- 1Department of Cardiology, Shanghai Tenth People’s Hospital, Tongji University School of Medicine, Shanghai, China
- 2Department of Pediatric Cardiovascular, Xin Hua Hospital, School of Medicine, Shanghai Jiao Tong University, Shanghai, China
- 3Department of Cardiology, Shanghai Tongji Hospital, Tongji University School of Medicine, Shanghai, China
- 4Shanghai East Hospital of Clinical Medical College, Nanjing Medical University, Shanghai, China
- 5Department of Cardiovascular Surgery, Shanghai East Hospital, Tongji University School of Medicine, Shanghai, China
- 6Department of Cardiovascular Surgery, National Clinical Research Center for Geriatric, West China Hospital, Sichuan University, Chengdu, China
- 7Department of Cardiovascular Surgery, Zhongshan Hospital, Fudan University, Shanghai, China
Atrial fibrillation (AF) is one of the most prevalent heart rhythm disorder. The causes of AF include age, male sex, diabetes, hypertension, valve disease, and systolic/diastolic dysfunction. But on molecular level, its mechanisms are largely unknown. In this study, we collected 10 patients with persistent atrial fibrillation, 10 patients with paroxymal atrial fibrillation and 10 healthy individuals and did Methylation EPICBead Chip and RNA sequencing. By analyzing the methylation and gene expression data using machine learning based feature selection method Boruta, we identified the key genes that were strongly associated with AF and found their interconnections. The results suggested that the methylation of KIF15 may regulate the expression of PSMC3, TINAG, and NUDT6. The identified AF associated methylation-expression regulations may help understand the molecular mechanisms of AF from a multi-omics perspective.
Introduction
Atrial fibrillation (AF), one of the most prevalent heart rhythm disorders, is a potential cause of heart failure and ischemic stroke with high morbidity and mortality (Ogawa et al., 2017; Asmarats et al., 2019). The cause of AF is multifactorial which include age, male sex, diabetes, hypertension, valve disease, and systolic/diastolic dysfunction (Schnabel et al., 2009; Lip et al., 2013; Voukalis et al., 2016). Depends on how often atrial fibrillation occurs and how it responds to treatment, AF is roughly divided into two major subtypes-paroxysmal atrial fibrillation (PAF) and persistent atrial fibrillation (PeAF). In the treatment of AF, drugs were the first choice, non-drug therapies were used only when drug therapy failed or patients could not tolerate the medication. In contrast to the extensive knowledge of etiology, the underlying mechanism of AF remains elusive. Further study of the potential mechanisms of AF could provide novel strategies for the treatment and management to increase quality of life and reduce economic burden of social (Chugh et al., 2001).
With the development of next generation sequencing (NGS) technologies, growing evidence have demonstrated that AF is a disease with a significant genetic contribution. Previous studies have filtered novel genetic variants and candidate genes including transcriptional factor genes (PITX2, PRRX1, ZFHX3, NKX2.5, TBX5), ion channel genes (KCNN3, HCN4, CACNA1C, SCN5A, KCNQ1, KCNH2), and caveolin genes (CAV1 and CAV2) (Ellinor et al., 2010; Olesen et al., 2014; Sinner et al., 2014; Ma et al., 2016; Nielsen et al., 2018). However, these genes explain only a small fraction of the biology and genetic underpinnings of AF.
Epidemiological studies have demonstrated that genetic, environmental, behavioral, and clinical factors contribute to AF pathogenic mechanism (Zhong et al., 2016). Emelia J. B performed genome-wide methylation using whole blood samples from 183 prevalent AF and 220 incident AF cases. They examined the association between DNA methylation and GWAS loci, suggesting DNA methylation might be a possible mechanism through which AF-specific genetic variations affect gene regulation (Lin et al., 2017). To date, only a few studies have investigated differential DNA methylation as a predictor biomarker at specific candidate loci that were previously associated with AF.
Therefore, we applied DNA methylation profiling study to identify the likely rare damaging variants and putative candidate genes from 10 patients with persistent atrial fibrillation (PeAF), 10 patients with paroxymal atrial fibrillation (PAF) and 10 healthy individuals. Interestingly, we identified top 10 genes (KIF15, ABCA3, FOXG1, VGF, PDE4D, EIF3C, CNTNAP5, SHOX2, VGF, TRIM59) as functional candidate genes and the expression level are significantly increased in PeAF and PAF patients than control. Given the importance of DNA methylation to gene expression, we investigated the gene expression of the same participants using RNA sequencing. We also defined top 10 genes (EPN3, EMD, SMCO4, F2RL2, TMED1, PSMC3, PDZD11, NUDT6, TINAG, GALNT5) in gene expression data and the expression pattern of these genes was significantly different between PeAF and PAF. These results have improved our understanding of the underlying mechanism and offer new insights into the potential pathway of AF, which could provide novel therapeutic option for this disease.
