- 1Environmental Biotechnology Laboratory, Department of Biotechnology, University of the Western Cape, Bellville, South Africa
- 2Department of Biochemistry, Faculty of Basic Medical Sciences, Olabisi Onabanjo University, Sagamu, Ogun State, Nigeria
- 3Department of Plant Sciences, Qwaqwa Campus, University of the Free State, Phuthadithjaba, South Africa
- 4Department of Plant Science, Faculty of Sciences, Olabisi Onabanjo University, Ago-Iwoye, Nigeria
- 5Plant Omics Laboratory, Department of Biotechnology, University of the Western Cape, Bellville, South Africa
Fusarium solani is worrisome because it severely threatens the agricultural productivity of certain crops such as tomatoes and peas, causing the general decline, wilting, and root necrosis. It has also been implicated in the infection of the human eye cornea. It is believed that early detection of the fungus could save these crops from the destructive activities of the fungus through early biocontrol measures. Therefore, the present work aimed to build a sensitive model of novel anti-Fusarium solani antimicrobial peptides (AMPs) against the fungal cutinase 1 (CUT1) protein for early, sensitive and accurate detection. Fusarium solani CUT1 receptor protein 2D secondary structure, model validation, and functional motifs were predicted. Subsequently, anti-Fusarium solani AMPs were retrieved, and the HMMER in silico algorithm was used to construct a model of the AMPs. After their structure predictions, the interaction analysis was analyzed for the Fusarium solani CUT1 protein and the generated AMPs. The putative anti-Fusarium solani AMPs bound the CUT1 protein very tightly, with OOB4 having the highest binding energy potential for HDock. The pyDockWeb generated high electrostatic, desolvation, and low van der Waals energies for all the AMPs against CUT1 protein, with OOB1 having the most significant interaction. The results suggested the utilization of AMPs for the timely intervention, control, and management of these crops, as mentioned earlier, to improve their agricultural productivity and reduce their economic loss and the use of HMMER for constructing models for disease detection.
Introduction
Fusarium solani severely threatens agricultural productivity worldwide due to reducing plant crops’ nutrients and harvest, resulting in tremendous economic losses (Hartman et al., 2011). It causes root rots of its host by penetrating plant cell walls and destroying the torus (Raaijmakers et al., 2009). Fusarium solani is a common soil fungus of a complex of more than twenty-six closely related filamentous fungi in the Nectriaceae family (Mavhunga, 2020). It is found in ponds, rivers, sewage facilities, water pipes, larvae and adults of the picnic beetle, and a symbiote of the ambrosia beetle (Šišić et al., 2018). It infects plants through developing plant roots to produce asexual macro- and microconidia dispersed through wind and rain (Shakeel et al., 2020). Morphologically, Fusarium solani is unique because, unlike most Fusarium species that form a pink or violet centre when cultured, it forms white and cottony colonies with a blue-green or bluish brown colour (Chehri et al., 2015). It is a common cause of diseases in plants such as peas, beans, potatoes, olive, soybeans, and many types of cucurbits and humans, resulting in either mycoses or the infection of the eye cornea. It can result in plant decline, wilting, and necrosis in plant roots (Kurt et al., 2020).
Several researchers have carried out work that focuses on the measures to reduce the menace of Fusarium solani on animals and crop plants (Bhat et al., 2016; Mahawar et al., 2019). One such work identified Fusarium solani to contain 5–17 chromosomes with a genome size of 45.81 Mbp and above (Coleman et al., 2009). Another research also identified the GC contents of its DNA to be 50% (Rasmey et al., 2020). The mycelium of Fusarium solani is rich in alanine and several fatty acids such as δ-aminobutyric-, palmitic-, oleic-, and linolenic acids (Nidiry et al., 2011). Potassium is necessary for the growth of Fusarium solani, and when the potassium level reduces to 3mM, it develops a feathery pattern (Mecteau et al., 2008). Fusarium solani can decompose cellulose at an optimal pH of 6.5 and a temperature of 30°C (Scully et al., 2012). It can metabolize steroids and lignin and reduce Fe3+ to Fe2+ (Hurst, 1995; Xiang et al., 2021). This fungus also produces several toxins such as mycotoxins (trichothecenes and fumonisins), and other toxins produced from citrus-associated F. solani include napthozarins, while certain toxic metabolites such as solaniol, neosolaniol, T-2 toxin, HT-2 toxin, and diacetoxyscirpenol (Rehman et al., 2012).
Fusarium solani is a stubborn plant pathogen because it is unaffected by the pH changes of the soil significantly, and soil fumigation increases its occurrence (Bhatti et al., 2013). This tendency allows Fusarium solani to persist in the soil for at least a decade to wreck its complete crop loss. Its virulence in plants is partly controlled by the cutinase 1 (CUT1) gene, upregulated by exposure to the plant’s cutin monomers (Li et al., 2002). A plethora of management practices exist which are developed independently due to the ubiquitous nature of the fungus. Despite these management practices, the menace of this fungus on plant crops is becoming alarming (Agnoli et al., 2012). It is of significant note to describe the functional and structural architecture of homologous cutinase 1 (CUT1) since its gene controls the virulence of the fungus. The circular dichroism and fluorescence profile at different pH ranges of 6–9 showed unique structural formation for the cutinase, indicating its stability to a wide range of pH (Pham et al., 2016). There is a high resemblance of the secondary structure of the cutinase using homology modelling for its structural study across Fusarium solani (Wei et al., 2014). However, the structural stability of the cutinase differs significantly in its tertiary structure, hydrophobicity, electrostatic parameters, and across different tolerance levels in folding during denaturation to aqueous guanidine hydrochloride. The four tryptophan residues in the protein is embedded in the inaccessible hydrophobic pockets. There is a different distribution of the aromatic amino acid on the surface of the enzyme (Kruithof, 2007).
Several methods exist for diagnosing Fusarium solani in the laboratories for its sensitive and timely detection. One of them relies on clinical observations such as hyaline hyphae in tissue, necrotic lesions in the skin and positive blood tests with fungal growth or the presence of fungal cell wall components to hint at fusariosis (van Diepeningen et al., 2015). Several laboratories also rely on morphological identification. However, multi-locus sequencing discriminates among species complex members (Zaccardelli et al., 2008). Diagnostic tools based on DNA identification have the best discriminatory power when based on translation elongation factor 1-α or the RNA polymerase II second largest subunit (He et al., 2011). The use of antimicrobial peptides could be used for disease diagnostics when modelled using powerful in silico tools such as HMMER (Tincho et al., 2016; Williams and Tincho, 2016; Bakare et al., 2020; Bakare et al., 2021a). Despite these interventions, the rapid test for the fungus has been suggested by authors for timely detection before it wreaks its havoc on the host plant.
