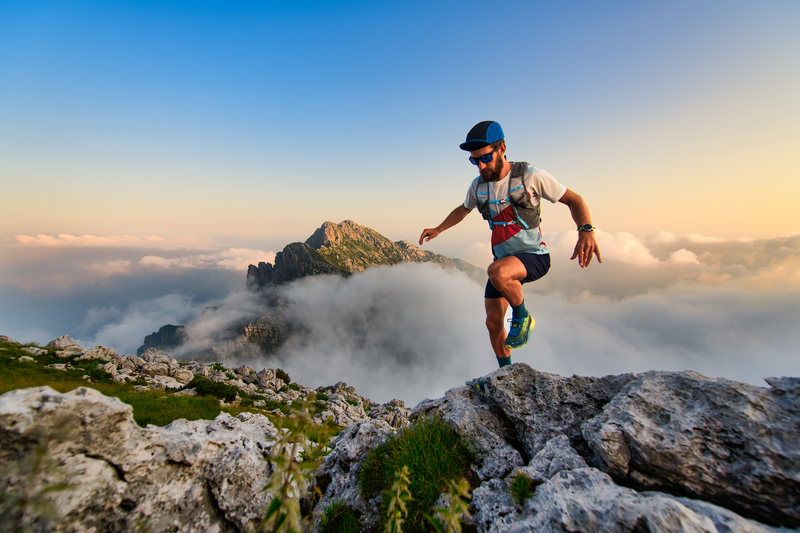
94% of researchers rate our articles as excellent or good
Learn more about the work of our research integrity team to safeguard the quality of each article we publish.
Find out more
ORIGINAL RESEARCH article
Front. Bioeng. Biotechnol. , 14 November 2019
Sec. Computational Genomics
Volume 7 - 2019 | https://doi.org/10.3389/fbioe.2019.00339
This article is part of the Research Topic Computational Methods in Inferring Cancer Tissue-of-Origin and Cancer Molecular Classification, Volume I View all 20 articles
Isocitrate dehydrogenase (IDH) is an oncogene, and the expression of a mutated IDH promotes cell proliferation and inhibits cell differentiation. IDH exists in three different isoforms, whose mutation can cause many solid tumors, especially gliomas in adults. No effective method for classifying gliomas on genetic signatures is currently available. DNA methylation may be applied to distinguish cancer cells from normal tissues. In this study, we focused on three subtypes of IDH-mutation gliomas by examining methylation data. Several advanced computational methods were used, such as Monte Carlo feature selection (MCFS), incremental feature selection (IFS), support machine vector (SVM), etc. The MCFS method was adopted to analyze methylation features, resulting in a feature list. Then, the IFS method incorporating SVM was applied to the list to extract important methylation features and construct an optimal SVM classifier. As a result, several methylation features (sites) were found to relate to glioma subclasses, which are annotated onto multiple genes, such as FLJ37543, LCE3D, FAM89A, ADCY5, ESR1, C2orf67, REST, EPHA7, etc. These genes are enriched in biological functions, including cellular developmental process, neuron differentiation, cellular component morphogenesis, and G-protein-coupled receptor signaling pathway. Our results, which are supported by literature reports and independent dataset validation, showed that our identified genes and functions contributed to the detailed glioma subtypes. This study provided a basic research on IDH-mutation gliomas.
Isocitrate dehydrogenase (IDH) exists in three different isoforms. IDH1 and DH2 catalyze the same reaction and use NADP+ as a cofactor instead of NAD+. IDH3 converts NAD+ to NADH in the mitochondria. IDH is an oncogene, and the expression of mutated IDH promotes cell proliferation and inhibits cell differentiation. Mutant IDH-derived (R)-2HG is a potential malignant substance and unwanted byproduct of cellular metabolism. 2HG dehydrogenase (2HGDH) prevents 2HG from accumulating in cells, and its intracellular levels in normal cells are maintained at <0.1 mM. The transformation induced by (R)-2HG is effective and reversible, suggesting that inhibiting 2HG has efficacy in the treatment of IDH mutant cancers. Mutations at Arg132 of IDH1 are present in five of six secondary glioblastoma (GBM) subtypes, and IDH mutations have been found in many other solid tumors (Losman and Kaelin, 2013).
Glioma in adults includes three main categories, namely, glioblastoma (GBM), astrocytoma, and oligodendroglioma. They are determined by genetic and histologic features. IDH1 and IDH2 mutations are generally detected in astrocytoma and oligodendroglioma but not in the GBM subtype. Thus, IDH-mutation is an important marker for glioma classification. Different subtypes of glioma have different mutation patterns. Mutations in ATRX and TP53 are usually identified in astrocytomas with mutant IDH, but TRET promoter variations and chromosome abnormality are generally identified in oligodendrogliomas (O-IDH) (Cancer Genome Atlas Research Network et al., 2015). Thus, A-IDH and O-IDH are two major subtypes of IDH-mutant gliomas distinguished by co-occurring genetic signatures and histopathology (Venteicher et al., 2017).