Materials and Methods
Atrial Fibrillation Patients
Ten patients with paroxymal atrial fibrillation (g1), 10 patients with persistent atrial fibrillation (continuous atrial fibrillation lasting more than 12 months) (g2) and 10 healthy individuals (g3) were enrolled in this study (Table 1). All patients were subjected to detailed medical evaluation, which included medical history, physical examination, electrocardiography (ECG), and echocardiography. Patients with chronic heart failure, coronary heart disease, cardiomyopathy, hyperthyroidism or chronic pulmonary heart disease were excluded.
The study was conducted in accordance with the Declaration of Helsinki, and the protocol used to collect human heart tissue was approved by the Ethics Committee of Shanghai East Hospital (DI: 0402017).
Written informed consents to participate in this study were provided by all the enrolled patients before operation of fibrillation ablation. The left atrial appendage tissues which were abandoned during isolated surgical ablation were collected. Normal left atrial appendages were collected from healthy male donors.
The Methylation Profiles
The DNA methylation status of 850K probes in the 30 samples was measured using Methylation EPICBead Chip. The raw data was quality controlled and preprocessed using R/Bioconductor package minfi1 (Aryee et al., 2014). The beta value ranged from 0 to 1 was calculated to represent how each position was methylated. 1 meant high methylation and 0 meant low methylation.
The RNA Sequencing Profiles
The total RNAs were extracted using RNeasy Mini Kit (Cat#74106, Qiagen) and the RNA integrity was checked using Agilent Bioanalyzer 2100 (Agilent technologies, Santa Clara, CA, United States). Qualified total RNA was further purified by RNAClean XP Kit (Cat A63987, Beckman Coulter Inc., Kraemer Boulevard, Brea, CA, United States) and RNase-Free DNase Set (Cat#79254, QIAGEN, GmBH, Germany). Pair-end sequencing reads were generated using Illumina data collection software. First, the reads were mapped onto human reference genome GRCh38 using Hisat2 (version:2.0.42) (Kim et al., 2015). Then, Stringtie (version:1.3.03) (Pertea et al., 2015) was used to calculate the FPKM (Fragments Per Kilobase of exon model per Million mapped reads).
Feature Selection Algorithm
There were 866,091 methylation probes and 50,868 RNAs. The number of features were extremely large. It was difficult to select key features using traditional statistical methods. Therefore, we adopted the latest machine learning based feature selection method Boruta to get the key methylation probes and RNAs.
Boruta (Kursa and Rudnicki, 2010) is a feature selection method based on random forest. It can select sample group relevant features effectively. First, it will shuffle the features to create many permuted datasets. Then, it will evaluate the importance score of each feature in the original actual dataset and permuted datasets. Then, it will compare the actual importance score with permuted scores and find the features with significantly higher actual importance scores than permuted scores. After multiple iterations, it will select all the sample group relevant features. The python code from https://github.com/scikit-learn-contrib/boruta_py was used to apply the Boruta feature selection algorithm.
Classification Predictor
To evaluate how well the selected features can classify the samples, we built an SVM (Support Vector Machine) classifier using the methylation data and another RNA-Seq data based SVM classifier. The svm function in R package e101714 (Chang and Lin, 2011) was used to apply the SVM classification algorithm.
LOOCV (leave-one-out cross validation) was used to objectively evaluate the classification performance. Each time, one sample was treated as test sample while all the other samples were used to train the model. After 30 rounds, all samples had been tested once and the overall accuracy was calculated based on the confusion matrix. In confusion matrix, the actual sample groups were compared with predicted sample groups.
Results
The Key Methylation Features Identified With Boruta
We ran Boruta feature selection algorithm on the methylation data and got 10 key methylation features. These 10 key methylation features were listed in Table 2. The probes were annotated to genome positions (Genome Build 37) and genes using the official annotation file from Illumina. Sometime, one position may be associated with multiple genes. Therefore, the 10 methylation probes can be mapped onto 15 gene symbols. We checked the GO annotation of these genes and found that cg16703882 (SHOX2) was associated with GO:0007507: heart development which was closely relevant to AF.
We plotted the heatmap of these 10 key methylation features in Figure 1. It can be seen that most of the 10 patients with paroxymal atrial fibrillation (g1), 10 patients with persistent atrial fibrillation (g2) and 10 healthy individuals (g3) were cluster into the right groups.