Antimicrobial peptides (AMPs) are small peptides that exist in nature and are part of many organisms’ innate systems with tested inhibition against bacteria, viruses, fungi, and other parasites (Bahar and Ren, 2013). The incidence of antibiotic-resistant microorganisms and the knowledge of the therapeutic effects have been well-described. It was discovered only recently that the wide-ranging functionality of the AMPs against diseases and infections expands the list of activities beyond antimicrobial effects attributed to them (Zhang and Gallo, 2016). AMPs have found applications in diagnostics where they are said to have a wide range of activities against HIV, bacterial and viral pneumonia, and Fusarium oxysporum (Tincho et al., 2016; Williams and Tincho, 2016; Bakare et al., 2020; Bakare et al., 2021a; Bakare et al., 2021b). Therefore, this work aimed to use in silico algorithms such as HMMER to build antimicrobial peptide models against Fusarium solani cutinase 1 for sensitive identification. This is important for its early detection for timely intervention, control, and management to improve agricultural productivity of crop plants such as peas, beans, potatoes, olive, soybeans, and many types of cucurbits and mycoses in humans.
Materials and methods
Retrieval of receptors
The gene for the receptor, CUT1 protein, was identified for Fusarium solani (isolate Cutin hydrolase 1; Flags: Precursor) and collected from the National Center for Biotechnology Information (NCBI) (https://www.ncbi.nlm.nih.gov/, accessed on 26 December 2021) (Pruitt et al., 2005), through literature mining. Thereafter, verification was performed using curation to ensure that the retrieved Fusarium solani gene was complete and specific for Fusarium solani. Translation of the reading frame of the coding portion of the gene into protein was performed using the Ex-PAsy translate tool (https://web.expasy.org/translate/, accessed on 27 December 2021) (Artimo et al., 2012). BLAST analysis was then performed using the UniProt interface (https://www.uniprot.org/help/uniprotkb, accessed on 23 January 2021) for further assurance of specificity such that the CUT1 protein of interest was specific for Fusarium solani.
2-D secondary structure prediction
The 2-D secondary structure prediction of the CUT1 protein was carried out using the PSIPRED server to ascertain their alpha-helices, beta-sheets, and random coils (http://bioinf.cs.ucl.ac.uk/psipred, accessed 30 December 2021) (McGuffin et al., 2000).
Protein model evaluation
The quality of the resulting CUT1 protein model was checked using PROCHECK (https://www.ebi.ac.uk/thornton-srv/software/PROCHECK/, accessed 31 December 2021) to predict parameters such as chain length, hydrogen bond geometry, planarity and angles of the peptide bonds (Laskowski et al., 2006).
Prediction of functional motifs
The MOTIF finder (http://www.genome.jp/tools/motif/, accessed 30 January 2022) was used to find the motifs present in the protein to enable its complete description (Carlson et al., 2007).
Anti-Fusarium solani AMPs collection
Collection of anti-Fusarium solani AMPs was carried out from antimicrobial peptide databases such as Antimicrobial Peptides Database (APD3) (https://aps.unmc.edu/database/anti) (Wang and Wang, 2004; Wang et al., 2005) and Collection of Antimicrobial Peptides (CAMP) (http://www.camp.bicnirrh.res.in/seqDb.php?page=0) (Thomas et al., 2010). Thereafter, the confirmation that the collected AMPs were either experimentally validated or predicted mining was carried out using literature mining. Removal of duplicate experimentally validated AMPs was then ensured from the list using the Cluster Database at High Identity with Tolerance (CD-HIT) (Li and Godzik, 2006).
Data partitioning
Random partitioning into two subsets (3/4 of the data utilized as the training partition and the remaining ¼ utilized as testing) of the screened experimentally validated AMPs was carried out to build a strong profile, including optimization/calibration of profiles.
Construction of profiles
Utilization of the Hidden Markov Models (HMMER) algorithm version 3.8 (Roddy, 2018) was carried out to build pathogen-specific profiles using the constructed datasets utilizing the terminal of the Ubuntu operating system version 12.04; (Canonical Ltd., London, United Kingdom) with the command line used for building the profile written:
For the first step, the training datasets were aligned using the Clustalo alignment tool (Fang, 2018). The alignment was carried out using the command line:
Clustalo –i FSTrainings. Fasta –o FSTrainings.sto --outfmt=st (i)
The command line simply states <<do an alignment of the sequences which are in the upper case found in the input file “dataset.fasta” with the Fasta, using Clustalo as multiple alignment tools and GCG Postscript output for graphical printing>>. The output of the command results in the construction of aligned sequences called “dataset.msf.” The aligned sequences were used as input in the next step.
The next step enhances the construction of the profiles of the target class sequences by showing the common motifs/signatures within the profiles. To achieve this, the “Build profiles” was run using the following command:
hmmbuild FSTrainings.hmm FSTrainings.sto (ii)
The resulting profiles “dataset.hmm” was used in evaluating the profiles’ performance by testing the created profiles on an independent AMP dataset. Figure 1 below shows the detailed representation of AMPs model construction.
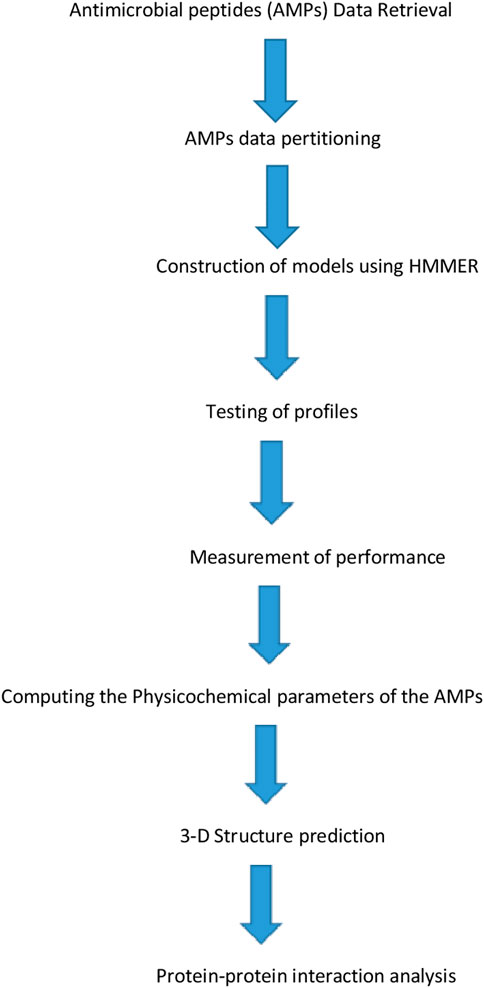
FIGURE 1. Detailed representative structural representation for Antimicrobial peptides (AMPs) model construction.
