- 1Institute of Chemistry, Academia Sinica, Taipei, Taiwan
- 2Department of Applied Mathematics, National Sun Yat-sen University, Kaohsiung, Taiwan
- 3Institute of Statistical Science, Academia Sinica, Taipei, Taiwan
The functions of biological macromolecules are often associated with conformational malleability of the structures. This phenomenon of chemically identical molecules with different structures is coined structural polymorphism. Conventionally, structural polymorphism is observed directly by structural determination at the density map level from X-ray crystal diffraction. Although crystallography approach can report the conformation of a macromolecule with the position of each atom accurately defined in it, the exploration of structural polymorphism and interpreting biological function in terms of crystal structures is largely constrained by the crystal packing. An alternative approach to studying the macromolecule of interest in solution is thus desirable. With the advancement of instrumentation and computational methods for image analysis and reconstruction, cryo-electron microscope (cryo-EM) has been transformed to be able to produce “in solution” structures of macromolecules routinely with resolutions comparable to crystallography but without the need of crystals. Since the sample preparation of single-particle cryo-EM allows for all forms co-existing in solution to be simultaneously frozen, the image data contain rich information as to structural polymorphism. The ensemble of structure information can be subsequently disentangled through three-dimensional (3D) classification analyses. In this review, we highlight important examples of protein structural polymorphism in relation to allostery, subunit cooperativity and function plasticity recently revealed by cryo-EM analyses, and review recent developments in 3D classification algorithms including neural network/deep learning approaches that would enable cryo-EM analyese in this regard. Finally, we brief the frontier of cryo-EM structure determination of RNA molecules where resolving the structural polymorphism is at dawn.
Introduction
The concept of structural polymorphism has been associated with structure research in the beginning. In material science, structural polymorphism depicts the existence of a solid material in more than one form or crystal structure. Biological macromolecules are “soft material” that can readily change forms. We thus refer to “one biomolecule with multiple structures” as “structural polymorphism”. Proteins offer the best known example of biomolecule to show structural polymorphism. The tertiary structure of a protein can undergo minor or even global conformational changes depending on the intrinsic properties of the protein and external stimuli. Some proteins exhibit the capacity to rearrange the structures in response to an environmental trigger (e.g., pH, temperature/salt ions, small molecules, redox). Remarkably, the alteration of a local structure is capable of inducing the change of a remote site in the same molecule. Such “allosteric behavior” of a protein is not only germane to mechanistic understanding of many fundamental biological processes, but also to understanding of the function of agonists/antagonists in the field of pharmacology. Thus structural polymorphism in proteins is an area of great interests.
Proteins, arguably the most versatile macromolecules in living systems, serve crucial functions in virtually all biological processes. Made up of linear polymers with building units of amino acids, proteins can fold up into three-dimensional structures determined by the sequence of amino acids. Based on the structure-function paradigm ushered in by Anfinsen in the 60s, the function of a protein is directly dependent on its three-dimensional structure (Anfinsen and Haber, 1961). In late 50s Koshland hypothesized structural change of an enzyme at the active center (Levy et al., 1959), which was used to explain the temperature dependence of ATP hydrolysis rate by myosin (Koshland, 1958) and other enzymes in general. Eventually, this line of research had further expanded into a branch in enzymology on how changes in conformation would have function consequence for proteins to assume different functions or switch between inactive and active states with high specificity and affinity.
In the structural biology community, structural polymorphism of proteins can be loosely classified as either structural changes or dynamical changes. The former would involve different protein conformations (i.e. distinct ensemble of one protein subunit), protein configurations (i.e. distinct ensemble of a protein with multiple subunits), and protein states (i.e. equilibrium states such as open and close state of an ion channel) among others. The latter may include allostery (i.e. non-equilibrium based structural changes) and transition from one state to another. Recently it has been uncovered that intrinsically disordered proteins (IDPs) comprise a significant fraction of the proteome. These proteins exhibit polymorphic ensemble of conformations rather than a unique structure (Kulkarni et al., 2018). In any case, the above mentioned classification of structural polymorphism is perhaps phenomenological.
At fundamental level, structural polymorphism can perhaps be described using the theory of energy landscape (Frauenfelder et al., 1991). Proposed by Frauenfelder in the 70s to explain the complex kinetics observed from photolysis experiments on myoglobin (Austin et al., 1975), this notion of protein energy landscape connects structural polymorphism to thermodynamics where the manner of transitions among sub-states would be determined by the energy barriers (Figure 1).
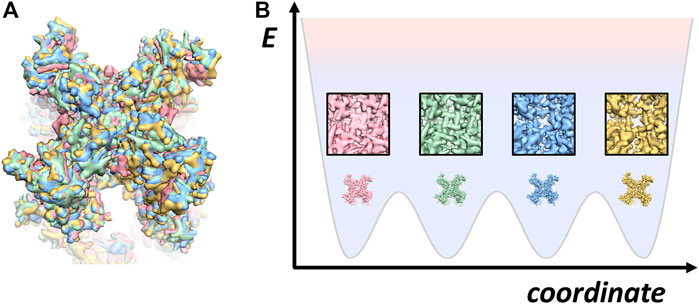
FIGURE 1. Snapshots of TRPV cryo-EM structures (A) four TRPV structures superimposed: apo followed by three different conformations bound with DkTx toxin (EMD-23136, PDB: 7L2P; EMD-23143; PDB: 7L2S; EMD-23141; PDB: 7L2T; EMD-23141; PDB: 7L2U) (Zhang et al., 2021). (B) a cartoon of energy landscape for illustrating the four different structures in (A).
However, visual evidence in support of multiple conformations from one protein had to come from X-ray crystal structure determination. The X-ray crystal diffraction approach with successful phasing provides observation of the electron density of an entire molecule to accurately define the positions of atoms in it. In X-ray crystallography, significantly different structures are largely derived from different crystal forms. Nonetheless, in a single crystal local motilities of a protein can be parameterized by temperature factors (B-factors) assigned to each atom when the resolution has reached 2.5 Å or beyond. These B-factors serve as indicators of the mobile chain segments, but cannot describe the exact motions during protein action (Vonrhein et al., 1995). Since most substantial chain displacements would affect packing contacts, the structural polymorphism could either destroy the crystals or change the crystal form─namely, a brand new structure often entails a new crystal form, but this new structure is again just another static structure (Schlichting et al., 1990). An example of structural polymorphism revealed by X-ray diffraction came from studies on the conformations of the enzyme adenylate kinase (AK). AK, ∼20 kDa in molecular weight, is a key signal transducing protein for controlling cellular energy homeostasis (Vonrhein et al., 1995) (see also reference therein). Heroic efforts in 1990’s on AK structure determination have produced a plethora of crystal structures (Vonrhein et al., 1995), demonstrating that protein conformational changes can be indeed drastic for this system (Schulz et al., 1990). Precisely, these conformational changes are characterized by the motions of internal chain segments relative to the main body constituted with the central parallel β-sheets (Vonrhein et al., 1995). These studies had set the quest for exploring the time trajectories of protein dynamics by single-molecule measurements (Roy et al., 2008; Tan et al., 2009) and molecular dynamics analysis (Formoso et al., 2015).
In this era of pandemic, it is important to recall early study on structural polymorphism of viral replication enzymes in relation to allostery by Peersen and his co-workers (Gong and Peersen, 2010). They assembled, purified, and crystallized poliovirus RNA-dependent RNA polymerase (RdRp) elongation complexes produced by multiple rounds of nucleotide incorporation. The crystal structures captured the active polymerase and its nucleotide triphosphate complexes in four distinct states, providing a working model describing the catalytic cycle of positive-strand RNA virus RdRps. Since RNA viruses encoding high- or low-fidelity RdRps are attenuated in the virulence, efforts were made to exploit the perturbed fidelity as the basis for rational drug design against RdRps. To do so, Hogle and Cameron (Moustafa et al., 2014) combined X-ray crystallography, spectroscopic and kinetic measurements to explain why some residues distant from the active site could affect the fidelity of nucleotide incorporation─they do so via altering the conformational dynamics of the active site.
Since its introduction in 1960s by Perutz (Perutz et al., 1968), X-ray protein crystallography had soon become the standard tool for determining the structure of proteins to atomic resolution. As the synchrotron light sources and computation software (Brünger et al., 1998) (see reference therein) were further advanced, the bottleneck of X-ray crystallography remains with crystallization. As mentioned earlier, a unique tertiary structure of a protein is likely to be output from a particular form of single crystal. In other words, a different structure would most likely come from a crystal of different symmetry or altered dimensions. This requirement of crystallization would thus impede easy access to structural polymorphism of biological macromolecules. More importantly, structural polymorphism obtained through X-ray crystallography is susceptible to criticism of not directly reflecting the functional states in solution. A notable case is yeast RNA polymerase II that manifests structural polymorphism (Fu et al., 1999; Cramer et al., 2000; Gnatt et al., 2001). As this polymorphism is associated with the swinging of a mobile domain involved in crystal packing, it was not clear at that time whether or not such polymorphism did bear direct physiological relevance. Therefore, there has been a cry for using an in solution approach to accessing the structure(s) of biological macromolecule.
Two structure technologies designed for imaging the structures of radiation-sensitive materials in non-crystalline states would answer the call─ cryo-electron microscopy (cryo-EM) (Dubochet et al., 1988; Henderson, 1995; Frank, 2002) and free electron X-ray laser (XFEL) (Ekeberg et al., 2015). The usage of XFEL single particle diffraction imaging is mostly limited to giant particles whereas the high-resolution application still requires crystals of small size. Compared to XFEL, Cryo-EM directly obtained the projection density of the target macromolecule without the need of phase retrieval. Cryo-EM, in particular the single particle analysis (Figure 2A), has undergone resolution revolution to become a mainstream structural biology method (Kühlbrandt, 2014; Nogales and Scheres, 2015). Since biological macromolecule is radiation sensitive, cryo-EM is a dose-limiting imaging technique. In this regards, the cryo-EM resolution revolution has been critically dependent on the high-sensitivity of CMOS direct electron detector (Xuong et al., 2007; McMullan et al., 2009). Camera made of such sensors that can count electrons has greatly improved the quantum efficiency (see the milestones in Figure 2B). In addition, empowered by fast signal transfer rate, the direct electron CMOS cameras produce movie data of images with very large number of pixels. This capability allows for image motion correction to rescue the loss of image contrast due to charge-induced specimen movement (Brilot et al., 2012; Li et al., 2013). Furthermore, stable electron optics and high-quality vacuum has enabled continued data collection on microscopy without human intervention for days, which plays a key role in supplying high volume of data to suffice subsequent computation of structures to near atomic resolution. Due to the simplicity of sample preparation and high-efficiency in data collection using automated cryo-EM (Tan et al., 2016), single particle cryo-EM has rapidly gained popularity in structural biology and made impact on life sciences in general by initiating structure-guided function studies. In a short period of 3 years, the percentage of atomic models in the Protein Data Bank (PDB) derived from high-quality cryo-EM maps has surpassed 10% while this figure is rapid climbing.
