- 1School of Biosciences and Technology, Vellore Institute of Technology, Vellore, India
- 2Department of Biomedical Sciences, College of Health and Sciences, QU Health, Qatar University, Doha, Qatar
Systemic lupus erythematosus (SLE) is an autoimmune inflammatory disorder that is clinically complex and has increased production of autoantibodies. Via emerging technologies, researchers have identified genetic variants, expression profiling of genes, animal models, and epigenetic findings that have paved the way for a better understanding of the molecular and genetic mechanisms of SLE. Our current study aimed to illustrate the essential genes and molecular pathways that are potentially involved in the pathogenesis of SLE. This study incorporates the gene expression profiling data of the microarray dataset GSE30153 from the Gene Expression Omnibus (GEO) database, and differentially expressed genes (DEGs) between the B-cell transcriptomes of SLE patients and healthy controls were screened using the GEO2R web tool. The identified DEGs were subjected to STRING analysis and Cytoscape to explore the protein–protein interaction (PPI) networks between them. The MCODE (Molecular Complex Detection) plugin of Cytoscape was used to screen the cluster subnetworks that are highly interlinked between the DEGs. Subsequently, the clustered DEGs were subjected to functional annotation with ClueGO/CluePedia to identify the significant pathways that were enriched. For integrative analysis, we used GeneGo MetacoreTM, a Cortellis Solution software, to exhibit the Gene Ontology (GO) and enriched pathways between the datasets. Our study identified 4 upregulated and 13 downregulated genes. Analysis of GO and functional enrichment using ClueGO revealed the pathways that were statistically significant, including pathways involving T-cell costimulation, lymphocyte costimulation, negative regulation of vascular permeability, and B-cell receptor signaling. The DEGs were mainly enriched in metabolic networks such as the phosphatidylinositol-3,4,5-triphosphate pathway and the carnitine pathway. Additionally, potentially enriched pathways, such as the signaling pathways induced by oxidative stress and reactive oxygen species (ROS), chemotaxis and lysophosphatidic acid signaling induced via G protein-coupled receptors (GPCRs), and the androgen receptor activation pathway, were identified from the DEGs that were mainly associated with the immune system. Four genes (EGR1, CD38, CAV1, and AKT1) were identified to be strongly associated with SLE. Our integrative analysis using a multitude of bioinformatics tools might promote an understanding of the dysregulated pathways that are associated with SLE development and progression. The four DEGs in SLE patients might shed light on the pathogenesis of SLE and might serve as potential biomarkers in early diagnosis and as therapeutic targets for SLE.
Introduction
Systemic lupus erythematosus (SLE), also known as lupus, is a rare systemic autoimmune disease that mostly affects middle-aged women, mainly of Asian, African, American, and Hispanic origin (Costa-Reis and Sullivan, 2013; Cui et al., 2013; Gurevitz et al., 2013). SLE affects an estimated 5 million people across the world, with an incidence of 1–10 per 100,000 person-years (Pons-Estel et al., 2010). SLE is characterized by a wide range of different autoantibodies, deposition of immune complexes, and immune system infiltration and inflammation within damaged organs. SLE autoantibodies invade the patient’s kidneys, heart, skin, joints, and brain, leading to various typical clinical symptoms. The most common clinical symptoms of lupus are rash, arthritis, and fatigue. Severe complications of SLE lead to nephritis, anemia, neurological symptoms, and thrombocytopenia, eventually leading to severe morbidity and mortality.
SLE is characterized by its clinical heterogeneity, with a wide range of clinical manifestations reflecting its complex etiopathogenesis (Tan et al., 1982). The clinical heterogeneity of SLE highlights the contribution of genetic and environmental factors to the susceptibility to the disease (Prokunina and Alarcon-Riquelme, 2004; Harley et al., 2009; Yang and Lau, 2015; Dang et al., 2016; Wang et al., 2017). To date, the reason for phenotypic variation in SLE is unknown. Understanding the molecular mechanisms behind the pathogenesis of SLE phenotypes could help in developing more efficient therapeutic approaches and preventive strategies.
With the extensive use of gene detection methods, high-throughput sequencing and extensive microarray data profiling studies on SLE have been conducted, and several differentially expressed genes (DEGs) and cellular pathways in SLE have been identified (Borrebaeck et al., 2014; Zhu et al., 2015). Nevertheless, until now, no particular gene has been recognized to act as a potential marker for the diagnosis of SLE. In addition, a large amount of data obtained from microarray technology and high-throughput sequencing have not been fully used. Ducreux et al. (2016) collected blood samples from SLE patients and healthy volunteers to identify differentially expressed genes (Ducreux et al., 2016). However, the interactions among differentially expressed genes and key genes involved in the signaling pathways of SLE remain to be elucidated. In addition, previous studies of genetic factors primarily focused on single genes; nevertheless, interactions among multiple genes may result in the multisystem invasion characteristics observed in SLE (Smith et al., 2017). Remarkably, studies have shown that disease−associated gene expression networks have a potential role in the immune response, which highlights their mechanism and therapeutic value for SLE (Deng and Tsao, 2010; Bentham et al., 2015).
Integrating and reanalyzing the data using bioinformatics methods may help in identifying gene regulatory pathways, essential genes, and their associated networks in SLE disease, which can provide new and valuable ideas for understanding the molecular mechanisms and identifying reliable diagnostic and therapeutic targets of SLE. Therefore, in this study, we first conducted a comprehensive collection of genes associated with SLE from the GEO dataset with ID GSE30153. Then, we performed a bioinformatics analysis of these genes with the MCODE (Molecular Complex Detection), GeneGo, and ClueGO tools. To further explore the pathogenesis of SLE in a more specific manner, functions and pathways identified by the modules were used to indicate the biological processes and biochemical pathways related to the immune system. Finally, the genes potentially associated with arthritis, pleurisy, and myocarditis, which are the common complications of SLE, were compared with SLE-related genes to identify common genes that participated in the development of SLE. To interpret the biological relevance of these changes in gene expression, we analyzed the microarray data via an integrated bioinformatic analysis expanding on traditional microarray analysis methods, namely, Gene Ontology (GO) and pathway analysis, thereby allowing the construction of interaction networks that might identify novel prognostic markers and therapeutic targets.
Materials and Methods
Acquisition of Array Data and Processing
Gene expression profiling data from microarray array analysis of the GSE30153 dataset were downloaded from the NCBI GEO database (Gene Expression Omnibus database)1. The database accommodates gene expression datasets from a variety of experiments, such as DNA-seq, ChIPs, RNA-seq, microarray, and high-throughput hybridization array (Edgar et al., 2002; Barrett et al., 2013). GSE30153 contains 26 samples, including 17 patients with SLE and 9 healthy controls of human sorted B-cells obtained by using the platform GPL570 (HG-U133_Plus_2) Affymetrix Human Genome U133 Plus 2.0 Array (Garaud et al., 2011). The downloaded gene expression profiling data are freely available in the public database, and there were no human or animal experiments conducted by any of the authors in this study.
Preprocessing of Data and DEG Identification
Using the robust multiarray standard model, the initial information from the dataset was subjected to quantile normalization, background correction, and log transition (Irizarry et al., 2003). Preprocessing included changing to gene symbols from probe IDs using the Gene ID converter from Entrez (Alibés et al., 2007). The statistical online tool GEO2R uses the R/Bioconductor, and limma package v3.26.8 was used to screen the raw gene expression data (Smyth, 2005; Barrett et al., 2013; Ritchie et al., 2015). We performed a Benjamini–Hochberg test (to determine the false discovery rate) and T-tests to compute the false discovery rate (FDR) and p-values to identify the DEGs between SLE patients and healthy control human sorted B-cells (Benjamini and Hochberg, 1995; Aubert et al., 2004). We set the primary criteria of | log (2 fold change) | > 1 and p < 0.05 to obtain significant DEGs from the dataset, whereas cutoffs of log2FC ≥ 1 and log2FC ≤ −1 were used to denote upregulated and downregulated DEGs, respectively. For high-throughput sequencing, a logarithm to base 2 is widely used and in the initial scaling, the doubling is equivalent to a log2FC of 1 (Love et al., 2014). A volcano plot was constructed using a web-based tool2. The resulting DEGs were used for further analysis.
Constructing PPI Networks
To assess the relationships between the DEGs from the GSE30153 dataset, we constructed a protein–protein interaction (PPI) network by using Search Tool for the Retrieval of Interacting Genes (STRING v11.0)3 (Szklarczyk et al., 2017, 2019). The cutoff criterion was set to a high confident interaction score of ≥0.7 to eliminate inconsistent PPIs from the dataset. We then incorporated the results from the STRING database into Cytoscape software (v3.7.2)4 to envisage the PPIs within the statistically relevant DEGs (Shannon et al., 2003). The MCODE plugin from Cytoscape was utilized to identify the interconnected regions or clusters from the PPI network. The cluster finding parameters were adopted, such as a degree cutoff of 2, a node score cutoff of 0.2, a kappa score (K-core) of 5, and a max depth of 100, which limits the cluster size for coexpressing networks (Bader and Hogue, 2003). The top clusters from MCODE were subjected to ClueGO v2.5.5/CluePedia v1.5.5 analysis to obtain comprehensive GO and pathway results from the PPI network. ClueGO combines GO and pathway analyses from KEGG and BioCarta and provides a fundamentally structured GO or pathway network from the PPI network (Bindea et al., 2009).
Metacore GeneGo Analysis of DEGs
Metacore, a Cortellis Solution software (Clarivate Analytics, London, United Kingdom)5, was used to perform curated pathway enrichment analysis and GO analysis. GeneGo facilitates the rapid assessment of metabolic pathways, protein biological networks, and pathway maps from high-throughput experimental data (MetaCoreLogin | Clarivate Analytics). Based on a significance threshold of p < 0.05, a pictorial representation of the molecular interactions of DEGs from the study groups is generated. Determination of a hypergeometric p-value enables the estimation of the chance that an intersection between DEGs and ontological elements is random. An FDR < 0.05 was used as a criterion to calculate if statistically significant DEGs constituted a processor pathway.
Results
Identification of DEGs From the Dataset
Our study contained the gene expression profiles of the GSE30153 dataset from the GEO database, which were submitted by Garaud et al. (2011) based on analysis with the GPL570 platform (Affymetrix Human Genome U133 Plus 2.0 Array) (Garaud et al., 2011). The dataset encompasses 26 samples, including 17 patients with SLE and 9 healthy controls (Table 1). By utilizing the GEO2R online tool, we obtained the differentially expressed genes (DEGs) from the GSE30153 dataset by comparing the SLE samples with control samples. By calculating p-values and | log2FC | values, the top 250 DEGs were identified. A volcano plot was constructed using the Rstudio web server ShinyVolcanoPlot to identify DEGs by comparing the SLE and control groups from the dataset. The volcano plot in Figure 1 depicts all the DEGs with a log2FC against the – log10 (p-value) between the two groups. With cutoffs of p < 0.05 and log2FC ≥ 1.0 or ≤−1, we found 4 and 13 genes that were upregulated and downregulated, respectively, between the two groups (Table 2). The genes that were differentially expressed between the two groups are shown in Supplementary Table S1.
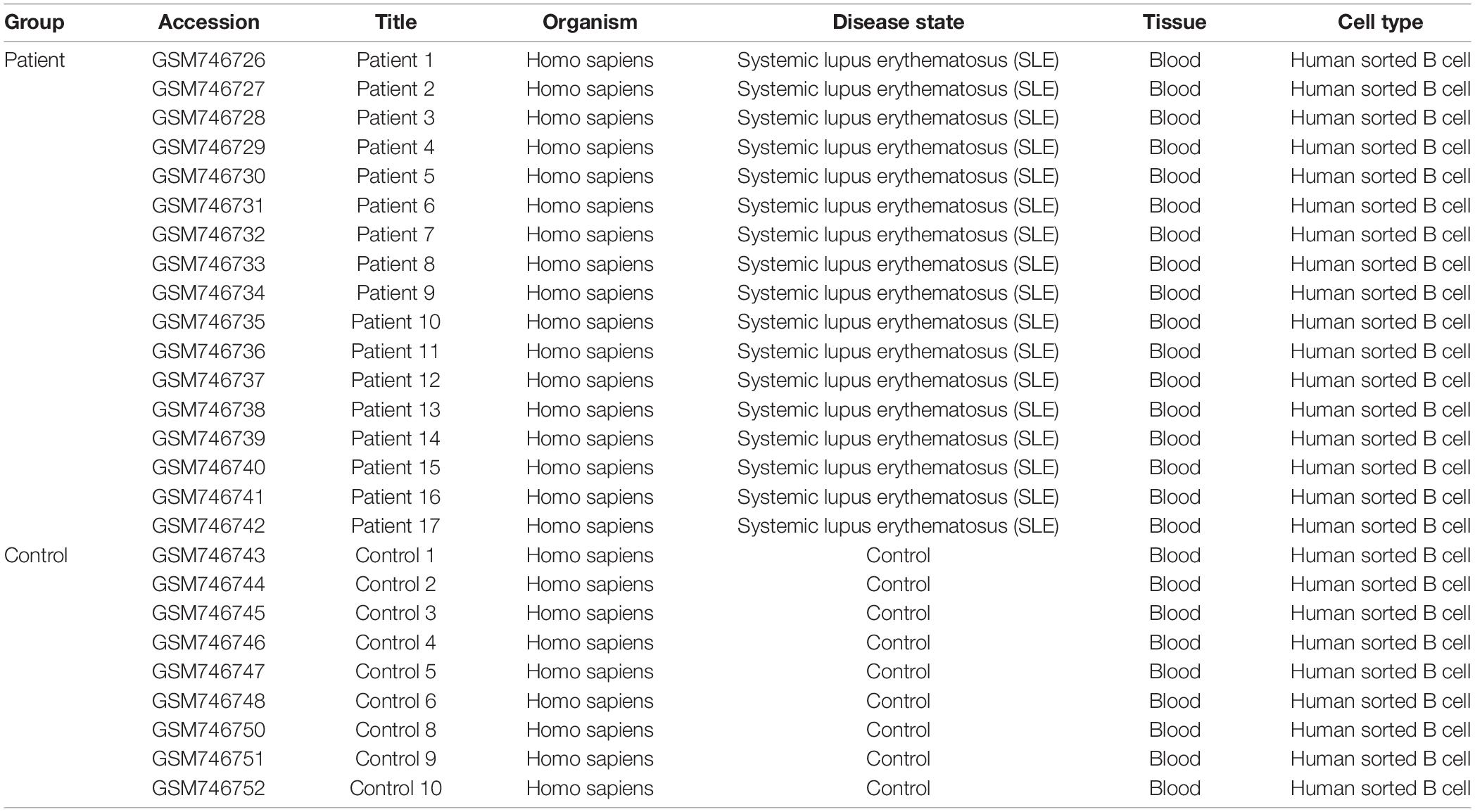
Table 1. The primary characteristics of 26 studies in GSE30153 procured from the Gene Omnibus Expression database.
