- 1Department of Nutrition, University of California Davis, Davis, CA, United States
- 2School of Medicine, University of California San Diego, La Jolla, CA, United States
Background: The N-glycan structure and composition of the spike (S) protein of SARS-CoV-2 are pertinent to vaccine development and efficacy.
Methods: We reconstructed the glycosylation network based on previously published mass spectrometry data using GNAT, a glycosylation network analysis tool. Our compilation of the network tool had 26 glycosyltransferase and glucosidase enzymes and could infer the pathway of glycosylation machinery based on glycans in the virus spike protein. Once the glycan biosynthesis pathway was generated, we simulated the effect of blocking specific enzymes—swainsonine or deoxynojirimycin for blocking mannosidase-II and indolizidine for blocking alpha-1,6-fucosyltransferase—to see how they would affect the biosynthesis network and the glycans that were synthesized.
Results: The N-glycan biosynthesis network of SARS-CoV-2 spike protein shows an elaborate enzymatic pathway with several intermediate glycans, along with the ones identified by mass spectrometric studies. Of the 26 enzymes, the following were involved—Man-Ia, MGAT1, MGAT2, MGAT4, MGAT5, B3GalT, B4GalT, Man-II, SiaT, ST3GalI, ST3GalVI, and FucT8. Blocking specific enzymes resulted in a substantially modified glycan profile of SARS-CoV-2.
Conclusion: Variations in the final N-glycan profile of the virus, given its site-specific microheterogeneity, are factors in the host response to the infection, vaccines, and antibodies. Heterogeneity in the N-glycan profile of the spike (S) protein and its potential effect on vaccine efficacy or adverse reactions to the vaccines remain unexplored. Here, we provide all the resources we generated—the glycans in the glycoCT xml format and the biosynthesis network for future work.
Introduction
Glycosylation is a very common and complex post-translational modification process of proteins (Shental-Bechor and Levy, 2008). About 70% of human proteins are likely glycosylated (An et al., 2009). N-glycosylation is one of the main types of glycosylation in humans (Reily et al., 2019). N-glycosylation has been shown to have an impact on protein folding and function in several cases (Parodi, 2000; Mitra et al., 2006). Specifically, the site of glycosylation and the type of the glycan—mannose-rich, hybrid, or complex—likely influence protein function and folding. Virus replication involves the host glycosylation machinery for post-translational modifications. So, it can be useful to understand which enzymes are critical to this process. Bioinformatics tools have been used to determine physiological processes in the field of genetics (Lindblom and Robinson, 2011) and cancer biology (Papaleo et al., 2017) successfully, and such tools are slowly coming into existence in the field of glycobiology (Liu and Neelamegham, 2015).
Given the current SARS-CoV-2 pandemic and the role of the spike (S) glycoprotein in the virus entry and infection of host cells (Ou et al., 2020), we chose to map the enzymatic machinery that is responsible for the spike (S) glycoprotein synthesis. The two novel mRNA vaccines that have shown the highest (> 90%) efficacies both train the immune system to produce antibodies against the spike (S) protein of SARS-CoV-2 (Baden et al., 2020; Jackson et al., 2020). However, the greater the degree to which the spike (S) protein is glycosylated, the greater the chances of vaccine failure (Wang et al., 2020). The high degree of glycosylation of the spike (S) protein also suggests the virus can change quickly, rendering vaccines less effective. Hence, understanding the glycosylation pathway more intricately could help improve drug development and assessment of vaccine efficacy. Once the pathway is understood better, it can determine where modified glycoproteins can be used in vitro with immune cells or immunoproteins, or simulation studies using molecular dynamic binding, determining the host response (Grant et al., 14991).
Several SARS-CoV-2 spike protein sequences have been reported (Liu et al., 2020), and the glycosylation profile of the spike (S) protein has also been reported by several independent research groups thus far, using sequencing, mass spectrometry, and imaging tools (Watanabe et al., 2020; Shajahan et al., 2020; Wang et al., 2020). We present a summary of the glycosites in SARS-CoV-2 in Table 1. There are two glycosites in the receptor-binding domain (RBD, which binds with ACE2 protein) between N319 and N541, which are N331 and N343. There is some built-in heterogeneity in the type and level of glycosylation at these glycosites (Shajahan et al., 2020). Most mass-spectrometry–based investigations suggest varying combinations of high mannose, hybrid, and complex glycans, with predominantly complex glycans in the RBD (Watanabe et al., 2020; Shajahan et al., 2020; Zhang et al., 2021; Sanda et al., 2021). A specific study on how this heterogeneity affects the pathogenicity or virulence of the virus has not been done yet. However, with the increase in genetic variants, the amino acid sequences in the spike (S) glycoprotein have also been shown to vary (Xu et al., 2020), and Li et al. evaluated about 80 spike glycoprotein variants, which resulted in 26 glycosite modifications (Li et al., 2020). Deletion of both N331 and N343 sites resulted in severe inability of the virus to infect the host cell, not to mention most glycosylation deletions reduced infectivity.
Several groups of researchers have developed bioinformatics tools that are useful in understanding glycosylation, both for glycosite prediction (Pitti et al., 2019) and to predict the biosynthetic pathway based on empirical data from mass spectrometry (Krambeck et al., 2017). GNAT (Liu et al., 2013), which is used in the current manuscript, is a tool that allows for selective identification of glycosylation pathways using the glycan profile of each protein based on specific enzyme rules and constraints. In this work, we examined the effect of blocking two different glycosylation enzymes, to see if we can modify the network of glycans developed as part of the virus spike glycoprotein. Specifically, we chose to simulate blocking mannosidase-II, which would be the effect if swainsonine or deoxynojirimycin were used, and to simulate blocking alpha-1,6-fucosyltransferase, which would be the effect if indolizidine were used. In fact, indolizidine extracts from the Tylophora genus of vines have previously been shown to be effective against SARS-CoV (Yang et al., 2010) but are yet to be tested in the current pandemic. Expanding on these blocking studies can help identify the ideal targets to choose that affect the virus replication, since those are dependent on the spike glycoprotein, without affecting the host.
