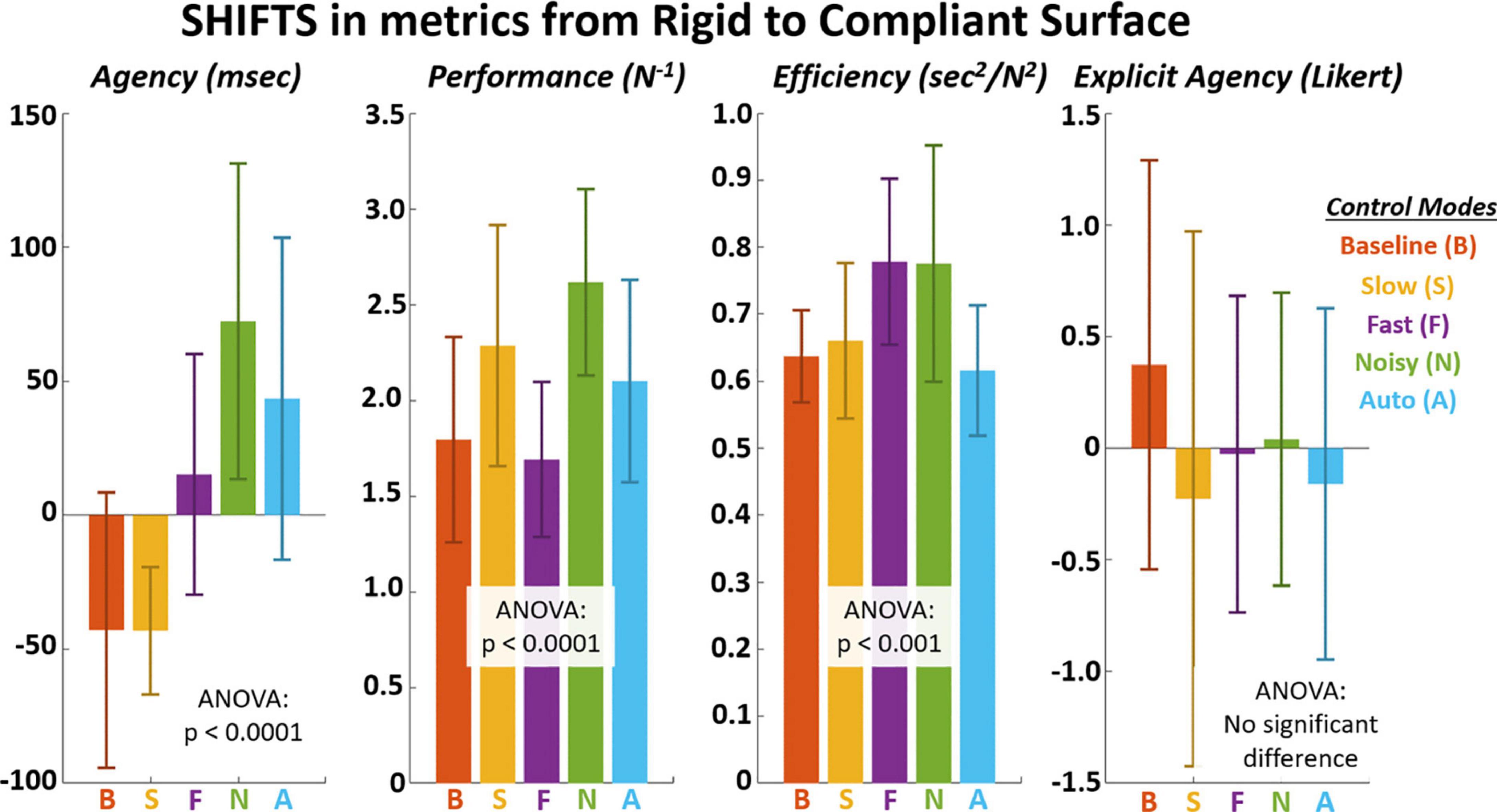
Figure 7. Shifts in metrics across control modes from rigid to compliant grasp surface. The surface-control mode interaction p-values are as follows: implicit agency = 1E-05, performance = 5E-05, efficiency = 2E-04, explicit agency = 0.57.

Table 5. Mean shifts from rigid to compliant surface for implicit agency (msec), performance (N–1), efficiency (sec2/N2), and explicit agency (Likert).
Analysis 3
The mean absolute force profiles across a 10-s trial for a single digit are shown for each dimension and for rigid versus compliant surfaces in Figure 8. These mean force trajectories are taken across both digits and all subjects for the “Baseline” control mode. As expected, the greatest force was applied in the direction normal to the grasping surface. Significant differences (p < 0.05) in force variability (standard deviation) between surfaces were observed in the lateral and normal dimensions.