Testing of profile
The query of the profile was carried out in a step called “Query profiles” utilizing the testing data against that profile using the command line as follows:
The independent testing of each created profile was performed in a step called “Query profiles.” The testing data were queried against the created profiles using the command line, with an E-value threshold of 95% or 0.05:
Hmmsearch –E5e-2 FSTrainings.hmm profile query.txt > resultfile.txt (iv)
Measurement of performance of the profile
Sensitivity, specificity, accuracy, and Matthews Correlation Coefficient as statistical parameters were carried out as described below, where TP indicates true positive, TN indicates true negative, FP indicates false positive, and FN indicates false negative:
Percentage sensitivity of the anti-Fusarium solani AMPs against Fusarium solani (testing sets) effectively predicted as anti- Fusarium solani AMPs (positive). The equation of the sensitivity is written below as (1):
Percentage specificity of the non-anti- Fusarium solani AMPs (negative sets) effectively predicted as non-anti- Fusarium solani AMPs (negative). The equation of the specificity is written below as (2):
Percentage accuracy of the effectively predicted peptides (anti- Fusarium solani AMPs and non-anti- Fusarium solani AMPs). The equation of the accuracy is written below as (3):
Matthew’s correlation coefficient (MCC) measures the sensitivity and specificity. MCC = 0 is an indication of absolutely random prediction, while MCC = 1 means perfect prediction. See the Eq. 4 as below:
Identification of putative anti- Fusarium solani AMPs
Proteome sequences were scanned using the profile with the list of all proteome sequences retrieved from the Ensembl database (http://www.ensembl.org/index.html, accessed on 22 April 2021) (Hubbard et al., 2002) and the UniProt database (http://www.uniprot.org/, accessed on 23 April 2021) (Consortium, 2015). An E-value cut-off was set to 0.05 for the discovery of putative anti- Fusarium solani AMPs. The task was accomplished using “hmmsearch” module of the HMMER software with the command line employed stated as follows:
Hmmsearch –E5e-2 FSTrainings.hmm profile query.txt > resultfile.txt (iv)
Specific FSTrainings.hmm in the profile, target class query.txt representing the species scanned against the profile and resultfile.txt is the output file acquired after testing the species against the constructed Fusarium solani (FS) profile.
Computation of the physicochemical properties of the putative anti- Fusarium solani AMPs and the Fusarium solani CUT1 protein
The anti- Fusarium solani AMPs physicochemical properties were calculated using the prediction interface of BACTIBASE (http://bactibase.pfba-lab-tun.org/physicochem, accessed on 31 June 2021) (Hammami et al., 2007; Hammami et al., 2010), DBAASP (https://dbaasp.org/, accessed on 31 July 2021) (Pirtskhalava et al., 2016), and APD3 (https://wangapd3.com/main.php, accessed on 28 August 2021) (Wang et al., 2009) and the receptor CUT1 protein was carried out using ProtParam tool (http://web.expasy.org/protparam/, accessed on 28 August 2021) from the ExPAsy server (Kyte and Doolittle, 1982).
Predictions of the putative anti- Fusarium solani AMPs and Fusarium solani protein structures
An example of a de novo peptide or protein structure prediction method was used to generate the putative anti- Fusarium solani AMPs, and the Fusarium solani CUT1 protein structures such as I-TASSER (Iterative Threading ASSembly Refinement) server was utilized (Eswar et al., 2009). In brief, uploading of each sequence onto the I-TASSER website was performed (Roy et al., 2010), and RasMol 2.7.5 Software (NextMove Software Ltd., Cambridge Science Park, United Kingdom) was then utilized to visualize the 3-D structures of the AMPs and the protein receptor (Sayle and Milner-White, 1995).
Putative anti- Fusarium solani AMPs and Fusarium solani protein interaction analysis
The pyDockWeb web-server which allows the docking of the protein-small ligand molecule, available at https://life.bsc.es/servlet/pydock/ (accessed on 31 March 2022) was used for the docking of the anti- Fusarium solani AMPs to the Fusarium solani CUT1 protein (Jiménez-García et al., 2013). In brief, the I-TASSER-generated PDB files for the 3-D structures of the anti- Fusarium solani putative AMPs and the Fusarium solani protein receptor were uploaded onto the pyDockWeb server. The interaction analysis of the complex between the anti- Fusarium solani putative AMPs and the CUT1 protein receptor was achieved using RasMol 2.7.5 Software (NextMove Software Ltd., Cambridge Science Park, United Kingdom) (Sayle and Milner-White, 1995). Subsequently, binding scores of the complex formed between the AMPs and the receptor protein were computed using the HDock server (http://hdock.phys.hust.edu.cn/, accessed on 3 March 2021) for comparison (Yan et al., 2020).
Results
2-D model structure prediction
The predicted result represented by PSIPRED server from Supplemetary1 revealed that the secondary structure of CUT1 protein contained 100 small non-polar, 46 hydrophobic, 61 polar, and 19 aromatics plus cysteine regions necessary to strengthen its activity.
The predicted result represented by the PSIPRED server further revealed that the secondary structure of CUT1 protein contained 6 beta-strands, 10 alpha-helices, and 16 random coils (Supplementary 2). The structure revealed that CUT1 protein belongs to an alpha-beta class, with a central beta-sheet of 4. The abundance of alpha-helices allowed the protein to perform its functions to act on carboxylic ester bonds and facilitate the fungus penetration into the plant cuticle.
3-D modelled structure validation
The structural quality of the modelled CUT1 was carried out using PROCHECK (https://servicesn.mbi.ucla.edu/ PROCHECK/). As indicated in Supplementary 3, the PROCHECK result analysis indicated that CUT1 had 92.6% residues in the most favored regions, 6.8% in the additional allowed regions, and 0.6% in the generously allowed and 0.0% at the disallowed regions. The distribution of the amino acid residues made its model of high quality.
Prediction of motif regions
Table 1 shows the probable functional motifs of CUT1 protein with three motifs in which the first one was located at position 46.222 in the amino acid sequence with an E value of 7e–53. Vir1 was located at position 118.171 in the amino acid sequence with an E value of 0.035, and the Mbeg1-like motif was located at position 120.16 in the amino acid sequence with an E value of 0.11.
The motif prediction of CUT1 protein Fusarium solani revealed that the fungus contains three small regions of the three-dimensional protein structure or amino acid sequence known as motifs which had virulence hydrolase, and (Figure 2).

FIGURE 2. Promising functional motifs present in CUT1 protein predicted by Motif finder (Number of motifs is 3, and it is virulence protein).
Antimicrobial peptides (AMPs) data collection and profile construction
Profile creation was carried out by random division of the experimentally validated anti-Fusarium solani antimicrobial peptides (AMPs) (Table 2). Subsequently, HMMER was used to cluster, build, and scan putative AMPs with the tendency to detect Fusarium solani. The experimentally validated anti-Fusarium solani AMPs were collected from CAMP, APD3, DBAASP, and BACTIBASE in which literature mining revealed the presence of 16 AMPs against Fusarium solani after removal of duplicate.
Testing of performance of the profile
The created profile of the anti-Fusarium solani AMPs was tested against the positive dataset, which was 25% of the total AMPs used to create the profile. A negative control dataset was also used, containing a random fragment of 17236 neuropeptides with no anti-Fusarium solani activities (Table 3). The result revealed that only three of the four positive testing datasets were true positive while the profile discriminated against all the 17236 negative datasets (neuropeptides). The performance results also revealed that the profile was sensitive, accurate, and specific, with much significant Matthews correlation coefficient (MCC) (Table 2).
Discovery of anti-Fusarium solani AMPs
Novel anti-Fusarium solani AMPs were discovered using HMMER with a set cut-off E value at 0.05. This yielded five AMPs across all proteomes scanned which adhered to the cut-off (Table 4). The AMPs were ranked according to their E values, with the lowest coming first on the list.