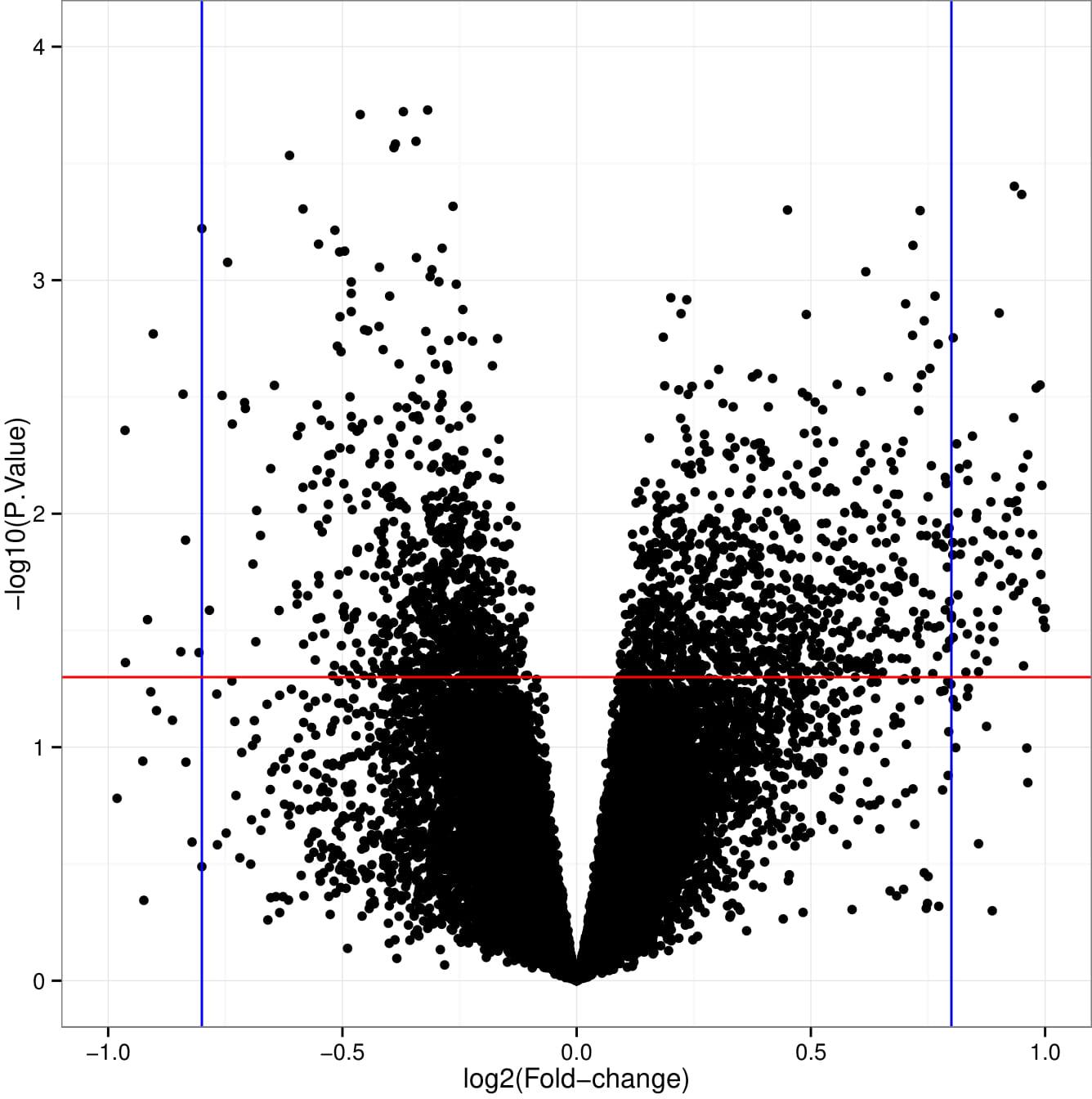
Figure 1. Pictorial representation of volcano plot for differentially expressed genes (DEGs) in systemic lupus erythematosus (SLE) compared to controls from the GSE30153 dataset. The X-axis represents Log2FC, large magnitude fold changes; Y-axis represents −log10 of a p-value, high statistical significance. Each black dot represents one gene. Black dots above red and beside blue line (left-sided and right-sided) are log2FC ≥ 1 and p-value <0.05, representing SLE related DEGs.
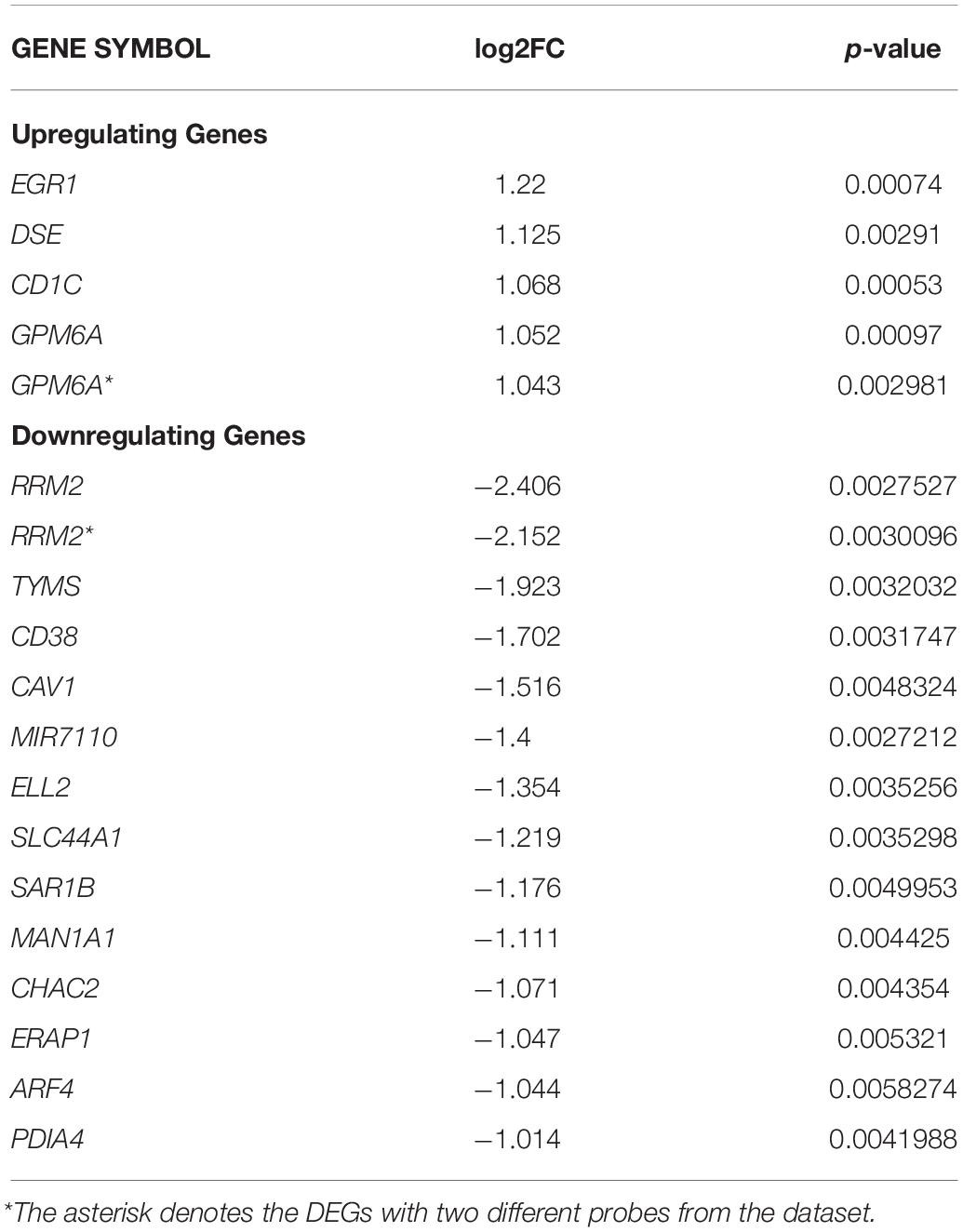
Table 2. Significantly upregulated and downregulated DEGs between two groups from GSE30153 dataset are tabulated.
Screening of Module and Construction of Interlinking PPI Network
To assess the protein–protein connections among the DEGs, we used the STRING tool to compute the protein interactions and plotted them using Cytoscape v3.7.2. Figure 2 depicts the PPI network with 103 nodes and 201 edges. The DEGs are represented as nodes, and the edges are interactions between the DEGs. A combined node score of >0.4 was considered to be significant. MCODE plugin v1.5.1 from Cytoscape was utilized to identify the densely interlinked regions within the protein network. As a result, we obtained the top two significant clusters from the DEGs protein network with MCODE scores of 5.043 and 3.625. A graphical representation of these clusters is shown in Figures 3A,B. The subnetworks, scores, number of nodes and edges, and node IDs are tabulated in Table 3.
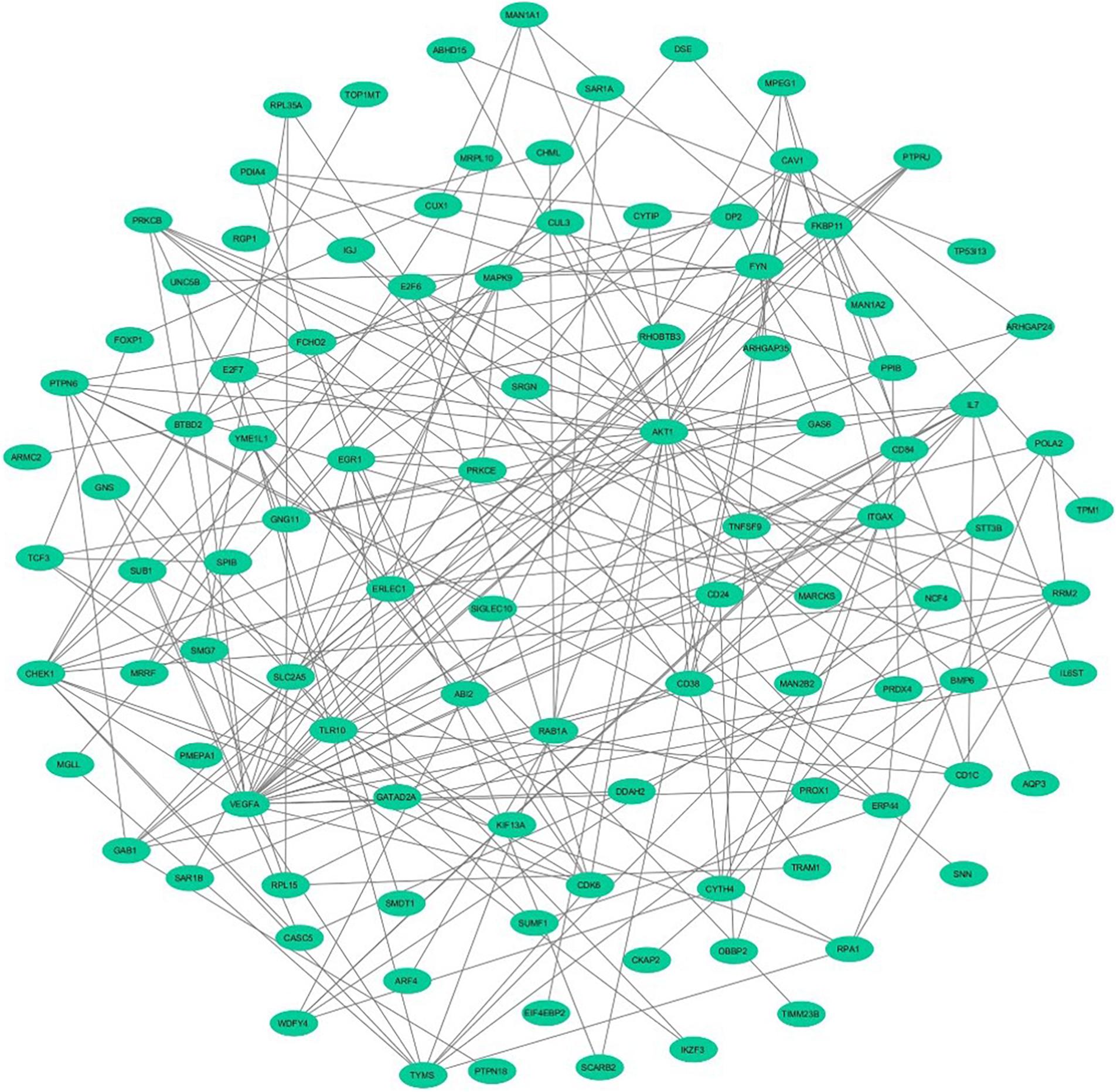
Figure 2. The network demonstrates the protein–protein interaction between the DEGs identified from GSE30153 using Cytoscape. The nodes represented as ellipse (robin’s blue) and edges as lines (gray).