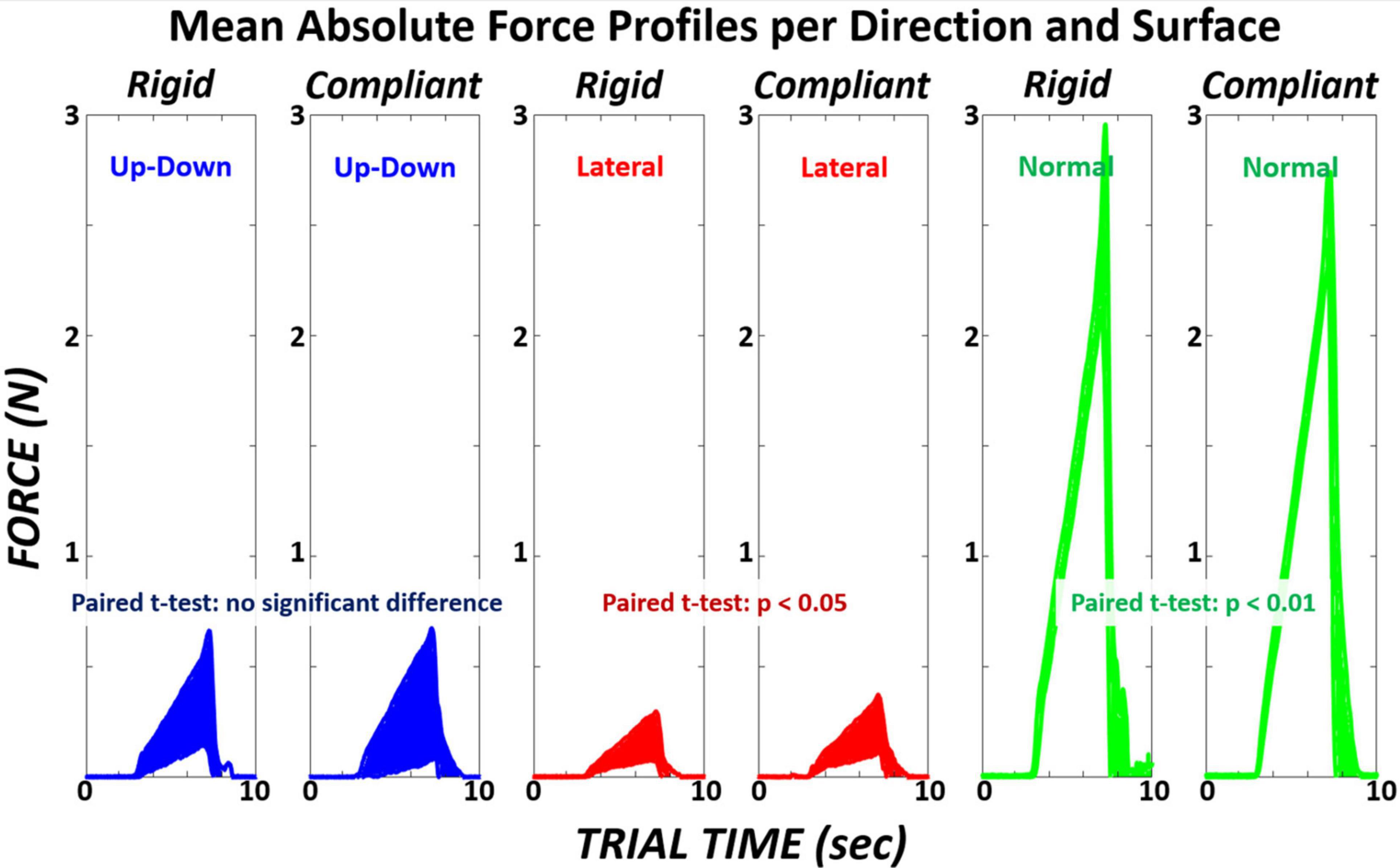
Figure 8. Mean absolute force profile across the 10-s trial for a single digit shown in each dimension (up-down, lateral, normal) for rigid versus compliant surfaces. Profile thickness indicates ±1standard deviation. Paired t-test performed across the average variability (standard deviation) for each dimension during ramp period (ttrial = 3–7 s). The mean force variability in each dimension for the rigid surface was 0.294, 0.098, and 0.256 N. The mean force variability in each dimension for the compliant surface was 0.291, 0.142, and 0.173 N.
Discussion
In this investigation of a precision grasp force task, we observed a positive relationship between implicit agency and performance and that both metrics can vary with modes of control. Furthermore, these general observations are consistent for grasp on either a rigid or compliant surface. While the positive dependence of performance on agency was significant and greater for the complaint surface, the regression fit was low. This suggests agency alone cannot “predict” performance and other explanatory variables are still needed. Furthermore, changes in control modes are required to elucidate this dependence, suggesting that agency can play a role in a dynamic framework for user-device adaptation. However, within a control mode whereby the user has presumably accommodated to a given condition (i.e., control mode), the performance-agency dependence may be diminished.
The dependence of performance on agency should motivate rehabilitation approaches that consider cognitive engagement beyond just entertainment and gamification (Sveistrup, 2004), but rather more efficient modes of physical therapy that restore neuromotor connectivity (Carter et al., 2012). Fostering motivation and engagement for greater participation is critical to ensure effective dosages of rehabilitation training (Hsieh et al., 2012; Stevenson et al., 2012). Our results additionally suggest that if cognition is systematically monitored and leveraged in real-time, the rehabilitation training sessions may further accelerate functional gains at a given level of participation.
Utilizing computerized interfaces, rehabilitation methods could readily leverage reliable, real-time cognitive measures with automated computational approaches. Optimization routines could be employed to systematically alter a VR training environment (Eddy and Lewis, 2002) with sensory feedback cues (visual, audio, haptic) that specifically enhance agency (Beck et al., 2017; Borhani et al., 2017; Wen et al., 2018). Presentation of avatars or goal-oriented tasks may be continually modified to elicit greater cognitive engagement while monitoring and promoting performance (Shin et al., 2014). Power-assistive exoskeletons and prosthetics driven by physiological commands (Lotte et al., 2012) may have input-output parameters [feedback gains, setpoint speeds, trajectories (Nataraj and van den Bogert, 2017)], customized to co-maximize agency and performance.