Methods
Simulated N-glycan biosynthetic network generation: We used the list of the most representative N-glycans per glycosite detected by Zhang et al. (obtained from Figure 3 in their manuscript) (Zhang et al., 2021) and the N-glycan profile of SARS-CoV-2 as reported by Shajahan et al. (obtained from Figure 4 in their manuscript) (Shajahan et al., 2020) to generate our primary glycosylation biosynthesis networks. Data from Zhang et al. (Zhang et al., 2021) and Shajahan et al. (Grant et al., 14991) were both obtained from recombinant viral proteins expressed in HEK293 cells, complete mass spectrometry data were available, and their identified glycosites were in agreement, as opposed to data from Sanda et al. (Sanda et al., 2021) that were also from HEK293 cells, but had a different set of glycosites predicted (see Table 1). We also did not use data from Watanabe et al. (Watanabe et al., 2020), since their spike glycoprotein was expressed in FreeStyle 293 cells, in order to keep our data source consistent. We generated the glycoCT xml version of these glycans using the glycanbuilder tool (Ceroni et al., 2008) and verified structures using the glycan chemNIST MS database (Glycan). Our supplemental documents provide all the glycans that were used and generated as part of this analysis in the glycoCT xml format.
GNAT and inferglycanpathway: As mentioned earlier, we used GNAT (Liu et al., 2013), with the additional enzymes developed by Hou et al. (Hou et al., 2016) to have a functional simulation tool for N-glycan biosynthesis with a total of 26 enzymes [c1galT1, b3galt6, GalNAcT-A, GalT-B, FucTH1, FucTH2, FucTLe, B3GalT, IGNT, ManIb, Man-Ia, SiaT, FucT, MGAT1, GNTE, MGAT2, MANI, Man-II, MGAT3, MGAT4, MGAT5, B4GalT, ST3GalI, ST3GalIV, ST3GalVI, and FUCT7]. The reverse inference algorithm derives the N-glycosylation pathway from a given set of reaction products and possible enzymes. For each enzyme, a set of rules and constraints is provided that defines its action. Given a glycan, it is then possible to identify a set of reactions that led to the production of the glycan, by examining all the enzyme rules with constraints in reverse. This will generate either a network of glycans that are predecessors of the given glycan to Man-9, which is the “parent” glycan for this pathway, or will result in an empty set, i.e., the given glycan has no predecessor leading the way to Man-9. Since we provided all the required enzymes to construct the entire glycosylation pathway, we were able to identify all the paths from Man-9 to all the glycans observed in the mass spectrometry studies. Since there is no good way to determine the linkage information (i.e., structure) from the mass-spectrometry–based composition (Klein and Zaia, 2019), there is likely structural heterogeneity that we failed to account for. This heterogeneity can also affect the pathway that was chosen or the enzyme that was involved in the biosynthesis, which is a limitation of our current approach.
Simulated inhibition of glycan biosynthesis pathway: Inhibition of all or parts of the glycosylation pathway is lately being considered a likely chemotherapeutic candidate for cancer (Kurosu, 2018) and Alzheimer’s disease (Haukedal and Freude, 2020), and treatment of the novel coronavirus infection could also be approached in a similar way. There are established natural and synthetic inhibitors for blocking or slowing down the rate of glycosylation biosynthetic reactions (Jeffrey et al., 2015–2017). The blocking of these pathways has been discussed and considered in the past (Wojtowicz et al., 2012; Vasconcelos-Dos-Santos et al., 2015). Tunicamycin, for instance, inhibits UDP-HexNAc, which catalyzes the first step of N-glycosylation (Merlie et al., 1982). It is originally considered an antibiotic and is now considered an anti-tumor agent in cancer (Wu et al., 2018). Furthermore, chloroquine and hydroxychloroquine are glycosylation inhibitors, which have been investigated in their effect against SARS-CoV (Vincent et al., 2005) and SARS-CoV-2 (Kalra et al., 2020), albeit their mechanisms are unclear. We identified the N-glycan biosynthesis pathway using GNAT before choosing common enzyme blocking targets. Based on the pathway we were able to detect for the biosynthesis of these specific glycans, we chose to simulate the blocking of Man-II (as done by swainsonine or deoxynojirimycin) and FucT8 (as done by indolizidine). We generated network graphs with glycans as nodes and enzymes as edges with and without the simulated enzyme inhibition.
Results
Figure 1 depicts the network of reactions (edges colored by enzymes) and glycans (nodes, numbered ones were identified by the mass spectrometry data) generated based on glycans from Zhang et al. The glycans detected by mass spectrometric analyses are numbered, and the intermediate ones generated in the biosynthesis pathway are not numbered. Our results suggest the involvement of the following nine enzymes: Man-Ia, Man-II, MGAT2, MGAT3, MGAT4, MGAT5, B4GalT, SiaT, and FucT8, and the network generated 279 glycans in total. In addition to the full network of biosynthetic reactions, Figure 1 also has panels depicting the effect of using chemical inhibitors swainsonine or deoxynojirimycin for blocking Man-II and indolizidine for blocking FucT8. In both cases, only three of the total 10 glycans were formed, thus modifying the glycan profile of the viral protein. In panels B and C, the network depicting three glycans (1, 4, and 9) that result in being traced back to the Man-9 residue (residue #102) will be formed. The other network that is independent of this (not containing residue#102) comprises all the glycans that would not be formed. Table 2 presents the glycans that could and could not be formed when Man-II and FucT8 were blocked. As previously mentioned, these two inhibitors were chosen because they have been established to specifically inhibit glycosylation enzymes.