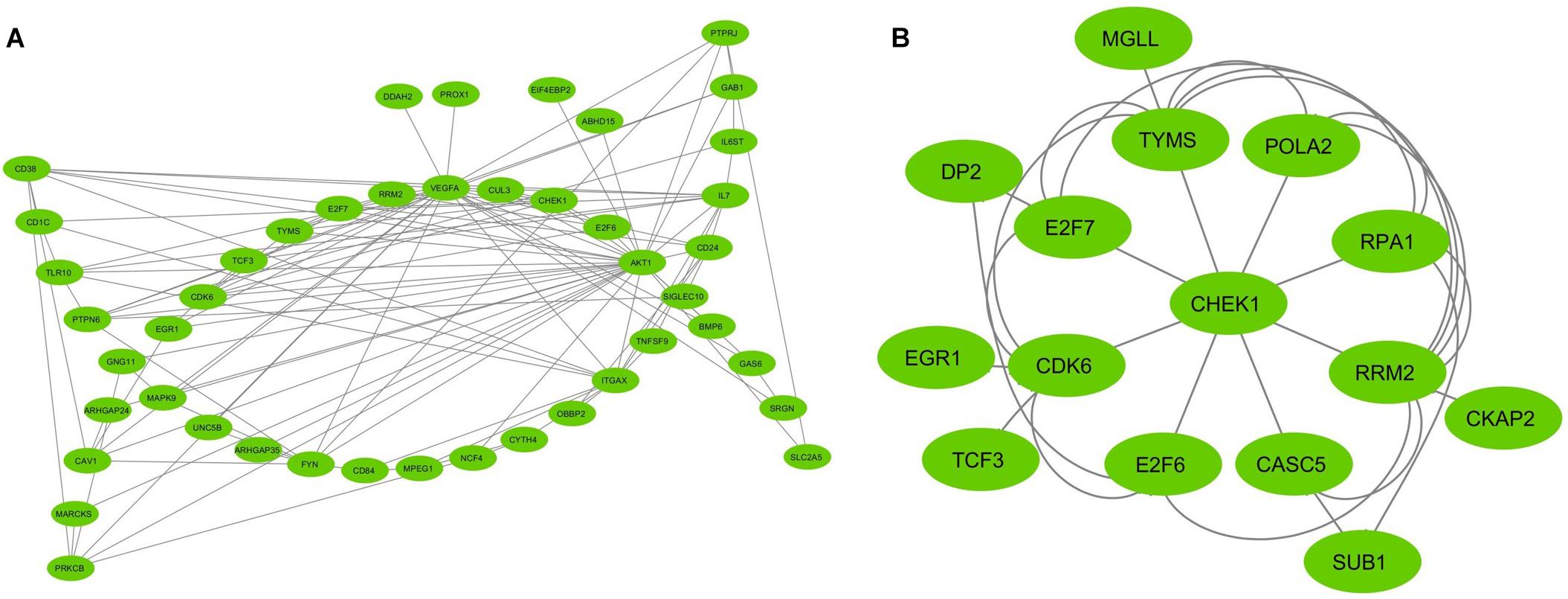
Figure 3. The MCODE (Molecular Complex Detection) plugin from Cytoscape analyzed the top two clusters derived from the network of interactions between protein and protein. (A) Cluster 1; (B) Cluster 2. The MCODE cluster score > 3. The nodes represented as ellipse (green) and edges as lines (gray).
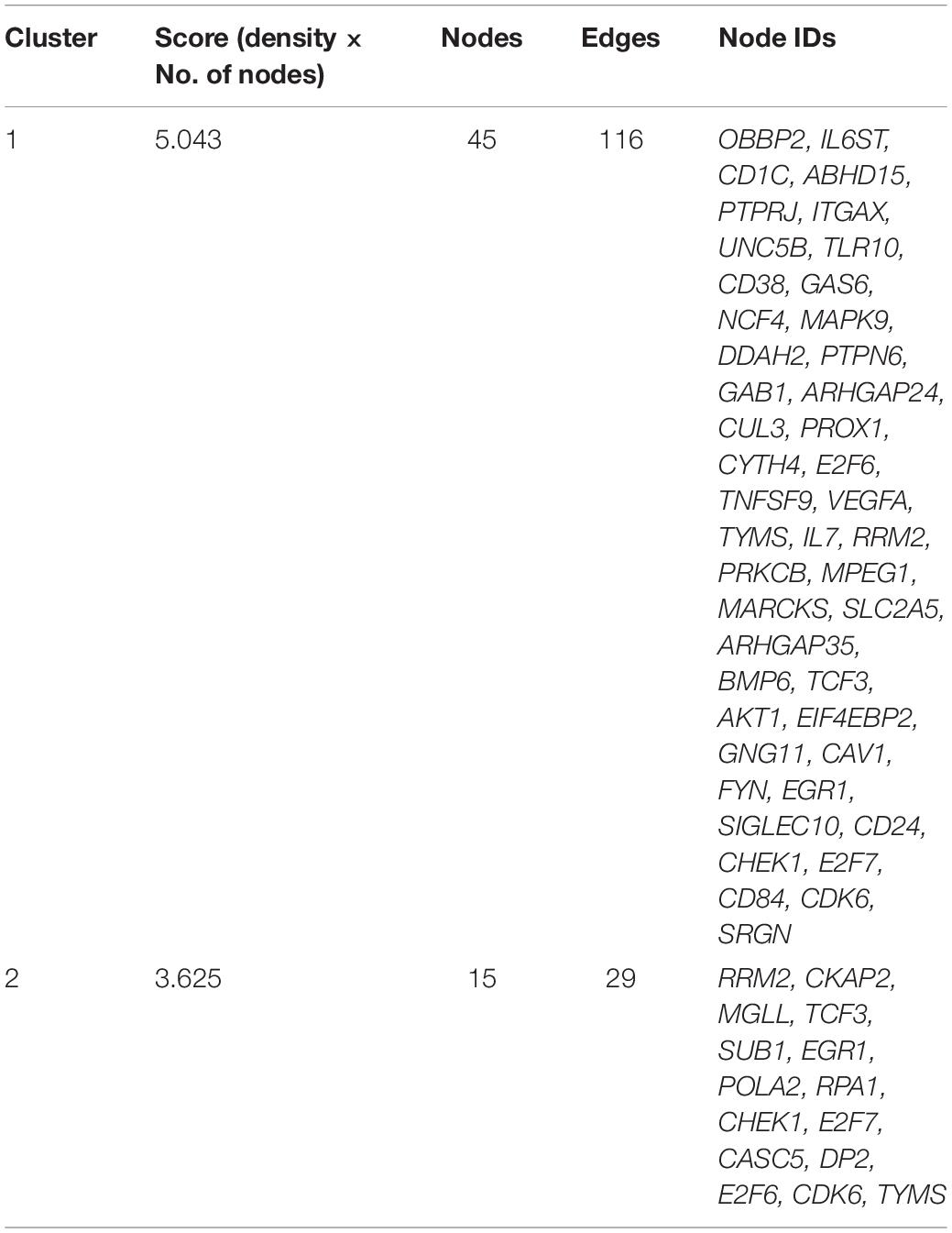
Table 3. The interconnected regions are clustered from the GSE30153 dataset using MCODE plugin in Cytoscape.
Enrichment Analysis by ClueGO
The top two subnetworks from MCODE were used as an input for analyzing the functional enrichment of PPI subnetworks using the ClueGO/CluePedia plugin from Cytoscape. In a biologically clustered subnetwork, ClueGO helps to visualize the biological terms of broad gene clusters. The subnetwork enrichment analyses of MCODE cluster 1 and cluster 2 are depicted in Figures 4A,B. For functional enrichment analysis, we set the statistical options based on a two-sided hypergeometric test with a Benjamini–Hochberg correction, p ≤ 0.05, and kappa scores ≥ 0.4 as criteria. The DEGs from cluster 1 were shown to be enriched mostly in T-cell costimulation (GO: 0031295), lymphocyte costimulation (GO: 0031294), negative regulation of vascular permeability (GO: 0043116), the metaphase/anaphase transition of the mitotic cell cycle (GO: 0007091), regulation of the transcription involved in the G1/S transition of the mitotic cell cycle (GO: 0000083), negative regulation of signal transduction in the absence of ligand (GO: 1901099), and KEGG pathways such as hematopoietic cell lineage (KEGG: 04640), B-cell receptor signaling pathway (KEGG: 04662), ErbB signaling pathway (KEGG: 04012), and AGE-RAGE (advanced glycation end products and receptor for AGE) signaling pathway in diabetic complications (Figure 4A). The DEGs from cluster 2 were mainly enriched in the regulation of the transcription involved in the G1/S transition of the mitotic cell cycle (GO: 0000083), the negative regulation of the G0 and G1 transitions (GO: 0070317), and the p53 signaling pathway (KEGG: 04115) (Figure 4B). The pathways that were activated in the enrichment analysis were highly related to B-cell pathophysiology, resulting in events associated with the immune system, vasculopathy, and kidney.
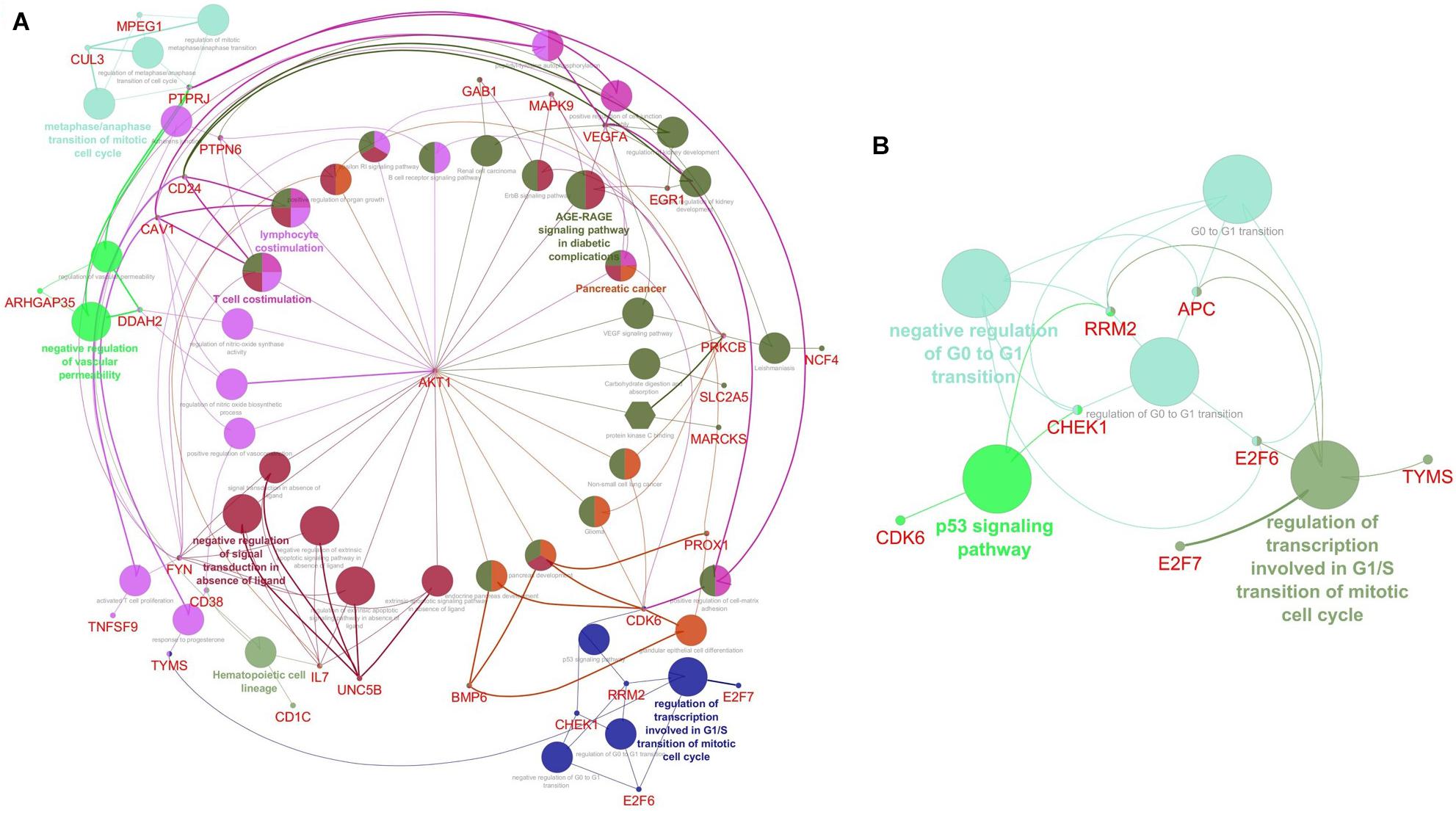
Figure 4. Visualization of Gene Ontology (GO) enrichment profiles from DEGs using Cytoscape software based on network analysis of ClueGO/CluePedia inferred from MCODE cluster 1 (A) and cluster 2 (B). The plugin provides a combined enrichment analysis of clusters, including the GO biological process, molecular function, and pathway from KEGG. The GO term/pathway network connectivity defined by edges and functional clusters on genes shared between terms (kappa score ≥ 0.4) and displaying pathways only with p≤ 0.05. The size of the node indicates the p-value. The color code of nodes represents the functional group that they belong to. The most important functional terms specify the pathway names within each class are indicated in bold colored characters. (A) The network enrichment analysis of cluster 1. Each node constitutes a precise term for cluster 1; (B) The network enrichment analysis of cluster 2. Each node constitutes a precise term for cluster 2.