Virtual reality may be an appropriate platform to identify training settings and device parameters that maximize function and perception of control for rehabilitative and assistive interfaces (Sutcliffe and Kaur, 2000). Depending on the nature and extent of neuromuscular deficit, the training paradigm may be aimed toward either rehabilitating independent function or improving outcomes with a powered assistive device. In this study, VR training implications are specific to rehabilitation or powered assistance of hand grasp. Prevalent clinical populations include persons with impaired grasp due to hemiparesis, cervical-level spinal cord injury, or upper-limb amputation (Ma et al., 2014). In this study, we observed grasp movement training through a computerized interface with variations in control modes and surface types.
The factors of control modes and surface type demonstrated significant interaction in having significant effects on implicit agency, performance, and control efficiency. As such, it was necessary to observe simple effects with each factor while holding the other factor constant. Participants in this study demonstrated significantly greater hand grasp performance, efficiency of performance, and performance dependence on agency when grasping a compliant surface. Several investigations in hand grasp robotics employ algorithms that command actuation based on compliance (Cutkosky and Kao, 1989; Prattichizzo et al., 2012; Deimel and Brock, 2016). These approaches facilitate digit-level synergies that accommodate several degrees of freedom against a variety of grasp object sizes, shapes, hardness, and surface friction (Michelman and Allen, 1993; Kim et al., 2003; Dollar and Howe, 2006; Dollar et al., 2010). Our study suggests that compliance-based approaches are not only flexibly functional, but they may also encourage users to execute grasp with greater perception of control. While the shift in agency with compliant surfaces was negative for the “Baseline” and “Slow” modes of control, the largest singular shift was positive and observed with the “Noisy” mode.
Noise in sensory feedback can enhance functional performance (Priplata et al., 2002). However, visual noise is typically a distractor to impair performance (Vasilakos and Beuter, 1993; Baldassi et al., 2006). In our study, visual noise was relatively mild (magnitude <5% of target maximum force) and did not generate a significant change in performance from “Baseline” for the rigid surface. Visual noise did significantly reduce agency for the rigid surface, suggesting participants readily dissociated the visual noise from their own true actions (Miele et al., 2011). However, for the compliant surface, both agency and performance significantly increased from “Baseline.” The increase in agency suggests participants perceptually embodied the noise of the trace into the actions of their own digits (Caspar et al., 2015). Facilitating body representation with complex visual feedback can enhance movement performance (Sanford et al., 2020). Furthermore, the inability to dissociate the noise may be explained by the increased uncertainty introduced with the compliant surface. However, this erroneous perception of control did not reduce performance, but rather enhanced it. It is conceivable that while the noise did not represent true actions, the compliant surface may have amplified participant perception of freedom to enact greater control. In turn, this enhanced perception may have effectively increased performance responsivity, akin to increasing feedback gains on true visual error (Wei et al., 2005).
Unlike “Noisy,” the “Auto” control mode significantly reduced both performance and agency compared to “Baseline” across both surfaces. This finding suggests a sensitivity to gradual automation in grasp that reduced self-agency. The reduced perception of control appeared to reduce independent performance despite display of excellent performance with “Auto.” We posit that this performance reduction was not due to conscious awareness of automation since no significant differences in explicit agency were observed. The major implication for assistive devices is the importance of continuous user control in restoration of hand grasp. Traditionally, users generate a command beyond a threshold, with electromyography (EMG) (Marasco et al., 2018) or mechanical switches (Farris et al., 2013), to trigger a “go” command to the movement device (Geethanjali, 2016). The device will then automatically complete a movement sequence, such as grasp closure (Hart et al., 1998) or a step (Hartigan et al., 2015), without further user input until movement completion. While efficient in executing preprogrammed functions, interfaces with greater automation may severely hinder sense of user control and engagement to the device. More complex functions require nuanced user commands that may need to be identified through machine learning classification of EMG patterns (Zhou et al., 2017; Burns et al., 2019). However, even relatively simple tasks, such as ramped grasp force, may be benefited by more continuous user control to enhance agency and performance.
The “Slow” and “Fast” control modes effectively served as higher and lower grasp force ramps relative to “Baseline.” Changes in visual display were effectively uniform once participants accommodated to the load rate required to accurately track the ramp. Since control modes were randomly presented and required grasp loads were relatively small, we do not attribute metric differences for these modes due to learning or fatigue. Participants demonstrated significant increase in agency and performance with “Slow” compared to “Baseline” suggesting greater engagement and capability with higher grasp force. Previous studies have suggested greater agency may be facilitated through greater effort (Demanet et al., 2013). It is not clear if an optimal force level exists for precision pinch from this study. Our objective only sought to specify a low force pinch task and subsequently identify the changes in performance and agency due to control modifications that include changes in force level, addition of noise, and level of automation.