No effective method for classifying gliomas on genetic signatures is currently available. By contrast, DNA methylation is used to distinguish cancer cells from normal tissues (Delpu et al., 2013). DNA methylation is a part of the normal epigenetic modification with potential regulatory significance, such as regulating gene expression patterns. In this study, we focused on three subtypes of IDH-mutation gliomas by methylation data, including astrocytomas with IDH mutations (A-IDH), astrocytoma with IDH mutation and enriched HG (A-IDH-HG), and oligodendrogliomas with IDH mutations (O-IDH). Our analyzing procedures used several advanced computational methods, like Monte Carlo feature selection (MCFS; Draminski et al., 2008), incremental feature selection (IFS; Liu and Setiono, 1998), and support machine vector (SVM; Cortes and Vapnik, 1995), etc. A feature list was produced by applying the MCFS method on the methylation data. Then, the IFS method followed to extract important methylation features by evaluating the performance of SVM on different feature subsets that consisted of top features in the list. As a result, we accessed some key methylation features (sites) related to the classification of gliomas annotated onto multiple genes, such as FLJ37543, LCE3D, FAM89A, ADCY5, ESR1, C2orf67, REST, EPHA7, etc. Furthermore, we obtained several biological functions related to the classification of glioma subtypes, which are also related to gene methylation and corresponding functions, such as cellular developmental process, neuron differentiation, cellular component morphogenesis, and G-protein-coupled receptor signaling pathway. We then validated these methylation signatures, genes, and functions on an independent dataset. We identified a group of methylation sites, genes, and functions by using our screening analysis method. This study provided a basic research on the detailed classification of A-IDH and O-IDH cases.
We downloaded the methylation profiles of patients with IDH-mutation glioma from GEO (Gene Expression Omnibus) under accession numbers GSE90496 and GSE109379, which were originally generated by Capper et al. (2018). The GSE90496 dataset was used as a training dataset, and the GSE109379 dataset was used as an independent test dataset. The training dataset had samples of 78 A-IDH subclasses, 46 high-grade astrocytoma (A-IDH-HG) subclasses, and 80 1p/19q co-deleted O-IDH subclasses. The test dataset had 94 A-IDH, 41 A-IDH-HG, and 83 O-IDH samples. The overlapped 42,383 methylation probes between training and test datasets were used to encode IDH-mutation glioma in each patient to investigate the methylation difference among different IDH-mutation glioma subclasses.
In this study, we first used MCFS (Chen et al., 2018a, 2019a,b; Pan et al., 2018, 2019a,b; Li et al., 2019) to rank the input features, and the ranked features were further selected through IFS (Zhang et al., 2015; Zhou et al., 2015; Chen et al., 2017b,c, 2018b; Wang et al., 2017; Li and Huang, 2018; Zhang T. M. et al., 2018) with a supervised classifier SVM (Cortes and Vapnik, 1995).
MCFS is a supervised feature selection method based on multiple decision trees (Draminski et al., 2008). We used it to generate m bootstrap sample sets and t feature subsets from original data. One decision tree was grown on the basis of each combination of bootstrap sets and feature subsets. A total of m × t decision trees was obtained. According to these trees, we calculated relative importance (RI) score for each feature. The main criterion is that the more frequent a feature is involved in splitting nodes of growing the m × t trees, the more important the feature will be; the accuracy of each decision tree is also considered for evaluating the importance of this feature. In detail, the RI score for one feature f is computed by
where wAcc stands for the weighted accuracy, nf(τ) represents a node of f in decision tree τ, the information gain of nf(τ) is denoted as IG(nf(τ)), no.in nf(τ) stands for the number of samples in nf(τ), no.in τ indicates the number of samples in τ. u and v are weighting factors, which were set to one in this study. After accessing the RI scores of all features, we ranked them in a list in terms of the decreasing order of their RI scores.
MCFS only ranked the input features but could not remove redundant features. The feature selection by an arbitrary cutoff of RI score was not the best method. Thus, IFS, which is a feature selection method with a supervised classifier, was further used to identify the optimum number of features for classification. IFS first generated a series of feature subsets with a step of 10 based on the ranked features from MCFS. The first feature subset consisted of the top 10 features, the second feature subset comprised the top 20 features, and so on. A supervised classifier was built and evaluated on the samples consisting of the features from each feature subset through 10-fold cross-validation. Lastly, we selected the optimum feature subset with the best performance.
We integrated IFS with SVM. To compare the performance baseline, we also evaluated the IFS with random forest (RF; Ho, 1995) and repeated incremental pruning to produce error reduction (RIPPER; Cohen, 1995).
SVM is a supervised classification algorithm based on statistical theory (Cortes and Vapnik, 1995). It finds a hyperplane with the maximum margin between two classes. SVM can handle linear and non-linear data. For non-linear data, SVM first maps the original data into a high-dimensional space by using kernels in which new data can be linearly separable. SVM is designed for binary classification, and one-vs.-the-rest strategy is used for multi-class classification. Multiple SVMs are trained, and each SVM is trained on positive samples from one class and negative samples from the remaining classes. A new sample is assigned a predicted class label corresponding to the highest probability score from one SVM.
RF is a supervised meta-classifier based on multiple decision trees (Ho, 1995). It grows multiple decision trees from bootstrap sets, and each decision tree is trained on a randomly selected feature subset. In contrast to SVM, RF can be directly applied to multiclass classification.
RIPPER is a rule-based classifier that greedily produces classification rules (Cohen, 1995). It first finds a good rule to cover training samples as much as possible and then removes the covered samples from the training set for mining the next rule. RIPPER repeats the above process until all the samples are covered by the produced classification rules.
To quickly implement above-mentioned three classification algorithms, three tools “SMO,” “RandomForest,” and “JRip” in Weka (Witten and Frank, 2005) were employed. Their default parameters were used.
To investigate whether the selected methylation probes were significantly enriched onto certain biological functions, we did the GO and KEGG enrichment analysis. The identified methylation probes were mapped onto genes based on the probe annotations of Illumina HumanMethylation450 BeadChip at GEO under the accession number GPL13534. The genes were enriched onto GO and KEGG terms by using hypergeometric test. We used R function phyper to perform the hypergeometric test. The KEGG database Release 86.0 was retrieved using R/Bioconductor package KEGGREST (https://bioconductor.org/packages/KEGGREST/) and the GO database with date stamp of 2017-Nov01 was provided in R/Bioconductor package org.Hs.eg.db (https://bioconductor.org/packages/org.Hs.eg.db/). The hypergeometric test P-values were adjusted to obtain their false discovery rate (FDR). The GO terms and KEGG pathways with FDR smaller than 0.05 were considered as significant and analyzed.