MetacoreTM GeneGo for Enrichment Analysis of DEGs
Further functional enrichment analysis was carried out using MetacoreTM GeneGo software from Clarivate Analytics to comprehensively dissect the pathways associated with the DEGs. Using the functional ontology feature in GeneGo, the IDs of potential genes that were involved in the target pathways were identified. Based on hypergeometric p-values, the probability that the intersection of a gene set and associated ontological objects was random was evaluated. A decreased p-value indicated that the entity would be more significant to the DEGs, suggesting a better score. The functional enrichment analysis of the DEGs defined the top 10 metabolic networks, and canonical pathway maps are depicted in Figures 5A,B. For each classification, the significant statistical data rely on a low p-value. The pathway maps with the lowest p-value are shown in Figures 6A–C. These are the top-scoring signaling pathways based on the gene enrichment distribution, which emphasizes that the DEGs from human sorted B-cells are triggered via oxidative stress and ROS-induced cellular signaling (Figure 6A), chemotaxis and lysophosphatidic acid signaling via GPCRs (Figure 6B), and androgen receptor activation and downstream signaling in prostate cancer (Figure 6C). The well-distinguished proteins and complexes of proteins are shown as specific symbols6; all experimental data are displayed and have corresponding thermometer-like symbols on all the maps. The upregulated genes are indicated by a red thermometer facing upwards.
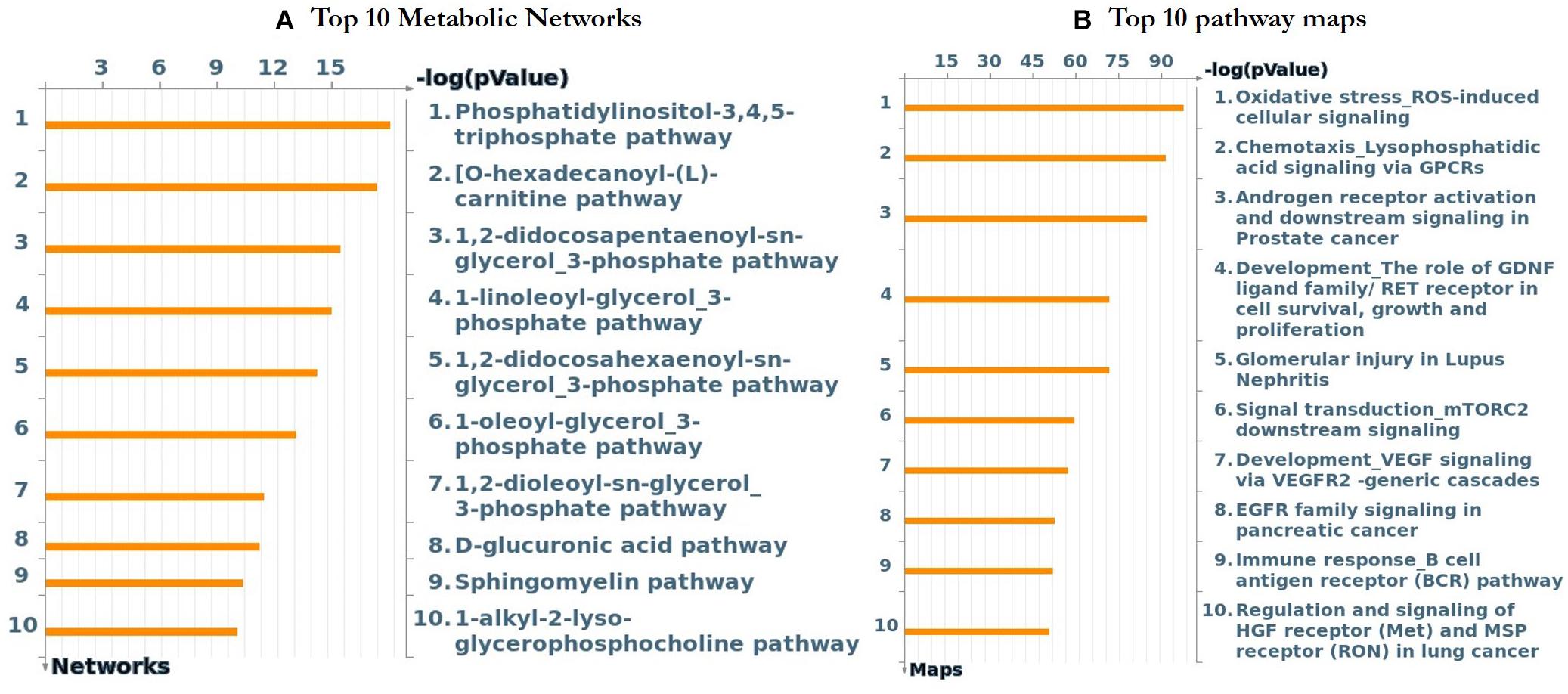
Figure 5. The top 10 metabolic networks and pathway maps were annotated using GeneGo enrichment analysis for the genes that are differentially expressed from SLE patients vs. healthy controls, respectively. (A) The content of these metabolic networks was annotated and defined by GeneGo Cortellis Solution software. Each process represents a pre-set network of protein interactions characteristic for the process, and sorting was performed for the metabolic networks that are statistically significant. (B) The pathway maps (canonical) of GeneGo display a series of signals and metabolic charts that cover human in a structured manner. The significant expression of a gene/protein represented in histogram height.
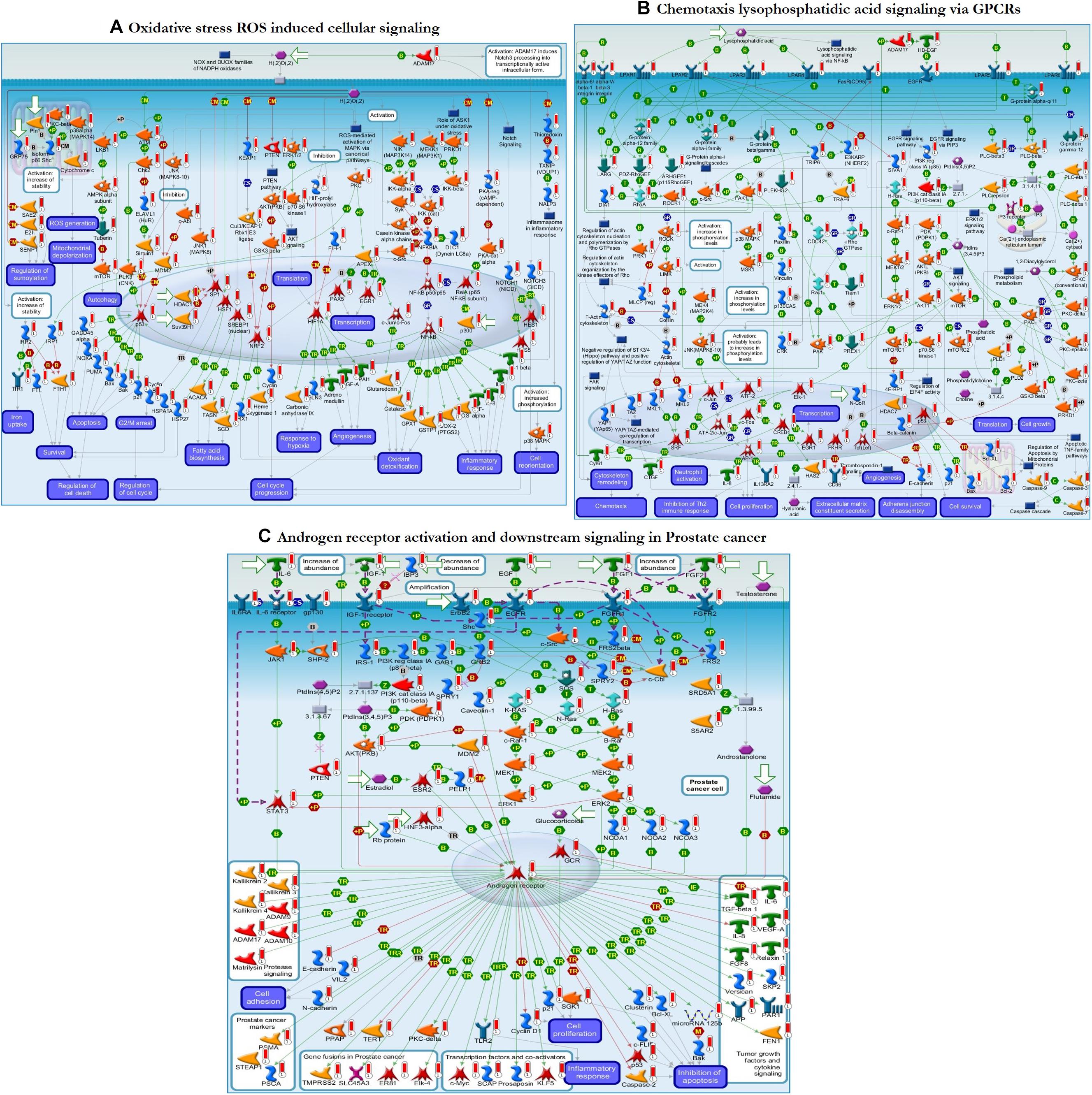
Figure 6. The enrichment analysis from GeneGo showed three regulated pathways with the highest score that are triggered in the SLE human sorted B-cells. (A) Oxidative stress ROS induced cellular signaling. (B) Chemotaxis lysophosphatidic acid signaling via G protein-coupled receptors (GPCRs). (C) Androgen receptor activation and downstream signaling in prostate cancer. The image depicts the protein and protein complexes that are well characterized as a specific symbol; laboratory data from all reports are correlated and shown on the maps as thermometer-like indicators. The red or blue color upward/downward thermometers indicate gene transcripts with upregulation/downregulation, respectively. The proteins connected by arrows demonstrate the stimulating and inhibitory effect of the protein. Further details are given at https://portal.genego.com/help/MC_legend.pdf.
Pathway Map Interaction Results From Clarivate
From the MetacoreTM results, we extracted the key genes from the enriched pathways that were differentially expressed, such as EGR1, CD38, CAV1, and AKT1. The differential expression of these genes was involved in the activation or inhibition of specific protein complexes in the enriched pathway maps (Figure 6 and Table 4). Early growth response 1 (EGR1) is a transcription factor that interacts with the IGF-2, APEX, SRD5A1, CD44, and EGFR genes and activates them through transcriptional regulation. The cyclic adenosine diphosphate (ADP) ribose hydrolase CD38 is an enzyme involved in the activation of the genes Semaphorin 4D, CD19, and c-Cbl through physical interactions. Caveolin 1 (CAV1) is a binding protein shown to interact with the ErbB2, MDR1, HTR2A, and androgen receptor genes, and inhibition or activation is followed by specific binding to its corresponding proteins. RAC-alpha serine/threonine-protein kinase (AKT1) is a protein kinase that interacts with the FKHR, mTOR, Bcl-10, FOXO3A, HNF3-beta, and GSK3 beta genes via phosphorylation, resulting in inhibition or activation. These genes were differentially expressed between sorted B-cells from controls and sorted B-cells from SLE patients and result in transcriptional regulation and inhibition of genes/proteins within the top-scored pathway maps.
Discussion
DNA microarrays and next generation sequencing (NGS) approaches are high-throughput technologies that have resulted in the emergence of new biomedical discoveries. Data from microarray and gene expression profiles have enabled a deeper understanding of the intrinsic molecular pathways of complex mechanisms of biological systems and their responses (Russo et al., 2003; Babu, 2004; Perez-Diez et al., 2013; Kumar et al., 2019). It is therefore highly relevant to examine the peripheral B-cell transcriptomes of SLE patients and healthy controls to determine genes that are differentially regulated and their target pathways. Our current study extracted DEGs from 17 SLE patients and 9 healthy controls from the GEO database (GSE30153) (Garaud et al., 2011). The top 250 DEGs were identified, including 4 upregulated and 13 downregulated genes from the groups through bioinformatics strategies (Table 2 and Supplementary Table S1). These identified DEGs were subjected to ClueGO and GeneGo MetacoreTM analysis for GO and pathway annotation, and constructed the interacting networks of PPI and used for cluster analysis. In the network, the nodes were considered proteins, and the edges were their interactions. Using network topology features, the PPI network can be analyzed to distinguish the core proteins that are involved in the pathways (Barabási and Oltvai, 2004; Ideker and Sharan, 2008; Keskin et al., 2016; Kumar et al., 2019). The identified DEGs from the present study were analyzed with STRING to exploit the complex interactions between the DEGs via text mining, evidence from experiments, and repositories (Figure 2). We performed module screening of the PPI networks using the MCODE plugin from Cytoscape. As a result, we obtained significant clusters that are densely interlinked regions in the PPI network (Figures 3A,B). Screening of these clusters from the network might help to identify the essential genes that are involved in the pathogenesis and progression of SLE. The obtained clusters mostly contained protein complexes or proteins present in the pathways in the PPI network, and cluster visualization is essential for comprehending the properties of the network functionally and systematically (Krogan et al., 2006; Rahman et al., 2013).