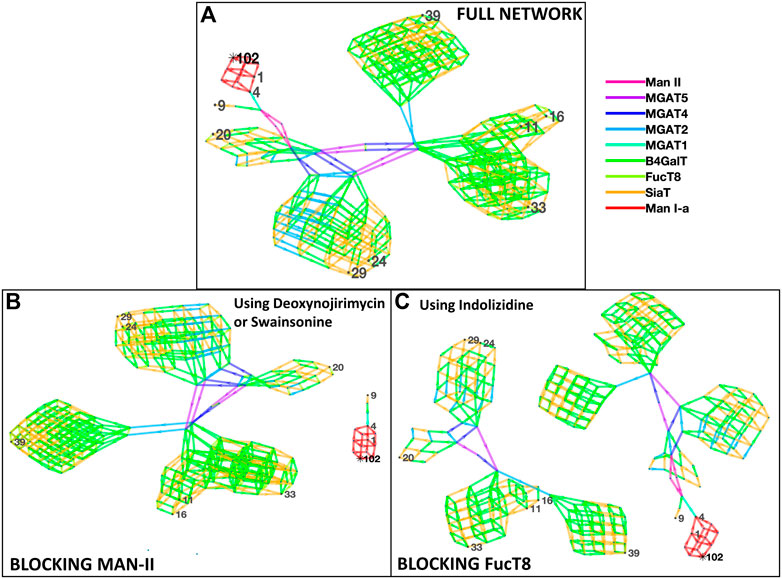
FIGURE 1. Network of glycans along with the enzymes involved in generating the 10 most abundant glycans as per Zhang et al. [panel (A)]. All the 10 glycans (identified by nodes 1, 4, 9, 11, 16, 20, 24, 29, 33, and 39) were traced back to their Man-9 parent glycan (node 102), and the biosynthesis pathway involved the use of nine enzymes as presented in the key to the right, as denoted by the colors. Panels (B) and (C) display disruption of the network of biosynthesis pathways when using the alpha-mannosidase (Man-II) blocking agent deoxynojirimycin or swainsonine [panel (B)] and when using the alpha-1,6-fucosyltransferase (FucT8) agonist indolizidine [panel (C)]. In the network generated with enzymes in panels (B) and (C), only three of the total 10 glycans are formed.
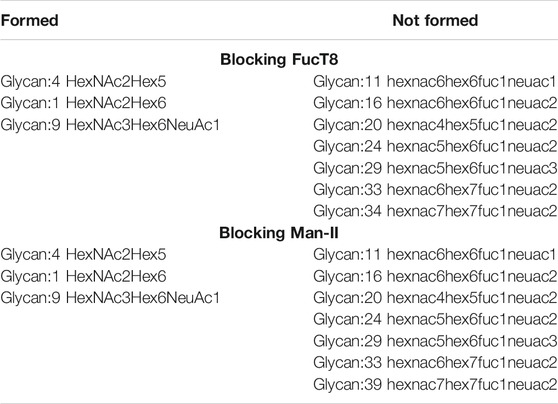
TABLE 2. List of glycans that were formed vs. not formed when specific enzymes were blocked when using data from Zhang et al. Glycan numbers indicate nodes in Figure 1.
Figure 2 depicts the network of reactions and glycans similar to Figure 1 but is based on glycans from Shajahan et al. Similar to Figure 1, the glycans detected by mass spectrometric analyses are numbered, and the intermediate ones generated in the biosynthesis pathway are not. Here, 12 enzymes were involved—Man-Ia, MGAT1, MGAT2, MGAT4, MGAT5, B3GalT, B4GalT, Man-II, SiaT, ST3GalI, ST3GalVI, and FucT8, and the network generated 292 glycans in total. Similar to Figure 1, blocking of Man-II and FucT8 resulted in a very different glycosylation profile of the overall protein since only the network of glycans that can be traced back to Man-9 (residue #15) will be formed, and the others (networks without residue #15) will not be formed. Table 3 presents the glycans that could and could not be formed when Man-II and FucT8 were blocked.
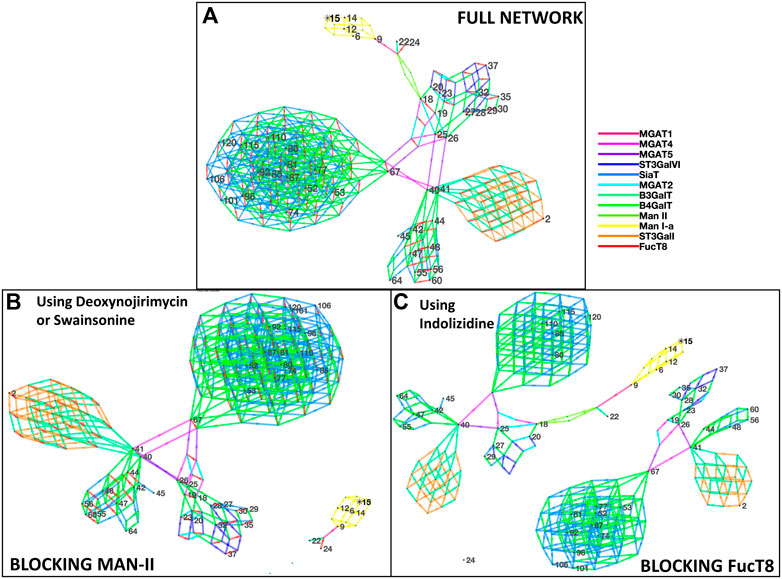
FIGURE 2. Network of glycans along with the enzymes involved in generating the glycans as per Shajahan et al. [panel (A)]. All the 48 glycans (identified by numbered nodes) were traced back to their Man-9 parent glycan (node 15). The colors (explained in the key) depict the different enzymes involved in the biosynthesis pathway. A total of 12 N-glycan biosynthesis enzymes were required to develop this profile of glycans. Panels (B) and (C) display disruption of the network of glycans generated when using the alpha-mannosidase (Man-II) blocking agent deoxynojirimycin or swainsonine [panel (B)] and when using the alpha-1,6-fucosyltransferase (FucT8) agonist indolizidine [panel (C)]. In the network generated with enzymes in panel (B), only five glycans are formed, and in panel (C), only 21 of the 48 are formed.