The major limitation of this study was examination of only one level for each control mode type. This study prioritized initial identification of agency and performance across a variety of mode types and two grasp surfaces. For fully customized deployment of an assistive device, it would be necessary to tune across multiple levels of each control mode concurrently. Control settings such as force magnitude, noise amplitude and frequency, and degree of automation may be specified independently or in unique combinations. The next phase of this research should establish methods that efficiently identify device settings such as optimal feedback gains with selective sampling (Nataraj et al., 2014). Optimal parameters could be determined to not only minimize performance errors for better movement (Neptune, 1999; Xiang et al., 2010). But to maximize quantifiable metrics for cognition. Our study suggests implicit agency may be such as agency for greater user-device integration during rehabilitation practices. An assumption of this study was that gender does not affect grasp performance or agency. Gender-based differences for movement agency and precision grasp have not been well established, but not considering gender as a factor is a potential study limitation.
Future studies should also consider alternative measurements of agency. While explicit metrics for agency have been commonly utilized in other studies (Dewey and Knoblich, 2014), variability was too large to identify significant differences in this study. This study did demonstrate that an implicit measure of agency, time-interval estimation for intentional binding, had correlation to performance in the aggregate and could be modulated across control modes. It remains unclear if this metric has sufficient sensitivity and resolution for customization of rehabilitation programs and devices to individual users. Furthermore, requiring each user to provide a verbal estimate of time-intervals would be tedious and cognitively fatiguing while adapting the training interface. The user should be able to devote greater attention to operating the interface while implicit agency is passively measured.
Physiological measures such as EMG and brain electroencephalography (EEG) may be monitored to reflect changes in cognitive agency (Tsakiris et al., 2006; Kang et al., 2015; Beyer et al., 2017). These measures need to be validated as a reliable surrogate for implicit agency and device-based rehabilitation interfaces. Computational optimization tools may be employed to concurrently adapt the user-device interface based on dynamic features of real-time EEG and EMG to assess cognitive integration. To this end, probabilistic methods that evaluate embodied cognition (Ryu and Torres, 2018) may be especially appropriate to extract perceptual features from stochastic physiological signals such as EEG and EMG. Reliable and validated physiological measures for agency would facilitate autonomous adaptation of rehabilitation interfaces for greater cognition. Interfaces include VR-based training paradigms and powered assistive devices such as prosthetics and exoskeletons.
Data Availability Statement
The underlying data supporting the conclusions of this article will be made available by the authors, without undue reservation.
Ethics Statement
The studies involving human participants were reviewed and approved by the Stevens Institutional Review Board. The patients/participants provided their written informed consent to participate in this study.
Author Contributions
RN contributed to designing and developing the experiment, analyzing the data, writing and revising the manuscript, and directing the project. SS contributed to recruiting participants, performing the data collections, and revising the manuscript. Both authors contributed to the article and approved the submitted version.
Funding
New Jersey Health Foundation award PC 53-19 assisted with fees related to computer supplies used for data analysis.
Conflict of Interest
The authors declare that the research was conducted in the absence of any commercial or financial relationships that could be construed as a potential conflict of interest.
Acknowledgments
The authors would like to acknowledge Aniket Shah and Mingxiao Liu who both assisted with data collection and management. This work was made possible by support from the Schaefer School of Engineering and Science, at the Stevens Institute of Technology and Research grant (PC 53-19) from the New Jersey Health Foundation.
References
Agostini, V., and Knaflitz, M. (2012). An algorithm for the estimation of the signal-to-noise ratio in surface myoelectric signals generated during cyclic movements. IEEE Trans. Biomed. Eng. 59, 219–225.
Antfolk, C., D’alonzo, M., Rosén, B., Lundborg, G., Sebelius, F., and Cipriani, C. (2013). Sensory feedback in upper limb prosthetics. Expert Rev. Med. Devices 10, 45–54. doi: 10.1586/erd.12.68
Baldassi, S., Megna, N., and Burr, D. C. (2006). Visual clutter causes high-magnitude errors. PLoS Biol. 4:e56. doi: 10.1371/journal.pbio.0040056
Beck, B., Di Costa, S., and Haggard, P. (2017). Having control over the external world increases the implicit sense of agency. Cognition 162, 54–60. doi: 10.1016/j.cognition.2017.02.002