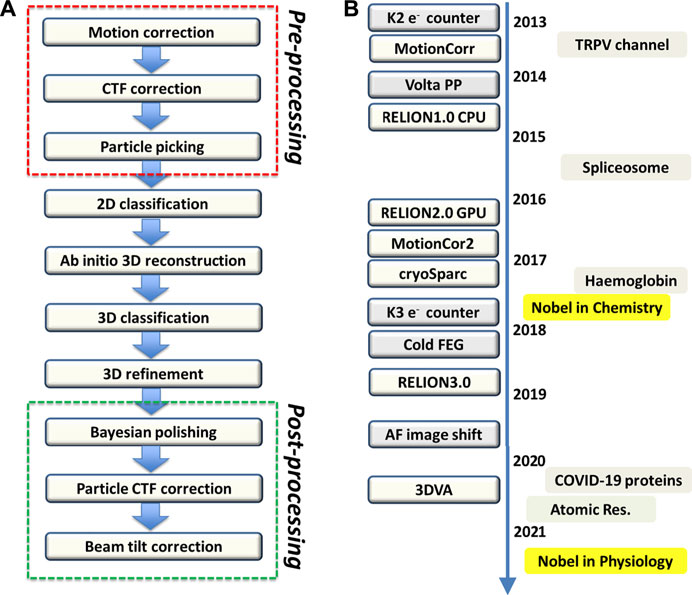
FIGURE 2. Single particle cryo-EM processing and milestones (A) Single particle processing. There are in general a total of 10 steps in the processing where the red box encloses the pre-processing steps, which can be executed in an on-the-fly manner. At the bottom, the post-processing steps encased by the green box are used to improve the overall resolution and local map quality as well. Bayesian polishing on RELION takes care of the dose weighting to compensate the frame-dependent radiation damage effect. Once a high-quality map is obtained, the variation of defocus and higher order aberration at per particle level can be further estimated and corrected. (B) Cryo-EM milestones. The boxes on the left of the timeline highlight key advancement of hardware and software where “AF image shift” stands for “aberration-free image shift.” The boxes on the right of the timeline indicate significant cryo-EM structures and events including recent rapid structure determination of COVID-19 proteins, achievement of atomic resolution with apo-ferritin, and the Nobel Physiology or Medicine in 2021 that recognizes the discoveries of TRPV and Piezo channels as heat and pressure sensors respectively where the structures were solely obtained by cryo-EM.
As single particle cryo-EM relaxes the need on using crystalline materials, it immediately opens the door for structure determination of biological macromolecules that are refractory to crystallization (e.g. TRPV in the milestones in Figure 2B). Importantly, as the targeted macromolecules are now free from the constraint of crystal packing, connecting cryo-EM structures with functional states is straightforward and legitimate. A vivid example provided by cryo-EM is the structures of ATP synthases (Guo et al., 2017; Murphy et al., 2019) where multiple structural intermediates corresponding to the snapshots of the machine in action were captured.
Now, as all possible sampled states in solution or native conditions are all recorded by cryo-EM imaging, the image data are complex in nature. The data consists of an ensemble of different structures. Making a single 3D reconstruction of a macromolecule using all its cryo-EM images that represent the ensemble of co-existing structures would obviously yield a blurred structure of the macromolecule. To disentangle the co-existing structures, Scheres, Frank, and Carazo made a pioneering effort (Scheres et al., 2007) in modeling the data as a mixture of a number of discrete structures using a likelihood approach (Sigworth et al., 2010; Grant et al., 2018). This likelihood-based 3D classification approach later evolved to a Bayesian approach (Scheres, 2012). Those disentangling algorithms immediately contributed to the breakthrough in the structure determination of spliceosome particles (Yan et al., 2015) (see the milestones in Figure 2B), which have poised an impasse for crystallography as the dynamical remodeling has resulted in overwhelming heterogeneity.
Earlier applications of single cryo-EM structural determination of proteins tended to employ this tool as a “crystal-free” PDB structure generator. Researchers would check out a dominant structure from the image data with the resolution better than 3.5 Å to suit de novo model building while ignoring other structures co-existing in the data that were less prominent. Recently, there has been increased interest in extracting as many possible structures from one cryo-EM study, particularly for elucidating the action of COVID-19 spike protein (Yang et al., 2021). This trend has attested the demand on the potential of cryo-EM in uncovering structural polymorphism. In this review, we focus on representative cases on well-folded proteins with high significance to illustrate this development. We exclude the discussion on IDPs (Kulkarni et al., 2018) in this review since a disordered structure would not emerge through the image averaging process in cryo-EM analyses. In the end, we highlight the progress in 3D classification algorithms that resolve the structural polymorphism registered in the data, and discuss the possibility of visualizing RNA structural polymorphism by cryo-EM where the challenges are much greater than that of protein.
Sub-100 kDa proteins
Since the high-resolution cryo-EM imaging is built upon phase contrast, visualizing small proteins is challenging. Considering proteins as small as adenylate kinase (AK), it could not generate sufficient signals under cryo-EM unless the targeted protein organizes into an oligomer. Currently, the smallest particles attainable by cryo-EM with near atomic resolution are represented by streptavidin (52 kDa) (Fan et al., 2019; Han et al., 2020) and haemoglobin (64 kDa) (Khoshouei et al., 2017; Herzik et al., 2019), both of which are in multimeric form.
Haemoglobin (Hgb) mediates oxygen transport in blood with four proteins organized as a dimer of αβ dimer in C2 symmetry; it is the first protein structure together with myoglobin solved using X-ray crystallography by the “isomorphous replacement” phasing technique,” invented by Max Perutz (Perutz et al., 1968). Almost 60 years later, the first cryo-EM image of haemoglobin (Hgb) was obtained by Danev and Baumeister (Khoshouei et al., 2017) (Figure 3A, adopted from Figure 1A in Khoshouei et al., 2017) with the usage of phase plate, which enables cryo-EM visualization of proteins smaller than 100 kDa (Chang et al., 2010; Wu et al., 2013). In the cryo-EM map of Hgb at 3.2 Å resolution, side-chain densities and prosthetic heme groups of Hgb are clearly resolved with C2 symmetry imposed (Khoshouei et al., 2017). The derived atomic model was compared with three conformers of ferrous (Fe2+) Hgb, tight (T), relaxed 1 (R1) and relaxed 2 (R2), obtained from the crystal structures by Shibayama et al. from Tame group in 2014 (Shibayama et al., 2014). Using rigid-body fitting to dock the α1 subunits, the authors found cross-correlation values of 43, 47 and 62% for T, R1 and R2 states, respectively (Khoshouei et al., 2017) (Figure 3B, adopted from Figure 1E in Khoshouei et al., 2017). This observation is consistent with that met-haemoglobin (metHgb), namely ferric (Fe3+) Hgb, can adopt an R-like state. It should be noted that this work did not invoke 3D classification analyses to disentangle the conformational sub-states that may co-exist.
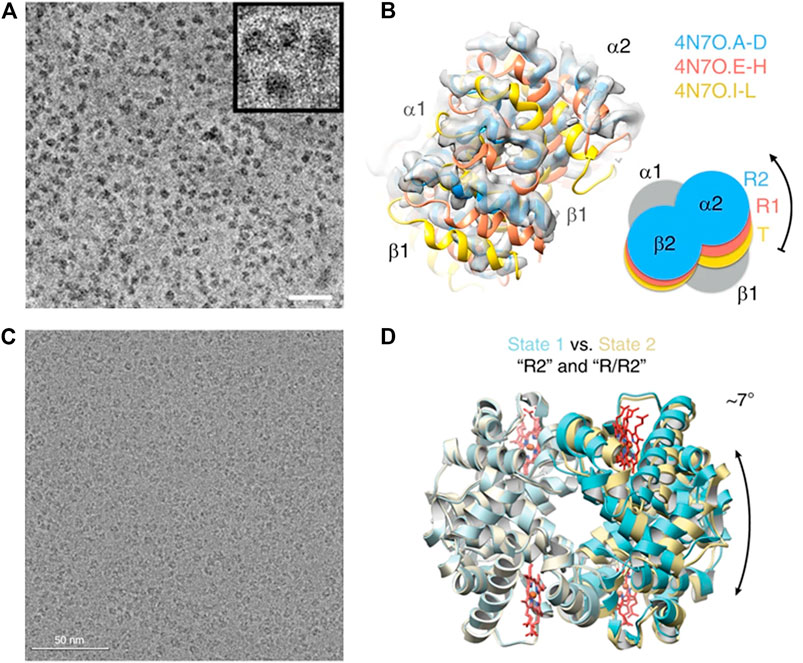
FIGURE 3. Single particle cryo-EM reveals co-exiting conformations of haemoglobin. (A) Visualization of haemoglobin with the aid of Volta phase plate (see the milestones in Figure 2B). (This figure is adopted from Figure 1A of Ref 41, an open access article distributed under the terms of the Creative Commons CC BY license. https://creativecommons.org/) (B) Modeling the cryo-EM map with known X-ray structures suggests the co-existing conformations. (This figure is adopted from Figure 1E of Ref 41, an open access article distributed under the terms of the Creative Commons CC BY license. https://creativecommons.org/). (C) Visualization of haemoglobin in thin ice without phase plate. This figure is adopted from Figure 1A of Ref 42. (D) Resolving the cryo-EM map with the usage of K-way 3D classification on RELION indicates the co-existing conformations. (This figure is adopted from Figure 1E of Ref 42, an open access article distributed under the terms of the Creative Commons CC BY license. https://creativecommons.org/).