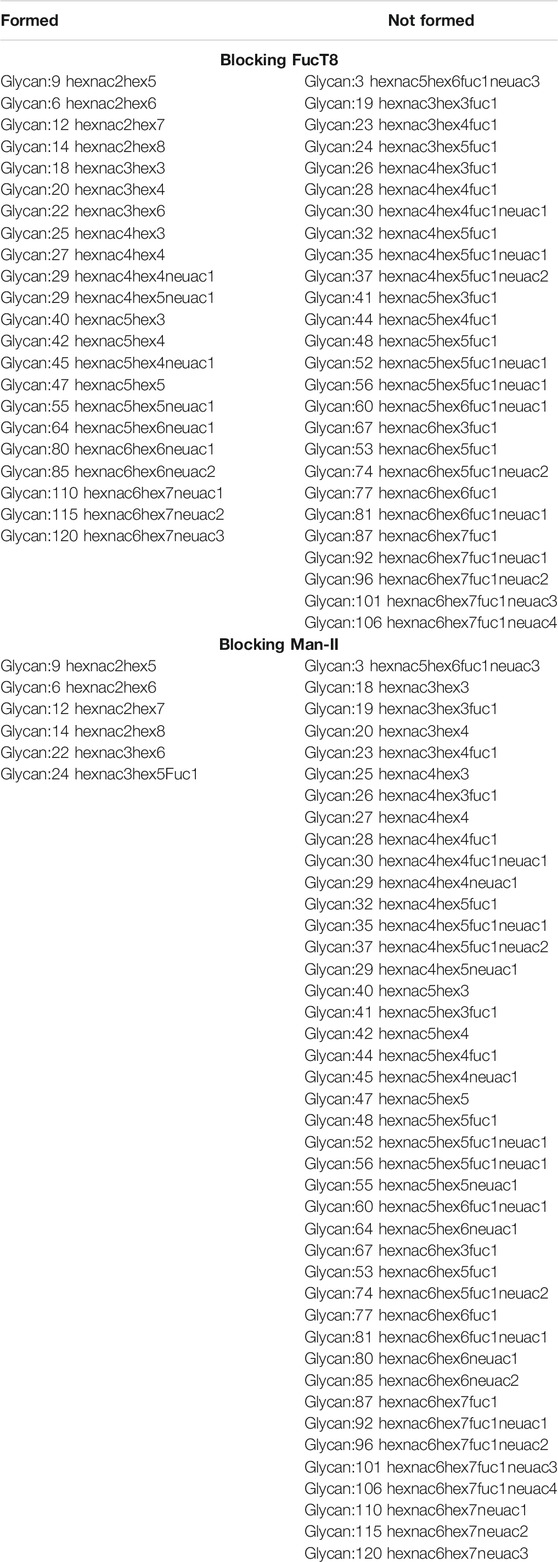
TABLE 3. List of glycans that were formed vs. not formed when specific enzymes were blocked when using data from Shajahan et al. Glycan numbers indicate nodes in Figure 2. Red font glycans are on the receptor-binding domain in subunit A of the spike glycoprotein S1.
The most abundant glycans reported by Zhang et al. (which were used to generate networks presented in Figure 1) were a subset of glycans reported by Shajahan et al. Thus, the network generated by Shajahan et al. was larger and included all the enzymes used by Zhang et al. For the sake of brevity, all information regarding the network is not presented in the manuscript; however, all supplementary information (glycans and high-resolution images of the network and network visualization scripts) can be found at https://github.com/girip/N-glycosylation-network-sars-cov2.git.
Discussion
Here, we present a preliminary N-glycan biosynthesis pathway of the spike (S) glycoprotein on SARS-CoV-2, which is not available currently for quick examination. We also share the individual glycan structures in a more easily accessible format for future glycoinformatics and molecular dynamics work. Based on our blocking simulation studies, the enzymes Man-II and FucT8 play important roles in the biosynthetic pathway, and without them, the glycans synthesized are altered, changing the glycoprotein profile. The N-glycan biosynthesis pathway is a highly conserved two-step process beginning in the endoplasmic reticulum and ending in the Golgi body (Aebi, 2013). It is now recognized that the host glycosylation process is very relevant to vaccine (Watanabe et al., 2020) and antibody development (Grant et al., 14991). Being able to identify the effect of modified glycans on the spike (S) protein could further aid in the development of vaccines, or in evaluating the efficacy of vaccines by comparing various glycovariant forms of the protein. This first step will pave the way for such future work.