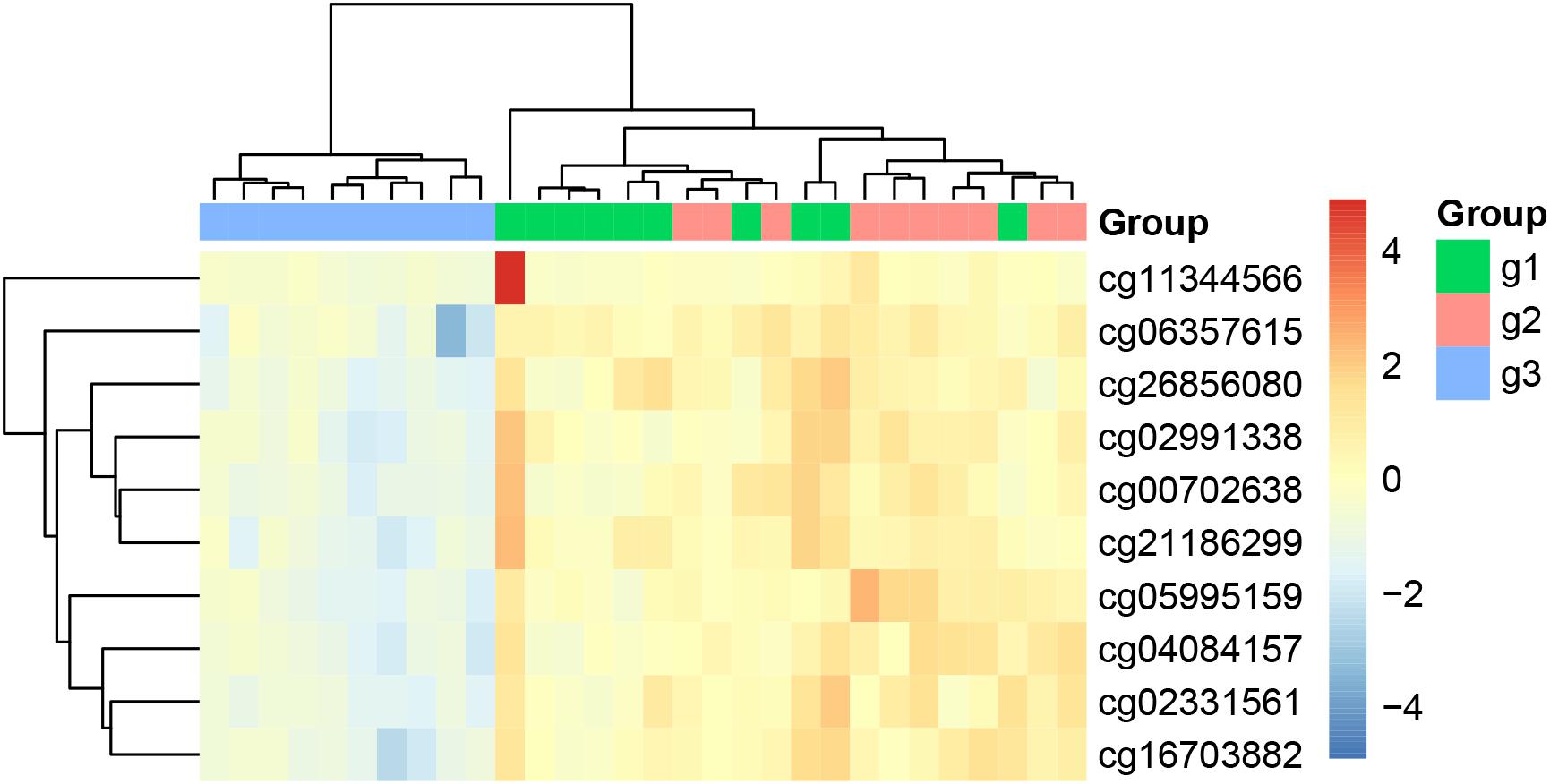
Figure 1. The heatmap of the key methylation features. The 30 samples were from three groups: 10 patients with paroxymal atrial fibrillation (g1), 10 patients with persistent atrial fibrillation (g2), and 10 healthy individuals (g3). It can be seen that most samples were cluster into the right groups.
The Key Gene Expression Features Identified With Boruta
Similarly, we ran Boruta feature selection algorithm on the RNA-Seq gene expression data and got 10 key gene expression features. The 10 key gene expression features were given in Table 3. We also plotted their heatmap in Figure 2. The clusters were also largely correct.
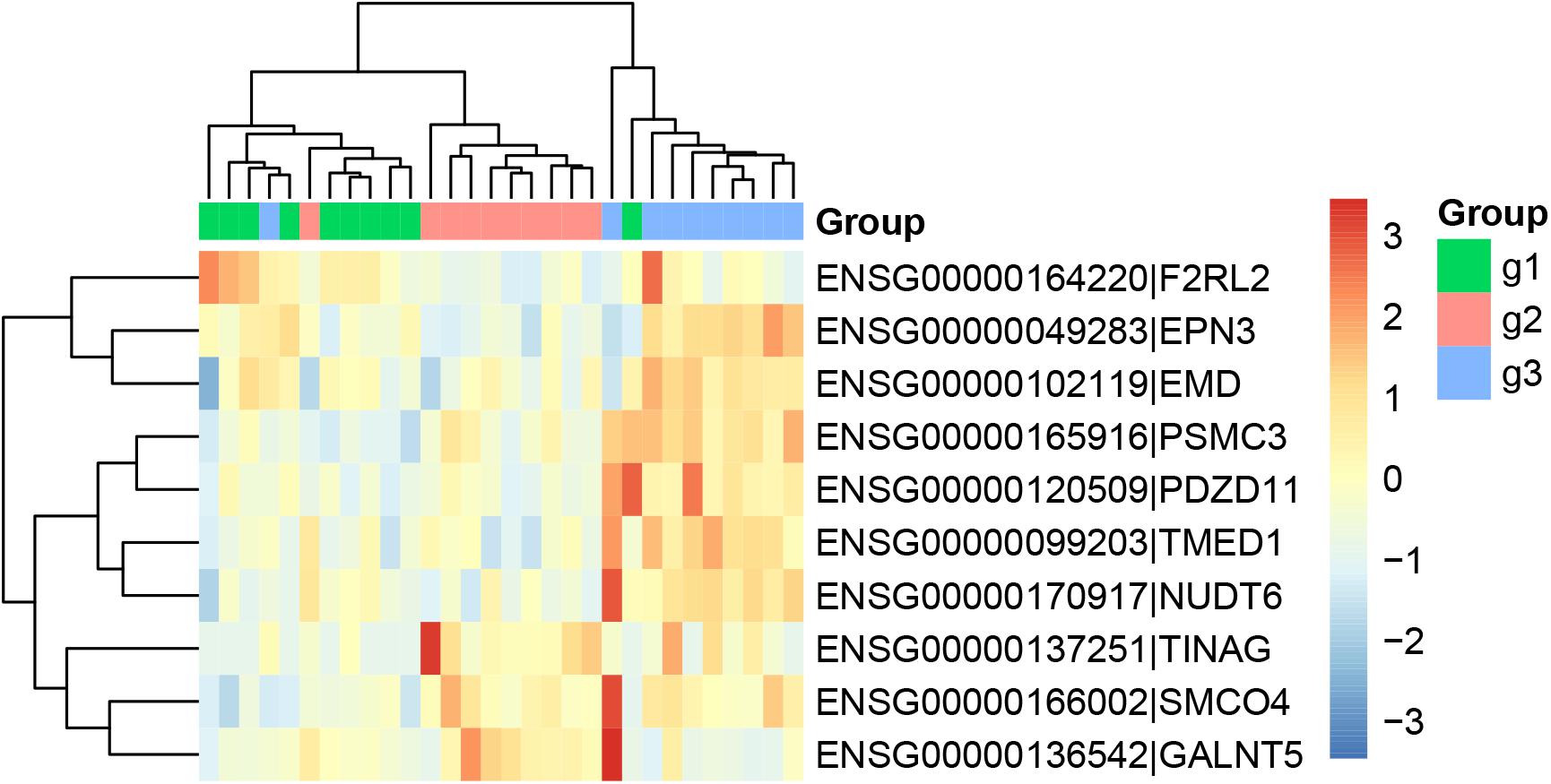
Figure 2. The heatmap of the key gene expression features. The 30 samples were from three groups: 10 patients with paroxymal atrial fibrillation (g1), 10 patients with persistent atrial fibrillation (g2), and 10 healthy individuals (g3). It can be seen that most samples were cluster into the right groups.
The Classification Performance of Methylation and Gene Expression
From Figures 1, 2, we can see that both methylation and gene expression features can correctly cluster most samples. But we would like to evaluate their performance objectively and quantitatively. Therefore, we applied LOOCV to test the SVM classifiers of methylation and gene expression features. The confusion matrixes of methylation features and gene expression features were listed in Tables 4, 5, respectively.
From Table 3, we can see that the AF patients (g1 + g2) and healthy individuals (g3) were perfectly classified using the methylation features, but the methylation data did not have great performance on classifying the subtype of AF (g1 and g2). From Table 4, we can see that the two subtype of