A subsequent cryo-EM study on haemoglobin was performed by Lander and co-workers (Herzik et al., 2019) with the intention of demonstrating the imaging capability of conventional defocusing cryo-EM using optimized samples (Figure 3C, adopted from Figure 2A in Herzik et al., 2019). Remarkably, through extensive parallel 3D classification analyses on the conventional cryo-EM images of Hgb, two distinct conformational states of metHgb were revealed (Figure 3D, adopted from Figure 3C in Herzik et al., 2019). State 1 attaining 2.8 Å resolution closely resembles the R2 state described by the crystal structure of Shibayama et al. (PDB: 4N7P) (CαRMSD 0.4 Å) (Shibayama et al., 2014), and state 2 with 3.2 Å resolution matches well with the “between R1 and R2” state (PDB: 4N7N) (CαRMSD 0.5 Å) (Shibayama et al., 2014). Comparing the two states by superposition of a αβ dimer from each molecule indicated a less than 10° rigid-body rotation of one αβ dimer relative to the other with the rotation axis centered about the dimer–dimer interface. Those observations were consistent with those movements observed in the crystal structures (Shibayama et al., 2014). The latter cryo-EM study of haemoglobin with thorough classification analyses clearly demonstrates that high-resolution single-particle cryo-EM is applicable to resolve distinct, biologically relevant conformational states of a sub-100 kDa complex.
Ion Channels
A variety of important cellular activities occur at the cell membranes including ion transport, signal transduction, and bioenergetics through the working of membrane proteins. Membrane proteins are notorious for crystallization. As a result, membrane protein crystal structures only comprise a small fraction in the PDB data bank. Ion channel, in particular, is a hard subject among membrane proteins as it cannot be easily prepared in large quantity because unwary over-expression would kill the host cells. Remarkably, as soon as the resolution of single particle cryo-EM was advanced with an electron counting camera (Li et al., 2013), Julius and Cheng immediately applied it to obtain a near atomic resolution structure of the ion channel of transient receptor potential (TRP) channel (Liao et al., 2013) with the protein stabilized by spider toxin and solubilized with detergent-like polymers.
Yet, membrane proteins are naturally embedded in lipid bilayers. To access the function and structure of membrane proteins in lipid bilayer, nanodisc was introduced by Sligar (Denisov and Sligar, 2016) (see Figure 4A). When a membrane protein is hosted in lipid bilayer encased by a scaffold protein that constitutes the nanodisc, it would allow the membrane protein in close-to-native environment to be characterized by NMR (Nasr et al., 2017) or visualized by cryo-EM (Flores et al., 2020) (Figure 4B, adopted from Figure 1 in Flores et al., 2020). Remarkably, as the resolution of cryo-EM imaging passes 2 Å, the protein-lipid interactions become visible (Flores et al., 2020) (Figure 4C, adopted from Figure 3 in Flores et al., 2020), demonstrating the possibility of seeing how lipids would participate in modulating the structure-function relationship of a membrane protein.
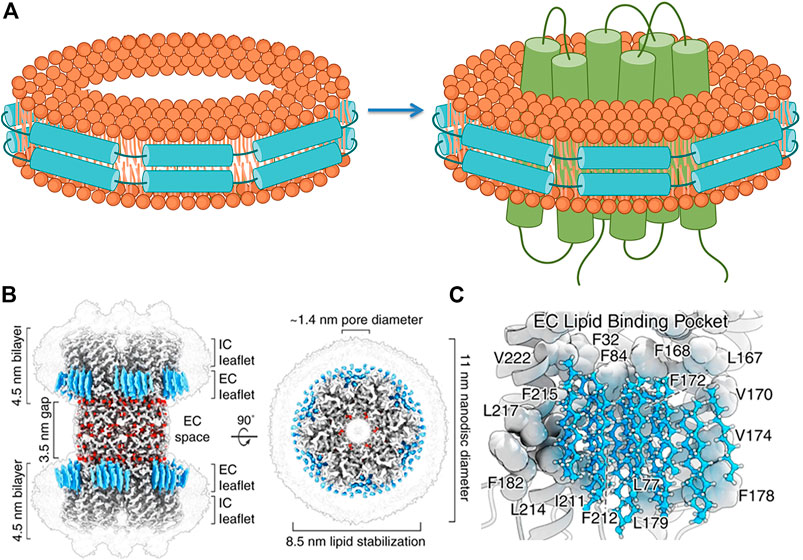
FIGURE 4. Nanodisc system facilitates cryo-EM analyses of membrane proteins in native environment. (A) Reconstitution of a nanodisc-membrane protein complex: lipids enclosed by membrane scaffold proteins (MSP) to form a nanodisc are assembled with a membrane protein to form a nanodisc-membrane protein complex. (This figure is made by modifying nanodisc cartoons available from BioRender with permission under a purchased license.) (B) Cryo-EM structure of a connexin protein in nanodisc. (C) Revelation of lipid-protein interactions (B and C) are adopted from Figures 1, 3 in Denisov and Sligar (2016) with permission from Creative Commons license).
Among ion channels, TRP channels are peculiar as some families of these ion channels respond to diverse stimuli to allow passage of either small or large cations; this puzzle of functional plasticity has drawn great attention. Of particular interest is the presumable conformation dynamics associated with the ion selectivity filter regulated by physiological reagents. Over years, it has been speculated that there would be structural intermediates behind such dynamic channel regulation. But can they be captured? Considering TRPV1, time trajectories from channel recording suggested this channel would alter its ion selectivity in response to binding of a plethora of small molecules of algogenic agents. To investigate the corresponding structures, Cheng and Julius reconstituted TRPV1 into nanodisc to perform cryo-EM imaging on the channel protein triggered with various agents including protons, vanilloid agonists, and peptide toxins (Zhang et al., 2021). As opposed to the previous study of TRPV1 (Liao et al., 2013) that selectively stabilized a conformation using a spider double-knot toxin (DkTx) together with a vanilloid agonist resiniferatoxin (RTX), this study sought to assay the possible intermediate states of TRPV1 bound with DkTx alone, of which the mode of action is puzzling as it targets the outer pore region of TRPV1, but opens the lower gate to evoke membrane currents. Remarkably, the authors identified a collection of sub-states within the same cryo-EM dataset featured by distinct toxin interactions, pore diameters, coordination of ions by amino acids in the selectivity filter. A total of 26 high-resolution 3D maps of TRPV1 were presented in this milestone study (please see Supplementary Figure S1 in Zhang et al., 2021). Those maps represent the sub-states of apo-TRPV1 under standard conditions, TRPV1 bound with a spider toxin with varied stoichiometry, and apo-TRPV1 in various acidic conditions. The massive amount of structure information output by cryo-EM at once not only uncovers new findings to explain the function of TRP channels as polymodal signal integrators, but also demonstrates the efficacy of cryo-EM in rapidly revealing structural polymorphism of native membrane proteins. This capability of cryo-EM in visualizing key structural features of receptor/channel proteins including ligand-receptor interactions, binding site stoichiometry and cooperativity, and the mode of competition, would perhaps trigger a tsunami that impacts molecular physiology and pharmacology.
Protein Machineries
Molecular machines are largely composed of large number of protein subunits, which may generate uncorrelated or correlated movements (Karplus and Kuriyan, 2005). GroEL, an Escherichia coli chaperonin (Hartl and Hayer-Hartl, 2002; Hayer-Hartl et al., 2016), is a molecular chaperone that assists the correct folding of proteins in the cell (Chaudhry et al., 2004); it is composed of 14 chemically identical protomers organized into two stacked seven-membered rings (see Hartl and Hayer-Hartl, 2002). Although the structure of GroEL has been previously characterized by both X-ray crystallography (Chaudhry et al., 2004; Bartolucci et al., 2005) and low-resolution cryo-EM in early days (Ludtke et al., 2004; Ludtke et al., 2008; Clare et al., 2012), those studies had applied D7 symmetry in the analysis of the structure by literally averaging over the protomers with assumption that they have identical conformations. In other words, those studies did not disclose any variations among the protomers in an oligomer.
Even with the resolution breakthrough in single particle cryo-EM, it is not trivial to derive the atomic structures of individual subunits within a molecular machine of homo-oligomer such as GroEL because the task is extremely computationally expensive. The first report regarding the conformation heterogeneity of the promoters of GroEL was thereby not available until 2017. Chiu and coworkers (Roh et al., 2017) first reconstructed apo-GroEL to close to a resolution of about 3.5 Å from approximately 40,000 particle images. At this resolution, 14 individual protein subunits were unambiguously isolated and segmented (Figure 5A, adopted from Figure 1C in Roh et al., 2017). In contrast to X-ray crystallography, cryo-EM structures provide information of local resolutions (Kucukelbir et al., 2014) in the maps in addition to a mean resolution. Local resolution analyses of the GroEL cryo-EM structure revealed that the apical domain was resolved to ∼4 Å and the equatorial domain to 3 Å (Figure 5B, adopted from Supplementary Figure S3 in Roh et al., 2017). This observation implied greater flexibility of the apical domain and suggested that structural variations may well exhibit among the 14 compositionally identical subunits of a GroEL oligomer. Since a naïve 3D reconstruction of GroEL without imposing symmetry would still treat the particle as a whole object, it may not be sensitive to subtle differences within a particle that vary from particle to particle, in particular in the “sea” of signals from an entire GroEL oligomer. Therefore, Chiu and coworkers forged a computationally expensive task of invoking focused 3D classification introduced by Scheres (Bai et al., 2015), and also independently by Huiskonen (Ilca et al., 2015)─in this approach, individual subunit in each particle image was computationally extracted, by which distinct conformations, if any, could be detected and resolved. This analysis allowed for sorting the subunits into a large number of classes based on the conformational similarity─approximately 70% of the subunits were categorized into three major conformation classes (Figure 5C, adopted from Figure 3 in Roh et al., 2017). The primary difference among the three conformers is localized to the apical domain, involved in substrate binding, where its orientation relative to the equatorial domain varies due to the movement of the intermediate domain that connects them. Interestingly, the spatial distributions of each conformation class differed among GroEL oligomers, with most oligomers containing 10–12 subunits in one of the three major conformations. In addition, correlation among subunits seems to exist in a GroEL oligomer as adjacent subunits were found to assume the same conformation. It is noted those three conformations match well with those found in GroEL X-ray structures, leading to the conclusion that the structural polymorphism previously observed in the crystal structures were not a crystallization artifact.