Behrman, A. L., Lawless-Dixon, A. R., Davis, S. B., Bowden, M. G., Nair, P., Phadke, C., et al. (2005). Locomotor training progression and outcomes after incomplete spinal cord injury. Phys. Ther. 85, 1356–1371. doi: 10.1093/ptj/85.12.1356
Beyer, F., Sidarus, N., Bonicalzi, S., and Haggard, P. (2017). Beyond self-serving bias: diffusion of responsibility reduces sense of agency and outcome monitoring. Soc. Cogn. Affect. Neurosci. 12, 138–145. doi: 10.1093/scan/nsw160
Blaya, J. A., and Herr, H. (2004). Adaptive control of a variable-impedance ankle-foot orthosis to assist drop-foot gait. IEEE Trans. Neural Syst. Rehab. Eng. 12, 24–31. doi: 10.1109/tnsre.2003.823266
Borhani, K., Beck, B., and Haggard, P. (2017). Choosing, doing, and controlling: implicit sense of agency over somatosensory events. Psychol. Sci. 28, 882–893. doi: 10.1177/0956797617697693
Burns, M. K., Pei, D., and Vinjamuri, R. (2019). Myoelectric control of a soft hand exoskeleton using kinematic synergies. IEEE Trans. Biomed. Circ. Syst. 13, 1351–1361. doi: 10.1109/tbcas.2019.2950145
Carter, A. R., Shulman, G. L., and Corbetta, M. (2012). Why use a connectivity-based approach to study stroke and recovery of function? Neuroimage 62, 2271–2280. doi: 10.1016/j.neuroimage.2012.02.070
Caspar, E. A., Cleeremans, A., and Haggard, P. (2015). The relationship between human agency and embodiment. Conscious. Cogn. 33, 226–236. doi: 10.1016/j.concog.2015.01.007
Childress, D. S. (1973). Powered limb prostheses: their clinical significance. IEEE Trans. Biomed. Eng. BME-20, 200–207. doi: 10.1109/tbme.1973.324273
Cutkosky, M. R., and Kao, I. (1989). Computing and controlling compliance of a robotic hand. IEEE Trans. Robot. Autom. 5, 151–165. doi: 10.1109/70.88036
Davoodi, R., Urata, C., Hauschild, M., Khachani, M., and Loeb, G. E. (2007). Model-based development of neural prostheses for movement. IEEE Trans. Biomed. Eng. 54, 1909–1918. doi: 10.1109/tbme.2007.902252
De Havas, J., Ito, S., Haggard, P., and Gomi, H. (2018). Low gain servo control during the kohnstamm phenomenon reveals dissociation between low-level control mechanisms for involuntary vs. voluntary arm movements. Front. Behav. Neurosci. 12:113. doi: 10.3389/fnbeh.2018.00113
Deimel, R., and Brock, O. (2016). A novel type of compliant and underactuated robotic hand for dexterous grasping. Int. J. Robot. Res. 35, 161–185. doi: 10.1177/0278364915592961
Delevoye-Turrell, Y., Giersch, A., and Danion, J.-M. (2002). A deficit in the adjustment of grip force responses in schizophrenia. Neuroreport 13, 1537–1539. doi: 10.1097/00001756-200208270-00010
Demanet, J., Muhle-Karbe, P. S., Lynn, M. T., Blotenberg, I, and Brass, M. (2013). Power to the will: how exerting physical effort boosts the sense of agency. Cognition 129, 574–578.
Dewey, J. A., and Knoblich, G. (2014). Do implicit and explicit measures of the sense of agency measure the same thing? PLoS One 9:e110118. doi: 10.1371/journal.pone.0110118
Dollar, A. M., and Howe, R. D. (2006). A robust compliant grasper via shape deposition manufacturing. IEEE/ASME Trans. Mechatronics 11, 154–161. doi: 10.1109/tmech.2006.871090
Dollar, A. M., Jentoft, L. P., Gao, J. H., and Howe, R. D. (2010). Contact sensing and grasping performance of compliant hands. Auton. Robots 28, 65. doi: 10.1007/s10514-009-9144-9
Doyle, P. J. (2002). Measuring health outcomes in stroke survivors. Arch. Phys. Med. Rehab. 83, S39–S43.
Eddy, J., and Lewis, K. (2002). “Multidimensional design visualization in multiobjective optimization,” in Proceedings of the 9th AIAA/ISSMO Symposium on Multidisciplinary Analysis and Optimization, (Atlanta, GA), 5621.
Evans, N., Gale, S., Schurger, A., and Blanke, O. (2015). Visual feedback dominates the sense of agency for brain-machine actions. PLoS One 10:e0130019. doi: 10.1371/journal.pone.0130019
Farris, R. J., Quintero, H. A., Murray, S. A., Ha, K. H., Hartigan, C., and Goldfarb, M. (2013). A preliminary assessment of legged mobility provided by a lower limb exoskeleton for persons with paraplegia. IEEE Trans. Neural Syst. Rehab. Eng. 22, 482–490. doi: 10.1109/tnsre.2013.2268320
Friedman, J., and Flash, T. (2007). Task-dependent selection of grasp kinematics and stiffness in human object manipulation. Cortex 43, 444–460. doi: 10.1016/s0010-9452(08)70469-6
Frith, C. D., and Haggard, P. (2018). Volition and the brain - revisiting a classic experimental study. Trends Neurosci. 41, 405–407. doi: 10.1016/j.tins.2018.04.009
Geethanjali, P. (2016). Myoelectric control of prosthetic hands: state-of-the-art review. Med. Devices (Auckl.) 9, 247. doi: 10.2147/mder.s91102
Haggard, P., Clark, S., and Kalogeras, J. (2002). Voluntary action and conscious awareness. Nat. Neurosci. 5, 382–385. doi: 10.1038/nn827
Hart, R. L., Kilgore, K. L., and Peckham, P. H. (1998). A comparison between control methods for implanted FES hand-grasp systems. IEEE Trans. Rehab. Eng. 6, 208–218. doi: 10.1109/86.681187
Hartigan, C., Kandilakis, C., Dalley, S., Clausen, M., Wilson, E., Morrison, S., et al. (2015). Mobility outcomes following five training sessions with a powered exoskeleton. Top. Spinal Cord Inj. Rehab. 21, 93–99. doi: 10.1310/sci2102-93
Hebert, J. S., Olson, J. L., Morhart, M. J., Dawson, M. R., Marasco, P. D., Kuiken, T. A., et al. (2013). Novel targeted sensory reinnervation technique to restore functional hand sensation after transhumeral amputation. IEEE Trans. Neural Syst. Rehab. Eng. 22, 765–773.