Physicochemical analysis of the anti-Fusarium solani AMPs and Fusarium solani CUT1 protein
Physicochemical parameters such as molecular weight, isoelectric point, percentage hydrophobicity, Boman index, net charge, and half-life were used to evaluate the resulting anti-Fusarium solani AMPs (Table 5). OOB1-5 had their most common amino acids: serine, glycine, threonine plus serine, arginine, and glutamate plus glycine. All the AMPs had significant hydrophobicity, with the lowest recorded for OOB3 (27%) and 5 (29%), which revealed the total percentage hydrophobicity as recorded for both APD3 and BACTIBASE. All the AMPs had positive charges with the exception of OOB2 and 5, which had 0 and −4, respectively. The isoelectric point of the AMPs was between 4.23 and 11.17, with the Boman index ranging from 2.14 to 2.99. Also, all the AMPs had a significant half-life, with the lowest recorded for OOB4 (1 h).
In Table 6 below, CUT1 protein of Fusarium solani had alanine as the most common amino acid, molecular weight of 23985.36 Da, 52% hydrophobicity, the isoelectric point of 8.13, net charge of +3 and a half=life of 30 h. The protein also had instability and aliphatic indices of 22.84 and 87.17, respectively.
Structure prediction of the anti-Fusarium solani AMPs and Fusarium solani CUT1 protein
The structures of the anti-Fusarium solani AMPs and Fusarium solani CUT1 protein were predicted utilizing parameters such as confidence score (C-score), Template modelling score (TM score), and Root means square deviation (RMSD) (Å) (Table 7). C-score is used to estimate the quality of the prediction by I-TASSER based on the significance of threading template alignments and the convergence parameters of the structure assembly simulations. C-score ranges from −5 to 2 for a model with high confidence (Zhang, 2008). All predicted models had significant C-score indicating that the 3-D structures of the putative AMPs and CUT1 protein were predicted with high confidence. Also, a TM score >0.5 indicates a model of correct topology and a TM-score < 0.17 means a random similarity. OOB2, 5, and CUT1 had correct topology, while OOB1, 3, and four had random similarities. The random similarity could be due to a lack of templates for predicting these molecules, an indication of their novelty (Bakare et al., 2020). For RMSD, 3-D structure prediction does not have a definitive RMSD value (Bakare et al., 2021a).
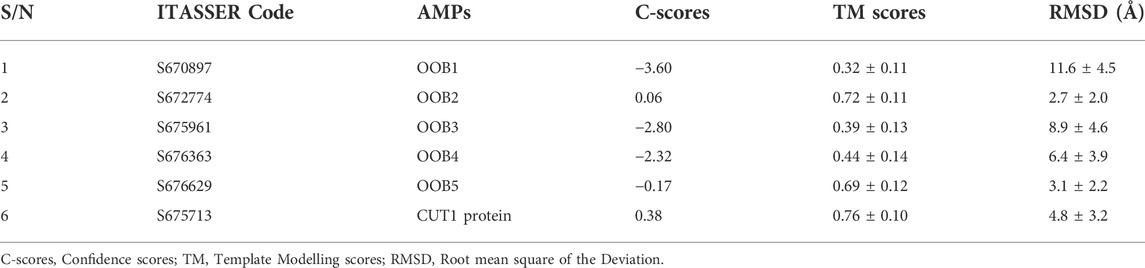
TABLE 7. Structure prediction of the anti-Fusarium solani AMPs and Fusarium solani CUT1 protein from I-TASSER.
The output images of the anti-Fusarium solani AMPs from the I-TASSER server and the CUT1 receptor are displayed in Figure 3. The representative 3-D structures showed that the putative AMPs and CUT1 protein displayed various secondary structures, α-helices, parallel β-sheet, anti-parallel β-sheet, extended, and loop conformational structures (Ramamoorthy et al., 2006; Sengupta et al., 2008).
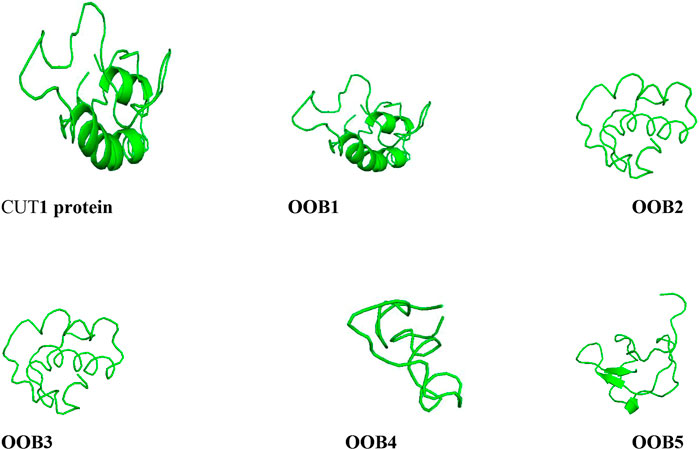
FIGURE 3. Structures of the anti-Fusarium solani AMPs and Fusarium solani cut1 protein all represented in ash colour visualized using PyMol.
Docking interaction analysis of the anti-Fusarium solani AMPs and Fusarium solani CUT1 protein
The docking interaction analysis between the anti-Fusarium solani AMPs and Fusarium solani CUT1 protein was predicted using pyDockWeb and HDock servers (Table 8). All the anti-Fusarium solani AMPs bound tightly with the CUT1 protein with the highest binding energy displayed for OOB4 in HDock. Despite high electrostatic, desolvation, and low van der Waals interaction energies generated for all-AMPs by pyDockWeb, OOB1 was ranked most significant.
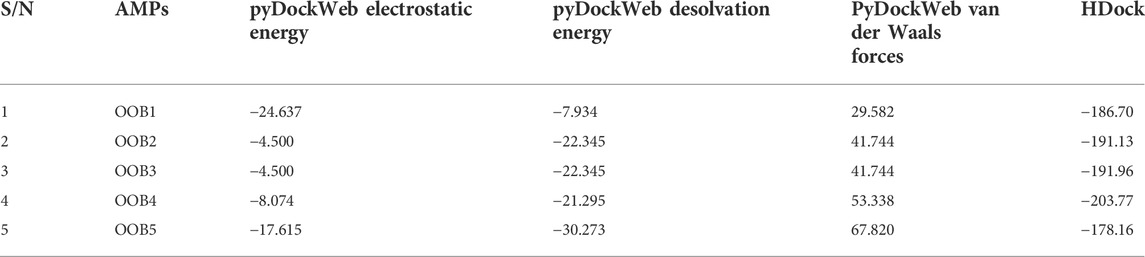
TABLE 8. Docking interaction analysis of the putative AMPs and CUT1 protein using pyDockWeb and HDock.
The output images from the HDock server showing the mode of binding and orientation of the anti-Fusarium solani AMPs along the Fusarium solani CUT1 protein are displayed in Figure 4. OOB1, 2, 3, and four had similar binding modes and orientations along the CUT1 protein, with only OOB5 binding differently. The observed differences in the orientation could be attributed to the interaction between the amino acid residues of the anti-Fusarium solani antimicrobial peptides and the CUT1 respectively (Jaakola et al., 2010).