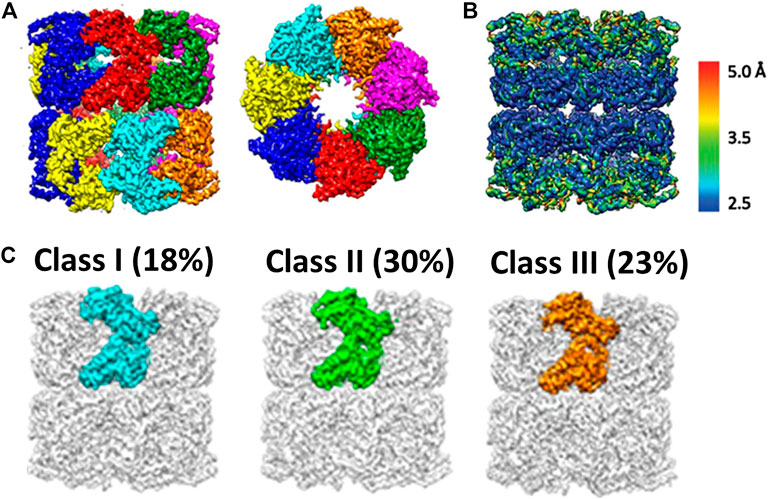
FIGURE 5. Cryo-EM analyses of GroEL, a multi-subunit protein machinery. (A) Segmentation of a high-resolution cryo-EM reconstruction. This allows extraction of individual subunit for further analysis. (B) Local Resolution map of GroEl. It shows the apical domain appears to be more dynamic as compared to equatorial domain. (C) Focused 3D classification on individual subunit. It reveals three major conformers (This set of figures is adopted from Roh et al (2017) with permission granted by NAS).
When chaperons are in action, they capture non-native polypeptides in the central cavity and rectify their folding with the fuel of ATP (Zhao et al., 2021). Kinetic measurements indicated ATP hydrolysis by such oligomeric complex exhibited both positive and negative cooperativity (Yifrach and Horovitz, 2000; Kafri et al., 2001; Kusmierczyk and Martin, 2003). The question is raised as to what would be the structure basis underlying the cooperation of subunits in ligand binding and/or catalysis. In the case of group I chaperons to which GroEL belongs, intra-ring conformational changes are thought to follow a concerted, Monod–Wyman–Changeux (MWC) model (Yifrach and Horovitz, 1995; Dyachenko et al., 2013; Horovitz, 2013). In this model, each ring is in equilibrium between two states that can interchange in an orchestrated manner. This is because there are steric repulsions between subunits in GroEL that conformation changes in individual subunits are dis-allowed except when the concerted intra-ring transition occurs (Ma and Karplus, 1998; Ma et al., 2000). In contrast, in group II chaperonins, as the open conformation exhibits little contacts between the intermediate or apical domains of neighboring subunits, there is no hindrance to prohibit independent conformation changes in individual subunits (Huo et al., 2010; Zhang et al., 2010; Yébenes et al., 2011; Zhang, 2011). This means that the cooperativity in MmCpn, an archaeal chaperonin complex that belongs to group II may follow a different intra-ring allosteric mechanism.
To test whether nucleotide binding to one subunit increases the probability of binding to an adjacent subunit more than remote subunits in the same particle of MmCpn, Zhao et al. (Zhao et al., 2021) used single particle cryo-EM with the focused 3D classification analysis to assess the number and the distribution of nucleotides bound to each subunit in this homo-oligomeric complex. As shown in Figure 3 in Zhao et al., 2021, eight major classes representing distinct sub-conformations are obtained with the distal tip of the apical domain moving inwards ∼35 Å between the most open conformation (class 1) and the most closed conformation (class 8). To assess the nucleotide occupancy in the 8 sub-conformations, difference mapping was performed. Interestingly, nucleotide density is present in the four more-closed conformations (class 5–8), but is absent in the four more-open conformations (class 1–4), consistent with the notion that the nucleotide binding and/or hydrolysis can cause the conformation of subunit to switch from the open to the closed form (Sekiguchi et al., 2013). Next, Zhao et al. further extended the analysis on individual ring of each particle so as to label each of the 8 subunits as apo- or ATP-bound according to the 3D class they belonged to. This way the experimental observations of the relative frequency distribution of nucleotide occupancy at a subunit level within a ring were obtained, and then compared to those based on that predicted from random binding (please see Figure 4B in Zhao et al., 2021). The remarkable quantitative agreement concluded that ATP binding to subunits in the case of MmCpn is stochastic, which is expected as the conformational change induced by ATP binding of a subunit is unfettered by inter-subunit interactions within a ring.
Temperature-Gated Cryo-Electron Microscope Studies
Those highlighted cases of structural polymorphism either stem from conformational sub-states associated with the protein dynamics or represent an outcome induced by ligand binding. The aforementioned samples used for cryo-EM studies, like nearly all other cryo-EM studies, were equilibrated at 4°C prior to plunger freezing, which is a common practice in cryo-EM community. As raising temperature can increase protein dynamics and 4°C is much far away from the point where most of enzymes are catalytically active, it is likely that the structures obtained from those cryo-EM studies may not closely reflect those of the macromolecules in action. To address this issue Chang et al. (Chang et al., 2021) modified the Vitrobot freezing device to enable the investigation of the structure of ketol-acid reductoisomerase (KARI) found in a hot spring bacteria (Sulfolobus acidocaldarius) that exhibits optimal enzyme activity in the range of 60–70°C (Chen et al., 2018). By clamping the temperature of vitrification at various temperatures ranging from 4 to 70°C, high-resolution structures of the enzyme at different temperatures were obtained (Chen et al., 2019). As shown in Figure 4 in Chen et al., 2019, the enzyme conformations remain largely unchanged in the range of 4–55°C. Strikingly, a major change was observed as the temperature was shifted to 70°C. The temperature-resolved structures seem to correlate well with the temperature-dependent activities. This study offers opportunities for dissecting the induced-fit mechanism by separating the contribution of ligand-induced from that of temperature-induced. Importantly, it demonstrates that cryo-EM can be used to obtain protein structures at functional temperatures. Along this line, a recent study of TRPV1 at elevated temperature has revealed the snapshots of heat-dependent opening of TRPV1 in the presence of capsaicin that underlies the mechanism of nociception (Kwon et al., 2021).
Discussion
In this focus review, we choose a few milestone cases of cryo-EM structures including sub-100 kDa proteins, membrane proteins, and protein machineries to illustrate the exciting frontier of using cryo-EM to visualize structural polymorphism of protein complexes. In the past, structural polymorphism was best approached by crystal studies, but the physiological relevance of those structures were often in question due to the potential artifact induced by crystal packing. Beyond removing crystal-associated artifact, cryo-EM opens a chapter for structural studies of biological macromolecules that are refractory to crystallization. In the case of TRPV channels, those ion or ligand induced polymorphism is largely inaccessible by X-ray crystallography as membrane proteins often resist crystallization. As the complexity increases, application of cryo-EM to protein machines of oligomeric subunits, as exemplified by GroEL, other chaperons, and proteasomes, has uncovered the spatial distribution of ligand occupancy to portray individual machine as “single molecule” and provide structure basis for the cooperativity models inferred from bulk biochemistry measurements. This new opportunity is perhaps completely outside the reach of crystallography, because crystallization of protein machine like chaperon would perhaps trap it in a unique configuration permitted by symmetry.
Though the opportunity of structural polymorphism has been permitted by cryo-EM in the beginning, conventional usage of cryo-EM since the resolution revolution mostly employs it as an alternative approach to X-ray crystallography in deriving a PDB model for a protein. In this classical approach, the role of 3D classification analyses is largely aimed to “purify” a subset of high structural homogeneity from the cryo-EM data to produce a density map of best quality or highest resolution. As such, researchers may discard other 3D reconstructions also uncovered from the data that were less prominent. However, there is a shift of focus that is taking place. Considering TRPV channel that has been crowned with Nobel Prize of Physiology or Medicine 2021, since its first cryo-EM structure was determined in 2013 (Shibayama et al., 2014), significant improvements in sample preparation, cryo-EM data collection and analyses have been made. Those advancements have transformed our capability of exploring structural sub-classes of nanodisc-embedded TRPV to shed lights into the mechanism.
So far, virtually all the cited examples were analyzed with the standard K-way 3D classification method using Relion (Scheres, 2012). Although the K-way 3D classification is effective for checking out a consensus image subset that is structurally homogeneous, it is limited to resolving discrete structural heterogeneity. Therefore, there has been continued interest in developing 3D classification methods for analyzing continuous conformations pertinent to the dynamics of the target systems since early days of single particle cryo-EM. When an approach can accommodate continuous motions, it has the potential to capture transient dynamics of the system of interest. This effort is perhaps crucial in establishing the cause and effect relationship between the SARS-Cov2 spike mutants (Yang et al., 2021) and gain- or loss-of-function in its infectious activities.
We list in Table 1 concurrent 3D classification approaches developed for resolving structural heterogeneity and make comments based on our hands-on test experience. They can be roughly categorized into 1) classical dimension reduction or linear sub-space modeling approach (Penczek et al., 2011; Tagare et al., 2015; Andén and Singer, 2018; Chung et al., 2020; Chung et al., 2021; Punjani and Fleet, 2021a), 2) novel manifold embedding technique with non-linear sub-space modeling (Dashti et al., 2020), and 3) artificial intelligence-based approach (Zhong et al., 2021; Chung et al., 2021; Punjani and Fleet, 2021b). The dimension reduction approach is best featured by principal component analysis (PCA eigen-analysis) that was developed earlier on (Penczek et al., 2011). The PCA-based methods also have the capacity of modeling both discrete conformations in addition to continuous conformations. In the case of discrete states, the number of states can be visualized in a plot of the space of eigenvectors (Figure 6 adopted from Figure 2 in Chung et al., 2020), where the eigenvectors often correspond to features providing biological insights. However, earlier algorithms developed for this approach were all heavy in computation as they invoked bootstrap large number of 3D reconstructions from random subsets of images or entailed solving covariance matrix of high dimension where the dimension grows with targeted resolution. Currently, there is active development to reduce the complexity from high dimension (Andén and Singer, 2018) whereas a stage-wise dimension reduction approach (Chung et al., 2020) has been successfully applied to experimental cryo-EM images. There is also novel approach within this framework that circumvents the need of solving covariant matrix (Chung et al., 2021). Due to the limitation by the issue of dimension, those PCA methods would be limited to solving heterogeneity at low resolution when computation resources are modest. It is noted that, 3DVA (Punjani and Fleet, 2021a), a recently released approach, enables fitting high-resolution linear subspace models to single particle cryo-EM data and has been demonstrated effective for resolving continuous flexibility. As 3DVA is implemented on cryoSparc (Punjani et al., 2017), another package as popular as Relion, it has received wide attention in the cryo-EM community to make immediate impact on COVID-19 research (Yang et al., 2021). It is also noted that a PCA approach developed by Stark group with implementation on COW has been available (Haselbach et al., 2017) for facilitating investigation on conformation dynamics (Haselbach et al., 2017) or energy landscape (Haselbach et al., 2018). For the latter case, allosteric regulation of human holo-proteosome was revealed (Haselbach et al., 2018). To reconstruct energy landscape, ManifoldEM (Dashti et al., 2020), developed by Ourmazd for analyzing cryo-EM images and XFEL diffraction images as well, employed manifold embedding and non-linear subspace mapping to position each cryo-EM image of a macromolecule on a complex energy surface. This tool allows the entire work-cycle of a molecular machine to be visualized as it passes through a continuum of states. However, it is challenging to endow the manifolds with biological meaning, while interpreting the structures on the manifold is time-consuming. In addition, to perform ManifoldEM analysis involves many sub-steps where the parameters at each step have to be fine-tuned.