Heo, P., Gu, G. M., Lee, S.-j., Rhee, K., and Kim, J. (2012). Current hand exoskeleton technologies for rehabilitation and assistive engineering. Int. J. Precis. Eng. Manuf. 13, 807–824. doi: 10.1007/s12541-012-0107-2
Hsieh, Y.-w, Wu, C.-y., Lin, K.-c., Yao, G., Wu, K.-y, and Chang, Y.-j (2012). Dose–response relationship of robot-assisted stroke motor rehabilitation: the impact of initial motor status. Stroke 43, 2729–2734. doi: 10.1161/strokeaha.112.658807
Hubbard, I. J., Parsons, M. W., Neilson, C., and Carey, L. M. (2009). Task−specific training: evidence for and translation to clinical practice. Occup. Ther. Int. 16, 175–189. doi: 10.1002/oti.275
Hughes, A.-M., Burridge, J. H., Demain, S. H., Ellis-Hill, C., Meagher, C., Tedesco-Triccas, L., et al. (2014). Translation of evidence-based assistive technologies into stroke rehabilitation: users’ perceptions of the barriers and opportunities. BMC Health Serv. Res. 14:124. doi: 10.1186/1472-6963-14-124
Jeannerod, M. (2009). The sense of agency and its disturbances in schizophrenia: a reappraisal. Exp. Brain Res. 192:527. doi: 10.1007/s00221-008-1533-3
Kang, S. Y., Im, C. H., Shim, M., Nahab, F. B., Park, J., Kim, D. W., et al. (2015). Brain networks responsible for sense of agency: an EEG study. PLoS One 10:e0135261. doi: 10.1371/journal.pone.0135261
Kazemi, M., Valois, J.-S., Bagnell, J. A., and Pollard, N. (2012). Robust Object Grasping Using Force Compliant Motion Primitives,” Robotics: Science and Systems (RSS). Sydney, NSW: MIT Press, 177–185.
Khalighinejad, N., Kunnumpurath, A., Bertini, C., Ladavas, E., and Haggard, P. (2017). Subliminal modulation of voluntary action experience: a neuropsychological investigation. Cortex 90, 58–70. doi: 10.1016/j.cortex.2017.02.012
Kilteni, K., Groten, R., and Slater, M. (2012). The sense of embodiment in virtual reality. Presence Teleoperators Vir. Environ. 21, 373–387. doi: 10.1162/pres_a_00124
Kim, B.-H., Yi, B.-J., Oh, S.-R., and Suh, I. H. (2003). Independent finger and independent joint-based compliance control of multifingered robot hands. IEEE Trans. Robot. Autom. 19, 185–199. doi: 10.1109/tra.2003.808846
Le Goff, K., Rey, A., Haggard, P., Oullier, O., and Berberian, B. (2018). Agency modulates interactions with automation technologies. Ergonomics 61, 1282–1297. doi: 10.1080/00140139.2018.1468493
Lotte, F., Faller, J., Guger, C., Renard, Y., Pfurtscheller, G., Lécuyer, A., et al. (2012). “Combining BCI with virtual reality: towards new applications and improved BCI,” in Towards Practical Brain-Computer Interfaces, eds B. Allison, S. Dunne, R. Leeb, J Del R. Millán, and A. Nijholt (Heidelberg: Springer), 197–220. doi: 10.1007/978-3-642-29746-5_10
Lucas, L., DiCicco, M., and Matsuoka, Y. (2004). An EMG-controlled hand exoskeleton for natural pinching. J. Robot. Mechatronics 16, 482–488. doi: 10.20965/jrm.2004.p0482
Ma, V. Y., Chan, L., and Carruthers, K. J. (2014). Incidence, prevalence, costs, and impact on disability of common conditions requiring rehabilitation in the United States: stroke, spinal cord injury, traumatic brain injury, multiple sclerosis, osteoarthritis, rheumatoid arthritis, limb loss, and back pain. Arch. Phys. Med. Rehab. 95, 986–995. e1.
Marasco, P. D., Hebert, J. S., Sensinger, J. W., Shell, C. E., Schofield, J. S., Thumser, Z. C., et al. (2018). Illusory movement perception improves motor control for prosthetic hands. Sci. Transl. Med. 10:eaao6990. doi: 10.1126/scitranslmed.aao6990
Michelman, P., and Allen, P. (1993). “Compliant manipulation with a dextrous robot hand,” in Proceedings of the 1993 IEEE International Conference on Robotics and Automation, (Atlanta, GA: IEEE), 711–716.