AF, paroxysmal atrial fibrillation (g1) and persistent atrial fibrillation (g2), had very different gene expression pattern. In other words, the methylation data and gene expression data complement each other. The methylation data can be used to predict the AF and the gene expression data can be used to classify the subtypes of AF.
We checked the wrongly predicted samples in Tables 3, 4. They were different. Within the 30 samples, 22 samples had the same predicted labels by expression and methylation. All these 22 samples were correctly predicted. For the 8 inconsistent samples between expression and methylation predictions, at least one of the two predictions (the expression-based prediction and the methylation-based prediction) was correct. In other words, all the samples can be corrected classified based on either expression or methylation. The expression-based prediction and the methylation-based prediction were complementary.
The Methylation-Expression Regulation Network
We mapped the genes of methylation features and gene expression features to the STRING network (Version 11.05) (Szklarczyk et al., 2018) and visualized the network using R package igraph (Csardi and Nepusz, 2006)6 to identify the potential relationship between two candidate genes sets. The methylation-expression regulation network was shown in Figure 3. In the network, the methylation and expression genes were marked in red and green. The methylation genes located in three clusters: EIF3CL-EIF3C, KIF15-TRIM59, and SHOX2-FOXG1-CNTNAP5. The three expression genes (PSMC3, TINAG, and NUDT6) were connected with methylation gene KIF15. Even EPN3 can be indirectly connected to KIF15. That made KIF15 at the center of the network. These results suggested that KIF5 may play important roles in the pathogenesis of AF.