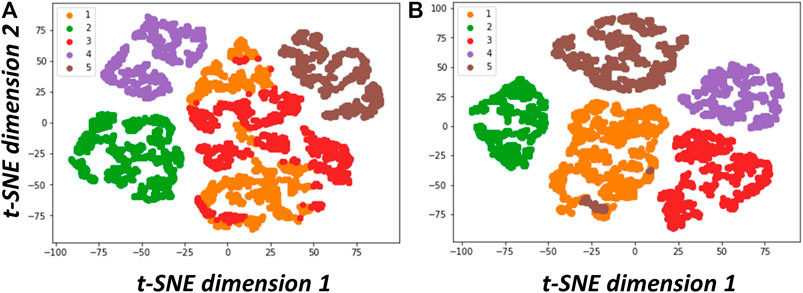
FIGURE 6. Data visualization of structural variability resolved via PCA analyses. (A) t-SNE plot of the results from classical PCA (Penczek et al., 2011). (B) t-SNE plot of the results from two-stage PCA (2SDR) (Chung et al., 2020). As stated in Chung et al., 2020, We followed the procedure in Penczek et al., 2011 to generate a dataset containing 9,453 simulated cryo-EM particle images projected from five 70S ribosome conformations with minor differences resulting from combinations of the absence or presence of tRNA (transfer RNA) and EF-G (elongation factor G). We then resampled these particle images to generate 11,000 3D volumes (density maps) on 75 × 75 × 75 voxels. Next, we solved the eigenvolumes using PCA (Penczek et al., 2011). or 2SDR (Chung et al., 2020). and compared the performance of these two methods using the factorial coordinates defined in Penczek et al., 2011. The t-SNE plots and k-means with 5 classes on their factorial coordinates indicate that the eigenvolumes solved by 2SDR have clearly resolved the structural variability (This set of figures is adopted from Chung et al., 2020 with permission given by International Press.).
Recently, there is a surge of neural network or deep learning based approaches to 3D classification, to name a few, CryoDrgn (Zhong et al., 2021), e2gmm (Chen and Ludtke, 2021), and 3DFlex (Punjani and Fleet, 2021b), where the codes have been released for usage except 3DFlex. CryoDrgn uses deep neural network to directly reconstruct continuous distributions of 3D density maps and map the variability at per-particle level of single-particle cryo-EM datasets. Also using deep leaning architect, e2gmm determines a conformational landscape for proteins using a 3D Gaussian mixture model mapped onto 2D particle images in known orientations; it can automatically resolve the structural heterogeneity within the protein complex and map particles onto a small latent space describing conformational and compositional changes. Compared to CryoDrgn, e2gmm is relatively faster, more intuitive, and easier to use as it is implemented on EMAN2. However, the attainable resolution with e2gmm strongly depends on the available computation resources (see the comment made by the authors in Table 1). The above-mentioned neural network approaches identify structural heterogeneity including conformation dynamics at the level of 3D reconstruction/3D classification. By contrast, DeepMap (Matsumoto et al., 2021), is a newly developed neural method that can work on already obtained 3D cryo-EM map of a static structure to uncover the hidden dynamics. Conventionally, such hidden dynamics has to be acquired experimentally using NMR, hydrogen-deuterium exchange mass-spectroscopy (HDX-MS), or computationally with molecular dynamics simulation on the structure. Remarkably, DeepMap can access to the dynamics of extremely large systems including virus particles and large protein complexes that cannot be solved using those conventional techniques. Of note, the neural network approach is now being actively developed for nearly all steps in cryo-EM image analysis workflow including micrograph de-noising (Tegunov and Cramer, 2019; Bepler et al., 2020; Palovcak et al., 2020) for particle picking, selecting good 2D classes (Li et al., 2020), 3D alignment (Jiménez-Moreno et al., 2021), and even for 3D map masking and sharpening (Sanchez-Garcia et al., 2021). Yet a user must bear in mind that any neural network approach would be time-consuming.
As there is increased interest in resolving structural heterogeneity, the demand on collecting more image data is likely to increase. To reconstruct a high-resolution single cryo-EM structure of low or no symmetry to near atomic resolution usually requires 105 particles of nearly homogeneous structure. This structure usually represents the dominant state that comprises a significant fraction of the image data whereas the remaining other structures corresponding to the less populated states would contain rich information as to structural polymorphism. In order to increase the map quality of those remaining sub-states, the size of dataset has to be enlarged, usually by many folds to an order of magnitude. With automated cryo-EM models such as Titan Krios and cryo-ARM, the current benchmark of throughput of data collection enabled by an imaging scheme of aberration-free image shift (Wu et al., 2019; Efremov and Stroobants, 2021), has reached a figure greater than 5,000 micrographs per day (Wu et al., 2019; Zhang et al., 2020). As a result, it is now possible to obtain sub-million to a million of particles on daily basis to afford the data volume to support fruitful mining on structural polymorphism. The drastic increase of the data volume would further burden the image analysis. Therefore, there will be expectation on developing efficient 3D classification algorithms so as to accelerate the rate of structure discoveries.
In this review, we mainly focus on structural polymorphism on well-behaved proteins and exclude those disease-associated amyloid proteins that are highly polymorphic in structures (Kollmer et al., 2019). Apart from proteins, RNAs are functional biological macromolecules─they fold into tertiary structures to exercise specific biological functions in addition to carrying genetic information coded in the sequence. Structural studies of RNA-only molecules have been extremely challenging with conventional structural methods such as X-ray crystallography and NMR. In the PDB, there are only ∼1,000 RNA-only structures compared to more than 10,000 protein structures since RNAs are refractory to crystallization. This issue perhaps traces its root to the extreme conformational heterogeneity inherent with RNA. A single strand RNA is prone to produce different sets of secondary structures that have comparable free-energy (Low and Weeks, 2010; Wang et al., 2021). Once the secondary structures fold into a tertiary structure, it would readily change conformations.
Despite that cryo-EM has made breakthroughs in high-resolution structural determination of large RNA-protein complexes previously unattainable by X-ray crystallography or NMR, a general view in the community of structural biology is that cryo-EM is ill-suited for most RNA molecules in the absence of protein partners as RNAs were too small or too flexible (Kappel et al., 2020). The developments of cryo-EM structure determination on RNA-only has thus been halted. Now, considering proteins again, in particular those without no or low symmetry, high-resolution cryo-EM determination is limited to 60 kDa, This would set the size of RNA-only molecules feasible with cryo-EM to be roughly 200 nucleotides (Kappel et al., 2020). Unfortunately, many functional noncoding RNAs are of similar size or smaller and thereby excluded from the reach of cryo-EM. Fortunately, as cryo-EM map describes the electrical potential of atoms, the small potential from RNA phosphate backbone allows nucleotide bases to be readily distinguished at moderate resolution (4 Å) (Wilkinson et al., 2018). Despite that at this resolution, purines (A, G) can be discerned from pyrimidines (U, C); only when the resolution is better than 3 Å, can adenosine be further distinguished from guanosine bases (Wilkinson et al., 2018). In other word, the resolution criteria required for modeling RNA from De Novo is more stringent than that for protein (Cheng, 2015).
To explore the potential of cryo-EM on RNA, Chiu and his colleagues initiated cryo-EM studies on RNA-only molecules since 2018. They tested a total of 18 functionally diverse RNAs with the size in the range 65–388 nucleotides (21–126 kDa) including Tetrahymena ribozyme, of which the complete structures were then unknown (Kappel et al., 2020). In order to enhance the visibility of those small RNAs, phase plate imaging technique was employed. The testing results show cryo-EM could resolve global folds of RNA molecules. Since the resolutions of the attained cryo-EM map (>4.7 Å) were not sufficient for De Novo model building, a pipeline (Ribosolve) that performs computer modeling by combining cryo-EM map and sequence data was developed for the structure determination of RNA molecules. Subsequently, Tetrahymena ribozyme in full-length was selected for the pursuit of high-resolution structure determination to reach 3.1 Å (Su et al., 2021). This result perhaps represents the RNA of highest resolution solved by cryo-EM. Concerning the potential of resolving structural polymorphism, there were a number of ribozymes and riboswitches together with human U1 snRNA were not pursued for 3D reconstruction as they could not generate meaningful 2D class averages (see Figure 2 in Kappel et al., 2020). Of particular interest is V. cholera glycine riboswitch. Using the K-way 3D classification, the authors have resolved at least four structurally distinct classes where the dominant group was further pursued to generate a cryo-EM map of 4.8 Å (see Extended Data Figure 3 in Kappel et al., 2020). These observations imply the existence of trackable structural polymorphism that merits further investigation. In conclusion, those recent exciting developments on RNA structures by cryo-EM have heralded new era of RNA structural biology where solving the issue of polymorphism related to its folding or dynamics is at dawn.
Author Contributions
WHC and IPT conceived the subject for this review. WHC wrote the manuscript. HHL and SHH contributed to case studies and figure making. SCC and IPT contributed to the testing on 3D classification algorithms.
Funding
This work was supported by Academia Sinica (AS) and Ministry of Science and Technology of Taiwan (MOST) with grant numbers AS-TP-110-L11, AS-TP-108-ML07, and MOST 109-2113-M-001-032 to WHC, and AS-IA-110-M05 and MOST 109-2118-M-001-006 to IPT.
Conflict of Interest
The authors declare that the research was conducted in the absence of any commercial or financial relationships that could be construed as a potential conflict of interest.
Publisher’s Note
All claims expressed in this article are solely those of the authors and do not necessarily represent those of their affiliated organizations, or those of the publisher, the editors and the reviewers. Any product that may be evaluated in this article, or claim that may be made by its manufacturer, is not guaranteed or endorsed by the publisher.