Miele, D. B., Wager, T. D., Mitchell, J. P., and Metcalfe, J. (2011). Dissociating neural correlates of action monitoring and metacognition of agency. J. Cogn. Neurosci. 23, 3620–3636. doi: 10.1162/jocn_a_00052
Moore, J., and Haggard, P. (2008). Awareness of action: inference and prediction. Conscious. Cogn. 17, 136–144. doi: 10.1016/j.concog.2006.12.004
Moore, J. W. (2016). What is the sense of agency and why does it matter? Front. Psychol. 7:1272. doi: 10.3389/fpsyg.2016.01272
Moore, J. W., and Fletcher, P. C. (2012). Sense of agency in health and disease: a review of cue integration approaches. Conscious. Cogn. 21, 59–68. doi: 10.1016/j.concog.2011.08.010
Moore, J. W., Middleton, D., Haggard, P., and Fletcher, P. C. (2012). Exploring implicit and explicit aspects of sense of agency. Conscious. Cogn. 21, 1748–1753. doi: 10.1016/j.concog.2012.10.005
Moore, J. W., and Obhi, S. S. (2012). Intentional binding and the sense of agency: a review. Conscious. Cogn. 21, 546–561. doi: 10.1016/j.concog.2011.12.002
Moore, J. W., Wegner, D. M., and Haggard, P. (2009). Modulating the sense of agency with external cues. Conscious. Cogn. 18, 1056–1064. doi: 10.1016/j.concog.2009.05.004
Nataraj, R. (2017). “Optimizing user integration for individualized rehabilitation,” in Biomimetic Prosthetics, ed. R. Vinjamuri (London: IntechOpen).
Nataraj, R., Audu, M. L., Kirsch, R. F., and Triolo, R. J. (2010). Comprehensive joint feedback control for standing by functional neuromuscular stimulation—A simulation study. IEEE Trans. Neural Syst. Rehab. Eng. 18, 646–657. doi: 10.1109/tnsre.2010.2083693
Nataraj, R., Audu, M. L., and Li, Z.-M. (2015). Digit mechanics in relation to endpoint compliance during precision pinch. J. Biomech. 48, 672–680. doi: 10.1016/j.jbiomech.2014.12.040
Nataraj, R., Audu, M. L., and Triolo, R. J. (2012b). Center of mass acceleration feedback control of standing balance by functional neuromuscular stimulation against external postural perturbations. IEEE Trans. Biomed. Eng. 60, 10–19. doi: 10.1109/tbme.2012.2218601
Nataraj, R., Audu, M. L., and Triolo, R. J. (2012a). Center of mass acceleration feedback control of functional neuromuscular stimulation for standing in the presence of internal postural perturbations. J. Rehab. Res. Dev. 49:889. doi: 10.1682/jrrd.2011.07.0127
Nataraj, R., Audu, M. L., and Triolo, R. J. (2014). Modified Newton-Raphson method to tune feedback gains of control system for standing by functional neuromuscular stimulation following spinal cord injury. Appl. Bion. Biomech. 11, 169–174. doi: 10.1155/2014/634509
Nataraj, R., Hollinger, D., Liu, M., and Shah, A. (2020c). Disproportionate positive feedback facilitates sense of agency and performance for a reaching movement task with a virtual hand. PLoS One 15:e0233175. doi: 10.1371/journal.pone.0233175
Nataraj, R., Sanford, S., Liu, M., Walsh, K., Wilder, S., Santo, A., et al. (2020b). “Cognitive and physiological intent for the adaptation of motor prostheses,” in Advances in Motor Neuroprostheses, ed. R. Vinjamuri (Cham: Springer), 123. doi: 10.1007/978-3-030-38740-2_8
Nataraj, R., Sanford, S., Shah, A., and Liu, M. (2020a). Agency and performance of reach-to-grasp with modified control of a virtual hand: implications for rehabilitation. Front. Hum. Neurosci. 14:126. doi: 10.3389/fnhum.2020.00126
Nataraj, R., Sanford, S., Shah, A., and Liu, M. (2020d). Agency and performance of reach-to-grasp with modified control of a virtual hand: implications for rehabilitation. Front. Hum. Neurosci. 14:126.
Nataraj, R., and van den Bogert, A. J. (2017). Simulation analysis of linear quadratic regulator control of sagittal-plane human walking—implications for exoskeletons. J. Biomech. Eng. 139:101009.
Neptune, R. R. (1999). Optimization algorithm performance in determining optimal controls in human movement analyses. J. Biomech. Eng. 121, 249–252. doi: 10.1115/1.2835111
Phillips, B., and Zhao, H. (1993). Predictors of assistive technology abandonment. Assist. Technol. 5, 36–45. doi: 10.1080/10400435.1993.10132205
Prattichizzo, D., Malvezzi, M., Gabiccini, M., and Bicchi, A. (2012). On the manipulability ellipsoids of underactuated robotic hands with compliance. Robot. Auton. Syst. 60, 337–346. doi: 10.1016/j.robot.2011.07.014
Priplata, A., Niemi, J., Salen, M., Harry, J., Lipsitz, L. A., and Collins, J. (2002). Noise-enhanced human balance control. Phys. Rev. Lett. 89:238101.