We used a multiclass classifier to classify samples from A-IDH, A-IDH-HG, and O-IDH and evaluated the trained classifiers by using 10-fold cross-validation (Kohavi, 1995; Chen et al., 2017c, 2018b; Li et al., 2019; Zhang et al., 2019; Zhou et al., 2019) on the training set. To further demonstrate the generalization ability of model learning, we examined the trained classifiers on an independent test set. We also considered Matthews correlation coefficient (MCC; Matthews, 1975; Gorodkin, 2004; Chen et al., 2017a; Zhao et al., 2018, 2019; Cui and Chen, 2019), accuracies of individual classes, and overall accuracy to measure model performance.
In this study, we adopted several advanced computational methods to investigate the methylation profiles of patients with three IDH-mutation glioma subclasses. The entire procedures are illustrated in Figure 1.
Figure 1. The entire procedures for investigating the methylation profiles of patients with three IDH-mutation glioma subclasses.
We first ranked 42,383 features (e.g., methylation sites) as the input by using MCFS. The RI scores of the input features are given in Table S1. A total of 19,692 features have RI scores >0, and the remaining 22,691 features have no any discriminative ability to classify samples from A-IDH, A-IDH-HG, and O-IDH. Thus, only 19,692 features were used for the tasks below.
Next, we evaluated the IFS with an SVM on the training set by using 10-fold cross-validation. Table 1 shows that we yielded the best MCC value of 0.977 when the top 750 features were used, with an overall accuracy of 0.985. The accuracies on three subclasses were 0.987, 0.957, and 1.000, respectively, indicating the good performance of SVM based on top 750 features. Figure 2B illustrates that the MCCs of SVMs changed with the number of the involved features. To justify why we selected SVM as the final classifier of IFS, we also evaluated the performance of IFS with RF and RIPPER. In Table 1, Figures 2A,C, IFS with RF yielded the best MCC value of 0.962 and an overall accuracy of 0.975 when the top 1,330 features were used. The accuracies on three subclasses were 0.987, 0.913, and 1.000, respectively. RF used more features but yielded a lower performance than SVM did. By contrast, the rule-based method RIPPER yielded lower performance than SVM and RF did, thereby achieving the MCC of 0.895 when the top 19,270 features were utilized. The accuracies on three subclasses were also lower than those of SVM and RF (see the last row of Table 1). RIPPER was worse than SVM and RF because RIPPER is a rule-based method that considers the balance between detecting interpretable classification rules and obtaining the high classification performance of “black-box.” The performance corresponding to the number of features of SVM, RF, and RIPPER is given in Table S2.
Table 1. The 10-fold cross-validation performance of IFS with different classifiers on the training set.
Figure 2. Performance of SVM, RF, and RIPPER that changed with the corresponding number of features. (A) RF performance, (B) RIPPER performance, and (C) SVM performance.
To further demonstrate the generalizability of our learned models, we further evaluated the IFS with SVM, RF, and RIPPER on the independent test set. Table 2 shows their performance on the independent test set, where the same number of optimum features identified on the training set was used for each classifier. The MCCs yielded by SVM, RF, and RIPPER were 0.899, 0.907, and 0.972, respectively. The three methods achieved a high performance, demonstrating the generalizability of the trained models. RIPPER yielded the lowest 10-fold cross-validation performance on the training set, but it yielded the highest performance on the independent test set. This result indicated that the simple rule-based method RIPPER might not easily suffer model overfitting compared with that of complicated classifiers SVM and RF, but too many features were used in this classifier.
As mentioned above, SVM with top 750 features yielded the best performance on the training set. However, when top 20 features were used, the SVM generated the MCC of 0.970, which was only 0.007 lower than that obtained by the SVM with top 750 features. Considering the efficiency of SVM, SVM with top 20 features was a more proper choice. Its performance on three classes is listed in Table 1, which was almost at the same level compared with that of the SVM with top 750 features. Furthermore, its performance on the test set is listed in Table 2, which was still acceptable.
We found 750 optimal features for distinguishing A-IDH, A-IDH-HG, and O-IDH with the help of SVM. However, considering the efficiency, SVM with top 20 features was a more suitable choice. Thus, it is believed that these 20 features were extremely important. Here, we gave an extensive discussion on these 20 features (Table 3), which were supported by previous studies. In addition, we further identified a group of detailed biological functions associated with different IDH-mutation glioma subclasses.
The top probe was cg04437966, marking gene FLJ37543. Also known as C5orf64, such gene has been widely reported to participate in tumorigenesis (Aschebrook-Kilfoy et al., 2015). As for its potential contribution on distinguishing different IDH subtypes, it has been reported to participate in multiscale modeling of oligodendrocytes in physical and pathological conditions, but not other neural cell subtypes (Mckenzie et al., 2017). Therefore, the expression level of such gene may actually contribution to the subtyping processes.
The next probe was cg14159026, identifying gene BVES. Encoding a specific member of the POP family of protein, such gene has been widely reported to participate in cell adhesion processes (Wada et al., 2001). As for its specific contribution on IDH-dependent glioma subtyping, it has been reported that such gene can participate in the development of different neural cells and functionally related to IDH (Lord et al., 1997; Ton et al., 2002). Therefore, although no direct reports confirmed its unique classification potentials for glioma subtyping, it is reasonable for us to regard such gene as a reference for IDH-dependent glioma subtyping. Apart from such probe, another effective probe named as cg17398252 is also designed to detect the methylation status of such gene, further confirming above results.
The third probe was cg22519158, detecting the methylation status of gene LCE3D. LCE3D is also a specific development associated gene, participating in the formation of stratum corneum (Bergboer et al., 2011). As for its potential relationship with IDH and its contribution on such subtyping, it has been reported that such gene is related to the expression of IDH and different subtypes of glioma at methylation level, corresponding with our results (Zhang M. et al., 2018).