We did not choose to focus only on the glycans in the receptor-binding domain (RBD) in the current report. Instead, we showed that the overall glycan profile is altered dramatically by simulating glycosylation pathway inhibitors. Owing to the site-specific microheterogeneity that all glycoproteins express, which is also true of the SARS-CoV-2 spike protein, it becomes important in subsequent steps to study the effect of this variability on its pathogenicity, with specific focus on the glycans in the RBD. However, the role of these glycans in overall protein folding and dynamics is unclear and still being studied. Several factors determine this microheterogeneity (Varki and et al., 2009), which in turn affects the structure, folding, and dynamics of the protein. So, it becomes important to not focus only on the glycans within the RBD of the spike (S) protein. Researchers have generated molecular dynamic simulations of the SARS-CoV-2 spike protein that represents site-specific microheterogeneity, based on mass spectrometry and imaging studies (Casalino et al., 2020; Chang and Zaia, 2019). Following up on our current approach, it would be possible to generate molecular dynamic simulation studies of the altered spike (S) glycoprotein that is generated by altering the glycosylation machinery.
Various levels of computational modeling of N-glycosylation have been used in the past. Here, we used a pathway construction computational model. Alternatively, simulations that include the kinetics of enzyme activity by including rate equations can be more representative of the physiological process (Krambeck et al., 2009). Using kinetics, it will further be possible to examine the effect of slowing down the rate of glycosylation of the spike (S) protein on overall viral replication (Kumar et al., 2020). Such an approach could shed light on potential pharmacological targets that would slow down both the host and the virus glycosylation pathways. If used appropriately, it may become possible to identify pharmacological targets that would affect the host the least and the virus the most. In addition, the time involved in testing several of these targets to identify ideal ones can be made short by using such network modeling tools before pre-clinical trials. However, this can be very challenging since the mammalian host post-translational glycosylation and its downstream effect on proteins and their functions are still an active area of investigation.
Yet another approach is to use Golgi-compartment representations within the modeling framework to model intra-organelle regional impacts on protein synthesis (Fisher et al., 2019). This could also aid in developing accurate representations of the enzymatic biosynthesis pathway. Alternately, for the current crisis, these approaches could evaluate competitive inhibitor glycans (natural or synthetic) and their effect on viral replication. By generating modified glycoproteins, it is possible to evaluate how they bind to or alter the immune response of the host since the host response to SARS-CoV-2 has recently been shown to be the determining factor in the severity of the manifested infection or for the development of life-threatening adverse complications (Mauro et al., 2020). The added benefit of modeling is to be able to quickly narrow down targets by simulating several at once while also knowing the underlying mechanism, which is not always possible in clinical studies. This makes computational modeling a useful tool in drug and vaccine development efforts.
Limitations
As mentioned above, there are several other approaches to construct N-glycosylation pathways. In this work, we used pathway construction that does not include dynamics of the glycosylation enzymatic processes. In addition to that, this work being computational is preliminary and requires further computational and experimental/basic/clinical work to identify the effect of simulated outcomes. HEK cells do not mimic lung epithelial or alveolar cells in producing a similar viral titer (Takayama, 2020), so the actual analyses may need to happen by using recombinant virus data from VERO-E6 cells lines, or from lung alveolar cells to match the viral titer or be more accurate. However, the glycosylation pathway enzyme machinery is only missing very few of the components that the mature cells express, making HEK293 cells a good cell line to use (Narimatsu et al., 2019). Another pertinent factor to consider is the wide variability of COVID-19 patient phenotypes contributed by genomic differences (Murray et al., 2020) that could include differences in the glycosylation machinery. We also did not conduct protein dynamic modeling studies, to determine if the altered glycan affects the protein and its downstream binding with mammalian receptors. While factoring in these limitations, we need novel approaches to hasten drug discovery and vaccine development.
Conclusion
We explored the use of a computational network analysis approach to determine a putative N-glycosylation pathway used by SARS-CoV-2 that results in the spike (S) glycoprotein. Even with emergency use authorization for several vaccines against this virus, mutant strains are being reported frequently (Lauring and Hodcroft, 2021). While the mutations at the genetic level are being reported, there are no efforts to understand how there is heterogeneity in glycosylation and how it affects vaccine efficacy or triggers adverse reactions. We presented here the first step in this process of eventually understanding this aspect better.
Data Availability Statement
The datasets presented in this study can be found in online repositories. The names of the repository/repositories and accession number(s) can be found in the article/Results section.
Author Contributions
SK and GK conceived the hypothesis and approach, SK identified chemical targets and verified glycosylation biochemical pathways, and GK ran the network analysis. Both the authors wrote the manuscript and have final responsibility.
Conflict of Interest
The authors declare that the research was conducted in the absence of any commercial or financial relationships that could be construed as a potential conflict of interest.
Acknowledgments
We would like to thank Prof. Sriram Neelamegham, University at Buffalo, for providing feedback on our manuscript and Prof John Yin, University of Wisconsin-Madison, for insight into virus replication processes. This work used the Extreme Science and Engineering Discovery Environment (XSEDE), which was supported by the National Science Foundation under grant number ACI-1548562.