Ritterband-Rosenbaum, A., Christensen, M. S., and Nielsen, J. B. (2012). Twenty weeks of computer-training improves sense of agency in children with spastic cerebral palsy. Res. Dev. Disabil. 33, 1227–1234. doi: 10.1016/j.ridd.2012.02.019
Ronsse, R., Vitiello, N., Lenzi, T., Van Den Kieboom, J., Carrozza, M. C., and Ijspeert, A. J. (2011). Human–robot synchrony: flexible assistance using adaptive oscillators. IEEE Trans. Biomed. Eng. 58, 1001–1012. doi: 10.1109/tbme.2010.2089629
Ryu, J., and Torres, E. B. (2018). Characterization of sensory-motor behavior under cognitive load using a new statistical platform for studies of embodied cognition. Front. Hum. Neurosci. 12:116. doi: 10.3389/fnhum.2018.00116
Saito, N., Takahata, K., Murai, T., and Takahashi, H. (2015). Discrepancy between explicit judgement of agency and implicit feeling of agency: Implications for sense of agency and its disorders. Conscious. Cogn. 37, 1–7. doi: 10.1016/j.concog.2015.07.011
Saleh, S., Fluet, G., Qiu, Q., Merians, A., Adamovich, S. V., and Tunik, E. (2017). Neural patterns of reorganization after intensive robot-assisted virtual reality therapy and repetitive task practice in patients with chronic stroke. Front. Neurol. 8:452. doi: 10.3389/fneur.2017.00452
Sanford, S., Liu, M., Selvaggi, T., and Nataraj, R. (2020). Effects of visual feedback complexity on the performance of a movement task for rehabilitation. J. Motor Behav. 1–15. doi: 10.1080/00222895.2020.1770670
Schofield, J. S., Shell, C. E., Thumser, Z. C., Beckler, D. T., Nataraj, R., and Marasco, P. D. (2019). Characterization of the sense of agency over the actions of neural-machine interface-operated prostheses. JoVE J. Vis. Exp. 143:e58702.
Shepherd, R. B. (2001). Exercise and training to optimize functional motor performance in stroke: driving neural reorganization? Neural Plast. 8, 121–129. doi: 10.1155/np.2001.121
Shin, J.-H., Ryu, H., and Jang, S. H. (2014). A task-specific interactive game-based virtual reality rehabilitation system for patients with stroke: a usability test and two clinical experiments. J. Neuroeng. Rehab. 11:32. doi: 10.1186/1743-0003-11-32
Stevenson, T., Thalman, L., Christie, H., and Poluha, W. (2012). Constraint-induced movement therapy compared to dose-matched interventions for upper-limb dysfunction in adult survivors of stroke: a systematic review with meta-analysis. Physiother. Can. 64, 397–413. doi: 10.3138/ptc.2011-24
Sutcliffe, A. G., and Kaur, K. D. (2000). Evaluating the usability of virtual reality user interfaces. Behav. Inform. Technol. 19, 415–426. doi: 10.1080/014492900750052679
Taylor, D. M., Tillery, S. I. H., and Schwartz, A. B. (2002). Direct cortical control of 3D neuroprosthetic devices. Science 296, 1829–1832. doi: 10.1126/science.1070291
Tsakiris, M., Prabhu, G., and Haggard, P. (2006). Having a body versus moving your body: how agency structures body-ownership. Conscious. Cogn. 15, 423–432. doi: 10.1016/j.concog.2005.09.004
Vasilakos, K., and Beuter, A. (1993). Effects of noise on a delayed visual feedback system. J. Theor. Biol. 165, 389–407. doi: 10.1006/jtbi.1993.1196
Velazquez, H. F., Walter, R. S., Gruttadauria, M. K., Huelskamp, T. J., Gara, D. P., Lynch, W. M., et al. (2008). Virtual reality system including personalized virtual environments Google Patents US20080162261A1.
Wege, A., Kondak, K., and Hommel, G. (2005). “Mechanical design and motion control of a hand exoskeleton for rehabilitation,” in Proceedings of the IEEE International Conference Mechatronics and Automation, 2005, (Niagara Falls, Ont: IEEE), 155–159.
Wegner, D. M. (2003). The mind’s best trick: how we experience conscious will. Trends Cogn. Sci. 7, 65–69. doi: 10.1016/s1364-6613(03)00002-0
Wei, Y., Bajaj, P., Scheidt, R., and Patton, J. (2005). “Visual error augmentation for enhancing motor learning and rehabilitative relearning,” in Proceedings of the 9th International Conference on Rehabilitation Robotics, ICORR 2005, (Chicago, IL: IEEE), 505–510.
Wen, W., Brann, E., Di Costa, S., and Haggard, P. (2018). Enhanced perceptual processing of self-generated motion: evidence from steady-state visual evoked potentials. Neuroimage 175, 438–448. doi: 10.1016/j.neuroimage.2018.04.019
Xiang, Y., Arora, J. S., and Abdel-Malek, K. (2010). Physics-based modeling and simulation of human walking: a review of optimization-based and other approaches. Struct. Multidiscipl. Optim. 42, 1–23. doi: 10.1007/s00158-010-0496-8
Keywords: cognitive agency, hand grasp force, movement rehabilitation, visual feedback, precision pinch
Citation: Nataraj R and Sanford S (2021) Control Modification of Grasp Force Covaries Agency and Performance on Rigid and Compliant Surfaces. Front. Bioeng. Biotechnol. 8:574006. doi: 10.3389/fbioe.2020.574006
Received: 18 June 2020; Accepted: 16 December 2020;
Published: 13 January 2021.
Edited by:
Borja Sañudo, University of Sevilla, SpainReviewed by:
Simone Tassani, Pompeu Fabra University, SpainMassimiliano Zingales, University of Palermo, Italy
Copyright © 2021 Nataraj and Sanford. This is an open-access article distributed under the terms of the Creative Commons Attribution License (CC BY). The use, distribution or reproduction in other forums is permitted, provided the original author(s) and the copyright owner(s) are credited and that the original publication in this journal is cited, in accordance with accepted academic practice. No use, distribution or reproduction is permitted which does not comply with these terms.
*Correspondence: Raviraj Nataraj, cm5hdGFyYWpAc3RldmVucy5lZHU=