FAM89A, as the following identified target gene is marked by the fourth probe, named cg12450347. There are no detailed reports on the biological functions of FAM89A. However, the abnormal expression level of such gene has also been screened out on some glioma gene expression profiling studies (Mascelli et al., 2013; Xie et al., 2017). Therefore, our screened-out probe definitely contributes to the IDH-dependent subtyping of glioma.
The next gene ADCY5, detected by probe cg17482114, is an enzyme that interacts with RGS2 in humans. ADCY5 is associated with various neurological syndromes in non-cancer tissues and can cause chorea, a type of neurological syndrome (Walker, 2016). The SNPs of ADCY5 are associated with elevated fasting glucose and increased type 2 diabetes risk. The DNA hypermethylation of ADCY5 induces a low mRNA expression pattern in malignant tissue samples (Sato et al., 2013).
ESR1, detected by probe cg08415493, was also identified to participate in IDH-dependent glioma subtyping. Encoding an estrogen receptor, such gene has been widely reported to participate in hormone related cell proliferation and differentiation (Dalvai and Bystricky, 2010; Mascelli et al., 2013). In glioma, such gene has been reported to be a specific biomarker for glioma subtyping on expression and methylation level (Uhlmann et al., 2003). Considering that such gene has also been identified to be functionally related to IDH, it is quite reasonable to regard such gene as a potential marker for such subtyping (Richardson et al., 2019).
C2orf67, as the target of probe cg12760041, was also identified in this study. According to recent publications, such gene has been reported to be effective as a serum metabolite measurement parameter (Ohyama et al., 2016; Aibara et al., 2018). As for the methylation status and expression pattern of such gene in different glioma subtypes, it has been identified as one of the potential markers reflecting the activation status of EGF signaling pathway (Trang et al., 2010). Considering that different IDH-dependent glioma subtypes have different EGF activation status (Roth and Weller, 2014; Thorne et al., 2016), it is reasonable to identify such gene and its targeted probe as one of the potential markers for such IDH-dependent subtyping.
REST, targeted by probes named as cg26694713 and cg04360458, is also predicted to participate in IDH-dependent glioma subtyping. REST is actually a transcriptional regulatory factor for neuronal genes (Zuccato et al., 2003). Apart from that, REST has also been identified as a specific marker for glioma subtyping due to its epigenetic alteration pattern (Zuccato et al., 2003). In the same report, the mutation status of IDH has also been validated to be functionally related to such methylation alteration (Zuccato et al., 2003).
The next two probes, named as cg21552709 and cg05427966, target Ephrin type-A receptor 7 (EPHA7). EPHA7, as a member of the ephrin receptor superfamily, mediates developmental events, particularly in the nervous system. During the embryonic development of the central nervous system, Ephs and ephrins have defined functions, such as axon mapping, neural crest cell migration, hindbrain segmentation, synapse formation, and physiological and abnormal angiogenesis. Eph and ephrins are frequently overexpressed in different tumor types, including GBM. An increased EphA7 expression is correlated with adverse outcomes in patients with primary and recurrent glioblastoma multiforme (Wang et al., 2008).
The next probe cg20138711 targeting ARHGEF3 was screened out in our study, which were deemed to contribute to IDH-dependent glioma subtyping. ARHGEF3 is a regulator for RhoA and RhoB GTPases (Hilgers and Webb, 2005). According to recent publications, mediating RhoA associated biological processes, ARHGEF3 has been confirmed to interact with IDH (Okada et al., 2003; Kloth et al., 2005) and has unique methylation status in glioma (Northcott et al., 2009). Therefore, it is quite reasonable to summary that such probe actually targets an effective regulatory gene for IDH-dependent glioma subtyping.
Probe cg03903398 is another informant feature targeting effective microRNA, coding gene named as MIR1275. MIR1275 is a functional microRNA coding gene, which has been directly reported to participate in multiple sclerosis (MS; Angerstein et al., 2012). As for its specific role for glioma subtyping, similar with gene ARHGEF3, such microRNA participates in TGF-beta signaling pathway (Yan et al., 2013) and has been validated to have different methylation status together with expression pattern in different IDH expression glioma subtypes (Kondo et al., 2014).
The following four probes cg19681793 (targeting THBS2), cg24215279 (targeting TPO), cg11235583 (targeting CLCNKB), and cg14158583 (targeting PVRL4) have also been confirmed to target effective genes with different methylation status in different IDH-dependent glioma subtypes. Apart from above-discussed eighteen probes, cg12930304 and cg11902641 were also identified to be significant for subtyping. However, according to the annotation, no actual genes are presented in such region, which may be induced by incomplete annotation reference or prediction redundancy. All in all, most genes corresponding to top ranked probes can be confirmed to have differential methylation patterns and corresponding contributions to A-IDH and O-IDH cases, validating the reliability of our findings.
The SVM with top 750 features yielded the best performance. These 750 features (methylation probes) were mapped onto genes, on which a GO and KEGG enrichment analysis was performed. Table 4 lists the significantly enriched GO/KEGG functions with FDR < 0.05. This section analyzed some of them.
Cellular development with hypergeometric test p-value of 2.48E-8 and FDR of 9.78E-5, is an important biological function that can be a marker to classify different glioma subclasses. The tyrosine kinase Fyn is an Src kinase family member essential for normal myelination and implicated in oligodendrocyte development (Ma et al., 2005). Fyn regulates oligodendroglial cell development in oligodendroglioma, considering that the neurogenesis of an adult brain is generally regulated by glial cells.