Abbreviations
B3GalT, ganglioside galactosyltransferase; b3galt6, beta-1,3-galactosyltransferase 6;B4GalT, beta-N-acetylglucosaminylglycopeptide beta-1,4-galactosyltransferase; c1galT1, core 1 synthase, glycoprotein-N-acetylgalactosamine 3-beta-galactosyltransferase, 1; FucT, glycoprotein 6-alpha-L-fucosyltransferase; FUCT7, 4-galactosyl-N-acetylglucosaminide 3-alpha-L-fucosyltransferase; FucTH1, type 1 galactoside alpha-(1,2)-fucosyltransferase; FucTH2, type 2 galactoside alpha-(1,2)-fucosyltransferase; FucTLe, 3-galactosyl-N-acetylglucosaminide 4-alpha-L-fucosyltransferase; GalNAcT-A, UDP-GalNAc:polypeptide N-acetylgalactosaminyltransferase A; GalT-B, fucosylgalactoside 3-alpha-galactosyltransferase; GNTE, N-acetyllactosaminide beta-1,3-N-acetylglucosaminyltransferase; IGNT, N-acetyllactosaminide beta-1,6-N-acetylglucosaminyltransferase; MANI, mannosyl-oligosaccharide 1,2-alpha-mannosidase; ManIb, alpha-1,4-glucan-protein synthase; Man-II, mannosyl-oligosaccharide 1,3-1,6-alpha-mannosidase; MGAT1, alpha-1,3-mannosyl-glycoprotein 2-beta-N-acetylglucosaminyltransferase; MGAT2, alpha-1,6-mannosyl-glycoprotein 2-beta-N-acetylglucosaminyltransferase; MGAT3, beta-1,4-mannosyl-glycoprotein 4-beta-N-acetylglucosaminyltransferase; MGAT4, alpha-1,3-mannosyl-glycoprotein 4-beta-N-acetylglucosaminyltransferase; MGAT5, alpha-1,6-mannosyl-glycoprotein 6-beta-N-acetylglucosaminyltransferase; SiaT, N-acetyllactosaminide alpha-2,3-sialyltransferase; ST3GalI, beta-galactoside alpha-2,3-sialyltransferase; ST3GalIV, N-acetyllactosaminide alpha-2,3-sialyltransferase; ST3GalVI, beta-galactoside alpha-(2,6)-sialyltransferase.
References
Aebi, M. (2013). N-linked Protein Glycosylation in the ER. Biochim. Biophys. Acta 1833, 2430–2437. doi:10.1016/j.bbamcr.2013.04.001
An, H. J., Froehlich, J. W., and Lebrilla, C. B. (2009). Determination of Glycosylation Sites and Site-specific Heterogeneity in Glycoproteins. Curr. Opin. Chem. Biol. 13, 421–426. doi:10.1016/j.cbpa.2009.07.022
Baden, L. R., El Sahly, H. M., Essink, B., Kotloff, K., Frey, S., Novak, R., et al. (2020). Efficacy and Safety of the mRNA-1273 SARS-CoV-2 Vaccine. N. Engl. J. Med. 384, 403–416. doi:10.1056/NEJMoa2035389
Casalino, L., Gaieb, Z., Goldsmith, J. A., Hjorth, C. K., Dommer, A. C., Harbison, A. M., et al. (2020). Beyond Shielding: The Roles of Glycans in SARS-CoV-2 Spike Protein. ACS Cent Sci. 6 (10), 1722–1734. doi:10.1021/acscentsci.0c01056
Ceroni, A., Maass, K., Geyer, H., Geyer, R., Dell, A., Haslam, S. M., et al. (2008). GlycoWorkbench: a Tool for the Computer-Assisted Annotation of Mass Spectra of Glycans. J. Proteome Res. 7, 1650–1659. doi:10.1021/pr7008252
Chang, D., and Zaia, J. (2019). Why Glycosylation Matters in Building a Better Flu Vaccine. Mol. Cel Proteomics 18, 2348–2358. doi:10.1074/mcp.R119.001491
Mauro, G. D., Cristina, S., Concetta, R., Francesco, R., and Annalisa, C. (2020). SARS-Cov-2 Infection: Response of Human Immune System and Possible Implications for the Rapid Test and Treatment. Int. Immunopharmacol 84, 106519. doi:10.1016/j.intimp.2020.106519
Fisher, P., Thomas-Oates, J., Wood, A. J., and Ungar, D. (2019). The N-Glycosylation Processing Potential of the Mammalian Golgi Apparatus. The. Front. Cel Dev Biol 7, 157. doi:10.3389/fcell.2019.00157
Glycan, M. S.NIST Library. Available at: https://chemdata.nist.gov/glycan/about (Accessed January 8, 2020).
Grant, O. C., Montgomery, D., Ito, K., and Woods, R. J. (1499). Analysis of the SARS-CoV-2 Spike Protein Glycan Shield Reveals Implications for Immune Recognition. Sci. Rep. 10, 144991. doi:10.1038/s41598-020-71748-7
Haukedal, H., and Freude, K. K. (2020). Implications of Glycosylation in Alzheimer's Disease. Front. Neurosci. 14, 625348. doi:10.3389/fnins.2020.625348
Hou, W., Qiu, Y., Hashimoto, N., Ching, W. K., and Aoki-Kinoshita, K. F. (2016). A Systematic Framework to Derive N-Glycan Biosynthesis Process and the Automated Construction of Glycosylation Networks. BMC Bioinformatics 17 (Suppl. 7), 240. doi:10.1186/s12859-016-1094-6
Jackson, L. A., Anderson, E. J., Rouphael, N. G., Roberts, P. C., Makhene, M., Coler, R. N., et al. (2020). An mRNA Vaccine against SARS-CoV-2 - Preliminary Report. N. Engl. J. Med. 383, 1920–1931. doi:10.1056/NEJMoa2022483
Jeffrey, D., Esko, C. B., Schnaar, R. L., and Cummings, R. D. (2015–2017). Editors J. D. Esko, and A. Varki. Cold Spring Harbor Laboratory Press
Kalra, R. S., Tomar, D., Meena, A. S., and Kandimalla, R. (2020). SARS-CoV-2, ACE2, and Hydroxychloroquine: Cardiovascular Complications, Therapeutics, and Clinical Readouts in the Current Settings. Pathogens 9, 546. doi:10.3390/pathogens9070546
Klein, J., and Zaia, J. (2019). Glypy: An Open Source Glycoinformatics Library. J. Proteome Res. 18, 3532–3537. doi:10.1021/acs.jproteome.9b00367
Krambeck, F. J., Bennun, S. V., Andersen, M. R., and Betenbaugh, M. J. (2017). Model-based Analysis of N-Glycosylation in Chinese Hamster Ovary Cells. PLoS One 12, e0175376. doi:10.1371/journal.pone.0175376
Krambeck, F. J., Bennun, S. V., Narang, S., Choi, S., Yarema, K. J., Betenbaugh, M. J., et al. (2009). A Mathematical Model to Derive N-Glycan Structures and Cellular Enzyme Activities from Mass Spectrometric Data. Glycobiology 19, 1163–1175. doi:10.1093/glycob/cwp081
Kumar, S., Maurya, V. K., Prasad, A. K., Bhatt, M. L. B., and Saxena, S. K. (2020). Structural, Glycosylation and Antigenic Variation between 2019 Novel Coronavirus (2019-nCoV) and SARS Coronavirus (SARS-CoV). Virusdisease 31, 13–21. doi:10.1007/s13337-020-00571-5
Kurosu, M. (2018). Inhibition of N-Glycosylation towards Novel Anti-Cancer Chemotherapeutics. J. Mol. Pharm. Org. Process. Res. 6, 141.