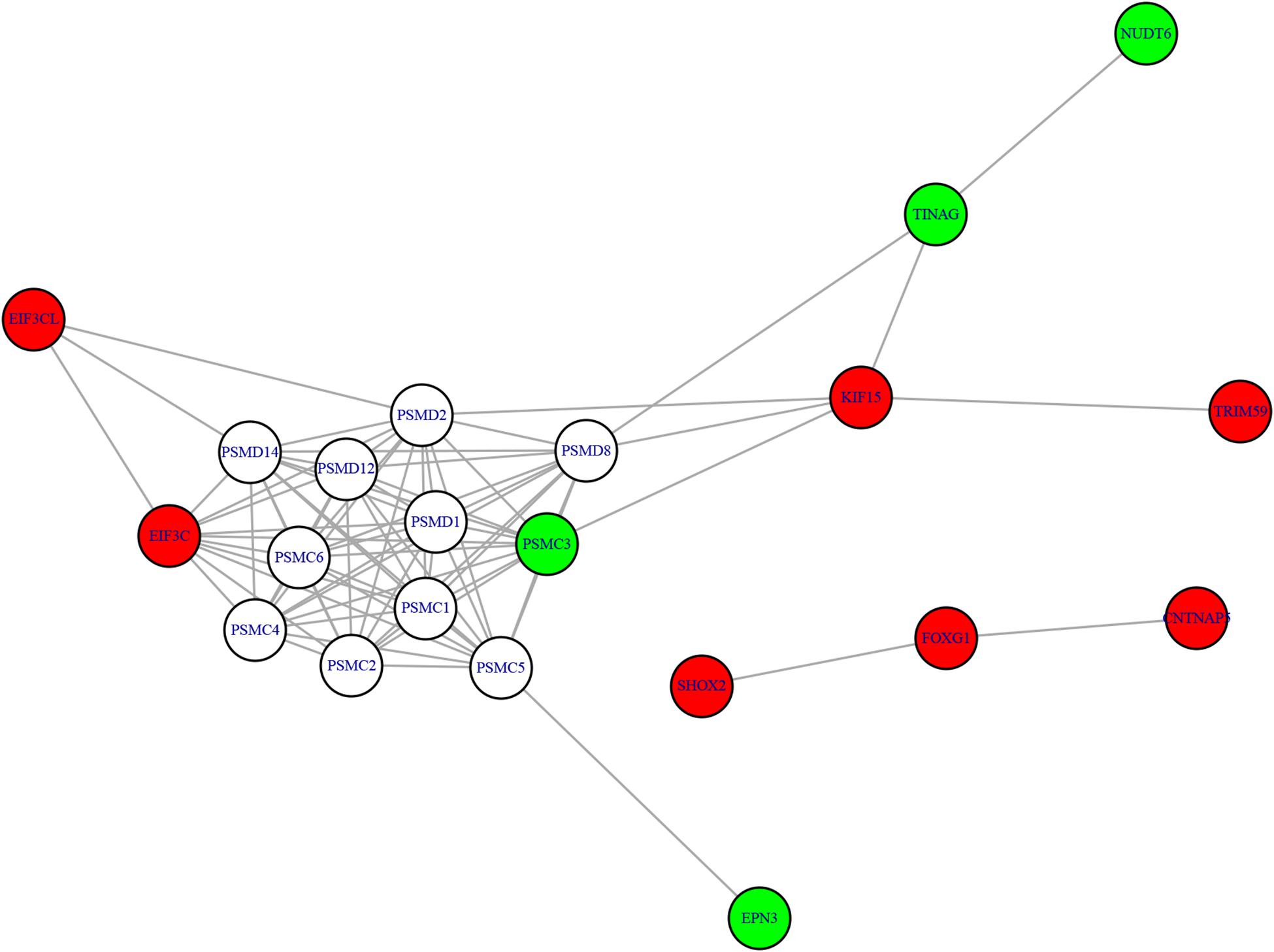
Figure 3. The methylation-expression regulation network. The red and green nodes were methylation and expression genes, respectively. The methylation genes located in three clusters: EIF3CL-EIF3C, KIF15-TRIM59, and SHOX2-FOXG1-CNTNAP5. KIF15 can directly or indirectly regulate the expression genes and may play important roles.
Discussion
DNA methylation, a pre-transcriptional modification characterized by the addition of methyl groups to specific nucleotides, regulates the stability of gene expression states and maintains genome integrity by collaborating with proteins that modify nucleosomes (Ma et al., 2014; Tao et al., 2016; Shen et al., 2017). Previous studies considered that changes in DNA methylation states contribute to the regulation of biological processes underlying AF, such as fibrosis, atrial dilatation, atrial fibroblast proliferation and differentiation from fibroblasts into myofibroblasts (Zhao et al., 2017). To further enhance the biological understanding of the atrial fibrillation, our study focused on DNA methylation, particularly with respect to how it relates to mRNA expression. Among our two gene sets of top 10 genes, we found PDED4, SHOX2, and EMD were the most important genes for AF which have been reported associated with AF in previous reference.
Atrial fibrillation is reported to be associated with a profound remodeling of membrane receptors and alterations in cAMP dependent regulation of Ca2+ handling. PDE4 is expressed in human atrial myocytes and accounts for approximately 15% of PDE (phosphodiesterase) activity (Molina et al., 2012). PDE4D encoded protein has 3’,5’-cyclic-AMP phosphodiesterase activity and degrades cAMP, which acts as a signal transduction molecule in multiple cell types and represents the major PDE4 subtype (Berk et al., 2016). The activity of PDEs decreased with age, and the relative PDED4 activity was lower in patients with permanent atrial fibrillation than in age-matched sinus rhythm controls (Milton et al., 2011). Previous study provided evidence that patients with pAF were found to have a decreased PDE4 activity as compared with patients in sinus rhythm (Yeh et al., 2007).