Neuron differentiation with hypergeometric test p-value of 7.14E-8 and FDR of 0.0009, can be another marker for classifying different glioma subclasses. The suppression of NSC (neural stem cells) differentiation and the promotion of its self-renewal capacity are controlled by the upregulation of PLAGL2. The inhibition of Wnt signaling partially restores the differentiation capacity of PLAGL2-expressing NSC (Zheng et al., 2010). These functions are consistent with a well-known hallmark of glioblastoma, e.g., strong self-renewal potential and immature differentiation state.
Cellular component morphogenesis with hypergeometric test p-value of 6.92E-5 and FDR of 0.0352, varies in different types of gliomas. Tumor cell metastasis mediated by abnormal extracellular matrix (ECM) regulations contributes to the rapid progression of GBM. As such, ECM may play an irreplaceable role during the invasion of GBM (Ulrich et al., 2009). Thus, cellular component morphogenesis may be a functional signature for characterizing different subtypes of gliomas.
G-protein-coupled receptor signaling pathway with hypergeometric test p-value of 6.91E-5 and FDR of 0.0352, coupled to a cyclic nucleotide second messenger, is an important pathway related to GBM. This pathway regulates glioma cells by interfering with calcium signaling processes. Its components, namely, P2Y1 and P2Y2 receptors, coexist in glioma C6 cells as an effective molecular identity of P2Y receptors (Ulrich et al., 2009). In terms of the specific role of this pathway in malignant diseases, Rho GTPase activation and angiogenesis are two typical pathological processes of the identified pathway to trigger tumorigenesis. Therefore, our enriched pathway may be effective and significant for the identification of different glioma subtypes (O'hayre et al., 2014).
The qualitatively analyzed genes help distinguish different glioma subclasses, and all the identified genes are supported by recent literature and related independent expression profiles. The functional enrichment of these genes further validates the differential functional characteristics of gliomas. Therefore, our new analysis method can help determine (methylation) signatures for glioma subclasses and establish a basis for further studying the detailed pathological mechanisms of these glioma subtypes at multiple omics levels.
Publicly available datasets were analyzed in this study. This data can be found here: https://www.ncbi.nlm.nih.gov/geo/query/acc.cgi?acc=GSE90496, https://www.ncbi.nlm.nih.gov/geo/query/acc.cgi?acc=GSE109379.
TH and Y-DC designed the study. XP and LC performed the experiments. TZ, FY, Y-HZ, LZ, and SW analyzed the results. XP and TZ wrote the manuscript. All authors contributed to the research and reviewed the manuscript.
This study was supported by Shanghai Municipal Science and Technology Major Project (2017SHZDZX01), National Key R&D Program of China (2018YFC0910403), National Natural Science Foundation of China (31701151), Natural Science Foundation of Shanghai (17ZR1412500), Shanghai Sailing Program (16YF1413800), the Youth Innovation Promotion Association of Chinese Academy of Sciences (CAS) (2016245), the fund of the key Laboratory of Stem Cell Biology of Chinese Academy of Sciences (201703), and Science and Technology Commission of Shanghai Municipality (STCSM) (18dz2271000).
The authors declare that the research was conducted in the absence of any commercial or financial relationships that could be construed as a potential conflict of interest.
The Supplementary Material for this article can be found online at: https://www.frontiersin.org/articles/10.3389/fbioe.2019.00339/full#supplementary-material
Table S1. RI scores of all input features ranked by MCFS.
Table S2. Ten-fold cross-validation performance of IFS with SVM, RF, and RIPPER that changed with the number of features.
Aibara, N., Ohyama, K., Hidaka, M., Kishikawa, N., Miyata, Y., Takatsuki, M., et al. (2018). Immune complexome analysis of antigens in circulating immune complexes from patients with acute cellular rejection after living donor liver transplantation. Transpl. Immunol. 48, 60–64. doi: 10.1016/j.trim.2018.02.011
Angerstein, C., Hecker, M., Paap, B. K., Koczan, D., Thamilarasan, M., Thiesen, H. J., et al. (2012). Integration of MicroRNA databases to study MicroRNAs associated with multiple sclerosis. Mol. Neurobiol. 45, 520–535. doi: 10.1007/s12035-012-8270-0
Aschebrook-Kilfoy, B., Argos, M., Pierce, B. L., Tong, L., Jasmine, F., Roy, S., et al. (2015). Genome-wide association study of parity in Bangladeshi women. PLoS ONE 10:e0118488. doi: 10.1371/journal.pone.0118488
Bergboer, J. G., Tjabringa, G. S., Kamsteeg, M., Van Vlijmen-Willems, I. M., Rodijk-Olthuis, D., Jansen, P. A., et al. (2011). Psoriasis risk genes of the late cornified envelope-3 group are distinctly expressed compared with genes of other LCE groups. Am. J. Pathol. 178, 1470–1477. doi: 10.1016/j.ajpath.2010.12.017