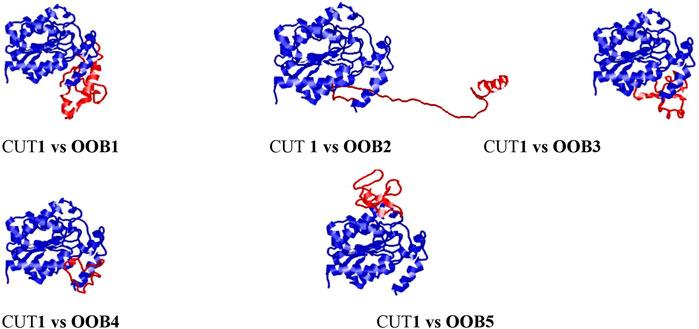
FIGURE 4. Docking interaction complexes between cut1 receptor protein (represented in blue) and anti-Fusarium solani AMPs (represented in red) visualized using RasMol.
Discussion
Identification of Fusarium solani through model construction of detection biomarkers could pave the way toward saving infected crop plants for an abundant harvest and greater economic value. The present research identified CUT1 protein as a target for detecting Fusarium solani during infection. CUT1 protein of Fusarium solani, as used in this research, is a stable protein based on its fortification with polar, non-polar, aromatic, and cysteine amino acids, which were contributed by the hydrophobic core, hydrogen bonding, net charge and the ionization state of the amino acid residues, a necessary criterion for receptor molecule used for identification purposes (Pace et al., 2014). This receptor protein had an excellent and unique model structure prediction in its secondary structure. The presence of chemical forces between protein and its immediate environment of CUT1 and the noncovalent bonds between amino acids could also explain its stability (Pace et al., 2014). The disulphide bridges in this receptor molecule were essential for protein stability, in which their disruption could result in loss of enzymatic activity (Pace et al., 2009).
The Fusarium solani CUT1 also displayed a high quality in its model validation with abundant most favoured regions for the reception of ligands capable of being biomarkers. Also, the three motifs of the fungal CUT1 protein are recognizable regions of protein structure with unique virulence functions for penetration into the host plant cuticle (Hanschen et al., 2014). The essence of the validation and motif finding steps was to ascertain that the CUT1 protein had evolutionarily more conserved regions for Fusarium solani than other regions of proteins in other organisms (Rost et al., 2003). Identifying motifs in proteins is necessary for classifying protein sequences and detecting functional annotation (Wu et al., 2003). Thus, CUT1 protein of Fusarium solani had promising functional motifs ranging from hydrolytic to virulent functions apart from being an evolutionarily conserved molecule.
AMPs have gained widespread attention from researchers as theranostic molecules because of their compensatory advantages over conventional antibodies during diagnosis and ease of penetration due to their favourable biochemical nature and small size (Tincho et al., 2016; Aruleba et al., 2018). The use of HMMER for the construction of models to identify pathogens as used in this research work is deemed appropriate in the field of diagnostics due to its correct prediction of models against specific organism types (Tincho et al., 2016). A model of the retrieved AMPs was constructed using HMMER after random partition into two datasets. The essence of the arbitrary partition exercise of the datasets into training and testing and the independent testing of the profile was to ascertain the robustness and the discriminatory power of the profile built by HMMER (Wu et al., 2003). The anti-Fusarium solani AMP model constructed was sensitive, accurate, and specific, with an excellent Matthews correlation coefficient using performance parameters. The model generated five anti-Fusarium solani AMPs across all proteomes of organisms scanned with significant E-values less than 0.05, with the lowest E-value recorded for 00B1.
The physicochemical parameters of the AMPs were computed to ascertain that they were bona vide AMPs. The Boman index was greater than 1 for all AMPs, indicating the greater capacity to bind to its receptor during pathogen detection with their percentage hydrophobicity above 30% except 00B3 and 5. The reduced hydrophobicity of OOB3 and 5 is the proportion of the polar amino acids above the non-polar ones. All the AMPs generated were cationic except OOB2 with a neutral charge. The absence of a positive charge does not interpret the absence of antimicrobial activity because some non-cationic AMPs have been reported with more excellent antimicrobial activities (Bakare et al., 2020).
The pyDockWeb generated three energy interactions: electrostatic, desolvation, and van der Waals interaction energies (Jiménez-García et al., 2013). The different structural formations such as alpha-helices and extended sheets of the putative anti-Fusarium solani AMPs in their 3-D structures can help folding complementation, insertion and intermolecular interaction (Aruleba et al., 2018). This is because alpha-helices exhibit efficient use of hydrogen bonds during the binding of the amino group hydrogen and the carboxyl group oxygen. Thus, the presence of alpha-helices in the AMPs makes them interact with another biomolecule for significant impact as targets during detection (Kumar et al., 2018). The 3-D structure of the CUT1 protein and the putative anti-Fusarium solani AMPs showed good quality, as indicated by the C-scores, TM-scores and RMSD values (Scholtz and Baldwin, 1992). Furthermore, the docking interaction study ascertained the binding energy displayed for HDock during detection with the putative anti- Fusarium solani AMP (OOB4) binding with the greatest affinity to Fusarium solani CUT1 protein (Zhang and Skolnick, 2004). Van der Waals forces gave the relatively weak electric forces that attracted neutral molecules between the anti-Fusarium solani AMPs and CUT1 protein (Hermann and Tkatchenko, 2020). The electrostatic energy referred to the electromagnetic gradient, which occurred when there were no moving electrical charges between the anti-Fusarium solani AMPs and Fusarium solani CUT1 protein (Wang et al., 2020). In the aqueous environment; desolvation energy was generated with the behavioural pattern of the CUT1 protein and anti-Fusarium solani AMPs (Hou et al., 2020). Overall, the pyDockWeb server had all the AMPs with significant values for these energy values, with OOB1 having the greatest electrostatic and desolvation energies and the lowest van der Waals interaction.
The tendency of the putative AMPs generated from this study to generate appreciable binding potential to CUT1 protein of Fusarium solani can be pursued for the rational design of a novel, selective and potent biomarker for the identification of Fusarium solani. Thus, this research produces new insights into the in silico modular architecture of the evolutionarily conserved host defense molecules such as AMPs with diagnostic helices associated with antimicrobial activity against fungal pathogens such as Fusarium solani.
Conclusion
The present research used in silico analysis to detect biomolecules for the sensitive identification of Fusarium solani. AMPs have shown great promise in circumventing the drawbacks associated with the current diagnostic systems. Five anti-Fusarium solani AMPs were identified with significant physicochemical parameters to detect Fusarium solani using CUT1 receptor protein as a target. The generated AMPs were sensitive and specific against Fusarium solani. OOB1 ranked the most significant binding energy interaction, while OOB1 had the most effective interaction with Fusarium solani CUT1 protein. The putative anti-Fusarium solani AMPs generated from this analysis could be used to prevent the catastrophic losses of the crops mentioned earlier due to the fungus, ranging from reducing the harvest quality and plant survivorship to aiding plant competitive ability.