Acknowledgments
The authors are indebted to Sunney Chan for critical reading of the manuscript. WHC acknowledges Yi-Min Wu, Jen-Wei Chang, Chun-Hsiung Wang, Chihong Song and Kazuyoshi Murata for sharing unpublished data that inspired this review.
References
Andén, J., and Singer, A. (2018). Structural Variability from Noisy Tomographic Projections. SIAM J. Imaging Sci. 11 (2), 1441–1492. doi:10.1137/17M1153509
Anfinsen, C. B., and Haber, E. (1961). Studies on the Reduction and Re-formation of Protein Disulfide Bonds. J. Biol. Chem. 236, 1361–1363. doi:10.1016/S0021-9258(18)64177-8
Austin, R. H., Beeson, K. W., Eisenstein, L., Frauenfelder, H., and Gunsalus, I. C. (1975). Dynamics of Ligand Binding to Myoglobin. Biochemistry 14, 5355–5373. doi:10.1021/bi00695a021
Bai, X. C., Rajendra, E., Yang, G., Shi, Y., and Scheres, S. H. (2015). Sampling the Conformational Space of the Catalytic Subunit of Human γ-secretase. eLife 4, e11182. doi:10.7554/eLife.11182
Bartolucci, C., Lamba, D., Grazulis, S., Manakova, E., and Heumann, H. (2005). Crystal Structure of Wild-type Chaperonin GroEL. J. Mol. Biol. 354, 940–951. doi:10.1016/j.jmb.2005.09.096
Bepler, T., Kelley, K., Noble, A. J., and Berger, B. (2020). Topaz-Denoise: General Deep Denoising Models for cryoEM and cryoET. Nat. Commun. 11, 5208. doi:10.1038/s41467-020-18952-1
Brilot, A. F., Chen, J. Z., Cheng, A., Pan, J., Harrison, S. C., Potter, C. S., et al. (2012). Beam-induced Motion of Vitrified Specimen on Holey Carbon Film. J. Struct. Biol. 177 (3), 630–637. doi:10.1016/j.jsb.2012.02.003
Brünger, A. T., Adams, P. D., Clore, G. M., DeLano, W. L., Gros, P., Grosse-Kunstleve, R. W., et al. (1998). Crystallography & NMR System: A New Software Suite for Macromolecular Structure Determination. Acta Crystallogr. D Biol. Crystallogr. 54, 905–921. doi:10.1107/s0907444998003254
Chang, W. H., Chiu, M. T., Chen, C. Y., Yen, C. F., Lin, Y. C., Weng, Y. P., et al. (2010). Zernike Phase Plate Cryoelectron Microscopy Facilitates Single Particle Analysis of Unstained Asymmetric Protein Complexes. Structure 18 (1), 17–27. doi:10.1016/j.str.2009.12.001
Chang, Y.-C., Chen, C.-Y., and Tsai, M.-D. (2021). Preparation of High-Temperature Sample Grids for Cryo- EM. J. Vis. Exp. 173, e62772. doi:10.3791/62772
Chaudhry, C., Horwich, A. L., Brunger, A. T., and Adams, P. D. (2004). Exploring the Structural Dynamics of the E.Coli Chaperonin GroEL Using Translation-Libration-Screw Crystallographic Refinement of Intermediate States. J. Mol. Biol. 342, 229–245. doi:10.1016/j.jmb.2004.07.015
Chen, C.-Y., Chang, Y.-C., Lin, B.-L., Huang, C.-H., and Tsai, M.-D. (2019). Temperature-resolved Cryo-EM Uncovers Structural Bases of Temperature-dependent Enzyme Functions. J. Am. Chem. Soc. 141 (51), 19983–19987. doi:10.1021/jacs.9b10687
Chen, C.-Y., Ko, T.-P., Lin, K.-F., Lin, B.-L., Huang, C.-H., Chiang, C.-H., et al. (2018). NADH/NADPH Bi-cofactor-utilizing and Thermoactive Ketol-Acid Reductoisomerase from Sulfolobus Acidocaldarius. Scientific Rep. 8, 7176. doi:10.1038/s41598-018-25361-4
Chen, M., and Ludtke, S. (2021). Deep Learning Based Mixed-Dimensional GMM for Characterizing Variability in CryoEM. Nat. Methods 18, 930–936. doi:10.1038/s41592-021-01220-5
Cheng, Y. (2015). Single-particle Cryo-EM at Crystallographic Resolution. Cell 161 (3), 450–457. doi:10.1016/j.cell.2015.03.049
Chung, S.-C., Wang, S.-H., Niu, B.-Y., Huang, S.-Y., Chang, W.-H., and Tu, I-P. (2020). Two-stage Dimension Reduction (2SDR) for Noisy High Dimensional Images and Application to Cryogenic Electron Microscopy. Ann. Math. Sci. Appl. 5 (2), 283–316. doi:10.4310/AMSA.2020.v5.n2.a4
Chung, S.-C., Wu, H.-Y., Chang, W.-H., and Tu, I-P. (2021). Grouping 3D Structure Conformations Using Network Analysis on 2D Cryo-EM Projection Images. Focus Microsc. 2021. http://www.focusonmicroscopy.org/2021/PDF/1145_Chung.pdf.
Clare, D. K., Vasishtan, D., Stagg, S., Quispe, J., Farr, G. W., Topf, M., et al. (2012). ATP-triggered Conformational Changes Delineate Substrate-Binding and -folding Mechanics of the GroEL Chaperonin. Cell 149, 113–123. doi:10.1016/j.cell.2012.02.047
Cramer, P., Bushnell, D. A., Fu, J., Gnatt, A. L., Maier-Davis, B., Thompson, N. E., et al. (2000). Architecture of RNA Polymerase II and Implications for the Transcription Mechanism. Science 288 (5466), 640–649. doi:10.1126/science.288.5466.640
Dashti, A., Mashayekhi, G., Shekhar, M., Hail, D. B., Salah, S., Schwander, P., et al. (2020). Retrieving Functional Pathways of Biomolecules from Single-Particle Snapshots. Nat. Commun 11, 4734. doi:10.1038/s41467-020-18403-x
Denisov, I. G., and Sligar, S. G. (2016). Nanodiscs for Structural and Functional Studies of Membrane Proteins. Nat. Struct. Mol. Biol. 23, 481–486. doi:10.1038/nsmb.3195
Kwon, D. H., Zhang, F., Suo, Y., Bouvette, J., Borgnia, M. J., and Lee, S .-Y. :Heat -dependentopening of TRPV1 in the Presence of Capsaicin .Nat. Struct. Mol. Biol. 28 ,554 ( 2021)doi :doi:10.1038/s41594-021-00616-3
Dubochet, J., Adrian, M., Chang, J. J., Homo, J. C., Lepault, J., McDowall, A. W., et al. (1988). Cryo-electron Microscopy of Vitrified Specimens. Q. Rev. Biophys. 21 (2), 129–228. doi:10.1017/s0033583500004297
Dyachenko, A., Gruber, R., Shimon, L., Horovitz, A., and Sharon, M. (2013). Allosteric Mechanisms Can Be Distinguished Using Structural Mass Spectrometry. Proc. Natl. Acad. Sci. U S A. 110, 7235–7239. doi:10.1073/pnas.1302395110
Efremov, R. G., and Stroobants, A. (2021). Coma-corrected Rapid Single-Particle Cryo-EM Data Collection on the CRYO ARM 300. Acta Crystallographica. Section D, Struct. Biol. 77 (Pt 5), 555–564. doi:10.1107/S2059798321002151
Ekeberg, T., Svenda, M., Abergel, C., Maia, F. R., Seltzer, V., Claverie, J. M., et al. (2015). Three-dimensional Reconstruction of the Giant Mimivirus Particle with an X-ray Free-Electron Laser. Phys. Rev. Lett. 114 (9), 098102. doi:10.1103/PhysRevLett.114.098102
Fan, X., Wang, J., Zhang, X., Yang, Z., Zhang, J. C., Zhao, L., et al. (2019). Single Particle Cryo-EM Reconstruction of 52 kDa Streptavidin at 3.2 Angstrom Resolution. Nat. Commun. 10 (1), 2386. doi:10.1038/s41467-019-10368-w
Flores, J. A., Haddad, B. G., Dolan, K. A., Myers, J. B., Yoshioka, C. C., Copperman, J., et al. (2020). Connexin-46/50 in a Dynamic Lipid Environment Resolved by CryoEM at 1.9 Å. Nat. Commun. 11, 4331. doi:10.1038/s41467-020-18120-5
Formoso, E., Limongelli, V., and Parrinello, M. (2015). Energetics and Structural Characterization of the Large-Scale Functional Motion of Adenylate Kinase. Sci. Rep. 5, 8425. doi:10.1038/srep08425
Frank, J. (2002). Single-particle Imaging of Macromolecules by Cryo-Electron Microscopy. Annu. Rev. Biophys. Biomol. Struct. 31, 303–319. doi:10.1146/annurev.biophys.31.082901.134202
Frauenfelder, H., Sligar, S. G., and Wolynes, P. G. (1991). The Energy Landscapes and Motions of Proteins. Science 254 (5038), 1598–1603. doi:10.1126/science.1749933
Fu, J., Gnatt, A. L., Bushnell, D. A., Jensen, G. J., Thompson, N. E., Burgess, R. R., et al. (1999). Yeast RNA Polymerase II at 5 A Resolution. Cell 98 (6), 799–810. doi:10.1016/s0092-8674(00)81514-7
Gnatt, A. L., Cramer, P., Fu, J., Bushnell, D. A., and Kornberg, R. D. (2001). Structural Basis of Transcription: an RNA Polymerase II Elongation Complex at 3.3 A Resolution. Science 292 (5523), 1876–1882. doi:10.1126/science.1059495
Gong, P., and Peersen, O. B. (2010). Structural Basis for Active Site Closure by the Poliovirus RNA-dependent RNA Polymerase. Proc. Natl. Acad. Sci. U S A. 107 (52), 22505–22510. doi:10.1073/pnas.1007626107
Grant, T., Rohou, A., and Grigorieff, N. (2018). cisTEM, User-Friendly Software for Single-Particle Image Processing. eLife 7, e35383. doi:10.7554/eLife.35383
Guo, H., Bueler, S. A., and Rubinstein, J. L. (2017). Atomic Model for the Dimeric FO Region of Mitochondrial ATP Synthase. Science 358 (6365), 936–940. doi:10.1126/science.aao4815