Cancer Genome Atlas Research Network, Brat, D. J., Verhaak, R. G., Aldape, K. D., Yung, W. K., Salama, S. R., et al. (2015). Comprehensive, Integrative Genomic Analysis of Diffuse Lower-Grade Gliomas. N. Engl. J. Med. 372, 2481–2498. doi: 10.1056/NEJMoa1402121
Capper, D., Jones, D. T. W., Sill, M., Hovestadt, V., Schrimpf, D., Sturm, D., et al. (2018). DNA methylation-based classification of central nervous system tumours. Nature 555, 469–474. doi: 10.1038/nature26000
Chen, L., Chu, C., Zhang, Y.-H., Zheng, M.-Y., Zhu, L., Kong, X., et al. (2017a). Identification of drug-drug interactions using chemical interactions. Curr. Bioinform. 12, 526–534. doi: 10.2174/1574893611666160618094219
Chen, L., Li, J., Zhang, Y. H., Feng, K., Wang, S., Zhang, Y., et al. (2018a). Identification of gene expression signatures across different types of neural stem cells with the Monte-Carlo feature selection method. J. Cell. Biochem. 119, 3394–3403. doi: 10.1002/jcb.26507
Chen, L., Pan, X., Hu, X., Zhang, Y.-H., Wang, S., Huang, T., et al. (2018b). Gene expression differences among different MSI statuses in colorectal cancer. Int J Cancer 143, 1731–1740. doi: 10.1002/ijc.31554
Chen, L., Pan, X., Zhang, Y.-H., Kong, X., Huang, T., and Cai, Y.-D. (2019a). Tissue differences revealed by gene expression profiles of various cell lines. J. Cell. Biochem. 120, 7068–7081. doi: 10.1002/jcb.27977
Chen, L., Wang, S., Zhang, Y.-H., Li, J., Xing, Z.-H., Yang, J., et al. (2017b). Identify key sequence features to improve CRISPR sgRNA efficacy. IEEE Access 5, 26582–26590. doi: 10.1109/ACCESS.2017.2775703
Chen, L., Zhang, S., Pan, X., Hu, X., Zhang, Y. H., Yuan, F., et al. (2019b). HIV infection alters the human epigenetic landscape. Gene Ther. 26, 29–39. doi: 10.1038/s41434-018-0051-6
Chen, L., Zhang, Y.-H., Lu, G., Huang, T., and Cai, Y.-D. (2017c). Analysis of cancer-related lncRNAs using gene ontology and KEGG pathways. Artif. Intell. Med. 76, 27–36. doi: 10.1016/j.artmed.2017.02.001
Cohen, W. W. (1995). “Fast effective rule induction,” in The Twelfth International Conference on Machine Learning (Tahoe City, CA), 115–123. doi: 10.1016/B978-1-55860-377-6.50023-2
Cortes, C., and Vapnik, V. (1995). Support-vector networks. Mach. Learn. 20, 273–297. doi: 10.1007/BF00994018
Cui, H., and Chen, L. (2019). A binary classifier for the prediction of EC numbers of enzymes. Curr. Proteomics 16, 381–389. doi: 10.2174/1570164616666190126103036
Dalvai, M., and Bystricky, K. (2010). Cell cycle and anti-estrogen effects synergize to regulate cell proliferation and ER target gene expression. PLoS ONE 5:e11011. doi: 10.1371/journal.pone.0011011
Delpu, Y., Cordelier, P., Cho, W. C., and Torrisani, J. (2013). DNA methylation and cancer diagnosis. Int. J. Mol. Sci. 14, 15029–15058. doi: 10.3390/ijms140715029
Draminski, M., Rada-Iglesias, A., Enroth, S., Wadelius, C., Koronacki, J., and Komorowski, J. (2008). Monte Carlo feature selection for supervised classification. Bioinformatics 24, 110–117. doi: 10.1093/bioinformatics/btm486
Gorodkin, J. (2004). Comparing two K-category assignments by a K-category correlation coefficient. Comput. Biol. Chem. 28, 367–374. doi: 10.1016/j.compbiolchem.2004.09.006
Hilgers, R. H., and Webb, R. C. (2005). Molecular aspects of arterial smooth muscle contraction: focus on Rho. Exp. Biol. Med. 230, 829–835. doi: 10.1177/153537020523001107
Ho, T. K. (1995). “Random decision forests,” in Proceedings of the 3rd International Conference on Document Analysis and Recognition (Montreal, QC).
Kloth, J. N., Fleuren, G. J., Oosting, J., De Menezes, R. X., Eilers, P. H., Kenter, G. G., et al. (2005). Substantial changes in gene expression of Wnt, MAPK and TNFalpha pathways induced by TGF-beta1 in cervical cancer cell lines. Carcinogenesis 26, 1493–1502. doi: 10.1093/carcin/bgi110
Kohavi, R. (1995). “A study of cross-validation and bootstrap for accuracy estimation and model selection,” in International Joint Conference on Artificial Intelligence (Montreal, QC: Lawrence Erlbaum Associates Ltd.), 1137–1145.
Kondo, Y., Katsushima, K., Ohka, F., Natsume, A., and Shinjo, K. (2014). Epigenetic dysregulation in glioma. Cancer Sci. 105, 363–369. doi: 10.1111/cas.12379
Li, J., and Huang, T. (2018). Predicting and analyzing early wake-up associated gene expressions by integrating GWAS and eQTL studies. Biochim. Biophys. Acta. Mol. Basis Dis. 1864, 2241–2246. doi: 10.1016/j.bbadis.2017.10.036
Li, J., Lu, L., Zhang, Y. H., Xu, Y., Liu, M., Feng, K., et al. (2019). Identification of leukemia stem cell expression signatures through Monte Carlo feature selection strategy and support vector machine. Cancer Gene Ther. doi: 10.1038/s41417-019-0105-y. [Epub ahead of print].