Data availability statement
The datasets presented in this study can be found in online repositories. The names of the repository/repositories and accession number(s) can be found in the article/Supplementary Material.
Author contributions
OB, AG, MJ, AK, and MK wrote the manuscript. OB, AG, and MK edited and checked the manuscript. All authors contributed to the article and approved the submitted version. All authors have read and agreed to the published version of the manuscript.
Funding
Financial support received by OB, a recipient of an NRF Post-Doctoral Fellowship (Grant number: 120712). This research was also financially supported by the NRF to MK (Grant numbers: 116346 and 109083), AK (Grant numbers: 107023 and 115280), and AG (Grant number: 129493). Part of the research was funded by a DST-NRF Centre of Excellence in Food Security award (Project ID: 170202) and GrainSA (GB0200065; GB0200066).
Acknowledgments
The authors would like to thank the various institutions, namely, the University of the Western Cape and the University of Free State, Olabisi Onabanjo University, for infrastructure and administrative support.
Conflict of interest
The authors declare that the research was conducted in the absence of any commercial or financial relationships that could be construed as a potential conflict of interest.
Publisher’s note
All claims expressed in this article are solely those of the authors and do not necessarily represent those of their affiliated organizations, or those of the publisher, the editors and the reviewers. Any product that may be evaluated in this article, or claim that may be made by its manufacturer, is not guaranteed or endorsed by the publisher.
Supplementary Material
The Supplementary Material for this article can be found online at: https://www.frontiersin.org/articles/10.3389/fbinf.2022.972529/full#supplementary-material
References
Agnoli, K., Schwager, S., Uehlinger, S., Vergunst, A., Viteri, D. F., Nguyen, D. T., et al. (2012). Exposing the third chromosome of Burkholderia cepacia complex strains as a virulence plasmid. Mol. Microbiol. 83 (2), 362–378. doi:10.1111/j.1365-2958.2011.07937.x
Artimo, P., Jonnalagedda, M., Arnold, K., Baratin, D., Csardi, G., de Castro, E., et al. (2012). ExPASy: SIB bioinformatics resource portal. Nucleic acids Res. 40 (W1), W597–W603. doi:10.1093/nar/gks400
Aruleba, R. T., Adekiya, T., Oyinloye, B., and Kappo, A. (2018). Structural studies of predicted ligand binding sites and molecular docking analysis of Slc2a4 as a therapeutic target for the treatment of cancer. Int. J. Mol. Sci. 19 (2), 386. doi:10.3390/ijms19020386
Bahar, A. A., and Ren, D. (2013). Antimicrobial peptides. Pharmaceuticals 6 (12), 1543–1575. doi:10.3390/ph6121543
Bakare, O. O., Gokul, A., and Keyster, M. (2021). PR-1-like protein as a potential target for the identification of Fusarium oxysporum: An in silico approach. Biotech 10 (2), 8. doi:10.3390/biotech10020008
Bakare, O. O., Keyster, M., and Pretorius, A. (2021). Building HMM and molecular docking analysis for the sensitive detection of anti-viral pneumonia antimicrobial peptides (AMPs). Sci. Rep. 11 (1), 20621–20715. doi:10.1038/s41598-021-00223-8
Bakare, O. O., Keyster, M., and Pretorius, A. (2020). Identification of biomarkers for the accurate and sensitive diagnosis of three bacterial pneumonia pathogens using in silico approaches. BMC Mol. Cell Biol. 21 (1), 82–13. doi:10.1186/s12860-020-00328-4
Bhat, M. N., Mesta, R., Yenjerappa, S., Tatagar, M., Sardana, H., Singh, D., et al. (2016). Biological control of Fusarium wilt of chillies using Trichoderma spp. Indian J. Hortic. 73 (1), 74–77. doi:10.5958/0974-0112.2016.00021.9
Bhatti, H. N., Batool, S., and Afzal, N. (2013). Production and characterization of a novel (beta)-glucosidase from Fusarium solani. Int. J. Agric. Biol. 15 (1).
Carlson, J. M., Chakravarty, A., DeZiel, C. E., and Gross, R. H. (2007). Scope: A web server for practical de novo motif discovery. Nucleic acids Res. 35 (2), W259–W264. doi:10.1093/nar/gkm310
Chehri, K., Salleh, B., and Zakaria, L. (2015). Morphological and phylogenetic analysis of Fusarium solani species complex in Malaysia. Microb. Ecol. 69 (3), 457–471. doi:10.1007/s00248-014-0494-2
Coleman, J. J., Rounsley, S. D., Rodriguez-Carres, M., Kuo, A., Wasmann, C. C., Grimwood, J., et al. (2009). The genome of nectria haematococca: Contribution of supernumerary chromosomes to gene expansion. PLoS Genet. 5 (8), e1000618. doi:10.1371/journal.pgen.1000618
Consortium, U. (2015). UniProt: A hub for protein information. Nucleic acids Res. 43 (D1), D204–D212. doi:10.1093/nar/gku989
Eswar, N., and Sali, A. (2009). “Protein structure modeling,” in From molecules to medicine, structure of biological macromolecules and its relevance in combating new diseases and bioterrorism. Editors J. L. Sussman, and P. Spadon (Dordrecht, Netherlands: Springer-Verlag), 139–151.
Fang, Q. (2018). Predicting functional alterations caused by non-synonymous variants in CHO using models based on phylogenetic tree and evolutionary preservation. University of Sheffield.
Hammami, R., Zouhir, A., Ben Hamida, J., and Fliss, I. (2007). Bactibase: A new web-accessible database for bacteriocin characterization. BMC Microbiol. 7 (1), 89–96. doi:10.1186/1471-2180-7-89
Hammami, R., Zouhir, A., Le Lay, C., Ben Hamida, J., and Fliss, I. (2010). BACTIBASE second release: A database and tool platform for bacteriocin characterization. BMC Microbiol. 10 (1), 22–25. doi:10.1186/1471-2180-10-22
Hanschen, F. S., Lamy, E., Schreiner, M., and Rohn, S. (2014). Reactivity and stability of glucosinolates and their breakdown products in foods. Angew. Chem. Int. Ed. 53 (43), 11430–11450. doi:10.1002/anie.201402639
Hartman, G. L., West, E. D., and Herman, T. K. (2011). Crops that feed the world 2. Soybean—worldwide production, use, and constraints caused by pathogens and pests. Food Secur. 3 (1), 5–17. doi:10.1007/s12571-010-0108-x
He, D., Hao, J., Zhang, B., Yang, Y., Song, W., Zhang, Y., et al. (2011). Pathogenic spectrum of fungal keratitis and specific identification of Fusarium solani. Invest. Ophthalmol. Vis. Sci. 52 (5), 2804–2808. doi:10.1167/iovs.10-5977
Hermann, J., and Tkatchenko, A. (2020). Density functional model for van der Waals interactions: Unifying many-body atomic approaches with nonlocal functionals. Phys. Rev. Lett. 124 (14), 146401. doi:10.1103/physrevlett.124.146401
Hou, Z., Tan, H., Gao, Y., Li, M., Lu, Z., and Zhang, B. (2020). Tailoring desolvation kinetics enables stable zinc metal anodes. J. Mat. Chem. A Mat. 8 (37), 19367–19374. doi:10.1039/d0ta06622b
Hubbard, T., Barker, D., Birney, E., Cameron, D., Chen, Y., Cox, T., et al. (2002). The Ensembl genome database project. Nucleic acids Res. 30 (1), 38–41. doi:10.1093/nar/30.1.38
Hurst, G. D. S. (1995). Fusarium solani in aqueous cutting fluids. United Kingdom: University of Surrey.