Furthermore, to identify the functional enrichment of these subnetworks from MCODE, we implemented the ClueGO plugin for analysis. This revealed that the DEGs were enriched in most essential pathways, which are highly associated with the immune system. The GO and KEGG enrichment analyses of the DEGs from cluster 1 showed that they were mostly enriched in T-cell costimulation, lymphocyte costimulation, negative regulation of vascular permeability, the metaphase/anaphase transition of the mitotic cell cycle, regulation of the transcription involved in the G1/S transition of mitotic cell cycle, the hematopoietic cell lineage, the B-cell receptor signaling pathway, the ErbB signaling pathway, the AGE-RAGE signaling pathway in diabetic complications, and pancreatic cancer. Interestingly, the costimulation of T-cell and lymphocyte receptors is recognized to be important in SLE pathogenesis by enabling communicating with B-cells for the production of autoantibodies (Shlomchik et al., 2001; Mak and Kow, 2014). In SLE, negative regulation of vascular permeability may be induced by different mechanisms; the dysregulated genes from the cluster 1 subnetwork might lead to endothelial cell damage and vasculopathy (Favero et al., 2014; Lee et al., 2019). The differential cell signaling results in the recruitment of various proteins and inappropriate activation of B-cells (Zhou et al., 2009; Comte et al., 2015). Oxidative stress is common in inflammatory disorders and results in the increased production of reactive carbonyl groups that are partially converted to AGEs, and the DEGs in the AGE-RAGE signaling pathway might also be involved in the accumulation of AGEs in SLE patients and lead to diabetic complications (de Leeuw et al., 2007; Li et al., 2007; Kurien and Scofield, 2008; Nienhuis et al., 2008). Interestingly, our enrichment analysis found that the identified differential expression of the genes (AKT1, VEGFA, CDK6, and MAPK9) that were involved in the risk of developing pancreatic cancer in SLE patients was due to chronic inflammation, suggesting that these genes might be involved in the pathogenesis of SLE. Our findings are therefore consistent with the roles of genes that are differentially expressed in SLE-causing pathways (Figure 4A). The enrichment analysis of the cluster 2 subnetwork showed that the DEGs were mostly enriched in the regulation of transcription involved in the G1/S transition of the mitotic cell cycle, the negative regulation of the G0 and G1 transitions, and the p53 signaling pathway. It has been reported that the proliferation of T-cells is followed by lowered levels of cyclin-dependent kinase (CDK) inhibitors, and alterations in the expression of CDKs in the G0/G1 phase were seen in the lymphocytes of SLE patients (Yamauchi and Bloom, 1997; Tang et al., 2009). The DEGs involved in the cluster 2 subnetwork might negatively regulate these pathways. Alterations in cyclin-CDK complex behavior and cyclin-dependent kinase inhibitors (CDKIs) have been reported to alter the proliferation of T-cells, oxidative stress, and immune responses (Santiago-Raber et al., 2001; Tang et al., 2009). p53 signaling is essential for various cellular mechanisms, and defects in this signaling pathway are associated with SLE development. Considerably elevated levels of p53 protein are found in SLE patients with active inflammatory disorders (Miret et al., 2003; Veeranki and Choubey, 2010). Apoptosis dysregulation appears to be another cause of SLE pathogenesis because the possible sources of autoantigens are cell debris from apoptosis in SLE, and excessive cellular senescence of the immune cells, especially T-cells, was reported in SLE patients with peripheral blood mononuclear cells (PBMCs) and skin lesions (Colonna et al., 2014; Sáenz-Corral et al., 2015). Thus, our identified DEGs (RRM2, APC, CHEK1, E2F6, TYMS, E2F7, and CDK6) from the cluster 2 subnetwork are highly related to and consistent with the members of the signaling pathways associated with the immune system, apoptosis, the cell cycle, and vasculopathy.
To clearly define the interactions between the proteins and signaling pathways examined from the interpretation of STRING, Cytoscape, MCODE, and ClueGO analyses, we implemented the GeneGo Metacore software, which incorporates extensive data on metabolic signaling pathways and their regulatory mechanisms and contains accurately complied networks of biological systems. By utilizing the GeneGo Metacore software, we obtained a detailed description of the DEGs that participate in SLE pathogenesis based on the determined p-values. Among the top 10 metabolic networks, the phosphatidylinositol-3,4,5-triphosphate pathway, O-hexadecanoyl-(L)-carnitine pathway, 1,2-didocosapentaenoyl-sn-glycerol 3-phosphate pathway, and 1-linoleoyl-glycerol-3-phosphate pathway were profoundly enriched and significant in the SLE DEGs (Figure 5A). The increased activity of phosphatidylinositol-3,4,5-triphosphate stimulates essential cell signaling pathways such as the pathways involved in cell division, survival, and the rapid increase in T-lymphocytes in SLE (Comte et al., 2015). PI3K (phosphatidylinositol 3-kinase) is a protein kinase that phosphorylates phosphatidylinositol 4,5-phosphate to regulate the signaling of T-lymphocytes; an increased amount of PI3K was also observed in an animal model of lupus (Liu et al., 1998; Grolleau et al., 2000; Niculescu et al., 2003; Joseph et al., 2014). Modification of the carnitine signaling pathway results in various organ failures by producing effective responses to pathogens (Famularo and De Simone, 1995; Famularo et al., 2004). Thus, the DEGs involved in the O-hexadecanoyl-(L)-carnitine pathway might lead to increased immune responses. In addition, the top three pathways associated with the DEGs of sorted B-cells from SLE patients were mostly enriched in oxidative stress- and ROS-induced cellular signaling (Figure 6A), chemotaxis and lysophosphatidic acid signaling via GPCRs (Figure 6B), and androgen receptor activation and downstream signaling in prostate cancer (Figure 6C). Recent findings have shown that oxidative stress and ROS induce molecular alterations that have adverse effects in SLE patients (Choi et al., 2016; Tsokos et al., 2016; Lightfoot et al., 2017). Elevated oxidative stress in SLE patients leads to the accumulation of higher amounts of oxidative lipoproteins, which are harmful in zebrafish models and cause additional oxidative damage to the system (Chung et al., 2007; Park et al., 2016; Lightfoot et al., 2017). Interestingly, our study identified the EGR1 gene as downregulated in the SLE patients in comparison to controls, and it also plays a role in ROS signaling. This clearly indicates that EGR1 might be required to maintain the oxidative stress and ROS signaling pathways.
Moreover, the DEGs involved in the oxidative stress signaling pathway might contribute to peripheral neuropathy, damage to blood vessels, and cardiovascular events, which are the prominent clinical conditions found in SLE patients. Chemotaxis and lysophosphatidic acid (LPA) signaling are essential pathways in autoimmune inflammatory disorders, and GPCRs are responsible for regulating immune cells via LPA receptors (Yang et al., 2005; Skoura and Hla, 2009). G2A gene knockout resulted in the hyperresponsiveness of T-cells to T-cell receptor stimulation, manifesting as an increased proliferation of T-cells, which may promote inflammatory phenotypes in G2A-deficient mice (Le et al., 2001). Various studies have suggested that LPA plays a vital role in atherosclerosis progression and development by promoting neutrophil and monocyte adherence and enhancing inflammatory events (Siess et al., 1999; Smyth et al., 2008; Skoura and Hla, 2009). The androgen receptor (AR) is a transcription factor that is activated by a ligand and is essential for cells targeted by the androgen response (Robeva et al., 2013; Gubbels Bupp and Jorgensen, 2018). AR also regulates immune function in SLE via transcriptional regulation of various genes. Our study identified the transcription factor AR, which positively regulates the c-Myc, SCAP, prosaposin, and KLF5 genes, which are responsible for inflammatory responses, and promotes tumor growth factors and cytokine signaling when activated (Figure 6C). The enriched terms from ClueGO modules and the GeneGo-identified terms correlated well in this study and validate the significance of the findings from the pathway maps. The combined results from these two enrichment analyses suggest that B-cells from SLE patients and B-cells from healthy controls undergo differential gene expression associated with positive regulation of kidney development, the hematopoietic cell lineage, positive regulation of vasoconstriction, T-cell costimulation, and regulation of the transcription involved in the G1/S transition of the mitotic cell cycle.
Furthermore, the interaction results from the GeneGo analysis provided the essential genes (EGR1, CD38, CAV1, and AKT1) from the pathway maps constructed from the DEGs. Among them, EGR1 (early growth response 1) is a transcription factor shown to interact with the IGF-2 (insulin-like growth factor 2), APEX (apurinic/apyrimidinic endodeoxyribonuclease 1), SRD5A1 (steroid 5 alpha-reductase 1), CD44 (cell surface glycoprotein CD44), and EGFR (epidermal growth factor receptor) genes and transcriptionally regulate them by activating or promoting their expression in sorted B-cells from patients with SLE (Liu et al., 1995; Recio and Merlino, 2003; Lee et al., 2005; Pines et al., 2005; Blanchard et al., 2007; Rui et al., 2008; Cullen et al., 2010; Sauer et al., 2010). The cyclic ADP ribose hydrolase (CD38) is also known as cluster of differentiation 38 protein, can be found on several immune cells, and activates SEMA4D (semaphorin-4D or cluster of differentiation 100), CD19 (B-lymphocyte antigen CD19 or cluster of differentiation 19), and c-Cbl (Casitas B-lineage lymphoma proto-oncogene) (Deaglio et al., 2005, 2007; Shen and Yen, 2008; Vences-Catalán et al., 2012). These interactions with CD38 result in the activation of B-lymphocytes and increase immune responses in SLE patients. The protein caveolin 1 (CAV1) has been shown to interact with the ErbB2 (Erb-B2 receptor tyrosine kinase 2), MDR1 (multidrug resistance protein 1), HTR2A (5-hydroxytryptamine receptor 2A), and AR (androgen receptor) genes. Several studies have suggested that caveolin 1 physically interacts with HER2, p-gp, HTR2A, and AR and activates/inhibits them by binding to their specific caveolin-binding motif (Couet et al., 1997; Lu et al., 2001; Razani and Lisanti, 2001; Bhatnagar et al., 2004; Bennett et al., 2010, 2014; Yu et al., 2012). AKT1 is a protein kinase that interacts with FKHR (Forkhead box protein O1), mTOR (mechanistic target of rapamycin), Bcl-10 (B-cell lymphoma/leukemia 10), FOXO3A (Forkhead box O3), HNF3-beta (hepatocyte nuclear factor 3-beta), and GSK3 beta (glycogen synthase kinase three beta). The protein kinase AKT1 inhibits FKHR via phosphorylation and decreases its activity (Biggs et al., 1999; Rena et al., 1999; Tang et al., 1999; Hay, 2011), whereas it increases its activity via phosphorylation of mTOR (Navé et al., 1999; Sekuliæ et al., 2000; Ikenoue et al., 2008; Thirumal Kumar et al., 2019). Additionally, AKT1 phosphorylates Bcl-10 at the specific residues Ser231 and Ser218, increasing its activity (Yeh et al., 2006), while it inhibits the action of FOXO3A via phosphorylation and decreases its activity, increasing the survival of cells (Brunet et al., 1999; Linding et al., 2007; Calnan and Brunet, 2008; Li et al., 2008; Tzivion et al., 2011). AKT1 decreases HNF3-beta activity by phosphorylating it at Thr156 (Wolfrum et al., 2003), whereas phosphorylation of GSK3-beta by AKT1 occurs at Ser9 to inhibit its activity (Brazil and Hemmings, 2001; Salas et al., 2004; Kuemmerle, 2005; Shin et al., 2006; Markou et al., 2008). This suggests the vital genes we identified from the DEGs of patients with SLE play essential roles in the development and progression of SLE via different signaling pathways to increase autoimmune responses.
In addition to the interaction analysis, we carried out interrelation analysis for the essential genes to determine the relationships between the genes, which implicitly or explicitly interacted with each other. Interestingly, the identified genes indirectly communicated with each other via molecular signaling pathways related to mTOR signaling, apoptosis, PI3K-Akt signaling, the hematopoietic cell lineage, positive regulation of vasoconstriction, signaling by receptor tyrosine kinases, AGE-RAGE signaling, and lymphocyte and T-cell costimulation (Figure 7). EGR1 and AKT1 are directly involved in oxidative stress via ROS and AGE-RAGE signaling, whereas CAV1 is directly involved in tyrosine kinase receptor signaling and lymphocyte and T-cell costimulation. CD38 is directly associated with the hematopoietic cell lineage and positive regulation of vasoconstriction. Overall, the dysregulation of the indicated pathways in SLE patients is a result of differential gene expression. The essential genes are differentially expressed between cells from patients with SLE and cells from healthy controls and are present in important signaling cascades, which could be a crucial factor for SLE development.