Liu, H. A., and Setiono, R. (1998). Incremental feature selection. Appl. Intell. 9, 217–230. doi: 10.1023/A:1008363719778
Lord, K. A., Wang, X. M., Simmons, S. J., Bruckner, R. C., Loscig, J., O'connor, B., et al. (1997). Variant cDNA sequences of human ATP:citrate lyase: cloning, expression, and purification from baculovirus-infected insect cells. Protein Expr. Purif. 9, 133–141. doi: 10.1006/prep.1996.0668
Losman, J. A., and Kaelin, W. G. Jr. (2013). What a difference a hydroxyl makes: mutant IDH, (R)-2-hydroxyglutarate, and cancer. Genes Dev. 27, 836–852. doi: 10.1101/gad.217406.113
Ma, D. K., Ming, G. L., and Song, H. (2005). Glial influences on neural stem cell development: cellular niches for adult neurogenesis. Curr. Opin. Neurobiol. 15, 514–520. doi: 10.1016/j.conb.2005.08.003
Mascelli, S., Barla, A., Raso, A., Mosci, S., Nozza, P., Biassoni, R., et al. (2013). Molecular fingerprinting reflects different histotypes and brain region in low grade gliomas. BMC Cancer 13:387. doi: 10.1186/1471-2407-13-387
Matthews, B. (1975). Comparison of the predicted and observed secondary structure of T4 phage lysozyme. Biochim. Biophys. Acta 405, 442–451. doi: 10.1016/0005-2795(75)90109-9
Mckenzie, A. T., Moyon, S., Wang, M., Katsyv, I., Song, W. M., Zhou, X., et al. (2017). Multiscale network modeling of oligodendrocytes reveals molecular components of myelin dysregulation in Alzheimer's disease. Mol. Neurodegener. 12:82. doi: 10.1186/s13024-017-0219-3
Northcott, P. A., Nakahara, Y., Wu, X., Feuk, L., Ellison, D. W., Croul, S., et al. (2009). Multiple recurrent genetic events converge on control of histone lysine methylation in medulloblastoma. Nat. Genet. 41, 465–472. doi: 10.1038/ng.336
O'hayre, M., Degese, M. S., and Gutkind, J. S. (2014). Novel insights into G protein and G protein-coupled receptor signaling in cancer. Curr. Opin. Cell Biol. 27, 126–135. doi: 10.1016/j.ceb.2014.01.005
Ohyama, K., Baba, M., Tamai, M., Yamamoto, M., Ichinose, K., Kishikawa, N., et al. (2016). Immune complexome analysis of antigens in circulating immune complexes isolated from patients with IgG4-related dacryoadenitis and/or sialadenitis. Mod. Rheumatol. 26, 248–250. doi: 10.3109/14397595.2015.1072296
Okada, K., Katagiri, T., Tsunoda, T., Mizutani, Y., Suzuki, Y., Kamada, M., et al. (2003). Analysis of gene-expression profiles in testicular seminomas using a genome-wide cDNA microarray. Int. J. Oncol. 23, 1615–1635. doi: 10.3892/ijo.23.6.1615
Pan, X., Chen, L., Feng, K. Y., Hu, X. H., Zhang, Y. H., Kong, X. Y., et al. (2019a). Analysis of expression pattern of snoRNAs in different cancer types with machine learning algorithms. Int. J. Mol. Sci. 20:2185. doi: 10.3390/ijms20092185
Pan, X., Hu, X., Zhang, Y.-H., Chen, L., Zhu, L., Wan, S., et al. (2019b). Identification of the copy number variant biomarkers for breast cancer subtypes. Mol. Genet. Genomics 294, 95–110. doi: 10.1007/s00438-018-1488-4
Pan, X., Hu, X., Zhang, Y. H., Feng, K., Wang, S. P., Chen, L., et al. (2018). Identifying patients with atrioventricular septal defect in down syndrome populations by using self-normalizing neural networks and feature selection. Genes (Basel). 9:208. doi: 10.3390/genes9040208
Richardson, T. E., Patel, S., Serrano, J., Sathe, A. A., Daoud, E. V., Oliver, D., et al. (2019). Genome-wide analysis of glioblastoma patients with unexpectedly long survival. J. Neuropathol. Exp. Neurol. 78, 501–507. doi: 10.1093/jnen/nlz025
Roth, P., and Weller, M. (2014). Challenges to targeting epidermal growth factor receptor in glioblastoma: escape mechanisms and combinatorial treatment strategies. Neuro Oncol. 16(Suppl. 8), viii14– viii19. doi: 10.1093/neuonc/nou222
Sato, T., Arai, E., Kohno, T., Tsuta, K., Watanabe, S., Soejima, K., et al. (2013). DNA methylation profiles at precancerous stages associated with recurrence of lung adenocarcinoma. PLoS ONE 8:e59444. doi: 10.1371/journal.pone.0059444
Thorne, A. H., Zanca, C., and Furnari, F. (2016). Epidermal growth factor receptor targeting and challenges in glioblastoma. Neuro Oncol. 18, 914–918. doi: 10.1093/neuonc/nov319
Ton, C., Stamatiou, D., Dzau, V. J., and Liew, C. C. (2002). Construction of a zebrafish cDNA microarray: gene expression profiling of the zebrafish during development. Biochem. Biophys. Res. Commun. 296, 1134–1142. doi: 10.1016/S0006-291X(02)02010-7
Trang, S. H., Joyner, D. E., Damron, T. A., Aboulafia, A. J., and Randall, R. L. (2010). Potential for functional redundancy in EGF and TGFalpha signaling in desmoid cells: a cDNA microarray analysis. Growth Factors 28, 10–23. doi: 10.3109/08977190903299387
Uhlmann, K., Rohde, K., Zeller, C., Szymas, J., Vogel, S., Marczinek, K., et al. (2003). Distinct methylation profiles of glioma subtypes. Int. J. Cancer 106, 52–59. doi: 10.1002/ijc.11175
Ulrich, T. A., De Juan Pardo, E. M., and Kumar, S. (2009). The mechanical rigidity of the extracellular matrix regulates the structure, motility, and proliferation of glioma cells. Cancer Res. 69, 4167–4174. doi: 10.1158/0008-5472.CAN-08-4859
Venteicher, A. S., Tirosh, I., Hebert, C., Yizhak, K., Neftel, C., Filbin, M. G., et al. (2017). Decoupling genetics, lineages, and microenvironment in IDH-mutant gliomas by single-cell RNA-seq. Science 355:eaai8478. doi: 10.1126/science.aai8478
Wada, A. M., Reese, D. E., and Bader, D. M. (2001). Bves: prototype of a new class of cell adhesion molecules expressed during coronary artery development. Development 128, 2085–2093.