Jaakola, V.-P., Lane, J. R., Lin, J. Y., Katritch, V., IJzerman, A. P., and Stevens, R. C. (2010). Ligand binding and subtype selectivity of the human A2A adenosine receptor: Identification and characterization of essential amino acid residues. J. Biol. Chem. 285 (17), 13032–13044. doi:10.1074/jbc.m109.096974
Jiménez-García, B., Pons, C., and Fernández-Recio, J. (2013). pyDockWEB: a web server for rigid-body protein–protein docking using electrostatics and desolvation scoring. Bioinformatics 29 (13), 1698–1699. doi:10.1093/bioinformatics/btt262
Kumar, P., Kizhakkedathu, J. N., and Straus, S. K. (2018). Antimicrobial peptides: Diversity, mechanism of action and strategies to improve the activity and biocompatibility in vivo. Biomolecules 8 (1), 4. doi:10.3390/biom8010004
Kurt, Ş., Uysal, A., Soylu, E. M., Kara, M., and Soylu, S. (2020). Characterization and pathogenicity of Fusarium solani associated with dry root rot of citrus in the eastern Mediterranean region of Turkey. J. Gen. Plant Pathol. 86 (4), 326–332. doi:10.1007/s10327-020-00922-6
Kyte, J., and Doolittle, R. F. (1982). A simple method for displaying the hydropathic character of a protein. J. Mol. Biol. 157 (1), 105–132. doi:10.1016/0022-2836(82)90515-0
Laskowski, R., MacArthur, M., and Thornton, J. (2006). Procheck: Validation of protein-structure coordinates. Int. Tables Crystallogr. 722–725. doi:10.1107/97809553602060000882
Li, D., Sirakova, T., Rogers, L., Ettinger, W. F., and Kolattukudy, P. (2002). Regulation of constitutively expressed and induced cutinase genes by different zinc finger transcription factors inFusarium solani f. sp. pisi (nectria haematococca). J. Biol. Chem. 277 (10), 7905–7912. doi:10.1074/jbc.m108799200
Li, W., and Godzik, A. (2006). Cd-Hit: A fast program for clustering and comparing large sets of protein or nucleotide sequences. Bioinformatics 22 (13), 1658–1659. doi:10.1093/bioinformatics/btl158
Mahawar, H., Prasanna, R., and Gogoi, R. (2019). Elucidating the disease alleviating potential of cyanobacteria, copper nanoparticles and their interactions in Fusarium solani challenged tomato plants. Plant Physiol. Rep. 24 (4), 533–540. doi:10.1007/s40502-019-00490-8
Mavhunga, M. (2020). A survey of Fusarium species occurring in the grassland biome of South Africa. South Africa: University of Johannesburg.
McGuffin, L. J., Bryson, K., and Jones, D. T. (2000). The PSIPRED protein structure prediction server. Bioinformatics 16 (4), 404–405. doi:10.1093/bioinformatics/16.4.404
Mecteau, M., Arul, J., and Tweddell, R. (2008). Effect of different salts on the development of Fusarium solani var. coeruleum, a causal agent of potato dry rot. phyto. 89 (1), 1–6. doi:10.7202/000377ar
Nidiry, E. S. J., Ganeshan, G., and Lokesha, A. (2011). Antifungal activity of Mucuna pruriens seed extractives and L-dopa. J. Herbs, Spices Med. Plants 17 (2), 139–143. doi:10.1080/10496475.2011.581135
Pace, C. N., Fu, H., Lee Fryar, K., Landua, J., Trevino, S. R., Schell, D., et al. (2014). Contribution of hydrogen bonds to protein stability. Protein Sci. 23 (5), 652–661. doi:10.1002/pro.2449
Pace, C. N., Grimsley, G. R., and Scholtz, J. M. (2009). Protein ionizable groups: pK values and their contribution to protein stability and solubility. J. Biol. Chem. 284 (20), 13285–13289. doi:10.1074/jbc.r800080200
Pham, C. L., Rey, A., Lo, V., Soules, M., Ren, Q., Meisl, G., et al. (2016). Self-assembly of MPG1, a hydrophobin protein from the rice blast fungus that forms functional amyloid coatings, occurs by a surface-driven mechanism. Sci. Rep. 6 (1), 25288–25316. doi:10.1038/srep25288
Pirtskhalava, M., Gabrielian, A., Cruz, P., Griggs, H. L., Squires, R. B., Hurt, D. E., et al. (2016). DBAASP v. 2: An enhanced database of structure and antimicrobial/cytotoxic activity of natural and synthetic peptides. Nucleic Acids Res. 44 (D1), 6503–D1112. doi:10.1093/nar/gkw243
Pruitt, K. D., Tatusova, T., and Maglott, D. R. (2005). NCBI reference sequence (RefSeq): A curated non-redundant sequence database of genomes, transcripts and proteins. Nucleic acids Res. 33 (1), D501–D504. doi:10.1093/nar/gki025
Raaijmakers, J. M., Paulitz, T. C., Steinberg, C., Alabouvette, C., and Moenne-Loccoz, Y. (2009). The rhizosphere: A playground and battlefield for soilborne pathogens and beneficial microorganisms. Plant Soil 321 (1), 341–361. doi:10.1007/s11104-008-9568-6
Ramamoorthy, A., Thennarasu, S., Tan, A., Gottipati, K., Sreekumar, S., Heyl, D. L., et al. (2006). Deletion of all cysteines in tachyplesin I abolishes hemolytic activity and retains antimicrobial activity and lipopolysaccharide selective binding. Biochemistry 45 (20), 6529–6540. doi:10.1021/bi052629q
Rasmey, A. H., Tawfik, M., and Abdel‐Kareem, M. (2020). Direct transesterification of fatty acids produced by Fusarium solani for biodiesel production: Effect of carbon and nitrogen on lipid accumulation in the fungal biomass. J. Appl. Microbiol. 128 (4), 1074–1085. doi:10.1111/jam.14540
Rehman, A., Rehman, A. U., Javed, N., Ullah Malik, A., and Mehboob, S. (2012). Toxin production by Fusarium solani from declining citrus plants and its management. Afr. J. Biotechnol. 11 (9), 2199–2203. doi:10.5897/AJB11.2125
Rost, B., Ofran, Y., Nair, R., and Liu, J. (2003). Automatic prediction of protein function. Cell. Mol. Life Sci. 60 (12), 2637–2650. doi:10.1007/s00018-003-3114-8
Roy, A., Kucukural, A., and Zhang, Y. (2010). I-TASSER: A unified platform for automated protein structure and function prediction. Nat. Protoc. 5 (4), 725–738. doi:10.1038/nprot.2010.5
Sayle, R. A., and Milner-White, E. J. (1995). Rasmol: Biomolecular graphics for all. Trends Biochem. Sci. 20 (9), 374–376. doi:10.1016/s0968-0004(00)89080-5
Scholtz, J. M., and Baldwin, R. L. (1992). The mechanism of alpha-helix formation by peptides. Annu. Rev. Biophys. Biomol. Struct. 21 (1), 95–118. doi:10.1146/annurev.bb.21.060192.000523
Scully, E. D., Hoover, K., Carlson, J., Tien, M., and Geib, S. M. (2012). Proteomic analysis of Fusarium solani isolated from the Asian longhorned beetle, Anoplophora glabripennis. PLoS One 7 (4), e32990. doi:10.1371/journal.pone.0032990
Sengupta, D., Leontiadou, H., Mark, A. E., and Marrink, S. J. (2008). Toroidal pores formed by antimicrobial peptides show significant disorder. Biochimica Biophysica Acta - Biomembr. 1778 (10), 2308–2317. doi:10.1016/j.bbamem.2008.06.007
Shakeel, Q., Wu, M., and Zhang, J. (2020). “Fungal diseases of cat palm (chamaedorea cataractarum), bamboo palm (chamaedorea seifrizii) and cluster palm (chamaedorea costaricana),” in Etiology and integrated management of economically important fungal diseases of ornamental palms (Springer), 125–140.