Han, Y., Fan, X., Wang, H., Zhao, F., Tully, C. G., Kong, J., et al. (2020). High-yield Monolayer Graphene Grids for Near-Atomic Resolution Cryoelectron Microscopy. Proc. Natl. Acad. Sci. U S A. 117 (2), 1009–1014. doi:10.1073/pnas.1919114117
Hartl, F. U., and Hayer-Hartl, M. (2002). Molecular Chaperones in the Cytosol: from Nascent Chain to Folded Protein. Science 295, 1852–1858. doi:10.1126/science.1068408
Haselbach, D., Komarov, I., Agafonov, D. E., Hartmuth, K., Graf, B., Dybkov, O., et al. (2018). Structure and Conformational Dynamics of Human Spliceosome Bact Complex. Cell 172, 454–464. doi:10.1016/j.cell.2018.01.010
Haselbach, D., Schrader, J., Lambrecht, F., Henneberg, F., Chari, A., Stark, H., et al. (2017). Long-range Allosteric Regulation of the Human 26S Proteasome by 20S Proteasome-Targeting Cancer Drugs. Nat. Commun. 8, 15578. doi:10.1038/ncomms15578
Hayer-Hartl, M., Bracher, A., and Hartl, F. U. (2016). The GroEL-GroES Chaperonin Machine: A Nano-Cage for Protein Folding. Trends Biochem. Sci. 41, 62–76. doi:10.1016/j.tibs.2015.07.009
Henderson, R. (1995). The Potential and Limitations of Neutrons, Electrons and X-Rays for Atomic Resolution Microscopy of Unstained Biological Molecules. Q. Rev. Biophys. 28 (2), 171–193. doi:10.1017/s003358350000305x
Herzik, M. A., Wu, M., and Lander, G. C. (2019). High-resolution Structure Determination of sub-100 kDa Complexes Using Conventional Cryo-EM. Nat. Commun. 10, 1032. doi:10.1038/s41467-019-08991-8
Horovitz, A. (2013). Putting Handcuffs on the Chaperonin GroEL. Proc. Natl. Acad. Sci. U S A. 110, 10884–10885. doi:10.1073/pnas.1309581110
Huo, Y., Hu, Z., Zhang, K., Wang, L., Zhai, Y., Zhou, Q., et al. (2010). Crystal Structure of Group II Chaperonin in the Open State. Structure 18, 1270–1279. doi:10.1016/j.str.2010.07.009
Ilca, S. L., Kotecha, A., Sun, X., Poranen, M. M., Stuart, D. I., and Huiskonen, J. T. (2015). Localized Reconstruction of Subunits from Electron Cryomicroscopy Images of Macromolecular Complexes. Nat. Commun. 6, 8843. doi:10.1038/ncomms9843
Jiménez-Moreno, A., Střelák, D., Filipovič, J., Carazo, J. M., and Sorzano, C. O. S. (2021). DeepAlign, a 3D Alignment Method Based on Regionalized Deep Learning for Cryo-EM. J. Struct. Biol. 213 (2), 107712. doi:10.1016/j.jsb.2021.107712
Kafri, G., Willison, K. R., and Horovitz, A. (2001). Nested Allosteric Interactions in the Cytoplasmic Chaperonin Containing TCP-1. Protein Sci. 10, 445–449. doi:10.1110/ps.44401
Kappel, K., Zhang, K., Su, Z., Watkins, A. M., Kladwang, W., Li, S., et al. (2020). Accelerated Cryo-EM-Guided Determination of Three-Dimensional RNA-Only Structures. Nat. Methods 17, 699–707. doi:10.1038/s41592-020-0878-9
Karplus, M., and Kuriyan, J. (2005). Molecular Dynamics and Protein Function. Proc. Natl. Acad. Sci. U S A. 102, 6679–6685. doi:10.1073/pnas.0408930102
Khoshouei, M., Radjainia, M., Baumeister, W., and Danev, R. (2017). Cryo-EM Structure of Haemoglobin at 3.2 Å Determined with the Volta Phase Plate. Nat. Commun. 8, 16099. doi:10.1038/ncomms.16099
Kollmer, K. M., Close, W., Funk, L., Rasmussen, J., Bsoul, A., Schierhorn, A., et al. (2019). Cryo-EM Structure and Polymorphism of Aβ Amyloid Fibrils Purified from Alzheimer’s Brain Tissue. Nat. Commun 10, 4760. doi:10.1038/s41467-019-12683-8
Koshland, D. E. (1958). Application of a Theory of Enzyme Specificity to Protein Synthesis. Proc. Natl. Acad. Sci. U S A. 44 (2), 98–104. doi:10.1073/pnas.44.2.98
Kucukelbir, A., Sigworth, F. J., and Tagare, H. D. (2014). Quantifying the Local Resolution of Cryo-EM Density Maps. Nat. Methods 11 (1), 63–65. doi:10.1038/nmeth.2727
Kühlbrandt, W. (2014). The Resolution Revolution. Science 343 (6178), 1443–1444. doi:10.1126/science.1251652
Kulkarni, P., Solomon, T. L., He, Y., Chen, Y., Bryan, P. N., and Orban, J. J. (2018). Structural Metamorphism and Polymorphism in Proteins on the Brink of Thermodynamic Stability. Protein Sci. 27 (9), 1557–1567. doi:10.1002/pro.3458
Kusmierczyk, A. R., and Martin, J. (2003). Nested Cooperativity and Salt Dependence of the ATPase Activity of the Archaeal Chaperonin Mm-Cpn. FEBS Lett. 547, 201–204. doi:10.1016/s0014-5793(03)00722-1
Levy, H. M., Sharon, N., and Koshland, D. E. (1959). Purified Muscle Proteins and the Walking Rate of Ants. Proc. Natl. Acad. Sci. U S A. 45 (6), 785–791. doi:10.1073/pnas.45.6.785
Li, X., Mooney, P., Zheng, S., Booth, C. R., Braunfeld, M. B., Gubbens, S., et al. (2013). Electron Counting and Beam-Induced Motion Correction Enable Near-Atomic-Resolution Single-Particle Cryo-EM. Nat. Methods 10, 584–590. doi:10.1038/nmeth.2472
Li, Y., Cash, J. N., Tesmer, J. J. G., and Cianfrocco, M. A. (2020). High-throughput Cryo-EM Enabled by User-free Preprocessing Routines. Structure 28 (7), 858e3–869. doi:10.1016/j.str.2020.03.008
Liao, M., Cao, E., Julius, D., and Cheng, Y. (2013). Structure of the TRPV1 Ion Channel Determined by Electron Cryo-Microscopy. Nature 504, 107–112. doi:10.1038/nature12822
Low, J. T., and Weeks, K. M. (2010). SHAPE-directed RNA Secondary Structure Prediction. Methods 52 (2), 150–158. doi:10.1016/j.ymeth.2010.06.007
Ludtke, S. J., Baker, M. L., Chen, D. H., Song, J. L., Chuang, D. T., and Chiu, W. (2008). De Novo backbone Trace of GroEL from Single Particle Electron Cryomicroscopy. Structure 16, 441–448. doi:10.1016/j.str.2008.02.007
Ludtke, S. J., Chen, D. H., Song, J. L., Chuang, D. T., and Chiu, W. (2004). Seeing GroEL at 6 A Resolution by Single Particle Electron Cryomicroscopy. Structure 12, 1129–1136. doi:10.1016/j.str.2004.05.006
Ma, J., and Karplus, M. (1998). The Allosteric Mechanism of the Chaperonin GroEL: a Dynamic Analysis. Proc. Natl. Acad. Sci. U S A. 95, 8502–8507. doi:10.1073/pnas.95.15.8502
Ma, J., Sigler, P. B., Xu, Z., and Karplus, M. (2000). A Dynamic Model for the Allosteric Mechanism of GroEL. J. Mol. Biol. 302, 303–313. doi:10.1006/jmbi.2000.4014
Matsumoto, S., Ishida, S., Araki, M., Kato, T., Terayama, K., Okuno, Y., et al. (2021). Extraction of Protein Dynamics Information from Cryo-EM Maps Using Deep Learning. Nat. Mach Intell. 3, 153–160. doi:10.1038/s42256-020-00290-y
McMullan, G., Faruqi, A. R., Henderson, R., Guerrini, N., Turchetta, R., Jacobs, A., et al. (2009). Experimental Observation of the Improvement in MTF from Backthinning a CMOS Direct Electron Detector. Ultramicroscopy 109 (9), 1144–1147. doi:10.1016/j.ultramic.2009.05.005
Moustafa, I. M., Korboukh, V. K., Arnold, J. J., Smidansky, E. D., Marcotte, L. L., Gohara, D. W., et al. (2014). Structural Dynamics as a Contributor to Error-Prone Replication by an RNA-dependent RNA Polymerase. J. Biol. Chem. 289 (52), 36229–36248. doi:10.1074/jbc.M114.616193
Murphy, B. J., Klusch, N., Langer, J., Mills, D. J., Yildiz, Ö., and Kühlbrandt, W. (2019). Rotary Substates of Mitochondrial ATP Synthase Reveal the Basis of Flexible F1-Fo Coupling. Science 364 (6446), eaaw9128. doi:10.1126/science.aaw9128
Nasr, M. L., Baptista, D., Strauss, M., Sun, Z. J., Grigoriu, S., Huser, S., et al. (2017). Covalently Circularized Nanodiscs for Studying Membrane Proteins and Viral Entry. Nat. Methods 14, 49–52. doi:10.1038/nmeth.4079
Nogales, E., and Scheres, S. H. (2015). Cryo-EM: A Unique Tool for the Visualization of Macromolecular Complexity. Mol. Cel 58 (4), 677–689. doi:10.1016/j.molcel.2015.02.019
Palovcak, E., Asarnow, D., Campbell, M. G., Yua, Z., and Cheng, Y. (2020). IUCrJ 7 (6), 1142–1150. doi:10.1107/S2052252520013184
Penczek, P. A., Kimmel, M., and Spahn, C. M. (2011). Identifying Conformational States of Macromolecules by Eigen-Analysis of Resampled Cryo-EM Images. Structure 19, 1582–1590. doi:10.1016/j.str.2011.10.003
Perutz, M. F., Muirhead, H., Cox, J. M., and Goaman, L. C. (1968). Three-dimensional Fourier Synthesis of Horse Oxyhaemoglobin at 2.8 A Resolution: the Atomic Model. Nature 219, 131–139. doi:10.1038/219131a0
Punjani, A., and Fleet, D. J. (2021). 3D Flexible Refinement: Structure and Motion of Flexible Proteins from Cryo-EM. BioRxiv. New york: CSHL (Cold Spring Harbor Laboratory). doi:10.1101/2021.04.22.440893