Walker, R. H. (2016). The non-Huntington disease choreas: five new things. Neurol. Clin. Pract. 6, 150–156. doi: 10.1212/CPJ.0000000000000236
Wang, L. F., Fokas, E., Juricko, J., You, A., Rose, F., Pagenstecher, A., et al. (2008). Increased expression of EphA7 correlates with adverse outcome in primary and recurrent glioblastoma multiforme patients. BMC Cancer 8:79. doi: 10.1186/1471-2407-8-79
Wang, S., Zhang, Y. H., Zhang, N., Chen, L., Huang, T., and Cai, Y. D. (2017). Recognizing and predicting thioether bridges formed by lanthionine and beta-methyllanthionine in lantibiotics using a random forest approach with feature selection. Comb. Chem. High Throughput Screen 20, 582–593. doi: 10.2174/1386207320666170310115754
Witten, I. H., and Frank, E. (eds.). (2005). Data Mining:Practical Machine Learning Tools and Techniques. San Francisco, CA: Morgan, Kaufmann, Elsevier.
Xie, L., Liao, Y., Shen, L., Hu, F., Yu, S., Zhou, Y., et al. (2017). Identification of the miRNA-mRNA regulatory network of small cell osteosarcoma based on RNA-seq. Oncotarget 8, 42525–42536. doi: 10.18632/oncotarget.17208
Yan, K., Yang, K., and Rich, J. N. (2013). The evolving landscape of glioblastoma stem cells. Curr. Opin. Neurol. 26, 701–707. doi: 10.1097/WCO.0000000000000032
Zhang, M., Pan, Y., Qi, X., Liu, Y., Dong, R., Zheng, D., et al. (2018). Identification of new biomarkers associated with IDH mutation and prognosis in astrocytic tumors using nanostring ncounter analysis system. Appl. Immunohistochem. Mol. Morphol. 26, 101–107. doi: 10.1097/PAI.0000000000000396
Zhang, P. W., Chen, L., Huang, T., Zhang, N., Kong, X. Y., and Cai, Y. D. (2015). Classifying ten types of major cancers based on reverse phase protein array profiles. PLoS ONE 10:e0123147. doi: 10.1371/journal.pone.0123147
Zhang, T. M., Huang, T., and Wang, R. F. (2018). Cross talk of chromosome instability, CpG island methylator phenotype and mismatch repair in colorectal cancer. Oncol. Lett. 16, 1736–1746. doi: 10.3892/ol.2018.8860
Zhang, X., Chen, L., Guo, Z.-H., and Liang, H. (2019). Identification of human membrane protein types by incorporating network embedding methods. IEEE Access 7, 140794–140805. doi: 10.1109/ACCESS.2019.2944177
Zhao, X., Chen, L., Guo, Z.-H., and Liu, T. (2019). Predicting drug side effects with compact integration of heterogeneous networks. Curr. Bioinform. 14:1. doi: 10.2174/1574893614666190220114644
Zhao, X., Chen, L., and Lu, J. (2018). A similarity-based method for prediction of drug side effects with heterogeneous information. Math. Biosci. 306, 136–144. doi: 10.1016/j.mbs.2018.09.010
Zheng, H., Ying, H., Wiedemeyer, R., Yan, H., Quayle, S. N., Ivanova, E. V., et al. (2010). PLAGL2 regulates Wnt signaling to impede differentiation in neural stem cells and gliomas. Cancer Cell 17, 497–509. doi: 10.1016/j.ccr.2010.03.020
Zhou, J.-P., Chen, L., and Guo, Z.-H. (2019). iATC-NRAKEL: an efficient multi-label classifier for recognizing anatomical therapeutic chemical (ATC) classes of drugs. Bioinformatics. doi: 10.1093/bioinformatics/btz757. [Epub ahead of print].
Zhou, Y., Zhang, N., Li, B. Q., Huang, T., Cai, Y. D., and Kong, X. Y. (2015). A method to distinguish between lysine acetylation and lysine ubiquitination with feature selection and analysis. J. Biomol. Struct. Dyn. 33, 2479–2490. doi: 10.1080/07391102.2014.1001793
Keywords: isocitrate dehydrogenase, methylation, IDH-mutation, gliomas, multi-class classification
Citation: Pan X, Zeng T, Yuan F, Zhang Y-H, Chen L, Zhu L, Wan S, Huang T and Cai Y-D (2019) Screening of Methylation Signature and Gene Functions Associated With the Subtypes of Isocitrate Dehydrogenase-Mutation Gliomas. Front. Bioeng. Biotechnol. 7:339. doi: 10.3389/fbioe.2019.00339
Received: 09 September 2019; Accepted: 30 October 2019;
Published: 14 November 2019.
Edited by:
Min Tang, Jiangsu University, ChinaReviewed by:
Xiao Chang, Children's Hospital of Philadelphia, United StatesCopyright © 2019 Pan, Zeng, Yuan, Zhang, Chen, Zhu, Wan, Huang and Cai. This is an open-access article distributed under the terms of the Creative Commons Attribution License (CC BY). The use, distribution or reproduction in other forums is permitted, provided the original author(s) and the copyright owner(s) are credited and that the original publication in this journal is cited, in accordance with accepted academic practice. No use, distribution or reproduction is permitted which does not comply with these terms.
*Correspondence: Tao Huang, dG9odWFuZ3Rhb0AxMjYuY29t; Yu-Dong Cai, Y2FpbF95dWRAMTI2LmNvbQ==
†These authors have contributed equally to this work
Disclaimer: All claims expressed in this article are solely those of the authors and do not necessarily represent those of their affiliated organizations, or those of the publisher, the editors and the reviewers. Any product that may be evaluated in this article or claim that may be made by its manufacturer is not guaranteed or endorsed by the publisher.
Research integrity at Frontiers
Learn more about the work of our research integrity team to safeguard the quality of each article we publish.