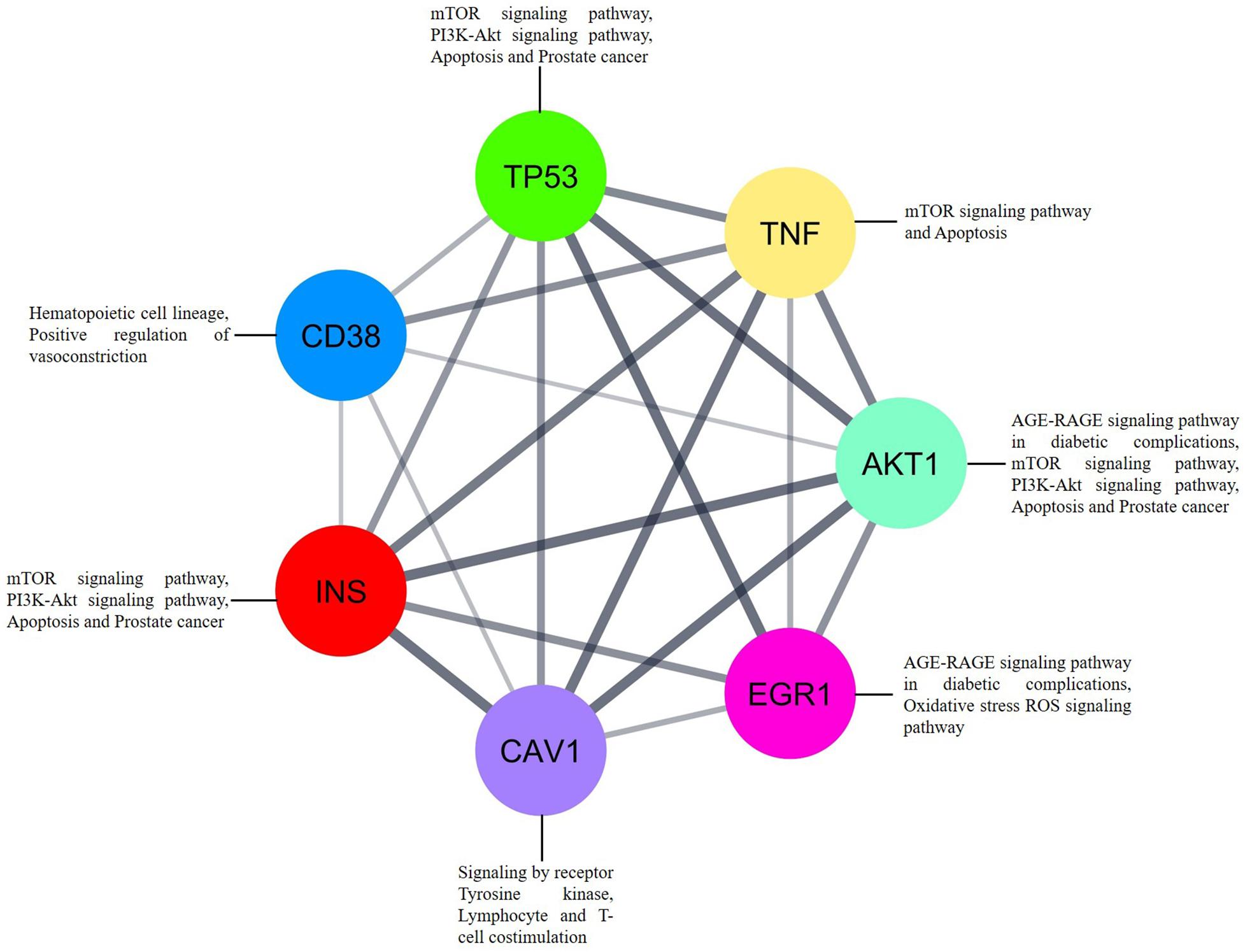
Figure 7. The interrelation analysis of genes EGR1, CD38, CAV1, and AKT1 that strongly associated to SLE. Each gene involved in different pathways via interacting to each other. Inbuilt color code was provided to all the genes based on the STRING tool from Cytoscape.
Conclusion
Taken together, the results of our comprehensive bioinformatics analysis showed that the DEGs identified between sorted B-cells from patients with SLE sorted B-cells from controls could play a significant role in the growth, progression, and development of SLE. This study identified 4 upregulated and 13 downregulated genes, including essential genes (EGR1, CD38, CAV1, and AKT1), from the pathway enrichment analysis. Indeed, the identified pathways from the enrichment analysis were strongly related to the immune system, vasculopathy, cardiovascular functions, and inflammatory responses, which are processes that can lead to the development of SLE. The broad understanding of SLE pathophysiology from this study will allow us to identify and develop therapies targeting SLE and contribute to personalized treatment strategies. Collectively, the study findings could aid in enhancing our understanding of the fundamental molecular processes of SLE and provide possible strategies for early diagnosis in SLE; in addition, combinatorial therapeutic strategies using oxidative stress and ROS cellular signaling and lysophosphatidic acid signaling via GPCRs might have symbiotic effects on the molecular events in SLE.
Data Availability Statement
Publicly available datasets were analyzed in this study. This data can be found here: https://www.ncbi.nlm.nih.gov/geo/query/acc.cgi?acc=GSE30153.
Author Contributions
SU, DT, HZ, and CG were involved in the design of the study and the acquisition, analysis, and interpretation of the data. SU, DT, CG, SY, NY, MS, and HZ were involved in the interpretation of the data and drafting the manuscript. CG, RS, and HZ supervised the entire study and were involved in study design, the acquisition, analysis, and interpretation of the data, and drafting the manuscript. The manuscript was reviewed and approved by all the authors.
Funding
This work was supported by Qatar University Grant# QUST-1-CHS-2020-2.
Conflict of Interest
The authors declare that the research was conducted in the absence of any commercial or financial relationships that could be construed as a potential conflict of interest.
Acknowledgments
We would like to take this opportunity to thank the management of VIT for providing the necessary facilities and encouragement to carry out this work.
Supplementary Material
The Supplementary Material for this article can be found online at: https://www.frontiersin.org/articles/10.3389/fbioe.2020.00276/full#supplementary-material
Footnotes
- ^ https://www.ncbi.nlm.nih.gov/geo/
- ^ https://paolo.shinyapps.io/ShinyVolcanoPlot/
- ^ http://www.string-db.org/
- ^ http://www.cytoscape.org/
- ^ https://clarivate.com/products/metacore/
- ^ https://portal.genego.com/help/MC_legend.pdf
References
Alibés, A., Yankilevich, P., Cañada, A., and Díaz-Uriarte, R. (2007). IDconverter and IDClight: conversion and annotation of gene and protein IDs. BMC Bioinformatics 8:9. doi: 10.1186/1471-2105-8-9
Aubert, J., Bar-Hen, A., Daudin, J. J., and Robin, S. (2004). Determination of the differentially expressed genes in microarray experiments using local FDR. BMC Bioinformatics 5:125. doi: 10.1186/1471-2105-5-125
Babu, M. M. (2004). An Introduction to Microarray Data Analysis. Cambridge: Horizon Bioscience, 225–249.
Bader, G. D., and Hogue, C. W. (2003). An automated method for finding molecular complexes in large protein interaction networks. BMC Bioinformatics 4:2. doi: 10.1186/1471-2105-4-2
Barabási, A.-L., and Oltvai, Z. N. (2004). Network biology: understanding the cell’s functional organization. Nat. Rev. Genet. 5, 101–113. doi: 10.1038/nrg1272
Barrett, T., Wilhite, S. E., Ledoux, P., Evangelista, C., Kim, I. F., Tomashevsky, M., et al. (2013). NCBI GEO: archive for functional genomics data sets–update. Nucleic Acids Res. 41, D991–D995. doi: 10.1093/nar/gks1193
Benjamini, Y., and Hochberg, Y. (1995). Controlling the false discovery rate: a practical and powerful approach to multiple testing. J. R. Statist. Soc. Ser. B (Methodol.) 57, 289–300.
Bennett, N. C., Gardiner, R. A., Hooper, J. D., Johnson, D. W., and Gobe, G. C. (2010). Molecular cell biology of androgen receptor signalling. Int. J. Biochem. Cell Biol. 42, 813–827. doi: 10.1016/j.biocel.2009.11.013
Bennett, N. C., Hooper, J. D., Johnson, D. W., and Gobe, G. C. (2014). Expression profiles and functional associations of endogenous androgen receptor and caveolin-1 in prostate cancer cell lines. Prostate 74, 478–487. doi: 10.1002/pros.22767
Bentham, J., Morris, D. L., Cunninghame Graham, D. S., Pinder, C. L., Tombleson, P., Behrens, T. W., et al. (2015). Genetic association analyses implicate aberrant regulation of innate and adaptive immunity genes in the pathogenesis of systemic lupus erythematosus. Nat. Genet. 47, 1457–1464. doi: 10.1038/ng.3434
Bhatnagar, A., Sheffler, D. J., Kroeze, W. K., Compton-Toth, B., and Roth, B. L. (2004). Caveolin-1 interacts with 5-HT2A serotonin receptors and profoundly modulates the signaling of selected Gαq-coupled protein receptors. J. Biol. Chem. 279, 34614–34623. doi: 10.1074/jbc.M404673200
Biggs, W. H., Meisenhelder, J., Hunter, T., Cavenee, W. K., and Arden, K. C. (1999). Protein kinase B/Akt-mediated phosphorylation promotes nuclear exclusion of the winged helix transcription factor FKHR1. Proc. Natl. Acad. Sci. U.S.A. 96, 7421–7426. doi: 10.1073/pnas.96.13.7421
Bindea, G., Mlecnik, B., Hackl, H., Charoentong, P., Tosolini, M., Kirilovsky, A., et al. (2009). ClueGO: a cytoscape plug-in to decipher functionally grouped gene ontology and pathway annotation networks. Bioinformatics 25, 1091–1093. doi: 10.1093/bioinformatics/btp101
Blanchard, Y., Seenundun, S., and Robaire, B. (2007). The promoter of the rat 5α-reductase type 1 gene is bidirectional and Sp1-dependent. Mol. Cell. Endocrinol. 264, 171–183. doi: 10.1016/j.mce.2006.11.007
Borrebaeck, C. A. K., Sturfelt, G., and Wingren, C. (2014). “Recombinant antibody microarray for profiling the serum proteome of SLE,” in Systemic Lupus Erythematosus: Methods and Protocols Methods in Molecular Biology, eds P. Eggleton and F. J. Ward (New York, NY: Springer), 67–78. doi: 10.1007/978-1-4939-0326-9_6
Brazil, D. P., and Hemmings, B. A. (2001). Ten years of protein kinase B signalling: a hard Akt to follow. Trends Biochem. Sci. 26, 657–664. doi: 10.1016/S0968-0004(01)01958-2
Brunet, A., Bonni, A., Zigmond, M. J., Lin, M. Z., Juo, P., Hu, L. S., et al. (1999). Akt promotes cell survival by phosphorylating and inhibiting a forkhead transcription factor. Cell 96, 857–868. doi: 10.1016/S0092-8674(00)80595-4
Calnan, D. R., and Brunet, A. (2008). The FoxO code. Oncogene 27, 2276–2288. doi: 10.1038/onc.2008.21
Choi, S.-C., Titov, A. A., Sivakumar, R., Li, W., and Morel, L. (2016). Immune cell metabolism in systemic lupus erythematosus. Curr. Rheumatol. Rep. 18:66. doi: 10.1007/s11926-016-0615-7
Chung, C. P., Avalos, I., Oeser, A., Gebretsadik, T., Shintani, A., Raggi, P., et al. (2007). High prevalence of the metabolic syndrome in patients with systemic lupus erythematosus: association with disease characteristics and cardiovascular risk factors. Ann. Rheum. Dis. 66, 208–214. doi: 10.1136/ard.2006.054973
Colonna, L., Lood, C., and Elkon, K. (2014). Beyond apoptosis in lupus. Curr Opin Rheumatol. 26, 459–466. doi: 10.1097/BOR.0000000000000083
Comte, D., Karampetsou, M. P., and Tsokos, G. C. (2015). T cells as a therapeutic target in SLE. Lupus 24, 351–363. doi: 10.1177/0961203314556139
Costa-Reis, P., and Sullivan, K. E. (2013). Genetics and epigenetics of systemic lupus erythematosus. Curr. Rheumatol. Rep. 15:369. doi: 10.1007/s11926-013-0369-4
Couet, J., Sargiacomo, M., and Lisanti, M. P. (1997). Interaction of a receptor tyrosine kinase, EGF-R, with caveolins. caveolin binding negatively regulates tyrosine and serine/threonine kinase activities. J. Biol. Chem. 272, 30429–30438. doi: 10.1074/jbc.272.48.30429
Cui, Y., Sheng, Y., and Zhang, X. (2013). Genetic susceptibility to SLE: Recent progress from GWAS. J. Autoimm. 41, 25–33. doi: 10.1016/j.jaut.2013.01.008
Cullen, E. M., Brazil, J. C., and O’Connor, C. M. (2010). Mature human neutrophils constitutively express the transcription factor EGR-1. Mol. Immunol. 47, 1701–1709. doi: 10.1016/j.molimm.2010.03.003
Dang, J., Li, J., Xin, Q., Shan, S., Bian, X., Yuan, Q., et al. (2016). Gene–gene interaction of ATG5, ATG7, BLK and BANK1 in systemic lupus erythematosus. Int. J. Rheum. Dis. 19, 1284–1293. doi: 10.1111/1756-185X.12768
de Leeuw, K., Graaff, R., de Vries, R., Dullaart, R. P., Smit, A. J., Kallenberg, C. G., et al. (2007). Accumulation of advanced glycation endproducts in patients with systemic lupus erythematosus. Rheumatology (Oxford) 46, 1551–1556. doi: 10.1093/rheumatology/kem215
Deaglio, S., Vaisitti, T., Bergui, L., Bonello, L., Horenstein, A. L., Tamagnone, L., et al. (2005). CD38 and CD100 lead a network of surface receptors relaying positive signals for B-CLL growth and survival. Blood 105, 3042–3050. doi: 10.1182/blood-2004-10-3873
Deaglio, S., Vaisitti, T., Billington, R., Bergui, L., Omede’, P., Genazzani, A. A., et al. (2007). CD38/CD19: a lipid raft–dependent signaling complex in human B cells. Blood 109, 5390–5398. doi: 10.1182/blood-2006-12-061812
Deng, Y., and Tsao, B. P. (2010). Genetic susceptibility to systemic lupus erythematosus in the genomic era. Nat. Rev. Rheumatol. 6, 683–692. doi: 10.1038/nrrheum.2010.176