Short Stature Homeobox 2 (SHOX2) is a member of the homeobox family of genes in which mutations associated with early-onset and familial AF (Hoffmann et al., 2019). SHOX2 is considered as a key regulator of sinus node development of which deficiency could lead to bradycardia in animal models (Vicente-Steijn et al., 2017). Previous study demonstrated SHOX2 was susceptible for SND and AF by screening 98 SND patients and 450 individuals with AF. In the heart development of mouse and zebrafish, they also proved SHOX2 plays an important role, the mutation of SHOX2 could lead to severe bradycardia (Blaschke et al., 2007; Ye et al., 2015).
Emerin (EMD) encodes a serine-rich nuclear membrane protein which located on the cytoplasmic surface of the inner nuclear membrane and related to X-linked Emery-Dreifuss muscular dystrophy (EDMD) (Capanni et al., 2009). Previous study found a nonsense mutation in EMD from two EDMD families which is associated with X-linked recessive inheritance, result in serious cardiac complication, including AF (Sakata et al., 2005). Cardiologic assessment revealed slow atrial fibrillation in a recent case of a 65-year-old male patient with a hemizygous duplication of 5 bases in exon 6 of the EMD, gene on the X chromosome (Kissel et al., 2009; Zhao et al., 2014; Brisset et al., 2019).
Data Availability Statement
The raw data supporting the conclusions of this article will be made available by the authors, without undue reservation, to any qualified researcher.
Ethics Statement
The studies involving human participants were reviewed and approved by the Ethics Committee of Shanghai East Hospital. The patients/participants provided their written informed consent to participate in this study. Written informed consent was obtained from the individual(s) for the publication of any potentially identifiable images or data included in this manuscript.
Author Contributions
YZ and CG conceived and designed the experiments. BL, XS, and KD performed the experiments. ML, YQ, and SZ analyzed the data. BL, XS, and YZ wrote the manuscript. All authors read and approved the final version of the manuscript.
Funding
The present study was funded by the National Key Research and Development Program (Grant No. 2018-YFC-1312505 to YZ), the National Natural Science Foundation of China (Grant No. 81770408 to CG), and (Grant No. 81900280 to XS).
Conflict of Interest
The authors declare that the research was conducted in the absence of any commercial or financial relationships that could be construed as a potential conflict of interest.
Footnotes
- ^ https://bioconductor.org/packages/minfi/
- ^ http://ccb.jhu.edu/software/hisat2/
- ^ http://ccb.jhu.edu/software/stringtie/
- ^ https://CRAN.R-project.org/package=e1071
- ^ http://string-db.org
- ^ https://CRAN.R-project.org/package=igraph
References
Aryee, M. J., Jaffe, A. E., Corrada-Bravo, H., Ladd-Acosta, C., Feinberg, A. P., Hansen, K. D., et al. (2014). Minfi: a flexible and comprehensive bioconductor package for the analysis of infinium DNA methylation microarrays. Bioinformatics 30, 1363–1369. doi: 10.1093/bioinformatics/btu049
Asmarats, L., Cruz-Gonzalez, I., Nombela-Franco, L., Arzamendi, D., Peral, V., Nietlispach, F., et al. (2019). Recurrence of device-related thrombus after percutaneous left atrial appendage closure. Circulation 140, 1441–1443. doi: 10.1161/CIRCULATIONAHA.119.040860
Berk, E., Christ, T., Schwarz, S., Ravens, U., Knaut, M., and Kaumann, A. J. (2016). In permanent atrial fibrillation, PDE3 reduces force responses to 5-HT, but PDE3 and PDE4 do not cause the blunting of atrial arrhythmias. Br. J. Pharmacol. 173, 2478–2489. doi: 10.1111/bph.13525
Blaschke, R. J., Hahurij, N. D., Kuijper, S., Just, S., Wisse, L. J., Deissler, K., et al. (2007). Targeted mutation reveals essential functions of the homeodomain transcription factor Shox2 in sinoatrial and pacemaking development. Circulation 115, 1830–1838. doi: 10.1161/CIRCULATIONAHA.106.637819
Brisset, M., Ben Yaou, R., Carlier, R. Y., Chanut, A., Nicolas, G., Romero, N. B., et al. (2019). X-linked Emery-Dreifuss muscular dystrophy manifesting with adult onset axial weakness, camptocormia, and minimal joint contractures. Neuromuscul. Disord. 29, 678–683. doi: 10.1016/j.nmd.2019.06.009
Capanni, C., Del Coco, R., Mattioli, E., Camozzi, D., Columbaro, M., Schena, E., et al. (2009). Emerin-prelamin a interplay in human fibroblasts. Biol. Cell 101, 541–554. doi: 10.1042/BC20080175
Chang, C.-C., and Lin, C.-J. (2011). LIBSVM: a library for support vector machines. ACM Trans. on Intell. Syst. Technol. 2, 1–27. doi: 10.1145/1961189.1961199
Chugh, S. S., Blackshear, J. L., Shen, W. K., Hammill, S. C., and Gersh, B. J. (2001). Epidemiology and natural history of atrial fibrillation: clinical implications. J. Am. Coll. Cardiol. 37, 371–378. doi: 10.1016/s0735-1097(00)01107-4
Csardi, G., and Nepusz, T. (2006). The igraph software package for complex network research. Inter J. Complex Syst. 1695, 1–9.