Lauring, A. S., and Hodcroft, E. B. (2021). Genetic Variants of SARS-CoV-2-What Do They Mean? JAMA 325, 529–531. doi:10.1001/jama.2020.27124
Li, Q., Wu, J., Nie, J., Zhang, L., Hao, H., Liu, S., et al. (2020). The Impact of Mutations in SARS-CoV-2 Spike on Viral Infectivity and Antigenicity. Cell 182, 1284–1294.doi:10.1016/j.cell.2020.07.012
Lindblom, A., and Robinson, P. N. (2011). Bioinformatics for Human Genetics: Promises and Challenges. Hum. Mutat. 32, 495–500. doi:10.1002/humu.21468
Liu, G., and Neelamegham, S. (2015). Integration of Systems Glycobiology with Bioinformatics Toolboxes, Glycoinformatics Resources, and Glycoproteomics Data. Wiley Interdiscip. Rev. Syst. Biol. Med. 7, 163–181. doi:10.1002/wsbm.1296
Liu, G., Puri, A., and Neelamegham, S. (2013). Glycosylation Network Analysis Toolbox: a MATLAB-Based Environment for Systems Glycobiology. Bioinformatics 29, 404–406. doi:10.1093/bioinformatics/bts703
Liu, Z., Xiao, X., Wei, W., Li, J., Yang, J., Tan, H., et al. (2020). Composition and Divergence of Coronavirus Spike Proteins and Host ACE2 Receptors Predict Potential Intermediate Hosts of SARS-CoV-2. J. Med. Virol. 92, 595–601. doi:10.1002/jmv.25726
Merlie, J. P., Sebbane, R., Tzartos, S., and Lindstrom, J. (1982). Inhibition of Glycosylation with Tunicamycin Blocks Assembly of Newly Synthesized Acetylcholine Receptor Subunits in Muscle Cells. J. Biol. Chem. 257, 2694–2701.
Mitra, N., Sinha, S., Ramya, T. N., and Surolia, A. (2006). N-linked Oligosaccharides as Outfitters for Glycoprotein Folding, Form and Function. Trends Biochem. Sci. 31, 156–163. doi:10.1016/j.tibs.2006.01.003
Murray, M. F., Kenny, E. E., Ritchie, M. D., Rader, D. J., and Bale, A. E. (2020). COVID-19 Outcomes and the Human Genome. Genet. Med. 22, 1175–1177. doi:10.1038/s41436-020-0832-3
Narimatsu, Y., Joshi, H. J., Nason, R., Coillie, J. V., Karlsson, R., Sun, L., et al. (2019). An Atlas of Human Glycosylation Pathways Enables Display of the Human Glycome by Gene Engineered Cells. Mol. Cel 75, 394–407. doi:10.1016/j.molcel.2019.05.017
Ou, X., Liu, Y., Lei, X., Li, P., Mi, D., Ren, L., et al. (2020). Characterization of Spike Glycoprotein of SARS-CoV-2 on Virus Entry and its Immune Cross-Reactivity with SARS-CoV. Nat. Commun. 11, 1620. doi:10.1038/s41467-020-15562-9
Papaleo, E., Gromova, I., and Gromov, P. (2017). Gaining Insights into Cancer Biology through Exploration of the Cancer Secretome Using Proteomic and Bioinformatic Tools. Expert Rev. Proteomics 14, 1021–1035. doi:10.1080/14789450.2017.1387053
Parodi, A. J. (2000). Role of N-Oligosaccharide Endoplasmic Reticulum Processing Reactions in Glycoprotein Folding and Degradation. Biochem. J. 348 Pt 1, 1–13.