Ducreux, J., Houssiau, F. A., Vandepapelière, P., Jorgensen, C., Lazaro, E., Spertini, F., et al. (2016). Interferon α kinoid induces neutralizing anti-interferon α antibodies that decrease the expression of interferon-induced and B cell activation associated transcripts: analysis of extended follow-up data from the interferon α kinoid phase I/II study. Rheumatology (Oxford) 55, 1901–1905. doi: 10.1093/rheumatology/kew262
Edgar, R., Domrachev, M., and Lash, A. E. (2002). Gene expression omnibus: NCBI gene expression and hybridization array data repository. Nucleic Acids Res. 30, 207–210. doi: 10.1093/nar/30.1.207
Famularo, G., and De Simone, C. (1995). A new era for carnitine? Immunol. Today 16, 211–213. doi: 10.1016/0167-5699(95)80159-6
Famularo, G., Simone, C., de, Trinchieri, V., and Mosca, L. (2004). Carnitines and its congeners: a metabolic pathway to the regulation of immune response and inflammation. Ann. N. Y. Acad. Sci. 1033, 132–138. doi: 10.1196/annals.1320.012
Favero, G., Paganelli, C., Buffoli, B., Rodella, L. F., and Rezzani, R. (2014). Endothelium and its alterations in cardiovascular diseases: life style intervention. Biomed. Res. Int. 2014:801896. doi: 10.1155/2014/801896
Garaud, J.-C., Schickel, J.-N., Blaison, G., Knapp, A.-M., Dembele, D., Ruer-Laventie, J., et al. (2011). B cell signature during inactive systemic lupus is heterogeneous: toward a biological dissection of lupus. PLoS One 6:e23900. doi: 10.1371/journal.pone.0023900
Grolleau, A., Kaplan, M. J., Hanash, S. M., Beretta, L., and Richardson, B. (2000). Impaired translational response and increased protein kinase PKR expression in T cells from lupus patients. J. Clin. Invest. 106, 1561–1568. doi: 10.1172/JCI9352
Gubbels Bupp, M. R., and Jorgensen, T. N. (2018). Androgen-Induced Immunosuppression. Front. Immunol. 9:794. doi: 10.3389/fimmu.2018.00794
Gurevitz, S., Snyder, J., Wessel, E., Frey, J., and Williamson, B. (2013). Systemic lupus erythematosus: a review of the disease and treatment options. Consult. Pharm. 28, 110–121. doi: 10.4140/TCP.n.2013.110
Harley, I. T. W., Kaufman, K. M., Langefeld, C. D., Harley, J. B., and Kelly, J. A. (2009). Genetic susceptibility to SLE: new insights from fine mapping and genome-wide association studies. Nat. Rev. Genet. 10, 285–290. doi: 10.1038/nrg2571
Hay, N. (2011). Interplay between FOXO, TOR, and Akt. Biochim. Biophys. Acta (BBA) Mol. Cell Res. 1813, 1965–1970. doi: 10.1016/j.bbamcr.2011.03.013
Ideker, T., and Sharan, R. (2008). Protein networks in disease. Genome Res. 18, 644–652. doi: 10.1101/gr.071852.107
Ikenoue, T., Inoki, K., Yang, Q., Zhou, X., and Guan, K.-L. (2008). Essential function of TORC2 in PKC and Akt turn motif phosphorylation, maturation and signalling. EMBO J. 27, 1919–1931. doi: 10.1038/emboj.2008.119
Irizarry, R. A., Bolstad, B. M., Collin, F., Cope, L. M., Hobbs, B., and Speed, T. P. (2003). Summaries of affymetrix genechip probe level data. Nucleic Acids Res. 31:e15. doi: 10.1093/nar/gng015
Joseph, N., Reicher, B., and Barda-Saad, M. (2014). The calcium feedback loop and T cell activation: How cytoskeleton networks control intracellular calcium flux. Biochim. Biophys. Acta (BBA) Biomemb. 1838, 557–568. doi: 10.1016/j.bbamem.2013.07.009
Keskin, O., Tuncbag, N., and Gursoy, A. (2016). Predicting protein–protein interactions from the molecular to the proteome level. Chem. Rev. 116, 4884–4909. doi: 10.1021/acs.chemrev.5b00683
Krogan, N. J., Cagney, G., Yu, H., Zhong, G., Guo, X., Ignatchenko, A., et al. (2006). Global landscape of protein complexes in the yeast Saccharomyces cerevisiae. Nature 440, 637–643. doi: 10.1038/nature04670
Kuemmerle, J. F. (2005). Endogenous IGF-I protects human intestinal smooth muscle cells from apoptosis by regulation of GSK-3β activity. Am. J. Physiol. Gastroin. Liver Physiol. 288, G101–G110. doi: 10.1152/ajpgi.00032.2004
Kumar, S. U., Kumar, D. T., Siva, R., Doss, C. G. P., and Zayed, H. (2019). Integrative bioinformatics approaches to map potential novel genes and pathways involved in ovarian cancer. Front. Bioeng. Biotechnol. 7:391. doi: 10.3389/fbioe.2019.00391
Kurien, B. T., and Scofield, R. H. (2008). Autoimmunity and oxidatively modified autoantigens. Autoimmun. Rev. 7, 567–573. doi: 10.1016/j.autrev.2008.04.019
Le, L. Q., Kabarowski, J. H., Weng, Z., Satterthwaite, A. B., Harvill, E. T., Jensen, E. R., et al. (2001). Mice lacking the orphan G protein-coupled receptor G2A develop a late-onset autoimmune syndrome. Immunity 14, 561–571. doi: 10.1016/s1074-7613(01)00145-5
Lee, W.-F., Wu, C.-Y., Yang, H.-Y., Lee, W.-I., Chen, L.-C., Ou, L.-S., et al. (2019). Biomarkers associating endothelial Dysregulation in pediatric-onset systemic lupus erythematous. Pediat. Rheumatol. 17:69. doi: 10.1186/s12969-019-0369-7
Lee, Y.-S., Jang, H.-S., Kim, J.-M., Lee, J.-S., Lee, J.-Y., Kim, K. L., et al. (2005). Adenoviral-mediated delivery of early growth response factor-1 gene increases tissue perfusion in a murine model of hindlimb ischemia. Mol. Ther. 12, 328–336. doi: 10.1016/j.ymthe.2005.03.027
Li, J.-T., Hou, F.-F., Guo, Z.-J., Shan, Y.-X., Zhang, X., and Liu, Z.-Q. (2007). Advanced glycation end products upregulate C-reactive protein synthesis by human hepatocytes through stimulation of monocyte IL-6 and IL-1β production. Scand. J. Immunol. 66, 555–562. doi: 10.1111/j.1365-3083.2007.02001.x
Li, Y., Wang, Z., Kong, D., Li, R., Sarkar, S. H., and Sarkar, F. H. (2008). Regulation of Akt/FOXO3a/GSK-3β/AR signaling network by isoflavone in prostate cancer cells. J. Biol. Chem. 283, 27707–27716. doi: 10.1074/jbc.M802759200
Lightfoot, Y. L., Blanco, L. P., and Kaplan, M. J. (2017). Metabolic abnormalities and oxidative stress in lupus. Curr. Opin. Rheumatol. 29, 442–449. doi: 10.1097/BOR.0000000000000413
Linding, R., Jensen, L. J., Ostheimer, G. J., Vugt, M. A. T. M., van Jørgensen, C., Miron, I. M., et al. (2007). Systematic discovery of in vivo phosphorylation networks. Cell 129, 1415–1426. doi: 10.1016/j.cell.2007.05.052
Liu, K.-Q., Bunnell, S. C., Gurniak, C. B., and Berg, L. J. (1998). T cell receptor–initiated calcium release is uncoupled from capacitative calcium entry in itk-deficient T cells. J. Exp. Med. 187, 1721–1727. doi: 10.1084/jem.187.10.1721
Liu, Z., Mittanck, D. W., Kim, S., and Rotwein, P. (1995). Control of insulin-like growth factor-II/mannose 6-phosphate receptor gene transcription by proximal promoter elements. Mol. Endocrinol. 9, 1477–1487. doi: 10.1210/mend.9.11.8584025
Love, M. I., Huber, W., and Anders, S. (2014). Moderated estimation of fold change and dispersion for RNA-seq data with DESeq2. Genome Biol. 15:550. doi: 10.1186/s13059-014-0550-8
Lu, M. L., Schneider, M. C., Zheng, Y., Zhang, X., and Richie, J. P. (2001). Caveolin-1 interacts with androgen receptor a positive modulator of androgen receptor mediated transactivation. J. Biol. Chem. 276, 13442–13451. doi: 10.1074/jbc.M006598200
Mak, A., and Kow, N. Y. (2014). The pathology of T cells in systemic lupus erythematosus. J. Immunol. Res. 2014:419029. doi: 10.1155/2014/419029
Markou, T., Cullingford, T. E., Giraldo, A., Weiss, S. C., Alsafi, A., Fuller, S. J., et al. (2008). Glycogen synthase kinases 3α and 3β in cardiac myocytes: regulation and consequences of their inhibition. Cell. Signal. 20, 206–218. doi: 10.1016/j.cellsig.2007.10.004
Miret, C., Molina, R., Filella, X., García-Carrasco, M., Claver, G., Ingelmo, M., et al. (2003). Relationship of p53 with other oncogenes, cytokines and systemic lupus erythematosus activity. TBI 24, 185–188. doi: 10.1159/000074428
Navé, B. T., Ouwens, M., Withers, D. J., Alessi, D. R., and Shepherd, P. R. (1999). Mammalian target of rapamycin is a direct target for protein kinase B: identification of a convergence point for opposing effects of insulin and amino-acid deficiency on protein translation. Biochem. J. 344, 427–431.
Niculescu, F., Nguyen, P., Niculescu, T., Rus, H., Rus, V., and Via, C. S. (2003). Pathogenic T cells in murine lupus exhibit spontaneous signaling activity through phosphatidylinositol 3-kinase and mitogen-activated protein kinase pathways. Arthr. Rheum. 48, 1071–1079. doi: 10.1002/art.10900
Nienhuis, H. L., de Leeuw, K., Bijzet, J., Smit, A., Schalkwijk, C. G., Graaff, R., et al. (2008). Skin autofluorescence is increased in systemic lupus erythematosus but is not reflected by elevated plasma levels of advanced glycation endproducts. Rheumatology (Oxford) 47, 1554–1558. doi: 10.1093/rheumatology/ken302
Park, J. K., Kim, J.-Y., Moon, J. Y., Ahn, E. Y., Lee, E. Y., Lee, E. B., et al. (2016). Altered lipoproteins in patients with systemic lupus erythematosus are associated with augmented oxidative stress: a potential role in atherosclerosis. Arthr. Res. Ther. 18:306. doi: 10.1186/s13075-016-1204-x
Perez-Diez, A., Morgun, A., and Shulzhenko, N. (2013). Microarrays for Cancer Diagnosis and Classification. Landes Bioscience. Available online at: https://www.ncbi.nlm.nih.gov/books/NBK6624/ (accessed July 19, 2019).
Pines, A., Bivi, N., Romanello, M., Damante, G., Kelley, M. R., Adamson, E. D., et al. (2005). Cross-regulation between Egr-1 and APE/Ref-1 during early response to oxidative stress in the human osteoblastic HOBIT cell line: Evidence for an autoregulatory loop. Free Radical Res. 39, 269–281. doi: 10.1080/10715760400028423
Pons-Estel, G. J., Alarcón, G. S., Scofield, L., Reinlib, L., and Cooper, G. S. (2010). Understanding the epidemiology and progression of systemic lupus erythematosus. Sem. Arthr. Rheum. 39, 257–268. doi: 10.1016/j.semarthrit.2008.10.007
Prokunina, L., and Alarcon-Riquelme, M. (2004). The genetic basis of systemic lupus erythematosus—knowledge of today and thoughts for tomorrow. Hum. Mol. Genet. 13, R143–R148. doi: 10.1093/hmg/ddh076
Rahman, K. M. T., Islam, M. F., Banik, R. S., Honi, U., Diba, F. S., Sumi, S. S., et al. (2013). Changes in Protein Interaction Networks Between Normal and Cancer Conditions: Total Chaos or Ordered Disorder? Network Biology. Available online at: http://agris.fao.org/agris-search/search.do?recordID=CN2013200002 (accessed November 19, 2019).