Punjani, A., and Fleet, D. J. (2021). 3D Variability Analysis: Directly Resolving Continuous Flexibility and Discrete Heterogeneity from Single Particle Cryo-EM. J. Struct. Biol. 213 (2), 107702. doi:10.1016/j.jsb.2021.107702
Punjani, A., L Rubinstein, J., J Fleet, D., and Brubaker, M. A. (2017). cryoSPARC: Algorithms for Rapid Unsupervised Cryo-EM Structure Determination. Nat. Methods 14, 290–296. doi:10.1038/nmeth.4169
Roh, S. H., Hryc, C. F., Jeong, H. H., Fei, X., Jakana, J., Lorimer, G. H., et al. (2017). Subunit Conformational Variation within Individual GroEL Oligomers Resolved by Cryo-EM. Proc. Natl. Acad. Sci. U S A. 114 (31), 8259–8264. doi:10.1073/pnas.1704725114
Roy, R., Hohng, S., and Ha, T. (2008). A Practical Guide to Single-Molecule FRET. Nat. Methods 5 (6), 507–516. doi:10.1038/nmeth.1208
Sanchez-Garcia, R., Gomez-Blanco, J., Cuervo, A., Carazo, J. M., Sorzano, C. O. S., Vargas, J., et al. (2021). DeepEMhancer: a Deep Learning Solution for Cryo-EM Volume post-processing. Commun. Biol. 4, 874. doi:10.1038/s42003-021-02399-1
Scheres, S. H., Gao, H., Valle, M., Herman, G. T., Eggermont, P. P., Frank, J., et al. (2007). Disentangling Conformational States of Macromolecules in 3D-EM through Likelihood Optimization. Nat. Methods 4 (1), 27–29. doi:10.1038/nmeth992
Scheres, S. H. (2012). RELION: Implementation of a Bayesian Approach to Cryo-EM Structure Determination. J. Struct. Biol. 180 (3), 519–530. doi:10.1016/j.jsb.2012.09.006
Schlichting, I., Almo, S. C., Rapp, G., Wilson, K., Petratos, K., Lentfer, A., et al. (1990). Time-resolved X-ray Crystallographic Study of the Conformational Change in Ha-Ras P21 Protein on GTP Hydrolysis. Nature 345, 309–315. doi:10.1038/345309a0
Schulz, G. E., Müller, C. W., and Diederichs, K. (1990). Induced-fit Movements in Adenylate Kinases. J. Mol. Biol. 213, 627–630. doi:10.1016/S0022-2836(05)80250-5
Sekiguchi, H., Nakagawa, A., Moriya, K., Makabe, K., Ichiyanagi, K., Nozawa, S., et al. (2013). ATP Dependent Rotational Motion of Group II Chaperonin Observed by X-ray Single Molecule Tracking. PLoS ONE 8, e64176. doi:10.1371/journal.pone.0064176
Shibayama, N., Sugiyama, K., Tame, J. R., and Park, S. Y. (2014). Capturing the Hemoglobin Allosteric Transition in a Single crystal Form. J. Am. Chem. Soc. 136, 5097–5105. doi:10.1021/ja500380e
Sigworth, F. J., Doerschuk, P. C., Carazo, J. M., and Scheres, S. H. (2010). An Introduction to Maximum-Likelihood Methods in Cryo-EM. Methods Enzymol. 482, 263–294. doi:10.1016/S0076-6879(10)82011-7
Su, Z., Zhang, K., Kappel, K., Li, S., Palo, M. Z., Pintilie, G. D., et al. (2021). Cryo-EM Structures of Full-Length Tetrahymena Ribozyme at 3.1 Å Resolution. Nature 596, 603–607. doi:10.1038/s41586-021-03803-w
Tagare, H. D., Kucukelbir, A., Sigworth, F. J., Wang, H., and Rao, M. (2015). Directly Reconstructing Principal Components of Heterogeneous Particles from Cryo-EM Images. J. Struct. Biol. 191 (2), 245–262. doi:10.1016/j.jsb.2015.05.007
Tan, Y. W., Hanson, J. A., and Yang, H. (2009). Direct Mg(2+) Binding Activates Adenylate Kinase from Escherichia coli. J. Biol. Chem. 284, 3306–3313. doi:10.1074/jbc.M803658200
Tan, Y. Z., Cheng, A., Potter, C. S., and Carragher, B. (2016). Automated Data Collection in Single Particle Electron Microscopy. Microscopy (Oxf) 65 (1), 43–56. doi:10.1093/jmicro/dfv369
Tegunov, D., and Cramer, P. (2019). Real-time Cryo-Electron Microscopy Data Preprocessing with Warp. Nat. Methods 16 (11), 1146–1152. doi:10.1038/s41592-019-0580-y
Vonrhein, C., Schlauderer, G. J., and Schulz, G. E. (1995). Movie of the Structural Changes during a Catalytic Cycle of Nucleoside Monophosphate Kinases. Structure 3, 483–490. doi:10.1016/s0969-2126(01)00181-2
Wang, X., Alnabati, E., Aderinwale, T. W., Subramaniya, S. R. M. V., Terashi, G., and Kihara, D. (2021). Detecting Protein and DNA/RNA Structures in Cryo-EM Maps of Intermediate Resolution Using Deep Learning. Nat. Commun 12, 2302. doi:10.1038/s41467-021-22577-3
Wilkinson, M. E., Lin, P.-C., Plaschka, C., and Nagai, K. (2018). Cryo-EM Studies of Pre-mRNA Splicing: from Sample Preparation to Model Visualization. Annu. Rev. Biophys. 47, 175–199. doi:10.1146/annurev-biophys070317-03341010.1146/annurev-biophys-070317-033410
Wu, C., Huang, X., Cheng, X, J., Zhu, D., and Zhang, X. (2019). High-quality, High-Throughput Cryo-Electron Microscopy Data Collection via Beam Tilt and Astigmatism-free Beam-Image Shift. J. Struct. Biol. 208 (3), 107396. doi:10.1016/j.jsb.2019.09.013
Wu, Y.-M., Wang, C.-H., Chang, J.-W., Chen, Y.-Y., Miyazaki, N., Murata, K., et al. (2013). Zernike Phase Contrast Cryo-Electron Microscopy Reveals 100 kDa Component in a Protein Complex. J. Phys. D: Appl. Phys. 46, 494008. doi:10.1088/0022-3727/46/49/494008
Xuong, N. H., Jin, L., Kleinfelder, S., Li, S., Leblanc, P., Duttweiler, F., et al. (2007). Future Directions for Camera Systems in Electron Microscopy. Methods Cel Biol 79, 721–739. doi:10.1016/S0091-679X(06)79028-8
Yan, C., Hang, J., Wan, R., Huang, M., Wong, C. C., and Shi, Y. (2015). Structure of a Yeast Spliceosome at 3.6-angstrom Resolution. Science 349 (6253), 1182–1191. doi:10.1126/science.aac7629
Yang, T.-J., Yu, P.-Y., Chang, Y.-C., Chang, N.-E., Tsai, Y.-X., Liang, K.-H., et al. (2021). Structure-activity Relationships of B.1.617 and Other SARS-CoV-2 Spike Variants. BioRxiv. New york: CSHL (Cold Spring Harbor Laboratory). doi:10.1101/2021.09.12.459978
Yébenes, H., Mesa, P., Muñoz, I. G., Montoya, G., and Valpuesta, J. M. (2011). Chaperonins: Two Rings for Folding. Trends Biochem. Sci. 36, 424–432. doi:10.1016/j.tibs.2011.05.003
Yifrach, O., and Horovitz, A. (2000). Coupling between Protein Folding and Allostery in the GroE Chaperonin System. Proc. Natl. Acad. Sci. U S A. 97, 1521–1524. doi:10.1073/pnas.040449997
Yifrach, O., and Horovitz, A. (1995). Nested Cooperativity in the ATPase Activity of the Oligomeric Chaperonin GroEL. Biochemistry 34, 5303–5308. doi:10.1021/bi00016a001
Zhang, J., Baker, M. L., Schröder, G. F., Douglas, N. R., Reissmann, S., Jakana, J., et al. (2010). Mechanism of Folding Chamber Closure in a Group II Chaperonin. Nature 463, 379–383. doi:10.1038/nature08701
Zhang, J. (2011). Cryo-EM Structure of a Group II Chaperonin in the Prehydrolysis ATP-Bound State Leading to Lid Closure. Structure 19, 633–639. doi:10.1016/j.str.2011.03.005
Zhang, K., Julius, D., and Cheng, Y. (2021). Structural Snapshots of TRPV1 Reveal Mechanism of Polymodal Functionality. Cell 184, 5138–5150. doi:10.1016/j.cell.2021.08.012
Zhang, K., Pintilie, G. D., Li, S., Schmid, M. F., and Chiu, W. (2020). Resolving Individual Atoms of Protein Complex by Cryo-Electron Microscopy. Cell Res 30 (12), 1136–1139. doi:10.1038/s41422-020-00432-2
Zhao, Y., Schmid, M. F., Frydman, J., and Chiu, W. (2021). CryoEM Reveals the Stochastic Nature of Individual ATP Binding Events in a Group II Chaperonin. Nat. Commun. 12, 4754. doi:10.1038/s41467-021-25099-0
Keywords: strucural analysis, classification, crystal, solution, conformation alanalysis, heterogeneity, cryo-TEM, x-ray
Citation: Chang W-H, Huang S-H, Lin H-H, Chung S-C and Tu I-P (2021) Cryo-EM Analyses Permit Visualization of Structural Polymorphism of Biological Macromolecules. Front. Bioinform. 1:788308. doi: 10.3389/fbinf.2021.788308
Received: 02 October 2021; Accepted: 16 November 2021;
Published: 08 December 2021.
Edited by:
Barbora Kozlikova, Masaryk University, CzechiaReviewed by:
Sai Ganesan, University of California, San Francisco, United StatesShruthi Viswanath, National Centre for Biological Sciences, India
Copyright © 2021 Chang, Huang, Lin, Chung and Tu. This is an open-access article distributed under the terms of the Creative Commons Attribution License (CC BY). The use, distribution or reproduction in other forums is permitted, provided the original author(s) and the copyright owner(s) are credited and that the original publication in this journal is cited, in accordance with accepted academic practice. No use, distribution or reproduction is permitted which does not comply with these terms.
*Correspondence: Wei-Hau Chang, weihau@gate.sinica.edu.tw