Ellinor, P. T., Lunetta, K. L., Glazer, N. L., Pfeufer, A., Alonso, A., Chung, M. K., et al. (2010). Common variants in KCNN3 are associated with lone atrial fibrillation. Nat. Genet. 42, 240–244. doi: 10.1038/ng.537
Hoffmann, S., Paone, C., Sumer, S. A., Diebold, S., Weiss, B., Roeth, R., et al. (2019). Functional characterization of rare variants in the shox2 gene identified in sinus node dysfunction and atrial fibrillation. Front. Genet. 10:648. doi: 10.3389/fgene.2019.00648
Kim, D., Langmead, B., and Salzberg, S. L. (2015). HISAT: a fast spliced aligner with low memory requirements. Nat. Methods 12, 357–360. doi: 10.1038/nmeth.3317
Kissel, J. T., Dimberg, E. L., Emslie-Smith, A. M., Selcen, D., and Keegan, B. M. (2009). A 49-year-old man with contractures, weakness, and cardiac arrhythmia. Neurology 72, 2036–2043. doi: 10.1212/01.wnl.0000350522.42635.09
Kursa, M., and Rudnicki, W. (2010). Feature selection with the boruta package. J. Statist. Softw. Artic. 36, 1–13. doi: 10.18637/jss.v036.i11
Lin, H., Yin, X., Xie, Z., Lunetta, K. L., Lubitz, S. A., Larson, M. G., et al. (2017). Methylome-wide association study of atrial fibrillation in framingham heart study. Sci. Rep. 7:40377. doi: 10.1038/srep40377
Lip, G. Y. H., Lane, D. A., Buller, H., and Apostolakis, S. (2013). Development of a novel composite stroke and bleeding risk score in patients with atrial fibrillation: the AMADEUS study. Chest 144, 1839–1847. doi: 10.1378/chest.13-1635
Ma, B., Wilker, E. H., Willis-Owen, S. A., Byun, H. M., Wong, K. C., Motta, V., et al. (2014). Predicting DNA methylation level across human tissues. Nucleic Acids Res. 42, 3515–3528. doi: 10.1093/nar/gkt1380
Ma, J. F., Yang, F., Mahida, S. N., Zhao, L., Chen, X., Zhang, M. L., et al. (2016). TBX5 mutations contribute to early-onset atrial fibrillation in chinese and caucasians. Cardiovasc. Res. 109, 442–450. doi: 10.1093/cvr/cvw003
Milton, A. G., Aykanat, V. M., Hamilton-Bruce, M. A., Nezic, M., Jannes, J., and Koblar, S. A. (2011). Association of the phosphodiesterase 4D (PDE4D) gene and cardioembolic stroke in an Australian cohort. Int. J. Stroke 6, 480–486. doi: 10.1111/j.1747-4949.2011.00616.x
Molina, C. E., Leroy, J., Richter, W., Xie, M., Scheitrum, C., Lee, I. O., et al. (2012). Cyclic adenosine monophosphate phosphodiesterase type 4 protects against atrial arrhythmias. J. Am. Coll. Cardiol. 59, 2182–2190. doi: 10.1016/j.jacc.2012.01.060
Nielsen, J. B., Thorolfsdottir, R. B., Fritsche, L. G., Zhou, W., Skov, M. W., Graham, S. E., et al. (2018). Biobank-driven genomic discovery yields new insight into atrial fibrillation biology. Nat. Genet. 50, 1234–1239. doi: 10.1038/s41588-018-0171-3
Ogawa, H., Senoo, K., An, Y., Shantsila, A., Shantsila, E., Lane, D. A., et al. (2017). Clinical features and prognosis in patients with atrial fibrillation and prior stroke: comparing the fushimi and darlington AF registries. eBio Med. 18, 199–203. doi: 10.1016/j.ebiom.2017.03.022
Olesen, M. S., Nielsen, M. W., Haunso, S., and Svendsen, J. H. (2014). Atrial fibrillation: the role of common and rare genetic variants. Eur. J. Hum. Genet. 22, 297–306. doi: 10.1038/ejhg.2013.139
Pertea, M., Pertea, G. M., Antonescu, C. M., Chang, T.-C., Mendell, J. T., and Salzberg, S. L. (2015). StringTie enables improved reconstruction of a transcriptome from RNA-seq reads. Nat. Biotechnol. 33, 290–295. doi: 10.1038/nbt.3122
Sakata, K., Shimizu, M., Ino, H., Yamaguchi, M., Terai, H., Fujino, N., et al. (2005). High incidence of sudden cardiac death with conduction disturbances and atrial cardiomyopathy caused by a nonsense mutation in the STA gene. Circulation 111, 3352–3358. doi: 10.1161/CIRCULATIONAHA.104.527184
Schnabel, R. B., Sullivan, L. M., Levy, D., Pencina, M. J., Massaro, J. M., D’Agostino, R. B., et al. (2009). Development of a risk score for atrial fibrillation (Framingham Heart Study): a community-based cohort study. Lancet 373, 739–745. doi: 10.1016/S0140-6736(09)60443-8