Razani, B., and Lisanti, M. P. (2001). Caveolins and caveolae: molecular and functional relationships. Exp. Cell Res. 271, 36–44. doi: 10.1006/excr.2001.5372
Recio, J. A., and Merlino, G. (2003). Hepatocyte growth factor/scatter factor induces feedback up-regulation of CD44v6 in melanoma cells through Egr-1. Cancer Res. 63, 1576–1582.
Rena, G., Guo, S., Cichy, S. C., Unterman, T. G., and Cohen, P. (1999). Phosphorylation of the transcription factor forkhead family member FKHR by protein kinase B. J. Biol. Chem. 274, 17179–17183. doi: 10.1074/jbc.274.24.17179
Ritchie, M. E., Phipson, B., Wu, D., Hu, Y., Law, C. W., Shi, W., et al. (2015). limma powers differential expression analyses for RNA-sequencing and microarray studies. Nucleic Acids Res. 43:e47. doi: 10.1093/nar/gkv007
Robeva, R., Tanev, D., Andonova, S., Kirilov, G., Savov, A., Stoycheva, M., et al. (2013). Androgen receptor (CAG)n polymorphism and androgen levels in women with systemic lupus erythematosus and healthy controls. Rheumatol. Int. 33, 2031–2038. doi: 10.1007/s00296-013-2687-2
Rui, C., Li, C., Xu, W., Zhan, Y., Li, Y., and Yang, X. (2008). Involvement of Egr-1 in HGF-induced elevation of the human 5α-R1 gene in human hepatocellular carcinoma cells. Biochem. J. 411, 379–386. doi: 10.1042/BJ20071343
Russo, G., Zegar, C., and Giordano, A. (2003). Advantages and limitations of microarray technology in human cancer. Oncogene 22:6497. doi: 10.1038/sj.onc.1206865
Sáenz-Corral, I., Vega-Memíje, M. E., Martínez-Luna, E., Cuevas-González, J. C., Rodríguez-Carreón, A. A., de la Rosa, J. J. B.-G., et al. (2015). Apoptosis in chronic cutaneous lupus erythematosus, discoid lupus, and lupus profundus. Int. J. Clin. Exp. Pathol. 8, 7260–7265.
Salas, T. R., Kim, J., Vakar-Lopez, F., Sabichi, A. L., Troncoso, P., Jenster, G., et al. (2004). Glycogen synthase kinase-3β is involved in the phosphorylation and suppression of androgen receptor activity. J. Biol. Chem. 279, 19191–19200. doi: 10.1074/jbc.M309560200
Santiago-Raber, M.-L., Lawson, B. R., Dummer, W., Barnhouse, M., Koundouris, S., Wilson, C. B., et al. (2001). Role of cyclin kinase inhibitor p21 in systemic autoimmunity. J. Immunol. 167, 4067–4074. doi: 10.4049/jimmunol.167.7.4067
Sauer, L., Gitenay, D., Vo, C., and Baron, V. T. (2010). Mutant p53 initiates a feedback loop that involves Egr-1/EGF receptor/ERK in prostate cancer cells. Oncogene 29, 2628–2637. doi: 10.1038/onc.2010.24
Sekuliæ, A., Hudson, C. C., Homme, J. L., Yin, P., Otterness, D. M., Karnitz, L. M., et al. (2000). A Direct linkage between the phosphoinositide 3-kinase-AKT signaling pathway and the mammalian target of rapamycin in mitogen-stimulated and transformed cells. Cancer Res. 60, 3504–3513.
Shannon, P., Markiel, A., Ozier, O., Baliga, N. S., Wang, J. T., Ramage, D., et al. (2003). Cytoscape: a software environment for integrated models of biomolecular interaction networks. Genome Res. 13, 2498–2504. doi: 10.1101/gr.1239303
Shen, M., and Yen, A. (2008). c-Cbl Interacts with CD38 and promotes retinoic acid–induced differentiation and G0 arrest of human myeloblastic leukemia cells. Cancer Res. 68, 8761–8769. doi: 10.1158/0008-5472.CAN-08-1058
Shin, S. Y., Chin, B. R., Lee, Y. H., and Kim, J.-H. (2006). Involvement of glycogen synthase kinase-3β in hydrogen peroxide-induced suppression of Tcf/Lef-dependent transcriptional activity. Cell. Signal. 18, 601–607. doi: 10.1016/j.cellsig.2005.06.001
Shlomchik, M. J., Craft, J. E., and Mamula, M. J. (2001). From T to B and back again: positive feedback in systemic autoimmune disease. Nat. Rev. Immunol. 1, 147–153. doi: 10.1038/35100573
Siess, W., Zangl, K. J., Essler, M., Bauer, M., Brandl, R., Corrinth, C., et al. (1999). Lysophosphatidic acid mediates the rapid activation of platelets and endothelial cells by mildly oxidized low density lipoprotein and accumulates in human atherosclerotic lesions. Proc. Natl. Acad. Sci. U.S.A. 96, 6931–6936.
Skoura, A., and Hla, T. (2009). Lysophospholipid receptors in vertebrate development, physiology, and pathology. J. Lipid Res. 50, S293–S298. doi: 10.1194/jlr.R800047-JLR200
Smith, S., Fernando, T., Wu, P. W., Seo, J., Ní Gabhann, J., Piskareva, O., et al. (2017). MicroRNA-302d targets IRF9 to regulate the IFN-induced gene expression in SLE. J. Autoimmu. 79, 105–111. doi: 10.1016/j.jaut.2017.03.003
Smyth, G. K. (2005). “limma: linear models for microarray data,” in Bioinformatics and Computational Biology Solutions Using R and Bioconductor Statistics for Biology and Health, eds R. Gentleman, V. J. Carey, W. Huber, R. A. Irizarry, and S. Dudoit (New York, NY: Springer), 397–420. doi: 10.1007/0-387-29362-0_23
Smyth, S. S., Cheng, H.-Y., Miriyala, S., Panchatcharam, M., and Morris, A. J. (2008). Roles of lysophosphatidic acid in cardiovascular physiology and disease. Biochim. Biophys. Acta (BBA) Mol. Cell Biol. Lipids 1781, 563–570. doi: 10.1016/j.bbalip.2008.05.008
Szklarczyk, D., Gable, A. L., Lyon, D., Junge, A., Wyder, S., Huerta-Cepas, J., et al. (2019). STRING v11: protein–protein association networks with increased coverage, supporting functional discovery in genome-wide experimental datasets. Nucleic Acids Res. 47, D607–D613. doi: 10.1093/nar/gky1131
Szklarczyk, D., Morris, J. H., Cook, H., Kuhn, M., Wyder, S., Simonovic, M., et al. (2017). The STRING database in 2017: quality-controlled protein–protein association networks, made broadly accessible. Nucleic Acids Res. 45, D362–D368. doi: 10.1093/nar/gkw937
Tan, E. M., Cohen, A. S., Fries, J. F., Masi, A. T., Mcshane, D. J., Rothfield, N. F., et al. (1982). The 1982 revised criteria for the classification of systemic lupus erythematosus. Arthr. Rheum. 25, 1271–1277. doi: 10.1002/art.1780251101
Tang, E. D., Nuñez, G., Barr, F. G., and Guan, K.-L. (1999). Negative regulation of the forkhead transcription factor FKHR by Akt. J. Biol. Chem. 274, 16741–16746. doi: 10.1074/jbc.274.24.16741
Tang, H., Tan, G., Guo, Q., Pang, R., and Zeng, F. (2009). Abnormal activation of the Akt-GSK3β signaling pathway in peripheral blood T cells from patients with systemic lupus erythematosus. Cell Cycle 8, 2789–2793. doi: 10.4161/cc.8.17.9446
Thirumal Kumar, D., Jain, N., Evangeline, J., Kamaraj, B., Siva, R., Zayed, H., et al. (2019). A computational approach for investigating the mutational landscape of RAC-alpha serine/threonine-protein kinase (AKT1) and screening inhibitors against the oncogenic E17K mutation causing breast cancer. Comput. Biol. Med. 115:103513. doi: 10.1016/j.compbiomed.2019.103513
Tsokos, G. C., Lo, M. S., Reis, P. C., and Sullivan, K. E. (2016). New insights into the immunopathogenesis of systemic lupus erythematosus. Nat. Rev. Rheumatol. 12, 716–730. doi: 10.1038/nrrheum.2016.186
Tzivion, G., Dobson, M., and Ramakrishnan, G. (2011). FoxO transcription factors; Regulation by AKT and 14-3-3 proteins. Biochim. Biophy. Acta (BBA) Mol. Cell Res. 1813, 1938–1945. doi: 10.1016/j.bbamcr.2011.06.002
Veeranki, S., and Choubey, D. (2010). Systemic lupus erythematosus and increased risk to develop B cell malignancies: role of the p200-family proteins. Immunol. Lett. 133, 1–5. doi: 10.1016/j.imlet.2010.06.008
Vences-Catalán, F., Rajapaksa, R., Levy, S., and Santos−Argumedo, L. (2012). The CD19/CD81 complex physically interacts with CD38 but is not required to induce proliferation in mouse B lymphocytes. Immunology 137, 48–55. doi: 10.1111/j.1365-2567.2012.03602.x
Wang, J., Liu, Y., Zhao, J., Xu, J., Li, S., and Qin, X. (2017). P-glycoprotein gene MDR1 polymorphisms and susceptibility to systemic lupus erythematosus in Guangxi population: a case-control study. Rheumatol. Int. 37, 537–545. doi: 10.1007/s00296-017-3652-2
Wolfrum, C., Besser, D., Luca, E., and Stoffel, M. (2003). Insulin regulates the activity of forkhead transcription factor Hnf-3β/Foxa-2 by Akt-mediated phosphorylation and nuclear/cytosolic localization. Proc. Natl. Acad. Sci. U.S.A. 100, 11624–11629. doi: 10.1073/pnas.1931483100
Yamauchi, A., and Bloom, E. T. (1997). Control of cell cycle progression in human natural killer cells through redox regulation of expression and phosphorylation of retinoblastoma gene product protein. Blood 89, 4092–4099. doi: 10.1182/blood.V89.11.4092
Yang, L. V., Radu, C. G., Wang, L., Riedinger, M., and Witte, O. N. (2005). Gi-independent macrophage chemotaxis to lysophosphatidylcholine via the immunoregulatory GPCR G2A. Blood 105, 1127–1134. doi: 10.1182/blood-2004-05-1916
Yang, W., and Lau, Y. L. (2015). Solving the genetic puzzle of systemic lupus erythematosus. Pediatr. Nephrol. 30, 1735–1748. doi: 10.1007/s00467-014-2947-8
Yeh, P. Y., Kuo, S.-H., Yeh, K.-H., Chuang, S.-E., Hsu, C.-H., Chang, W. C., et al. (2006). A pathway for tumor necrosis factor-α-induced Bcl10 nuclear translocation Bcl10 is up-regulated by nf-κb and phosphorylated by Akt1 and then complexes with bcl3 to enter the nucleus. J. Biol. Chem. 281, 167–175. doi: 10.1074/jbc.M511014200
Yu, J., Akishita, M., Eto, M., Koizumi, H., Hashimoto, R., Ogawa, S., et al. (2012). Src kinase-mediates androgen receptor-dependent non-genomic activation of signaling cascade leading to endothelial nitric oxide synthase. Biochem. Biophys. Res. Commun. 424, 538–543. doi: 10.1016/j.bbrc.2012.06.151
Zhou, Y., Yuan, J., Pan, Y., Fei, Y., Qiu, X., Hu, N., et al. (2009). T cell CD40LG gene expression and the production of IgG by autologous B cells in systemic lupus erythematosus. Clin. Immunol. 132, 362–370. doi: 10.1016/j.clim.2009.05.011
Keywords: systemic lupus erythematosus, protein–protein interactions, Metacore, microarray and bioinformatics, expression profiling data, biomarkers, functional enrichment analysis
Citation: Udhaya Kumar S, Thirumal Kumar D, Siva R, George Priya Doss C, Younes S, Younes N, Sidenna M and Zayed H (2020) Dysregulation of Signaling Pathways Due to Differentially Expressed Genes From the B-Cell Transcriptomes of Systemic Lupus Erythematosus Patients – A Bioinformatics Approach. Front. Bioeng. Biotechnol. 8:276. doi: 10.3389/fbioe.2020.00276
Received: 06 January 2020; Accepted: 16 March 2020;
Published: 30 April 2020.
Edited by:
Manoj Kumar Kashyap, Amity University Gurgaon, IndiaReviewed by:
Jian Yu, Beihang University, ChinaNoor Ahmad Shaik, King Abdulaziz University, Saudi Arabia
Copyright © 2020 Udhaya Kumar, Thirumal Kumar, Siva, George Priya Doss, Younes, Younes, Sidenna and Zayed. This is an open-access article distributed under the terms of the Creative Commons Attribution License (CC BY). The use, distribution or reproduction in other forums is permitted, provided the original author(s) and the copyright owner(s) are credited and that the original publication in this journal is cited, in accordance with accepted academic practice. No use, distribution or reproduction is permitted which does not comply with these terms.
*Correspondence: C. George Priya Doss, Z2VvcmdlcHJpeWFkb3NzQHZpdC5hYy5pbg==; Hatem Zayed, aGF0ZW0uemF5ZWRAcXUuZWR1LnFh