Pitti, T., Chen, C. T., Lin, H. N., Choong, W. K., Hsu, W. L., and Sung, T. Y. (2019). N-GlyDE: a Two-Stage N-Linked Glycosylation Site Prediction Incorporating Gapped Dipeptides and Pattern-Based Encoding. Sci. Rep. 9, 15975. doi:10.1038/s41598-019-52341-z
Reily, C., Stewart, T. J., Renfrow, M. B., and Novak, J. (2019). Glycosylation in Health and Disease. Nat. Rev. Nephrol. 15, 346–366. doi:10.1038/s41581-019-0129-4
Sanda, M., Morrison, L., and Goldman, R. (2021). N- and O-Glycosylation of the SARS-CoV-2 Spike Protein. Anal. Chem. 93, 2003–2009. doi:10.1021/acs.analchem.0c03173
Shajahan, A., Supekar, N. T., Gleinich, A. S., and Azadi, P. (2020). Deducing the N- and O- Glycosylation Profile of the Spike Protein of Novel Coronavirus SARS-CoV-2. Glycobiology 30, 981–988. doi:10.1093/glycob/cwaa042
Shental-Bechor, D., and Levy, Y. (2008). Effect of Glycosylation on Protein Folding: a Close Look at Thermodynamic Stabilization. Proc. Natl. Acad. Sci. U S A. 105, 8256–8261. doi:10.1073/pnas.0801340105
Takayama, K. (2020). Vitro and Animal Models for SARS-CoV-2 Research. Trends Pharmacol. Sci. 41, 513–517. doi:10.1016/j.tips.2020.05.005
Varki, A., Cummings, R. D., Esko, J. D., Stanley, P., Hart, G. W., Aebi, M., et al. (2009). Essentials of Glycobiology [Internet]. 3rd edn Cold Spring Harbor, NY: Cold Spring Harbor Laboratory Press
Vasconcelos-Dos-Santos, A., Oliveira, I. A., Lucena, M. C., Mantuano, N. R., Whelan, S. A., Dias, W. B., et al. (2015). Biosynthetic Machinery Involved in Aberrant Glycosylation: Promising Targets for Developing of Drugs against Cancer. Front. Oncol. 5, 138. doi:10.3389/fonc.2015.00138
Vincent, M. J., Bergeron, E., Benjannet, S., Erickson, B. R., Rollin, P. E., Ksiazek, T. G., et al. (2005). Chloroquine Is a Potent Inhibitor of SARS Coronavirus Infection and Spread. Virol. J. 2, 69. doi:10.1186/1743-422X-2-69
Wang, D., Baudys, J., Bundy, J. L., Solano, M., Keppel, T., and Barr, J. R. (2020). Comprehensive Analysis of the Glycan Complement of SARS-CoV-2 Spike Proteins Using Signature Ions-Triggered Electron-Transfer/Higher-Energy Collisional Dissociation (EThcD) Mass Spectrometry. Anal. Chem. 92, 14730–14739. doi:10.1021/acs.analchem.0c03301
Wang, F., Kream, R. M., and Stefano, G. B. (2020). An Evidence Based Perspective on mRNA-SARS-CoV-2 Vaccine Development. Med. Sci. Monit. 26, e924700. doi:10.12659/MSM.924700
Watanabe, Y., Allen, J. D., Wrapp, D., McLellan, J. S., and Crispin, M. (2020). Site-specific Glycan Analysis of the SARS-CoV-2 Spike. Science 369, 330–333. doi:10.1126/science.abb9983
Wojtowicz, K., Szaflarski, W., Januchowski, R., Zawierucha, P., Nowicki, M., Zabel, M., et al. (2012). Inhibitors of N-Glycosylation as a Potential Tool for Analysis of the Mechanism of Action and Cellular Localisation of Glycoprotein P. Acta Biochim. Pol. 59, 445–450.
Wu, J., Chen, S., Liu, H., Zhang, Z., Ni, Z., Chen, J., et al. (2018). Tunicamycin Specifically Aggravates ER Stress and Overcomes Chemoresistance in Multidrug-Resistant Gastric Cancer Cells by Inhibiting N-Glycosylation. J. Exp. Clin. Cancer Res. 37, 272. doi:10.1186/s13046-018-0935-8
Xu, W., Wang, M., Yu, D., and Zhang, X. (2020). Variations in SARS-CoV-2 Spike Protein Cell Epitopes and Glycosylation Profiles during Global Transmission Course of COVID-19. Front. Immunol. 11, 565278. doi:10.3389/fimmu.2020.565278
Yang, C. W., Lee, Y. Z., Kang, I. J., Barnard, D. L., Jan, J. T., Lin, D., et al. (2010). Identification of Phenanthroindolizines and Phenanthroquinolizidines as Novel Potent Anti-coronaviral Agents for Porcine Enteropathogenic Coronavirus Transmissible Gastroenteritis Virus and Human Severe Acute Respiratory Syndrome Coronavirus. Antivir. Res 88, 160–168. doi:10.1016/j.antiviral.2010.08.009
Keywords: N-glycan biosynthesis pathway, SARS-CoV-2, glyco-informatics, computational modeling, glycan heterogeneity
Citation: Krishnan S and Krishnan GP (2021) N-Glycosylation Network Construction and Analysis to Modify Glycans on the Spike (S) Glycoprotein of SARS-CoV-2. Front. Bioinform. 1:667012. doi: 10.3389/fbinf.2021.667012
Received: 11 February 2021; Accepted: 11 May 2021;
Published: 08 June 2021.
Edited by:
Hongmin Cai, South China University of Technology, ChinaReviewed by:
Yejun Wang, Shenzhen University, ChinaYves Vandenbrouck, Commissariat à l'Energie Atomique et aux Energies Alternatives (CEA), France
Copyright © 2021 Krishnan and Krishnan. This is an open-access article distributed under the terms of the Creative Commons Attribution License (CC BY). The use, distribution or reproduction in other forums is permitted, provided the original author(s) and the copyright owner(s) are credited and that the original publication in this journal is cited, in accordance with accepted academic practice. No use, distribution or reproduction is permitted which does not comply with these terms.
*Correspondence: Sridevi Krishnan, c3Jpa3Jpc2huYW5AdWNkYXZpcy5lZHU=