Shen, K., Tu, T., Yuan, Z., Yi, J., Zhou, Y., Liao, X., et al. (2017). DNA methylation dysregulations in valvular atrial fibrillation. Clin. Cardiol. 40, 686–691. doi: 10.1002/clc.22715
Sinner, M. F., Tucker, N. R., Lunetta, K. L., Ozaki, K., Smith, J. G., Trompet, S., et al. (2014). Integrating genetic, transcriptional, and functional analyses to identify 5 novel genes for atrial fibrillation. Circulation 130, 1225–1235. doi: 10.1161/CIRCULATIONAHA.114.009892
Szklarczyk, D., Gable, A. L., Lyon, D., Junge, A., Wyder, S., Huerta-Cepas, J., et al. (2018). STRING v11: protein-protein association networks with increased coverage, supporting functional discovery in genome-wide experimental datasets. Nucleic Acids Res. 47, D607–D613. doi: 10.1093/nar/gky1131
Tao, H., Shi, K. H., Yang, J. J., and Li, J. (2016). Epigenetic mechanisms in atrial fibrillation: new insights and future directions. Trends Cardiovasc. Med. 26, 306–318. doi: 10.1016/j.tcm.2015.08.006
Vicente-Steijn, R., Kelder, T. P., Tertoolen, L. G., Wisse, L. J., Pijnappels, D. A., Poelmann, R. E., et al. (2017). RHOA-ROCK signalling is necessary for lateralization and differentiation of the developing sinoatrial node. Cardiovasc. Res. 113, 1186–1197. doi: 10.1093/cvr/cvx104
Voukalis, C., Lip, G. Y., and Shantsila, E. (2016). Emerging Tools for Stroke Prevention in Atrial Fibrillation. eBio Med. 4, 26–39. doi: 10.1016/j.ebiom.2016.01.017
Ye, W., Wang, J., Song, Y., Yu, D., Sun, C., Liu, C., et al. (2015). A common Shox2-Nkx2-5 antagonistic mechanism primes the pacemaker cell fate in the pulmonary vein myocardium and sinoatrial node. Development 142, 2521–2532. doi: 10.1242/dev.120220
Yeh, Y. H., Ehrlich, J. R., Qi, X., Hebert, T. E., Chartier, D., and Nattel, S. (2007). Adrenergic control of a constitutively active acetylcholine-regulated potassium current in canine atrial cardiomyocytes. Cardiovasc. Res. 74, 406–415. doi: 10.1016/j.cardiores.2007.01.020
Zhao, G., Zhou, J., Gao, J., Liu, Y., Gu, S., Zhang, X., et al. (2017). Genome-wide DNA methylation analysis in permanent atrial fibrillation. Mol. Med. Rep. 16, 5505–5514. doi: 10.3892/mmr.2017.7221
Zhao, J., Liu, T., and Li, G. (2014). Relationship between two arrhythmias: sinus node dysfunction and atrial fibrillation. Arch. Med. Res. 45, 351–355. doi: 10.1016/j.arcmed.2014.04.005
Keywords: atrial fibrillation, methylation, multi-omics, feature selection, classification
Citation: Liu B, Shi X, Ding K, Lv M, Qian Y, Zhu S, Guo C and Zhang Y (2020) The Joint Analysis of Multi-Omics Data Revealed the Methylation-Expression Regulations in Atrial Fibrillation. Front. Bioeng. Biotechnol. 8:187. doi: 10.3389/fbioe.2020.00187
Received: 19 December 2019; Accepted: 26 February 2020;
Published: 12 March 2020.
Edited by:
Peilin Jia, The University of Texas Health Science Center at Houston (UTHealth), United StatesReviewed by:
Yan Yao, Chinese Academy of Medical Sciences, ChinaJian Zhong Zhou, The First Affiliated Hospital of Chongqing Medical University, China
Copyright © 2020 Liu, Shi, Ding, Lv, Qian, Zhu, Guo and Zhang. This is an open-access article distributed under the terms of the Creative Commons Attribution License (CC BY). The use, distribution or reproduction in other forums is permitted, provided the original author(s) and the copyright owner(s) are credited and that the original publication in this journal is cited, in accordance with accepted academic practice. No use, distribution or reproduction is permitted which does not comply with these terms.
*Correspondence: Changfa Guo, Z3VvLmNoYW5nZmFAenMtaG9zcGl0YWwuc2guY24=; Yangyang Zhang, emhhbmd5YW5neWFuZ193eUB2aXAuc2luYS5jb20=; emhhbmd5YW5neWFuZ193eUB0b25namkuZWR1LmNu
†These authors have contributed equally to this work