Šišić, A., Al-Hatmi, A. M. S., Bacanovic-Sisic, J., Ahmed, S. A., Dennenmoser, D., de Hoog, G. S., et al. (2018). Two new species of the Fusarium solani species complex isolated from compost and hibiscus (Hibiscus sp.). Antonie Leeuwenhoek 111 (10), 1785–1805. doi:10.1007/s10482-018-1068-y
Thomas, S., Karnik, S., Barai, R. S., Jayaraman, V. K., and Idicula-Thomas, S. (2010). Camp: A useful resource for research on antimicrobial peptides. Nucleic acids Res. 38 (1), D774–D780. doi:10.1093/nar/gkp1021
Tincho, M., Gabere, M., and Pretorius, A. (2016). In silico identification and molecular validation of putative antimicrobial peptides for HIV therapy. J. AIDS Clin. Res. 7 (9). doi:10.4172/2155-6113.1000606
van Diepeningen, A. D., Brankovics, B., Iltes, J., van der Lee, T. A. J., and Waalwijk, C. (2015). Diagnosis of Fusarium infections: Approaches to identification by the clinical mycology laboratory. Curr. Fungal Infect. Rep. 9 (3), 135–143. doi:10.1007/s12281-015-0225-2
Wang, G., Li, X., and Wang, Z. (2009). APD2: The updated antimicrobial peptide database and its application in peptide design. Nucleic acids Res. 37 (1), D933–D937. doi:10.1093/nar/gkn823
Wang, J. T.-L., Wang, X., Shasha, D., and Zhang, K. (2005). Metricmap: An embedding technique for processing distance-based queries in metric spaces. IEEE Trans. Syst. Man. Cybern. B 35 (5), 973–987. doi:10.1109/tsmcb.2005.848489
Wang, P.-J., Zhou, D., Li, J., Pang, L. X., Liu, W. F., Su, J. Z., et al. (2020). Significantly enhanced electrostatic energy storage performance of P (VDF-HFP)/BaTiO3-Bi (Li0. 5Nb0. 5) O3 nanocomposites. Nano Energy 78, 105247. doi:10.1016/j.nanoen.2020.105247
Wang, Z., and Wang, G. (2004). Apd: The antimicrobial peptide database. Nucleic acids Res. 32 (1), D590–D592. doi:10.1093/nar/gkh025
Wei, R., Oeser, T., and Zimmermann, W. (2014). Synthetic polyester-hydrolyzing enzymes from thermophilic actinomycetes. Adv. Appl. Microbiol. 89, 267–305. doi:10.1016/b978-0-12-800259-9.00007-x
Williams, M. E., and Tincho, M. (2016). Molecular validation of putative antimicrobial peptides for improved human immunodeficiency virus diagnostics via HIV protein p24. J. AIDS Clin. Res. 7 (571), 2. doi:10.4172/2155-6113.1000571
Wu, C. H., Huang, H., Yeh, L. S. L., and Barker, W. C. (2003). Protein family classification and functional annotation. Comput. Biol. Chem. 27 (1), 37–47. doi:10.1016/s1476-9271(02)00098-1
Xiang, L., Wang, M., Pan, F., Wang, G., Jiang, W., Wang, Y., et al. (2021). Transcriptome analysis Malus domestica ‘M9T337’root molecular responses to Fusarium solani infection. Physiological Mol. Plant Pathology 113, 101567. doi:10.1016/j.pmpp.2020.101567
Yan, Y., Tao, H., He, J., and Huang, S. Y. (2020). The HDOCK server for integrated protein–protein docking. Nat. Protoc. 15 (5), 1829–1852. doi:10.1038/s41596-020-0312-x
Zaccardelli, M., Vitale, S., Luongo, L., Merighi, M., and Corazza, L. (2008). Morphological and molecular characterization of Fusarium solani isolates. J. Phytopathology 156 (9), 534–541. doi:10.1111/j.1439-0434.2008.01403.x
Zhang, L.-j., and Gallo, R. L. (2016). Antimicrobial peptides. Curr. Biol. 26 (1), R14–R19. doi:10.1016/j.cub.2015.11.017
Zhang, Y. (2008). I-TASSER server for protein 3D structure prediction. BMC Bioinforma. 9 (1), 40–48. doi:10.1186/1471-2105-9-40
Keywords: Fusarium solani, protein, in silico, energies, fungus
Citation: Bakare OO, Gokul A, Jimoh MO, Klein A and Keyster M (2022) In silico discovery of biomarkers for the accurate and sensitive detection of Fusarium solani. Front. Bioinform. 2:972529. doi: 10.3389/fbinf.2022.972529
Received: 18 June 2022; Accepted: 22 August 2022;
Published: 30 September 2022.
Edited by:
Pu-Feng Du, Tianjin University, ChinaReviewed by:
Xiaolei Zhu, Anhui Agricultural University, ChinaRodrigo Ligabue-Braun, Federal University of Health Sciences of Porto Alegre, Brazil
Copyright © 2022 Bakare, Gokul, Jimoh, Klein and Keyster. This is an open-access article distributed under the terms of the Creative Commons Attribution License (CC BY). The use, distribution or reproduction in other forums is permitted, provided the original author(s) and the copyright owner(s) are credited and that the original publication in this journal is cited, in accordance with accepted academic practice. No use, distribution or reproduction is permitted which does not comply with these terms.
*Correspondence: Olalekan Olanrewaju Bakare, lekanbakare77@gmail.com; Marshall Keyster, mkeyster@uwc.ac.za